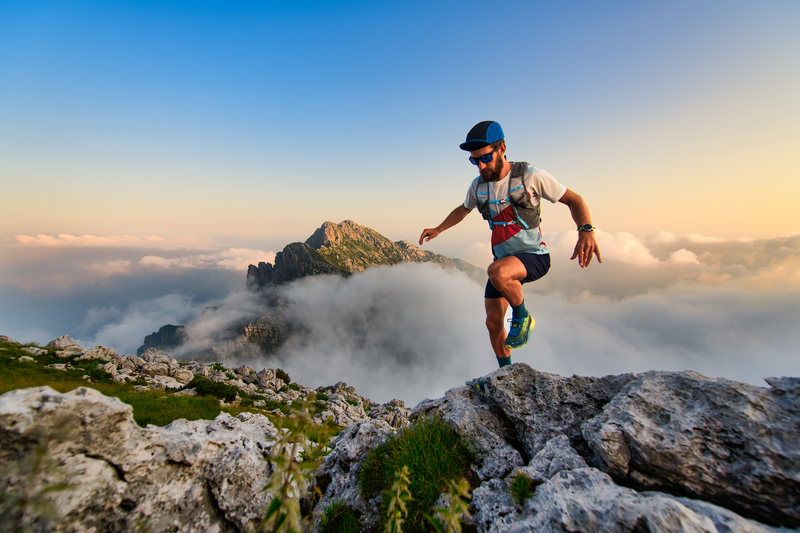
95% of researchers rate our articles as excellent or good
Learn more about the work of our research integrity team to safeguard the quality of each article we publish.
Find out more
REVIEW article
Front. Oncol. , 08 July 2024
Sec. Breast Cancer
Volume 14 - 2024 | https://doi.org/10.3389/fonc.2024.1400498
This article is part of the Research Topic Insights in Raman Microscopy for Bio-imaging Applications View all articles
Breast cancer is the most commonly diagnosed cancer in women globally and a leading cause of cancer-related mortality. However, current detection methods, such as X-rays, ultrasound, CT scans, MRI, and mammography, have their limitations. Recently, with the advancements in precision medicine and technologies like artificial intelligence, liquid biopsy, specifically utilizing Surface-Enhanced Raman Spectroscopy (SERS), has emerged as a promising approach to detect breast cancer. Liquid biopsy, as a minimally invasive technique, can provide a temporal reflection of breast cancer occurrence and progression, along with a spatial representation of overall tumor information. SERS has been extensively employed for biomarker detection, owing to its numerous advantages such as high sensitivity, minimal sample requirements, strong multi-detection ability, and controllable background interference. This paper presents a comprehensive review of the latest research on the application of SERS in the detection of breast cancer biomarkers, including exosomes, circulating tumor cells (CTCs), miRNA, proteins and others. The aim of this review is to provide valuable insights into the potential of SERS technology for early breast cancer diagnosis.
In 2022, There will be an estimated 357200 new cases of breast cancer in China, resulting in 75000 deaths (1). Breast cancer is classified into four major molecular subtypes based on immunohistochemical classification: luminal A and luminal B (expressing the estrogen receptor (ER)), basal-like and human epidermal growth factor receptor 2 (HER2)-enriched (without ER expression) (2, 3). The choice of clinical treatment and prognosis is closely related to breast cancer subtypes, highlighting the importance of early detection. Patients with early-stage breast cancer have a survival rate of 98%, while late-stage patients’ survival drops to 27% (4). Triple-negative breast cancer is highly invasive and metastatic, associated with a poor prognosis and high recurrence rates (5), whereas the Luminal A/B subtype is suitable for hormone therapy because of endoplasmic reticulum enrichment (6). Breast cancer cells exhibit heterogeneity in molecular and morphological characteristics. However, conventional imaging methods such as mammography, ultrasound, mammography, CT, and MRI have limitations. Mammography, the most widely used method, has a sensitivity ranging from 36% to 98%, with significant false positive and negative results. Furthermore, despite advancements in imaging technology, its application is limited by unnecessary radiation exposure and biopsies (7, 8). Invasive tissue biopsy, the current gold standard for breast cancer diagnosis, fails to capture sufficient information about the tumor’s overall characteristics and is not suitable for dynamic monitoring of cancer development and treatment efficacy (9, 10). Consequently, new diagnostic technologies are urgently needed to overcome these limitations. The latest advancements in less invasive liquid biopsy, driven by new technologies, are leading the way toward precision medicine. Components of liquid biopsy include CTCs, microRNA, extracellular vesicle (EV), proteins, and metabolites secreted by tumor cells (11). As a minimally invasive approach, liquid biopsy is highly valued for its ability to reflect the overall heterogeneity of systemic tumor burden, monitor disease progression, and evaluate treatment efficacy in real-time (12).
SERS is a versatile analytical technique that provides rich molecular information, high sensitivity, narrow spectral bandwidth, strong multi-detection capability, and minimal interference from water (13, 14). Consequently, it holds great promise as a detection tool for disease diagnosis by analyzing body fluids. Notably, SERS has shown practicality in breast cancer diagnosis, as evidenced by recent studies. The mechanisms underlying SERS can be broadly classified into two categories: electromagnetic enhancement (15) and chemical enhancement (16). In summary, the performance of SERS biosensors relies on various components, including the substrate, reporter molecule, stabilizer molecule, conjugated material, and fabrication method. Numerous studies have been conducted on the SERS detection of breast cancer biomarkers, aiming to enhance the sensitivity, specificity, and repeatability of SERS. These improvements have been achieved through strategies such as enhancing substrate affinity, employing labels for detection, and targeting substrates with oligonucleotides.
Exosomes (EXO), ranging in size from 30-150nm, are extracellular vesicles that have been extensively studied for their involvement in various aspects of cancer (17, 18). including initiation, metastasis, pre-metastatic niche preparation, angiogenesis, immunity, and drug resistance (19). These vesicles carry specific biological and genetic information from their parent cells, selectively enriching certain nucleic acids, proteins, and lipids, thereby providing a comprehensive compositional profile related to cancer pathogenesis and status (20). Notably, characteristic miRNAs found in the nucleic acid cargo of exosomes have been associated with breast cancer invasiveness (21), angiogenesis (22), metastasis (23), and drug resistance (24). Moreover, the expression of exosomal proteins, such as PD-L1, has been closely linked to cancer occurrence, development, and prognosis (25, 26). The stability of exosomes, attributed to their lipid bilayer structure, has attracted attention as a potential biomarker (26–28). However, the heterogeneity of exosomes poses challenges in isolating them from complex biological samples and conducting accurate analyses. Currently, there are two broad categories of techniques for detecting breast cancer exosomes: targeting molecules on the exosome surface using methods such as protein imprinting, enzyme-linked immunosorbent assay (ELISA), polymerase chain reaction (PCR), and various optical approaches; and comprehensive analysis of exosomes, which involves complex instruments and datasets, making clinical application difficult.
In the subsequent sections, we will delve into the most recent studies that employ Surface-Enhanced Raman Spectroscopy (SERS) in conjunction with other approaches to identify exosomes. This integration presents a hopeful avenue for diagnosing breast cancer in its early stages, albeit with the caveat that further enhancements are necessary.
Substrates play a crucial role in capturing biomolecules, and their capacity varies depending on the substrate. Noble metals such as gold and silver are commonly used in SERS for the detection of breast cancer exosomes due to their ability to enhance sensitivity through local surface plasmon resonance (LSPR) (29). In addition, various other properties of the substrate, such as the size, shape, number and uniformity of nanoparticles (NP) or metals, strongly influence the function of SERS (30). The exposure of a metal surface to a laser beam scatters conduction electrons into frequency electrons, also known as plasmons, that generate an additional electric field corresponding to the electrons of the laser. In nanostructures, these electrons oscillate all over the surface and, thus, are commonly defined as localized surface plasmons, thereby increasing the intensity of the local electromagnetic field, a phenomenon known as localized SPR(LSPR). Given that LSPR depends on the NP size, smaller NPs display poor polarization and result in loss of LSPR properties. Spherical NPs have lower efficiency because of the uniform spread of electric field density (hotspots). Changing the shape from spherical to cubical NPs improves SERS efficiency, mainly because of the focused non-uniform field close to the sharp ends (31). Importantly, more hotspots are created with NP aggregation compared with single NPs (32). In a study by Ferreira et al. (33), low-cost and environmentally friendly SERS substrates were developed. Specifically, in-situ synthesis of silver nanoparticles was performed, and these particles were anchored on bacterial cellulose (BC) membranes. The use of nata de coco as a source for BC film production offers a cost-effective and simple alternative to traditional methods. When combined with Principal Component Analysis (PCA), this approach can effectively distinguish exosomes from the MCF-10A and MDA-MB-231 cell lines, demonstrating improved peak differentiation, reduced background noise, and enhanced detectability of analytes due to minimal interference from cellulose peaks. Furthermore, advancements in nanofiber alignment achieved through nano-structuring techniques have enhanced the reproducibility of SERS signals when compared to ordinary cellulose.
Various methods facilitate interactions between exosomes and nanoparticles, including physical anchoring to solid carriers or chemical reaction mediation in a suspension. However, these simple methods can often result in irregular arrangements, leading to inconsistent SERS vibrational spectra. To address this issue, advanced nanofabrication techniques have been employed to improve physical anchoring. Additionally, advancements in nanomachining technology have allowed for precise customization of SERS substrates. Pramanik et al. (34) have reported a heterogeneous SERS platform that employed plasmonic gold nano-stars attached to two-dimensional graphene oxide, resulting in significant Raman enhancement. This platform successfully generated nano-sized “hotspots” through plasmon-exciton coupling, thereby greatly improving Raman efficiency (Table 1).
Table 1 Detection Limits (LOD) and Dynamic Ranges of Different Methods for Detecting Breast Cancer Exosomes.
The detection of large biomolecules, such as proteins and nucleic acids, is typically conducted after drying on SERS substrates. However, this transition from the hydrated state to the dry state often leads to significant structural changes, resulting in increased spectral variability (38). Yang (36) and Tian (42) investigated the transition state between wet and dry states during the evaporation process of metal nanoparticle sols. In this state, a three-dimensional liquid “hotspot” matrix is formed, substantially increasing the intensity of SERS signals. Additionally, a super-wetting superhydrophobic porous SERS platform (43) addressed limitations by confining exosomes to ordered nanopores, ensuring uniform distribution for stable and reproducible SERS signals (Table 1).
