- Department of Hematology, The Second Affiliated Hospital of Xi’an Jiaotong University, Xi’an, Shaanxi, China
Background: Imatinib is the most widely used tyrosine kinase inhibitor (TKI) in patients with newly diagnosed chronic-phase chronic myeloid leukemia(CML-CP). However, failure to achieve optimal response after imatinib administration, and subsequent switch to second-generation TKI therapy results in poor efficacy and induces drug resistance. In the present study, we developed and validated a nomogram to predict the efficacy of imatinib in the treatment of patients newly diagnosed with CML-CP in order to help clinicians truly select patients who need 2nd generation TKI during initial therapy and to supplement the risk score system.
Methods: We retrospectively analyzed 156 patients newly diagnosed with CML-CP who met the inclusion criteria and were treated with imatinib at the Second Affiliated Hospital of Xi’an Jiao Tong University from January 2012 to June 2022. The patients were divided into a poor-response cohort (N = 60)and an optimal-response cohort (N = 43) based on whether they achieved major molecular remission (MMR) after 12 months of imatinib treatment. Using univariate and multivariate logistic regression analyses, we developed a chronic myeloid leukemia imatinib-poor treatment (CML-IMP) prognostic model using a nomogram considering characteristics like age, sex, HBG, splenic size, and ALP. The CML-IMP model was internally validated and compared with Sokal, Euro, EUTOS, and ELTS scores.
Results: The area under the curve of the receiver operator characteristic curve (AUC)of 0.851 (95% CI 0.778–0.925) indicated satisfactory discriminatory ability of the nomogram. The calibration plot shows good consistency between the predicted and actual observations. The net reclassification index (NRI), continuous NRI value, and the integrated discrimination improvement (IDI) showed that the nomogram exhibited superior predictive performance compared to the Sokal, EUTOS, Euro, and ELTS scores (P < 0.05). In addition, the clinical decision curve analysis (DCA) showed that the nomogram was useful for clinical decision-making. In predicting treatment response, only Sokal and CML-IMP risk stratification can effectively predict the cumulative acquisition rates of CCyR, MMR, and DMR (P<0.05).
Conclusion: We constructed a nomogram that can be effectively used to predict the efficacy of imatinib in patients with newly diagnosed CML-CP based on a single center, 10-year retrospective cohort study.
1 Introduction
Since the introduction of the tyrosine kinase inhibitor (TKI); imatinib, the prognosis of patients with chronic phase chronic myeloid leukemia(CML-CP) has greatly improved, with a 10-year overall survival (OS) of 80–90% (1, 2). The emergence of 2nd generation TKI drugs (e.g., nilotinib and dasatinib) has enabled patients with CML to achieve faster and deeper molecular responses (3–6). Imatinib mesylate is the most widely used drug in patients with chronic-phase CML owing to its safety, efficacy, and pharmacoeconomic advantages. Data show that patients who were initiated with imatinib therapy but failed to meet certain response landmarks such as those proposed in the 2020 European Leukemia Net (ELN) criteria and who were switched to a 2nd or 3rd generation TKI had poor outcomes and easily developed drug resistance, which led to a poor long-term survival (7–11). Therefore, it is extremely important to help clinicians select patients who need 2nd generation TKI treatment as an initial therapy.
Current prognostic scoring systems such as the Sokal, Euro, EUTOS, and ELTS are widely used in clinical practice but they have limitations (12–15). The Sokal and Euro scoring systems were established before the advent of imatinib, and the OS of patients was considered the endpoint of the study. After the advent of imatinib, the survival rate was greatly improved, and the pursuit of survival was quicker molecular responses and higher rates of major molecular response (MMR, ≤ 0.1% BCR::ABLIS) and deep molecular response (DMR,MR4.5 ≤ 0.0032% BCR::ABLIS). However, the EUTOS and ELTS were established after the introduction of imatinib and they cannot effectively identify patients with poor efficacy of imatinib as a first-line treatment.
Therefore, efforts have been made to explore new risk stratification methods to meet the needs of clinical practice. In order to select patients with first-line imatinib treatment failure (IMTF), Zhang Xiaoshuai et al. first proposed a predictive model for IMTF. The model consists of analyzing WBC count, hemoglobin concentration, blood basophil count, and ELTS score, which clinicians can use to estimate the probability of imatinib treatment failure (16). However, the model was established based on clinical data from a single center in Beijing, and there has been no relevant research in other regions of China. In addition, when clinicians treat patients with a warning of efficacy, they also recommend the replacement with 2nd generation TKIs. Therefore, with the development of a new model, we aimed to effectively screen patients with poor efficacy of first-line imatinib and provide guidance for clinicians for the selection of first-line therapy.
Most researchers investigated that the achievement of MMR (BCR::ABLIS ≤ 0.1%) at 12 months is associated with a very low probability of subsequent loss of response and a high likelihood of achieving a subsequent DMR (MR4.0, BCR::ABLIS ≤ 0.01%), which may facilitate discontinuation of TKI therapy. Simultaneously, the significance of achieving MMR within 12 months has been established in various studies to correlate with event free survival (EFS) (17). This hallmark is recognized by the European LeukemiaNet as an optimal response and the National Comprehensive Cancer Network considers IS BCR::ABLIS ≥ 0.1% as an indicator for possible changes in therapy (18).
Hence, we defined patients with CML-CP who did not achieve MMR after 12 months of imatinib treatment as having poor efficacy. We explored the factors influencing the poor efficacy of imatinib as a first-line treatment in patients with CML-CP at 12 months by integrating sociodemographic and laboratory tests. Our study offers a simple but a novel tool to predict imatinib efficacy at 12 months in patients, which can be used for better stratification based on current risk classifications and as a guidance for clinicians for the selection of first-line TKI.
