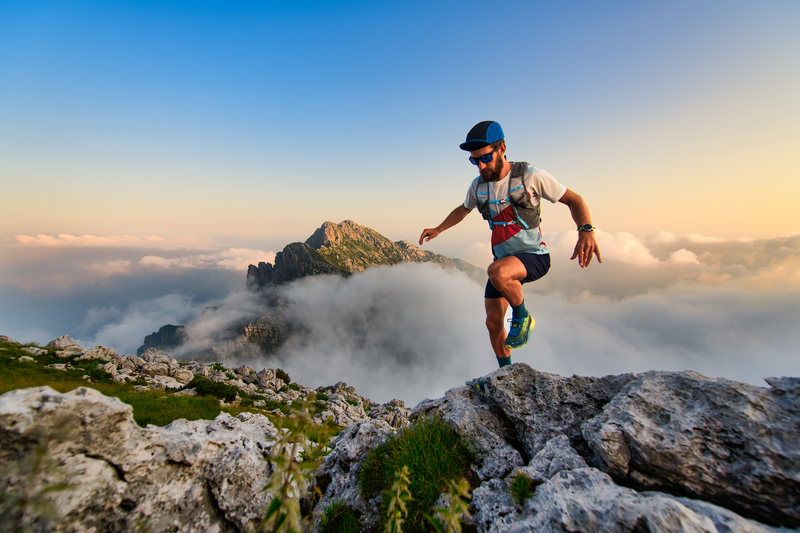
95% of researchers rate our articles as excellent or good
Learn more about the work of our research integrity team to safeguard the quality of each article we publish.
Find out more
ORIGINAL RESEARCH article
Front. Oncol. , 16 December 2024
Sec. Molecular and Cellular Oncology
Volume 14 - 2024 | https://doi.org/10.3389/fonc.2024.1408697
This article is part of the Research Topic Deciphering the Role of Signature Genes in Cancer Prognosis and Therapy Resistance View all 10 articles
Introduction: The transcriptomic characteristics of ROS1+ non-small cell lung cancer (NSCLC) represent a crucial aspect of its tumor biology. These features provide valuable insights into key dysregulated pathways, potentially leading to the discovery of novel targetable alterations or biomarkers.
Methods: From The Cancer Genome Atlas (TCGA) and the Gene Expression Omnibus (GEO) databases, all available ROS1+ (n = 10), ALK+ (n = 5) and RET+ (n = 5) NSCLC tumor and ROS1+ cell line (n = 7) RNA-sequencing files were collected. In addition, 10 healthy lung RNA-seq samples were included. Differential gene expression with DESeq2 (R package) and gene co-expression (WGCNA, R package) analyses were performed. Functional annotation was performed through Gene Set Enrichment Analysis (GSEA) using Webgestalt and RNAseqChef, Over-Representation Analysis (ORA) through Enrichr. iRegulon was used to identify enriched transcription factors that regulate a gene co-expression module.
Results: ROS1+ NSCLC samples were significantly enriched for the nucleotide synthesis and cell adhesion KEGG pathways compared to ALK+ and RET+ samples. Moreover, NOTCH1 was significantly downregulated in ROS1+ NSCLC and PD-L1 was weakly expressed. When comparing ROS1+ tumor versus cell line transcriptomes, an upregulation of MYC and MET was found in cell lines together with a significantly decreased expression of HER3, HER4 and BRAF. Within ROS1-tumors, GJB2 was overexpressed in the CD74- and CLTC-ROS1+ subgroups. The differential expression of IL20RB and GJB2 in cell lines was confirmed through RT-qPCR. Finally, the gene co-expression analysis unveils a gene cluster involving cell cycle-related genes which significantly correlates with the disease stage of patients. In addition, we propose TFDP1 and ISL1 as key ROS1-specific transcription factors.
Conclusion: This study highlights cell adhesion and nucleotide synthesis as crucial signatures in ROS1+ NSCLC. The upregulation of GJB2 may serve as a prognostic biomarker, along with IL20RB, a known mediator of bone metastases. Furthermore, TDFP1 and ISL1 were identified as relevant transcription factors that could potentially regulate the biological processes in ROS1-rearranged NSCLC.
ROS1+ non-small cell lung cancer (NSCLC) is a molecular subgroup of malignancies which account for approximately 2% of newly diagnosed lung cancer cases every year (1, 2). Chromosomal rearrangements that involve the 6q22 locus harboring ROS1 result in the formation of oncogenic fusion proteins. Thus, ROS1+ NSCLC belongs to the category of oncogene-addicted tumors like ALK+, RET+ and NTRK+ NSCLC, among others (3, 4). The overexpression of a constitutively activated tyrosine kinase-containing fusion protein promotes cell growth, proliferation and migration through the stimulation of the MAPK, PI3K/mTOR and JAK/STAT signaling pathways (1). Oncogene-addicted lung adenocarcinomas are predominantly diagnosed in young, non-smoker patients. They present a low tumor mutational burden (TMB) and show a poor response towards immune checkpoint inhibitors (5). Concerning the treatment of ROS1+ NSCLC, tyrosine kinase inhibitors (TKIs) are the most effective targeted therapies in first line. However, resistance is observed in the majority of patients after a certain period of treatment, caused by intrinsic (e.g. kinase point mutations that prevent inhibitor binding) or extrinsic resistance mechanisms, such as histological transformation (e.g. to small cell lung cancer) or the activation of bypass signaling cascades such as EGFR or c-MET (6–9). Although last-generation TKIs such as repotrectinib and the investigational NVL-520 have shown potent activity against the aggressive ROS1 kinase domain point mutations like G2032R (10, 11), heavily pre-treated patients presenting either extrinsic resistance or brain, bone or liver metastases represent a clinical challenge. Unless other targetable alterations are present driving resistance, patients in this setting rely on chemotherapy-based regimes, with a limited benefit (12). In addition, a remarkable heterogeneity in disease outcomes and metastatic patterns is typically observed among patients, highlighting the need for a deeper understanding of the ROS1+ NSCLC tumor biology (13, 14).
Intriguingly, the biological processes defining ROS1+ NSCLC beyond the tyrosine kinase-mediated signaling remain largely underexplored. This pathway canonically induces the neoplastic transformation of alveolar type II cells. However, the dysregulation of molecular processes not directly orchestrated by the ROS1 signaling requires further elucidation. It is known that ROS1 rearrangements, due to their pro-tumorigenic role, are mainly mutually exclusive with alterations affecting homologous kinases such as ALK, RET and NTRK. Despite converging in the same cellular signaling axes, each oncogenic fusion type results in different disease trajectories. For instance, ALK+ and RET+ NSCLC patients are at higher risk of developing brain metastases compared to ROS1+ NSCLC during treatment (15). Moreover, the presence of additional mutations that might explain the tumor evolution, such as tumor-suppressor inactivating variants or copy number gains of oncogenes, has not been fully characterized within the ROS1+ NSCLC setting. Although keystone findings in the initiation and evolution of lung adenocarcinoma have been recently published, little attention is paid to ROS1-driven tumors (16). This can be explained by the low prevalence of ROS1 rearrangements across NSCLC patients; which restricts the access to the substantial amount of tumor specimens required to conduct solid studies.
The culprits behind the heterogeneous disease outcomes, poor response to immune checkpoint inhibitors and metastases development of ROS1+ NSCLC have not been highlighted yet. Nevertheless, developmental signaling pathways such as the Notch and the Sonic Hedgehog (Shh) pathways are widely known to be aberrantly activated in NSCLC, and co-exist with driver oncogene mutations (17–21). Importantly, Notch signaling orchestrates the epithelial-to-mesenchymal transition (EMT) of NSCLC cells, a critical process at the initial phase of metastasis and drug resistance (22). On top of that, non-canonical oncogenic signaling pathways have lately drawn the attention to the lung cancer field, as exemplified by the SPP1 (osteopontin/phosphoprotein 1) pathway (23). A study by Liu et al. unveiled through single cell RNA-sequencing the pro-tumorigenic role of GJB2 in LUAD (24). This gene encodes the gap junction beta-2 protein belonging to the connexin family, which was described to modulate intercellular communication and extracellular matrix remodeling. Hence, assessing the expression levels of genes involved in the Notch and Shh pathways together with GJB2 will shed light on the ROS1+ NSCLC pathomechanisms.
Some specific insights into ROS1+ tumor evolution were provided in a recent study by Neel et al., demonstrating that the subcellular localization determined by the ROS1 fusion partner gene modulates the downstream signaling pathway that will be activated (25). This phenomenon will likely be reflected at a gene expression level. Besides contrasting these findings using publicly available data, in this study we hypothesized that ROS1+ NSCLC is defined by specific transcriptomic signatures compared to other oncogene-driven tumors like ALK+ or RET+ lung adenocarcinomas. In parallel to the deeper exploration of the biology of ROS1+ NSCLC, the second goal of this exploratory analysis consists in identifying candidate genes whose dysregulated expression in a ROS1+-specific manner might be considered as novel therapeutic targets or biomarkers.
Gene expression RNA-seq data files were obtained from publicly available databases Gene Expression Omnibus (GEO) (fastq) and The Cancer Genome Atlas (TCGA) (raw gene counts) after selecting for ROS1-fusion containing lung cancer RNA-seq samples. The raw read counts for GEO samples were acquired adhering to the specifications outlined in the published GDC mRNA quantification analysis pipeline, which was also utilized for TCGA samples (GRCh38.d1.vd1_gencode.v36 GDC reference genome, STAR 2.7.5c).