Surface modifications are necessary to enhance the capture efficiency of exosomes by simple SERS substrates. Previous studies have focused on constructing trapping substrate-exosome-detection probe structures through immunoaffinity. However, the use of antibodies presents challenges in terms of preservation, stability, and their impact on detection. Therefore, recent studies have shifted towards the development of aptamers and capture molecules that target the exosomal phospholipid membrane structure non-specifically. Zhang et al. (44) developed a dual-aptamer ratio SERS biosensor that demonstrated high affinity and specificity for surface proteins (Epithelial cell adhesion molecule (EpCAM) (45), Prostate specific antigen (PSA) (46), carcino-embryonic antigen (CEA) (47) etc) on exosomes derived from breast cancer cells, resulting in improved sensitivity (48). The strategy involved linking the aptamers targeting two proteins (EpCAM and EGFR2) with 3’ end-modified Rhodamine through short complementary DNA, forming V-shaped DNA. This V-shaped DNA was attached to the Au@Ag NPs/GO substrate surface to selectively recognize exosomes with these two proteins. This approach provided numerous DNA adsorption sites on the GO layer, enhancing sensitivity. In terms of SERS detection of exosomes, multiple Raman probes or machine learning models are typically employed to achieve more precise diagnoses (49). However, these methods often involve cumbersome procedures and lack adequate sensitivity for early cancer diagnosis. In contrast, proportional SERS can effectively reduce the impact of background fluctuations, resulting in high sensitivity, accurate quantification, good reproducibility, and eliminating the need for nucleic acid amplification (50, 51). Thus, proportional SERS holds significant application value in the early diagnosis of cancer.
Sandwich detection methods, such as those that incorporate aptamers, can result in decreased detection efficiency due to spatial blocking (52). To overcome this limitation, a SERS strategy was developed for the sensitive detection of exosome PD-L1 (53). This approach utilized the non-selective recognition ability of MXene and the specific recognition ability of Au@MPBA@SiO. MXene was applied onto a gold chip, facilitating its interaction with the phospholipid membrane of exosomes. By doing so, the MXene was able to non-selectively capture exosomes, effectively overcoming the issue of spatial blocking (54). This novel method significantly enhanced the detection efficiency and sensitivity. Additionally, Daassi et al. (55) have demonstrated the significance of ExoPD-L1 in tumor immune evasion, further supporting the relevance of this platform’s ability to specifically recognize PD-L1 on exosomes. Notably, distinct Raman spectra were observed between healthy individuals and patients, as well as between different cell lines, validating the potential of this approach for distinguishing PD-L1 levels in exosomes from breast cancer patients compared to those of the normal population and between different cell lines.
Raman tags have the ability to bind to specific target molecules, resulting in the generation of strong and easily identifiable Raman signals (35). Numerous SERS tags with diverse structures and chemical properties have been documented in the literature. Some examples include 4-mercaptobenzoate (56), 4-Pyridinethiol (57), 4-MBA (58), and 4-Aminothiophenol (59). The utilization of multiple Raman tags not only facilitates the achievement of narrower Raman bandwidths but also expands the range of targets that can be analyzed. Consequently, this approach enhances the sensitivity and specificity for molecular analysis of exosomes (56–58).
Kwizera et al. employed the use of the organic dye QSY21, specifically designed as a Raman reporter label, to successfully monitor eight target proteins (EpCAM, CD44, HER2, EGFR, IGF1R, CD81, CD63, and CD9) found in exosomes. Their findings exhibited a strong correlation with ELISA, boasting an R2 value of 0.97 (60). Additionally, the researchers employed 3D printed array templates for the capture of exosomes via antibody arrays. This innovative approach provided rapid results, with a remarkable turnaround time of just 2 hours, making it highly promising for potential applications in clinical testing (Table 1).
On account of the co-expression of proteins of exosomes and subtle variations in expression across different cancer subtypes, Su et al. (61) developed the iREX (Integrated Raman Spectroscopy EXO) biosensor, which is capable of multiple quantitatively analyzing proteins of exosomes (MUC1, HER2 and CEA) in clinical plasma samples from breast cancer patients (Figure 1). The study revealed distinct expression profiles of these proteins in different cell subtypes, providing a precise molecular diagnosis of breast cancer subtypes. The iREX biosensor not only ensures accurate detection and diagnosis, but also minimizes false negative results by overcoming the hook effect (39, 41, 62). This biosensor’s ability to simultaneously detect multiple proteins from exosomes on a single vertically flowing NC membrane further enhances its detection capabilities. The study demonstrated that MUC1, HER2, and CEA had distinct expression levels in exosomes derived from MCF-7, SKBR-3, MDA-MB-231, and BT474 cell lines, and these findings were validated using clinical serum samples (Table 1).
Figure 1 This figure Outlines the novel SERS biosensor technology in terms of breast cancer biomarkers including exosomes, circulating tumor cells, mirnas, various proteins, etc.
In summary, the utilization of SERS in conjunction with a diverse range of strategies offers significant improvements in sensitivity, specificity, and reproducibility for the analysis of exosomes derived from breast cancer. These approaches hold great potential for early detection of cancer, thereby facilitating advancements in clinical practice. Nevertheless, it is important to emphasize that further enhancements in SERS technology are imperative to achieve optimal results.
Efficient and reliable methods for the isolation and identification of exosomes have been highly regarded, as competitive adsorption of other molecules to the metal surface can lead to challenging SERS spectra analysis. The integration of exosomes with reporter tags for Raman signals presents challenges for exosome isolation. Inadequate separation may result in the retention of excessive free Raman signal reporter tags, leading to false-positive outcomes (63). Immunomagnetic bead separation technology offers immunoaffinity-mediated exosome recognition and convenient magnetic collection, enabling efficient exosome enrichment. Li et al. (64) combined immunomagnetic bead separation technology with SERS, establishing a magnetic SERS platform. This platform demonstrated sensitive and specific detection of exosomes, enabling differentiation between two distinct breast cancer cell lines (MCF-7 and MDA-MB-231) or exosomes from serum samples with 100% sensitivity and specificity within a 95% confidence interval.
Kwizera et al. (60) constructed a droplet-based immunoassay method for the quantification of exosomes. In this method, the trapping antibody was attached to magnetic beads, enabling the adsorption of target exosomes. Subsequently, the magnetic beads were encapsulated within microdroplets using a microfluidic platform. The concentration of exosomes was estimated by measuring the fluorescence emission resulting from enzyme-catalyzed reactions. This technique exhibited the ability to differentiate the expression levels of target proteins on individual exosomes within the droplets (Table 1).
The complex composition of exosomes leads to intricate vibrational spectra patterns. To achieve more precise analysis of these spectra, SERS in combination with multivariate mathematical and statistical methods such as principal component analysis (PCA), partial least squares discriminant analysis (PLS-DA), and artificial intelligence have been employed. Xie et al. (65) utilized spectral deconvolution of complicated SERS spectra (66) collected from a SERS sensor to quantify serum exosomes. They applied the MCR-ALS algorithm for spectral separation, enabling dual detection using SERS. Furthermore, they accurately determined the concentrations of SKBR and MCF exosomes in clinical serum samples across various pathological conditions, thus demonstrating the clinical applicability of this method.
Additionally, Xie et al. (65) reported an artificial intelligence-assisted SERS strategy for label-free spectral analysis (Figure 2). Figure 3 The artificial neural network (ANN) algorithm was employed to capture the characteristic composition spectrum of serum exosomes in the SERS dataset. This algorithm demonstrated excellent predictive capabilities for distinguishing exosomes originating from different breast cancer cell lines (MDA-MB-231, MCF-7, BT474, and SKBR-3) as well as exosomes from human patients with different cancer subtypes. To assess the efficacy of the strategy, the Mahalanobis distance between the exosome clusters derived from serum samples and those from cancer cells was measured, enabling a quantitative evaluation of the similarity between the plasma and cancer cell exosome data. The results indicated that the relative similarity was reduced following surgical removal of tumors, suggesting the necessity of surgery in the context of breast cancer. Overall, this deep learning-assisted SERS integration strategy demonstrates the potential to accurately diagnose and prognosis breast cancer through the analysis of exosomes (Table 1).
Figure 2 (A) (i) Overview of the architecture and (ii) the real image of the iREX biosensor, which comprises a sample injector, a VFA device and a plastic cassette. The VFA device is stacked with a conjugate pad, a test pad of four spatially separated test spots and an absorbent pad orderly from the top to the bottom. (B) Illustration of multiplexed detection of exosomal proteins in serum samples. The aptamers immobilized in the corresponding test spots capture various target EXOs through the specific binding to the corresponding exosomal proteins, and SERS probes are bound onto EXOs via the specific recognition of the CD63 conjugation aptamer to the common exosomal CD63, forming sandwich complexes for the subsequent SERS profiling. (C) Schematic of the iREX biosensor based multiplexed detection of exosomal proteins in the presence of no EXOs (negative test) and multiple EXOs (positive test).
Figure 3 Overview of Deep Learning-Assisted SERS Spectroscopic Analysis of Serum Exosomes for Diagnosis and Postoperative Assessment of Breast Cancer. (A) The schematic workflow involving the collection of clinical samples from patients, isolation of serum exosomes, SERS measurement, and deep learning-assisted analysis. (B) The deep learning model for cancer diagnosis and postoperative assessment in which the ANN algorithm is first trained and validated using SERS data from cancerous exosomes derived from different cancer cell lines and then employed for analysis of serum exosomes from patients. Adapted from Artificial Intelligent Label-Free SERS Profiling of Serum Exosomes for Breast Cancer Diagnosis and Postoperative Assessment by Y. Xie, X. Su, Y. Wen, (C) Zheng, M. Li, Nano Lett. 22 (2022) 7910–7918. https://doi.org/10.1021/acs.nanolett.2c02928.
In a related study, Shin et al. (37) presented a deep learning approach using a CNN model to detect and classify cancer specimens, as well as determine the specific type of cancer. The method proved successful, achieving accurate differentiation among six different early cancer types, with diagnostic sensitivity and specificity surpassing 90%. While the inclusion of additional training samples and the resolution of the SERS detection chip manufacturing issue are necessary, the system offers a straightforward and rapid approach that requires minimal samples, thus offering a convenient tool for early diagnosis by clinicians.
CTCs constitute a diverse group capable of generating metastatic tumors in distant sites through epithelial-mesenchymal transition (EMT) (40, 67–69). Numerous studies have established an association between CTCs and adverse prognostic indicators such as poorer prognosis, reduced disease-free survival, and overall survival rates in breast cancer (70–73). SWOG trial (SWOG protocol S0500) results (74) highlighted that modifications in chemotherapy did not yield a significant impact on the overall survival of patients with elevated CTC levels compared to those without. While CTCs have shown promise as an independent prognostic indicator with clinical validity (dividing a population into distinct groups with markedly different clinical outcomes), their clinical utility in enhancing patient outcomes remains elusive.
