- 1Department of Urology, University Hospitals Leuven, Leuven, Belgium
- 2Laboratory of Experimental Urology, Urogenital, Abdominal and Plastic Surgery, Department of Development and Regeneration, KU Leuven, Leuven, Belgium
- 3Laboratory for Cytogenetics and Genome Research, KU Leuven, Leuven, Belgium
- 4Center for Human Genetics, University Hospitals Leuven, Leuven, Belgium
- 5Department of Pathology, AZ Klina, Brasschaat, Belgium
Bladder cancer (BC) is the most common malignancy of the urinary tract. About 75% of all BC patients present with non-muscle-invasive BC (NMIBC), of which up to 70% will recur, and 15% will progress in stage and grade. As the recurrence and progression rates of NMIBC are strongly associated with some clinical and pathological factors, several risk stratification models have been developed to individually predict the short- and long-term risks of disease recurrence and progression. The NMIBC patients are stratified into four risk groups as low-, intermediate-, high-risk, and very high-risk by the European Association of Urology (EAU). Significant heterogeneity in terms of oncological outcomes and prognosis has been observed among NMIBC patients within the same EAU risk group, which has been partly attributed to the intrinsic heterogeneity of BC at the molecular level. Currently, we have a poor understanding of how to distinguish intermediate- and (very-)high-risk NMIBC with poor outcomes from those with a more benign disease course and lack predictive/prognostic tools that can specifically stratify them according to their pathologic and molecular properties. There is an unmet need for developing a more accurate scoring system that considers the treatment they receive after TURBT to enable their better stratification for further follow-up regimens and treatment selection, based also on a better response prediction to the treatment. Based on these facts, by employing a multi-layered –omics (namely, genomics, epigenetics, transcriptomics, proteomics, lipidomics, metabolomics) and immunohistopathology approach, we hypothesize to decipher molecular heterogeneity of intermediate- and (very-)high-risk NMIBC and to better stratify the patients with this disease. A combination of different –omics will provide a more detailed and multi-dimensional characterization of the tumor and represent the broad spectrum of NMIBC phenotypes, which will help to decipher the molecular heterogeneity of intermediate- and (very-)high-risk NMIBC. We think that this combinatorial multi-omics approach has the potential to improve the prediction of recurrence and progression with higher precision and to develop a molecular feature-based algorithm for stratifying the patients properly and guiding their therapeutic interventions in a personalized manner.
1 Introduction and background
Bladder cancer (BC) is the most common malignancy of the urinary tract and is the sixth most commonly diagnosed form of cancer in Europe, while it ranked ninth worldwide (1, 2). Moreover, the estimated number of new cases will almost double from 2022 to 2050 (3). Although BC is approximately four times more commonly seen in males, females are more frequently diagnosed with advanced-stage disease when compared to males in the same age group (4). This gender discrepancy is partially attributed to gender differences in smoking, which can also explain the increasing incidence of BC in women in developed countries. Tobacco smoking is by far the principal risk factor for the development of BC, accounting for approximately 50-65% of new cases each year. Occupational exposure to carcinogens (including aromatic amines, polycyclic aromatic hydrocarbons, and chlorinated hydrocarbons) is the second most frequent preventable risk factor for BC in industrialized countries. These compounds are commonly found in industries where dyes, paint, metal, rubber, and petroleum products are produced (5, 6). Apart from a history of pelvic radiotherapy, the other risk factors for BC, for which the International Agency for Research on Cancer (IARC) has reported sufficient evidence, are environmental exposures (arsenic, X or gamma radiation), medications (cyclophosphamide, chlornaphazine), opium consumption, and Schistosoma infection (7). Moreover, candidate association studies have unveiled that the gene polymorphisms of N-acetyltransferase (NAT-2) and glutathione S transferases (GSTM1 and GSTT1) (detoxifying arylamines and polycyclic hydrocarbons), and of arsenic (+3 oxidation state) methyltransferase (AS3MT) are associated with a higher risk of BC (6, 8). At present, Lynch syndrome, caused by germline mutations in DNA mismatch repair (MMR) genes (MSH2, MSH6, MLH1, PSM2, and EPCAM), remains the only identified hereditary cancer syndrome associated with a higher BC risk (8).
More than 90% of BCs in Europe and North America are urothelial carcinomas (UC), derived from the urothelium. Confirmation of the diagnosis and clinical staging is performed by transurethral resection of the bladder tumor (TURBT). At initial presentation, 25% of cases are diagnosed with muscle-invasive BC (MIBC). These patients have aggressive disease, as approximately one-third have undetected metastases, while 25% have lymph node involvement (9). Treatment modalities are well defined by international guidelines, with aggressive combination treatments, such as radical cystectomy with (neo-)adjuvant chemotherapy, to be recommended (9). The remaining 75% of BC patients present with non–muscle-invasive BC (NMIBC), disease confined to the mucosa (stage Ta, carcinoma in situ [CIS]) or submucosa (stage T1). Up to 70% of the NMIBC cases will recur, and 15% will progress in stage and grade (10). Therefore, NMIBC patients are scheduled to undergo frequent monitoring, currently based on cystoscopy and cytology, which makes BC one of the costliest of all cancers to manage (11).
The recurrence and progression rates of NMIBC are strongly associated with several clinical and pathological factors. To individually predict the short- and long-term risks of disease recurrence and progression, the European Organization for Research and Treatment of Cancer (EORTC) Genito-Urinary Cancer Group (GUCG) has developed a risk calculator consisting of a scoring system and risk tables (12). The EORTC risk calculator is the result of a post-hoc statistical analysis of 2596 patients from seven separate prospective trials with 291 to 517 included patients. These patients, treated between 1979 and 1989, were categorized by the old (pre-2004) World Health Organization (WHO) grading system. Because only a minority of patients (n=171) in the EORTC cohort were treated with bacillus Calmette-Guérin (BCG), and none of them received maintenance treatment (which is now considered mandatory for at least 12 months to lead to effect), the Spanish CUETO (Club Urologico Español de Tratamiento Oncologico) consortium developed another risk stratification model based on a total of 1062 patients treated with BCG between February 1990 and May 1999 in 4 prospective trials (13). Both risk calculators have poor discrimination for prognostic outcomes in external validation (14, 15). In a Brazilian cohort, the discriminative ability of the EORTC model overestimated the short- and long-term progression rates, especially in high-risk patients (14). Another retrospective multicentric study demonstrated that both the EORTC and the CUETO risk calculators overestimated the risk of disease recurrence and progression in high-risk patients. Moreover, it was observed that these overestimations remained in the BCG-treated patients, especially when the EORTC model was used (15).
Based on clinically available prognostic factors and, in particular, the data from the EORTC risk tables, the European Association of Urology (EAU) Guidelines recommend stratification of NMIBC patients into four risk groups: low, intermediate, high, and very high risk. Specific treatment recommendations have been defined for these groups (9). Low-risk patients have a low risk of disease progression and a low to moderate risk of recurrence. With the defined treatment modalities, these patients have excellent survival. Management of intermediate- and (very-)high-risk NMIBC consists of TURBT and bladder instillations with chemotherapeutics or BCG plus intensive follow-up. Despite this intensive treatment and follow-up schedule, these patients have a high risk for disease recurrence (73-84%) and a moderate risk for progression to MIBC (8.1-14%) at 5-years. On the other hand, very high-risk tumors have a very high probability of disease progression (29-54%). Equally, the rate of BCG non-responders amounts to 40% (9).
Patients with NMIBCs in the same EAU risk group can have significant heterogeneity in terms of oncological outcomes and prognosis. The failure to accurately predict outcomes of this scoring system, which is solely based on clinico-pathological features, may be partly attributed to the intrinsic heterogeneity of BC at the molecular level. The generation of large-scale, high-throughput molecular data and the development of new profiling technologies and analytical algorithms have led to molecular subtyping of the disease (16). Early molecular profiling revealed evidence for a dual-track model according to which BC develops from two distinct pathways - the papillary and the non-papillary pathway (17–19). The papillary NMIBCs develop via urothelial hyperplasia and are associated with disruption of the PI3K-AKT-mTOR (Phosphoinositide 3-kinase/Protein kinase B/Mammalian target of rapamycin) pathway and mutations in the FGFR3 and HRAS genes (20, 21). The non-papillary MIBCs develop from flat dysplasia and CIS and are characterized by genetic alterations in tumor suppressor genes that regulate cell cycle and apoptosis (TP53, CDKN2A, CCND1, CDKN1B, and RB1) (20, 21). Although this model includes many characteristic features of BC, it does not fully address the heterogeneity of the disease (18, 19).
Based on gene expression, the first molecular classification of mixed samples of MIBC and NMIBC revealed five different subtypes (22). Later, two distinct subtypes, basal-like and non-basal-like, were identified again through transcriptomic analysis of MIBC with NMIBC (23). Molecular classification of only MIBC based on gene expression showed two main distinct subtypes: luminal and basal (24). Further research revealed first three different subtypes and then four subtypes, similar to those of breast cancer (Figures 1A, B) (25–27). The revised classifications by The Cancer Genome Atlas and Lund University showed five and six subtypes, respectively (Figure 1C) (28, 29). However, these subclassifications were based on DNA/RNA analyses performed mainly on retrospective cohorts of MIBC patients, with very little data from NMIBC patients. With the intense work on this subclassification, a consensus has been recently reached, which showed six different molecular subtypes in MIBC (Figure 1D) (30).
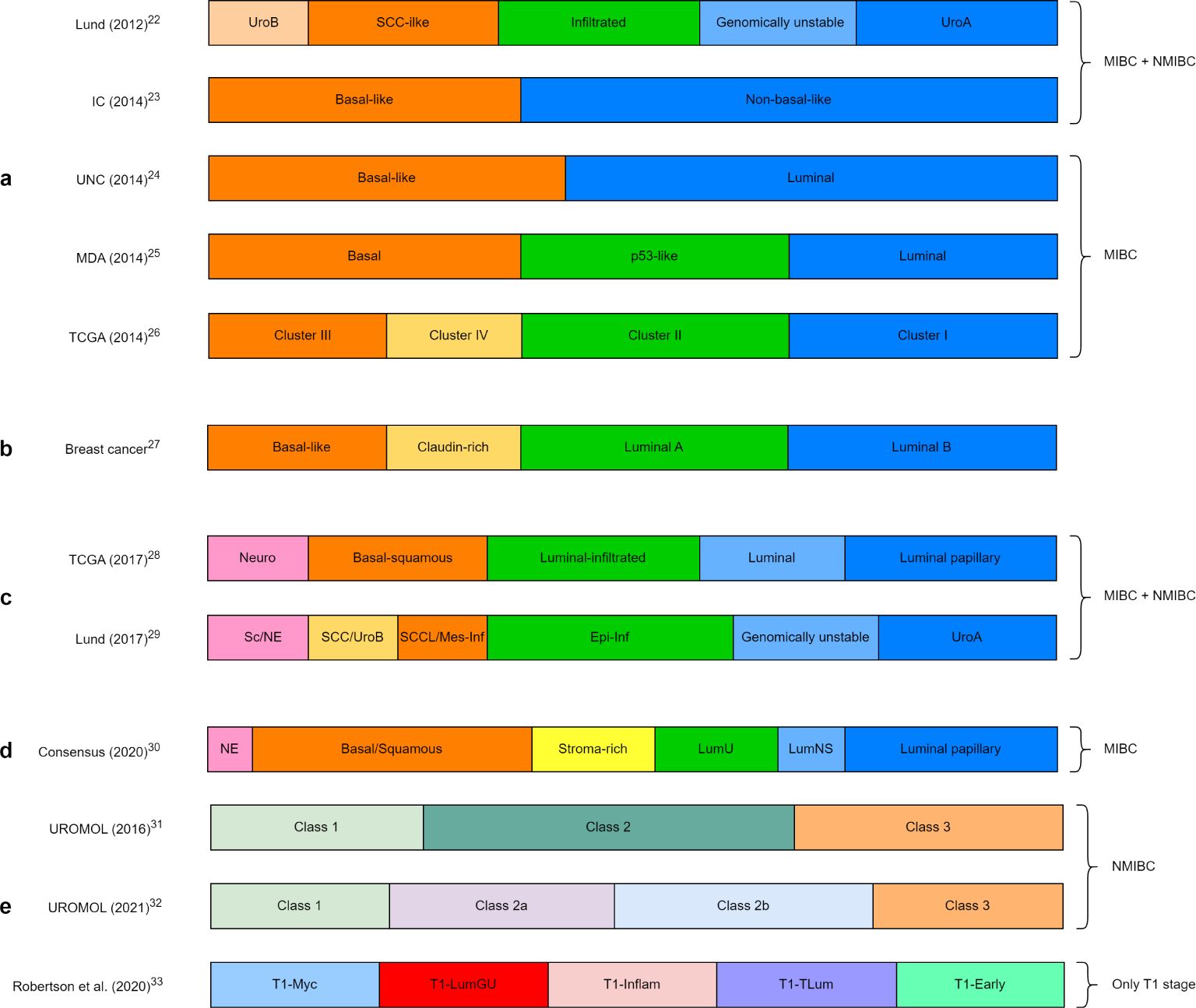
Figure 1. Molecular subclassification of bladder cancer by different research groups: (A) initial molecular classification of bladder cancer, (B) molecular classification of breast cancer, (C) recent molecular classification of MIBC, (D) consensus classification of MIBC, (E) molecular classification of only NMIBC and only T1 stage. Lund, Lund University; IC, Institute Curie; UNC, University of North Carolina; MDA, MD Anderson Cancer Center; TCGA, The Cancer Genome Atlas; UroA, Urobasal A; UroB, Urobasal B; SCC, Squamous cell carcinoma; Neuro, Neuronal; Epi-Inf, Epithelial-infiltrated; SCCL, SCC-like; Mes-Inf, Mesanchymal-infiltrated; Sc, Small-cell; NE, neuro-endocrine-like; LumU, Luminal unstable; LumNS, Luminal non-specified; LumGU, Luminal genomically unstable; Inflam, Inflammed; TLum, True luminal.
Comprehensive transcriptome profiling has revealed the presence of three biological subclasses in NMIBC (UROMOL Class 1, 2, and 3), thus different from that of MIBC (Figure 1E) (31). With the recent update of this cohort with an integrated multi-omics analysis (genomics, transcriptomics, and spatial proteomics), four subclasses (UROMOL2021 Class 1, 2a, 2b, and 3) were identified (Figure 1E) (32). Another recent subclassification of only the T1 stage showed five different molecular subtypes (Figure 1E) (33).