2 Methods
2.1 Cohort selection
We retrospectively enrolled 156 consecutive patients newly diagnosed with chronic-phase CML at our institution between January 2012 and June 2022. All patients were aged at least 18 years, diagnosed with CML-CP, receiving imatinib, followed up for at least 12 months, and who underwent analysis of BCR::ABL transcript type at 12 months of imatinib treatment.
2.2 Data collection
The baseline characteristics, such as sociodemographic characteristics, clinical co-variates, and treatment information were collected. Sociodemographic data included sex, age, annual household income, marital status, level of education, household registration, and comorbidity. The clinical co-variates data included white blood cell (WBC, ×109/L), hemoglobin (HGB, g/L), platelet (PLT, ×109/L), red blood cell distribution width (RDW, %), lymphocyte (LYM, ×109/L), monocyte (MONO, ×109/L), basophils (BAS, %), eosinophil (EOS, %), lactate dehydrogenase (LDH, U/L), alkaline phosphatase (ALP,U/L), marrow blasts (%), spleen size (cm), and blood blasts (%). The treatment information included the analysis of BCR::ABL transcript type at 12 months of age with imatinib mesylate treatment.
2.3 Diagnosis, responses and outcomes
The diagnoses, therapy responses, and outcomes followed the ELN recommendations. Complete cytogenetic response (CCyR) is equivalent to a negative FISH test (+/− 2%) and BCR::ABL≤ 1%.MMR was defined as BCR::ABL ≤ 0.1%. DMR was defined as BCR::ABL ≤ 0.01%/0.0032%. A poor response was defined as an unachieved MMR after 12 months of imatinib mesylate treatment.
2.4 Statistical methods
We summarized the patients’ baseline characteristics using descriptive statistics. Before variable selection, we transformed the continuous variables into categorical variables according to whether they followed a normal distribution and then selected the median or mean as cutoff points. Missing data on candidate prognostic variables were handled using multivariate imputation by chained equation (MICE).
Categorical data were presented as numbers (percentages) and analyzed using the chi-square test or nonparametric test for comparisons. Covariates with P < 0.20 in the uni-variable analyses were included in the multivariate analyses and backward stepwise regression (P<0.10)was used to select variables for inclusion in the nomogram.
A total of 1000 bootstrap resamples and leave-one-out cross validation were used to validate the nomogram internally. We assessed the discriminative ability and predictive accuracy of the model using the time-dependent area under the curve (AUC) of the receiver operating characteristic (ROC) curve, calibration plot, and Hosmer-Lemeshow curve. To assess the improvement in prediction compared with the Sokal, Euro, EUTOS, and ELTS scores, we used integrated discrimination improvement (IDI),continuous net reclassification index (NRI), continuous NRI, and decision curve analysis (DCA), which suggested that the new model had an improvement in predictive capacity compared with the old model when they were greater than zero (19–22).
We divided the patients into low and high risk subgroups according to optimal cut points calculated by the ROC curve and constructed chronic myeloid leukemia imatinib poor treatment (CML-IMP) risk stratification. We verify the applicability of the five risk scoring systems in evaluating cumulative CCyR, MMR and DMR by chi-square test and Fisher test.
All statistical analyses were performed using SPSS 22.0 and R version 4.0.2.
3 Results
3.1 Patients’ characteristics
We collected data from 156 patients who received imatinib mesylate initially. Out of the 156 patients, the following patients were excluded from the study: 26 patients with no available key clinical information; 18 patients with a follow-up period < 12 months; 2 patients treated with imatinib for more than 6 months after diagnosis; 5 patients who did not undergo molecular monitoring at 12 months of treatment with imatinib; and 2 patients below 18 years old. Finally, 103 patients were included in the study. We presented the baseline characteristics of the development cohort in the Table 1. The flowchart of the research design is shown in Figure 1.
3.2 Development of a CML-IMP nomogram
The patients were divided into poor response cohort (N = 60) and optimal response cohort (N = 43), depending on whether they achieved MMR after 12 months of imatinib treatment. In uni-variable analyses, there were 13 variables with statistically significant differences between groups (P < 0.20): sex, age, annual household income, level of education, co-morbidity, white blood cell (WBC, ×109/L), hemoglobin (HGB, g/L), platelet (PLT,×109/L), red blood cell distribution width (RDW,%), monocyte (MONO, ×109/L), eosinophil (EOS,%), alkaline phosphatase (ALP,U/L),and spleen size (cm) (Table 2). We selected five variables to construct a new prognostic model in the form of a nomogram by using the multivariate logistic regression analysis (Table 3). The nomogram consisted of sex, age, hemoglobin levels, spleen size, and alkaline phosphatase levels and comprised a new prognostic model called the CML-IMP model for predicting the probability of poor efficacy of imatinib as a first-line treatment in patients with CML-CP at 12 months (Figure 2).