HCC-78 cells were obtained from the German Collection of Microorganisms and Cell Cultures GmbH (DSMZ, Germany). CUTO-28, CUTO-37, CUTO-38 and CUTO-27 were kindly provided by Prof. Dr. Robert C. Doebele (Division of Medical Oncology, University of Colorado School of Medicine, Anschutz Medical Campus). Cells were cultured in RPMI 1640 medium supplemented with 10% fetal bovine serum (FBS) and L-glutamine 1% v/v; at 37°C, 5% CO2 in a humidified incubator.
To validate the chosen significant differentially expressed genes (DEGs), 2 μg of total RNA extracted with QIAgen RNeasy RNA isolation kit was employed to synthesize the complementary DNA (cDNA) using the SuperScriptTM III First-Strand Synthesis kit (Thermo Fisher, cat. # 18080051). The following primers were designed to assess the expression levels of the genes (5’➔3’): GJB2 (FWD: TGGTGGACCTACACAAGCA, REV: TGGAGAAGCCGTCGTACAT), IL20RB (FWD: CTGAAGGTCCTGAGTGTGATG, REV: GAGGTCTGTGAGCCCAATG), GAPDH (FWD: TGCACCACCAACTGCTTAGC, REV:
GGCATGGACTGTGGTCATGAG), HPRT1 (FWD: TGACACTGGCAAAACAATGCA, REV:
GGTCCTTTTCACCAGCAAGCT) and YWHAZ (FWD: CGAAGCTGAAGCAGGAGAAG, REV: TTTGTGGGACAGCATGGATG). The quantitative PCR was performed using the SYBR Green Master Mix 2x (Eurogentec, cat. # RT-SN2X-03+) in a Bio-Rad CFX96 real-time PCR system. Data was analyzed with the Bio-Rad CFX Maestro software (Bio-Rad v2.3) and qbasePLUS (Biogazelle). Gene expression levels are reported as calibrated and normalized relative quantities (CNRQ) ± standard error (SE) obtained from the gene normalization considering GAPDH, HPRT1 and YWHAZ as reference target genes.
The read counts associated with protein coding genes from all samples were consolidated into a matrix, serving as input for the DESeq2, R package (26). Gene counts related to Y chromosome, mitochondrial, and ribosomal RNA were excluded from subsequent analysis. In the differential gene expression analysis, the batch effect correction was applied using the removeBatchEffect function from the limma R package (27). Multiple contrasts were defined to assess differential expression across various groups within the study (Supplementary File 1). In each comparison, genes with a p-value less than 0.05, following multiple testing correction using the Benjamini-Hochberg (BH) method, were considered to be statistically significant and thus identified as differentially expressed. The cut-offs for the BH method include log2(fold change) = |2| and false-discovery rate (FDR) < 0.05. The clustering of the cell line samples was performed through the K-means method. K=7 was chosen given that 7 different cell lines were analyzed and the three biological replicates of each sample clustered together, as verified upon the DESeq2 analysis. Correlation between ROS1 expression and oncogenes of interest across ROS1+ tumor samples was calculated using the Spearman rank method in RNAseqChef (28). Regarding the RT-qPCR experiments, technical triplicates were used per each reaction and every RT-qPCR was performed twice. Differences in GJB2 and IL20RB expression between cell lines were assessed using 1-way ANOVA, a Bonferroni correction for multiple comparisons and α=0.05 in GraphPad Prism v9.
The investigation of dysregulated genes involved the utilization of Weighted Gene Co-expression Network Analysis (WGCNA, R package) to identify co-expressed modules (i.e. clusters of genes exhibiting similar expression patterns across samples) and hub genes (i.e. genes playing a central and highly connected role within a co-expression module) (29). It is based on the assumption that highly correlated genes within a module (cluster) are involved in common biological processes. For this analysis, we selected the 70% most variable genes from the gene expression data, after filtering, normalization and batch correction. Then, a signed correlation matrix was created by calculating the biweight midcorrelation (a robust alternative to the Pearson correlation) across all gene pairs. This adjacency matrix was obtained using a soft threshold power of 8 to establish a scale-free topology. The topological overlap measure (TOM) was then computed for all genes, considering both direct pairwise correlations and shared correlations with other genes. Unsupervised clustering of genes in the hierarchical cluster tree, based on a dissimilarity threshold of 1-TOM, resulted in the formation of gene modules. In this study, the minimum module size was defined as 100 genes, and the module-merging cut height was set at 0.3.
The functional characterization of the differentially expressed genes was carried out through different methods. RNAseqChef was used to perform a gene set enrichment analysis (GSEA) and over-representation analysis (ORA) together with Webgestalt and Enrichr (30, 31).
Survival data obtained from the lung adenocarcinoma (LUAD) cohort available at TCGA database was plotted with GEPIA2 (32). All the subtypes were included in the cohort: proximal inflammatory (PI), proximal proliferative (PP) and terminal respiratory unit (TRU).
With regard to the ROS1+ patient characteristics shown in Table 1, among samples gathered in this study (n=10), the median age at diagnosis was 63 years-old and both sexes are represented in a balanced manner, with 50% males and 50% females respectively. Diverse ethnicities are also present in the study, with 50% of Caucasian (5/10), 33% of Asian (3/10) and 10% of African-American (1/10) descent. Concerning disease stage, half of the sequenced samples were categorized as a IIB (5/10), followed by stage IIIA and IB in equal proportions each (2/10) and only one sample (1%) was collected at stage IV (1/10). Finally, previous treatment received by the patients was not reported for all samples. Within the available annotations, chemotherapy alone or in combination with radiotherapy or immunotherapy was the most common approach (3/10). Only one patient received radiation, immuno- and chemotherapy previously.
To begin with, we sought to confirm the overexpression of the rearranged tyrosine kinase in each of the three tumor types under investigation (Figure 1A). While oncogene overexpression was evident across all subtypes, statistical significance was achieved in the following comparisons: ALK+ vs ROS1+ tumors (p < 0.001), RET+ vs ROS1+ (p < 0.001), ROS1+ vs RET+ tumors (p = 0.01), and ROS1+ vs normal lung tissue (p < 0.001).
Figure 1. ROS1+ NSCLC signature. (A) Gene expression levels of the oncogenic kinases in ALK+, RET+, ROS1+ tumors and normal lung tissue. (B) Venn diagram reflecting the significant differentially expressed genes (DEGs) between two comparisons: (1) ROS1+ tumor specimens and cell lines versus normal lung tissue and (2) ROS1+ tumor specimens and cell lines versus ALK+ and RET+ tumor samples. (C) Circos plot reflecting the top 10 enriched biological processes in ROS1+ NSCLC. (D) IL20RB mRNA levels in HCC-78, CUTO-28 and CUTO-37 cell lines expressed as calibrated and normalized relative mRNA quantity (CNRQ) ± SEM. A 1-way ANOVA test was performed considering a Bonferroni correction and a chosen α = 0.05. (E) Over-representation analysis (ORA) depicting the key enriched pathways in ROS1+ tumor samples and cell lines versus normal lung tissue. (F) GSEA summarizing the dysregulated KEGG pathways in ROS1+ NSCLC compared to normal lung tissue and ALK+/RET+ tumor specimens.
Next, in an effort to profile a ROS1+ specific expression signature, transcriptomes of ROS1+ tumor samples and ROS1+ patient-derived cell lines were compared to normal lung tissue or ALK/RET+ NSCLC specimens. The number of overlapping significant differentially expressed genes in both contrasts are shown in Figure 1B. 118 genes were differentially expressed in ROS1+ compared to both normal tissue and non-ROS1+ NSCLC. 805 genes were found to be specifically and significantly dysregulated in ROS1+ samples compared to normal lung tissue. In addition, 169 specifically and significantly differentially expressed genes (DEGs) were obtained from the comparison between ROS1 and ALK/RET-driven tumors. We then proceeded with an over-representation analysis (ORA) for the 118 ROS1+ specific DEGs to determine which biological functions were enriched in ROS1-rearranged tumors and cell lines based on the overlapping 118 gene group. The top 10 enriched categories and the log2(fold change) of the genes associated with each biological function are illustrated in Figure 1C. Firstly, metabolic processes related to nucleotide synthesis, such as the response to purine-containing compound, amine or pyridine-containing metabolic process and the response to organophosphorus, were the most enriched. The ROS1-specific overexpression of NMNAT2, an enzyme involved in nicotinamide adenine dinucleotide (NAD) synthesis, and ISL1, a crucial transcription factor regulating glycolysis and tumorigenesis, highlight the metabolic dependencies of ROS1+ tumor specimens and cell lines. Moreover, several significantly overexpressed genes, including IL20RB, were associated with the positive regulation of cytokine production. Considering the role of IL20RB in promoting bone metastasis in lung adenocarcinoma (33), we selected this gene for further validation. RT-qPCR was performed to confirm the expression of IL20RB in cell lines, as shown in Figure 1D. Furthermore, the analysis revealed an overrepresentation of biological processes related to cell adhesion, including integrin activation (characterized by RASIP1 overexpression), homotypic cell-cell interaction, and receptor-mediated endocytosis.
To obtain a more comprehensive understanding of the gene expression landscape, we explored the primary differences between ROS1+ NSCLC samples and cell lines compared to normal lung tissue. This approach aimed to highlight the crucial pathways that may contribute to the malignant transformation process in this specific tumor subtype. For this purpose, we carried out an over-representation analysis (ORA). As shown in Figure 1E, the MAPK signaling pathway (Gene ratio = 1.78, p = 0.4) and the focal adhesion KEGG pathways (GR = 2.31, p = 0.15) contained the highest amount of the significant DEGs. In addition, the choline metabolism in cancer (GR = 3.2, p = 0.15), the neurotrophin signaling pathway (GR = 2.56, p = 0.24) and the Vibrio cholerae infection were also over-represented categories (GR = 3.97, p = 0.18). To complement the initial findings, we expanded the GSEA-based analysis to identify significantly upregulated or downregulated pathways in ROS1+ lung adenocarcinomas and cell lines in comparison to ALK/RET+ tumor samples (Figure 1F). Interestingly, the analysis revealed an upregulation of gene sets associated with the cell cycle and homologous recombination, accompanied by a downregulation of gene sets related to tyrosine and beta-alanine metabolism, lysosome function, and the chemokine signaling pathway (all p-values < 2e-16).
