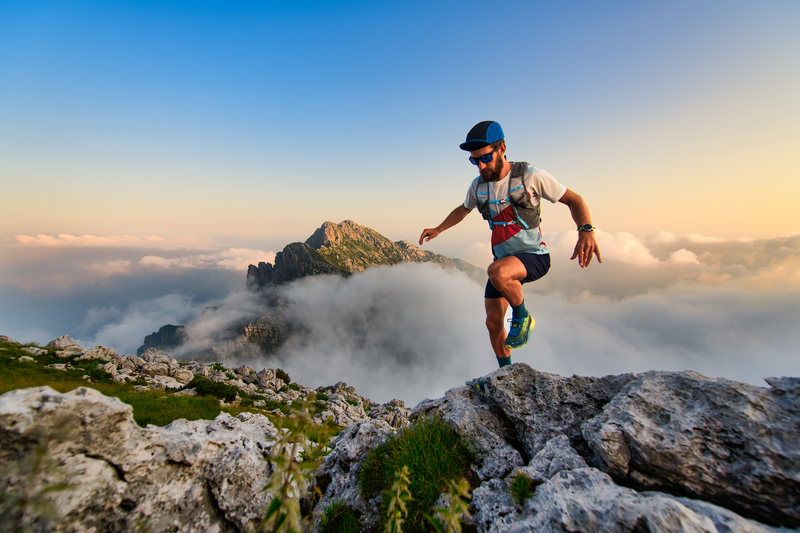
95% of researchers rate our articles as excellent or good
Learn more about the work of our research integrity team to safeguard the quality of each article we publish.
Find out more
ORIGINAL RESEARCH article
Front. Oncol. , 02 September 2024
Sec. Cancer Genetics
Volume 14 - 2024 | https://doi.org/10.3389/fonc.2024.1428025
Objective: The causal relationship and mechanisms between lipids and glioblastoma (GBM) remain unclear. This study aims to investigate the independent causal relationship between liposomal phosphatidylcholine 16:0_22:6 (PC16) and GBM, and to identify the potential mediating role of the inflammatory factor-fibroblast growth factor 21(FGF21).
Methods: Utilizing summary statistics from genome-wide association studies (GWAS) of lipids (179 types in 7174 Finnish individuals), GBM (243 cases and 287137 controls), and inflammatory factors (91 types in 14824 European individuals), a two-sample Mendelian Randomization (MR) approach was employed to establish the causal link between liposomal PC16 and GBM. Additionally, a two-step MR method was used to quantify the proportion of the causal effect of PC16 on GBM that is mediated by the inflammatory factor FGF21.
Results: MR analyses revealed a strong causal relationship between PC16 and GBM (OR=1.72, 95% CI: 1.11-2.68, P=0.016), but no reverse causality was observed from GBM to PC16 (OR=1.01, 95% CI: 0.99-1.02, P=0.38). Mediation analysis showed a strong causal relationship between PC16 and the FGF21 (OR = 0.94, 95% CI: 0.89-0.99, P=0.018) as well as between FGF21 and GBM (OR = 0.42, 95% CI: 0.25-0.71, P=0.001), with the mediation effect accounting for 9.78% of the total effect. This suggests that the causal relationship between PC16 and GBM is likely mediated by the intermediary factor FGF21. No evidence of pleiotropy was found in the sensitivity analysis of these positive results.
Conclusion: In summary, the findings of this study suggest that liposomal PC16 may increase the risk of GBM occurrence, and FGF21 may play a significant mediating role in this causal relationship.
Brain tumors are among the most difficult malignant tumors to cure, with gliomas being one of the most biologically invasive, complex, and heterogeneous brain tumors in the central nervous system of adults (1, 2). Glioblastoma (GBM), a World Health Organization (WHO) grade IV glioma, accounts for 12-15% of all brain tumors. Patients have a median survival of only 14-16 months, with a 5-year survival rate of approximately 5% (3–5). GBM exhibits high malignancy with low cure rates and high recurrence rates. The main clinical treatments are surgery, radiotherapy, and chemotherapy. However, due to its complexity, variable biological characteristics, and limited understanding of the developmental causes of glioblastoma, the clinical prognosis is very poor (6). Therefore, further exploration of the pathogenesis of GBM, identification of its endogenous biomarkers, determination of potential targets, and development of effective treatment methods are of significant importance for improving patient survival rates.