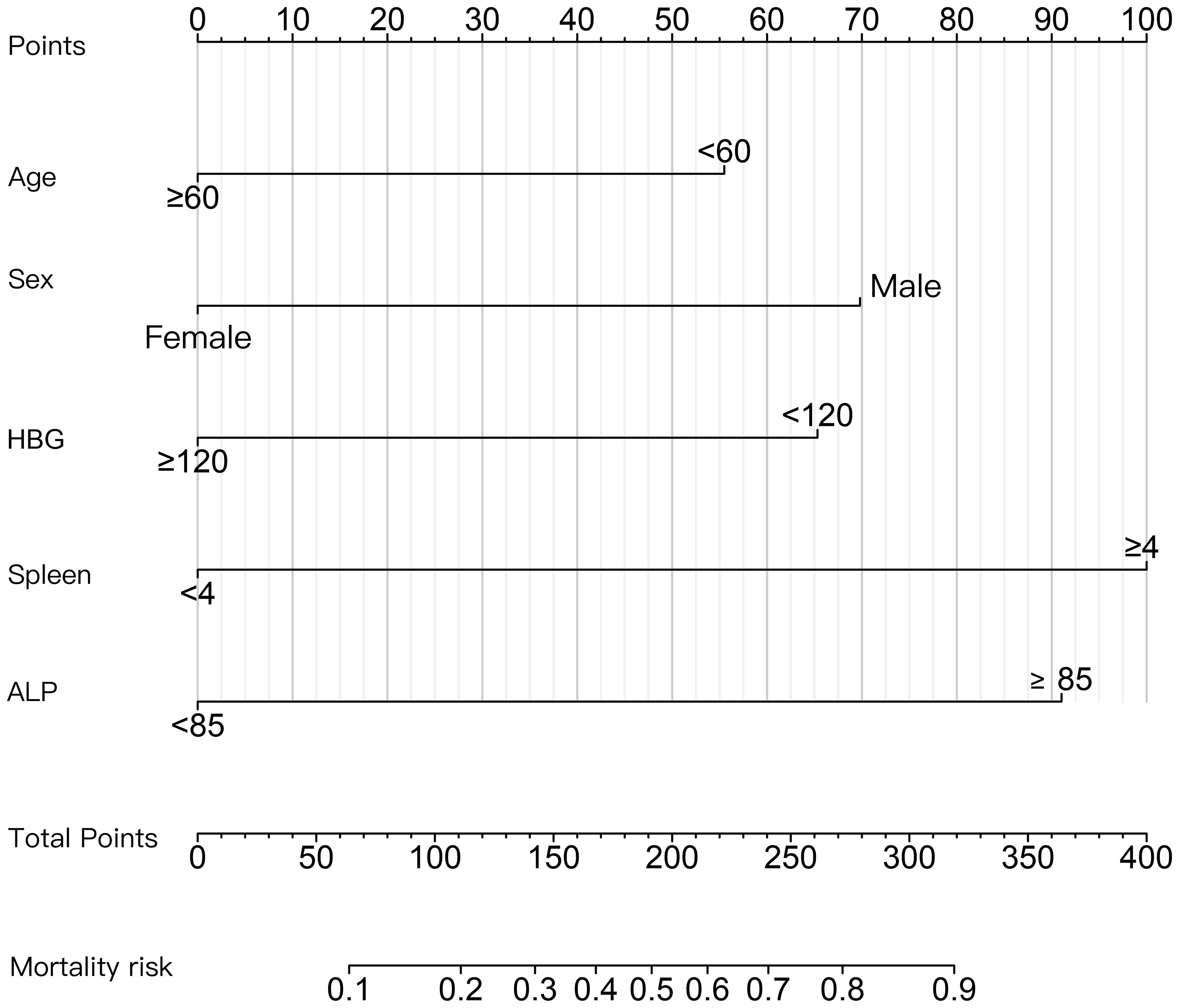
Figure 2 Nomogram for predicting the probability of poor efficacy of imatinib in the first-line treatment of CML-CP patients at 12 months. The value of each variable was scored on a point scale from 0 to 100, after which the scores for each variable were added together. That sum is located on the total points axis, which enables us to predict the probability of poor efficacy of imatinib.
3.3 Validation of the CML-IMP model
In the development of cohorts, 1,000 bootstrap resamples were used to internally validate the CML-IMP model. Leave-one-out cross validation indicated the CML-IMP model could classify 77.7% cases accurately. As for discrimination, time-dependent AUC was 0.851 (95%CI = 0.778~0.925) (Figure 3A). In addition, the results of the Hosmer–Lemeshow test showed that, χ2 = 5.492 (P = 0.704), proving that the model has good goodness of fit (Figure 3B). Moreover, the probabilities predicted by the nomogram matched well with the clinical outcomes (Figure 3B), and the decision curve showed that the model has potential clinical application value (Figure 3C).
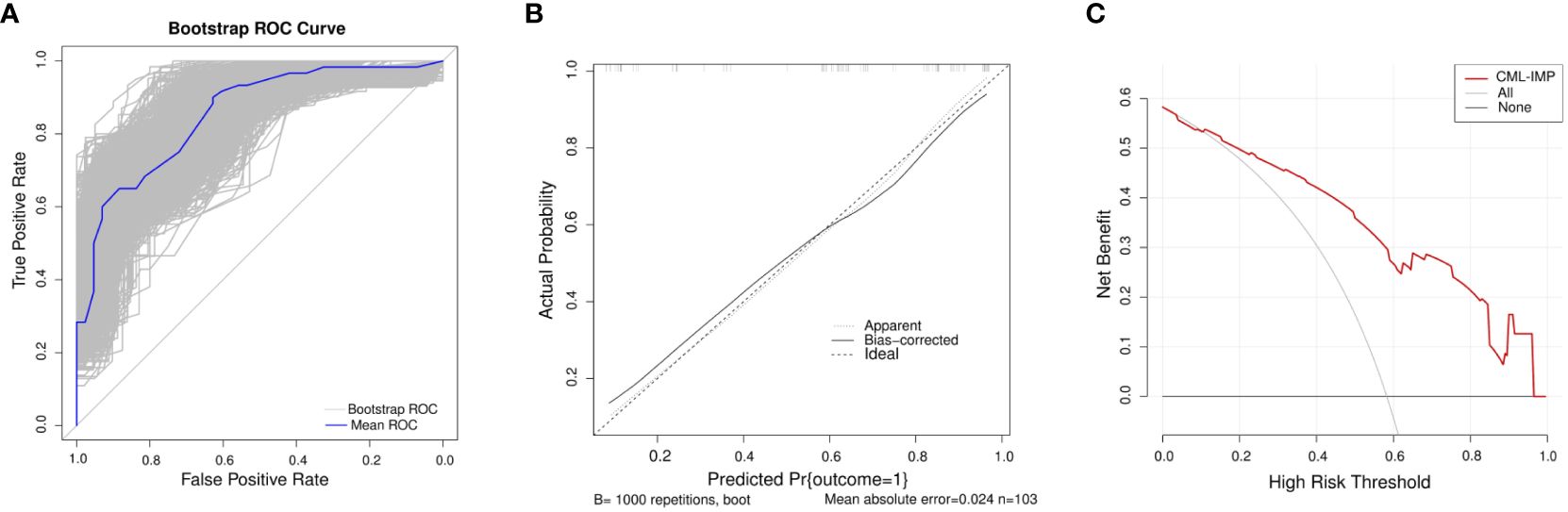
Figure 3 Evaluation of the CML-IMP model (A) Receiver operating characteristic curve for the CML-IMP model generated using bootstrap resampling (1000 times) (B) CML-IMP calibration plot. When the solid line (performance nomogram) was closer to the dotted line (ideal model), the prediction accuracy of the CML-IMP was better. (C) Decision curve analysis for the prediction model. The red solid line is for the prediction model, the gray line is for all patients with CML-CP, and the solid horizontal line indicates no patients have CML-CP. The graph depicts the expected net benefit per patient relative to the CML-IMP prediction of risk of poor efficacy of imatinib. The net benefit increases as the model curve is extended.