Whole tumor transcriptome analysis enables accurate profiling of both the tumor cells and the stromal compartment, which comprise a heterogeneous population of cells that work together to enhance the fitness of the tumor cells. Therefore, directly comparing ROS1+ lung adenocarcinomas with ALK+ or RET+ tumors, while excluding cell lines, provides further insights into the tumor microenvironment. Figure 2A shows the heatmap resulting from clustering ROS1+ and ALK+ tumor transcriptomes based on the significant DEGs between the two groups. Samples clustered together according to their driver mutation, indicating an oncogene-dependent modulation of the gene expression profile. Subsequent GSEA revealed a significant upregulation of the interleukin-17 signaling pathway, the ribosome signature and the systemic lupus erythematosus KEGG pathways in ROS1+ tumors compared to ALK+ specimens (Figure 2B). In addition, downregulation of the cGMP-PKG signaling pathway, the aldosterone synthesis and secretion signature together with primary immunodeficiency and vascular smooth muscle contraction gene sets was observed in ROS1+ vs ALK+ tumors. Thus, both up and downregulated gene sets implicate differences concerning inflammatory and protein synthesis pathways. Similarly, ROS1+ and RET+ specimens were collated, revealing a mixed clustering pattern (Figure 2C). In this case, three out of the four RET+ samples grouped together whilst one shared higher similarity with ROS1+ samples. GSEA unveiled significant upregulation of immune response, hematopoietic cell lineage, cell adhesion and cholesterol metabolism pathways in ROS1-rearranged tumors. Interestingly, no significantly upregulated gene sets were observed in RET+ specimens (Figure 2D).
Figure 2. Differences between ROS1+ and ALK+/RET+ tumors. (A) Clustergram reflecting the differences between ROS1+ and ALK+ tumor specimens. (B) GSEA resulting from the significantly DEGs between ROS1+ and ALK+ tumors. (C) Clustergram reflecting the differences between ROS1+ and RET+ tumor specimens. (D) GSEA resulting from the significantly DEGs between ROS1+ and RET+ tumors. (E) Gene expression levels of the NOTCH family and (F) canonical tumor-suppressor genes and oncogenes.
We explored the impact of the Notch signaling pathway within ROS1+ NSCLC, as it is known to exert a pro-tumorigenic role in NSCLC (Figure 2E). Although no genes regulated through the Notch signaling pathway were significantly dysregulated, we found that NOTCH1 expression decreased significantly in ROS1+ tumor samples compared to RET+ specimens (p=0.04). With regard to the other members of the NOTCH gene family, NOTCH2 and NOTCH3 reflected similar expression trends across tumor types. Interestingly, NOTCH4 exhibited a modest expression in ROS1+ tumors compared to ALK+ and RET+ specimens, although the difference did not reach statistical significance. Considering the role of the Notch pathway in promoting epithelial-to-mesenchymal transition (EMT), we conducted a comprehensive analysis to explore the association between NOTCH1 expression and the metastatic characteristics of lung adenocarcinoma (LUAD) patients across multiple studies. As illustrated in Supplementary Figure 3A, the group exhibiting low NOTCH1 expression levels comprised a significantly higher proportion of individuals with TNM stage M0 (p=0.0021), suggesting an absence of metastatic activity in the tumor. To extend our understanding of the patterns of metastatic progression, we retrieved the clinical details of ROS1+, ALK+ and RET+ NSCLC patients from the “Metastatic NSCLC study” by Jee et al. (34). Supplementary Figure 3B shows that ROS1+ NSCLC patients presented lower rates of extrathoracic metastases, being the central nervous system (CNS) and liver the most common sites. Contrarily, ALK+ and RET+ NSCLC patients were diagnosed with bone and soft tissue metastatic lesions as well as CNS and liver. Thus, higher NOTCH1 expression in RET+ specimens might be related to the EMT-promoting effect and enhanced metastatic disease in RET+ NSCLC patients.
In addition, changes in expression of canonical tumor-suppressor genes such as BRCA1, BRCA2 and TP53 as well as oncogenes like BRAF, EGFR, KRAS, MET and MYC were assessed (Figure 2F). No significant differences were observed between the various tumor types, suggesting that the expression of these genes is not dependent on the specific rearrangement. Furthermore, we investigated the expression levels of CD274, a gene that encodes the programmed death-ligand 1 (PD-L1), a cell surface protein expressed by neoplastic cells that interacts with its receptor, programmed cell death protein 1 (PD-1). The latter is present in activated T, natural killer (NK) and B lymphocytes, macrophages, dendritic cells (DCs) and monocytes. The interaction between PD-1 and PD-L1 leads to the suppression of cellular immunity against tumor cells. Across the three tumor types, a relatively low level of normalized CD274 counts was observed, with no significant differences detected. This finding supports the classification of ROS1+, ALK+, and RET+ NSCLC as “cold tumors,” which is consistent with the modest benefit of immunotherapy observed in these patient subsets (35, 36).
Given that both ROS1+ NSCLC tumor specimens and ROS1+ patient-derived cell lines were used in this study, evaluating the key transcriptomic differences between tumor and cell line samples is particularly important. As shown in Figure 3A, CUTO and HCC-78 cell lines cluster separately from ROS1+ NSCLC tumor samples. This remarkable difference is reflected by the 6,803 significantly DEGs. Interestingly, the HCC-78 cell line gene expression pattern clustered between the one of CUTO-23 and CUTO-38 cell lines, indicating that HCC-78 cells share common transcriptomic traits with CUTO cell lines. Among the significant DEGs shown in in Figure 3B, tumor specimens overexpressed genes like MUC5B, encoding for the glycoprotein mucin, AEBP1 (AE binding protein 1), SPARCL1 (SPARC-like protein 1), RBX1 (ring box-1) and ELN (elastin). In contrast, genes such as ALDOA (aldolase A), U2AF1 (U2 small nucleolar RNA auxiliary factor 1), MFSD14A (major facilitator superfamily domain containing 14A) and FKBP2 (FKBP prolyl isomerase 2) were upregulated in patient-derived cell lines.
Figure 3. Transcriptomic differences between ROS1+ tumors and patient-derived cell lines. (A) Clustergram highlighting the differences between ROS1+ tumor specimens and patient-derived cell lines. (B) Volcano plot containing more than 6,000 DEGs. (C) Differential expression of relevant oncogenes and tumor-suppressor genes across ROS1+ NSCLC tumor subtypes and patient-derived cell lines. (D) Distribution across chromosomes of the top 500 significant up- and downregulated genes between tumor specimens and cell lines. (E) GSEA elucidating the KEGG pathways up- or down-regulated in patient-derived cell lines versus tumor specimens.
In order to showcase the representativity of the patient-derived cell lines used in the study, special attention was paid to genes involved in extrinsic resistance mechanisms towards TKIs described in ROS1+ NSCLC patients (Figure 3C). The statistical tests were performed relatively to CD74-ROS1 tumor specimens given that it constitutes the most frequent rearrangement subtype. EGFR was significantly overexpressed in CUTO-38 cells (p < 0.001), CUTO-37 (p = 0.012), CUTO-33 (p = 0.04) and CUTO-23 (p = 0.003). ERBB2, whose product is HER2, was significantly downregulated in CLTC-ROS1 tumors (p < 0.001), CUTO-37, CUTO-28, CUTO-27 and CUTO-33 (p < 0.001). Contrarily, it was upregulated in CUTO38 cells (p = 0.001). A similar trend was observed in ERBB3 expression, except for a significant downregulation in HCC-78 cells (p = 0.006) instead of an overexpression in CUTO-38 cells. GJB2 was clearly expressed in CD74- and CLTC-ROS1 tumors, being significantly higher in the latter case (p < 0.001). In contrast, this gene was practically not expressed in the remaining tumor subtypes and cell lines. In parallel, MET was upregulated in CUTO38 and CUTO-37 (p < 0.001) together with CUTO-33 (p = 0.024) and CUTO28 (p = 0.011). MYC was overexpressed in HCC-78 cells (p = 0.021), CUTO33 (p = 0.017) and CUTO28 (p < 0.001). No differences were detected concerning NOTCH4 expression. However, the endogenous ROS1 levels varied across samples. SLC34A2-ROS1 tumors reflected an upregulation (p < 0.001) whilst CUTO-33, CUTO-28, CUTO-27 and CUTO-23 showed lower ROS1 levels (p = 0.01). Finally, TP53 was upregulated in EZR-ROS1 tumors (p<0.001) and downregulated in CUTO33 (p = 0.007), CUTO-28 (p = 0.004) and CUTO-27 cells (p = 0.005).
To investigate whether specific genomic regions contained a notable concentration of differentially expressed genes (DEGs), the top 500 significant DEGs were mapped to their corresponding locations on the genome (Figure 3D). This visualization aimed to provide a graphical representation of the DEG distribution. The analysis revealed that the distribution of these genes was relatively uniform across the genome, with the exception of chromosome 7, which exhibited a higher density of DEGs compared to other chromosomes. GSEA that included the 6,803 DEGs and using the “chromosomalLocation” function revealed a significant enrichment of overexpressed genes in cell lines located in the cytogenetic band chr7p22.3 (Supplementary Figure 1). Interestingly, copy number gains involving this genomic region known to harbor oncogenes such as UNCX, FAM20C, MAD1L1 and PDGFA have been reported during the immortalization process of patient-derived small cell lung cancer lines (37).