The current significant challenges and unmet needs are to better understand and model the disease at the molecular level, to unveil and validate new subtype-specific molecular biomarkers, and to improve the current stratification models by integrating new molecular information. Molecular data is starting to emerge in NMIBC; however, it will still take time to incorporate this data into current models. Further research is required, and then the translation of the novel findings into the clinical routine by independent randomized studies is needed for an accurate stratification of intermediate-/(very-)high-risk NMIBC to identify which patients are at the highest risk for disease progression, to predict treatment response, to identify novel targets for treatment, and to improve existing management modalities. Besides genetic alterations (mutations, copy number alterations [CNA], single nucleotide variations [SNV], insertions-deletions [indels], loss of heterozygosity [LOH], translocations, tumor mutational burden [TMB], microsatellite instability [MSI]), additional layers of information can be gained by studying control of gene activity and expression (DNA methylation, histone modification or short/long non-coding RNAs – epigenetics), RNA-RNA and RNA-protein interactions (transcriptomics), protein composition, structure and activity, and protein-protein interactions (proteomics), and unique chemical fingerprints of specific cellular processes (metabolomics-lipidomics), which might expand the current typing even further.
2 The hypothesis
It is now well known that cell functions, such as the synthesis of peptides/proteins or other metabolites, are more complex processes than the ones explained by the central dogma of molecular biology. As Figure 2 depicts, alterations in each step, including replication, transcription, and translation, e.g., epigenetic regulations of genes, transcriptional regulations (RNA processing), translational regulations, and post-translational modifications of proteins, and crosstalk between different processes can all be associated with the development of cancer. Apart from the ‘in-tumor’ processes, there are also other biological pathways that are driven by different cells (such as tumor microenvironment [TME], immune response, etc.) and external stimuli (carcinogens, lifestyle, radiation, infection, etc.), which play a role in the manifestation of cancer.
Several pathways, commonly altered in oncogenesis and cancer progression in general, also play a significant role in BC (summarized in Figure 3):
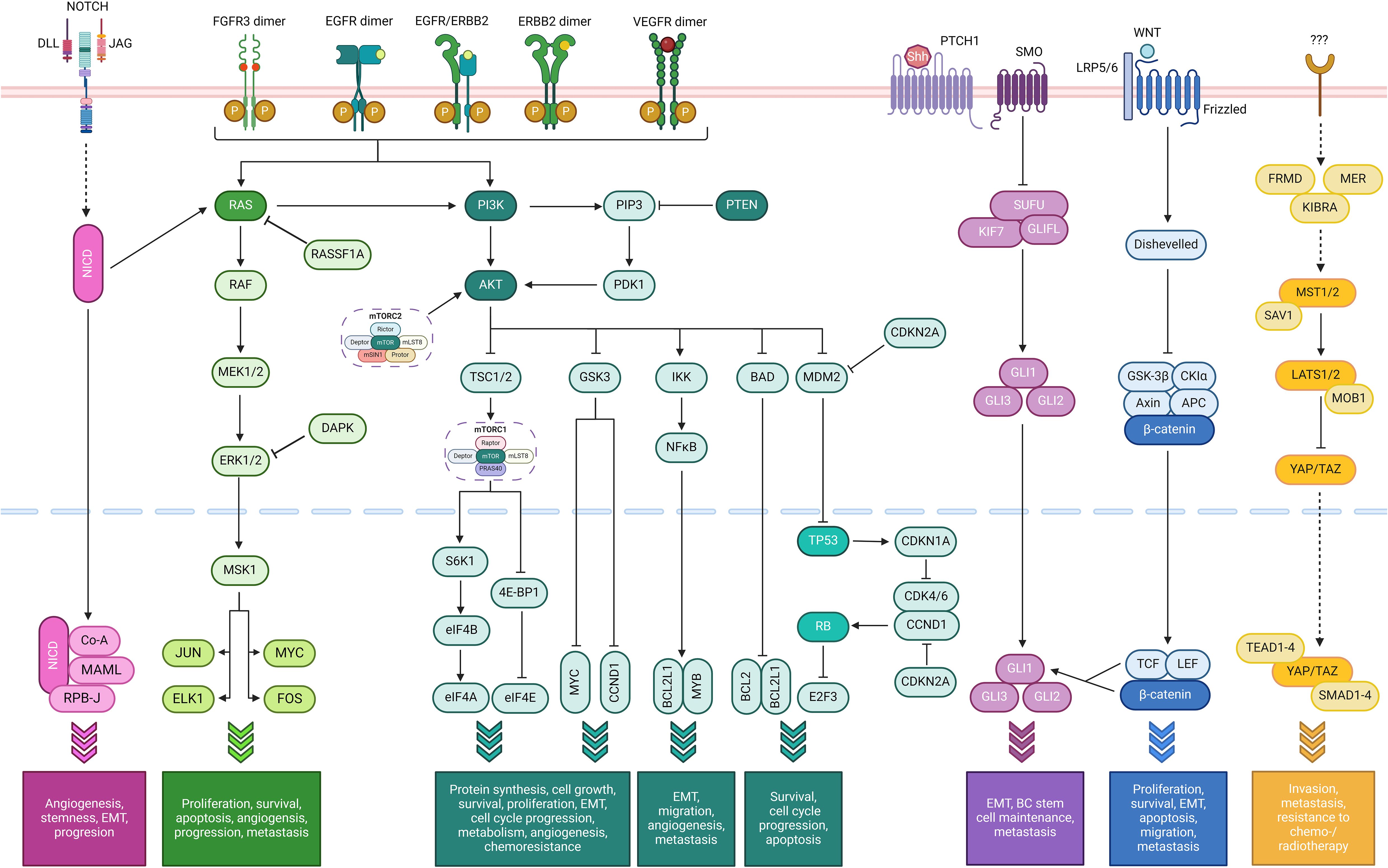
Figure 3. Simplified summary of oncogenic pathways playing a role in bladder cancer. From left to right: the NOTCH pathway (fuchsia), Ras/Raf/MEK/ERK pathway (bright green), the PI3K/AKT/mTOR pathway (dark green) with the TP53 and cell cycle pathways as a part of it (light green, at the very right of the pathway), the sonic hedgehog (SHH) pathway (purple), the Wnt/β-catenin pathway (dark blue), and the Hippo-YAP pathway (orange). The FGFR, VEGF, and ErbB pathways use the Ras/Raf/MEK/ERK and/or the PI3K/AKT/mTOR pathways (Created with BioRender.com).
2.1 TP53 pathway
The tumor suppressor gene TP53, also known as ‘the guardian of the genome’, is the most frequently mutated gene in human tumors, and the process of tumor development is strongly related to the dysfunctions caused by TP53 mutations. The protein coded by this gene, p53, functions primarily as a transcription factor and regulates various cell functions such as cell cycle, apoptosis, autophagy, DNA repair, and metabolism. Mutant TP53 promotes the development and progression of BC through inhibition of apoptosis, alteration of DNA methylation patterns, activation of oncogenic pathways (e.g., PI3K/AKT/mTOR pathway), induction of multiple metabolic changes, modulation of TME with immunosuppressive changes and enhancement of metastatic potential (26, 34–37).
2.2 Cell cycle pathway
The cell cycle is a highly regulated pathway enabling cell growth, duplication of genetic material, and cell division. The cell cycle machinery, composed of proteins (cyclins) and their catalytic partners called cyclic-dependent kinases, drives progression from one cell-cycle phase to another. Interestingly, these proteins play a role in tumorigenesis by affecting not only tumor cells but also TME (e.g., anti-tumor immune response). Dysregulation of this pathway, through amplification or rearrangements of genes encoding D-, E-, A-, and B-cyclins (CCND1, CCND2, CCND3, CCNE1, CCNE2, CCNA1, CCNA2, CCNB1), CDK4, CDK6, CDK2, CDK1, and CDK7, has been documented in a vast number of cancers, including BC (26, 34, 35).
2.3 PI3K/AKT/mTOR pathway
This pathway is essential in regulating the cell cycle. Dysregulation of this pathway, through mutations or amplification in PIK3CA, loss or inactivation of PTEN, hyperactivation of AKT or mTOR, or inactivating mutations in TSC1, can lead to uncontrolled cell growth and survival, altered metabolism, increased angiogenesis and epithelial-to-mesenchymal transition (EMT), and chemoresistance (38, 39).
2.4 Ras/Raf/MEK/ERK pathway (also known as MAPK [mitogen-activated protein kinase] pathway)
It is a critical signal transduction cascade that transmits signals from extracellular stimuli to the nucleus, which in turn influences gene expression and impacts cell proliferation, differentiation, survival, and apoptosis. It is crucial for normal cell functions and is highly conserved evolutionarily across different organisms. It is one of the most commonly altered pathways in cancer, including BC, and its mutations or dysregulations can lead to uncontrolled cell proliferation and reduced apoptosis, which are foundational hallmarks of cancer. In BC, mutations in the components of this pathway, such as HRAS, lead to constitutive activation of the pathway, which promotes tumor initiation and development. This continuous activation also contributes to tumor growth and progression. It can also evade apoptosis by influencing transcription factors that regulate pro-survival genes when activated. The chronic activation of this pathway is also linked to angiogenesis, metastasis, and resistance to specific therapies, particularly those targeting upstream components like tyrosine kinase receptors (38, 40).
2.5 Fibroblast growth factor receptor pathway
This pathway is a critical signaling cascade involved in various cellular processes such as cell proliferation, differentiation, migration, and survival. Its activation is initiated by binding one of 22 defined fibroblast growth factors (FGF) to one of four FGFRs, which leads to the dimerization of receptor and autophosphorylation of the tyrosine kinase domain. Aberrant activation of the FGFR signaling, often due to mutations or overexpression, initiates continuous activation of several downstream pathways, including the Ras/MAPK, PI3K/AKT, and PLCγ (phospholipase C gamma). These pathways promote cellular proliferation, inhibit apoptosis, influence cell behaviors (e.g., loss of contact inhibition, anchorage-independent growth), enhance cell survival mechanisms, and alter the TME, which contributes to oncogenesis and progression of BC (41).
2.6 ErbB pathway
The ErbB family proteins function as cell membrane receptor tyrosine kinases, which are activated following ligand (epidermal growth factor [EGF], transforming growth factor-alpha [TGF-α], neuregulins, etc.) binding and receptor dimerization, regulate several essential cell functions such as cell proliferation, migration, differentiation, apoptosis, and motility. Their organ-specific expression plays a role in cardiac development, synaptic formation, and proliferation/differentiation of glial cells. Overexpression of ERBB1 (encoding epidermal growth factor receptor [EGFR]=ErbB1=Her1) or ERBB2 (encoding ErbB2=Her2) or both contributes to shorter recurrence periods and earlier disease progression in early-stage BCs (38).
2.7 Vascular endothelial factor pathway (also known as angiogenesis pathway)
Activation of the vascular endothelial factor receptors (VEGFRs, particularly VEGFR2) triggers angiogenesis through promoting several processes such as endothelial cell proliferation and migration, increased vascular permeability, endothelial progenitor cell mobilization, and anti-apoptotic effects, which in turn end up with tumor growth, progression, and metastasis (38, 40).
2.8 NOTCH pathway
It is a highly conserved cell signaling system present in most animals and plays a major role in neurogenesis and regulation of embryonic development. This pathway has a dual function in BC, where it suppresses proliferation by upregulating dual-specificity phosphatases when activated and leads to tumorigenesis by ERK1/2 phosphorylation when inactivated. Inactivation of this pathway, through loss-of-function mutations in NOTCH1 and NOTCH2, loss of HES1 expression, and overexpression of NOTCH3 and JAGGED2, contributes to tumor angiogenesis, stemness, EMT, and cancer progression (42).
2.9 Sonic hedgehog pathway
It plays a critical role in organ development, acting as a morphogen involved in patterning many systems, such as several parts of the central nervous system, lungs, teeth, limbs, and digits, and regulating the proliferation and differentiation of adult stem cells. Dysregulation of this pathway by means of mutations in genes encoding its components (e.g., PTCH1, SMO, GLI), epigenetic modifications in the promoter regions of the pathway genes, overexpression of pathway components (SHH ligands, transcription factors), and crosstalk with other signaling pathways, drives key processes such as EMT, BC stem cell maintenance, and lymph node or distant metastasis (43).
2.10 Wnt/β-catenin pathway
This pathway is highly conserved across multiple species and critical to both embryological development and adult tissue homeostasis regeneration. Aberrant activation of this pathway, often through mutations or epigenetic alterations in the pathway components (e.g., APC, CTNNB1, PTEN, Wnt ligands or antagonists), leads to nuclear accumulation of β-catenin and subsequent transcriptional activation of Wnt target genes (e.g., MYC [c-Myc], CCND1), which in turn contribute to uncontrolled proliferation, evasion of apoptosis, promoting EMT, and enhanced metastatic potential (44).
2.11 Hippo-YAP pathway (also known as MST/WW45/LATS pathway)
It is involved in cell growth, apoptosis, homeostasis, and controlling organ size during embryonic development. Dysregulation of this pathway, through, e.g., overexpression of transcription factor YAP (yes-associated protein) or transcription coactivator TAZ (PDZ-binding motif) or decreased expression of MST1/2 and LATS1, plays a role in invasion, metastasis, and resistance to the cytotoxic effects of chemotherapy (especially cisplatin) and radiotherapy (26, 35, 40).
2.12 Histone modification (chromatin regulatory) pathway
Histone modifications such as acetylation, methylation, phosphorylation, ubiquitination, SUMOylation, and ADP-ribosylation regulate chromatin structure and gene expression. Dysregulation of specific histone-modifying enzymes such as histone acetyltransferases, histone deacetylases (HDACs), histone demethylases or their catalytic subunits (e.g., EZH2), through overexpression and loss-of-function mutations in the encoding genes (KDM6A, HDAC1, HDAC2, HDAC3, EZH2, MLL2 [KMT2D], SETD2), plays role in cancer initiation, increased genomic instability and aggressiveness, stemness, progression, and metastasis (26, 35, 38).
2.13 SWI/SNF (SWItch/Sucrose Non-Fermentable) complex
This complex is a subfamily of ATP-dependent chromatin remodeling proteins and regulates transcription of specific genes by altering the chromatin structure and functions as a tumor suppressor in cancers. Mutations in the genes encoding the subunits of the SWI/SNF complex such as ARID1A (the most frequent), ARID1B, SMARCA4, SMARCA2, SMARCC2, SMARCC1, and PBRM1, promote several key hallmarks of cancer such as cell proliferation and survival, invasion, stemness, and interactions with the other oncogenic pathways, which in turn increases the aggressiveness of BC (26, 40).