3.4 Comparison of the CML-IMP models with standard prediction algorithms
To determine the most predictive model in clinical settings, we compared the CML-IMP model with the Sokal, Euro, EUTOS, and ELTS scores. Figure 4A showed that CML-IMP model improved the model accuracy such that the AUC compared with other models. We also assessed clinical effect by DCA which showed that the CML-IMP model could achieve positive net benefit over a wider range of risk threshold, with higher area under the decision curve analysis (AUDC) than Sokal, EUTOS, and ELTS score (Figure 4B). In addition, we evaluated the IDI, NRI, and continuous NRI to test the improvement in the prediction efficiency of the CML-IMP model and found that the new model improved the prediction of newly diagnosed CML-CP compared to the Sokal, Euro, EUTOS, and ELTS scores (Table 4).
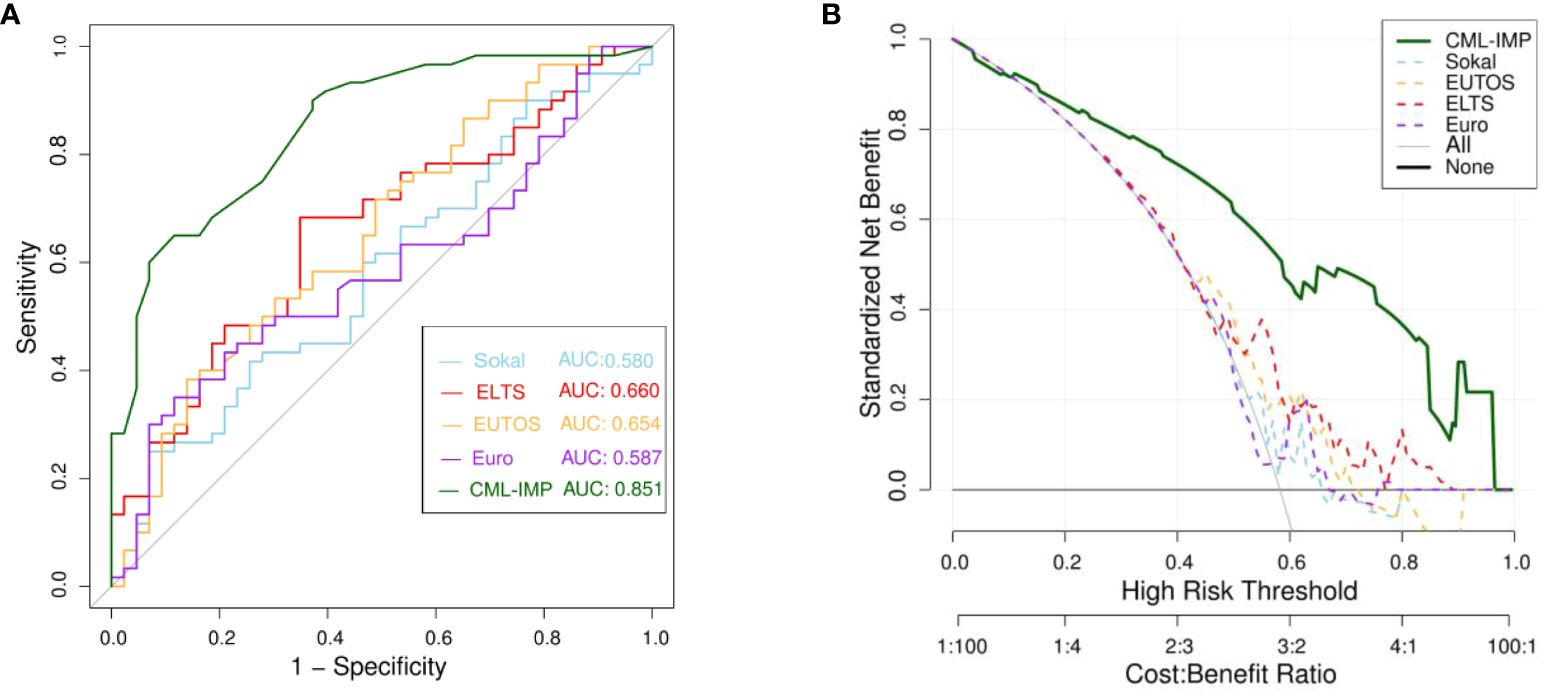
Figure 4 (A) Receiver operating characteristic curve (ROC) analyses for the prediction of probability of poor efficacy of imatinib of five models (B) The DCA was used to estimate clinical usefulness of the four models. The improvement in prediction precision of the CML-IMP model was compared to the Sokal, Euro, EUTOS, and ELTS.