The CellSearch technology is widely regarded as the gold standard for detecting and separating CTCs in breast cancer. This method targets the epithelial marker protein EpCAM on the surfaces of CTCs. However, CTCs that express EpCAM to a lesser extent or not at all may go undetected, leading to false negatives. To address this limitation, a new microfluidic chip was developed, known as the antibody-functionalized microfluidic (AFM) chip. This chip is capable of capturing not only EpCAM but also CK19, CD45, and DAPI, which enhances the accuracy of CTC detection (75). Another study by Wilson et al. (76) demonstrated the use of a microfluidic platform combined with immunomagnetic separation technology for SERS detection of CTCs in breast cancer. SERS is a highly promising technique for multiple detections due to its narrow peak, addressing the disadvantage of CellSearch, which only targets EpCAM. The use of immunomagnetic separation in a microfluidic device minimizes sample contamination and loss, while its automation reduces human intervention and operational errors. In contrast to quantitative methods for miRNA detection, this platform utilized chemometric least squares (CLS) regression to calculate weight factors for each label. These weight factors correspond to the relative intensity of SERS spectra of nanotags on cells compared to the reference. The authors of this study utilized SERS spectra of precisely defined nanotags to analyze individual cells. By quantifying the SERS signal, they were able to determine the quantity of nanolabels bound to surface proteins, thus enabling the differentiation of various types of breast cancer cells. This platform, which combined microfluidics, immunomagnetic separation, and SERS technology, proved to be an efficient method for detecting CTCs in breast cancer.
MicroRNAs (miRNAs), which are small non-coding RNAs, are actively involved in regulating key cellular processes such as cell proliferation, differentiation, apoptosis, and cancer development (77). Interestingly, numerous miRNAs are found within chromosomal regions associated with tumors (78). In particular, miRNAs exhibit significant expression variances between normal and tumor tissues, influencing critical aspects of breast tumor cell proliferation, infiltration, and migration (79). Notably, miR-21 has been reported to be upregulated in human breast cancer cell lines and is crucial throughout all stages of breast cancer pathogenesis (80, 81). Additionally, miR-221/222 clusters (82), along with miR-9, miR-10b, miR-29a, among others, are found to be overexpressed in breast cancer, while miR-30a, miR-31, miR34, miR-93, miR-125, and miR-126 are down-regulated (83–87).
Currently, common methods for detecting miRNA include probe-targeted hybridization or amplification techniques. The former includes northern Western blotting and microarray chip technology, while the latter includes RT-qPCR and sequencing. Northern blotting (88) is considered the gold standard for miRNA detection, but it is time-consuming, has low sensitivity, and requires expensive reagents. RT-qPCR (89) is highly automated, but designing miRNA primers is challenging due to base pairing mismatches during amplification (90). Sequencing technology is suitable for mass screening of early-stage breast cancer, but it is also time-consuming and costly (91, 92). Microarray (93) Target labeling is challenging due to the short probe target (94). Furthermore, miRNA has intrinsic characteristics such as short sequences, highly homology (95), low abundance, (accounting for only 0.01% of total RNA in plasma) (96, 97), and it exhibits a dynamic range of at least four orders of magnitude (98), requiring a highly sensitive detection method. As mentioned above, SERS has been shown to possess advantages such as ultra-high sensitivity, narrow spectral bandwidth, and strong multiple detection capability, making it a promising tool for miRNA detection.
In the context of miRNA detection, similar to exosome detection, innovative techniques are utilized in surface-enhanced Raman scattering (SERS) to enhance sensitivity. These methods include the use of novel substrates, targeted labels, and oligonucleotides. Droplet detection (99) presents several advantages, such as compartmentalization, small volume analysis, and high throughput capacities. The integration of SERS with liquid droplet arrays markedly minimizes liquid volume requirements. In contrast to super-wetting microchips described in exosome detection (100, 101) in exosome detection, liquid droplet array microcolumns effectively secure and concentrate small-volume droplets. Homogeneously dispersed miRNA within these arrays can significantly amplify SERS signal intensity (38, 102, 103). This platform (104)has successfully distinguished four breast cancer-related miRNAs, demonstrating promising applications in early breast cancer diagnostics.
Lee et al. (105) demonstrated the development of a SERS biosensor utilizing plasma-headed velvet gold nanorods. This unique configuration induced the aggregation of neighboring nanorods, leading to a reduction in the interparticle gaps and the formation of self-assembled hotspots (106, 107). The biosensor exhibited remarkable sensitivity, enabling the detection of miR-21, miR-222, and miR-200c of exosomes with low limits of detection, as well as a wide dynamic range, without the need for amplification processes.
To achieve the stability of the interaction between the capture probe and miRNA, oligonucleotide-based strategies, specifically hybridization, were employed. The sandwich hybridization strategy (108–110) proved effective only when the target miRNA sequence perfectly complemented both Locked Nucleic Acid (LNA) probes. Due to the separation of the Raman dye from the substrate caused by the hybridization reaction between the DNA probe and the amplified miRNA product, the Raman signal weakened, resulting in a gradual decrease in the height of the Raman signature peak with increasing miRNA concentration. Therefore, miRNA can be quantified through the fitting of a concentration-dependent Raman intensity curve, allowing for a precise quantitative analysis of miRNA.
In contrast to exosome detection, several studies have reported strategies that combine isothermal amplification of miRNA with SERS. Xu et al. (111) described a sandwich SERS platform utilizing Au@Ag core-shell nanorods. A significant feature of this sensor is the integration of double-stranded specific nucleases (DSNSA) for signal amplification. DSN is a protein enzyme known for its selective degradation of double-stranded DNA (112, 113). This unique property makes it highly advantageous for miRNA detection. Utilizing the hydrolysis reaction of DSN, the target molecule miRNA can be recycled and reused, allowing for the involvement of trace amounts of miRNA in multiple DSN digestion reactions (114), which improved the sensitivity and amplified the signal. By monitoring Raman signal attenuation, the sensor simultaneously measured miR-21, miR-155, and let 7b through three different Raman reporting tags, respectively.
Meng et al. (115) reported a novel approach to enhance the sensitivity of as SERS biosensor by employing isothermal amplification of microRNA (miRNA) using a nuclease enzyme. In contrast to existing techniques, this method generates a three-dimensional (3D) SERS holographic image through computer processing, which enables visualization, offers excellent reproducibility, and reduces processing time (116). The developed biosensor successfully detects nine breast cancer-related miRNAs in both standard and clinical samples, exhibiting superior accuracy when compared to RT-qPCR.
Weng et al. (113) described the application of catalytic hairpin assembly (CHA) technology in a SERS biosensor for the highly sensitive detection of miRNA-21 and miRNA-155. In comparison to enzymatic DNA loop reactions, CHA offers several advantages such as low cost and simplicity in reaction conditions, as well as easy storage (117). Through CHA, DNA molecules generated were capable of binding reporter genes to SERS substrates. Consequently, the intensity of the SERS signal was directly proportional to the concentration of DNA products associated with the target miRNAs. This platform achieved remarkably low detection limits of 0.398 fM and 0.215 fM for miRNA-21 and miRNA-155, respectively, by exploiting the synergistic effects of RNA cycling amplification and SERS “hotspots”.
Zhang et al. (118) proposed a novel Fluorescent-Raman Binary Star Ratio Probe (BSR) that utilizes enzyme-free amplification to enhance the sensitivity of surface-enhanced Raman scattering (SERS). The researchers also conducted fluorescence imaging experiments to evaluate the stability of the BSR probes in complex microenvironments and their potential for imaging single living cells. The concentration of miRNA-203 in single cells determined by the SERS signal intensity ratio of Cy3 and Rox was found to be consistent with previous findings. By implementing dual Raman reporter molecules, the platform effectively reduced background interference and exhibited improved stability in complex biological environments. The probe successfully detected miRNA-203 in single cells, thereby offering a promising tool for precise medical imaging.
Similarly, Wang et al. (119) recently reported the development of a SERS biosensor that utilizes a reverse molecular sentinel (iMS) nanoprobe. This nanoprobe incorporates miRNA molecules, causing the displacement of a single DNA placeholder chain and the formation of a stem-ring structure. Subsequently, the nanoprobe binds to the surface of plasma active nanostars (AuNS@Ag), resulting in a significant enhancement of the SERS signal (ON state). Conversely, when the placeholder chain is intact, the increased distance between the nanoprobe and the nanostars leads to a weaker SERS signal (OFF state). To achieve differentiation between miR-21 and miR-34a without the need for target labeling or subsequent washing steps, a suitable occupying chain is designed with a short poly(T) tail at its 3’ end. This occupying chain demonstrates high thermal stability and consistently generates a stable OFF signal.
Coupled with isothermal amplification offers a notable advantage in reducing sample consumption and enhancing sensitivity. Nevertheless, it encounters complexities in intricate biomedical analyses, often necessitating time-intensive reactions. Furthermore, in the enzyme-assisted DNA cycle reaction, there is a susceptibility for enzyme adsorption onto the electrode surface, potentially compromising sensitivity. Microfluidic technology emerges as a viable strategy to address such separation challenges. Wang et al. (94)presented a novel approach that integrates SERS with alternating current and microfluidics (120). The enrichment of TMAS (Triggerable Mutually Amplified Signal) probes in a microfluidic reaction chamber leads to significant fluid flow, which is a result of the combined action of electrothermal and alternating current electroosmotic force. This phenomenon promotes the sensitivity of the reaction, as evidenced by a low limit of detection (LOD) of miR-21 at 2.33 fM. In addition, microfluidic chips that employ AC electric flow technology demonstrate efficient mixing capabilities, resulting in improved uniformity and DNA cross rate (121). This, in turn, significantly reduces the time required for detection (122). A comparison between this approach and the traditional microfluidic method (123), reveals shorter measurement times, higher signal uniformity, and enhanced detection sensitivity and reproducibility.
Furthermore, Zhang (124) et al. presented an innovative multimodal spectral approach for early diagnosis of breast cancer using attenuated total reflection Fourier Transform infrared spectroscopy (ATR-FTIR) and SERS data. The authors employed a set of 32 machine learning models to extract complementary information and enhance the accuracy of miRNA detection. The verification accuracy rate achieved was 95.1% while the test accuracy stood at 91.6%.
Research on urine samples, as well as serum samples, is currently being conducted. Kim et al. (125) introduced a platform that utilizes silver nanorods grown on SNP, leading to a significant enhancement in sensitivity for the simultaneous detection of two miRNAs (miRNA-21/155). Through the use of nanocolumns grown on SNPs, this platform is able to detect multiple targets at one point, resulting in improved sensitivity with detection limits (LOD) of 451 zmol and 1.65 amol, respectively. The platform also allowed for the quantification of the miRNAs in various samples, enabling the classification of four cancer cell lines (MCF-7, HCC1143, MDA-MB-231, HCC1954).