GSEA identified pathways whose activation differed between tumor samples and cell lines (Figure 3E). As expected, immune response-related gene sets like allograft rejection, Leishmania infection and viral myocarditis together with cell adhesion molecules (CAMs) were significantly overexpressed in tumor specimens. Contrarily, gene sets corresponding to the cell cycle, DNA replication, DNA repair and RNA transport were enriched in CUTO and HCC-78 cell lines. Therefore, these results highlight the major biological differences between tumor samples and cell lines and can be summarized in cell cycle, DNA repair and inflammatory signatures.
The subsequent exploratory analysis focused exclusively on ROS1+ NSCLC patient-derived cell lines. As illustrated in Figure 4A, these cell lines exhibit a remarkable phenotypic heterogeneity. Their morphology and colony formation do not adhere to a single pattern, leading us to hypothesize that such differences might be reflected at the transcriptomic level. Despite the limited sample size, the differential gene expression analysis unveiled intriguing features, as depicted in the principal component analysis (PCA) shown in Figure 4B. The majority of cell lines clustered together, with a clear separation along the first principal component, which accounted for 72.44% of the variance. CUTO-37 and CUTO-38 were the two cell lines that clustered more distinctly from the rest. This observation is further supported by the dendrogram presented in Figure 4C.
Figure 4. Transcriptomic traits of ROS1+ patient-derived NSCLC cell lines. (A) Phenotypes of some of the cell lines characterized in the study. Phase contrast microscopy images taken at 10X magnification. (B) Principal component plot. (C) Dendrogram and (D) clustergram reflecting the differences across cell lines. (E) GSEA indicating the significantly enriched hallmarks in each cell line. (F) Enriched hallmarks in CUTO-28 and (G) CUTO-37 cell lines.
Next, we identified similar expression patterns per each cell line through a k-means sample clustering. Assuming that each cell line constitutes a separate group and given that the biological replicates clustered together in each line, a K=7 was chosen. The resulting clustergram is shown in Figure 4D, and the following clusters are: 1: HCC-78 (450 genes), 2: CUTO-23 (314 genes), 3: CUTO-38 (764 genes), 4: CUTO-28 (1094 genes), 5: CUTO-37 (1127 genes), 6: CUTO-27 (164 genes) and 7: CUTO-33 (724 genes). The subsequent GSEA performed in order to identify the significantly enriched hallmarks in each cluster is shown in Figure 4E. HCC-78 transcriptome was enriched in KRas-mediated signaling and interferon responses, CUTO-38 cells showed an enrichment in cholesterol and reactive oxygen species (ROS) together with androgen response and bile acid metabolism. CUTO-37 cells were enriched in the EMT, hypoxia and TNFα signaling via NF-ƙB, among other hallmarks. CUTO-33 cells were EMT- and UV response-enriched hallmarks. CUTO-28 cells showed an enrichment in estrogen responses, Myc targets, mTORC1 signaling and E2F targets hallmarks. Finally, CUTO-27 depicted an upregulation in TNFα signaling via NF-ƙB hallmark. No significant hallmarks were identified in CUTO-23 cells. The networks of the GSEA hallmarks identified in the two most distant cell lines according to the dendrogram, CUTO-28 and CUTO-37 are represented in Figure 4F (CUTO-28) and Figure 4G (CUTO-37) respectively.
The molecular subtypes defined by the ROS1 fusion partner genes have been shown to influence downstream signaling due to the different subcellular localizations of the resulting fusion proteins (25). Therefore, we hypothesized that the differential activation of signaling pathways might be reflected at the gene expression level. Three out of the four ROS1-rearranged tumor subtypes were included in the analysis, as there were at least two independent samples per fusion type (CD74-ROS1, SLC34A2-ROS1, and EZR-ROS1). The PCA plot containing PC1 and PC2 indicates that EZR-ROS1 tumors cluster remarkably further from CD74- and SLC34A2-ROS1 tumor specimens, as shown in Figure 5A. The complementary dendrogram showcases the similarity between CD74- and SLC34A2-ROS1 tumors (Figure 5B).
Figure 5. Impact of the ROS1 fusion partner in the tumor transcriptome. (A) Principal component analysis (PCA) of CD74-, SLC34A2- and EZR-ROS1 tumor samples. (B) Clustergram of the cell lines based on their transcriptomic traits (C) GSEA depicting the enriched hallmarks in CD74-ROS1 and (D) EZR-ROS1 NSCLC samples.
Next, a DEG analysis was conducted to unveil the distinctive traits of each ROS1 rearrangement type, done through pairwise comparisons. The cut-off conditions were log2(fold change |2|) > and FDR < 0.05. The obtained volcano plots and heatmaps per each rearrangement are shown in Supplementary Figure 2B, for the CD74-ROS1 signature, 2c for SLC34A2-ROS1 signature and 2d for EZR-ROS1 signature respectively. The functional annotation of the DEGs in each subtype revealed a significant upregulation of CD74-ROS1 tumors in EMT hallmark and a downregulation of TGF-β signaling (Figure 5C). In addition, EZR-ROS1 tumor specimens were characterized by an enrichment in β-catenin signaling and the downregulation of a G2M checkpoint hallmark (Figure 5D). It is worth noting that no significant enrichment was observed in the SLC34A2-ROS1 subtype.
Finally, the correlation between ROS1 expression and selected oncogenes of interest was determined for the CD74-ROS1, SLC34A2-ROS1, and EZR-ROS1 subtypes (Supplementary Figure 2). Notably, MET (Spearman correlation coefficient = 0.964), BRAF (0.963), MYC (0.714), and EGFR (0.39) exhibited positive correlations with ROS1 expression. Conversely, the expression of transcription factors known to drive epithelial-mesenchymal transition (EMT), such as SNAI1 (-0.678) and TWIST1 (-0.785), negatively correlated with ROS1 expression. However, these correlations did not reach statistical significance, which might be attributed to the limited sample size in each group.
Based on our hypothesis, we proceeded to evaluate GJB2 expression as a potential prognostic marker for ROS1-rearranged NSCLC, considering its upregulation compared to ALK+ and RET+ tumors, as well as normal adjacent lung tissue. Statistical significance was achieved only when compared to normal lung tissue (p = 0.042) (Figure 6A). This observation might be attributed to the fact that GJB2 overexpression occurs only in specific ROS1 fusion subtypes. Figure 6B illustrates the normalized GJB2 counts across the investigated ROS1 rearrangement types, revealing that GJB2 expression was present only in CD74-ROS1 and CLTC-ROS1 samples, with a significant upregulation in the latter subtype (p = 0.016). These findings were validated through RT-qPCR in our cell line models, where transcript levels are expressed as calibrated and normalized relative mRNA quantities (CNRQ) ± SEM (Figure 6C). GJB2 expression was not detected in HCC-78 cells harboring the SLC34A2-ROS1 fusion, in contrast to CUTO-28 (TPM3-ROS1) and CUTO-37 (CD74-ROS1) cell lines, which showed significant GJB2 expression (p < 0.0001 in both comparisons). Consequently, the higher levels of GJB2 transcript in CUTO-28 and CUTO-37 lines suggest that GJB2 is actively expressed by tumor cells and that its expression varies depending on the specific ROS1 fusion type.
Figure 6. The role of GJB2 expression in ROS1+ NSCLC. (A) GJB2 expression levels across oncogene-driven NSCLC and normal lung tissue. (B) GJB2 expression across different ROS1 rearrangements in tumor specimens. (C) GJB2 mRNA levels in HCC-78, CUTO-28 and CUTO-37 cell lines expressed as calibrated and normalized relative mRNA quantity (CNRQ) ± SEM. A 1-way ANOVA test was performed considering a Bonferroni correction and a chosen α = 0.05. (D) Kaplan-Meier survival plot of The Cancer Genome Atlas (TCGA) lung adenocarcinoma (LUAD) cohort divided in GJB2-high or low expression levels. (E) GJB2 single-nucleotide variants identified in LUAD patients.
Moreover, we evaluated the prognostic significance of high GJB2 expression in the lung adenocarcinoma (LUAD) cohort from The Cancer Genome Atlas (TCGA) (Figure 6D). LUAD patients with GJB2 overexpression exhibited a poorer prognosis compared to those in the low-GJB2 expression group (Hazard ratio for high GJB2 expression = 1.8; p-value for high GJB2 expression < 0.001). Next, we validated this finding in an independent LUAD patient cohort that combined several studies. As shown in Supplementary Figure 4, the results were concordant with the TCGA cohort. Taking these observations into account, GJB2 expression might explain the variability among ROS1+ NSCLC patient disease outcomes. As reported by Liu et al., the potential mechanism behind the pro-tumorigenic role of high GJB2 expression might be the upregulation of the SPP1 signaling pathway (24). Furthermore, we mapped the reported GJB2 variants within the same TCGA LUAD cohort onto the gene structure, as illustrated in Figure 6E, to identify potential gain-of-function mutations. Among the variants, four were missense mutations: L10M and G130C, located in the connexin domain; and R165Q and V178M, present in the cysteine-rich connexin domain of GJB2. Interestingly, only the V178M variant has been clinically described in the literature, reported as a pathogenic mutation identified in patients with autosomal-recessive hearing loss (38). This suggests that V178M is unlikely to be a gain-of-function mutation in the context of hearing loss. However, the impact of the GJB2 V178M variant on tumor cell biology remains to be elucidated.
