- 1Department of Radiology, Affiliated Hospital of North Sichuan Medical College, Nanchong, China
- 2MRI Research Institute, Huaxi MR Research Center (HMRRC), Chengdu, Sichuan, China
- 3Department of Radiology, Affiliated Hospital of North Sichuan Medical College, Nanchong, Sichuan, China
Background and purpose: Differentiating high-grade gliomas (HGGs) from solitary brain metastases (SBMs) using conventional magnetic resonance imaging (MRI) remains challenging due to their similar imaging features. This study aimed to evaluate the diagnostic performance of advanced diffusion models, such as neurite orientation dispersion and density imaging (NODDI) and mean apparent propagator magnetic resonance imaging (MAP-MRI), incomparison to traditional techniques like diffusion-weighted imaging (DWI), diffusion tensor imaging (DTI), and diffusion kurtosis imaging (DKI) for distinguishing HGGs from SBMs.
Methods: In total, 17 patients with HGGs and 26 patients with SBMs were prospectively recruited based on the established inclusion and exclusion criteria. Structural MRI sequences and diffusion spectrum imaging (DSI) were utilized to assess quantitative parameter models, including NODDI, MAP-MRI, DWI, DTI, and DKI. Quantitative parameters were measured for both the tumor parenchymal area and the peritumoral edema area. The quantitative parameters of the two patient groups were compared using either the independent Student’s t-test or the Mann–Whitney U test. The effectiveness of each model was evaluated using receiver operating characteristic (ROC) curves and calculating the area under the curve (AUC). Finally, the DeLong test was employed to compare the diagnostic performance of each model through pairwise comparisons of ROC curves.
Results: Isotropic volume fraction (Viso) based on NODDI; mean squared displacement (MSD) and the return to plane probabilities (RTPP) based on MAP-MRI; radial diffusivity (RDk) and mean diffusivity (MDk) based on DKI; and axial diffusivity (AD), radial diffusivity (RD), and mean diffusivity (MD) based on DTI of the peritumoral edema tumor were significantly different between HGGs and SBMs (p < 0.05). The optimal single discriminant parameters for each model are NODDI_Viso, MAP-MRI_MSD, DKI_MDk, and DTI_AD. Among these, the AUC of Viso (0.809) exceeds that of MSD (0.733), MDk (0.718), and AD (0.779). The combined model, which incorporates DTI_AD, DKI_RD, and NODDI_Viso, demonstrated superior diagnostic performance (0.897).
Conclusions: Advanced diffusion MRI quantitative parameters derived from NODDI, such as Viso, have the potential to enhance the differentiation between HGGs and SBMs. The integrated utilization of these models is anticipated to enhance diagnostic accuracy and refine MRI protocols for brain tumor assessment.
Introduction
Gliomas, the most malignant subtype of neuroepithelial tumors, represent the predominant incidence of primary brain tumors (1). According to the latest Fifth edition of the World Health Organization (WHO) classification of central nervous system (CNS) tumors, gliomas are stratified into four grades (2). High-grade gliomas (HGGs), encompassing WHO grades III and IV, entail a grim prognosis, with a 5-year survival rate ranging from 25.9% to 49.4% for grade III and a mere 4.7% for grade IV (3). Brain metastases are prevalent malignant neoplasms in adults (4), occurring 10 times more frequently than primary brain malignancies (5). In certain instances, patients presenting multiple cerebral lesions with a history of primary malignancy may undergo a straightforward diagnosis of brain metastases. Nonetheless, solitary brain metastases (SBMs) manifest initially in nearly 30% of patients with systemic malignancies (6), potentially serving as the inaugural symptom of undiagnosed extracranial malignant tumors. Additionally, gliomas may manifest in patients with systemic cancer. Given the differences in medical staging, clinical management, and prognosis between HGGs and SBMs, accurately distinguishing these two types of malignant tumors is of great value for clinical decision-making.
Currently, magnetic resonance imaging (MRI) stands as the premier noninvasive modality for diagnosing intracranial tumors. Accurately distinguishing between HGGs and SBMs poses a challenge when patients present with isolated and markedly heterogeneously enhanced brain lesions, as both commonly display akin imaging features and enhancement patterns—including cysts, necrosis, circular enhancement, and peritumoral edema—on conventional MRI. This often leads to misdiagnoses in over 40% of cases (7). In such scenarios, while postoperative histopathological biopsy remains the gold standard for differentiation, it is associated with certain limitations, including surgical complications or infeasibility due to patients’ poor physical condition, tumor invasiveness, or proximity to critical brain regions. Consequently, resorting to noninvasive techniques becomes imperative for distinguishing between HGGs and SBMs (8, 9).
Many previous studies have explored various methodologies to tackle this issue, including MR perfusion-weighted imaging (PWI) (10–12), magnetic resonance spectroscopy (MRS) (10, 12), diffusion-weighted imaging (DWI) (13, 14), and diffusion tensor imaging (DTI) (10, 15). Notably, among these techniques, the DWI-based apparent diffusion coefficient (ADC) and DTI-based fractional anisotropy (FA) values are the most widely utilized metrics. However, there are controversial results regarding the ability of ADC and FA to distinguish between HGGs and SBMs.
In recent years, traditional MRI technology has increasingly struggled to meet the practical needs of clinical work. However, innovative diffusion MRI techniques, such as neurite orientation dispersion and density imaging (NODDI) (16) and the diffusion spectrum imaging (DSI)-based mean apparent propagator (MAP)-MRI (17), have demonstrated enhanced sensitivity in detecting changes in the microstructure of brain tissue. Promising results have been obtained using NODDI and MAP-MRI in multiple sclerosis (18), glioma grading (19), and Alzheimer’s disease (20). However, it remains uncertain whether these techniques outperform the more commonly used non-Gaussian-based diffusion kurtosis imaging (DKI) and Gaussian diffusion models such as DTI and DWI in differentiation between HGGs and SBMs. Therefore, this study aims to evaluate the effectiveness of NODDI, MAP-MRI, DKI, DTI, and DWI in distinguishing HGGs from SBMs.
Materials and methods
Study design and participants
This study adhered to the principles outlined in the Helsinki Declaration and received approval from the ethics review committee of our institution. All participants provided written informed consent prior to undergoing MRI examinations. From December 2022 to November 2023, we recruited 76 patients suspected of having brain tumors based on MRI findings or other clinical assessments. The inclusion criteria were as follows: (1) patients with isolated brain lesions exhibiting significant, uneven enhancement on structural MRI; (2) patients diagnosed with HGGs or SBMs via postoperative pathological biopsy or stereotactic biopsy, in accordance with the 2016 WHO classification criteria for brain tumors; and (3) some SBM patients who were not subjected to surgical or pathological biopsy were clinically confirmed through follow-up (confirmation criteria included: histopathologically confirmed malignant tumors elsewhere in the body, with or without metastasis, and a significant reduction in intracranial lesions following radiotherapy and chemotherapy). The exclusion criteria were as follows: (1) patients with other brain tumors confirmed via histopathology that were not HGGs or SBMs; (2) insufficient peritumoral edema for further analysis; (3) any pretreatment of brain lesions prior to the MRI examination; and (4) poor MRI image quality due to motion artifacts. Ultimately, our study included 43 patients (27 men and 16 women, with an average age of 57.64 years and an age range of 17– 84 years). The selection process for research subjects is depicted in Figure 1.