In their study, Liu et al. (126) investigated the use of copper phthalocyanine (CuPc) molecules as a diagnostic and therapeutic nanoplatform for breast cancer miRNA detection employing surface-enhanced Raman scattering (SERS). The authors demonstrated that CuPc molecules played a dual role in SERS detection capability and photodynamic therapy (PDT). Firstly, the SERS signal enhancement factor (EF) value of CuPc was estimated to be 7.42×104, which surpassed that of other 2D nanomaterials (127). Secondly, PDT (128, 129) combined with CuPc@HG@BN showed promising therapeutic effects. For instance, when the tumor was at an early stage, complete clearance was achieved with a concentration of only 25 μg/mL of CuPc@HG@BN. However, for tumors that had been growing for 9 days, they observed only a slight reduction in miR-21 levels after 3 days of treatment. Even with a drug concentration of 200 μg/mL, the tumor was no longer eliminated, highlighting the importance of this platform in the early diagnosis and treatment of breast cancer in clinical settings. This study not only employed CuPc, but also implemented a ratio strategy and ring amplification of miRNA to improve the accuracy and sensitivity of the platform. The use of ratio strategy enhanced the early diagnosis capability, while the ring amplification improved the sensitivity of the platform, achieving an intracellular miR-21 reaction concentration as low as 0.7 fM. In summary, this study presents a multifunctional platform for the diagnosis and treatment of breast cancer utilizing SERS, contributing to the advancement of SERS applications in breast cancer research.
HER2 is typically distinguished among different subtypes of breast cancer through assessment using a grading system of four levels based on staining intensity. The current gold standard method, immunohistochemistry (IHC), is relatively inexpensive but lacks objectivity in results (130). Fluorescence in situ hybridization (FISH) can detect the HER2 gene copy number in each cell nucleus, but it requires expensive reagents and laboratory equipment (131). SERS possesses highly efficient optical properties such as narrow bandwidth Raman peaks. Murali (132), Xie (133), Mo (134), Verdin (135), Téllez-Plancarte (136), et al. have utilized SERS to detect HER2 levels in samples from various sources. Their proportional grading reduces background interference, yielding detection results highly similar to FISH. SERS enables simultaneous, objective, and quantitative detection of multiple receptor proteins rapidly, presenting a promising means for future receptor protein analysis. Similarly, Kapara et al. (137) conducted research on identifying breast cancer subtypes by detecting different levels of ER and PR expression.
Matrix metalloproteinases (MMPs) constitute a large family of proteases (138). Elevated synthesis and activity levels of MMPs in cancer have been shown to result in alterations in extracellular matrix protein hydrolysis (139). The expression of MT1-MMP (140), MMP-2 (141), and MMP-9 (142, 143) is closely associated with cancer invasion and metastasis. Zhong et al. (144) proposed a ratio-type SERS nanoprobe, where the SERS intensity of a substrate peptide labeled with Rh B on the nanoprobe decreased specifically under MMP-2 digestion, while the 2-NT signal served as an internal standard for proportional imaging. Differential expression of MMP-2 in breast cancer of varying malignancy grades enabled the identification of different breast cancer subtypes. Zhu et al. (145) further localized SERS nanoprobe labeling of MT1-MMP in MDA-MB-231 cells, achieving marked and imaging.
Liu et al. (146) developed a SERS biosensing platform revealing real-time changes in MMP-9 secretion during intercellular communication, offering a new strategy for real-time monitoring of MMP-9 secretion during cell communication processes, laying the foundation for future research in breast cancer microenvironments and metastasis.
Low levels of serum albumin have high application value in determining the prognosis of breast cancer (147). In previous studies, SERS analysis of serum albumin showed great promise in cancer detection and screening, such as colorectal cancer, gastric cancer, nasopharyngeal cancer, and liver cancer (148–150). Lin et al. (151) used hydroxyapatite particles to separate albumin and then obtained its signal through SERS. Through PLS analysis of collected SERS data and using LDA to determine the functional relationship, they achieved an ideal diagnostic sensitivity (100%) and specificity (97.5%).
The relatively low concentration of biomarkers in the peripheral blood of early breast cancer patients limits many current detection methods, while SERS possesses the advantages of trace analysis and multiple detections. Zhen et al. (120) developed a SERS biosensor based on a microfluidic chip that can simultaneously detect three important breast cancer biomarkers: CA153, CA125, and CEA. It holds significant medical significance for early detection of breast cancer and has great application value. Positive margins are a significant cause of breast cancer recurrence.
Breast cancer cells can overexpress CD47 as a means to evade the immune system. Davis et al. (152) collected data on the level of binding of SERS nanoparticles conjugated with CD47-specific antibodies to various breast cancer cell lines as well as margin specimens during surgery. They successfully differentiated clinically different breast cancer cell lines and tumor samples from normal adjacent tissues, although further exploration of their clinical application value is required.
In contrast to ductal carcinoma in situ (DCIS), infiltrating breast cancer (IBC) exhibits a significant increase in polysulfides in the stroma due to the decrease in bisulfide, laying the foundation for distinguishing DCIS and IBC by detecting different levels of polysulfides. Kubo et al. (153) used SERS imaging based on gold nanoparticles to monitor and analyze polysulfides and proposed a strategy for automated diagnosis using machine learning. This provides a potential means for monitoring the hyperplasia of connective tissue occurring in cancer-related stromal regions.
Sialic acid (SA) is a component that can bind to salivary glycolipids and glycoproteins (154). Its abnormal expression is believed to be associated with the occurrence and development of tumors. Obtaining sialic acid is simpler and less invasive than other biomarkers. Liang et al. (155) developed a SERS molecular strategy based on MPBA@AgNP nanoprobes. They also utilized big data analysis to increase the reliability of SERS data results. Peak ratio at 1074 and 1572 cm-1 indicates that the expression level of sialic acid on tumor cells is higher than that on normal cells, thus achieving early diagnosis of breast cancer. Similarly, Hernández-Arteaga et al. (156) validated the increased level of sialic acid in the saliva of breast cancer patients compared to benign tumors using a citrate-Ag-NP-based SERS biosensor. These studies contribute significantly to the field of early diagnosis of breast cancer through the use of sialic acid as a biomarker.
At present, several issues hinder the clinical application of SERS in breast cancer detection. Firstly, the utilization of SERS technology to simultaneously detect multiple biomarkers in clinical samples (e.g., tissues, serum) is hindered by prolonged detection time and subtle differences in target biomarkers. To address this, combining the complex data obtained by different SERS sensors with artificial intelligence and statistical technology has emerged as a general trend. However, a lack of standard detection procedures persists. Secondly, exosomes, miRNAs, and CTCS exhibit low abundance and necessitate isolation from other small molecules in body fluids, such as cell fragments, lipoproteins, and protein aggregates. Thirdly, while the amalgamation of SERS with immunomagnetic separation and microfluidic technology partially resolves this limitation, studies on this topic remain scarce. There is insufficient research data to definitively establish which substrate type can generate more hotspots and achieve higher detection sensitivity. Furthermore, although some studies have investigated the use of SERS for detecting breast cancer samples in tears, saliva, urine, and other liquid sources, limited research exists on the detection of exosomes, miRNAs, or CTCS in these samples. Consequently, it is imperative to develop SERS strategies that are capable of detecting exosomes, miRNAs, and CTCS, while also ensuring the acquisition of high-quality and reproducible Raman signals. Consequently, this novel avenue of research focusing on liquid biopsy via SERS holds significant promise for obtaining comprehensive diagnostic information in the future.
XL: Investigation, Writing – original draft, Writing – review & editing. YJ: Investigation, Writing – original draft, Writing – review & editing. CZ: Conceptualization, Funding acquisition, Supervision, Writing – review & editing.
The author(s) declare financial support was received for the research, authorship, and/or publication of this article. This research was primarily granted funding from the Shandong Provincial Natural Science Foundation (ZR2022MH034), the Taishan Scholars Program of Shandong Province (tsqn201812135), the China Postdoctoral Science Foundation (2021T140408 and 2021M691934), and Jinan Clinical Medical Science and technology Innovation plan (202225071), and Young Physician Research Award Fund of China Breast Surgery (2020-CHPASLP-01).
The authors declare that the research was conducted in the absence of any commercial or financial relationships that could be construed as a potential conflict of interest.
All claims expressed in this article are solely those of the authors and do not necessarily represent those of their affiliated organizations, or those of the publisher, the editors and the reviewers. Any product that may be evaluated in this article, or claim that may be made by its manufacturer, is not guaranteed or endorsed by the publisher.