Whats’more,we divided the development cohort into low and high risk subgroups according to optimal cut points calculated by the ROC curve.The low risk subgroup predicted the probability of poor outcome ≤0.6628,and the high subgroup predicted the probability of poor outcome >0.6628. There are 59 patients (57.3%)in low risk group and 44 patients(42.7%) in high risk group according to CML-IMP risk stratification. In predicting treatment response, only Sokal and CML-IMP scoring systems can effectively predict the cumulative acquisition rates of CCyR, MMR, and DMR (P<0.05) (Table 5).
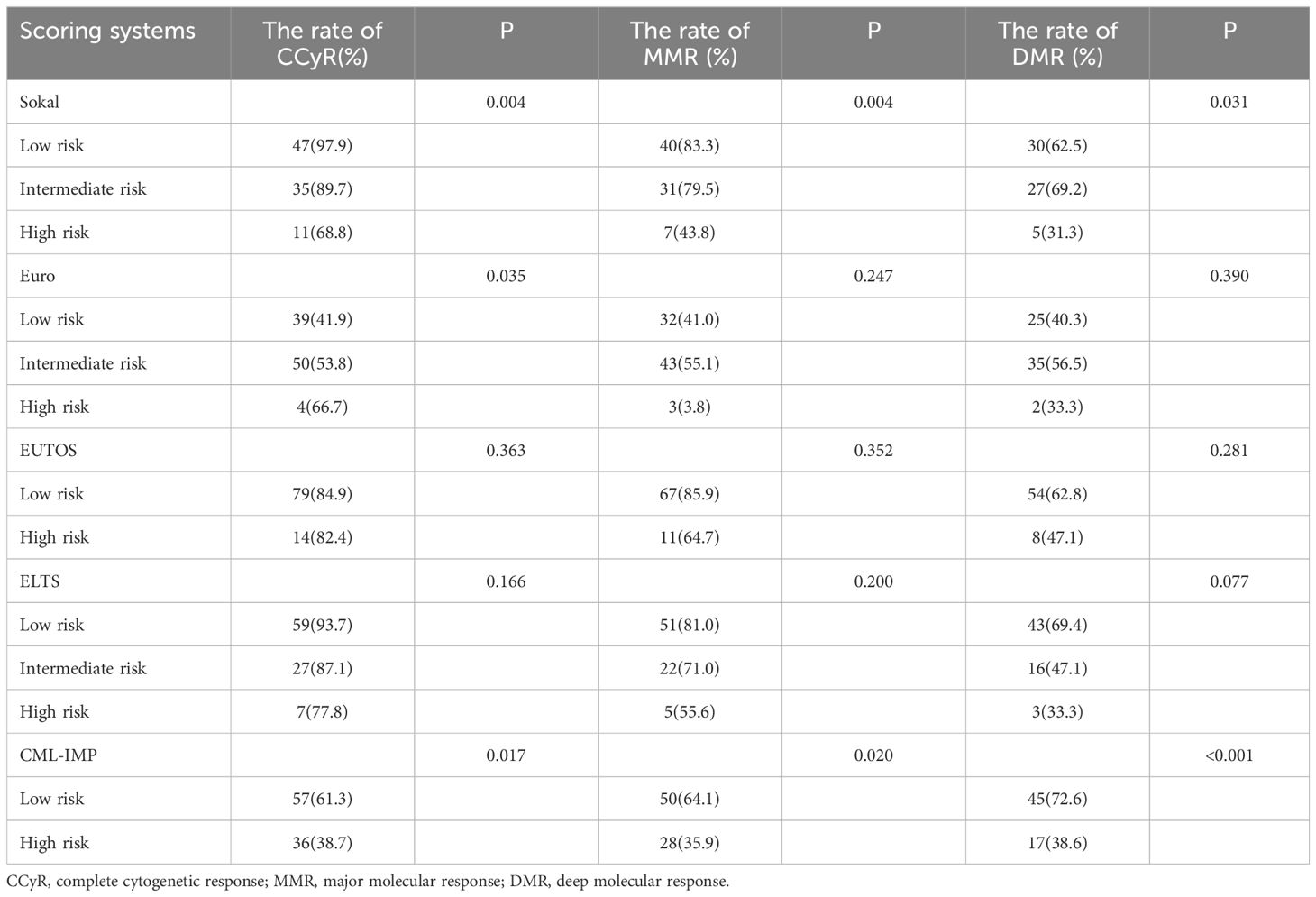
Table 5 Evaluation of molecular response achievement in patients with CML-CP using five scoring systems.
4 Discussion
In this retrospective study, we established and validated a CML-IMP model that included characteristics such as, age, sex, HBG, spleen size, and ALP. The novel CML-IMP model performed well in terms of discrimination, calibration, clinical usefulness, and improvement in prediction, suggesting a good prognostic value in predicting the probability of poor efficacy of imatinib as a first-line treatment strategy for patients with CML-CP at 12 months.
Current models such as Sokal, Euro, EUTOS, and ELTS scores predict TKI treatment response and outcomes based on the clinical characteristics of patients, WBC count at initial diagnosis, HGB, comorbidities, BCR::ABL transcript types, and high-risk additional chromosomal abnormalities. Previous studies have confirmed that sociodemographic factors are significantly associated with the prognosis of patients with chronic myeloid leukemia (23–28). Therefore, in this model, sociodemographic factors were considered, including patient age, sex, annual household income, marital status, level of education, household registration, and other factors. According to research reports, the OS rate of patients with CML in the United States is significantly lower in low-income populations, males, and unmarried patients (23). Similarly, in our study, sex, age, annual household income, and level of education were found to be significant in the univariate analysis; however, in the multivariate analysis, annual household income and level of education were not included, which may be related to our small sample size and confounding factors.