Apart from the abovementioned ones, there are also other pathways involved in tumorigenesis and progression of BC, which play a role in cancer cell metabolism and cancer stem cells such as IL6/IL6R/STAT3, COX2/PGE2/SOX2, ALDH1A1/TUBB3, ARRB/ALDH/CD44 pathways (40). External carcinogenic stimuli such as cigarette smoke and occupational exposure cause BC development through several mechanisms, including formation of DNA abducts and oxidative DNA damage (e.g., 8-oxodeoxyguanosine), accumulation of somatic mutations, aberrant DNA methylation (hypermethylation of tumor suppressor genes, hypomethylation of oncogenes), histone modifications (acetylation, methylation, other post-translational modifications), microRNA dysregulation, platelet-activating factor (PAF) accumulation, activation of multiple pathways (e.g., STAT3 and ERK1/2 by nicotine), and affecting EMT process (45, 46). Even though the effect of smoking on disease recurrence has been widely studied, it is less investigated for disease progression, and the results have been ambiguous (47). Very few studies could find an association between smoking and progression. In contrast, the others have failed to do so, probably due to the limited power of studies because of a relatively small number of patients and/or events and progression being not the primary endpoint of those studies. A recent systematic review and meta-analysis has found that the risk of progression was not increased for smokers vs. never-smokers, while ever-smokers had a compromised progression-free survival both for all patients and subgroups of high-risk and BCG-treated patients (48). On the other hand, several studies have shown that cigarette smoke induced the initiation and progression of BC and mediated the EMT and ERK1/2 pathway (49). A very recent study using single-cell and multi–omics analyses identified 33 tobacco carcinogens-related genes and constructed a prognostic score that showed high-risk patients had significantly worse overall survival. This study also highlighted that cancer-associated fibroblasts mediated the crosstalk between EMT and immune evasion, which in turn played a role in disease progression (50). A serum metabolic profiling study identified 40 metabolites, including an increased abundance of amino acids (tyrosine, phenylalanine, proline, serine, valine, isoleucine, glycine, and asparagine) and taurine, in smoker BC patients. An integromic analysis of differential metabolomic gene signature and transcriptomics data revealed an intersection of 17 genes (catechol-O-methyltransferase, iodotyrosine deiodinase, and tubulin tyrosine ligase being the most important ones) that showed a significant correlation with the survival of smokers with BC (51).
Even though our current knowledge about how these oncogenic pathways is dysregulated has increased enormously with recent studies, there are still unknowns. More importantly, revealing these pathways individually is not enough, as the crosstalks between these pathways make the whole process much more complicated. Understanding the missing parts and the relationships of these pathways with each other can facilitate the development of novel diagnostic and therapeutic strategies. Moreover, our knowledge is fragmented as most studies focus on only one specific oncogenic pathway. We need to look broader to understand the whole picture. Current technology allows for such multiplatform analyses.
There is an unmet need to develop a more accurate classification system in NMIBC. A better patient stratification for specific follow-up regimens and selected treatments, based on prediction of disease prognosis and response to treatment, is needed. This will require the assessment of multiple biological parameters. Based on these assumptions, by employing a multi-layered –omics (namely, genomics, epigenetics, transcriptomics, proteomics, lipidomics, metabolomics) and immunohistopathological approach, we hypothesize to demonstrate molecular heterogeneity of intermediate- and (very-)high-risk NMIBC and to stratify the patients better.
Overestimation of the risk of disease recurrence and progression in high-risk patients by the EORTC and the CUETO risk calculators and the fact that some researchers have failed to validate these calculators externally can be explained by the inherent disease heterogeneity. The molecular characterization of MIBC has already shown several subgroups with clearly different characteristics (21–29). The same has recently been proved to be true also for NMIBC (30–32).
These facts support our hypothesis that a combinatorial multi–omics approach will be more powerful for detecting and explaining existing disease heterogeneity. Each –omics layer provides unique and different but limited information; however, only combining several –omics with high-performance data linkage using powerful bioinformatics will unravel a better, more detailed, and multi-dimensional characterization of disease mechanism and (novel) treatment targets. Meanwhile, the transfer of the results of multi–omics into immunohistopathology will allow us to use the new data in routine daily practice, even without the need for sophisticated infrastructure.
For this reason, we propose to perform a comprehensive multilayer assessment of the genome, epigenome, transcriptome, proteome, lipidome, metabolome, and immunohistopathological characteristics that represent the broad spectrum of NMIBC phenotypes. This has the potential to improve the prediction of recurrence and progression and to develop an algorithm (based on clinical, pathological, and molecular features) to adequately stratify patients and guide therapeutic interventions in a personalized manner. The lack of predictive value of the currently used risk calculators results in under- or over-treatment of patients, which ultimately leads to poor quality of life, observed high BC fatality rates, and increased treatment costs (11, 52, 53). Moreover, a comprehensive depiction of the mechanism(s) of non-responsiveness to intravesical BCG treatment of NMIBC will reveal unique molecular pathways that can further guide drug development in the future by identifying novel therapeutic targets.
3 Evaluation of the hypothesis
Recent technological improvements in molecular biology have tremendously increased our knowledge of the biological character of cancer, including BC. The data from different –omics studies help us unravel the molecular complexity of BC more efficiently.
3.1 Genomics
Increasing evidence suggests that genetic mutations (germline or somatic) significantly influence the incidence of BC (54, 55). For this reason, most research has focused on detecting genomic alterations. The most recent studies performed on a large number of patients with NMIBC showed that the most frequently altered genes are TERT promoter (73%), FGFR3 (49%-34%), KDM6A (38%-18%), PIK3CA (26%-25%), STAG2 (23%-10%), ARID1A (21%-5%), TP53 (21%-9%), FAT1 (15%-11%), KMT2D (24%-12%), and KMT2C (11%-10%) (the first and second percentages come from Ref. #56 and #32, respectively). RTK/PI3K, TP53/cell cycle, chromatin modification, and DNA damage repair (DDR) are frequently altered pathways (32, 56). The most frequent CNAs in BC involve CDKN2A, TP53, FGFR3, HRAS, ERBB2, TSC1, RB1, PTEN, CCND1, MDM2, and E2F3 (56–58).
TMB is defined as the total number of somatic missense mutations per megabase (Mb) of the tumor’s genomic DNA. It serves as a biomarker for predicting response to immunotherapy, with higher TMB often correlating with better prognosis and increased sensitivity to immune checkpoint inhibitors. In BC, a threshold of 10 mutations/Mb is commonly used to distinguish high from low TMB. Key genes involved in BC with high TMB include TP53, KMT2D, KDM6A, ARID1A, KMT2C, PIK3CA, FAT4, EP300, and RB1. Additionally, some of the MMR (MSH2, MSH6), DDR (ATM, BRCA2, POLQ, CDK12, ATR, BRIP1), and polymerase-encoding genes (POLE, POLD1) are frequently altered in TMB high tumors (59, 60). In the 100,000 Genome Project, the UC of the bladder was found to have median mutations of 7.2/Mb, and 11.9% of the cases had a high TMB, defined as >20 mutations/Mb (61).
Resulting from genomic hypermutability, variations in the length of repetitive sequences (microsatellites) in the entire genomic structure are known as MSI. In tumor cells with MSI, DNA mismatches in microsatellites cannot be repaired due to a deficient MMR machinery, which results in the accumulation of mutations in tumor suppressor genes and/or oncogenes (62). Recent studies have shown that MSI can be used as a predictor of response to immune checkpoint inhibition in various solid organ tumors as well as BC (62). Microsatellite analysis (MSA) can be used to identify both initial or recurrent tumors, with a better sensitivity and specificity than urine cytology, where low-grade and low-stage disease can be detected as accurately as high-grade and high-stage disease (62). LOH is identified by comparing the DNA isolated from tumor tissue to normal DNA, generally isolated from blood, with the MSA. LOH at 9p, 17p, 9q, 8p, 13q, 11p, and 4p have been shown to have prognostic value in NIBC (62).
3.2 Epigenetics
Although genetic mutations are mainly investigated, epigenetics represents more prevalent DNA alterations that can lead to the development and progression of cancer. Epigenetic alterations can be defined as stable molecular changes of the phenotype of a cell that are inheritable during somatic cell divisions (and sometimes germ line transmissions) but do not involve changes in the DNA sequence itself. The major epigenetic phenomena in cancer cells are mediated by several molecular mechanisms comprising DNA hypermethylation, histone modifications, nucleosome remodeling, and RNA-associated silencing (Figure 3).
The most studied epigenetic mechanism of these is DNA hypermethylation that occurs in CpG islands (a cytosine that precedes guanine in a CpG dinucleotide) in promoter regions. Besides the methylated genes common to various cancer types (GSTP1, CDKN2A, RB1, MLH1, APC, PTEN, DAPK1, MSH6, MGMT, RASSF1A, TIMP3, BRCA1, CDH1, VHL, CDKN2B, FHIT, TWIST1, ONECUT2, WIF1, HIC1, PRAC1, SFRP5, RUNX3, SOCS1, etc.) and more specific to BC (ZNF154, HOXA9, POU4F2, EOMES, ACOT11, PCDHGA12, CA3, PTGDR, TBX4, FGFR3, PMF1, PCDH8, PCDH17, GDF15, KISS1, ISL1, ALDH1A3, TBX3, etc.), new candidate genes will be found as further studies are performed, which can be used for screening, diagnostic and prognostic purposes (63–65).
3.3 Transcriptomics
The majority of the molecular research performed on BC depends on transcriptomic analyses. With gene expression studies by using messenger (m) RNA, several molecular subclassifications of BC have been performed. First, binary subtyping, namely luminal and basal, has been proposed (22–24). Later, with further research, up to six subtypes have been identified by different research groups (25–29). With an effort to mitigate the differences and inconsistencies among these molecular subtypings, a consensus subclassification has been performed, which showed six different molecular subtypes: luminal papillary, luminal non-specified, luminal unstable, stroma-rich, basal/squamous, and neuro-endocrine-like (30). Transcriptomic profiling of only NMIBC identified three molecular subtypes (UROMOL2016 Class 1, 2, and 3), which has been recently updated with employing multi-omics and revealed four different subclasses (UROMOL2021 Class 1, 2a, 2b, and 3) (31, 32).
Apart from molecular subtyping studies, numerous research focused on the differential expression of specific genes and their effect on BC formation, progression to muscle-invasive or metastatic disease, prognosis, and response to chemotherapy or immunotherapy. Recent research on micro (mi) RNAs revealed their roles in stratifying patients, detecting disease progression, and predicting clinical outcomes in BC, which have the potential to be used as promising biomarkers (66, 67). An increasing number of recent studies on long non-coding (lnc) RNAs showed their roles in proliferation, differentiation, migration, invasion, apoptosis, and metabolism (e.g., glycolysis) of tumor cells, resistance to cisplatin, stemness, and EMT (68, 69). Additionally, circular (circ) RNAs, another type of small non-coding RNAs, have emerging oncogenic and anti-oncogenic functions, particularly regulating migration, invasion, and drug resistance, in BC (70, 71).
3.4 Proteomics
Proteomics can be broadly classified into discovery and targeted proteomics, which are highly complementary to each other. Discovery proteomics is predominantly conducted using mass spectrometry (MS)-based technologies, which allow comprehensive analysis of proteins and post-translational modifications without the requirement of generating target-specific antibodies. However, it still has several technical challenges, and newer methods are being developed to make it more efficient by increasing its dynamic range of peptide sampling and resolution (72, 73). Liquid chromatography-coupled tandem mass spectrometry (LC-MS/MS) is the gold standard for current proteomics research; however, it cannot identify and quantify specific proteins in complex mixtures with a similar scale and sensitivity to that of next-generation DNA sequencing. The proteomic data can be used for several purposes, such as screening (detection of new or recurrent disease), patient stratification, prediction of treatment response, and identification of novel drugs/drug targets (74).
A recent systematic review has identified the top ten enriched pathways of proteomic biomarkers for BC, namely the immune system, innate immune system, complement cascade, integrin beta 3 cell surface interactions, mesenchymal-to-epithelial transition, EMT, FGFR signaling, c-Myb transcription factor network, endogenous TLR signaling, and Trk receptor signaling mediated by the MAPK pathway (75). Strogglios et al. reported the first proteomic classification of 98 NMIBC samples based on an unbiased comprehensive LC-MS/MS approach, in which three NMIBC proteomic subtypes (NPS) were identified: NPS1 (mostly high stage/grade/risk samples) was the smallest group (17.3%) and overexpressed proteins reflective of an immune/inflammatory phenotype, involved in cell proliferation, unfolded protein response, and DNA damage response. While NPS2 (mixed stage/grade/risk composition) presented with an infiltrated/mesenchymal profile, NPS3 had differentiated/luminal phenotype, in line with its pathological composition (mostly low stage/grade/risk samples) (76). Based on The Cancer Genome Atlas (TCGA) dataset, the immune-related prognostic signature (IRPS) was constructed with seven immune-related genes (STAT3, TGFB1, CTSG, NFKB1, SNRPD2, PDCD1, and TAP1). It was related to poor five-year overall and disease-free survival (77). Dressler et al. have analyzed 242 tumor samples from different stages and identified five proteomic subtypes: PAULA (Proteomic Analysis of the Urothelial cancer LAndscape) 1 was a low-risk cluster with the highest number of samples and the longest survival, where PAULA IIa/IIb/IIc were the intermediate-risk clusters, and PAULA III was the high-risk cluster with the shortest survival (78). While some studies present proteins specifically for disease stage (e.g., coded by PRDX1, UMP/CMPK, GSTM1, PGAM1, PRDX6, PSME1, HSPB-1, ANXA1, and CAPG for NMIBC; BLVRB, PRDX2, and HPGD for MIBC), recent studies reveal novel potential biomarkers for BC in general such as RET, PVRL4, AREG, FGFBP1, WFDC2, ESM-1, SPR, AK1, CD2AP, ADGFR1, GMPS, and C8A (79–81).
3.5 Lipidomics & metabolomics
Even though the exact mechanism is not still clearly unraveled, it has been known for a long time that most cancer cells produce their energy predominantly through anaerobic glycolysis followed by lactic acid fermentation, which is known as the Warburg effect, instead of the usual citric acid cycle and oxidative phosphorylation. Recent research has proven that both cancer development and metastatic disease progression are characterized by a unique reprogramming of cellular energy, glucose, and lipid metabolisms, which is required for the maintenance of rapid proliferation of cancer cells (82–85). Moreover, the deregulation of cellular metabolism is now considered one of the hallmarks of cancer (86).
Recent studies have shown that a variety of characteristic metabolic changes, including increased glucose utilization for glycolysis and de novo fat synthesis, elevated sorbitol pathway intermediates, oxidative metabolism imbalance, glutamine consumption, altered metabolism of membrane lipids, and differential derivation of nucleic acid components pyrimidine and purine, are observed in NMIBC and MIBC (87–89). Piyarathna et al. have reported a progressive decrease in the levels of phosphatidylserine, phosphatidylethanolamines, and phosphocholines, whereas an increase in the levels of diacylglycerols with increasing tumor stage in UC. The levels of diacylglycerols and lyso-phosphatidylethanolamines were significantly elevated in tumors with lymphovascular invasion and lymph node metastasis, respectively (90). Comparative lipidomic profiling of two isogenic human T24 BC cell lines showed reprogrammed lipid metabolism was associated with cisplatin resistance (91). These findings encourage further research to identify various potential biomarkers for non-invasive diagnosis and also for prediction of recurrence and progression in BC.