1. Han B, Zheng R, Zeng H, Wang S, Sun K, Chen R, et al. Cancer incidence and mortality in China, 2022. J Natl Cancer Cent. (2024) 4: 47–53. doi: 10.1016/j.jncc.2024.01.006
2. Harbeck N, Penault-Llorca F, Cortes J, Gnant M, Houssami N, Poortmans P, et al. Breast cancer. Nat Rev Dis Primer. (2019) 5:1–31. doi: 10.1038/s41572-019-0111-2
3. Stastny I, Zubor P, Kajo K, Kubatka P, Golubnitschaja O, Dankova Z. Aberrantly methylated cfDNA in body fluids as a promising diagnostic tool for early detection of breast cancer. Clin Breast Cancer. (2020) 20:e711–22. doi: 10.1016/j.clbc.2020.05.009
4. Song M, Giovannucci E. Preventable incidence and mortality of carcinoma associated with lifestyle factors among whites in the United States. JAMA Oncol. (2016) 2:1154–61. doi: 10.1001/jamaoncol.2016.0843
5. Garrido-Castro AC, Lin NU, Polyak K. Insights into molecular classifications of triple-negative breast cancer: improving patient selection for treatment. Cancer Discovery. (2019) 9:176–98. doi: 10.1158/2159-8290.CD-18-1177
6. Tsang JYS, Tse GM. Molecular classification of breast cancer. Adv Anat Pathol. (2020) 27:27. doi: 10.1097/PAP.0000000000000232
7. Drukteinis JS, Mooney BP, Flowers CI, Gatenby RA. Beyond mammography: new frontiers in breast cancer screening. Am J Med. (2013) 126:472–9. doi: 10.1016/j.amjmed.2012.11.025
8. Kolb TM, Lichy J, Newhouse JH. Comparison of the performance of screening mammography, physical examination, and breast US and evaluation of factors that influence them: an analysis of 27,825 patient evaluations. Radiology. (2002) 225:165–75. doi: 10.1148/radiol.2251011667
9. Wang R, Li X, Zhang H, Wang K, He J. Cell-free circulating tumor DNA analysis for breast cancer and its clinical utilization as a biomarker. Oncotarget. (2017) 8:75742–55. doi: 10.18632/oncotarget.v8i43
10. Appierto V, Di Cosimo S, Reduzzi C, Pala V, Cappelletti V, Daidone MG. How to study and overcome tumor heterogeneity with circulating biomarkers: The breast cancer case. Semin Cancer Biol. (2017) 44:106–16. doi: 10.1016/j.semcancer.2017.04.007
11. Bardelli A, Pantel K, Biopsies L. What we do not know (Yet). Cancer Cell. (2017) 31:172–9. doi: 10.1016/j.ccell.2017.01.002
12. Hessvik NP, Llorente A. Current knowledge on exosome biogenesis and release. Cell Mol Life Sci. (2018) 75:193–208. doi: 10.1007/s00018-017-2595-9
13. Li M, Cushing SK, Wu N. Plasmon-enhanced optical sensors: A review. Analyst. (2015) 140:386–406. doi: 10.1039/C4AN01079E
14. Shan B, Pu Y, Chen Y, Liao M, Li M. Novel SERS labels: Rational design, functional integration and biomedical applications. Coord Chem Rev. (2018) 371:11–37. doi: 10.1016/j.ccr.2018.05.007
15. Li M, Cushing SK, Zhou G, Wu N. Molecular hot spots in surface-enhanced Raman scattering. Nanoscale. (2020) 12:22036–41. doi: 10.1039/D0NR06579J
16. Zhang Y, Mi X, Tan X, Xiang R. Recent progress on liquid biopsy analysis using surface-enhanced raman spectroscopy. Theranostics. (2019) 9:491–525. doi: 10.7150/thno.29875
17. Kalluri R, LeBleu VS. The biology, function, and biomedical applications of exosomes. Science. (2020) 367:eaau6977. doi: 10.1126/science.aau6977
18. van Niel G, D’Angelo G, Raposo G. Shedding light on the cell biology of extracellular vesicles. Nat Rev Mol Cell Biol. (2018) 19:213–28. doi: 10.1038/nrm.2017.125
19. Guo Y, Ji X, Liu J, Fan D, Zhou Q, Chen C, et al. Effects of exosomes on pre-metastatic niche formation in tumors. Mol Cancer. (2019) 18:39. doi: 10.1186/s12943-019-0995-1
20. Schmelz K, Toedling J, Huska M, Cwikla MC, Kruetzfeldt L-M, Proba J, et al. Spatial and temporal intratumour heterogeneity has potential consequences for single biopsy-based neuroblastoma treatment decisions. Nat Commun. (2021) 12:6804. doi: 10.1038/s41467-021-26870-z
21. Eichelser C, Stückrath I, Müller V, Milde-Langosch K, Wikman H, Pantel K, et al. Increased serum levels of circulating exosomal microRNA-373 in receptor-negative breast cancer patients. Oncotarget. (2014) 5:9650–63. doi: 10.18632/oncotarget.v5i20
22. O’Brien K, Rani S, Corcoran C, Wallace R, Hughes L, Friel AM, et al. Exosomes from triple-negative breast cancer cells can transfer phenotypic traits representing their cells of origin to secondary cells. Eur J Cancer. (2013) 49:1845–59. doi: 10.1016/j.ejca.2013.01.017
23. Tominaga N, Kosaka N, Ono M, Katsuda T, Yoshioka Y, Tamura K, et al. Brain metastatic cancer cells release microRNA-181c-containing extracellular vesicles capable of destructing blood–brain barrier. Nat Commun. (2015) 6:6716. doi: 10.1038/ncomms7716
24. Chen W, Liu X, Lv M, Chen L, Zhao J, Zhong S, et al. Exosomes from drug-resistant breast cancer cells transmit chemoresistance by a horizontal transfer of microRNAs. PloS One. (2014) 9:e95240. doi: 10.1371/journal.pone.0095240
25. Chen H, Huang C, Wu Y, Sun N, Deng C. Exosome metabolic patterns on aptamer-coupled polymorphic carbon for precise detection of early gastric cancer. ACS Nano. (2022) 16:12952–63. doi: 10.1021/acsnano.2c05355
26. Tan J, Wen Y, Li M. Emerging biosensing platforms for quantitative detection of exosomes as diagnostic biomarkers. Coord Chem Rev. (2021) 446:214111. doi: 10.1016/j.ccr.2021.214111
27. Jin Y, Du N, Huang Y, Shen W, Tan Y, Chen YZ, et al. Fluorescence analysis of circulating exosomes for breast cancer diagnosis using a sensor array and deep learning. ACS Sens. (2022) 7:1524–32. doi: 10.1021/acssensors.2c00259
28. Song F, Wang C, Wang C, Gao J, Liu H, Zhang Y, et al. Enrichment-detection integrated exosome profiling biosensors promising for early diagnosis of cancer. Anal Chem. (2021) 93:4697–706. doi: 10.1021/acs.analchem.0c05245
29. Zeng Y, Madsen SJ, Yankovich AB, Olsson E, Sinclair R. Comparative electron and photon excitation of localized surface plasmon resonance in lithographic gold arrays for enhanced Raman scattering. Nanoscale. (2020) 12:23768–79. doi: 10.1039/D0NR04081A
30. Chauhan P, Bhargava A, Kumari R, Ratre P, Tiwari R, Kumar R Srivastava, et al. Surface-enhanced Raman scattering biosensors for detection of oncomiRs in breast cancer. Drug Discovery Today. (2022) 27:2121–36. doi: 10.1016/j.drudis.2022.04.016
31. Li T, Zhang Y, He B, Yang B, Huang Q. Periodically hydrologic alterations decouple the relationships between physicochemical variables and chlorophyll-a in a dam-induced urban lake. J Environ Sci. (2021) 99:187–95. doi: 10.1016/j.jes.2020.06.014
32. Lapresta-Fernández A, Nefeli Athanasopoulou E, Jacob Silva P, Pelin Güven Z, Stellacci F. Site-selective surface enhanced Raman scattering study of ligand exchange reactions on aggregated Ag nanocubes. J Colloid Interface Sci. (2022) 616:110–20. doi: 10.1016/j.jcis.2022.02.051
33. Ferreira N, Marques A, Águas H, Bandarenka H, Martins R, Bodo C, et al. Label-free nanosensing platform for breast cancer exosome profiling. ACS Sens. (2019) 4:2073–83. doi: 10.1021/acssensors.9b00760
34. Pramanik A, Mayer J, Patibandla S, Gates K, Gao Y, Davis D, et al. Mixed-dimensional heterostructure material-based SERS for trace level identification of breast cancer-derived exosomes. ACS Omega. (2020) 5:16602–11. doi: 10.1021/acsomega.0c01441
35. Han XX, Zhao B, Ozaki Y. Surface-enhanced Raman scattering for protein detection. Anal Bioanal Chem. (2009) 394:1719–27. doi: 10.1007/s00216-009-2702-3
36. Liu H, Yang Z, Meng L, Sun Y, Wang J, Yang L, et al. Three-dimensional and time-ordered surface-enhanced raman scattering hotspot matrix. J Am Chem Soc. (2014) 136:5332–41. doi: 10.1021/ja501951v
37. Shin H, Choi BH, Shim O, Kim J, Park Y, Cho SK, et al. Single test-based diagnosis of multiple cancer types using Exosome-SERS-AI for early stage cancers. Nat Commun. (2023) 14:1644. doi: 10.1038/s41467-023-37403-1
38. Hernandez-Perez R, Fan ZH, Garcia-Cordero JL. Evaporation-driven bioassays in suspended droplets. Anal Chem. (2016) 88:7312–7. doi: 10.1021/acs.analchem.6b01657
39. Rosenbohm JM, Klapperich CM, Cabodi M. Tunable duplex semi-quantitative detection of nucleic acids with a visual lateral flow immunoassay readout. Anal Chem. (2022) 94:3956–62. doi: 10.1021/acs.analchem.1c05039
40. Yu M, Bardia A, Wittner BS, Stott SL, Smas ME, Ting DT, et al. Circulating breast tumor cells exhibit dynamic changes in epithelial and mesenchymal composition. Science. (2013) 339:580–4. doi: 10.1126/science.1228522
41. Xiong E, Jiang L, Tian T, Hu M, Yue H, Huang M, et al. Simultaneous dual-gene diagnosis of SARS-coV-2 based on CRISPR/cas9-mediated lateral flow assay. Angew Chem Int Ed. (2021) 60:5307–15. doi: 10.1002/anie.202014506
42. Ge M, Li P, Zhou G, Chen S, Han W, Qin F, et al. General surface-enhanced raman spectroscopy method for actively capturing target molecules in small gaps. J Am Chem Soc. (2021) 143:7769–76. doi: 10.1021/jacs.1c02169
43. Zhu K, Zhou T, Chen P, Zong S, Wu L, Cui Y, et al. Long-lived SERS matrix for real-time biochemical detection using “Frozen” Transition state. ACS Sens. (2023) 8:3360–9. doi: 10.1021/acssensors.3c00302
44. Zhang Q, Ma R, Zhang Y, Zhao J, Wang Y, Xu Z. Dual-aptamer-assisted ratiometric SERS biosensor for ultrasensitive and precise identification of breast cancer exosomes. ACS Sens. (2023) 8:875–83. doi: 10.1021/acssensors.2c02587