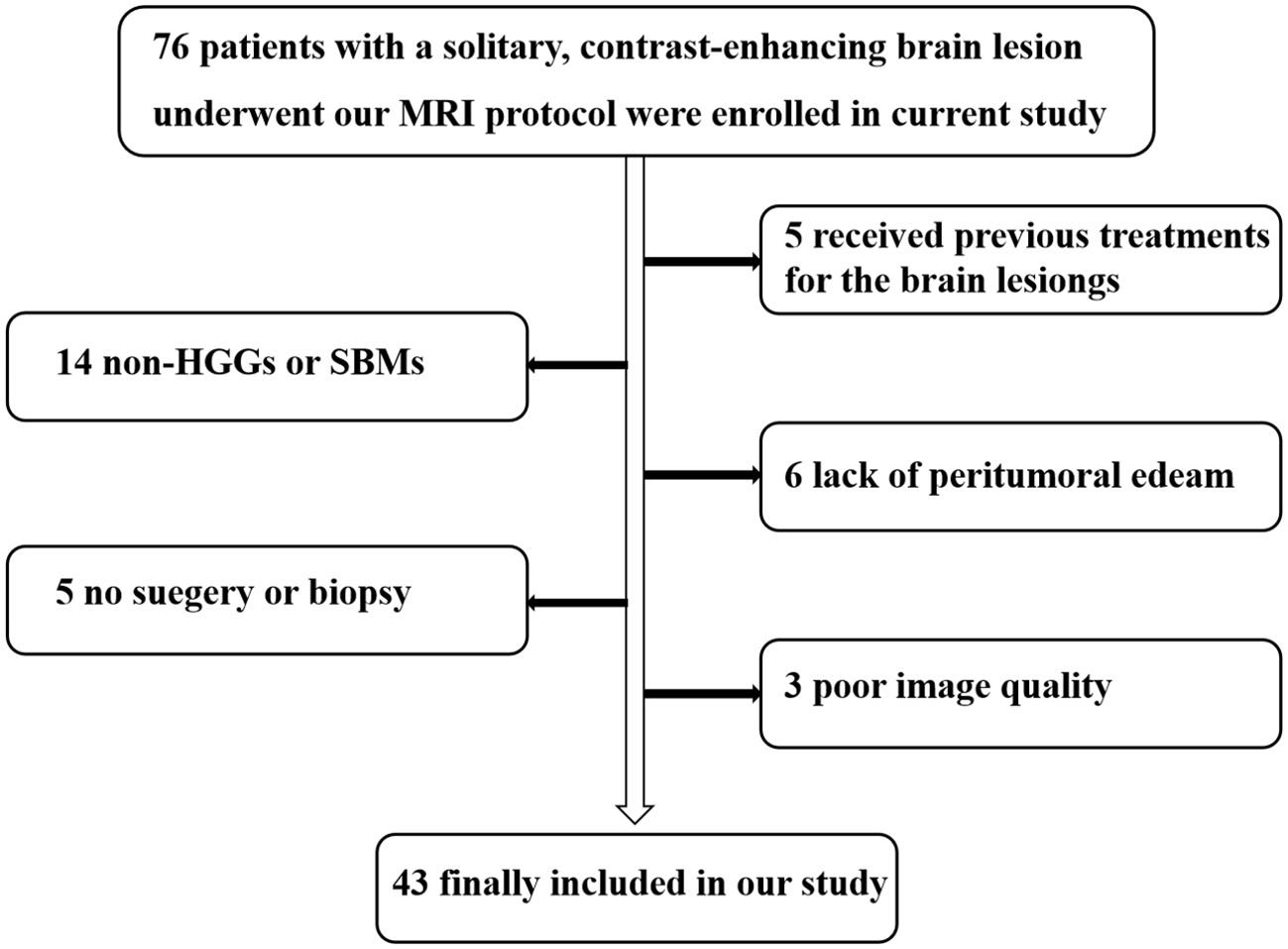
Figure 1. Flowchart illustrating the selection of the study population. HGGs, high-grade gliomas; SBMs, solitary brain metastases.
MRI protocols
In this study, all participants underwent MRI using a 3-T scanner (MAGNETOM Skyra, Siemens Healthineers, Erlangen, Germany) with a 32-channel head coil. Patients were positioned in a standard supine orientation for structural and diffusion-weighted MRI scans. The structural MRI protocol comprised axial T1-weighted images (T1WI), axial T2-weighted images (T2WI), axial DWI images, and axial contrast-enhanced 3D T1WI. The diffusion-weighted imaging protocol involved axial DSI conducted prior to the injection of the contrast agent, capturing 99 diffusion directions and 11 b-values (specifically, 0 s/mm2, 350 s/mm2, 650 s/mm2, 950 s/mm2, 1,000 s/mm2, 1,350 s/mm2, 1,650 s/mm2, 1,700 s/mm2, 2,000 s/mm2, 2,700 s/mm2, and 3,000 s/mm2) over a duration of 12 min. Table 1 provides details on the specific parameters for each MRI sequence. The imaging coverage was designed to encompass the entire brain comprehensively, maintaining consistency across all axial sequences. The orientation of the scanning plane was aligned parallel to the anterior commissure–posterior commissure (AC-PC) line.
Reconstruction and segmentation
All DSI data, initially stored in DICOM format, were converted to NIfTI format utilizing the DCM2NII tool. Subsequently, diffusion parameter models of DTI, DKI, MAP-MRI, and NODDI were reconstructed using in-house postprocessing software (NeuDiLab), which is based on the Diffusion Imaging in Python (DIPY) framework (https://dipy.org). The Elastix software (http://Elastix.isi.uu.nl/) was used to register all diffusion parameter models with axial contrast-enhanced T1W images, ensuring alignment within the same imaging space. For the registration of diffusion images, baseline data with contrast-enhanced T1W images were used. The quantitative analysis was conducted by a radiologist with 3 years of experience in neuroradiology, under the mentorship of a senior radiologist with 10 years of clinical neuroradiology experience in MRI, for the delineation of the regions of interest (ROIs Both radiologists were blind to the histological findings. Discrepancies were resolved through consensus. The ITK-SNAP (www.itk-snap.org) was used for the manual delineation of ROIs on contrast-enhanced T1WI and T2WI images. In identifying the ROIs, the area of significant enhancement within the maximum cross-sectional area of the tumor parenchyma was marked as the contrast-enhancing tumor ROIs, while the high-signal area on T2WI without enhancement was designated as the peritumoral edema ROIs. Regions suspected of containing necrotic, calcified, or cystic components were manually excluded. Ultimately, the delineated ROIs were transferred to the corresponding registered diffusion parameter model diagrams for the same patient using ITK-SNAP software, facilitating the calculation of average quantitative parameters for both the contrast-enhancing tumor area and peritumoral edema areas.