We retrospectively enrolled 156 patients newly diagnosed with CML-CP. The median age of patients with CML-CP at diagnosis was 53 years (18–77). Patients with CML-CP included 60 males and 43 females. Our analysis showed that it is easier to achieve an optimal response (MMR) after 12 months of imatinib treatment in elderly patients (29). In China, a study based on a large population found that with an increase in age, high WBC levels, low hemoglobin concentrations, high percentage of blood basophils decreases at the time of initial diagnosis, indicating that the degree of proliferation and invasion of the disease is gradually decreasing, which also explains why it is easier for elderly patients to achieve MMR when treated with imatinib for 12 months. Contrarily, increased age is a risk factor in the Sokal and ELTS scores, but this may be due to different endpoints of the scores; Sokal score considers the patient’s OS as the study endpoint whereas the ELTS score considers CML-related death as the study endpoint. It has been suggested that the incidence of serious hematological and non-hematological adverse reactions in elderly patients increased, resulting in a significant increase in the proportion of patients who have reduced, interrupted, or discontinued TKI treatment. In addition, there were more comorbidities in the elderly, which may explain the difference in the results between the two studies. Moreover, many real-world retrospective cohort studies have identified poor prognostic factors in male patients with CML, consistent with our findings (23, 30).
Previous studies have proposed factors potentially affecting the prognosis of patients with CML-CP, such as high WBC count, low hemoglobin concentration, high percentage of blood basophils, high ELTS risk score, high-risk ACAs, and possibly some BCR::ABL transcript types (31–33). However, these prognostic factors are controversial and have low prediction accuracy, whereas our new model chose several factors with high prediction discrimination, calibration, and clinical applicability. Additionally, ALP levels were independently associated with imatinib efficacy in our study. ALP hydrolyzes phosphate esters to produce phosphoric acid in an alkaline environment and is a hallmark enzyme after neutrophil mitosis. It has been reported that there is a certain relationship between the ALP level in patients with CML and the changes in the patient’s condition and clinical stage (34).
Furthermore, the CML-IMP model has a greater ability to recognize the risks affecting the efficacy of imatinib as a first-line treatment strategy in patients with CML-CP than the conventional staging system. The nomogram demonstrated its potential value in clinical practice. It can help clinicians select patients who need 2nd generation TKI treatment during initial therapy.
Although the CML-IMP model performed well, the present study has some limitations. First, this was a single-center study of Chinese patients from a single region, which may have limited its generalization. Although our nomogram was validated using bootstraps with 1000 resamples, future prospective multicenter studies are still needed for external validation. Secondly, sociodemographic factors such as marital status, annual household income, educational level, etc., may change after the diagnosis of the disease. Thirdly, we excluded patients who were not regularly followed up at the time of study inclusion, which may lead to selection bias. Finally, this is a real-world retrospective cohort study that inevitably shows some missing values, but we processed the missing data by using multiple imputation methods to minimize the impact of data deficiencies. Therefore, prospective studies are needed in the future to reduce the impact of selection bias and data loss on the conclusions, and further improve the predictive ability of the model.
5 Conclusions
In summary, we developed a user-friendly nomogram with increased accuracy, good clinical utility, and more precise prognostic prediction than the conventional staging system, which could potentially predict the probability of poor efficacy of imatinib mesylate in the first-line treatment of patients with CML-CP at 12 months. It can help clinicians accurately select patients who need 2nd generation TKI treatment as the initial therapy in clinical practice and complement the current risk stratification of patients with CML-CP.
Data availability statement
The raw data supporting the conclusions of this article will be made available by the authors, without undue reservation.
Ethics statement
The studies involving humans were approved by the Ethics Committee of the Second Affiliated Hospital of Xi’an Jiaotong University. The studies were conducted in accordance with the local legislation and institutional requirements. The participants provided their written informed consent to participate in this study.
Author contributions
YXL: Project administration, Funding acquisition, Formal analysis, Visualization, Resources, Conceptualization, Supervision, Investigation, Writing – review & editing, Validation, Software, Methodology, Data curation, Writing – original draft. YLZ: Software, Visualization, Writing – original draft, Data curation, Writing – review & editing. JW: Writing – review & editing, Methodology, Data curation. ALH: Writing – review & editing, Methodology, Data curation. WGZ: Data curation, Writing – review & editing, Methodology. XMC: Methodology, Data curation, Writing – review & editing. YXC: Writing – review & editing, Methodology, Data curation. JL: Data curation, Methodology, Writing – review & editing. PYZ: Writing – review & editing, Methodology, Data curation. JLW: Data curation, Writing – review & editing, Methodology. WHZ: Writing – review & editing, Methodology, Data curation. YY: Writing – review & editing, Methodology, Data curation. XM: Software, Resources, Writing – review & editing. SPC: Resources, Writing – review & editing, Software. LJZ: Resources, Software, Writing – review & editing. TW: Software, Writing – review & editing, Resources. XGW: Resources, Writing – review & editing, Software. XRM: Supervision, Writing – original draft, Investigation, Writing – review & editing, Visualization, Methodology, Formal analysis, Data curation.
Funding
The author(s) declare that no financial support was received for the research, authorship, and/or publication of this article.
Acknowledgments
The authors thank the support from all the medical and nursing staff, Department of Hematology, the Second Affiliated Hospital of Xi’an Jiaotong University, Xi’an, Shaanxi,China.
Conflict of interest
The authors declare that the research was conducted in the absence of any commercial or financial relationships that could be construed as a potential conflict of interest.
Publisher’s note
All claims expressed in this article are solely those of the authors and do not necessarily represent those of their affiliated organizations, or those of the publisher, the editors and the reviewers. Any product that may be evaluated in this article, or claim that may be made by its manufacturer, is not guaranteed or endorsed by the publisher.