45. Wang H, Chen H, Huang Z, Li T, Deng A, Kong J. DNase I enzyme-aided fluorescence signal amplification based on graphene oxide-DNA aptamer interactions for colorectal cancer exosome detection. Talanta. (2018) 184:219–26. doi: 10.1016/j.talanta.2018.02.083
46. Chen W-J, Shao Y-H, Li C-N, Liu M-Z, Wang Z, Deng N-Y. ν-projection twin support vector machine for pattern classification. Neurocomputing. (2020) 376:10–24. doi: 10.1016/j.neucom.2019.09.069
47. An Y, Li R, Zhang F, He P. Magneto-mediated electrochemical sensor for simultaneous analysis of breast cancer exosomal proteins. Anal Chem. (2020) 92:5404–10. doi: 10.1021/acs.analchem.0c00106
48. Gotrik MR, Feagin TA, Csordas AT, Nakamoto MA, Soh HT. Advancements in aptamer discovery technologies. Acc Chem Res. (2016) 49:1903–10. doi: 10.1021/acs.accounts.6b00283
49. Wang J, Wuethrich A, Lobb RJ, Antaw F, Sina AAI, Lane RE, et al. Characterizing the heterogeneity of small extracellular vesicle populations in multiple cancer types via an ultrasensitive chip. ACS Sens. (2021) 6:3182–94. doi: 10.1021/acssensors.1c00358
50. Wu Y, Xiao F, Wu Z, Yu R. Novel aptasensor platform based on ratiometric surface-enhanced raman spectroscopy. Anal Chem. (2017) 89:2852–8. doi: 10.1021/acs.analchem.6b04010
51. Chen J, Wu Y, Fu C, Cao H, Tan X, Shi W, et al. Ratiometric SERS biosensor for sensitive and reproducible detection of microRNA based on mismatched catalytic hairpin assembly. Biosens Bioelectron. (2019) 143:111619. doi: 10.1016/j.bios.2019.111619
52. Gao F, Jiao F, Xia C, Zhao Y, Ying W, Xie Y, et al. A novel strategy for facile serum exosome isolation based on specific interactions between phospholipid bilayers and TiO2. Chem Sci. (2019) 10:1579–88. doi: 10.1039/C8SC04197K
53. Zhou Y, Chen Q, Zhong S, Liu H, Koh K, Chen H. Ti3C2Tx MXene -facilitated non-selective trapping effect: Efficient SERS detection of exosomal PD-L1. Biosens Bioelectron. (2023) 237:115493. doi: 10.1016/j.bios.2023.115493
54. You Q, Zhuang L, Chang Z, Ge M, Mei Q, Yang L, et al. Hierarchical Au nanoarrays functionalized 2D Ti2CTx MXene membranes for the detection of exosomes isolated from human lung carcinoma cells. Biosens Bioelectron. (2022) 216:114647. doi: 10.1016/j.bios.2022.114647
55. Daassi D, Mahoney KM, Freeman GJ. The importance of exosomal PDL1 in tumour immune evasion. Nat Rev Immunol. (2020) 20:209–15. doi: 10.1038/s41577-019-0264-y
56. Pham X-H, Hahm E, Kang E, Son BS, Ha Y, Kim H-M, et al. Control of silver coating on raman label incorporated gold nanoparticles assembled silica nanoparticles. Int J Mol Sci. (2019) 20:1258. doi: 10.3390/ijms20061258
57. Yu Z, Smith ME, Zhang J, Zhou Y, Zhang P. Determination of trichloroethylene by using self-referenced SERS and gold-core/silver-shell nanoparticles. Microchim Acta. (2018) 185: 330. doi: 10.1007/s00604-018-2870-y
58. Huang X-B, Wu S-H, Hu H-C, Sun J-J. AuNanostar@4-MBA@Au core–shell nanostructure coupled with exonuclease III-assisted cycling amplification for ultrasensitive SERS detection of ochratoxin A. ACS Sens. (2020) 5:2636–43. doi: 10.1021/acssensors.0c01162
59. Zhou T, Fan M, You R, Lu Y, Huang L, Xu Y, et al. Fabrication of Fe3O4/Au@ATP@Ag Nanorod sandwich structure for sensitive SERS quantitative detection of histamine. Anal Chim Acta. (2020) 1104:199–206. doi: 10.1016/j.aca.2020.01.017
60. Kwizera EA, O’Connor R, Vinduska V, Williams M, Butch ER, Snyder SE, et al. Molecular detection and analysis of exosomes using surface-enhanced raman scattering gold nanorods and a miniaturized device. Theranostics. (2018) 8:2722–38. doi: 10.7150/thno.21358
61. Su X, Liu X, Xie Y, Chen M, Zheng C, Zhong H, et al. Integrated SERS-vertical flow biosensor enabling multiplexed quantitative profiling of serological exosomal proteins in patients for accurate breast cancer subtyping. ACS Nano. (2023) 17:4077–88. doi: 10.1021/acsnano.3c00449
62. Gomez-Martinez J, Silvy M, Chiaroni J, Fournier-Wirth C, Roubinet F, Bailly P, et al. Multiplex lateral flow assay for rapid visual blood group genotyping. Anal Chem. (2018) 90:7502–9. doi: 10.1021/acs.analchem.8b01078
63. Kang Y-T, Purcell E, Palacios-Rolston C, Lo T-W, Ramnath N, Jolly S, et al. Isolation and profiling of circulating tumor-associated exosomes using extracellular vesicular lipid-protein binding affinity based Microfluidic device. Small Weinh Bergstr Ger. (2019) 15:e1903600. doi: 10.1002/smll.201903600
64. Li G, Zhu N, Zhou J, Kang K, Zhou X, Ying B, et al. A magnetic surface-enhanced Raman scattering platform for performing successive breast cancer exosome isolation and analysis. J Mater Chem B. (2021) 9:2709–16. doi: 10.1039/D0TB02894K
65. Xie Y, Su X, Wen Y, Zheng C, Li M. Artificial intelligent label-free SERS profiling of serum exosomes for breast cancer diagnosis and postoperative assessment. Nano Lett. (2022) 22:7910–8. doi: 10.1021/acs.nanolett.2c02928
66. Felten J, Hall H, Jaumot J, Tauler R, de Juan A, Gorzsás A. Vibrational spectroscopic image analysis of biological material using multivariate curve resolution–alternating least squares (MCR-ALS). Nat Protoc. (2015) 10:217–40. doi: 10.1038/nprot.2015.008
67. Bidard F-C, Michiels S, Riethdorf S, Mueller V, Esserman LJ, Lucci A, et al. Circulating tumor cells in breast cancer patients treated by neoadjuvant chemotherapy: A meta-analysis. JNCI J Natl Cancer Inst. (2018) 110:560–7. doi: 10.1093/jnci/djy018
68. Gkountela S, Castro-Giner F, Szczerba BM, Vetter M, Landin J, Scherrer R, et al. Circulating tumor cell clustering shapes DNA methylation to enable metastasis seeding. Cell. (2019) 176:98–112.e14. doi: 10.1016/j.cell.2018.11.046
69. Micalizzi DS, Maheswaran S, Haber DA. A conduit to metastasis: circulating tumor cell biology. Genes Dev. (2017) 31:1827–40. doi: 10.1101/gad.305805.117
70. Rack B, Schindlbeck C, Jückstock J, Andergassen U, Hepp P, Zwingers T, et al. Circulating tumor cells predict survival in early average-to-high risk breast cancer patients. J Natl Cancer Inst. (2014) 106:dju066. doi: 10.1093/jnci/dju066
71. Zhang L, Riethdorf S, Wu G, Wang T, Yang K, Peng G, et al. Meta-analysis of the prognostic value of circulating tumor cells in breast cancer. Clin Cancer Res. (2012) 18:5701–10. doi: 10.1158/1078-0432.CCR-12-1587
72. Janni WJ, Rack B, Terstappen LWMM, Pierga J-Y, Taran F-A, Fehm T, et al. Pooled analysis of the prognostic relevance of circulating tumor cells in primary breast cancer. Clin Cancer Res. (2016) 22:2583–93. doi: 10.1158/1078-0432.CCR-15-1603
73. Sparano J, O’Neill A, Alpaugh K, Wolff AC, Northfelt DW, Dang CT, et al. Association of circulating tumor cells with late recurrence of estrogen receptor–positive breast cancer: A secondary analysis of a randomized clinical trial. JAMA Oncol. (2018) 4:1700–6. doi: 10.1001/jamaoncol.2018.2574
74. Nanou A, Miao J, Coumans FAW, Dolce EM, Darga E, Barlow W, et al. Tumor-derived extracellular vesicles as complementary prognostic factors to circulating tumor cells in metastatic breast cancer. JCO Precis Oncol. (2023) 7:e2200372. doi: 10.1200/PO.22.00372
75. Abdulla A, Zhang Z, Ahmad KZ, Warden AR, Li H, Ding X. Rapid and efficient capturing of circulating tumor cells from breast cancer Patient’s whole blood via the antibody functionalized microfluidic (AFM) chip. Biosens Bioelectron. (2022) 201:113965. doi: 10.1016/j.bios.2022.113965
76. Wilson RE, O’Connor R, Gallops CE, Kwizera EA, Noroozi B, Morshed BI, et al. Immunomagnetic capture and multiplexed surface marker detection of circulating tumor cells with magnetic multicolor surface-enhanced raman scattering nanotags. ACS Appl Mater Interfaces. (2020) 12:47220–32. doi: 10.1021/acsami.0c12395
77. Mulrane L, McGee SF, Gallagher WM, O’Connor DP. miRNA dysregulation in breast cancer. Cancer Res. (2013) 73:6554–62. doi: 10.1158/0008-5472.CAN-13-1841
78. Nemeth K, Bayraktar R, Ferracin M, Calin GA. Non-coding RNAs in disease: from mechanisms to therapeutics. Nat Rev Genet. (2024) 25:211–32. doi: 10.1038/s41576-023-00662-1
79. Ramassone A, Pagotto S, Veronese A, Visone R. Epigenetics and microRNAs in cancer. Int J Mol Sci. (2018) 19:459. doi: 10.3390/ijms19020459
80. Zhang ZJ, Ma SL. miRNAs in breast cancer tumorigenesis (Review). Oncol Rep. (2012) 27:903–10. doi: 10.3892/or.2011.1611
81. Corcoran C, Friel AM, Duffy MJ, Crown J, O’Driscoll L. Intracellular and extracellular microRNAs in breast cancer. Clin Chem. (2011) 57:18–32. doi: 10.1373/clinchem.2010.150730
82. Piva R, Spandidos DA, Gambari R. From microRNA functions to microRNA therapeutics: Novel targets and novel drugs in breast cancer research and treatment. Int J Oncol. (2013) 43:985–94. doi: 10.3892/ijo.2013.2059
83. Hu J, Xu J, Wu Y, Chen Q, Zheng W, Lu X, et al. Identification of microRNA-93 as a functional dysregulated miRNA in triple-negative breast cancer. Tumor Biol. (2015) 36:251–8. doi: 10.1007/s13277-014-2611-8
84. Zhao F, Dou Y, Wang X, Han D, Lv Z, Ge S, et al. Serum microRNA-195 is down-regulated in breast cancer: a potential marker for the diagnosis of breast cancer. Mol Biol Rep. (2014) 41:5913–22. doi: 10.1007/s11033-014-3466-1
85. Kim S-J, Shin J-Y, Lee K-D, Bae Y-K, Sung KW, Nam SJ, et al. MicroRNA let-7a suppresses breast cancer cell migration and invasion through downregulation of C-C chemokine receptor type 7. Breast Cancer Res BCR. (2012) 14:R14. doi: 10.1186/bcr3098