Statistical analysis
The diffusion parameters of contrast-enhancing tumors and peritumoral edema were quantified. The Shapiro–Wilk test and the Levene F-test were used to assess normality and homogeneity of variance across all diffusion metrics. To evaluate differences in individual diffusion parameters, either an independent Student’s t-test or the Mann–Whitney U test was utilized. Furthermore, quantitative indicators showing significant statistical differences between HGGs and SBMs were integrated and analyzed using multiple logistic regression to develop a combined model. The performance of each parameter and the combined models was evaluated using the receiver operating characteristic (ROC) and the corresponding area under the ROC curve (AUC). Statistical metrics, including the calculation of the best threshold, specificity, sensitivity, AUC, and the 95% confidence interval (CI), were conducted using SPSS software (version 26.0, IBM, Armonk, NY, USA) to determine their efficacy in distinguishing between HGGs and SBMs. Pairwise comparisons of the ROC curves were also executed using the DeLong test. A two-tailed p < 0.05 was considered statistically significant.
Results
Characteristics of the study participants
Among the 43 enrolled patients, 17 (10 men and seven women; mean age, 58.11 years; age, 17–84 years) were confirmed to have HGGs, while 26 (17 men and nine women; mean age, 57.07 years; age, 26–73 years) were diagnosed with SBM based on histopathological examination or clinical follow-up. The primary sites of SBMs included 15 patients with lung carcinoma, three with breast cancer, two with ovarian cancer, one with esophageal cancer, one with gastric cancer, one with colon cancer, one with renal cancer, one with nasopharyngeal carcinoma, and one with atrial lymphoma.
Comparison of diffusion parameters between HGGs and SBMs groups
The mappings of diffusion parameters, including DWI_ADC, DTI_AD, DTI_RD, DTI_MD, DTI_FA, DKI_AK, DKI_RK, DKI_MK, DKI_AD, DKI_RD, DKI_MD, DKI_FA, MAP_NG, MAP_NGax, MAP_NGrad, MAP_MSD, MAP_QIV, MAP_RTOP, MAP_RTAP, MAP_RTPP, NODDI_Vic, NODDI_Viso, and NODDI_ODI, from one patient with HGG and one with SBM are shown in Table 2. As shown in Table 2, DTI_AD, DTI_RD, DTI_MD, DKI_RD, DKI_MD, MAP_MSD, and NODDI_Viso of the peritumoral edema were significantly lower in the HGGs compared to SBMs (p = 0.004, p = 0.030, p = 0.026, p = 0.044, p = 0.026, p = 0.017, and p = 0.001, respectively). In contrast, MAP_RTPP of the peritumoral edema was significantly higher in the HGGs than in the SBMs (p = 0.039). No obvious differences were found among all other diffusion parameters in the contrast-enhancing tumors or peritumoral edema between the two groups (p > 0.05). Figure 2 shows the violin diagram of the significant diffusion parameters in peritumoral edema.
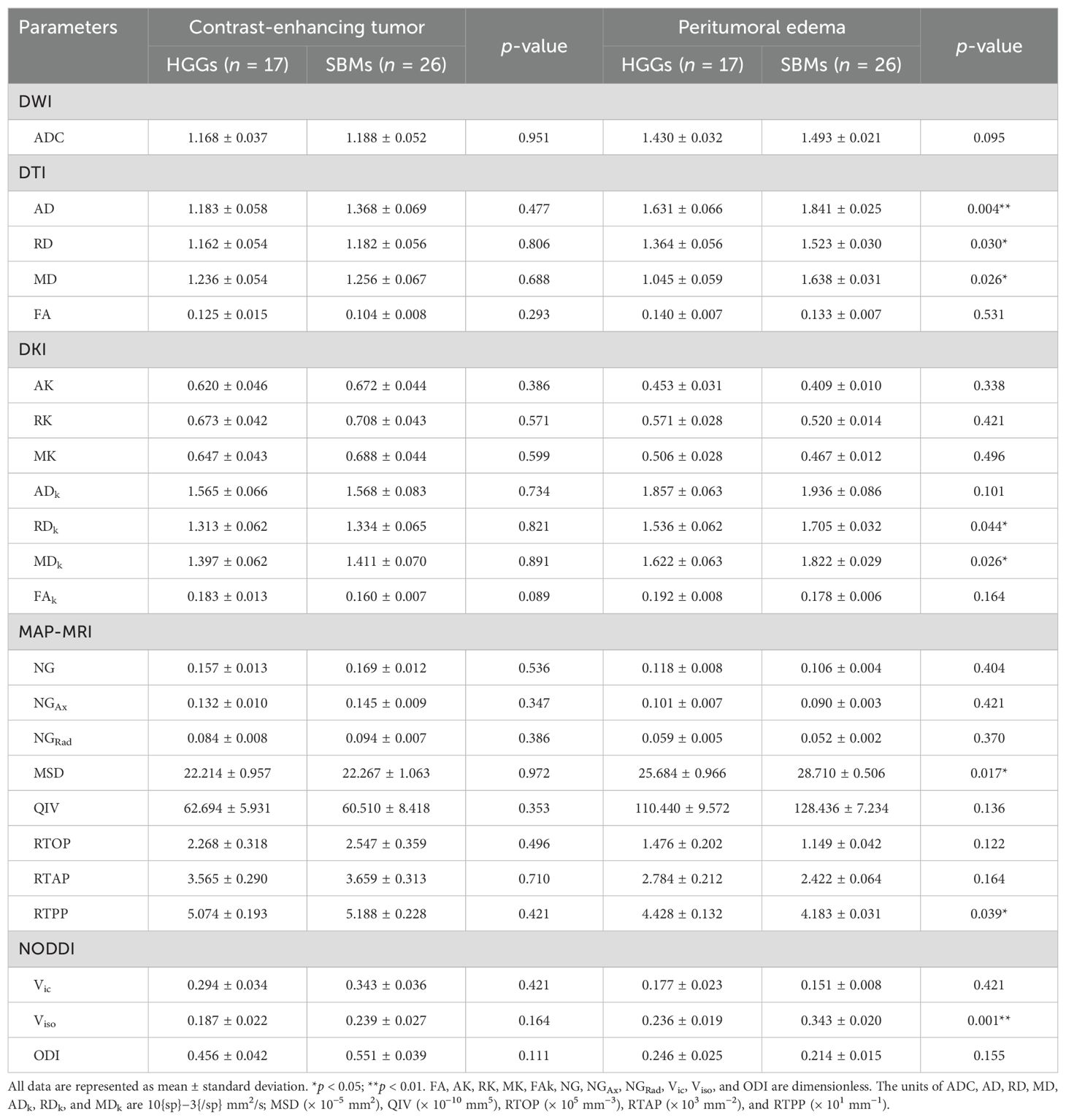
Table 2. All diffusion parameters in the contrast-enhancing tumor or peritumoral edema between HGGs and SBMs.