Phosphatidylcholine and its derivatives, a class of phospholipids, are one of the main components of cellular biological membranes and help maintain cell structure and function (7). They are also sources of lipid second messengers and determinants of the cell cycle process. The choline metabolites produced by their synthesis and catabolism contribute to proliferative growth and programmed cell death (8). Lipids have a variety of biological functions, reflecting the physiological and pathological states of cells, tissues, and organs. Alterations in lipid metabolism are among the most significant metabolic changes in the development of cancer. Under the influence of carcinogenic and environmental factors, cancer cells widely modify their metabolism to survive and develop in the constantly changing microenvironment (9). Studies have shown that total choline metabolites, including choline, phosphocholine, and phosphatidylcholine, are elevated in various tumors and cancer cells (10, 11). Therefore, this study aims to explore the potential association between phosphatidylcholine expression levels and the risk of GBM.
Fibroblast growth factor 21(FGF21), an intermediary factor, is a novel regulator of glucose and lipid metabolism produced by the liver or other tissues, skeletal muscle, and pancreatic beta cells. It plays multiple roles in development, organogenesis, and metabolism (12). For example, the FGF21/FGFR signal promotes neurite outgrowth in an L1-dependent manner and plays an important role in neural development (13). FGF21 has potent protective properties in neurons, such as inhibiting glutamate-induced death of primary rat brain neurons and D-galactose-induced brain aging in mice (14, 15). This suggests that FGF21 not only affects peripheral system glucose and lipid metabolism but also has significant neurobiological consequences in brain cells, including neurons and glial cells. This also hints that FGF21 might be an intermediary factor affecting the causal relationship between PC16 and GBM. Therefore, this study uses Mendelian Randomization (MR) to analyze the causal relationship between PC16 and GBM and to verify whether FGF21 mediates the relationship between PC16 and GBM.
MR studies are a method of causal inference that uses single nucleotide polymorphisms (SNPs) associated with exposure as instrumental variables (IVs) to infer causal associations between exposure and outcome (16). MR leverages Mendel’s laws of genetics, as genetic variants are randomly distributed during gamete formation and are mostly unaffected by environmental or lifestyle factors, thus reducing bias from unmeasured confounders or confounding factors while avoiding reverse causality (17). Therefore, our goal is to determine through MR analysis whether PC16 has a causal relationship with GBM and to clarify whether FGF21 as an intermediary participates in mediating this causal relationship and to quantify its mediation proportion.
This study utilizes publicly available genome-wide association study (GWAS) summary statistics. Phosphatidylcholine 16:0 (PC16) was considered the exposure factor, glioblastoma multiforme (GBM) as the outcome, and fibroblast growth factor 21 (FGF21) was included for mediation analysis. Initially, the total causal effect of PC16 on GBM was analyzed, followed by the causal relationship between PC16 and FGF21. Subsequent causal inference was made between FGF21 and GBM, and finally, the proportion of the effect mediated by the intermediary factor in the causal relationship between PC16 and GBM was assessed (Figure 1).
Figure 1. Overview of this Mendelian randomization (MR) analysis, including study design and main findings.
The Mendelian randomization (MR) study utilized data from large-scale GWAS. The lipidomic data were derived from a genome-wide analysis of 179 lipid species in 7174 Finnish individuals by Ottensmann et al. (accession numbers: GCST90277238-GCST90277416) (18). Data on inflammation markers came from a genetic study on circulating inflammatory proteins by Zhao, J.H et al., including 14824 participants of European descent (accession numbers: GCST90274758 - GCST90274848) (19). GBM data were obtained from the FinnGen database, which included 243 cases and 287137 controls, all of European descent (20). Each study had corresponding ethical review approvals and patient informed consent, eliminating the need for additional ethical approval.