86. Shukla K, Sharma AK, Ward A, Will R, Hielscher T, Balwierz A, et al. MicroRNA-30c-2-3p negatively regulates NF-κB signaling and cell cycle progression through downregulation of TRADD and CCNE1 in breast cancer. Mol Oncol. (2015) 9:1106–19. doi: 10.1016/j.molonc.2015.01.008
87. Jang K, Ahn H, Sim J, Han H, Abdul R, Paik SS, et al. Loss of microRNA-200a expression correlates with tumor progression in breast cancer. Transl Res. (2014) 163:242–51. doi: 10.1016/j.trsl.2013.11.005
88. Válóczi A, Hornyik C, Varga N, Burgyán J, Kauppinen S, Havelda Z. Sensitive and specific detection of microRNAs by northern blot analysis using LNA-modified oligonucleotide probes. Nucleic Acids Res. (2004) 32:e175. doi: 10.1093/nar/gnh171
89. Chen C, Ridzon DA, Broomer AJ, Zhou Z, Lee DH, Nguyen JT, et al. Real-time quantification of microRNAs by stem–loop RT–PCR. Nucleic Acids Res. (2005) 33:e179. doi: 10.1093/nar/gni178
90. Kang T, Zhu J, Luo X, Jia W, Wu P, Cai C. Controlled self-assembly of a close-packed gold octahedra array for SERS sensing exosomal microRNAs. Anal Chem. (2021) 93:2519–26. doi: 10.1021/acs.analchem.0c04561
91. Si Y, Xu L, Wang N, Zheng J, Yang R, Li J. Target microRNA-responsive DNA hydrogel-based surface-enhanced raman scattering sensor arrays for microRNA-marked cancer screening. Anal Chem. (2020) 92:2649–55. doi: 10.1021/acs.analchem.9b04606
92. Wen S, Su Y, Dai C, Jia J, Fan G-C, Jiang L-P, et al. Plasmon coupling-enhanced raman sensing platform integrated with exonuclease-assisted target recycling amplification for ultrasensitive and selective detection of microRNA-21. Anal Chem. (2019) 91:12298–306. doi: 10.1021/acs.analchem.9b02476
93. Li Q, Lowey B, Sodroski C, Krishnamurthy S, Alao H, Cha H, et al. Cellular microRNA networks regulate host dependency of hepatitis C virus infection. Nat Commun. (2017) 8:1789. doi: 10.1038/s41467-017-01954-x
94. Wang Z, Ye S, Zhang N, Liu X, Wang M. Triggerable mutually amplified signal probe based SERS-microfluidics platform for the efficient enrichment and quantitative detection of miRNA. Anal Chem. (2019) 91:5043–50. doi: 10.1021/acs.analchem.8b05172
95. Roush S, Slack FJ. The let-7 family of microRNAs. Trends Cell Biol. (2008) 18:505–16. doi: 10.1016/j.tcb.2008.07.007
96. Dong H, Lei J, Ding L, Wen Y, Ju H, Zhang X. MicroRNA: function, detection, and bioanalysis. Chem Rev. (2013) 113:6207–33. doi: 10.1021/cr300362f
97. Mitchell PS, Parkin RK, Kroh EM, Fritz BR, Wyman SK, Pogosova-Agadjanyan EL, et al. Circulating microRNAs as stable blood-based markers for cancer detection. Proc Natl Acad Sci. (2008) 105:10513–8. doi: 10.1073/pnas.0804549105
98. Williams Z, Ben-Dov IZ, Elias R, Mihailovic A, Brown M, Rosenwaks Z, et al. Comprehensive profiling of circulating microRNA via small RNA sequencing of cDNA libraries reveals biomarker potential and limitations. Proc Natl Acad Sci. (2013) 110:4255–60. doi: 10.1073/pnas.1214046110
99. Chin LK, Son T, Hong J-S, Liu A-Q, Skog J, Castro CM, et al. Plasmonic sensors for extracellular vesicle analysis: from scientific development to translational research. ACS Nano. (2020) 14:14528–48. doi: 10.1021/acsnano.0c07581
100. Xu T, Xu L-P, Zhang X, Wang S. Bioinspired superwettable micropatterns for biosensing. Chem Soc Rev. (2019) 48:3153–65. doi: 10.1039/C8CS00915E
101. Xu T, Shi W, Huang J, Song Y, Zhang F, Xu L-P, et al. Superwettable microchips as a platform toward microgravity biosensing. ACS Nano. (2017) 11:621–6. doi: 10.1021/acsnano.6b06896
102. De Angelis F, Gentile F, Mecarini F, Das G, Moretti M, Candeloro P, et al. Breaking the diffusion limit with super-hydrophobic delivery of molecules to plasmonic nanofocusing SERS structures. Nat Photonics. (2011) 5:682–7. doi: 10.1038/nphoton.2011.222
103. Lu L-Q, Zheng Y, Qu W-G, Yu H-Q, Xu A-W. Hydrophobic Teflon films as concentrators for single-molecule SERS detection. J Mater Chem. (2012) 22:20986–90. doi: 10.1039/c2jm33955b
104. Song X, Xu T, Song Y, He X, Wang D, Liu C, et al. Droplet array for open-channel high-throughput SERS biosensing. Talanta. (2020) 218:121206. doi: 10.1016/j.talanta.2020.121206
105. Lee JU, Kim WH, Lee HS, Park KH, Sim SJ. Quantitative and specific detection of exosomal miRNAs for accurate diagnosis of breast cancer using a surface-enhanced raman scattering sensor based on plasmonic head-flocked gold nanopillars. Small. (2019) 15:1804968. doi: 10.1002/smll.201804968
106. Hu M, Ou FS, Wu W, Naumov I, Li X, Bratkovsky AM, et al. Gold nanofingers for molecule trapping and detection. J Am Chem Soc. (2010) 132:12820–2. doi: 10.1021/ja105248h
107. Park S-G, Mun C, Xiao X, Braun A, Kim S, Giannini V, et al. Surface energy-controlled SERS substrates for molecular concentration at plasmonic nanogaps. Adv Funct Mater. (2017) 27:1703376. doi: 10.1002/adfm.201703376
108. Lee JM, Jung Y. Two-temperature hybridization for microarray detection of label-free microRNAs with attomole detection and superior specificity. Angew Chem Int Ed. (2011) 50:12487–90. doi: 10.1002/anie.201105605
109. Wang K, Sun Z, Feng M, Liu A, Yang S, Chen Y, et al. Design of a sandwich-mode amperometric biosensor for detection of PML/RARα fusion gene using locked nucleic acids on gold electrode. Biosens Bioelectron. (2011) 26:2870–6. doi: 10.1016/j.bios.2010.11.030
110. Novara C, Chiadò A, Paccotti N, Catuogno S, Esposito CL, Condorelli G, et al. SERS-active metal-dielectric nanostructures integrated in microfluidic devices for label-free quantitative detection of miRNA. Faraday Discuss. (2017) 205:271–89. doi: 10.1039/C7FD00140A
111. Xu W, Zhang Y, Hou D, Shen J, Dong J, Gao Z, et al. Quantitative SERS detection of multiple breast cancer miRNAs based on duplex specific nuclease-mediated signal amplification. Anal Methods. (2023) 15:2915–24. doi: 10.1039/D3AY00583F
112. Zhang S, Liu R, Xing Z, Zhang S, Zhang X. Multiplex miRNA assay using lanthanide-tagged probes and the duplex-specific nuclease amplification strategy. Chem Commun. (2016) 52:14310–3. doi: 10.1039/C6CC08334J
113. Weng S, Lin D, Lai S, Tao H, Chen T, Peng M, et al. Highly sensitive and reliable detection of microRNA for clinically disease surveillance using SERS biosensor integrated with catalytic hairpin assembly amplification technology. Biosens Bioelectron. (2022) 208:114236. doi: 10.1016/j.bios.2022.114236
114. Shagin DA, Rebrikov DV, Kozhemyako VB, Altshuler IM, Shcheglov AS, Zhulidov PA, et al. A novel method for SNP detection using a new duplex-specific nuclease from crab hepatopancreas. Genome Res. (2002) 12:1935–42. doi: 10.1101/gr.547002
115. Meng S, Chen R, Xie J, Li J, Cheng J, Xu Y, et al. Surface-enhanced Raman scattering holography chip for rapid, sensitive and multiplexed detection of human breast cancer-associated MicroRNAs in clinical samples. Biosens Bioelectron. (2021) 190:113470. doi: 10.1016/j.bios.2021.113470
116. Wu L, Wang Z, Fan K, Zong S, Cui Y. A SERS-assisted 3D barcode chip for high-throughput biosensing. Small. (2015) 11:2798–806. doi: 10.1002/smll.201403474
117. Zhang K, Lv S, Lin Z, Tang D. CdS: Mn quantum dot-functionalized g-C3N4 nanohybrids as signal-generation tags for photoelectrochemical immunoassay of prostate specific antigen coupling DNAzyme concatamer with enzymatic biocatalytic precipitation. Biosens Bioelectron. (2017) 95:34–40. doi: 10.1016/j.bios.2017.04.005
118. Zhang J, Zhang H, Ye S, Wang X, Ma L. Fluorescent-raman binary star ratio probe for microRNA detection and imaging in living cells. Anal Chem. (2021) 93:1466–71. doi: 10.1021/acs.analchem.0c03491
119. Wang H-N, Crawford BM, Fales AM, Bowie ML, Seewaldt VL, Vo-Dinh T. Multiplexed detection of microRNA biomarkers using SERS-based inverse molecular sentinel (iMS) nanoprobes. J Phys Chem C Nanomater Interfaces. (2016) 120:21047–50. doi: 10.1021/acs.jpcc.6b03299
120. Zheng Z, Wu L, Li L, Zong S, Wang Z, Cui Y. Simultaneous and highly sensitive detection of multiple breast cancer biomarkers in real samples using a SERS microfluidic chip. Talanta. (2018) 188:507–15. doi: 10.1016/j.talanta.2018.06.013
121. Wong PK, Chen C-Y, Wang T-H, Ho C-M. Electrokinetic bioprocessor for concentrating cells and molecules. Anal Chem. (2004) 76:6908–14. doi: 10.1021/ac049479u
122. Green NG, Ramos A, González A, Castellanos A, Morgan H. Electrothermally induced fluid flow on microelectrodes. J Electrost. (2001) 53:71–87. doi: 10.1016/S0304-3886(01)00132-2
123. Kang T, Kim H, Lee JM, Lee H, Choi Y-S, Kang G, et al. Ultra-specific zeptomole microRNA detection by plasmonic nanowire interstice sensor with bi-temperature hybridization. Small. (2014) 10:4200–6. doi: 10.1002/smll.201400164
124. Zhang S, Wu SQY, Hum M, Perumal J, Tan EY, Lee ASG, et al. Complete characterization of RNA biomarker fingerprints using a multi-modal ATR-FTIR and SERS approach for label-free early breast cancer diagnosis. RSC Adv. (2024) 14:3599–610. doi: 10.1039/D3RA05723B
125. Kim J, Park J, Ki J, Rho HW, Huh Y-M, Kim E, et al. Simultaneous dual-targeted monitoring of breast cancer circulating miRNA via surface-enhanced Raman spectroscopy. Biosens Bioelectron. (2022) 207:114143. doi: 10.1016/j.bios.2022.114143