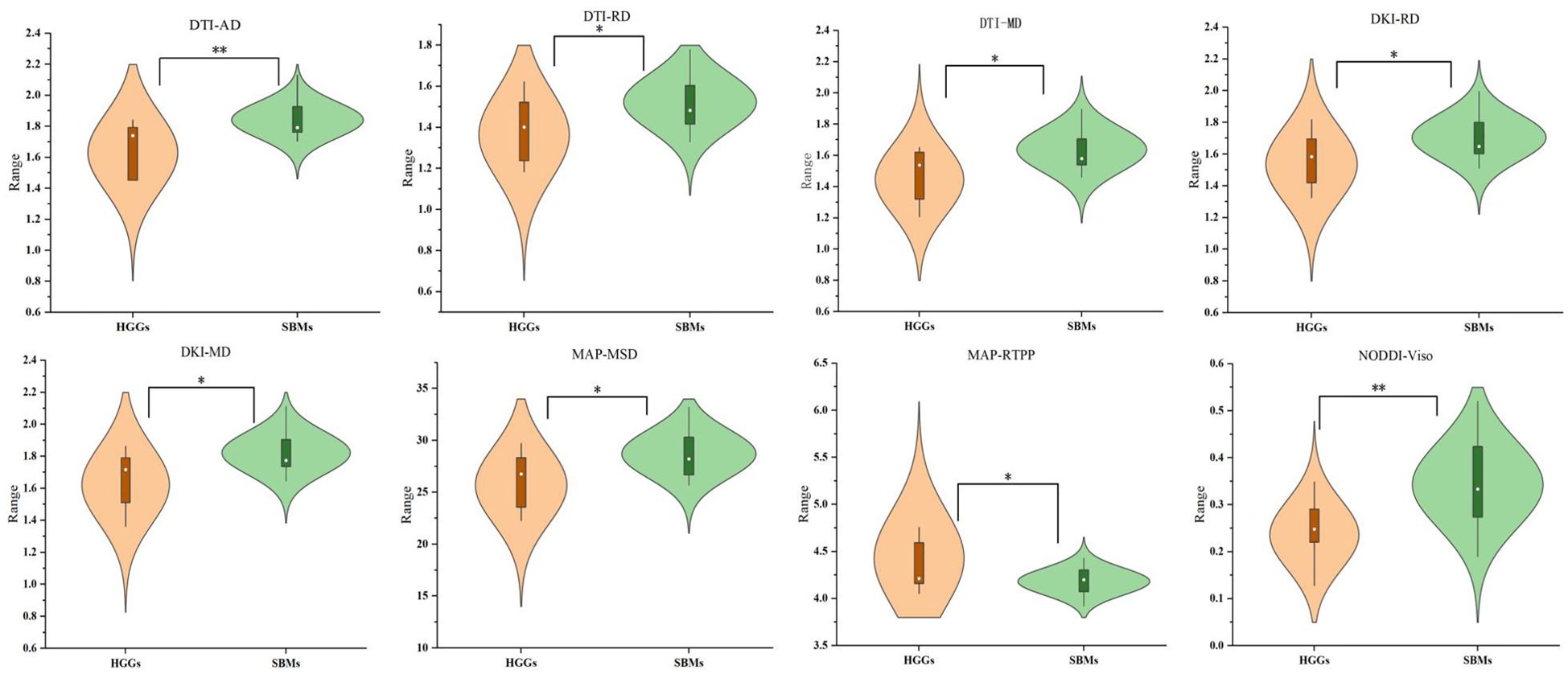
Figure 2. Violin diagram of significant diffusion parameters in peritumoral edema. *p < 0.05; **p < 0.01.
Distinguishing performance of diffusion parameters between HGGs and SBMs groups
ROC curve analyses of the significant diffusion parameters of the peritumoral edema are shown in Table 3 and Figure 3. The best individual metrics for DTI, DKI, MAP-MRI, and NODDI were DTI_AD, DKI_MD, MAP_MSD, and NODDI_Viso, respectively (AUC = 0.779, 0.718, 0.733, and 0.809, respectively). Among these metrics, NODDI_Viso had the highest AUC of 0.809, achieving 93.3% sensitivity and 59.9% specificity, with a 95% CI of 0.671 to 0.947, and the best cut-off value of 0.302 for distinguishing HGGs. The DeLong test was used for pairwise comparison of the ROC curves, revealing no significant differences between the parameters (all p > 0.05). When combined to create a multiple logistic regression analysis model, the combined model of DTI_AD, DKI_RD, and NODDI_Viso showed better diagnostic efficacy (AUC: 0.897, 95% CI: 0.799 to 0.995, sensitivity: 86.7%, specificity: 77.3%) compared to any single parameter models, although this difference was not statistically significant. The typical cases of HGGs and SBMs are shown in Figures 4, 5.
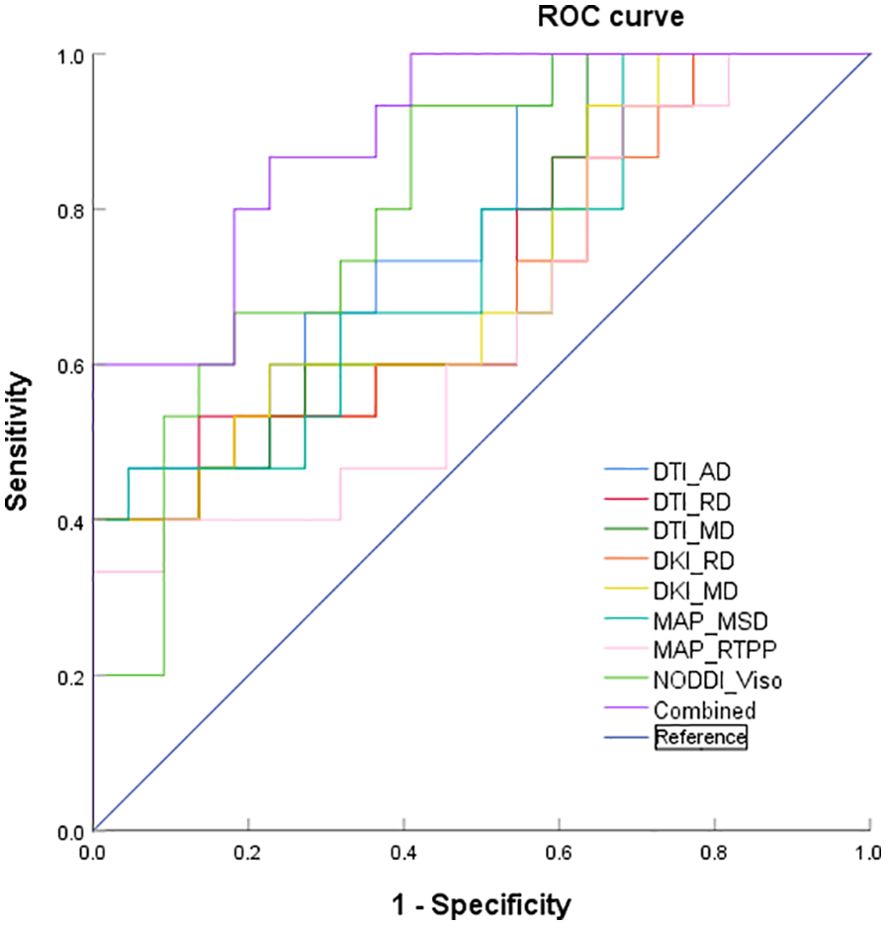
Figure 3. ROC curves of DTI_AD, DTI_RD, DTI_MD, DKI_RD, DKI_MD, MAP_MSD, MAP_RTPP, NODDI_Viso, and the combined model of the peritumoral edema.