Unsupervised methods, such as gene co-expression analysis, enable the identification of gene clusters with positively or negatively correlated expression patterns, independent of sample category or experimental condition. In this study, we identified 18 gene clusters, each characterized by a correlation coefficient and a p-value associated with a specific trait or phenotype. Supplementary Figure 5 illustrates the resulting matrix, with columns representing the traits of the tumor samples included in the analysis. The strongest correlation was observed for the green-yellow cluster (coefficient = -0.48, p = 0.007) with respect to disease stage, suggesting that the expression patterns of genes within this cluster collectively explain a portion of the variability attributed to the disease stage of the samples. The observed eigengene expression patterns of the green-yellow module across samples (Figure 7A) highlight the variability in the direction and magnitude of gene expression among different sample types. These discrepancies can be attributed to the moderate correlation coefficient of the module, indicating that while the genes within the cluster share a common expression pattern, other factors may also influence their expression. The functional annotation of this gene cluster through over-representation analysis (ORA) revealed the involvement of genes in critical cellular processes such as cell cycle, DNA repair and replication, and the P53 signaling pathway (Figure 7B). These findings suggest that the disease stage of tumor samples can be partially explained by the coordinated expression of genes regulating these essential pathways.
Figure 7. Gene co-expression analysis. (A) Eigengene expression patterns of the green-yellow module across samples. (B) ORA comprising the green-yellow gene module and (C) red module. (D) Workflow performed to identify key transcription factors (TFs) that regulate the expression of genes in a given co-expression module. (E) ORA performed with the TFDP1 regulon.
The following module which showed moderate correlation between the genes is the red one (0.44, p=0.01). The trait that defines this gene cluster is the comparison between ROS1+ tumor specimens and normal or healthy lung samples. When carrying out ORA, the steroid biosynthesis, amino sugar and nucleotide sugar metabolism together with the small cell lung cancer KEGG pathways were identified (Figure 7C). Finally, with the aim to unveil master regulatory transcription factors that orchestrate the ROS1+ NSCLC transcriptome, an iRegulon-based analysis upon the red module was performed followed by an ORA annotation (Figure 7D). The top-ranked transcription factor (TF) for the red gene module was TFDP1. Considering only the target genes of the aforementioned TF, ORA was performed (Figure 7E) and it unveiled that DNA replication, homologous recombination, cell cycle, spliceosome and protein processing in ER were the significantly over-represented KEGG pathways in the TFDP1 regulon.
The biology of oncogene-addicted lung neoplasms has been heterogeneously approached within the last decades. Partially due to differences in the prevalence of each molecular subtype, restricting access to patient-derived samples. In consequence, study of ROS1-, ALK- and RET- rearranged tumors remains modest beyond the kinase domain of their oncogenic fusion proteins. To unveil distinctive traits between oncogene-addicted lung adenocarcinomas, particularly between rare subtypes like ROS1+ NSCLC, aspects such as tumor transcriptome profiling might be enlightening. Although existing transcriptome studies lack the necessary depth and specificity required for a comprehensive characterization of ROS1-driven NSCLC, we hypothesized that reanalysis based on combined data can yield a more representative characterization of this tumor type (39–41).
Our findings point towards a relevant contribution of the nucleotide synthesis and cell adhesion pathways to the proliferation of ROS1+ lung adenocarcinomas. The highest overexpressed gene in ROS1+ tumor specimens and cell lines, ISL1 provides new insights about this molecular subtype. This gene encodes a LIM homeodomain transcription factor which regulates insulin expression during homeostasis. Moreover, its pathological role in tumorigenesis has been reported through the regulation of cyclin D1 and c-Myc genes in neuroblastoma, gastric cancer and non-Hodgkin lymphoma (42–44). ISL1 has been recently reported to mediate lung morphogenesis, particularly, the adequate branching of the respiratory epithelium through the Sonic hedgehog (Shh) signaling pathway (45). In addition, ISL1 is known to regulate processes like epithelial cell differentiation, adhesion and migration in NSCLC (46, 47). Importantly, Shh mediates processes like EMT, therefore a dysregulation of ISL1 expression might enhance tumorigenesis via the acquisition of a mesenchymal phenotype of neoplastic cells. Li et al. provided evidence supporting this hypothesis by demonstrating that triptolide-mediated inhibition of the Sonic hedgehog (Shh) pathway could reverse chemoresistance in NSCLC cells (48). Regarding the upregulated cell adhesion signature in ROS1+ NSCLC, the gene encoding Ras interacting protein 1 (RASIP1) was found to be significantly overexpressed. RASIP1 plays a crucial role in the integrin activation process, which is essential for cell migration (49). Therefore, pharmacological inhibition of RASIP1 or its associated pathways might represent a potential strategy to prevent or reduce the development of metastasis in ROS1+ NSCLC.
Additionally, the significantly increased levels of NOTCH1 in RET+ tumors might partially explain the higher rates of extrathoracic metastases in RET+ compared to ROS1+ NSCLC patients. The potential underlying mechanism might be the epithelial-to-mesenchymal transition (EMT)-promoting role of the Notch pathway that enhances cell migration (19, 22, 50). As depicted by two studies in NSCLC, Notch-1 and Notch-3 respectively promote EGFR-TKI resistance and maintain a stem-like status. Remarkably, their pharmacological inhibition decreased cell growth and migration, autophagy and increased the cell apoptotic activity (18, 20, 21). Hence, the Notch pathway constitutes a crucial player regarding the regulation of EMT in NSCLC, becoming a drug target for metastatic disease.
It is known that higher GJB2 expression levels in a subset of cancer-associated fibroblasts (CAFs) induce stromal tumor fibrosis, enhancing chemotherapy resistance in solid tumors (51). These observations were complemented by the significant positive correlation between GJB2 expression and the tumor infiltration by CAFs (52). Specifically in LUAD, the promoter of GJB2 was found to be hypomethylated, possibly leading to gene overexpression. Moreover, the functional annotation of DEGs in GJB2-overexpressing LUAD revealed an enrichment of the PI3K/AKT and ECM-receptor interaction KEGG pathways (53). However, the functional characterization of GJB2 missense variants identified in patients is currently lacking, limiting our ability to draw definitive conclusions regarding their impact on gene function and disease pathogenesis. In parallel, the upregulation of IL20RB in ROS1+ NSCLC tumors and cell lines might constitute one of the mechanisms that mediate bone metastasis. According to a recent study performed in The Netherlands, around 1/3 of stage IV ROS1+ NSCLC patients present bone metastases at diagnosis (54). IL20RB has been described to activate the JAK/STAT pathway upon stimulation with interleukin 19 (IL-19). In consequence, phosphorylated STAT3 translocates to the nucleus of tumor cells promoting the secretion of granulocyte-macrophage colony stimulating factor (GM-CSF), a cytokine that induces IL-19 synthesis in osteoclasts (33). Importantly, IL20RB neutralization with antibodies reduced the metastatic potential of tumor cells in vivo; therefore this becomes a relevant strategy to be evaluated in clinical trials in order to manage bone metastases in advanced stages.
The identification of the enriched systemic lupus erythematosus (SLE) signature in ROS1+ NSCLC might be a priori a puzzling finding. SLE is an autoimmune disease characterized by a dysregulation of cytokines, T cells, B cells and macrophages (55). However, this signature has been previously identified in lung cancer with a higher incidence in the adenocarcinoma histological subtype and non-smoker women (56). Although the causal explanation of this findings is not fully understood, the manifestations of the SLE signature are consistent with the higher risk of thromboembolic events reported in ROS1+ NSCLC patients (57–59). Mechanistically, SLE-induced coagulopathy is caused by autoantibodies which target endothelial cells. The resulting damage triggers the coagulation cascade (60). Therefore, in the context of ROS1+ NSCLC, the expression of a SLE gene signature might be mediated by cell adhesion molecules; another important gene set identified in this study (61, 62). The potential aberrant expression of cell adhesion molecules like integrins and gap junction proteins could initiate the coagulation cascade, explaining the increased susceptibility of thromboembolic events observed in patients.
Importantly, the main limitation of this study is the low sample size of ROS1-, ALK- and RET-rearranged tumors, which is explained by the low prevalence of each tumor subtype. The limited number of tumor specimens and cell lines included in our analysis may affect the generalizability of our findings, necessitating further validation using additional patient-derived samples and experimental models. Moreover, the patients involved in the study received various treatment regimens, which likely impacted gene expression profiles, introducing another potential limitation of our study. However, the identified signatures emerged from analyzing tumor samples and patient-derived cell lines independently collected and sequenced, increasing the robustness of the presented evidence. By employing RT-qPCR, we validated the expression of IL20RB in our ROS1+ cell lines as well as the fusion-specific GJB2 expression pattern. Consequently, we confirm the validity of such findings in independent samples and using an orthogonal method to NGS like RT-qPCR. In addition, complementing the dataset with patient-derived cell lines provides two main advantages. First, the confirmation of the observations in tumor samples, a biologically comprehensive sample type. Second, a detailed assessment of the cell line transcriptomes unveiled converging gene expression patterns with tumor samples, which can be summarized in the enrichment of cell adhesion molecules and nucleotide synthesis signatures. The shared traits offer a new insight about the most representative features of cell lines’ tumor physiology, shown by a dependency on ISL1 expression which links its activity with glucose metabolism and the acquisition of a mesenchymal phenotype.
As seen in our study, differences were noticed between tumor samples and cell lines, reflected by the more than 6000 significantly DEGs. Among them, key oncogenes like MYC and MET were found to be upregulated in cell lines; possibly as a result of the spontaneous immortalization of cell lines. Moreover, the observed cluster of overexpressed genes on chromosome 7, in line with previously reported copy number gains of 7p22.3 in cell lines, must be considered when extrapolating results obtained in vitro. Because this region is rich in oncogenes, differences should be closely regarded when designing experiments involving pathways in which these genes participate (16, 37). It is also worth noting that HCC-78 cells clustered among CUTO lines, indicating that their transcriptomes are very similar despite being cell lines established in different time points. Nevertheless, the phenotypical and transcriptomic variability among ROS1+ patient-derived cell lines supports the heterogeneous ROS1+ NSCLC patient outcomes in the clinical setting, reinforcing them as valuable experimental models.