MR studies require that the instrumental variables (IV) satisfy three assumptions: (1) IV is significantly associated with the exposure; (2) IV is not associated with any confounders; (3) IV influences the outcome only through the exposure. To meet these criteria, SNPs that reached genome-wide significance thresholds were extracted as IVs. For lipidomic PC16 and inflammatory marker FGF21, we selected SNPs with p < 1e-5, and for GBM, SNPs with p < 5e-5. We retained SNPs without linkage disequilibrium (LD) (kb=10000, R2 <0.001) to ensure the SNPs were not closely related. SNPs significantly associated with the outcome at the genome-wide level (P<5e-8) were excluded. Variance (R2) and F-statistics were used to estimate the association strength between the selected IVs and the exposure, with weak instruments (F-statistic <10) being removed to avoid bias. SNPs with allele frequencies inconsistent between exposure and outcome, as well as palindromic SNPs with intermediate allele frequencies, were excluded. The rigorously filtered SNPs were used for the final causal analysis.
The main method used for this analysis was the inverse-variance weighted (IVW) method. IVW is powerful for causal detection but requires that genetic variants impact the outcome only through the exposure in the study. Despite attempts to exclude known confounder-related SNPs, many unknown confounders could still introduce bias. Alternative methods, including MR-Egger, weighted median, and Bayesian MR (BWMR), were also utilized to assess the robustness of the results. However, if the results among the different methods were inconsistent, IVW was prioritized as the primary method for estimating causal effects, given its common use in MR analysis and its robustness in the absence of directional pleiotropy (21).
Cochran’s Q was used to assess heterogeneity among SNPs, indicating no heterogeneity when P>0.05. MR-Egger and MR-PRESSO were employed for pleiotropy testing. The “leave-one-out” approach was used to assess the influence of individual SNPs on the causal relationship. Sensitivity analysis was undertaken to verify the reliability of the results.
The total effect of PC16 on GBM was decomposed into the direct effect of PC16 on GBM and the indirect effect mediated by the intermediary variable. A two-step MR design was further used for mediation analysis, exploring whether FGF21 mediated the causal pathway from PC16 to GBM. The percentage of the mediation effect was calculated by dividing the indirect effect by the total effect.
The “Two-Sample MR” package was used for MR causal estimation analysis, and the “MR-PRESSO” package for MR pleiotropy and heterogeneity analysis. MR estimates were presented as odds ratios (OR) with 95% confidence intervals (CI). All statistical computations were performed using R version 4.2.3.
After removing palindromic and ambiguous SNPs, and those with linkage disequilibrium, a total of 23 SNPs were included in this study. The F-statistics of the PC16-exposed SNPs ranged from 19.8 to 53.9, all exceeding the threshold of 10, showing a strong intensity. This indicates no weak IVs bias, which means the results are reliable (Supplementary Table S1). The causal relationship between genetically predicted PC16 and GBM was estimated through regression methods such as Inverse Variance Weighting (IVW), MR-Egger, weighted median, and BWMR. Among all MR methods, the IVW method showed a significant positive correlation between genetically predicted PC16 expression and GBM (OR=1.72, 95% CI: 1.11-2.68, P= 0.016, Figures 2, 3), and BWMR showed the same result (OR=1.82, 95% CI: 1.09-3.02, P= 0.02, Figure 2). However, after extracting the reliable genetic instrumental variables of GBM (Supplementary Table S2), a reverse MR analysis was conducted. The results showed that GBM has no reverse causal relationship with PC16 expression (IVW: OR=1.01, 95% CI: 0.99-1.02, P= 0.38; BWMR: OR=1.00, 95% CI: 0.99-1.01, P= 0.98, Figure 2).