References
1. O’Brien SG, Guilhot F, Larson RA, Gathmann I, Baccarani M, Cervantes F, et al. Imatinib compared with interferon and low-dose cytarabine for newly diagnosed chronic-phase chronic myeloid leukemia. N Engl J Med. (2003) 348:994–1004. doi: 10.1056/NEJMoa022457
2. Druker BJ, Guilhot F, O’Brien SG, Gathmann I, Kantarjian H, Gattermann N, et al. Five-year follow-up of patients receiving imatinib for chronic myeloid leukemia. N Engl J Med. (2006) 355:2408–17. doi: 10.1056/NEJMoa062867
3. Jabbour E, Kantarjian HM, Saglio G, Steegmann JL, Shah NP, Boqué C, et al. Early response with dasatinib or imatinib in chronic myeloid leukemia: 3-year follow-up from a randomized phase 3 trial (DASISION). Blood. (2014) 123:494–500. doi: 10.1182/blood-2013-06-511592
4. Doggrell SA, Christensen AM. Are there better Bcr-Abl kinase inhibitors for chronic myeloid leukaemia than imatinib? Evaluation of Saglio G, Kim D-W, Issaragrisil S, et al. Nilotinib versus imatinib for newly diagnosed chronic myeloid leukemia. N Engl J Med. (2010) 362:2251–9. doi: 10.1517/14656566.2011.534780
5. Kantarjian HM, Hochhaus A, Saglio G, De Souza C, Flinn IW, Stenke L, et al. Nilotinib versus imatinib for the treatment of patients with newly diagnosed chronic phase, Philadelphia chromosome-positive, chronic myeloid leukaemia: 24-month minimum follow-up of the phase 3 randomised ENESTnd trial. Lancet Oncol. (2011) 12:841–51. doi: 10.1016/S1470-2045(11)70201-7
6. Hochhaus A, Saglio G, Hughes TP, Larson RA, Kim DW, Issaragrisil S, et al. Long-term benefits and risks of frontline nilotinib vs imatinib for chronic myeloid leukemia in chronic phase: 5-year update of the randomized ENESTnd trial. Leukemia. (2016) 30:1044–54. doi: 10.1038/leu.2016.5
7. Kantarjian HM, Hughes TP, Larson RA, Kim DW, Issaragrisil S, le Coutre P, et al. Long-term outcomes with frontline nilotinib versus imatinib in newly diagnosed chronic myeloid leukemia in chronic phase: ENESTnd 10-year analysis. Leukemia. (2021) 35:440–53. doi: 10.1038/s41375-020-01111-2
8. Cortes JE, Saglio G, Kantarjian HM, Baccarani M, Mayer J, Boqué C, et al. Final 5-year study results of DASISION: the dasatinib versus imatinib study in treatment-naïve chronic myeloid leukemia patients trial. J Clin Oncol. (2016) 34:2333–40. doi: 10.1200/JCO.2015.64.8899
9. Cortes JE, Khoury HJ, Kantarjian HM, Lipton JH, Kim DW, Schafhausen P, et al. Long-term bosutinib for chronic phase chronic myeloid leukemia after failure of imatinib plus dasatinib and/or nilotinib. Am J Hematol. (2016) 91:1206–14. doi: 10.1002/ajh.24536
10. Shah NP, Rousselot P, Schiffer C, Rea D, Cortes JE, Milone J, et al. Dasatinib in imatinib-resistant or -intolerant chronic-phase, chronic myeloid leukemia patients: 7-year follow-up of study CA180–034. Am J Hematol. (2016) 91:869–74. doi: 10.1002/ajh.24423
11. Shah NP, Guilhot F, Cortes JE, Schiffer CA, le Coutre P, Brümmendorf TH, et al. Long-term outcome with dasatinib after imatinib failure in chronic-phase chronic myeloid leukemia: follow-up of a phase 3 study. Blood. (2014) 123:2317–24. doi: 10.1182/blood-2013-10-532341
12. Sokal JE, Cox EB, Baccarani M, Tura S, Gomez GA, Robertson JE, et al. Prognostic discrimination in “good-risk” chronic granulocytic leukemia. Blood. (1984) 63:789–99. doi: 10.1182/blood.V63.4.789.789
13. Hasford J, Baccarani M, Hoffmann V, Guilhot J, Saussele S, Rosti G, et al. Predicting complete cytogenetic response and subsequent progression-free survival in 2060 patients with CML on imatinib treatment: the EUTOS score. Blood. (2011) 118:686–92. doi: 10.1182/blood-2010-12-319038
14. Hasford J, Pfirrmann M, Hehlmann R, Allan NC, Baccarani M, Kluin-Nelemans JC, et al. A new prognostic score for survival of patients with chronic myeloid leukemia treated with interferon alfa. Writing Committee for the Collaborative CML Prognostic Factors Project Group. J Natl Cancer Inst. (1998) 90:850–8. doi: 10.1093/jnci/90.11.850
15. Pfirrmann M, Baccarani M, Saussele S, Guilhot J, Cervantes F, Ossenkoppele G, et al. Prognosis of long-term survival considering disease-specific death in patients with chronic myeloid leukemia. Leukemia. (2016) 30:48–56. doi: 10.1038/leu.2015.261
16. Zhang XS, Gale RP, Zhang MJ, Huang XJ, Jiang Q. A predictive scoring system for therapy-failure in persons with chronic myeloid leukemia receiving initial imatinib therapy. Leukemia. (2022) 36:1336–42. doi: 10.1038/s41375-022-01527-y
17. Shanmuganathan N, Hughes TP. Molecular monitoring in CML: how deep? How often? How should it influence therapy? Blood. (2018) 132:2125–33. doi: 10.1182/blood-2018-05-848630
18. Deininger MW, Shah NP, Altman JK, Berman E, Bhatia R, Bhatnagar B, et al. Chronic myeloid leukemia, version 2.2021, NCCN clinical practice guidelines in oncology. J Natl Compr Canc Netw. (2020) 18:1385–415. doi: 10.6004/jnccn.2020.0047
19. Uno H, Tian L, Cai T, Kohane IS, Wei LJ. A unified inference procedure for a class of measures to assess improvement in risk prediction systems with survival data. Stat Med. (2013) 32:2430–42. doi: 10.1002/sim.5647