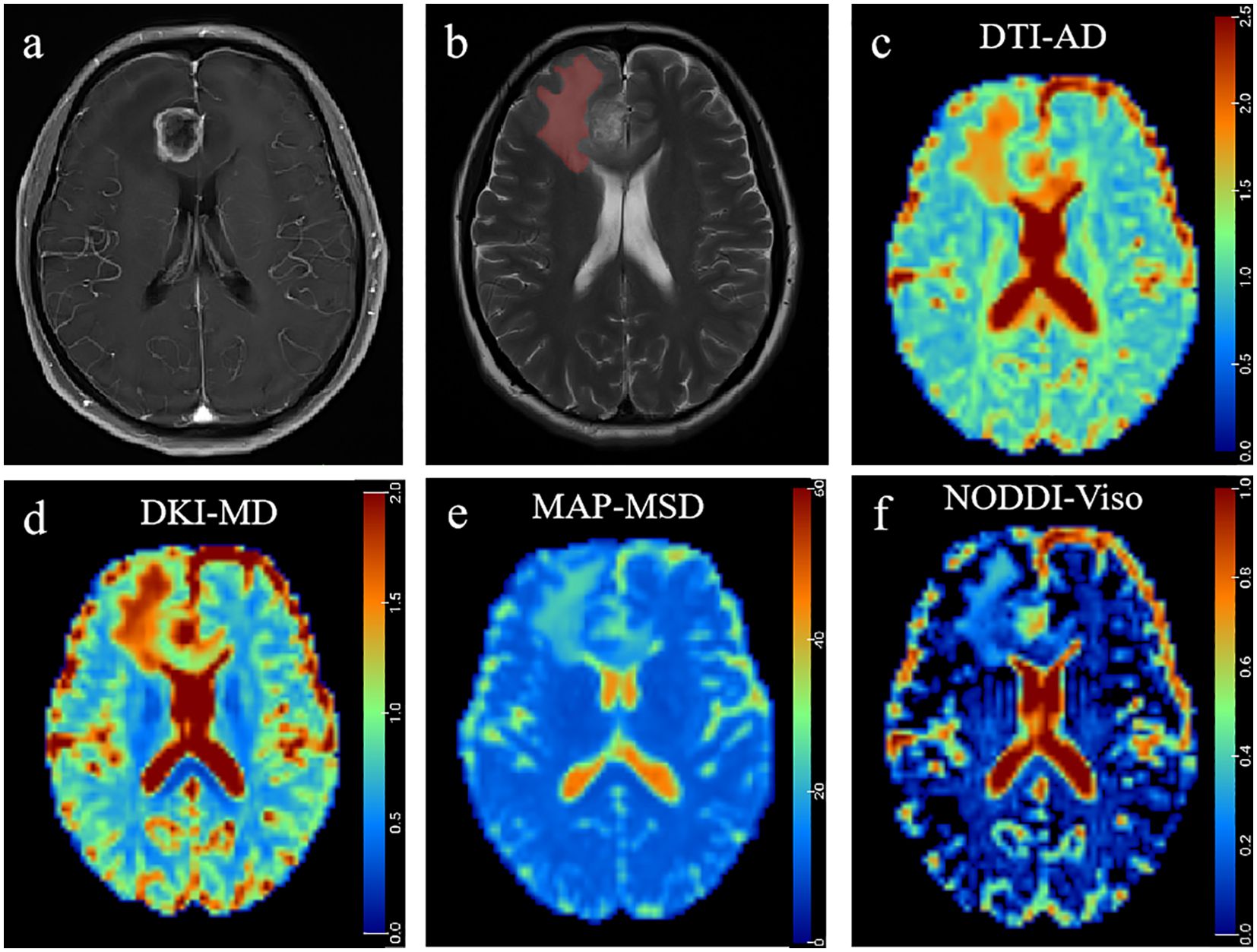
Figure 4. A 41-year-old man histopathologically confirmed to have isolated brain metastases from lung carcinoma. (A) Postcontrast T1W images and (B) T2W images represent an isolated contrast-enhancing tumor with peritumoral edema in the right frontal lobe. The red area represents the ROI of peritumoral edema. Pseudocolorful maps show the lesion AD (C), MDk (D), MSD (E), and Viso (F) having a slight increase compared to the contralateral normal white matter.