Figure 2. MR analysis results. PC16, phosphatidylcholine 16:0_22:6; GBM, glioblastoma; FGF21, fibroblast growth factor 21; IVW, inverse variance weighted; BWMR, bayesian weighted mendelian randomization; OR, odds ratio; CI, confidence interval; SNPs, single nucleotide polymorphisms.
Figure 3. (A) Forest plot to visualize causal effect of each single SNP on total GBM risk; (B) Scatter plots to visualize causal relationship between FGF21 and GBM from different methods. SNP, single nucleotide polymorphism; MR, mendelian randomization; GBM, glioblastoma.
After screening for strong instrumental variables and removing palindromic and ambiguous SNPs, we extracted 23 genome-wide significant SNPs as instrumental variables. The F-statistics of the genetic instrumental variables of PC16 ranged from 19.8 to 53.9 (Supplementary Table S3). Based on the IVW method, genetically predicted PC16 and FGF21 are negatively correlated (OR = 0.94, 95% CI:0.89-0.99, P=0.018) (Figures 2, 4A). MR - Egger (OR =0.90, 95% CI:0.82-0.98, P=0.03) and BWMR (OR =0.94, 95% CI:0.89-1.00, P=0.038) yielded results consistent with the IVW method (Figures 2, 4B).
Figure 4. (A) Forest plot to visualize causal effect of each single SNP on FGF21; (B) the scatter plots to visualize causal relationship between PC16 and FGF21 from different methods. SNP, single nucleotide polymorphism; MR, mendelian randomization; PC16, phosphatidylcholine 16:0_22:6; FGF21, fibroblast growth factor 21.
After removing palindromic and ambiguous SNP, we extracted 24 genome-wide significant SNPs as instrumental variables. The F-statistics of the instrumental variables of FGF21 ranged from 19.6 to 156.6 (Supplementary Table S4). Based on the IVW method, FGF21 is significantly negatively correlated with GBM (OR = 0.42, 95% CI:0.25-0.71, P=0.001; Figures 2, 5A). The other two Supplementary Methods, weighted median (OR =0.45, 95% CI:0.22-0.96, P=0.038) and BWMR (OR =0.44, 95% CI:0.26-0.73, P=0.0018), showed results consistent with the IVW method (Table 1, Figure 5B).
Figure 5. (A) Forest plot to visualize causal effect of each single FGF21 SNP on GBM; (B) the scatter plots to visualize causal relationship between FGF21 and GBM from different methods. SNP, single nucleotide polymorphism; MR, mendelian randomization; GBM, glioblastoma; FGF21, fibroblast growth factor 21.
We analyzed the mediating effect of FGF21 in the causal relationship between PC16 and GBM. We found that PC16 is associated with a decrease in FGF21, and a decrease in FGF21 is associated with an increased risk of GBM. This mediating effect accounts for 9.78% of the total causal effect (Table 1).
Several sensitivity analysis methods were used in this study to detect possible pleiotropy. The results of Cochran’s Q test showed no evidence of heterogeneity or asymmetry among these SNPs. In our study, the tests of MR-Egger and MR-PRESSO showed no horizontal pleiotropy (Table 2). Leave-one-out analysis was used to validate the influence of each SNP on the overall causal estimate. After removing each SNP, a systemic MR analysis was conducted again on the remaining SNPs, and the results remained consistent, indicating that all SNP estimations contribute significantly to the causal association. This also indicates that no dominant SNPs exist for PC16 with GBM, PC16 with FGF21, and FGF21 with GBM, and previous MR results are valid (Supplementary Figures S1–S3).
This study employs the statistical result data from large-scale GWAS to analyze the causal relationship between PC16 and GBM, using a two-step Mendelian Randomization (MR) approach to assess the mediating effect of FGF21. The results suggest that PC16 is a risk factor for GBM, which can be mitigated through the mediation of FGF21 expression, thereby increasing the risk of GBM. MR-Egger and MR-PRESSO test analyses indicate that the MR findings are not affected by pleiotropy, and no dominant SNPs were present.