126. Liu J, Zheng T, Tian Y. Functionalized h-BN nanosheets as a theranostic platform for SERS real-time monitoring of microRNA and photodynamic therapy. Angew Chem Int Ed. (2019) 58:7757–61. doi: 10.1002/anie.201902776
127. Ling X, Fang W, Lee Y-H, Araujo PT, Zhang X, Rodriguez-Nieva JF, et al. Raman enhancement effect on two-dimensional layered materials: graphene, h-BN and moS2. Nano Lett. (2014) 14:3033–40. doi: 10.1021/nl404610c
128. Ma Y, Li X, Li A, Yang P, Zhang C, Tang B. H2S-activable MOF nanoparticle photosensitizer for effective photodynamic therapy against cancer with controllable singlet-oxygen release. Angew Chem Int Ed. (2017) 56:13752–6. doi: 10.1002/anie.201708005
129. Lan G, Ni K, Xu R, Lu K, Lin Z, Chan C, et al. Nanoscale metal-organic layers for deeply penetrating X-ray induced photodynamic therapy. Angew Chem Int Ed Engl. (2017) 56:12102–6. doi: 10.1002/anie.201704828
130. Dixon AR, Bathany C, Tsuei M, White J, Barald KF, Takayama S. Recent developments in multiplexing techniques for immunohistochemistry. Expert Rev Mol Diagn. (2015) 15:1171–86. doi: 10.1586/14737159.2015.1069182
131. Cornejo KM, Kandil D, Khan A, Cosar EF. Theranostic and molecular classification of breast cancer. Arch Pathol Lab Med. (2014) 138:44–56. doi: 10.5858/arpa.2012-0442-RA
132. Murali VP, Karunakaran V, Murali M, Lekshmi A, Kottarathil S, Deepika S, et al. A clinically feasible diagnostic spectro-histology built on SERS-nanotags for multiplex detection and grading of breast cancer biomarkers. Biosens Bioelectron. (2023) 227:115177. doi: 10.1016/j.bios.2023.115177
133. Xie Y, Wen Y, Su X, Zheng C, Li M. Label-free plasmon-enhanced spectroscopic HER2 detection for dynamic therapeutic surveillance of breast cancer. Anal Chem. (2022) 94:12762–71. doi: 10.1021/acs.analchem.2c02419
134. Mo W, Ke Q, Zhou M, Xie G, Huang J, Gao F, et al. Combined morphological and spectroscopic diagnostic of HER2 expression in breast cancer tissues based on label-free surface-enhanced raman scattering. Anal Chem. (2023) 95:3019–27. doi: 10.1021/acs.analchem.2c05067
135. Verdin A, Malherbe C, Eppe G. Spatially resolved determination of the abundance of the HER2 marker in microscopic breast tumors using targeted SERS imaging. Microchim Acta. (2021) 188:288. doi: 10.1007/s00604-021-04943-6
136. Téllez-Plancarte A, Haro-Poniatowski E, Picquart M, Morales-Méndez JG, Lara-Cruz C, Jiménez-Salazar JE, et al. Development of a nanostructured platform for identifying HER2-heterogeneity of breast cancer cells by surface-enhanced raman scattering. Nanomaterials. (2018) 8:549. doi: 10.3390/nano8070549
137. Kapara A, Brunton VG, Graham D, Faulds K. Characterisation of estrogen receptor alpha (ERα) expression in breast cancer cells and effect of drug treatment using targeted nanoparticles and SERS. Analyst. (2020) 145:7225–33. doi: 10.1039/D0AN01532F
138. Gonzalez-Avila G, Sommer B, Mendoza-Posada DA, Ramos C, Garcia-Hernandez AA, Falfan-Valencia R. Matrix metalloproteinases participation in the metastatic process and their diagnostic and therapeutic applications in cancer. Crit Rev Oncol Hematol. (2019) 137:57–83. doi: 10.1016/j.critrevonc.2019.02.010
139. Kessenbrock K, Plaks V, Werb Z. Matrix metalloproteinases: regulators of the tumor microenvironment. Cell. (2010) 141:52–67. doi: 10.1016/j.cell.2010.03.015
140. Lodillinsky C, Fuhrmann L, Irondelle M, Pylypenko O, Li X-Y, Bonsang-Kitzis H, et al. Metastasis-suppressor NME1 controls the invasive switch of breast cancer by regulating MT1-MMP surface clearance. Oncogene. (2021) 40:4019–32. doi: 10.1038/s41388-021-01826-1
141. Nakopoulou L, Tsirmpa I, Alexandrou P, Louvrou A, Ampela C, Markaki S, et al. MMP-2 protein in invasive breast cancer and the impact of MMP-2/TIMP-2 phenotype on overall survival. Breast Cancer Res Treat. (2003) 77:145–55. doi: 10.1023/A:1021371028777
142. Stawarski M, Rutkowska-Wlodarczyk I, Zeug A, Bijata M, Madej H, Kaczmarek L, et al. Genetically encoded FRET-based biosensor for imaging MMP-9 activity. Biomaterials. (2014) 35:1402–10. doi: 10.1016/j.biomaterials.2013.11.033
143. Wu L, Wang Z, Zhang Y, Fei J, Chen H, Zong S, et al. In situ probing of cell–cell communications with surface-enhanced Raman scattering (SERS) nanoprobes and microfluidic networks for screening of immunotherapeutic drugs. Nano Res. (2017) 10:584–94. doi: 10.1007/s12274-016-1316-2
144. Zhong Q, Zhang K, Huang X, Lu Y, Zhao J, He Y, et al. In situ ratiometric SERS imaging of intracellular protease activity for subtype discrimination of human breast cancer. Biosens Bioelectron. (2022) 207:114194. doi: 10.1016/j.bios.2022.114194
145. Zhu D, Li A, Di Y, Wang Z, Shi J, Ni X, et al. Interference-free SERS nanoprobes for labeling and imaging of MT1-MMP in breast cancer cells. Nanotechnology. (2021) 33:115702. doi: 10.1088/1361-6528/ac4065
146. Liu X, Gu J, Wang J, Zhang W, Wang Y, Xu Z. Cell membrane-anchored SERS biosensor for the monitoring of cell-secreted MMP-9 during cell–cell communication. ACS Sens. (2023) 8:4307–14. doi: 10.1021/acssensors.3c01663
147. Lis C, Grutsch J, Vashi P, Lammersfeld C. Is serum albumin an independent predictor of survival in patients with breast cancer? J Parenter Enter Nutr. (2003) 27:10–5. doi: 10.1177/014860710302700110
148. Lin J, Chen R, Feng S, Pan J, Li Y, Chen G, et al. A novel blood plasma analysis technique combining membrane electrophoresis with silver nanoparticle-based SERS spectroscopy for potential applications in noninvasive cancer detection. Nanomed Nanotechnol Biol Med. (2011) 7:655–63. doi: 10.1016/j.nano.2011.01.012
149. Wang J, Lin D, Lin J, Yu Y, Huang Z, Chen Y, et al. Label-free detection of serum proteins using surface-enhanced Raman spectroscopy for colorectal cancer screening. J Biomed Opt. (2014) 19:87003. doi: 10.1117/1.JBO.19.8.087003
150. Yu Y, Lin Y, Xu C, Lin K, Ye Q, Wang X, et al. Label-free detection of nasopharyngeal and liver cancer using surface-enhanced Raman spectroscopy and partial lease squares combined with support vector machine. Biomed Opt Express. (2018) 9:6053–66. doi: 10.1364/BOE.9.006053
151. Lin Y, Gao S, Zheng M, Tang S, Lin K, Xie S, et al. A microsphere nanoparticle based-serum albumin targeted adsorption coupled with surface-enhanced Raman scattering for breast cancer detection. Spectrochim Acta A Mol Biomol Spectrosc. (2021) 261:120039. doi: 10.1016/j.saa.2021.120039
152. Davis RM, Campbell JL, Burkitt S, Qiu Z, Kang S, Mehraein M, et al. A raman imaging approach using CD47 antibody-labeled SERS nanoparticles for identifying breast cancer and its potential to guide surgical resection. Nanomater Basel Switz. (2018) 8:953. doi: 10.3390/nano8110953
153. Kubo A, Masugi Y, Hase T, Nagashima K, Kawai Y, Takizawa M, et al. Polysulfide serves as a hallmark of desmoplastic reaction to differentially diagnose ductal carcinoma in situ and invasive breast cancer by SERS imaging. Antioxidants. (2023) 12:240. doi: 10.3390/antiox12020240
154. Cheng B, Xie R, Dong L, Chen X. Metabolic remodeling of cell-surface sialic acids: principles, applications, and recent advances. ChemBioChem. (2016) 17:11–27. doi: 10.1002/cbic.201500344
155. Liang L, Shen Y, Zhang J, Xu S, Xu W, Liang C, et al. Identification of breast cancer through spectroscopic analysis of cell-membrane sialic acid expression. Anal Chim Acta. (2018) 1033:148–55. doi: 10.1016/j.aca.2018.04.072
156. Hernández-Arteaga AC, de Jesús Zermeño-Nava J, Martínez-Martínez MU, Hernández-Cedillo A, Ojeda-Galván HJ, José-Yacamán M, et al. Determination of salivary sialic acid through nanotechnology: A useful biomarker for the screening of breast cancer. Arch Med Res. (2019) 50:105–10. doi: 10.1016/j.arcmed.2019.05.013
Keywords: SERS, biomarker, liquid biopsy, breast cancer, early diagnosis
Citation: Liu X, Jia Y and Zheng C (2024) Recent progress in Surface-Enhanced Raman Spectroscopy detection of biomarkers in liquid biopsy for breast cancer. Front. Oncol. 14:1400498. doi: 10.3389/fonc.2024.1400498
Received: 13 March 2024; Accepted: 24 June 2024;
Published: 08 July 2024.
Edited by:
Ana Maria Da Costa Ferreira, University of São Paulo, BrazilReviewed by:
Zufang Huang, Fujian Normal University, ChinaCopyright © 2024 Liu, Jia and Zheng. This is an open-access article distributed under the terms of the Creative Commons Attribution License (CC BY). The use, distribution or reproduction in other forums is permitted, provided the original author(s) and the copyright owner(s) are credited and that the original publication in this journal is cited, in accordance with accepted academic practice. No use, distribution or reproduction is permitted which does not comply with these terms.
*Correspondence: Chao Zheng, Y2hhb3poZW5nQHNkdS5lZHUuY24=
†ORCID: Chao Zheng, orcid.org/0000-0003-2075-0186
Disclaimer: All claims expressed in this article are solely those of the authors and do not necessarily represent those of their affiliated organizations, or those of the publisher, the editors and the reviewers. Any product that may be evaluated in this article or claim that may be made by its manufacturer is not guaranteed or endorsed by the publisher.
Research integrity at Frontiers
Learn more about the work of our research integrity team to safeguard the quality of each article we publish.