While these ‘bulk’ profiling methods have offered invaluable insights into the key biological events and the molecular characteristics of mechanistic pathways involved in BC, they lack the ability to show intratumoral heterogeneity, as tissue specimens are processed as a whole and the data originating from different components of the tumor (e.g., tumor cells, immune cells, endothelium, connective and/or muscle tissue cells) cannot be recognized separately. At this point, state-of-the-art technologies such as single-cell/-nucleus sequencing and spatial –omics help fill the existing gaps and increase our knowledge. Very recent research using single-cell and/or spatial transcriptomics, proteomics, and metabolomics has substantially augmented our pre-existing corpus of knowledge by improving our perception of the molecular basis of the intratumoral heterogeneity and tumor cell-TME interaction in BC (92–95).
Even though it has been less than a decade since these two technologies have been commercially available, the number of techniques has increased tremendously, and every single new method tries to compensate for the disadvantages or hurdles of the existing approaches (96). However, there are still some aspects that need to be improved, such as detection efficiency, transcriptome-wide profiling, spatial resolution, sequenced tissue section area, cost, and tissue compatibility/usability. Most of these methods are applicable only to fresh-frozen (FF) tissues, while very few techniques have been implemented in formalin-fixed paraffin-embedded (FFPE) tissues, such as deterministic barcoding in tissue sequencing (DBiT-seq), CellScape (Canopy, Biosciences, St. Louis, MO, USA), and Visium Spatial and Xenium In Situ (10x Genomics, Pleasanton, CA, USA) (97). While FF tissues are disadvantageous as they are inappropriate for prolonged storage, prone to deformation over time, and gene diffusion during tissue permeabilization, the RNA in FFPE samples is of inadequate quality.
On the other hand, whether it is spatial or not, sequencing only one of the abovementioned –omics provides information from a single aspect of the tumor. It would be more promising if altered (mutated, under-/overexpressed) genes, control mechanism(s) and mediator(s) of their (in)activation, expressed and (possibly) modified proteins and their interaction between each other, altered metabolites of the cellular functions, and histopathological anatomy of the cancer tissue could be viewed simultaneously, preferably from the same slide. This holistic approach would extensively deepen our knowledge of cancer pathophysiology. By providing invaluable information from different aspects of the tumor, the (spatial) multi–omics technology provides a comprehensive understanding of the functions and regulations of driver genes, expressed proteins, and metabolites (mid-/end-products of different pathways) for cancer initiation and progression. When it is implemented for NMIBC, this multi-layered large-scale data will help to improve molecular and clinical subtyping, delineate tumor cell behavior, predict tumor response to treatments, find novel druggable targets, detect tumor development, recurrence, or progression with more efficient liquid biopsies, and to support clinical decision-making.
For testing the combinatorial approach of multi–omics in NMIBC, biosamples (urine, blood, and BC tissue) should be collected prospectively from all patients who are planning to undergo TURBT, as some of the –omics studies mentioned here can only be performed on FF biosamples. According to predefined standard operating procedures for each –omics study, biosamples should be collected and stored immediately at -80°C till analyses are performed. Multi–omics data have value only when combined with long-term follow-up data, which should be collected simultaneously from the same patient cohort. Furthermore, longitudinal molecular profiling of cancer tissue, urine, or blood during patient follow-up will reveal the disease’s evolution when there is a recurrence or progression. At this point, accurately identifying novel biomarkers showing the presence of recurrence and/or progression can potentially decrease the number of cystoscopies and/or imaging performed during the follow-up.
It is obvious that an enormous set of data points will be generated with the combination of the abovementioned multi–omics approach. Here, researchers face another big challenge: Previous approaches, such as the Pearson/Spearman correlation and the Kaplan-Meier method, could only perform pairwise data integration and were insufficient to process multi-layered big data. Recent advancements in mathematical methods, such as matrix deconvolution, network approaches, and machine learning, have significantly enhanced multi–omics data integration. Bioinformaticians have developed new, specialized bioinformatic pipelines necessary for collecting, processing, and manipulating –omics data to integrate their associations with clinico-pathological features. Key steps for data collection, preparation, representation, and clinical use in a multi–omics approach can be summarized as follows (98):
I. Data collection: Raw data, consistent in experimental conditions and data format, are collected from different –omics platforms and then converted into quantitative data.
II. Data cleaning and quality assessment: Missing values and outliers are identified, and data quality control metrics are used to ensure data reliability before downstream analyses.
III. Normalization: Data normalization methods are used for different –omics layers to mitigate biases and to ensure comparability and compatibility.
IV. Feature selection: Genomic and epigenetic alterations, differential gene expression, and other proteomic/metabolomic/lipidomic abnormalities are identified, and relevant features are selected based on biological and/or statistical significance. Filter methods (e.g., correlation analysis), wrapper methods (e.g., recursive feature elimination), and embedded methods (e.g., LASSO regression) are commonly used feature selection approaches. Dimensionality reduction methods such as principal component analysis (PCA), t-distributed stochastic neighbor embedding (t-SNE), uniform manifold approximation and projection (UMAP) are used to mitigate computational complexity and to facilitate appropriateness of data input for different analysis tools.
V. Data integration: Frequently used computational methods and their available tools (in brackets) include PCA [Scikit-learn], canonical component analysis (CCA) [MixOmics], independent component analysis (ICA) [FastICA], non-negative matrix factorization (NMF) [NIMFA], Tensor factorization [TensorFlow], multiple kernel learning (MKL) [MKLpy], regularized regression models [GLMNET], ensemble methods [DIABLO], network-based integration [OmicsNet], and deep learning models [Keras, TensorFlow].
VI. Annotation: The integrated data are annotated with relevant biological and functional information such as gene ontology terms, gene regulatory network, metabolic pathways, and signaling pathways. There are various publicly available pathway databases for different purposes, such as KEGG (Kyoto Encyclopedia of Genes and Genomes), WikiPathways, Reactome, PANTHER (Protein ANalysis THrough Evolutionary Relationships), TRANSFAC (TRANScription FACtor database), Pathway commons, etc.
VII. Data fusion: The integrated data are fused into a cohesive representation so that the information from different –omics layers is combined.
VIII. Clustering & subtyping: Unsupervised or supervised clustering techniques are employed to identify clusters or subtypes within the integrated data, which in turn provide insights into tumor heterogeneity.
IX. Machine learning modeling: Unsupervised (k-means clustering, hierarchical clustering, Gaussian mixture models) or supervised (support vector machines, random forests, neural networks, logistic regression, decision trees) learning techniques are used to group samples based on similar –omics profiles and predict outcomes or uncover patterns within the multi–omics data.
X. Visualization: Several data visualization tools, such as scatterplots, heatmaps, network plots, circos plots, hexbins, are used to understand the variability and subpopulations within datasets. Interactive visualization tools enable researchers to explore and analyze multi–omics data interactively.
XI. Interpretation: Interpreting the findings in the context of biological knowledge, pathways, and functional relevance helps not only understand the biological significance of observed patterns or specific subpopulations but also find novel information and decipher biological heterogeneity.
XII. Validation: The validity, robustness, reproducibility, and generalizability of the findings from the integrated data should be ensured using rigorous validation methods, cross-validation techniques, and independent datasets.
Various multi–omics tools such as iCluster, PARADIGM (PAthway Recognition Algorithm using Data Integration on Genomic Models), MetScape 2, BCC (Bayesian Consensus Clustering), SNF (Similarity Network Fusion), LRAcluster (Low Rank Approximation based multi–omics data clustering), PaintOmics 3, iOmicsPASS, SALMON (Survival Analysis Learning with Multi-Omics Neural Networks), NEMO (NEighborhood based Multi-Omics clustering), MONET (Multi Omic clustering by Non-Exhaustive Types), PIntMF (Penalized Integrative Matrix Factorization), MergeOmics 2.0, OmicsAnalyst, Arena3D, NeDRex, OmicsNet 2.0, DriverDBv4, are currently being used to integrate multi–omics data (99–102). However, these tools rely on different mathematical theories and computational approaches from each other and can support different data types. Therefore, to reach the defined goal with the multi–omics data, researchers should select appropriate multi–omics tool(s) according to their data type. Moreover, they are not very user-friendly and require advanced skills and experience in R, Python, or MATLAB. As the data are different from bulk multi–omics data, specific computational methods have been developed for the integration of data originating from single-cell multi–omics platforms such as MOFA+ (Multi-Omics Factor Analysis), scAI (single-cell Aggregation and Inference), scMVAE (single-cell Multimodal Variational Autoencoder), DCCA (Deep Cross-omics Cycle Attention), citeFUSE, and Seurat v4 (103, 104). With the recent developments in artificial intelligence (AI), various AI-based computational tools for multi–omics data integration have been developed for different purposes such as molecular subtyping, prediction of drug response, survival prediction, patient clustering (e.g., OmiEmbed, MetaCancer, DeepDRK, PathME, DeFusion, AKLIMATE, PRODeepSyn, etc.) (105). However, there is still ample room for further studies to develop newer computational methods for better, more proper, and robust multi–omics data integration, enabling systematic assessment of multi-layered findings.
When the multi–omics pipelines are used for NMIBC, the integrated multi-layered data would allow to identify significant associations of genome, epigenome, transcriptome, proteome, lipidome, metabolome, and immunohistopathological profiles to improve stratification of intermediate- and (very-)high-risk patients, and to develop classifiers for predicting disease outcomes and response to treatment (e.g., discriminating high-risk tumor profiles that have a higher potential to progress to MIBC and not to respond to intravesical BCG treatment) (Figure 4). The clinical performance of these classifiers should be tested (specificity, selectivity) and compared to the currently used criteria (EAU risk groups and risk stratification according to Gontero et al.) (12, 106). The analytical assay should be validated regarding repeatability, intermediate precision, and reproducibility.
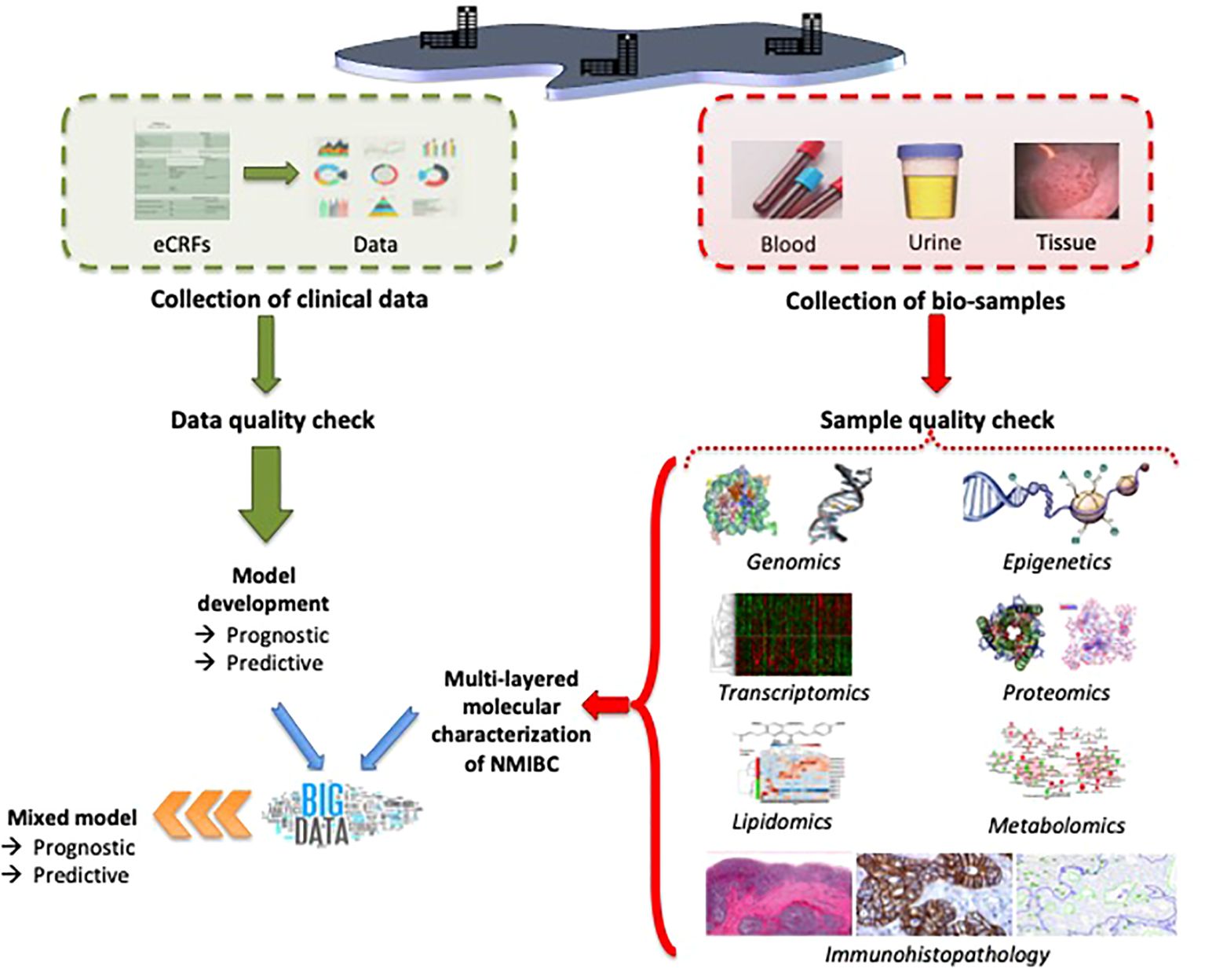
Figure 4. Possible setup of the experimental method for the characterization of intermediate- and high-risk NMIBC by using multi–omics.
We know that testing this hypothesis in the abovementioned setting will have some limitations. As the number of parameters that will come out from each –omics is not precise in the beginning, it may not be easy to determine the number of patients required for the training and validation cohorts. This would affect the power analysis and create a risk of ‘over-fitting’; however, this risk can be eliminated using the Bonferroni correction and more sophisticated computational methods.
4 Consequences of the hypothesis and discussion
Intensive work is currently being done in the field of BC markers with the goal of characterizing BC earlier, both at the initial diagnosis and at recurrence and/or progression. Although various –omics biomarkers have been identified for disease recurrence and progression up to now, the study of BC biomarkers is still in its developmental state.
The current major challenge and unmet need are twofold. First, there is a need for real-life contemporary data on NMIBC patients, which can be provided by setting up a multicentric organization that merges the results of already existing datasets. The second unmet need is the development of a more accurate scoring system, which takes into account the treatment they received after TURBT, to enable better stratification of the intermediate- and (very-)high-risk NMIBC patients for treatment selection and further follow-up scheduling, also based on a better prediction of response to treatment.