Phosphatidylcholine is not only a major component of cellular biomembranes but also plays a significant role in cellular signaling pathways. To accommodate the increased proliferation rate of cancer cells, an activation of lipid biosynthesis is observed to provide sufficient fatty acids for new membrane generation (22). Previous in vivo and in vitro MRS studies have shown that higher-grade gliomas exhibit elevated total choline levels compared to lower-grade gliomas (23–25), with other various tumors also demonstrating increased cellular total choline metabolites, including choline, phosphocholine, and phosphatidylcholine (10, 11). However, the potential causal relationship and mechanisms of action between PC16 and GBM have not been studied. This study establishes a causal relationship between the two.
FGF21, a hormone secreted by the liver, regulates simple sugar intake via signal transduction through the FGF21 receptor in the paraventricular nucleus of the hypothalamus, and is associated with a reduction in dopamine neurotransmission in the nucleus accumbens. FGF21 acts as a downstream metabolic target of oncogenes or as a corrective metabolic suppressor in tumor lesions (26, 27), with its overexpression antagonizing the development of liver and pancreatic cancers (28, 29). Furthermore, FGF21 inhibits pro-tumorigenic factors, including ER stress, ROS stress, and mitochondrial dysfunction (30–32). However, in GBM, upregulation of FGF21 gene expression promotes glioma cell protrusion elongation (33). Additionally, FGF21 may be inactivated by cleavage by Fibroblast Activation Protein (FAP), affecting GBM cell metabolic regulation and tumor progression (34, 35), This aligns with our findings that FGF21 may mediate the causal relationship between PC16 and GBM as an intermediary factor.
This study exhibits several strengths. To the best of our knowledge, this is the first MR study to investigate the causal relationship between PC16 and GBM, incorporating FGF21 to analyze the mediation proportion in this causal relationship. We minimized confounding factors and reverse causality to avoid potential biases inherent in observational studies. Alternative methods like MR-Egger, weighted median, and BWMR were used, and results were validated through sensitivity analysis, providing robust outcomes.
This study also has certain limitations. Firstly, although the data used encompass a large sample size, they are sourced from European ancestry populations. The expression of PC16, incidence of GBM, and expression of FGF21 vary among different populations. Whether the study results can be generalized to other populations requires further research using relevant GWAS data from diverse populations. Secondly, while the study employed an MR design and strived to rule out known confounders, the results may still be influenced by unconsidered potential confounders. Finally, while MR is a theoretical method for analyzing causal relationships, further laboratory research is needed to explore the specific biological connections between PC16 and GBM.
In conclusion, this study confirmed the causal relationship between PC16 and GBM through MR analysis and identified that FGF21 plays a vital mediating role in this causal relationship.
The original contributions presented in the study are included in the article/Supplementary Material. Further inquiries can be directed to the corresponding authors.
PW: Supervision, Writing – review & editing. XZ: Writing – original draft. BX: Data curation, Writing – review & editing. JO: Data curation, Writing – review & editing. JZ: Formal Analysis, Writing – review & editing. XP: Conceptualization, Methodology, Writing – review & editing.
The author(s) declare that financial support was received for the research, authorship, and/or publication of this article. This study was supported by President Foundation of The Fifth Affiliated Hospital, Southern Medical University (No. YZ2020MS04).
Summary statistics for the genetic associations with PC16, FGF21, and GBM were obtained from GWAS respectively by Ottensmann et al., Zhao, J.H et al. and FennGenn consortium. We would like to thank the researchers and study participants for their contributions.
The authors declare that the research was conducted in the absence of any commercial or financial relationships that could be construed as a potential conflict of interest.
All claims expressed in this article are solely those of the authors and do not necessarily represent those of their affiliated organizations, or those of the publisher, the editors and the reviewers. Any product that may be evaluated in this article, or claim that may be made by its manufacturer, is not guaranteed or endorsed by the publisher.