The mild impact of different ROS1 fusion partner genes contrasts with the work by Neel et al. They demonstrated SLC34A2-ROS1 and SDC4-ROS1 fusions strongly activate the MAPK pathway, which was not seen in CD74-ROS1, due to their differential subcellular localization. The discordance with our results might be explained by three reasons. First, the included data did not include determination of phosphorylated Erk 1/2 protein through immunoblotting, which would have been the most specific method to determine the activation levels of the MAPK pathway. Second, the overlap between the target genes of the MAPK pathway and other ROS1 downstream signaling pathways, such as the JAK/STAT or mTOR/AKT, might mask the differential MAPK pathway activation levels from a transcriptome perspective. Third, Neel et al. also mentioned that a shorter CD74-ROS1 isoform can localize in endosomes and plasma membrane instead of the ER, and as such activate the MAPK pathway as well (25).
Nonetheless, the GJB2 overexpression found in CLTC-ROS1 and CD74-ROS1 subtypes, might have implications in disease progression. First because GJB2, expressed by tumor cells and cancer-associated fibroblasts (CAFs), contributes to ECM remodeling and activates the SPP1/PI3K/AKT signaling pathway in lung adenocarcinoma (24, 63). Second, due to the pro-metastatic role of KRT16, which was found to be upregulated in the same two patient subsets. (Supplementary Figure 6). This cytokeratin upregulates the synthesis of vimentin in lung cancer cells (64). Consequently, this hypothesis is concordant with the increased likelihood of CD74-ROS1+ NSCLC patients to develop brain metastases (65). On top of that, Wang et al. identified that CD74-ROS1+ bone metastatic NSCLC cells secreted CCL5 through STAT3 activation to recruit macrophages. In this interaction, the tumor-promoting M2 macrophages stimulate tumor cells and induce EMT via TGF-β pathway stimulation (66). These results align with the upregulated EMT signature that we report in CD74-ROS1 specimens. Similarly, the downregulation of the TGF-β pathway that we found in tumor specimens can be explained by the lack of interaction between tumor cells and the bone niche in the samples that we analyzed.
Applying gene co-expression analysis in the tumor samples lead to the detection of the cell cycle and amino sugar and nucleotide sugar metabolism pathway over representation within the ROS1+ lung adenocarcinoma subtype. This approach allowed an unsupervised characterization of samples aimed to identify functionally related genes (67). The confirmation of these observations in additional samples, experimental models and independent cohorts might open the door to find new actionable targets in a ROS1-specific manner. In parallel, this approach combined with the iRegulon tool pointed towards TFDP1, a transcription factor known to interact with E2F, another essential regulator whose targets were significantly enriched in CUTO-28 cells. Moreover, TFDP1 amplification has been described in lung cancer and esophageal squamous cell carcinomas (68). It is important to note that an activation of the TFDP1/E2F1 axis in lung cancer results in the attenuation of the p53 pathway, mediated by COMMD9 (69). Thus, this transcription factor represents a novel research object holding therapeutic potential.
Focused efforts concerning ROS1+ NSCLC patients are currently oriented towards refining the treatment scheme upon improved patient stratification, overcoming drug resistance and understanding the disease risk factors (70). Our study contributes to two of these aspects. First, treatment-wise, combination strategies of TKIs and monoclonal antibodies targeting IL20RB might be beneficial to treat bone metastases. Second, further functional studies are required to confirm the role of the nucleotide synthesis pathway in ROS1+ NSCLC, its targeting might enhance the sensitivity towards checkpoint inhibitors as reported by Wu et al. (71) Besides, the inhibition of nucleotide synthesis through mTORC1/IMPDH targeting is known to induce replication stress, ultimately resulting in apoptosis (72). Thus, exploring combinations of TKIs and IMPDH inhibitors could be useful to address heavily pre-treated cases. Third, GJB2 expression could be employed as a prognostic biomarker. Collectively, such observations have the potential to enhance the tailoring of therapies to different patient subsets and help to predict disease outcomes.
In the present study we perform an in-depth characterization of ROS1+ NSCLC using two complementary approaches: differential gene expression and gene co-expression analysis. Our results point towards IL20RB, the nucleotide synthesis and cell adhesion pathways as specific signatures compared to ALK+ and RET+ tumors. Importantly, they constitute targetable alterations which could be co-inhibited together with ROS1. Moreover, we report differences in oncogene expression such as MYC, MET and BRAF between ROS1+ tumor samples and cell lines, which should be taken into account when interpreting in vitro experiments. Finally, we propose ISL1 and TFDP1 as candidate transcription factors that complement the oncogenic dependencies of ROS1+ NSCLC through cyclin D1, c-Myc and the Sonic hedgehog (Shh) pathway. Furthermore, the identification of the enriched systemic lupus erythematosus (SLE) signature might be related to the higher risk of thromboembolic events in ROS1+ NSCLC patients. In addition, GJB2 was found overexpressed in CD74- and CLTC-ROS1+ tumor specimens and cell lines, which positively correlates with patients presenting a poor prognosis. Despite the limited sample size, the robustness of our evidences is supported by the independent validation of IL20RB and GJB2 expression using RT-qPCR. Collectively, the present study broadens our understanding of the molecular alterations in ROS1+ NSCLC, paving the path towards novel therapeutic strategies.
The original contributions presented in the study are included in the article/Supplementary Material. Further inquiries can be directed to the corresponding author.
Ethical approval was not required for the studies on humans in accordance with the local legislation and institutional requirements because only commercially available established cell lines were used. Ethical approval was not required for the studies on animals in accordance with the local legislation and institutional requirements because only commercially available established cell lines were used.
MT: Data curation, Formal analysis, Investigation, Methodology, Writing – original draft. KO: Conceptualization, Funding acquisition, Project administration, Supervision, Writing – review & editing. GV: Conceptualization, Funding acquisition, Project administration, Supervision, Writing – review & editing. GV: Conceptualization, Funding acquisition, Project administration, Supervision, Writing – review & editing. LM: Data curation, Formal analysis, Investigation, Methodology, Resources, Software, Validation, Writing – review & editing.
The author(s) declare financial support was received for the research, authorship, and/or publication of this article. This project was founded by FWO (Research Foundation Flanders) -”Kom op tegen kanker”. Project number: G094820N.
We would like to thank Prof. Dr. Robert C. Doebele and his team for kindly providing us the CUTO cell lines, as part of a research collaboration agreement with the University of Colorado.
The authors declare that the research was conducted in the absence of any commercial or financial relationships that could be construed as a potential conflict of interest.
All claims expressed in this article are solely those of the authors and do not necessarily represent those of their affiliated organizations, or those of the publisher, the editors and the reviewers. Any product that may be evaluated in this article, or claim that may be made by its manufacturer, is not guaranteed or endorsed by the publisher.