With this hypothesis, the aim is to define the tumor at the molecular level using high-resolution multi-layered –omics profiling and to use the molecular and clinical data to guide therapeutic intervention at a personalized level for NMIBC. A comprehensive depiction of the mechanism(s) of non-responsiveness to intravesical BCG treatment of NMIBC will reveal unique molecular pathways that can further guide drug development in the future by providing novel therapeutic targets (Figure 5).
A rapidly increasing number of research has been recently published in which disease heterogeneity, patient stratification, predictive and/or prognostic biomarkers, and cancer drug response were targeted to be improved using the bi-/tri-/multi–omics approach. Some of these studies with important molecular or clinical implications are summarized here (Figure 6). Among these critical researches, the most important can be emphasized as the tri–omics (genomics, transcriptomics, proteomics) approach improving the existing UROMOL2016 subclassification of NMIBC by identifying four different prognostic molecular subtypes (class 1, 2a, 2b, and 3 in UROMOL2021) (32). Anurag et al. showed that CIS samples had a 46-gene expression signature in which the druggable targets MTOR, TYK2, AXIN1, CTP1B, GAK, and PIEZO1 were selectively upregulated while BRD2 and NDUFB2 were selectively downregulated (107). With stage-stratified multi–omics profiling of NMIBC (Ta vs. T1), unsupervised clustering of copy number data revealed four clusters (CN1-CN4) within all tumor samples. Furthermore, Hurst et al. showed that there was sufficient molecular heterogeneity in both stages and, therefore, proposed to divide the Ta and T1 stages into three and four expression groups (TaE1-TaE3 vs. T1E1-T1E4), respectively, which provided prognostic information (108). Strandgaard et al. observed that post-BCG CD8 T-cell exhaustion was associated with post-BCG high-grade (HG) recurrence. They found that pre-BCG tumors of patients with HG recurrence had high expression of genes related to cell division and immune function, and the post-BCG urine of these patients had higher concentrations of immunoinhibitory proteins (CD70, PD1, CD5). A high pre-BCG exhaustion score, calculated based on the mean expression of five immunoinhibitory processes-related genes (PDCD1, CTLA4, LAG3, HAVCR2, and KLGR1), was associated with worse post-BCG recurrence-free survival (109). Using multiplatform mutational, proteomic, and metabolomic spatial mapping on a whole-organ scale, Czerniak et al. identified the molecular evolution of BC from mucosal field effects, which might span nearly 30 years and can be divided into two phases: The dormant phase was characterized by the gradual development of α mutations. The progressive phase lasted approximately five years and was signified by the β mutations, while the γ mutations developed during the last 2-3 years of disease progression to MIBC (110). By employing mutation, CNA, methylation, mRNA, and lncRNA profiling, Lu et al. refined the consensus classification of MIBC (30) and identified four robust integrative consensus subtypes (iCS1-iCS4) which had distinctive molecular patterns and were associated with stratified prognosis, different tumor immune microenvironment, and distinct sensitivity to immune checkpoint inhibitor therapy (111). Another multi–omics approach (mutation, methylation, mRNA, miRNA, and lncRNA) integrated with machine learning revealed three cancer subtypes (CS1-CS3) in MIBC that were related to prognosis and identified 12 hub genes that constituted a consensus machine learning-driven signature (CMLS). The low-CMLS group exhibited a more favorable prognosis and responded better to immunotherapy, while the high-CMLS group had a poor prognosis and a lower likelihood of benefitting from immunotherapy (112). In a recent multi–omics study, linoleic acid metabolism was found to be associated with variations in trained immunity induced by distinct BCG strains (113). With proteogenomic characterization, Groeneveld et al. demonstrated five unsupervised proteomic groups (uPG_A-uPG_E) in NMIBC and MIBC. They also identified the enrichment of proteins involved in tumor necrosis factor-related apoptosis-inducing ligand (TRAIL)-mediated apoptosis in FGFR3-mutated tumors, which could not be captured through transcriptomics (114).
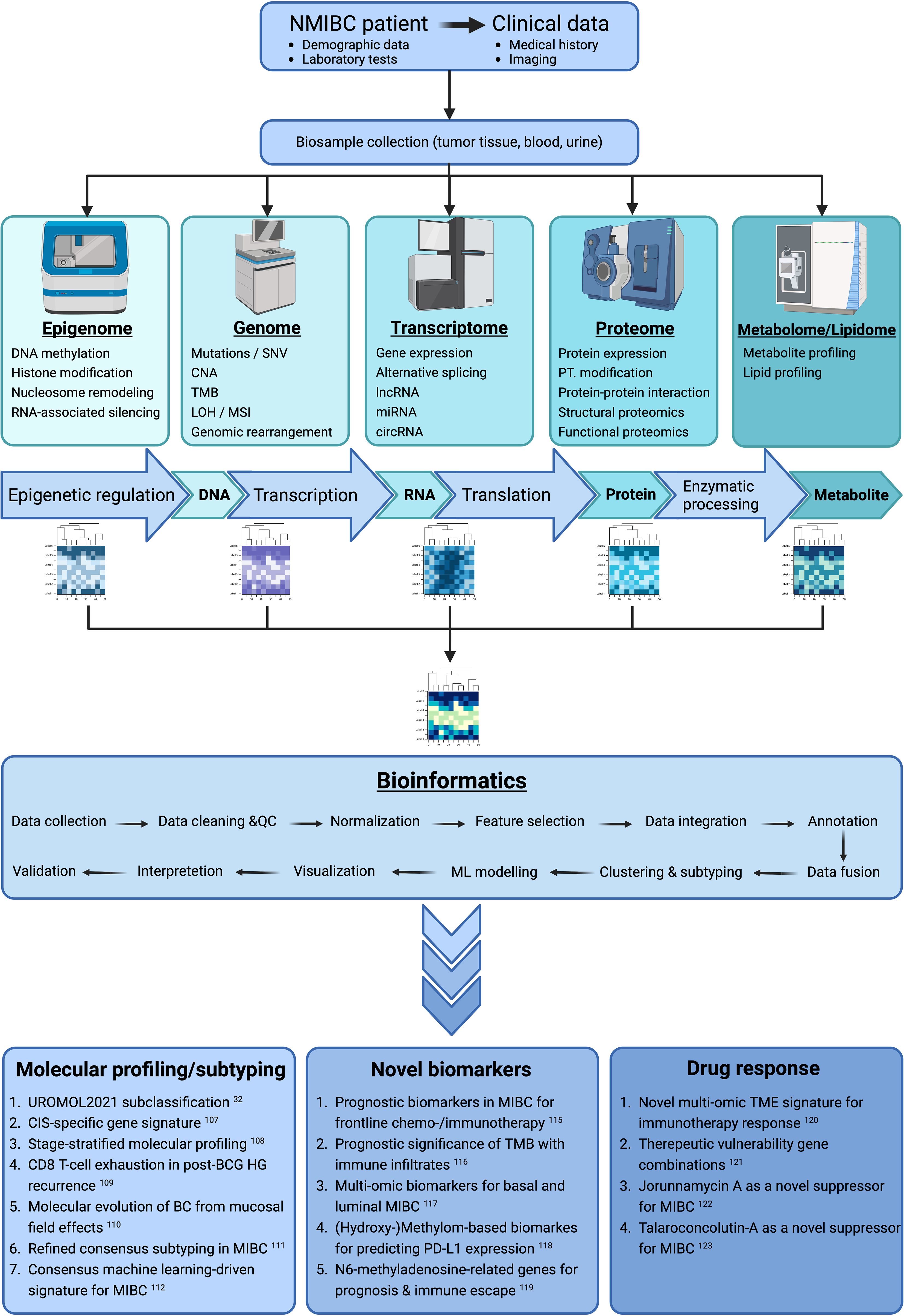
Figure 6. Simplified schema for multi–omics integration strategies in BC with examples of recently published papers for the use of findings (Created with BioRender.com). BC, Bladder cancer; BCG, Bacillus Calmette-Guérin; circRNA, Circular RNA; CIS, Carcinoma in situ; CNA, copy number alteration; HG, High grade; LOH, Loss of heterozygosity; lncRNA, Long non-coding RNA; MIBC, Muscle-invasive bladder cancer; miRNA, Micro RNA; ML, Machine learning; MSI, Microsatellite instability; NMIBC, Non-muscle-invasive bladder cancer; PD-L1, Programmed death ligand 1; PT, Posttranslational; QC, Quality control; SNV, Single nucleotide variation; TMB, Tumor mutational burden; TME, Tumor microenvironment.
Further multi–omics studies depicted distinct predictive or prognostic biomarkers for prognosis, programmed death ligand 1 (PD-L1) expression, response to chemotherapy and immunotherapy, immune escape of BC, and immune infiltrates (115–119). A bi–omics study revealed that a TME score (low vs. high) can predict the prognosis and the response to immunotherapy (120). Another study defined therapeutic vulnerability gene combinations and prognostic risk of BC by integrating multi–omics and clinical data (121). In two other studies, jorunnamycin A and talaroconvolutin-A were found to suppress MIBC via targeting fatty acid synthase (FASN) and topoisomerase 1 (TOP1), and cell cycle and ferroptosis, respectively, which could be a potential candidate for treating BC (122, 123). These existing results can be interpreted as proof that multi–omics will provide us a better understanding of BC.
The following impacts can be expected in the management of NMIBC with the success of a multi–omics approach:
1. Improvement of the disease outcome of NMIBC by tailoring available treatment options according to the tumor’s molecular profile and predicted treatment response. As such, the proposed approach has a significant direct impact on patient-relevant issues: survival and quality of life.
A. Clinical risk models currently used for intermediate- and high-risk NMIBC patients are based on clinical parameters and lack accuracy. Moreover, they only provide an estimation of the risk of a tumor to recur or progress and offer physicians predictions that are not accurate enough for precision medicine. Therefore, urologists/multidisciplinary teams treat their patients based on ‘subjective’ decisions (interpretation of a certain percentage risk). Incorporating biomarkers into the risk-scoring systems may provide accurate and individual predictions. Thus, through better risk stratification, physicians will avoid over- and under-treatment of NMIBC patients and change the diagnostic and therapeutic decision tree. By improved prediction of the risk of progressing to muscle-invasive disease at the time of initial TURBT, patients who are not likely to respond to conservative treatments (such as intravesical BCG) may be directed to early radical cystectomy in a timely manner and may be offered an increased probability of cure. Better risk prediction may also improve treatment guidelines and lead to more ‘objective’ treatment decisions.
B. Accurate prediction of the risk of progressing to MIBC would also allow the identification of a subgroup of patients in whom conservative treatment modalities may be considered safe. These patients represent the majority of the intermediate- and (very-)high-risk NMIBC group, and accurate risk prediction would allow them to avoid aggressive and invasive treatments (radical cystectomy, chemotherapy, radiotherapy). As a result, their quality of life would be retained. Moreover, for patients in whom the risk of developing future MIBC would be estimated to be elevated by the use of biomarkers, cancer-specific and overall survival may increase as they could be offered early curative treatment.
2. The interactions between putative causative factors, individual features of recurrence and progression, and the spectrum of molecular alterations underlying disease heterogeneity in NMIBC (e.g., response to BCG treatment) will be revealed. In this way, the data would enable the identification of novel therapeutic targets in NMIBC and guiding caregivers in directing the patients who are not responding to gold-standard treatments.
3. If patients suitable for conservative treatment could be determined more accurately and objectively by using biomarkers, the cost of treatment may decrease with a patient-tailored treatment and follow-up scheme. Besides, better risk stratification of NMIBC patients would significantly increase the quality of BC treatment.
The risk stratification developed based on this hypothesis will require external validation. This will prove its scientific accuracy and potential for use in clinical routine practice. In addition to this, it will need valorization in a real-life clinical setting through evaluation of its added value to the quality of treatment and modification of treatment and follow-up, which will impact the cost of disease management and the quality of life of the patients (Figure 5).
In conclusion, it is evident that there is ample room for further research to develop a better and more accurate stratification of intermediate- and (very-)high-risk NMIBC. Multi-layered –omics studies can provide the ‘missing’ information necessary for increasing the quality of treatment and the quality of life of these patients, as well as for determining novel therapeutic targets.
Data availability statement
The original contributions presented in the study are included in the article/supplementary material, further inquiries can be directed to the corresponding author/s.
Author contributions
MA: Conceptualization, Data curation, Project administration, Writing – original draft, Writing – review & editing. TJ: Data curation, Writing – review & editing. TM: Writing – review & editing. TG: Writing – review & editing. SJ: Conceptualization, Supervision, Writing – review & editing. FA: Conceptualization, Supervision, Writing – review & editing.
Funding
The author(s) declare that no financial support was received for the research, authorship, and/or publication of this article.
Acknowledgments
This manuscript is a part of the doctoral thesis of Dr. Murat Akand. Steven Joniau is a senior clinical researcher at the Research Foundation of Flanders (FWO).
Conflict of interest
The authors declare that the research was conducted in the absence of any commercial or financial relationships that could be construed as a potential conflict of interest.
Publisher’s note
All claims expressed in this article are solely those of the authors and do not necessarily represent those of their affiliated organizations, or those of the publisher, the editors and the reviewers. Any product that may be evaluated in this article, or claim that may be made by its manufacturer, is not guaranteed or endorsed by the publisher.