The Supplementary Material for this article can be found online at: https://www.frontiersin.org/articles/10.3389/fonc.2024.1428025/full#supplementary-material
Supplementary Figure 1 | the leave-one-out plots of the sensitivity analysis for PC16 on GBM. PC16, phosphatidylcholine 16:0_22:6; GBM, glioblastoma; MR, Mendelian randomization analysis.
Supplementary Figure 2 | the leave-one-out plots of the sensitivity analysis for PC16 on FGF21. PC16, phosphatidylcholine 16:0_22:6; FGF21, fibroblast growth factor 21; MR, Mendelian randomization analysis.
Supplementary Figure 3 | the leave-one-out plots of the sensitivity analysis for FGF21 on GBM. FGF21, fibroblast growth factor 21; GBM, glioblastoma; MR, Mendelian randomization analysis.
1. Ostrom QT, Price M, Neff C, Cioffi G, Waite KA, Kruchko C, et al. CBTRUS statistical report: primary brain and other central nervous system tumors diagnosed in the United States in 2015–2019. Neuro-Oncology. (2022) 24:v1–v95. doi: 10.1093/neuonc/noac202
2. Ostrom QT, Bauchet L, Davis FG, Deltour I, Fisher JL, Langer CE, et al. The epidemiology of glioma in adults: a ‘state of the science’ review. Neuro Oncol. (2014) 16:896–913. doi: 10.1093/neuonc/nou087
3. Louis DN, Ohgaki H, Wiestler OD, Cavenee WK, Burger PC, Jouvet A, et al. The 2007 WHO classification of tumours of the central nervous system. Acta Neuropathol. (2007) 114:97–109. doi: 10.1007/s00401-007-0243-4
4. Schaff LR, Mellinghoff IK. Glioblastoma and other primary brain Malignancies in adults: A review. JAMA. (2023) 329:574–87. doi: 10.1001/jama.2023.0023
5. McKinnon C, Nandhabalan M, Murray SA, Plaha P. Glioblastoma: clinical presentation, diagnosis, and management. BMJ. (2021) 374:n1560. doi: 10.1136/bmj.n1560
6. Ou A, Yung WKA, Majd N. Molecular mechanisms of treatment resistance in glioblastoma. IJMS. (2020) 22:351. doi: 10.3390/ijms22010351
7. Aronson JK. Phosphatidylcholine. In: Meyler’s side effects of drugs. Elsevier Science Press. p. 722–3. doi: 10.1016/B978-0-444-53717-1.00147-5
8. Ridgway ND. The role of phosphatidylcholine and choline metabolites to cell proliferation and survival. Crit Rev Biochem Mol Biol. (2013) 48:20–38. doi: 10.3109/10409238.2012.735643
9. Butler LM, Perone Y, Dehairs J, Lupien LE, de Laat V, Talebi A, et al. Lipids and cancer: Emerging roles in pathogenesis, diagnosis and therapeutic intervention. Adv Drug Delivery Rev. (2020) 159:245–93. doi: 10.1016/j.addr.2020.07.013
10. Glunde K, Ackerstaff E, Mori N, Jacobs MA, Bhujwalla ZM. Choline phospholipid metabolism in cancer: consequences for molecular pharmaceutical interventions. Mol Pharmaceutics. (2006) 3:496–506. doi: 10.1021/mp060067e
11. Katz-Brull R, Seger D, Rivenson-Segal D, Rushkin E, Degani H. Metabolic markers of breast cancer: enhanced choline metabolism and reduced choline-ether-phospholipid synthesis. Cancer Res. (2002) 62:1966–70.