20. Pencina MJ, D’Agostino RB, Steyerberg EW. Extensions of net reclassification improvement calculations to measure usefulness of new biomarkers. Stat Med. (2011) 30:11–21. doi: 10.1002/sim.4085
21. Cho H, Yoon DH, Lee JB, Kim SY, Moon JH, Do YR, et al. Comprehensive evaluation of the revised international staging system in multiple myeloma patients treated with novel agents as a primary therapy. Am J Hematol. (2017) 92:1280–6. doi: 10.1002/ajh.24891
22. Fitzgerald M, Saville BR, Lewis RJ. Decision curve analysis. Jama. (2015) 313:409–10. doi: 10.1001/jama.2015.37
23. Perry AM, Brunner AM, Zou T, McGregor KL, Amrein PC, Hobbs GS, et al. Association between insurance status at diagnosis and overall survival in chronic myeloid leukemia: A population-based study. Cancer. (2017) 123:2561–9. doi: 10.1002/cncr.30639
24. Smith AG, Painter D, Howell DA, Evans P, Smith G, Patmore R, et al. Determinants of survival in patients with chronic myeloid leukaemia treated in the new era of oral therapy: findings from a UK population-based patient cohort. BMJ Open. (2014) 4:e004266. doi: 10.1136/bmjopen-2013-004266
25. Bencomo-Alvarez AE, Gonzalez MA, Rubio AJ, Olivas IM, Lara JJ, Padilla O, et al. Ethnic and border differences on blood cancer presentation and outcomes: A Texas population-based study. Cancer. (2021) 127:1068–79. doi: 10.1002/cncr.33347
26. Lee JP, Birnstein E, Masiello D, Yang D, Yang AS. Gender and ethnic differences in chronic myelogenous leukemia prognosis and treatment response: a single-institution retrospective study. J Hematol Oncol. (2009) 2:30. doi: 10.1186/1756-8722-2-30
27. Rego MN, Metze K, Lorand-Metze I. Low educational level but not low income impairs the achievement of cytogenetic remission in chronic myeloid leukemia patients treated with imatinib in Brazil. Clinics (Sao Paulo). (2015) 70:322–5. doi: 10.6061/clinics/2015(05)03
28. Larfors G, Sandin F, Richter J, Själander A, Stenke L, Lambe M, et al. The impact of socio-economic factors on treatment choice and mortality in chronic myeloid leukaemia. Eur J Haematol. (2017) 98:398–406. doi: 10.1111/ejh.12845
29. Wu J, Lu AD, Zhang LP, Zuo YX, Jia YP. Study of clinical outcome and prognosis in pediatric core binding factor-acute myeloid leukemia. Zhonghua Xue Ye Xue Za Zhi. (2019) 40:52–7. doi: 10.3760/cma.j.issn.0253-2727.2019.01.010
30. Ciftciler R, Haznedaroglu IC. Tailored tyrosine kinase inhibitor (TKI) treatment of chronic myeloid leukemia (CML) based on current evidence. Eur Rev Med Pharmacol Sci. (2021) 25:7787–98. doi: 10.26355/eurrev_202112_27625
31. Castagnetti F, Gugliotta G, Breccia M, Iurlo A, Levato L, Albano F, et al. The BCR-ABL1 transcript type influences response and outcome in Philadelphia chromosome-positive chronic myeloid leukemia patients treated frontline with imatinib. Am J Hematol. (2017) 92:797–805. doi: 10.1002/ajh.24774
32. Qin YZ, Jiang Q, Jiang H, Lai YY, Zhu HH, Liu YR, et al. Combination of White Blood Cell Count at Presentation With Molecular Response at 3 Months Better Predicts Deep Molecular Responses to Imatinib in Newly Diagnosed Chronic-Phase Chronic Myeloid Leukemia Patients. Med (Baltimore). (2016) 95:e2486. doi: 10.1097/MD.0000000000002486
33. Zhang XS, Gale RP, Huang XJ, Jiang Q. Is the Sokal or EUTOS long-term survival (ELTS) score a better predictor of responses and outcomes in persons with chronic myeloid leukemia receiving tyrosine-kinase inhibitors? Leukemia. (2022) 36:482–91. doi: 10.1038/s41375-021-01387-y
Keywords: chronic phase chronic myeloid leukemia, imatinib, prognostic model, nomogram, Sokal score, Euro score, EUTOS score, ELTS score
Citation: Li YX, Zhang YL, Wang J, He AL, Zhang WG, Cao XM, Chen YX, Liu J, Zhang PY, Wang JL, Zhao WH, Yang Y, Meng X, Chen SP, Zhang LJ, Wang T, Wang XG and Ma XR (2024) Development and validation of a nomogram to predict poor efficacy of imatinib in the treatment of newly diagnosed chronic phase chronic myeloid leukemia patients. Front. Oncol. 14:1418417. doi: 10.3389/fonc.2024.1418417
Received: 16 April 2024; Accepted: 11 June 2024;
Published: 24 June 2024.
Edited by:
Raffaele Palmieri, University of Rome Tor Vergata, ItalyReviewed by:
Anna M. Eiring, Texas Tech University Health Sciences Center El Paso, United StatesWeiming Li, Huazhong University of Science and Technology, China
Copyright © 2024 Li, Zhang, Wang, He, Zhang, Cao, Chen, Liu, Zhang, Wang, Zhao, Yang, Meng, Chen, Zhang, Wang, Wang and Ma. This is an open-access article distributed under the terms of the Creative Commons Attribution License (CC BY). The use, distribution or reproduction in other forums is permitted, provided the original author(s) and the copyright owner(s) are credited and that the original publication in this journal is cited, in accordance with accepted academic practice. No use, distribution or reproduction is permitted which does not comply with these terms.
*Correspondence: Xiaorong Ma, 425966345@qq.com