Glossary
4E-BP1: Eukaryotic translation initiation factor 4E (eIF4E)-binding protein 1
ACOT11: Acyl-CoA thioesterase 11
ADGRF1: Adhesion G protein-coupled receptor F1
AK1: Adenylate kinase 1
AKT: RAC(Rho family)-alpha serine/threonine-protein kinase (also known as Protein kinase B)
ALDH1A3: Aldehyde dehydrogenase 1 family member A3
ANXA1: Annexin A1
APC: Adenomatous polyposis coli
AREG: Amphiregulin
ARID1A: AT-rich interactive domain-containing protein 1A
ATM: Ataxia-telangiectasia mutated
ATR: Ataxia-telangiectasia and Rad3-related protein
BAD: BCL2-associated agonist of cell death
BC: Bladder cancer
BCC: Bayesian consensus clustering
BCG: Bacillus Calmette-Guérin
BCL2: B-cell lymphoma 2
BCL2L1: BCL-2-like protein 1
BLVRB: Biliverdin reductase B
BRCA1: Breast cancer type 1 susceptibility protein
BRCA2: Breast cancer type 2 susceptibility protein
BRIP1: BRCA1-interacting protein
C8A: Complement C8 alpha chain
CA3: Carbonic anhydrase 3
CAPG: Macrophage-capping protein
CCA: Canonical component analysis
CCND1: Cyclin D
CD2AP: CD2-associated protein
CDH1: Cadherin-1 (E-cadherin= epithelial cadherin)
CDK4/6: Cyclin-dependent kinase 4/6
CDK12: Cyclin-dependent kinase 12
CDKN1A: Cyclin-dependent kinase inhibitor 1A
CDKN2A: Cyclin-dependent kinase inhibitor 2A
CDKN2B: Cyclin-dependent kinase 4 inhibitor B
circRNA: Circular RNA
CIS: Carcinoma in situ; CMLS, Consensus machine learning-driven signature
CKIα: Cyclin-dependent kinase inhibitor α
CNA: Copy number alteration
Co-A: Co-activator
CTLA4: Cytotoxic T-lymphocyte associated protein 4
CTSG: Cathepsin G
CUETO: Club Urologico Español de Tratamiento Oncologico
DAPK: Death-associated protein kinase
DAPK1: Death-associated protein kinase 1
DCCA: Deep cross-omics cycle attention
Deptor: DEP domain-containing mTOR-interacting protein
DDR: DNA damage repair
DBiT-seq: Deterministic barcoding in tissue sequencing
DLL: Delta-like ligand
E2F3: Transcription factor E2F3
EAU: European Association of Urology
EGF: Epidermal growth factor
EGFR: Epidermal growth factor receptor
eIF4B/E: Eukaryotic translation initiation factor 4A/B/E
ELK1: ETS-like 1
EMT: Epithelial-to-mesenchymal transition
EOMES: Eomesodermin
EORTC: European Organization for Research and Treatment of Cancer
EP300: E1A-associated protein p300
ERBB2: Erythroblastosis oncogene B2 (human epidermal growth factor 2=HER2)
ERK1/2: Extracellular signal-regulated kinase ½
ESM1: Endothelial cell-specific molecule 1
FASN: Fatty acid synthase
FAT4: FAT tumor suppressor homolog 4
FF: Fresh frozen
FFPE: Formalin-fixed paraffin-embedded
FGF: Fibroblast growth factor
FGFBP1: Fibroblast growth factor-binding protein 1
FGFR: Fibroblast growth factor receptor
FGFR3: Fibroblast growth factor receptor 3
FHIT: Fragile histidine triad protein (Bis[5’-adenosyl]-triphosphatase)
FOS: Finkel–Biskis–Jinkins murine osteogenic sarcoma virus
FRMD: FERM domain-containing protein
GDF15: Growth differentiation factor 15
GLI1/2/3: Glioma-associated oncogene 1/2/3
GLIFL: Full-length glioma-associated oncogene
GMPS: Guanine monophosphate synthase
GSK3: Glycogen synthase kinase 3
GSTM1: Glutathione S-transferase mu 1
GSTP1: Glutathione S-transferase pi 1
GUCG: Genito-Urinary Cancer Group
HAVCR2: Hepatitis A virus cellular receptor 2
HIC1: Hypermethylated in cancer 1 (ZBTB transcriptional repressor 1)
HG: High grade
HOXA9: Homeobox A9
HPGD: Hydroxyprostaglandin dehydrogenase 15
HSPB1: Heat shock protein family B (small) member 1
IARC: International Agency for Research on Cancer
ICA: Independent component analysis
iCS: Integrative consensus subtypes
IKK: Inhibitor of nuclear factor κ
Indel: Insertion-deletion
ISL1: ISL LIM homeobox 1 (Islet1)
IRPS: Immune-related prognostic signature
JAG: Jagged
KDM6A: Lysine demethylase 6A
KEGG: Kyoto Encyclopedia of Genes and Genomes
KIBRA: Kidney and brain expressed protein
KIF7: Kinesin family member 7
KLRG1: Killer cell lectin-like receptor subfamily G member 1
KMT2C: Lysine methyltransferase 2C
KMT2D: Lysine methyltransferase 2D
KISS1: KiSS-1 metastasis suppressor (kisspeptin)
LAG3: Lymphocyte-activating 3
LATS1/2: Large tumor suppressor kinase 1
LC: Liquid chromatography
LEF: Lymphoid enhancer-binding factor
lncRNA: Long non-coding RNA
LOH: Loss of heterozygosity
LRAcluster: Low rank approximation based multi-omics data clustering
LRP5/6: Low-density lipoprotein receptor-related protein 5/6
MAML: Mastermind-like protein
MAPK: Mitogen-activated protein kinase
Mb: Megabase
MDM2: Mouse double minute 2 homolog
MEK1/2: Mitogen-activated protein kinase 1/2
MER: MER proto-oncogene
MGMT: O-6-methylguanine-DNA methyltransferase
MIBC: Muscle-invasive bladder cancer
miRNA: Micro RNA
MKL: Multiple kernel learning
MLH1: MutL homolog 1
mLST8: mammalian lethal with SEC13 protein 8
MOB1: Mps one binder 1
MOFA+: Multi-omics factor analysis
MONET: Multi-omic clustering by non-exhaustive types
mRNA: Messenger RNA
MS: Mass spectrometry
MSH2: MutS homolog 2
MSH6: MutS homolog 6
MSI: Microsatellite instability
mSIN1: mammalian stress-activated protein kinase interacting protein 1
MSK1: Mitogen- and stress-activated kinase 1
MST1/2: Macrophage-stimulating 1/2
mTOR: Mammalian target of rapamycin
mTORC1/2: Mammalian target of rapamycin complex 1/2
MYB: Myeloblastosis
MYC: Myelocytomatosis
NEMO: Neighborhood-based multi-omics clustering
NFκB: Nuclear factor kappa-light-chain-enhancer of activated B cells
NFKB1: Nuclear factor kappa B subunit 1
NICD: NOTCH intracellular domain
NMIBC: Non-muscle-invasive bladder cancer
NMF: Non-negative matrix factorization
NOTCH: Neurogenic locus notch homolog protein
NPS: NMIBC proteomic subtype
ONECUT2: One cut homeobox 2
PANTHER: Protein analysis through evolutionary relationships
PARADIGM: Pathway recognition algorithm using data integration on genomic models
PCA: Principal component analysis
PCDH8: Protocadherin 8
PCDH17: Protocadherin 17
PCDHGA12: Protocadherin gamma subfamily A, 12
PDCD1: Programmed cell death 1
PDK1: Protein 3-phosphoinositide-dependent protein kinase-1
PD-L1: Programmed death ligand 1
PGAM1: Phosphoglycerate mutase 1
PI3K: Phosphoinositide 3-kinase
PIK3CA: Phosphatidylinositol-4,5-biphosphanate 3-kinase, catalytic subunit alpha
PIP3: Phosphatidylinositol (3,4,5)-triphosphate
PLCγ: Phospholipase C gamma
PIntMF: Penalized integrative matrix factorization
PMF1: Polyamide modulated factor 1
POLD1: DNA polymerase delta 1, catalytic subunit
POLE: DNA polymerase epsilon, catalytic subunit
POLQ: DNA polymerase theta
POU4F2: POU class 4 homeobox 2
PRAC1: PRAC1 small nuclear protein
PRAS40: Proline-rich AKT1 substrate
PRDX1: Peroxiredoxin 1
PRDX2: Peroxiredoxin 2
PRDX6: Peroxiredoxin 6
Protor: Protein observed with Rictor
PSME1: Proteasome activator subunit 1
PTCH1: Patched 1
PTEN: Phosphatase and tensin homolog
PTGDR: Prostaglandin D2 receptor
PVRL4: Poliovirus-receptor-like 4 (Nectin 4=Nectin cell adhesion molecule 4)
RAF: Rapidly accelerated fibrosarcoma
Raptor: Regulatory-associated protein of mTOR
RAS: Rat sarcoma
RASSF1: Ras-associated domain family member 1
RASSF1A: Ras association domain-containing protein 1A
RB: Retinoblastoma protein
RB1: RB transcriptional corepressor 1
Rictor: Rapamycin-insensitive companion of mTOR
RPB-J: Recombination signal binding protein for immunoglobulin kappa J region
RUNX3: RUNX family transcription factor 3
S6K1: Ribosomal protein S6 kinase beta-1
SALMON: Survival analysis learning with multi-omics neural networks
SAV1: Protein Salvador homolog 1
scAI: Single-cell aggregation and inference scMVAE, Single-cell multimodal variational autoencoder
SFRP5: Secreted frizzled-related protein 5
SHH: Sonic hedgehog
SMAD1-4: Mothers against decapentaplegic homolog 1-4
SMO: Smoothened
SNF: Similarity network fusion
SNRPD2: Small nuclear ribonucleoprotein D2 polypeptide
SNV: Single nucleotide variation
SOCS1: Suppressor of cytokine signaling 1
SPR: Sepiapterin reductase
SUFU: Suppressor of fused kinase
SUMO: Small ubiquitin-like modifier
SWI/SNF: SWItch/Sucrose Non-Fermentable
STAT3: Signal transducer and activator of transcription 3
TAP1: Transporter 1, ATP binding cassette subfamily B member
TAZ: Transcriptional coactivator with PDZ-binding motif
TBX3: T-box transcription factor 3
TBX4: T-box transcription factor 4
TCF: T-cell factor
TCGA: The Cancer Genome Atlas
TEAD1-4: Telomere ends-associated domain family member 1-4
TGF-α: Transforming growth factor-alpha
TGFB1: Transforming growth factor beta 1
TIMP3: TIMP metallopeptidase inhibitor 3
TLR: Toll-like receptor
TMB: Tumor mutational burden
TME: Tumor microenvironment
TOP1: Topoisomerase 1
TP53: Tumor protein 53
TRAIL: Tumor necrosis factor-related apoptosis-inducing ligand
TRANSFAC: Transcription Factor database
TRK: Tyrosine receptor kinase
TSC1/2: Tuberous sclerosis 1/2
t-SNE: T-distributed stochastic neighbor embedding
TURBT: Transurethral resection of the bladder tumor
TWIST1: Twist-related protein 1
UC: Urothelial carcinoma
UMAP: Uniform manifold approximation and projection
UMP/CMPK: Cytidine/uridine monophosphate kinase 1
uPG: Unsupervised proteomic group
VEGF: Vascular endothelial factor
VEGFR: Vascular endothelial factor receptor
VHL: Von Hippel-Lindau tumor suppressor
WFDC2: WAP four-disulfide core domain protein 2 (human epididymis protein 4)
WHO: World Health Organization
WIF1: Wnt inhibitory factor 1
Wnt: Wingless-Int 1
YAP: Yes-associated protein
ZNF154: Zinc finger protein 154
References
1. Bray F, Laversanne M, Sung H, Ferlay J, Siegel RL, Soerjomataram I, et al. Global cancer statistics 2022: GLOBOCAN estimates of incidence and mortality worldwide for 36 cancers in 185 countries. CA Cancer J Clin. (2024) 74:229–63. doi: 10.3322/caac.21834
2. Cancer today. Available online at: http://gco.iarc.who.int/today/en (Accessed July 2, 2024).
3. Cancer tomorrow. Available online at: http://gco.iarc.who.int/tomorrow/en (Accessed July 2, 2024).
4. Donsky H, Coyle S, Scosyrev E, Messing EM. Sex differences in incidence and mortality of bladder and kidney cancers: national estimates from 49 countries. Urol Oncol. (2014) 32:40.e23–31. doi: 10.1016/j.urolonc.2013.04.010
5. Saginala K, Barsouk A, Aluru JS, Rawla P, Padala SA, Barsouk A. Epidemiology of bladder cancer. Cancers. (2020) 8:15. doi: 10.3390/medsci8010015
6. Jubber I, Ong S, Bukavina L, Black PC, Compérat E, Kamat AM, et al. Epidemiology of bladder cancer in 2023: a systematic review of risk factors. Eur Urol. (2023) 84:176–90. doi: 10.1016/j.eururo.2023.03.029
7. International Agency for Research on Cancer. List of classifications by cancer sites with sufficient or limited evidence in humans. IARC monographs volumes 1-132 . Available online at: http://monographs.iarc.who.int/wp-content/uploads/2019/07/Classifications_by_cancer_site.pdf (Accessed July 2, 2024).