12. Fisher FM, Maratos-Flier E. Understanding the physiology of FGF21. Annu Rev Physiol. (2016) 78:223–41. doi: 10.1146/annurev-physiol-021115-105339
13. Huang X, Hu J, Li Y, Zhuyun Yang Z, Zhu H, Zhou L, et al. The cell adhesion molecule L1 regulates the expression of FGF21 and enhances neurite outgrowth. Brain Res. (2013) 1530:13–21. doi: 10.1016/j.brainres.2013.07.043
14. Leng Y, Wang Z, Tsai LK, Leeds P, Fessler EB, Wang J, et al. FGF-21, a novel metabolic regulator, has a robust neuroprotective role and is markedly elevated in neurons by mood stabilizers. Mol Psychiatry. (2015) 20:215–23. doi: 10.1038/mp.2013.192
15. Yu Y, Bai F, Wang W, Liu Y, Yuan Q, Qu S, et al. Fibroblast growth factor 21 protects mouse brain against d-galactose induced aging via suppression of oxidative stress response and advanced glycation end products formation. Pharmacol Biochem Behav. (2015) 133:122–31. doi: 10.1016/j.pbb.2015.03.020
16. Lawlor DA, Harbord RM, Sterne JAC, Timpson N, Davey Smith G. Mendelian randomization: Using genes as instruments for making causal inferences in epidemiology. Stat Med. (2008) 27:1133–63. doi: 10.1002/sim.3034
17. Emdin CA, Khera AV, Kathiresan S. Mendelian randomization. JAMA. (2017) 318:1925. doi: 10.1001/jama.2017.17219
18. Ottensmann L, Tabassum R, Ruotsalainen SE, Gerl MJ, Klose C, Widén E, et al. Genome-wide association analysis of plasma lipidome identifies 495 genetic associations. Nat Commun. (2023) 14:6934. doi: 10.1038/s41467-023-42532-8
19. Zhao JH, Stacey D, Eriksson N, Macdonald-Dunlop E, Hedman ÅK, Kalnapenkis A, et al. Genetics of circulating inflammatory proteins identifies drivers of immune-mediated disease risk and therapeutic targets. Nat Immunol. (2023) 24:1540–51. doi: 10.1038/s41590-023-01588-w
20. Kurki MI, Karjalainen J, Palta P, Sipilä TP, Kristiansson K, Donner KM, et al. FinnGen provides genetic insights from a well-phenotyped isolated population. Nature. (2023) 613:508–18. doi: 10.1038/s41586-022-05473-8
21. Bowden J, Del Greco M F, Minelli C, Davey Smith G, Sheehan N, Thompson J, et al. A framework for the investigation of pleiotropy in two-sample summary data Mendelian randomization. Stat Med. (2017) 36:1783–802. doi: 10.1002/sim.7221
22. Kuhajda FP. Fatty acid synthase and cancer: new application of an old pathway. Cancer Res. (2006) 66:5977–80. doi: 10.1158/0008-5472.CAN-05-4673
23. Howe FA, Barton SJ, Cudlip SA, Stubbs M, Saunders DE, Murphy M, et al. Metabolic profiles of human brain tumors using quantitative in vivo1 H magnetic resonance spectroscopy. Magnetic Resonance Med. (2003) 49:223–32. doi: 10.1002/mrm.10367
24. Sabatier J, Gilard V, Malet-Martino M, Ranjeva JP, Terral C, Breil S, et al. Characterization of choline compounds with in vitro 1H magnetic resonance spectroscopy for the discrimination of primary brain tumors. Invest Radiol. (1999) 34:230–5. doi: 10.1097/00004424-199903000-00013
25. Righi V, Roda JM, Paz J, Mucci A, Tugnoli V, Rodriguez-Tarduchy G, et al. 1 H HR-MAS and genomic analysis of human tumor biopsies discriminate between high and low grade astrocytomas. NMR Biomed. (2009) 22:629–37. doi: 10.1002/nbm.1377
26. Singhal G, Kumar G, Chan S, Fisher FM, Ma Y, Vardeh HG, et al. Deficiency of fibroblast growth factor 21 (FGF21) promotes hepatocellular carcinoma (HCC) in mice on a long term obesogenic diet. Mol Metab. (2018) 13:56–66. doi: 10.1016/j.molmet.2018.03.002