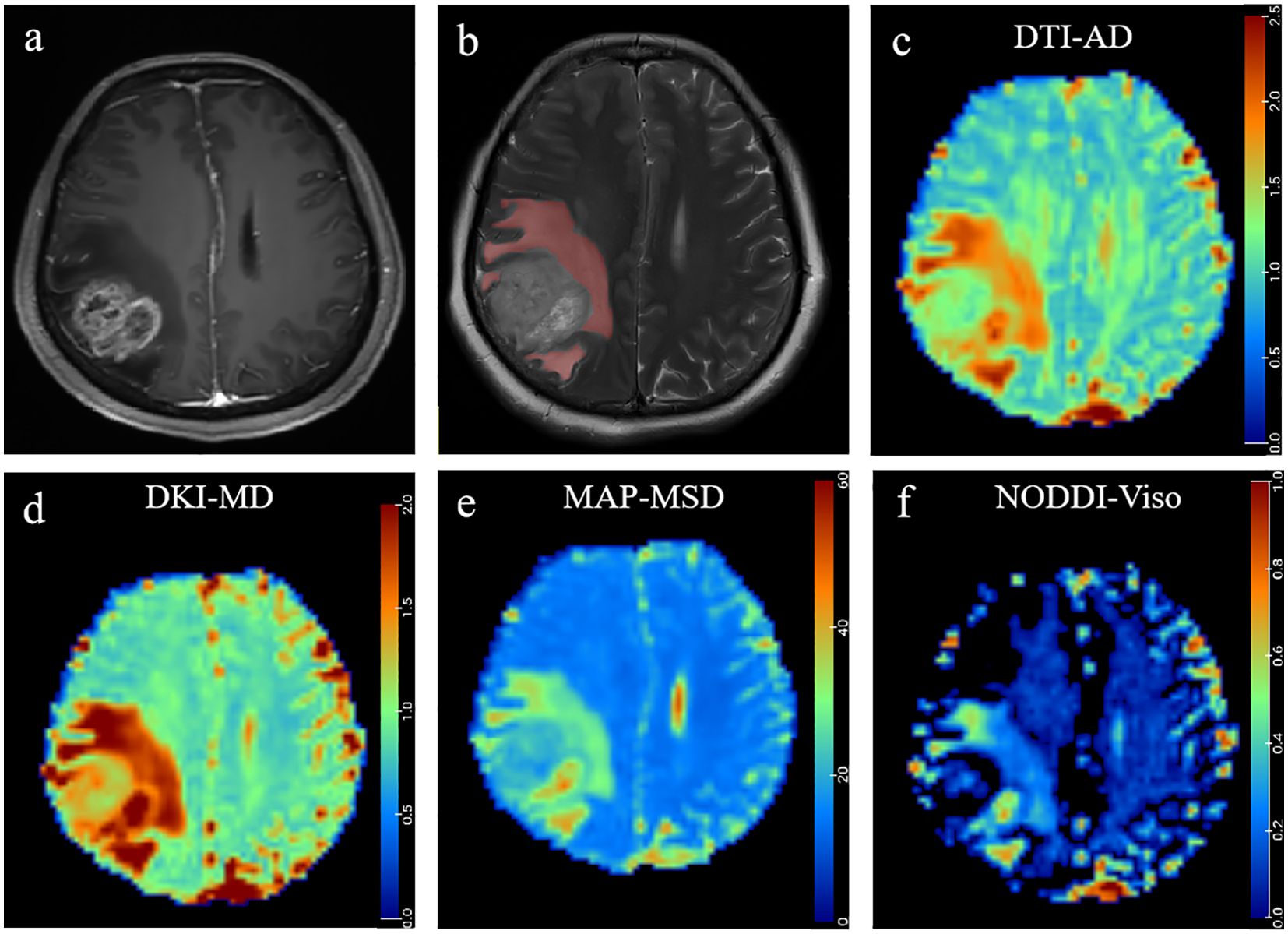
Figure 5. A 48-year-old woman histopathologically confirmed to have glioblastoma (WHO grade IV). (A) Postcontrast T1W images and (B) T2W images represent contrast-enhancing tumors with peritumoral edema in the right parietal occipital lobe. The red area represents the ROI of peritumoral edema. Pseudocolorful maps show the lesion AD (C), MDk (D), MSD (E), and Viso (F) having a slight increase compared to the contralateral normal white matter.
Discussion
This study used a 12-min diffusion sequence to investigate the ability of NODDI, MAP, DKI, DTI, and DWI to distinguish between GBM and SBM. All parameters of NODDI, MAP, DKI, DTI, and DWI were reconstructed using specialized postprocessing tools. Our results confirmed that HGGs and SBMs showed distinctive NODDI, MAP-MRI, DKI, and DTI-based diffusion parameters in the peritumoral edema region, while no differences were observed for any of the diffusion parameters in the contrast-enhancing tumor region. NODDI-based peritumoral Viso is useful for distinguishing GBM and SBM, and the combination of DTI_AD, DKI_RD, and NODDI_Viso further enhances classification accuracy.
Patients diagnosed with HGG or SBM exhibit divergent treatment pathways and survival rates, underscoring the critical importance of noninvasive and accurate differential diagnoses (21). Relying solely on conventional MRI techniques to differentiate between these malignancies poses considerable difficulties (7). Recent advancements in diffusion-weighted MRI technology have significantly enhanced our capability to detect microstructural alterations within brain tissue. Traditional Gaussian probability distribution models inadequately capture the intricate microanatomy of complex brain tissues. In contrast, non-Gaussian diffusion models, such as NODDI, MAP-MRI, and DKI offer a more accurate representation of water molecule dispersion, thereby more effectively reflecting the heterogeneity and complexity of the tissue microenvironment (22).
NODDI is an increasingly popular DWI technique with significant potential for clinical studies (23), as it provides a three-compartment microstructure model (intracellular, extracellular, and cerebrospinal fluid (CSF)) for each voxel. This approach can simultaneously describe the microstructural characteristics of dendrites and axons in both gray and white matter, providing more specific neuronal change data than standard DTI analysis (16). In our study, we demonstrated that NODDI-based Viso probably outperformed other non-Gaussian or Gaussian diffusion metrics in differentiating between HGGs and SBMs. Although no single diffusion parameter can completely capture the complexity of neural tissue, our results suggest that Viso may serve as a sensitive imaging biomarker in neurooncology research and warrants further investigation (8).
NODDI-Viso represents isotropic Gaussian diffusion within the tissue. Caverzasi et al. (24) applied the NODDI model to evaluate various brain lesions. Unlike tumor-infiltrated edema, they found that qualitative results of NODDI color images can be used to distinguish vascular edema (e.g., brain metastases, lymphoma, and toxoplasmosis), which is characterized by a significant increase in Viso. Their findings are consistent with our results. Yoshihito et al. (25) applied the NODDI model to distinguish glioblastoma from brain metastases and found that the Viso of the peritumoral edema was significantly lower in glioblastoma than in brain metastases. They suggested that the increased signal on the Viso map is related to vascular edema. This result can be explained by the degradation of the extracellular matrix by acetyl heparanase and matrix metalloproteinases, which allows metastatic brain tumors to grow into the brain parenchyma in a dilated and noninfiltrating manner, resulting in a high degree of isotropy (26). Our data support their discovery and align with the hypotheses regarding vascular edema and invasive edema (27).
MAP-MRI is a novel magnetic resonance diffusion model based on DSI that more accurately reflects changes in the structure of white matter fiber bundles in the brain. Our study found that HGGs showed lower mean-squared displacement (MSD) and higher return-to-plane probabilities (RTPP) values than SBMs. MSD is a second-order displacement indicator of molecular diffusion distance and is more sensitive than the mean diffusivity (MD) in DTI (28). RTPP represents the probability of water molecules returning to the radial direction of the main diffusion direction (29). This phenomenon may occur because GBM tumor cells produce a large amount of specific extracellular matrix, mainly tenascin (30), which accumulates in the extracellular matrix and acts as a component of cell adhesion and migration (31). As a result, water molecules are more likely to return to their starting positions, leading to more restricted diffusion.
For DTI, we found that SBMs showed higher peritumoral axial diffusivity (AD), radial diffusivity (RD), and MD values than SBMs. MD reveals the rate of diffusion motion of water molecules, while AD and RD reflect the diffusion rates of water vertical and parallel scalars along the white matter tracts, respectively (32). MD, AD, and RD are all negatively correlated with the number of tumor cells (33, 34), which helps explain why metastatic lesions often form simple vascular edema around the tumor, resulting in lower cell density. As an extension of DTI, DKI-based RD and MD in our study showed similar change patterns with DTI-based RD and MD.