8. Lobo N, Afferi L, Moschini M, Mostafid H, Porten H, Psutka SP, et al. Epidemiology, screening, and prevention of bladder cancer. Eur Urol Oncol. (2022) 5:628–39. doi: 10.1016/j.euo.2022.10.003
9. Babjuk M, Burger M, Capoun O, Cohen D, Comperat EM, Dominguez Escrig JL, et al. European Association of Urology guidelines on non-muscle-invasive bladder cancer (Ta, T1, and carcinoma in situ). Eur Urol. (2022) 81:75–94. doi: 10.1016/j.eururo.2021.08.010
10. Mariappan P, Smith G. A surveillance schedule for G1Ta bladder cancer allowing efficient use of check cystoscopy and safe discharge at 5yr based on 25-yr prospective database. J Urol. (2005) 173:1108–11. doi: 10.1097/01.ju.0000149163.08521.69
11. Ploeg M, Aben KK, Kiemeney LA. The present and future burden of urinary bladder cancer in the world. World J Urol. (2009) 27:289–93. doi: 10.1007/s00345-009-0383-3
12. Sylvester RJ, van der Meijden AP, Oosterlinck W, Witjes JA, Bouffioux C, Denis L, et al. Predicting recurrence and progression in individual patients with stage Ta, T1 bladder cancer using EORTC risk tables: a combined analysis of 2596 patients from seven EORTC trials. Eur Urol. (2006) 49:466–75. doi: 10.1016/j.eururo.2005.12.031
13. Fernandez-Gomez J, Madero R, Solsona E, Unda M, Martinez-Pineiro L, Gonzalez M, et al. Predicting nonmuscle invasive bladder cancer recurrence and progression in patients treated with Bacillus Calmette-Guerin: the CUETO scoring model. J Urol. (2009) 182:2195–203. doi: 10.1016/j.juro.2009.07.016
14. Busato Júnior WF, Almeida GL, Ribas CA, Ribas Filho JM, De Cobelli O. EORTC risk model to predict progression in patients with non-muscle-invasive bladder cancer: is it safe to use in clinical practice? Clin Genitourin Cancer. (2015) 14:176–82. doi: 10.1016/j.clgc.2015.09.005
15. Xylinas E, Kent M, Kluth L, Pycha A, Comploj E, Svatek RS, et al. Accuracy of the EORTC risk tables and of the CUETO scoring model to predict outcomes in non-muscle-invasive urothelial carcinoma of the bladder. Br J Cancer. (2013) 109:1460–6. doi: 10.1038/bjc.2013.372
16. Zhao L, Lee VHF, Ng MK, Yan H, Bijlsma M. Molecular subtyping of cancer: current status and moving toward clinical applications. Brief Bioinform. (2019) 20:572–84. doi: 10.1093/bib/bby026
17. Czerniak B, Dinney C, McConkey D. Origins of bladder cancer. Annu Rev Pathol. (2016) 11:149–74. doi: 10.1146/annurev-pathol-012513-104703
18. Knowles MA, Hurst CD. Molecular biology of bladder cancer: new insights into pathogenesis and clinical diversity. Nat Rev Cancer. (2015) 15:25–41. doi: 10.1038/nrc3817
19. Zhu S, Yu W, Yang X, Wu C, Cheng F. Traditional classification and novel subtyping systems for bladder cancer. Front Oncol. (2020) 10:102. doi: 10.3389/fonc.2020.00102
20. Netto GJ. Molecular biomarkers in urothelial carcinoma of the bladder: are we there yet? Nat Rev Urol. (2012) 9:41–51. doi: 10.1038/nrurol.2011.193
21. Hurst C, Rosenberg J, Knowles M. SnapShot: bladder cancer. Cancer Cell. (2018) 34:350–1. doi: 10.1016/j.ccell.2018.07.013
22. Sjödahl G, Lauss M, Lövgren K, Chebil G, Gudjonsson S, Veerla S, et al. A molecular taxonomy for urothelial carcinoma. Clin Cancer Res. (2012) 18:3377–86. doi: 10.1158/1078-0432.CCR-12-0077-T
23. Biton A, Bernard-Pierrot I, Lou Y, Krucker C, Chapeaublanc E, Rubio-Pérez C, et al. Independent component analysis uncovers the landscape of the bladder tumor transcriptome and reveals insights into luminal and basal types. Cell Rep. (2014) 9:1235–45. doi: 10.1016/j.celrep.2014.10.035
24. Damrauer JS, Hoadley KA, Chism DD, Fan C, Tiganelli CJ, Wobker SE, et al. Intrinsic subtypes of high-grade bladder cancer reflect the hallmarks of breast cancer biology. PNAS. (2014) 111:3110–5. doi: 10.1073/pnas.1318376111
25. Choi W, Porten S, Kim S, Willis D, Plimack ER, Hoffman-Censits J, et al. Identification of distinct basal and luminal subtypes of muscles-invasive bladder cancer with different sensitivities to frontline chemotherapy. Cancer Cell. (2014) 25:152–65. doi: 10.1016/j.ccr.2014.01.009
26. Cancer Genome Atlas Research Network. Comprehensive molecular characterization of urothelial bladder carcinoma. Nature. (2014) 507:315–22. doi: 10.1038/nature12965
27. Perou CM, Sorlie T, Eisen MB, van de Rijn M, Jeffrey SS, Rees CA, et al. Molecular portraits of human breast tumors. Nature. (2000) 406:747–52. doi: 10.1038/35021093
28. Robertson AG, Kim J, Al-Ahmadie H, Bellmunt J, Guo G, Cherniack AD, et al. Comprehensive molecular characterization of muscle-invasive bladder cancer. Cell. (2017) 171:540–556.e25. doi: 10.1016/j.cell.2017.09.007
29. Sjödahl G, Erikson P, Liedberg F, Höglund M. Molecular classification of urothelial carcinoma: global mRNA classification versus tumor-cell phenotype classification. J Pathol. (2017) 242:113–25. doi: 10.1002/path.4886
30. Kamaoun A, de Reynies A, Allory Y, Sjödahl G, Robertson AG, Seiler R, et al. A consensus molecular classification of muscle-invasive bladder cancer. Eur Urol. (2020) 77:420–33. doi: 10.1016/j.eururo.2019.09.006
31. Hedegaard J, Lamy P, Nordentoft I, Algaba F, Hoyer S, Ulhoi BP, et al. Comprehensive transcriptional analysis of early-stage urothelial carcinoma. Cancer Cell. (2016) 30:27–42. doi: 10.1016/j.ccell.2016.05.004
32. Lindskrog SV, Prip F, Lamy P, Taber A, Groeneveld CS, Birkenkamp-Demtröder K, et al. An integrated multi-omics analysis identifies prognostic molecular subtypes of non-muscle-invasive bladder cancer. Nat Commun. (2021) 12:2301. doi: 10.1038/s41467-021-22465-w
33. Robertson AG, Groeneveld CS, Jordan B, Lin X, McLaughlin KA, Das A, et al. Identification of differential tumor subtypes of T1 bladder cancer. Eur Urol. (2020) 78:533–7. doi: 10.1016/j.eururo.2020.06.048
34. Sanchez-Vega F, Mina M, Armenia J, Chatila WK, Luna A, La KC, et al. Oncogenic signaling pathways in the cancer genome atlas. Cell. (2018) 173:321–7. doi: 10.1016/j.cell.2018.03.035
35. Lokeshwar SD, Lopez M, Sarcan S, Agulair K, Morera DS, Shaheen DM, et al. Molecular oncology of bladder cancer from inception to modern perspective. Cancers. (2022) 14:2578. doi: 10.3390/cancers14112578
36. Ferguson HR, Smith MP, Francavilla C. Fibroblast growth factor receptors (FRGRs) and noncanonical partners in cancer signaling. Cells. (2021) 10:1021. doi: 10.3390/cells10051201
37. Peng Y, Wang Y, Zhou C, Mei W, Zeng C. PI3K/AKT/mTOR pathway and its role in cancer therapeutics: are we making headway? Front Oncol. (2022) 12:819128. doi: 10.3389/fonc.2022.819128
38. Thomas J, Sonpavde G. Molecularly targeted therapy towards genetic alterations in advanced bladder cancer. Cancers. (2022) 14:1795. doi: 10.3390/cancers14071795
39. Kung CP, Weber JD. It’s getting complicated – a fresh look at p53-MDM2-ARF triangle in tumorigenesis and cancer therapy. Front Cell Dev Biol. (2022) 10:818744. doi: 10.3389/fcell.2022.818744
40. Peng M, Chu X, Peng Y, Li D, Zhang Z, Wang W, et al. Targeted therapies in bladder cancer: signaling pathways, applications, and challenges. MedComm (2020). (2023) 4:e455. doi: 10.1002/mco2.455
41. Wang H, Guo M, Wei H, Chen Y. Targeting p53 pathways: mechanisms, structures, and advances in therapy. Signal Transduct Target Ther. (2023) 8:92. doi: 10.1038/s41392-023-01347-1
42. Shi Q, Xue C, Zeng Y, Yuan X, Chu Q, Jiang S, et al. Notch signaling pathway in cancer: from mechanistic insights to targeted therapies. Signal Transduct Target Ther. (2024) 9:128. doi: 10.1038/s41392-024-01828-x
43. Berrino C, Omar A. Unravelling the mysteries of the sonic hedgehog pathway in cancer stem cells: activity, crosstalk and regulation. Curr Issues Mol Biol. (2024) 46:5397–419. doi: 10.3390/cimb46060323
44. Shah K, Kazi JU. Phosphorylation-dependent regulation of WNT/beta-catenin signaling. Front Oncol. (2022) 12:858782. doi: 10.3389/fonc.2022.858782
45. Cani M, Turco F, Butticè S, Vogl UM, Buttigliero C, Novello S, et al. How does environmental and occupational exposure contribute too carcinogenesis in genitourinary and lung cancers? Cancers. (2023) 15:2836. doi: 10.3390/cancers15102836
46. Wu Y, Shu J, Yuan Y, Zhou D. Tobacco smoke and bladder cancer: the current research status and the future challenges. Int J Clin Exp Med. (2019) 12:12633–9.
47. Zuniga KB, Graff RE, Feiger DB, Meng MV, Porten SP, Kenfield SA. Lifestyle and non-muscle invasive bladder cancer recurrence, progression, and mortality: available research and future directions. Bladder Cancer. (2020) 6:9–23. doi: 10.3233/BLC-190249
48. Slusarczyk A, Zapala P, Zapala L, Radziszewski P. The impact of smoking on recurrence and progression of non-muscle invasive bladder cancer: a systematic review and meta-analysis. J Cancer Res Clin Oncol. (2023) 149:2673–91. doi: 10.1007/s00432-022-04464-6
49. Hayashi T, Fujita K, Hayashi Y, Hatano K, Kawashima A, McConkey DJ, et al. Mutational landscape and environmental effects in bladder cancer. Int J Mol Sci. (2020) 21:6072. doi: 10.3390/ijms.21176072
50. Wu J, Gao F, Meng R, Li H, Mao Z, Xiao Y, et al. Single-cell and multi-omics analyses highlight cancer-associated fibroblasts-induced immune evasion and epithelial mesenchymal transition for smoking bladder cancer. Toxicology. (2024) 504:153782. doi: 10.1016/j.tox.2024.153782
51. Amara CS, Ambati CR, Vantaku V, Piyarathna DWB, Donepudi SR, Ravi SS, et al. Serum metabolic profiling identified a distinct metabolic signature in bladder cancer smokers: a key metabolic enzyme associated with patient survival. Cancer Epidemiol Biomarkers Prev. (2019) 28:770–81. doi: 10.1158/1055-9965.EPI-18-0936
52. Botteman MF, Pashos CL, Redaelli A, Laskin B, Hauser R. The health economics of bladder cancer: a comprehensive review of the published literature. Pharmacoeconomics. (2003) 21:1315–30. doi: 10.1007/BF03262330
53. Burger M, Catto JW, Dalbagni G, Grossman HB, Herr H, Karakiewicz P, et al. Epidemiology and risk factors of urothelial bladder cancer. Eur Urol. (2013) 63:234–41. doi: 10.1016/j.eururo.2012.07.033
54. Vlachostergios P, Faltas BM, Carlo MI, Nassar AH, Alaiwi SA, Sonpavde G. The emerging landscape of germline variants in urothelial carcinoma; implications for genetic testing. Cancer Treat Res Commun. (2020) 23:100165. doi: 10.1016/j.ctarc.2020.100165
55. Zhang X, Zhang Y. Bladder cancer and genetic mutations. Cell Biochem Biophys. (2015) 73:65–9. doi: 10.1007/s12013-015-0574-z
56. Pietzak EJ, Bagrodia A, Cha EK, Drill EN, Iyer G, Isharwal S, et al. Next-generation sequencing of nonmuscular invasive bladder cancer reveals potential biomarkers and rational therapeutic targets. Eur Urol. (2017) 72:952–9. doi: 10.1016/j.eururo.2017.05.032
57. Choi W, Ochoa A, McConkey DJ, Aine M, Hoglund M, Kim WY, et al. Genetic alterations in the molecular subtypes of bladder cancer: illustrations in the Cancer Genome Atlas dataset. Eur Urol. (2017) 72:354–65. doi: 10.1016/j.eururo.2017.03.010
58. Soave A, Kluwe L, Yu H, Rink M, Gild P, Vetterlein MW, et al. Copy number variations in primary tumor, serum and lymph node metastasis of bladder cancer patients treated with radical cystectomy. Sci Rep. (2020) 10:21562. doi: 10.1038/s41598-020-75869-x
59. Voutsadakis IA. Urothelial bladder carcinomas with high tumor mutational burden have a better prognosis and targetable molecular defects beyond immunotherapies. Curr Oncol. (2022) 29:1390–407. doi: 10.3390/curroncol29030117
60. Halbert B, Einstein DJ. Hot or not: tumor mutational burden (TMB) as a biomarker of immunotherapy response in genitourinary tumors. Urology. (2021) 147:119–26. doi: 10.1016/j.urology.2020.10.030
61. Chalmers ZR, Connelly CF, Fabrizio D, Gay L, Ali SM, Ennis R, et al. Analysis of 100,000 human cancer genomes reveals the landscape of tumor mutational burden. Genome Med. (2017) 9:34. doi: 10.1186/s13073-017-0424-2
62. Moon C, Gordon M, Moon D, Reynolds T. Microsatellite instability analysis (MSA) for bladder cancer: past history and future directions. Int J Mol Sci. (2021) 22:12864. doi: 10.3390/ijms.222312864
63. Sharma A, Reutter H, Ellinger J. DNA methylation and bladder cancer: where genotype does not predict phenotype. Curr Genomics. (2020) 21:34–6. doi: 10.2174/1389202921666200102163422
64. Gilyazova I, Enikeeva K, Rafikova G, Kagirova E, Sharifyanova Y, Asadullina D, et al. Epigenetic and immunological features of bladder cancer. Int J Mol Sci. (2023) 24:9854. doi: 10.3390/ijms24129854
65. Li Y, Sun L, Guo X, Mo N, Zhang J, Li C. Frontiers in bladder cancer genomic research. Front Oncol. (2021) 11:670729. doi: 10.3389/fonc.2021.670729
66. Torres-Bustamante MI, Vazquez-Urrutia JR, Solorzano-Ibarra F, Ortiz-Lazareno PC. The role of miRNAs to detect progression, stratify, and predict relevant clinical outcomes in bladder cancer. Int J Mol Sci. (2024) 25:2178. doi: 10.3390/ijms25042178
67. El-Mahdy HA, Elsakka EGE, El-Husseiny AA, Ismail A, Yehia AM, Abdelmaksoud NM, et al. miRNAs role in bladder cancer pathogenesis and targeted therapy: signaling pathways interplay – a review. Pathol Res Pract. (2023) 242:154316. doi: 10.1016/j.prp.2023.154316
68. Li HJ, Gong X, Li ZK, Qin W, He CX, Xing L, et al. Role of long non-coding RNAs on bladder cancer. Front Cell Dev Biol. (2021) 9:672679. doi: 10.3389/fcell.2021.672679
69. Mehmandar-Oskuie A, Jahankhani K, Rostamlou A, Arabi S, Razavi ZS, Mardi A. Molecular landscape of lncRNAs in bladder cancer: from drug resistance to novel lncRNA-based therapeutic strategies. BioMed Pharmacother. (2023) 165:115242. doi: 10.1016/j.biopha.2023.115242
70. Sun K, Wang D, Yang BB, Ma J. The emerging functions of circular RNAs in bladder cancer. Cancers. (2021) 13:4618. doi: 10.3390/cancers13184618