The Supplementary Material for this article can be found online at: https://www.frontiersin.org/articles/10.3389/fonc.2024.1408697/full#supplementary-material
1. Drilon A, Jenkins C, Iyer S, Schoenfeld A, Keddy C, Davare MA. ROS1-dependent cancers - biology, diagnostics and therapeutics. Nat Rev Clin Oncol. (2021) 18:35–55. doi: 10.1038/s41571-020-0408-9
2. Parikh DA, Walia G, Freeman-Daily J, Hennink M, Tomalia T, Buonanno L, et al. Characteristics of patients with ROS1+ Cancers: results from the first patient-designed, global, pan-cancer ROS1 data repository. JCO Oncol Pract. (2020) 16:e183–9. doi: 10.1200/JOP.19.00135
3. Russo A, Lopes AR, McCusker MG, Garrigues SG, Ricciardi GR, Arensmeyer KE, et al. New targets in lung cancer (excluding EGFR, ALK, ROS1). Curr Oncol Rep. (2020) 22:48. doi: 10.1007/s11912-020-00909-8
4. Remon J, Pignataro D, Novello S, Passiglia F. Current treatment and future challenges in ROS1- and ALK-rearranged advanced non-small cell lung cancer. Cancer Treat Rev. (2021) 95:102178. doi: 10.1016/j.ctrv.2021.102178
5. Choudhury NJ, Schneider JL, Patil T, Zhu VW, Goldman DA, Yang SR, et al. Response to immune checkpoint inhibition as monotherapy or in combination with chemotherapy in metastatic ROS1-rearranged lung cancers. JTO Clin Res Rep. (2021) 2. doi: 10.1016/j.jtocrr.2021.100187
6. Roys A, Chang X, Liu Y, Xu X, Wu Y, Zuo D. Resistance mechanisms and potent-targeted therapies of ROS1-positive lung cancer. Cancer Chemother Pharmacol. (2019). doi: 10.1007/s00280-019-03902-6
7. Dziadziuszko R, Le AT, Wrona A, Jassem J, Camidge DR, Varella-Garcia M, et al. An activating KIT mutation induces crizotinib resistance in ROS1-positive lung cancer. J Thorac Oncol. (2016) 11:1273–81. doi: 10.1016/j.jtho.2016.04.001
8. Ku BM, Bae YH, Lee KY, Sun J-M, Lee S-H, Ahn JS, et al. Entrectinib resistance mechanisms in ROS1-rearranged non-small cell lung cancer. Invest New Drugs. (2020) 38:360–8. doi: 10.1007/s10637-019-00795-3
9. Lin JJ, Langenbucher A, Gupta P, Yoda S, Fetter IJ, Rooney M, et al. Small cell transformation of ROS1 fusion-positive lung cancer resistant to ROS1 inhibition. NPJ Precis Oncol. (2020) 4:21. doi: 10.1038/s41698-020-0127-9
10. Drilon A, Ou S-HI, Cho BC, Kim D-W, Lee J, Lin JJ, et al. Repotrectinib (TPX-0005) is a next-generation ROS1/TRK/ALK inhibitor that potently inhibits ROS1/TRK/ALK solvent- front mutations. Cancer Discovery. (2018) 8:1227–36. doi: 10.1158/2159-8290.CD-18-0484
11. Drilon A, Horan JC, Tangpeerachaikul A, Besse B, Ou SHI, Gadgeel SM, et al. NVL-520 is a selective, TRK-sparing, and brain-penetrant inhibitor of ROS1 fusions and secondary resistance mutations. Cancer Discovery. (2023) 13:598–615. doi: 10.1158/2159-8290.CD-22-0968
12. Ettinger DS, Wood DE, Aisner DL, Akerley W, Bauman JR, Bharat A, et al. NCCN guidelines insights: non-small cell lung cancer, version 2.2021. J Natl Compr Cancer Network. (2021) 19:254–66. doi: 10.6004/jnccn.2021.0013
13. Park S, Ahn B-C, Lim SW, Sun J-M, Kim HR, Hong MH, et al. Characteristics and outcome of ROS1-positive non-small cell lung cancer patients in routine clinical practice. J Thorac Oncol. (2018) 13:1373–82. doi: 10.1016/j.jtho.2018.05.026
14. Chang X, Liu Z, Man S, Roys A, Li Z, Zuo D, et al. Metastasis manners and the underlying mechanisms of ALK and ROS1 rearrangement lung cancer and current possible therapeutic strategies. RSC Adv. (2019) 9:17921–32. doi: 10.1039/C9RA02258A
15. Drilon A, Lin JJ, Filleron T, Ni A, Milia J, Bergagnini I, et al. Frequency of brain metastases and multikinase inhibitor outcomes in patients with RET–rearranged lung cancers. J Thorac Oncol. (2018) 13:1595–601. doi: 10.1016/j.jtho.2018.07.004
16. Al Bakir M, Huebner A, Martínez-Ruiz C, Grigoriadis K, Watkins TBK, Pich O, et al. The evolution of non-small cell lung cancer metastases in TRACERx. Nature. (2023) 616:534–42. doi: 10.1038/s41586-023-05729-x
17. Du J, Chen W, Yang L, Dai J, Guo J, Wu Y, et al. Disruption of SHH signaling cascade by SBE attenuates lung cancer progression and sensitizes DDP treatment. Sci Rep. (2017) 7. doi: 10.1038/s41598-017-02063-x
18. Xie M, He CS, Wei SH, Zhang L. Notch-1 contributes to epidermal growth factor receptor tyrosine kinase inhibitor acquired resistance in non-small cell lung cancer. Vitro vivo. Eur J Cancer. (2013) 49:3559–72. doi: 10.1016/j.ejca.2013.07.007
19. Galluzzo P, Bocchetta M. Notch signaling in lung cancer. Expert Rev Anticancer Ther. (2011) 11:533–40. doi: 10.1586/era.10.158
20. Ma Y, Li M, Si J, Xiong Y, Lu F, Zhang J, et al. Blockade of Notch3 inhibits the stem-like property and is associated with ALDH1A1 and CD44 via autophagy in non-small lung cancer. Int J Oncol. (2016) 48:2349–58. doi: 10.3892/ijo.2016.3464
21. Mur EB, Bernardo S, Papon L, Mancini M, Fabbrizio E, Goussard M, et al. Notch inhibition overcomes resistance to tyrosine kinase inhibitors in EGFR-driven lung adenocarcinoma. J Clin Invest. (2020) 130:612–24. doi: 10.1172/JCI126896
22. Yuan X, Wu H, Han N, Xu H, Chu Q, Yu S, et al. Notch signaling and EMT in non-small cell lung cancer: Biological significance and therapeutic application. J Hematol Oncol. (2014) 7. doi: 10.1186/s13045-014-0087-z
23. Ji X, Liu Y, Mei F, Li X, Zhang M, Yao B, et al. SPP1 overexpression is associated with poor outcomes in ALK fusion lung cancer patients without receiving targeted therapy. Sci Rep. (2021) 11:14031. doi: 10.1038/s41598-021-93484-2
24. Liu Z, Xiao Z, Wang X, Zhang L, Zhang Z. Ion channel gene GJB2 influences the intercellular communication by Up-regulating the SPP1 signaling pathway identified by the single-cell RNA sequencing in lung adenocarcinoma. Front Oncol. (2023) 13:1146976. doi: 10.3389/fonc.2023.1146976
25. Neel DS, Allegakoen DV, Olivas V, Mayekar MK, Hemmati G, Chatterjee N, et al. Differential subcellular localization regulates oncogenic signaling by ROS1 kinase fusion proteins. Cancer Res. (2019) 79:546–56. doi: 10.1158/0008-5472.CAN-18-1492
26. Love MI, Huber W, Anders S. Moderated estimation of fold change and dispersion for RNA-seq data with DESeq2. Genome Biol. (2014) 15. doi: 10.1186/s13059-014-0550-8
27. Ritchie ME, Phipson B, Wu D, Hu Y, Law CW, Shi W, et al. Limma powers differential expression analyses for RNA-sequencing and microarray studies. Nucleic Acids Res. (2015) 43:e47. doi: 10.1093/nar/gkv007
28. Etoh K, Nakao M. A web-based integrative transcriptome analysis, RNAseqChef, uncovers the cell/tissue type-dependent action of sulforaphane. J Biol Chem. (2023) 299. doi: 10.1016/j.jbc.2023.104810
29. Langfelder P, Horvath S. WGCNA: An R package for weighted correlation network analysis. BMC Bioinf. (2008) 9. doi: 10.1186/1471-2105-9-559
30. Liao Y, Wang J, Jaehnig EJ, Shi Z, Zhang B. WebGestalt 2019: gene set analysis toolkit with revamped UIs and APIs. Nucleic Acids Res. (2019) 47:W199–205. doi: 10.1093/nar/gkz401
31. Kuleshov MV, Jones MR, Rouillard AD, Fernandez NF, Duan Q, Wang Z, et al. Enrichr: a comprehensive gene set enrichment analysis web server 2016 update. Nucleic Acids Res. (2016) 44:W90–7. doi: 10.1093/nar/gkw377
32. Tang Z, Li C, Kang B, Gao G, Li C, Zhang Z. GEPIA: A web server for cancer and normal gene expression profiling and interactive analyses. Nucleic Acids Res. (2017) 45:W98–W102. doi: 10.1093/nar/gkx247
33. He Y, Luo W, Liu Y, Wang Y, Ma C, Wu Q, et al. IL-20RB mediates tumoral response to osteoclastic niches and promotes bone metastasis of lung cancer. J Clin Invest. (2022) 132. doi: 10.1172/JCI157917
34. Jee J, Lebow ES, Yeh R, Das JP, Namakydoust A, Paik PK, et al. Overall survival with circulating tumor DNA-guided therapy in advanced non-small-cell lung cancer. Nat Med. (2022) 28:2353–63. doi: 10.1038/s41591-022-02047-z
35. Negrao MV, Skoulidis F, Montesion M, Schulze K, Bara I, Shen V, et al. Oncogene-specific differences in tumor mutational burden, PD-L1 expression, and outcomes from immunotherapy in non-small cell lung cancer. J Immunother Cancer. (2021) 9. doi: 10.1136/jitc-2021-002891
36. Lee J, Park CK, Yoon H-K, Sa YJ, Woo IS, Kim HR, et al. PD-L1 expression in ROS1-rearranged non-small cell lung cancer: A study using simultaneous genotypic screening of EGFR, ALK, and ROS1. Thorac Cancer. (2019) 10:103–10. doi: 10.1111/1759-7714.12917
37. Coe BP, Lee EHL, Chi B, Girard L, Minna JD, Gazdar AF, et al. Gain of a region on 7p22.3, containing MADILI, is the most frequent event in small-cell lung cancer cell lines. Genes Chromosomes Cancer. (2006) 45:11–9. doi: 10.1002/gcc.20260
38. Posukh OL. Genetic etiology of hearing loss in Russia. Hum Genet. (2022) 141:649–63. doi: 10.1007/s00439-021-02327-7
39. Seo JS, Ju YS, Lee WC, Shin JY, Lee JK, Bleazard T, et al. The transcriptional landscape and mutational profile of lung adenocarcinoma. Genome Res. (2012) 22:2109–19. doi: 10.1101/gr.145144.112