Prior research has used various diffusion metrics in efforts to distinguish tumor parenchymal areas between HGGs and SBMs; however, the findings remain controversial (8, 25, 35, 36). In the current study, despite utilizing five different diffusion models, no significant differences were observed in the diffusion parameters among the contrast-enhancing tumors of HGG and SBM. These results imply that diffusion metrics may not effectively discern the heterogeneity inherent to these distinct types of malignant tumors.
Our study encountered several limitations. Firstly, it was constrained by a relatively small sample size. Secondly, the majority of SBMs analyzed originated from lung cancer, suggesting potential deviations in results when assessing SBMs from other primary sites. Thirdly, the analysis was limited to mean diffusion metrics within ROIs, and it was noted that the tumor volume in HGG patients exceeded that in SBM patients. Lastly, this research did not incorporate other functional MR techniques, such as perfusion imaging and MR spectroscopy, which are essential for comprehensive comparative studies.
Conclusions
Advanced diffusion MRI quantitative parameters derived from NODDI, such as Viso, have the potential to enhance the capability to differentiate between HGGs and SBMs. The integrated utilization of these models is anticipated to enhance diagnostic accuracy and refine MRI protocols for brain tumor assessment.
Data availability statement
The original contributions presented in the study are included in the article/supplementary material. Further inquiries can be directed to the corresponding author.
Ethics statement
The studies involving humans were approved by Medical Ethics Committee of Chuanbei Medical College Affiliated Hospital. The studies were conducted in accordance with the local legislation and institutional requirements. The participants provided their written informed consent to participate in this study. Written informed consent was obtained from the individual(s), and minor(s)’ legal guardian/next of kin, for the publication of any potentially identifiable images or data included in this article.
Author contributions
LH: Data curation, Investigation, Methodology, Software, Writing – original draft, Writing – review & editing. MC: Data curation, Software, Writing – review & editing. HL: Investigation, Software, Writing – review & editing. XS: Data curation, Writing – original draft. ZQ: Data curation, Writing – original draft. XX: Data curation, Investigation, Software, Writing – original draft, Writing – review & editing.
Funding
The author(s) declare that no financial support was received for the research, authorship, and/or publication of this article.
Conflict of interest
The authors declare that the research was conducted in the absence of any commercial or financial relationships that could be construed as a potential conflict of interest.
Publisher’s note
All claims expressed in this article are solely those of the authors and do not necessarily represent those of their affiliated organizations, or those of the publisher, the editors and the reviewers. Any product that may be evaluated in this article, or claim that may be made by its manufacturer, is not guaranteed or endorsed by the publisher.
References
1. Giaquinto AN, Miller KD, Tossas KY, Winn RA, Jemal A, Siegel RL. Cancer statistics for african american/black people 2022. CA Cancer J Clin. (2022) 72:202–29. doi: 10.3322/caac.21718
2. Louis DN, Perry A, Wesseling P, Brat DJ, Cree IA, Figarella-Branger D, et al. The 2021 WHO classification of tumors of the central nervous system: a summary. Neuro Oncol. (2021) 23:1231–51. doi: 10.1093/neuonc/noab106
3. Omuro A, DeAngelis LM. Glioblastoma and other Malignant gliomas: a clinical review. JAMA. (2013) 310:1842–50. doi: 10.1001/jama.2013.280319
4. Louis DN, Perry A, Reifenberger G, von Deimling A, Figarella-Branger D, Cavenee WK, et al. The 2016 world health organization classification of tumors of the central nervous system: a summary. Acta Neuropathol. (2016) 131:803–20. doi: 10.1007/s00401-016-1545-1
5. Lapointe S, Perry A, Butowski NA. Primary brain tumours in adults. Lancet. (2018) 392:432–46. doi: 10.1016/S0140-6736(18)30990-5
6. Schiff D. Single brain metastasis. Curr Treat Options Neurol. (2001) 3:89–99. doi: 10.1007/s11940-001-0027-4
7. Blanchet L, Krooshof PW, Postma GJ, Idema AJ, Goraj B, Heerschap A, et al. Discrimination between metastasis and glioblastoma multiforme based on morphometric analysis of MR images. AJNR Am J Neuroradiol. (2011) 32:67–73. doi: 10.3174/ajnr.A2269
8. Mao J, Zeng W, Zhang Q, Yang Z, Yan X, Zhang H, et al. Differentiation between high-grade gliomas and solitary brain metastases: a comparison of five diffusion-weighted MRI models. BMC Med Imaging. (2020) 20:124. doi: 10.1186/s12880-020-00524-w
9. Jackson RJ, Fuller GN, Abi-Said D, Lang FF, Gokaslan ZL, Shi WM, et al. Limitations of stereotactic biopsy in the initial management of gliomas. Neuro Oncol. (2001) 3:193–200. doi: 10.1093/neuonc/3.3.193
10. Martin-Noguerol T, Mohan S, Santos-Armentia E, Cabrera-Zubizarreta A, Luna A. Advanced MRI assessment of non-enhancing peritumoral signal abnormality in brain lesions. Eur J Radiol. (2021) 143:109900. doi: 10.1016/j.ejrad.2021.109900
11. Scola E, Desideri I, Bianchi A, Gadda D, Busto G, Fiorenza A, et al. Assessment of brain tumors by magnetic resonance dynamic susceptibility contrast perfusion-weighted imaging and computed tomography perfusion: a comparison study. Radiol Med. (2022) 127:664–72. doi: 10.1007/s11547-022-01470-z
12. Sparacia G, Gadde JA, Iaia A, Sparacia B, Midiri M. Usefulness of quantitative peritumoural perfusion and proton spectroscopic magnetic resonance imaging evaluation in differentiating brain gliomas from solitary brain metastases. Neuroradiol J. (2016) 29:160–7. doi: 10.1177/1971400916638358
13. Caravan I, Ciortea CA, Contis A, Lebovici A. Diagnostic value of apparent diffusion coefficient in differentiating between high-grade gliomas and brain metastases. Acta Radiol. (2018) 59:599–605. doi: 10.1177/0284185117727787