71. Ghafouri-Fard S, Najafi S, Hussen BM, Basiri A, Hidayat HJ, Taheri M, et al. The role of circular RNAs in the carcinogenesis of bladder cancer. Front Oncol. (2022) 12:801841. doi: 10.3389/fonc.2022.801841
72. Aebersold P, Mann M. Mass-spectrometric exploration of proteome structure and function. Nature. (2016) 537:347–55. doi: 10.1038/nature19949
73. Yates JR 3rd. Recent technical advances in proteomics. F1000Res. (2019) 8(F1000 Faculty Rev):351. doi: 10.12688/f1000research.16987.1
74. Latosinska A, Frantzi M, Vlahou A, Merseburger AS, Mischak H. Clinical proteomics for precision medicine: the bladder cancer case. Proteomics Clin Appl. (2018) 12:1700074. doi: 10.1002/prca.201700074
75. Khan AA, Al-Mahrouqi N, Al-Yahyaee A, Al-Sayegh H, Al-Harthy M, Al-Zadjali S. Deciphering urogenital cancers through proteomic biomarkers: a systematic review and meta-analysis. Cancers. (2024) 16:22. doi: 10.3390/cancers16010022
76. Stroggilos R, Mokou M, Latosinska A, Makridakis M, Lygirou V, Mavrogeorgis E, et al. Proteome-based classification of nonmuscle invasive bladder cancer. Int J Cancer. (2020) 146:281–94. doi: 10.1002/ijc.32556
77. Jiang L, Chen S, Pan Q, Zheng J, He J, Sun J, et al. The feasibility of proteomics sequencing based immune-related prognostic signature for predicting clinical outcomes of bladder cancer patients. BMC Cancer. (2022) 22:676. doi: 10.1186/s12885-022-09783-y
78. Dressler FF, Diedrichs F, Sabtan D, Hinrichs S, Krisp C, Gemoll T, et al. Proteomic analysis of the urothelial cancer landscape. Nat Commun. (2024) 15:4513. doi: 10.1038/s41467-024-48096-5
79. Tabaei S, Haghshenas MR, Ariafar A, Gilany K, Stensballe A, Farjadian S, et al. Comparative proteomics analysis in different stages of urothelial bladder cancer for identification of potential biomarkers: highlighted role for antioxidant activity. Clin Proteomics. (2023) 20:28. doi: 10.1186/s12014-023-09419-8
80. Kong T, Qu Y, Zhao T, Niu Z, Lv X, Wang Y, et al. Identification of novel protein biomarkers from the blood and urine for the early diagnosis of bladder cancer via proximity extension analysis. J Transl Med. (2024) 22:314. doi: 10.1186/s12967-024-04951-z
81. Chang Q, Chen Y, Yin J, Wang T, Dai Y, Wu Z, et al. Comprehensive urinary proteome profiling analysis identifies diagnosis and relapse surveillance biomarkers for bladder cancer. J Proteome Res. (2024) 23:2241–52. doi: 10.1021/acs.jproteome.4c00199
82. Martinez-Reyes I, Chandel NS. Cancer metabolism: looking forward. Nat Rev Cancer. (2021) 21:669–80. doi: 10.1038/s41568-021-00378-6
83. Melo LMN, Lesner NP, Sabatier M, Ubellacker JM, Tasdogan A. Emerging metabolomic tools to study cancer metastasis. Trends Cancer. (2022) 8:988–1001. doi: 10.1016/j.trecan.2022.07.003
84. Beloribi-Djefaflia S, Vasseur S, Guillaumond F. Lipid metabolic reprogramming in cancer cells. Oncogenesis. (2016) 5:e189. doi: 10.1038/oncsis.2015.49
85. Vasseur S, Guillaumond F. Lipids in cancer: a global view of the contribution of lipid pathways to metastatic formation and treatment resistance. Oncogenesis. (2022) 11:46. doi: 10.1038/s41389-022-00420-8
86. Hanahan D, Weinberg RA. Hallmarks of cancer: the next generation. Cell. (2011) 144:646–74. doi: 10.1016/j.cell.2011.02.013
87. Massari F, Ciccarese C, Santoni M, Iacovelli R, Mazzucchelli R, Piva F, et al. Metabolic phenotype of bladder cancer. Cancer Treat Rev. (2016) 45:46–57. doi: 10.1016/j.ctrv.2016.03.005
88. Sahu D, Lotan Y, Wittmann B, Neri B, Hansel DE. Metabolomics analysis reveals distinct profiles of nonmuscle-invasive and muscle-invasive bladder cancer. Cancer Med. (2017) 6:2106–20. doi: 10.1002/cam4.1109
89. Amara CS, Vantaku V, Lotan Y, Putluri N. Recent advances in the metabolomic study of bladder cancer. Expert Rev Proteomics. (2019) 16:315–24. doi: 10.1080/14789450.2019.1583105
90. Piyarathna DWB, Rajendiran TM, Putluri V, Vantaku V, Soni T, von Rundstedt FC, et al. Distinct lipidomics landscapes associated with clinical stages of urothelial cancer of the bladder. Eur Urol Focus. (2018) 4:907–15. doi: 10.1016/j.euf.2017.04.005
91. Lee MY, Yeon A, Shahid M, Cho E, Sairam V, Figlin R, et al. Reprogrammed lipid metabolism in bladder cancer with cisplatin resistance. Oncotarget. (2018) 9:13231–43. doi: 10.18632/oncotarget.24229
92. Lindskrog SV, Schmokel SS, Nordentoft I, Lamy P, Knudsen M, Prip F, et al. Single-nucleus and spatially resolved intratumor subtype heterogeneity in bladder cancer. Eur Urol Open Sci. (2023) 51:78–88. doi: 10.1016/j.eururos.2023.03.006
93. Long F, Wang W, Li S, Wang B, Hu X, Wang J, et al. The potential crosstalk between tumor and plasma cells and its association with clinical outcome and immunotherapy response in bladder cancer. J Transl Med. (2023) 21:298. doi: 10.1186/s12967-023-04151-1
94. Feng C, Wang X, Tao Y, Xie Y, Lai Z, Li Z, et al. Single-cell proteomic analysis dissects the complexity of tumor microenvironment in muscle invasive bladder cancer. Cancers. (2021) 13:5440. doi: 10.3390/cancers13215440
95. Tu A, Said N, Muddiman DC. Spatially resolved metabolomic characterization of muscle invasive bladder cancer by mass spectrometry imaging. Metabolomics. (2021) 17:70. doi: 10.1007/s11306-021-01819-x
96. Moses L, Pachter L. Museum of spatial transcriptomics. Nat Methods. (2022) 19:534–46. doi: 10.1038/s41592-022-01409-2
97. Liu Y, Yang M, Deng Y, Su G, Enninful A, Guo CC, et al. High-spatial-resolution multi-omics sequencing via deterministic barcoding in tissue. Cell. (2020) 183:1665–81. doi: 10.1016/j.cell.2020.10.026
98. Chakraborty S, Sharma G, Karmakar S, Banerjee S. Multi-OMICS approaches in cancer biology: new era in cancer therapy. Biochim Biophys Acta Mol Basis Dis. (2024) 1870:167120. doi: 10.1016/j.bbadis.2024.167120
99. Heo YJ, Hwa C, Lee GH, Park JM, An JY. Integrative multi-omics approaches in cancer research: from biological networks to clinical subtypes. Mol Cells. (2021) 44:433–43. doi: 10.14348/molcells.2021.0042
100. Zhou G, Pang Z, Lu Y, Ewald J, Xia J. OmicsNet 2.0: a web-based platform for multi-omics integration and network visual analytics. Nucleic Acids Res. (2022) 50:W527–33. doi: 10.1093/nar/gkac376
101. Lan W, Liao H, Chen Q, Zhu L, Pan Y, Chen YPP. DeepKEGG: a multi-omics data integration framework with biological insights for cancer recurrence prediction and biomarker discovery. Brief Bioinform. (2024) 25:bbae185. doi: 10.1093/bib/bbae185
102. Wieder C, Cooke J, Frainay C, Poupin N, Bowler R, Jourdan F, et al. PathIntegrate: multivariate modeling approaches for pathway-based multi-omics data integration. PloS Comput Biol. (2024) 20:e1011814. doi: 10.1371/journal.pcbi.1011814
103. Stanojevic S, Li Y, Ristivojevic A, Garmire LX. Computational methods for single-cell multi-omics integration and alignment. Genomics Proteomics Bioinf. (2022) 20:836–49. doi: 10.1016/j.gpb.2022.11.013
104. Flynn E, Almonte-Loya A, Fragiadakis GK. Single-cell multiomics. Annu Rev BioMed Data Sci. (2023) 6:313–37. doi: 10.1146/annurev-biodatasci-020422-050645
105. Li J, Li L, You P, Wei Y, Xu B. Towards artificial intelligence to multi-omics characterization of tumor heterogeneity in esophageal cancer. Semin Cancer Biol. (2023) 91:35–49. doi: 10.1016/j.semcancer.2023.02.009
106. Gontero P, Slyvester R, Pisano F, Joniau S, Vander Eeckt K, Serretta V, et al. Prognostic factors and risk groups in T1G3 non-muscle-invasive bladder cancer patients initially treated with Bacillus Calmette-Guérin: results of a retrospective multicenter study of 2451 patients. Eur Urol. (2015) 67:74–82. doi: 10.1016/j.eururo.2014.06.040
107. Anurag M, Strandgaard T, Kim SH, Dou Y, Comperat E, Al-Ahmadie H, et al. Multi-omics profiling of urothelial carcinoma in situ reveals CIS-specific gene signature and immune characteristics. iScience. (2024) 27:109179. doi: 10.1016/j.isci.2024.109179
108. Hurst CD, Cheng G, Platt FM, Castro MAA, Marzouka NS, Eriksson P, et al. Stage-stratified molecular profiling of non-muscle-invasive bladder cancer enhances biological, clinical, and therapeutic insight. Cell Rep Med. (2021) 2:100472. doi: 10.1016/j.xcrm.2021.100472
109. Strandgaard T, Lindskrog SV, Nordentoft I, Christensen E, Birkenkamp-Demtroder K, Andreasen TG, et al. Elevated T-cell exhaustion and urinary tumor DNA levels are associated with bacillus Calmette-Guérin failure in patients with non-muscle-invasive bladder cancer. Eur Urol. (2022) 82:646–56. doi: 10.1016/j.eururo.2022.09.008
110. Czerniak B, Lee S, Jung SY, Kus P, Bondaruk J, Lee J, et al. Inferring bladder cancer evolution from mucosal field effects by whole-organ spatial mutational, proteomic, and metabolomic mapping. Res Sq. (2024) 10:rs.3.rs-3994376. doi: 10.21203/rs.3.rs-3994376/v1
111. Lu X, Meng J, Su L, Jiang L, Wang H, Zhu J, et al. Multi-omics consensus ensemble refines the classification of muscle-invasive bladder cancer with stratified prognosis, tumor microenvironment and distinct sensitivity to frontline therapies. Clin Transl Med. (2021) 11:e601. doi: 10.1002/ctm2.601
112. Chu G, Ji X, Wang Y, Niu H. Integrated multiomics analysis and machine learning refine molecular subtypes and prognosis for muscle-invasive urothelial cancer. Mol Ther Nucleic Acids. (2023) 33:110–26. doi: 10.1016/j.omtn.2023.06.001
113. Xu JC, Chen ZY, Huang XJ, Wu J, Huang H, Niu LF, et al. Multi-omics analysis reveals that linoleic acid metabolism is associated with variations of trained immunity induced by distinct BCG strains. Sci Adv. (2024) 10:eadk8093. doi: 10.1126/sciadv.adk8093
114. Groeneveld CS, Sanchez-Quiles V, Dufour F, Shi M, Dingli F, Nicolle R, et al. Proteogenomic characterization of bladder cancer reveals sensitivity to apoptosis induced by tumor necrosis factor-related apoptosis-inducing ligand in FGFR3-mutated tumors. Eur Urol. (2024) 85:486–94. doi: 10.1016/j.eururo.2023.05.037
115. Mo Q, Li R, Adeegbe DO, Peng G, Chan KS. Integrative multi-omics analysis of muscle-invasive bladder cancer identifies prognostic biomarkers for frontline chemotherapy and immunotherapy. Commun Biol. (2020) 3:784. doi: 10.1038/s42003-020-01491-2
116. Zhang C, Shen L, Qi F, Wang JC, Luo J. Multi-omics analysis of tumor mutational burden combined with immune infiltrates in bladder urothelial carcinoma. J Cell Physiol. (2020) 235:3849–63. doi: 10.1002/jcp.29279
117. Feng C, Pan L, Tang S, He L, Wang X, Tao Y, et al. Integrative transcriptomic, lipidomic, and metabolomic analysis reveals potential biomarkers of basal and luminal muscle invasive bladder cancer subtypes. Front Genet. (2021) 12:695662. doi: 10.3389/fgene.2021.695662
118. Shi ZD, Han XX, Song ZJ, Dong Y, Pang K, Wang XL, et al. Integrative multi-omics analysis depicts the methylome and hydroxymethylome in recurrent bladder cancers and identifies biomarkers for predicting PD-L1 expression. Biomark Res. (2023) 11:47. doi: 10.1186/s40364-023-00488-3
119. Liu Y, Pang Z, Wang J, Wang J, Ji B, Xu Y, et al. Multi-omics comprehensive analysis reveals the predictive value of N6-methyladenosine-related genes in prognosis and immune escape of bladder cancer. Cancer Biomark. (2024) 40:79–94. doi: 10.3233/CBM-230286
120. Chu G, Shan W, Ji X, Wang Y, Niu H. Multi-omics analysis of novel signature for immunotherapy response and tumor microenvironment regulation patterns in urothelial cancer. Front Cell Dev Biol. (2021) 9:764125. doi: 10.3389/fcell.2021.764125
121. Xu Y, Sun X, Liu G, Li H, Yu M, Zhu Y. Integration of multi-omics and clinical treatment data reveals bladder cancer therapeutic vulnerability gene combinations and prognostic risks. Front Immunol. (2024) 14:1301157. doi: 10.3389/fimmu.2023.1301157
122. Chen R, Hao X, Chen J, Zhang C, Fan H, Lian F, et al. Integrated multi-omics analyses reveal jorunnamycin A as a novel suppressor for muscle-invasive bladder cancer by targeting FASN and TOP1. J Transl Med. (2023) 21:549. doi: 10.1186/s12967-023-04400-3
123. Xia Y, Xiang L, Yao M, Ai Z, Yang W, Guo J, et al. Proteomics, transcriptomics, and phosphoproteomics reveal the mechanism of talaroconvolutin-A suppressing bladder cancer via blocking cell cycle and triggering ferroptosis. Mol Cell Proteomics. (2023) 22:100672. doi: 10.1016/j.mcpro.2023.100672
Keywords: bladder cancer, recurrence, progression, non-muscle-invasive, -omics, genomics, epigenetics, transcriptomics
Citation: Akand M, Jatsenko T, Muilwijk T, Gevaert T, Joniau S and Van der Aa F (2024) Deciphering the molecular heterogeneity of intermediate- and (very-)high-risk non–muscle-invasive bladder cancer using multi-layered –omics studies. Front. Oncol. 14:1424293. doi: 10.3389/fonc.2024.1424293
Received: 27 April 2024; Accepted: 13 September 2024;
Published: 21 October 2024.
Edited by:
Badrinath Konety, Allina Health, United StatesReviewed by:
Jera Jeruc, University of Ljubljana, SloveniaChandra Sekhar Amara, Baylor College of Medicine, United States
Copyright © 2024 Akand, Jatsenko, Muilwijk, Gevaert, Joniau and Van der Aa. This is an open-access article distributed under the terms of the Creative Commons Attribution License (CC BY). The use, distribution or reproduction in other forums is permitted, provided the original author(s) and the copyright owner(s) are credited and that the original publication in this journal is cited, in accordance with accepted academic practice. No use, distribution or reproduction is permitted which does not comply with these terms.
*Correspondence: Frank Van der Aa, ZnJhbmsudmFuZGVyYWFAdXpsZXV2ZW4uYmU=
†ORCID: Murat Akand, orcid.org/0000-0001-8840-5771
Tim Muilwijk, orcid.org/0000-0002-6965-389X
Steven Joniau, orcid.org/0000-0003-3195-9890
Frank Van Der Aa, orcid.org/0000-0002-4617-3666