40. Martínez-Ruiz C, Black JRM, Puttick C, Hill MS, Demeulemeester J, Larose Cadieux E, et al. Genomic–transcriptomic evolution in lung cancer and metastasis. Nature. (2023) 616:543–52. doi: 10.1038/s41586-023-05706-4
41. Dhanasekaran SM, Balbin OA, Chen G, Nadal E, Kalyana-Sundaram S, Pan J, et al. Transcriptome meta-analysis of lung cancer reveals recurrent aberrations in NRG1 and Hippo pathway genes. Nat Commun. (2014) 5. doi: 10.1038/ncomms6893
42. Liu Z, Hu W, Qin Y, Sun L, Jing L, Lu M, et al. Isl1 promotes gene transcription through physical interaction with Set1/Mll complexes. Eur J Cell Biol. (2023) 102. doi: 10.1016/j.ejcb.2023.151295
43. Li M, Sun C, Bu X, Que Y, Zhang L, Zhang Y, et al. ISL1 promoted tumorigenesis and EMT via Aurora kinase A-induced activation of PI3K/AKT signaling pathway in neuroblastoma. Cell Death Dis. (2021) 12. doi: 10.1038/s41419-021-03894-3
44. Zhang Q, Yang Z, Jia Z, Liu C, Guo C, Lu H, et al. ISL-1 is overexpressed in non-Hodgkin lymphoma and promotes lymphoma cell proliferation by forming a p-STAT3/p-c-Jun/ISL-1 complex. Mol Cancer. (2014) 13:181. doi: 10.1186/1476-4598-13-181
45. Huang R, Zhang C, Zheng Y, Zhang W, Huang H, Qiu M, et al. ISL1 regulates lung branching morphogenesis via Shh signaling pathway. J Biol Chem. (2023) 299. doi: 10.1016/j.jbc.2023.105034
46. Li H, Yin C, Zhang B, Sun Y, Shi L, Liu N, et al. PTTG1 promotes migration and invasion of human non-small cell lung cancer cells and is modulated by miR-186. Carcinogenesis. (2013) 34:2145–55. doi: 10.1093/carcin/bgt158
47. Wooten DJ, Groves SM, Tyson DR, Liu Q, Lim JS, et al. Systems-level network modeling of Small Cell Lung Cancer subtypes identifies master regulators and destabilizers. 15(10):e1007343 doi: 10.1371/journal.pcbi.1007343
48. Li LB, Yang LX, Liu L, Liu FR, Li AH, Zhu YL, et al. Targeted inhibition of the HNF1A/SHH axis by triptolide overcomes paclitaxel resistance in non-small cell lung cancer. Acta Pharmacol Sin. (2024) 45:1060–76. doi: 10.1038/s41401-023-01219-y
49. Chen Y, Zhang L, Liu L, Sun S, Zhao X, Wang Y, et al. Rasip1 is a RUNX1 target gene and promotes migration of NSCLC cells. Cancer Manag Res. (2018) 10:4537–52. doi: 10.2147/CMAR.S168438
50. Gainor JF, Tseng D, Yoda S, Dagogo-Jack I, Friboulet L, Lin JJ, et al. Patterns of metastatic spread and mechanisms of resistance to crizotinib in ROS1-positive non-small-cell lung cancer. JCO Precis Oncol. (2017) 2017. doi: 10.1200/PO.17.00063
51. Cho SJ, Oh JH, Baek J, Shin Y, Kim W, Ko J, et al. Intercellular cross-talk through lineage-specific gap junction of cancer-associated fibroblasts related to stromal fibrosis and prognosis. Sci Rep. (2023) 13. doi: 10.1038/s41598-023-40957-1
52. Jia Y, Guo B, Zhang W, Wang F, Zhang Y, Zhang Q, et al. Pan-cancer analysis of the prognostic and immunological role of GJB2: a potential target for survival and immunotherapy. Front Oncol. (2023) 13:1110207. doi: 10.3389/fonc.2023.1110207
53. Lu A, Shi Y, Liu Y, Lin J, Zhang H, Guo Y, et al. Integrative analyses identified ion channel genes GJB2 and SCNN1B as prognostic biomarkers and therapeutic targets for lung adenocarcinoma. Lung Cancer. (2021) 158:29–39. doi: 10.1016/j.lungcan.2021.06.001
54. ten Berge DMHJ, Damhuis RAM, Aerts JGJV, Dingemans AMC. Real-world treatment patterns and survival of patients with ROS1 rearranged stage IV non-squamous NSCLC in the Netherlands. Lung Cancer. (2023) 181. doi: 10.1016/j.lungcan.2023.107253
55. Kaul A, Gordon C, Crow MK, Touma Z, Urowitz MB, Van Vollenhoven R, et al. Systemic lupus erythematosus. Nat Rev Dis Primers. (2016) 2. doi: 10.1038/nrdp.2016.39
56. Rosenberger A, Sohns M, Friedrichs S, Hung RJ, Fehringer G, McLaughlin J, et al. Gene-set meta-analysis of lung cancer identifies pathway related to systemic lupus erythematosus. PloS One. (2017) 12. doi: 10.1371/journal.pone.0173339
57. Muñoz-Unceta N, Zugazagoitia J, Manzano A, Jiménez-Aguilar E, Olmedo ME, Cacho JD, et al. High risk of thrombosis in patients with advanced lung cancer harboring rearrangements in ROS1. Eur J Cancer. (2020) 141:193–8. doi: 10.1016/j.ejca.2020.10.002
58. Beninato T, Lo Russo G, Garassino MC, De Braud F, Platania M. Recurrent thrombosis followed by Lazarus response in ROS1 rearranged NSCLC treated with crizotinib: a case report. Tumori. (2020) 106(6):300891620905665. doi: 10.1177/0300891620905665
59. Nichetti F, Russo G, Prelaj A, Provenzano L, de Braud F, Cabiddu M, et al. ALK/ROS1 rearrangements: A real hallmark for thromboembolic events in cancer patients? Thromb Res. (2020) 194:176–7. doi: 10.1016/j.thromres.2020.06.041
60. Moschetti L, Piantoni S, Vizzardi E, Sciatti E, Riccardi M, Franceschini F, et al. Endothelial dysfunction in systemic lupus erythematosus and systemic sclerosis: A common trigger for different microvascular diseases. Front Med (Lausanne). (2022) 9:849086. doi: 10.3389/fmed.2022.849086
61. Nieswandt B, Varga-Szabo D, Elvers M. Integrins in platelet activation. J Thromb Haemostasis. (2009) 7:206–9. doi: 10.1111/j.1538-7836.2009.03370.x
62. Vaiyapuri S, Jones CI, Sasikumar P, Moraes LA, Munger SJ, Wright JR, et al. Gap junctions and connexin hemichannels underpin hemostasis and thrombosis. Circulation. (2012) 125:2479–91. doi: 10.1161/CIRCULATIONAHA.112.101246
63. Tang Y, Zhang YJ, Wu ZH. High GJB2 mRNA expression and its prognostic significance in lung adenocarcinoma: A study based on the TCGA database. Med (United States). (2020) 99. doi: 10.1097/MD.0000000000019054
64. Wang W, Zhu L, Zhou J, Liu X, Xiao M, Chen N, et al. Targeting the KRT16-vimentin axis for metastasis in lung cancer. Pharmacol Res. (2023) 193. doi: 10.1016/j.phrs.2023.106818
65. Li Z, Shen L, Ding D, Huang J, Zhang J, Chen Z, et al. Efficacy of crizotinib among different types of ROS1 fusion partners in patients with ROS1-rearranged non–small cell lung cancer. J Thorac Oncol. (2018) 13:987–95. doi: 10.1016/j.jtho.2018.04.016
66. Wang Z, Lei Z, Wang Y, Wang S, Wang J-P, Jin E, et al. Bone-metastatic lung adenocarcinoma cells bearing CD74-ROS1 fusion interact with macrophages to promote their dissemination. Oncogene. (2024) 43:2215–27. doi: 10.1038/s41388-024-03072-7
67. van Dam S, Võsa U, van der Graaf A, Franke L, de Magalhães JP. Gene co-expression analysis for functional classification and gene-disease predictions. Brief Bioinform. (2018) 19:575–92. doi: 10.1093/bib/bbw139
68. Zhan W, Wang W, Han T, Xie C, Zhang T, Gan M, et al. COMMD9 promotes TFDP1/E2F1 transcriptional activity via interaction with TFDP1 in non-small cell lung cancer. Cell Signal. (2017) 30:59–66. doi: 10.1016/j.cellsig.2016.11.016
69. Tang M, Burgess JT, Fisher M, Boucher D, Bolderson E, Gandhi NS, et al. Targeting the COMMD4–H2B protein complex in lung cancer. Br J Cancer. (2023) 129:2014–24. doi: 10.1038/s41416-023-02476-8
70. Boulanger MC, Schneider JL, Lin JJ. Advances and future directions in ROS1 fusion-positive lung cancer. Oncologist. (2024). doi: 10.1093/oncolo/oyae205
71. Wu Hl, Gong Y, Ji P, Xie Yf, Jiang YZ, Liu G. Targeting nucleotide metabolism: a promising approach to enhance cancer immunotherapy. J Hematol Oncol. (2022) 15. doi: 10.1186/s13045-022-01263-x
Keywords: ROS1+ NSCLC, RNA-sequencing, gene co-expression, nucleotide synthesis, cell adhesion, prognostic biomarker
Citation: Terrones M, Op de Beeck K, Van Camp G, Vandeweyer G and Mateiu L (2024) Transcriptomic analysis of ROS1+ non-small cell lung cancer reveals an upregulation of nucleotide synthesis and cell adhesion pathways. Front. Oncol. 14:1408697. doi: 10.3389/fonc.2024.1408697
Received: 28 March 2024; Accepted: 29 November 2024;
Published: 16 December 2024.
Edited by:
Dalila Luciola Zanette, Oswaldo Cruz Foundation (Fiocruz), BrazilCopyright © 2024 Terrones, Op de Beeck, Van Camp, Vandeweyer and Mateiu. This is an open-access article distributed under the terms of the Creative Commons Attribution License (CC BY). The use, distribution or reproduction in other forums is permitted, provided the original author(s) and the copyright owner(s) are credited and that the original publication in this journal is cited, in accordance with accepted academic practice. No use, distribution or reproduction is permitted which does not comply with these terms.
*Correspondence: Geert Vandeweyer, Z2VlcnQudmFuZGV3ZXllckB1emEuYmU=
Disclaimer: All claims expressed in this article are solely those of the authors and do not necessarily represent those of their affiliated organizations, or those of the publisher, the editors and the reviewers. Any product that may be evaluated in this article or claim that may be made by its manufacturer is not guaranteed or endorsed by the publisher.
Research integrity at Frontiers
Learn more about the work of our research integrity team to safeguard the quality of each article we publish.