14. Aslan K, Gunbey HP, Tomak L, Incesu L. Multiparametric MRI in differentiating solitary brain metastasis from high-grade glioma: diagnostic value of the combined use of diffusion-weighted imaging, dynamic susceptibility contrast imaging, and magnetic resonance spectroscopy parameters. Neurol Neurochir Pol. (2019) 53:227–37. doi: 10.5603/PJNNS.a2019.0024
15. Tan Y, Wang XC, Zhang H, Wang J, Qin JB, Wu XF, et al. Differentiation of high-grade-astrocytomas from solitary-brain-metastases: Comparing diffusion kurtosis imaging and diffusion tensor imaging. Eur J Radiol. (2015) 84:2618–24. doi: 10.1016/j.ejrad.2015.10.007
16. Zhang H, Schneider T, Wheeler-Kingshott CA, Alexander DC. NODDI: practical in vivo neurite orientation dispersion and density imaging of the human brain. Neuroimage. (2012) 61:1000–16. doi: 10.1016/j.neuroimage.2012.03.072
17. Ozarslan E, Koay CG, Shepherd TM, Komlosh ME, İrfanoğlu MO, Pierpaoli C, et al. Mean apparent propagator (MAP) MRI: a novel diffusion imaging method for mapping tissue microstructure. Neuroimage. (2013) 78:16–32. doi: 10.1016/j.neuroimage.2013.04.016
18. Wood H. NODDI reveals brain microstructural changes in multiple sclerosis. Nat Rev Neurol. (2022) 18:1. doi: 10.1038/s41582-021-00600-x
19. Sun Y, Su C, Deng K, Hu X, Xue Y, Jiang R. Mean apparent propagator-MRI in evaluation of glioma grade, cellular proliferation, and IDH-1 gene mutation status. Eur Radiol. (2022) 32:3744–54. doi: 10.1007/s00330-021-08522-4
20. Moody JF, Dean DR 3rd, Kecskemeti SR, Blennow K, Zetterberg H, Kollmorgen G, et al. Associations between diffusion MRI microstructure and cerebrospinal fluid markers of Alzheimer’s disease pathology and neurodegeneration along the Alzheimer’s disease continuum. Alzheimers Dement (Amst). (2022) 14:e12381. doi: 10.1002/dad2.12381
21. Singh K, Saxena S, Khosla AA, McDermott MW, Kotecha RR, Ahluwalia MS. Update on the management of brain metastasis. Neurotherapeutics. (2022) 19:1772–81. doi: 10.1007/s13311-022-01312-w
22. Jensen JH, Helpern JA. MRI quantification of non-Gaussian water diffusion by kurtosis analysis. NMR BioMed. (2010) 23:698–710. doi: 10.1002/nbm.v23:7
23. Kamiya K, Hori M, Aoki S. NODDI in clinical research. J Neurosci Methods. (2020) 346:108908. doi: 10.1016/j.jneumeth.2020.108908
24. Caverzasi E, Papinutto N, Castellano A, Zhu AH, Scifo P, Riva M, et al. Neurite orientation dispersion and density imaging color maps to characterize brain diffusion in neurologic disorders. J Neuroimaging. (2016) 26:494–8. doi: 10.1111/jon.2016.26.issue-5
25. Kadota Y, Hirai T, Azuma M, Hattori Y, Khant ZA, Hori M, et al. Differentiation between glioblastoma and solitary brain metastasis using neurite orientation dispersion and density imaging. J Neuroradiol. (2020) 47:197–202. doi: 10.1016/j.neurad.2018.10.005
26. Preusser M, Capper D, Ilhan-Mutlu A, Berghoff AS, Birner P, Bartsch R, et al. Brain metastases: pathobiology and emerging targeted therapies. Acta Neuropathol. (2012) 123:205–22. doi: 10.1007/s00401-011-0933-9
27. Fordham AJ, Hacherl CC, Patel N, Jones K, Myers B, Abraham M, et al. Differentiating glioblastomas from solitary brain metastases: an update on the current literature of advanced imaging modalities. Cancers (Basel). (2021) 13(12):2960. doi: 10.3390/cancers13122960
28. Ning L, Westin CF, Rathi Y. Estimating diffusion propagator and its moments using directional radial basis functions. IEEE Trans Med Imaging. (2015) 34:2058–78. doi: 10.1109/TMI.42
29. Fick R, Wassermann D, Caruyer E, Deriche R. MAPL: Tissue microstructure estimation using Laplacian-regularized MAP-MRI and its application to HCP data. Neuroimage. (2016) 134:365–85. doi: 10.1016/j.neuroimage.2016.03.046
30. Zamecnik J. The extracellular space and matrix of gliomas. Acta Neuropathol. (2005) 110:435–42. doi: 10.1007/s00401-005-1078-5
31. Pope WB, Mirsadraei L, Lai A, Eskin A, Qiao J, Kim HJ, et al. Differential gene expression in glioblastoma defined by ADC histogram analysis: relationship to extracellular matrix molecules and survival. AJNR Am J Neuroradiol. (2012) 33:1059–64. doi: 10.3174/ajnr.A2917
32. Kodiweera C, Alexander AL, Harezlak J, McAllister TW, Wu YC. Age effects and sex differences in human brain white matter of young to middle-aged adults: A DTI, NODDI, and q-space study. Neuroimage. (2016) 128:180–92. doi: 10.1016/j.neuroimage.2015.12.033
33. Yuan W, Holland SK, Jones BV, Crone K, Mangano FT. Characterization of abnormal diffusion properties of supratentorial brain tumors: a preliminary diffusion tensor imaging study. J Neurosurg Pediatr. (2008) 1:263–9. doi: 10.3171/PED/2008/1/4/263
34. Marini C, Iacconi C, Giannelli M, Cilotti A, Moretti M, Bartolozzi C. Quantitative diffusion-weighted MR imaging in the differential diagnosis of breast lesion. Eur Radiol. (2007) 17:2646–55. doi: 10.1007/s00330-007-0621-2
35. Gao E, Gao A, Kit KW, Shi L, Bai J, Zhao G, et al. Histogram analysis based on diffusion kurtosis imaging: Differentiating glioblastoma multiforme from single brain metastasis and comparing the diagnostic performance of two region of interest placements. Eur J Radiol. (2022) 147:110104. doi: 10.1016/j.ejrad.2021.110104
Keywords: glioma, brain metastasis, magnetic resonance imaging, neurite orientation dispersion and density imaging (NODDI), mean apparent propagator magnetic resonance imaging (MAP-MRI), diffusion magnetic resonance imaging (dMRI)
Citation: He L, Chen M, Li H, Shi X, Qiu Z and Xu X (2024) Differentiation between high-grade gliomas and solitary brain metastases based on multidiffusion MRI model quantitative analysis. Front. Oncol. 14:1401748. doi: 10.3389/fonc.2024.1401748
Received: 15 March 2024; Accepted: 23 September 2024;
Published: 14 October 2024.
Edited by:
Alfredo Conti, University of Bologna, ItalyCopyright © 2024 He, Chen, Li, Shi, Qiu and Xu. This is an open-access article distributed under the terms of the Creative Commons Attribution License (CC BY). The use, distribution or reproduction in other forums is permitted, provided the original author(s) and the copyright owner(s) are credited and that the original publication in this journal is cited, in accordance with accepted academic practice. No use, distribution or reproduction is permitted which does not comply with these terms.
*Correspondence: Xiaoxue Xu, bmNsaXR0bGVzbm93bmNAMTYzLmNvbQ==