27. Lu W, Li X, Luo Y. FGF21 in obesity and cancer: New insights. Cancer Lett. (2021) 499:5–13. doi: 10.1016/j.canlet.2020.11.026
28. Huang X, Yu C, Jin C, Yang C, Xie R, Cao D, et al. Forced expression of hepatocyte-specific fibroblast growth factor 21 delays initiation of chemically induced hepatocarcinogenesis. Mol Carcinog. (2006) 45:934–42. doi: 10.1002/(ISSN)1098-2744
29. Luo Y, Yang Y, Liu M, Wang D, Wang F, Bi Y, et al. Oncogenic KRAS reduces expression of FGF21 in acinar cells to promote pancreatic tumorigenesis in mice on a high-fat diet. Gastroenterology. (2019) 157:1413–1428.e11. doi: 10.1053/j.gastro.2019.07.030
30. Ye M, Lu W, Wang X, Wang C, Abbruzzese JL, Liang G, et al. FGF21-FGFR1 coordinates phospholipid homeostasis, lipid droplet function, and ER stress in obesity. Endocrinology. (2016) 157:4754–69. doi: 10.1210/en.2016-1710
31. Planavila A, Redondo-Angulo I, Ribas F, Garrabou G, Casademont J, Giralt M, et al. Fibroblast growth factor 21 protects the heart from oxidative stress. Cardiovasc Res. (2015) 106:19–31. doi: 10.1093/cvr/cvu263
32. Wang X-M, Xiao H, Liu LL, Cheng D, Li XJ, Si LY, et al. FGF21 represses cerebrovascular aging via improving mitochondrial biogenesis and inhibiting p53 signaling pathway in an AMPK-dependent manner. Exp Cell Res. (2016) 346:147–56. doi: 10.1016/j.yexcr.2016.06.020
33. Leng Y, Wang J, Wang Z, Liao HM, Wei M, Leeds P, et al. Valproic acid and other HDAC inhibitors upregulate FGF21 gene expression and promote process elongation in glia by inhibiting HDAC2 and 3. IJNPPY. (2016) 19:pyw035. doi: 10.1093/ijnp/pyw035
34. Dunshee DR, Bainbridge TW, Kljavin NM, Zavala-Solorio J, Schroeder AC, Chan R, et al. Fibroblast activation protein cleaves and inactivates fibroblast growth factor 21. J Biol Chem. (2016) 291:5986–96. doi: 10.1074/jbc.M115.710582
Keywords: PC16, FGF21, Mendelian randomization, mediation, GBM
Citation: Wang P, Zhang X, Xiao B, Ouyang J, Zhang J and Peng X (2024) Role of FGF21 in mediating the effect of phosphatidylcholine on GBM. Front. Oncol. 14:1428025. doi: 10.3389/fonc.2024.1428025
Received: 05 May 2024; Accepted: 15 August 2024;
Published: 02 September 2024.
Edited by:
Alison Colquhoun, University of São Paulo, BrazilReviewed by:
Juliano Andreoli Miyake, Federal University of Santa Catarina, BrazilCopyright © 2024 Wang, Zhang, Xiao, Ouyang, Zhang and Peng. This is an open-access article distributed under the terms of the Creative Commons Attribution License (CC BY). The use, distribution or reproduction in other forums is permitted, provided the original author(s) and the copyright owner(s) are credited and that the original publication in this journal is cited, in accordance with accepted academic practice. No use, distribution or reproduction is permitted which does not comply with these terms.
*Correspondence: Xiaobin Peng, cHhiMjAxMTA2MDdAc211LmVkdS5jbg==; Jingjing Zhang, emhhbmdqamluZzIwMjNAMTYzLmNvbQ==
†These authors share first authorship
Disclaimer: All claims expressed in this article are solely those of the authors and do not necessarily represent those of their affiliated organizations, or those of the publisher, the editors and the reviewers. Any product that may be evaluated in this article or claim that may be made by its manufacturer is not guaranteed or endorsed by the publisher.
Research integrity at Frontiers
Learn more about the work of our research integrity team to safeguard the quality of each article we publish.