- 1Department of Medical Imaging, Xuzhou Clinical School of Xuzhou Medical University, Xuzhou, China
- 2Department of Radiology, Xuzhou Central Hospital, Xuzhou, China
- 3Department of Radiology, Xuzhou Universal Medical Imaging Diagnostic Center, Xuzhou, China
- 4Department of Radiology, Affiliated Hospital of Xuzhou Medical University, Xuzhou, China
Background: Cervical cancer (CC) remains the second leading cause of cancer-related death in women, and the ability to accurately anticipate the presence or absence of lymphovascular space invasion (LVSI) is critical to maintaining optimal patient outcomes. The objective of this study was to establish and verify an MRI radiomics-based model to predict the status of LVSI in patients with operable CC.
Methods: The current study performed a retrospective analysis, with 86 patients in the training cohort and 38 patients in the testing group, specifically focusing on patients with CC. The radiomics feature extraction process included ADC, T2WI-SPAIR, and T2WI sequences. The training group data were used for the initial radionics-based model building, and the model predictive performance was subsequently validated using data from patients recruited in the experimental group.
Results: The development of the radiomics scoring model has been completed with 17 selected features. The study found several risk factors associated with LVSI. These risk factors included moderate tumor differentiation (P = 0.005), poor tumor differentiation (P = 0.001), and elevated combined sequence-based radiomics scores (P = 0.001). Radiomics scores based on predictive model, combined sequences, ADC, T2WI-SPAIR, and T2WI exhibited AUCs of 0.897, 0.839, 0.815, 0.698, and 0.739 in the training cohort, respectively, with corresponding testing cohort values of 0.833, 0.833, 0.683, 0.692, and 0.725. Excellent consistency was shown by the calibration curve analysis, which showed a higher degree of agreement between the actual and anticipated LVSI status. Moreover, the decision curve analysis outcomes demonstrated the medical application of this prediction model.
Conclusion: This investigation indicated that the MRI radiomics model was successfully developed and validated to predict operable CC patient LVSI status, attaining high overall diagnostic accuracy. However, further external validation and more deeper analysis on a larger sample size are still needed.
Introduction
At the global level, cervical cancer (CC) remains the second deadliest form of cancer among women (1), with an estimated 530,000 diagnoses and 250,000 deaths annually (2). Surprisingly, patients in underdeveloped countries account for almost 80% of CC-related mortality (2). Despite recent therapy advancements, long-term CC patient outcomes are poor (3).
The occurrence of lymph node (LN) metastasis (LNM) is strongly connected with the prognosis of CC patients (4–6), and evidence of lymphovascular space invasion (LVSI) is significantly related to the LNM status of a given page. In patients with early-stage CC without LNM, positive LVSI status implies the necessity for lymph node dissection (7). Postoperative radiotherapy is also recommended in some LVSI-positive CC cases as per the Sedlis criteria (8). The ability to preoperatively gauge the LVSI status of a given patient is thus vital to support therapeutic decision-making. However, only postoperative pathology investigations may now confirm the presence of LVSI. Magnetic resonance imaging (MRI) techniques commonly diagnose CC because they provide excellent soft tissue resolution. However, standard MRI scans are poorly suited for diagnosing patient LVSI status (9–11).
Radiomics provides an alternate method for characterizing microscopic, invisible features to the human eye by analyzing clinical images to obtain high-dimensional quantitative data (12). MRI radiomics approaches have been deployed to predict tumor staging, LNM status, and therapeutic efficacy in patients with CC (4, 13–15). Currently, there have been no MRI radiomics studies that have explored investigated the ability to predict the status of LVSI in cervical cancer patients before surgery.
In order to fill this knowledge gap, the current study was conducted to develop and validate an MRI radiomics-based model that can accurately predict the state of LVSI in patients with operable CC.
Study methodology
Study design
This retrospective study was approved by the Ethics Committee of Xuzhou Central Hospital, with the need for informed consent being waived by the ethics committee. The training group consisted of 86 consecutive patients with CC who underwent an MRI examination from June 2016 to June 2021. Additionally, a separate experimental group comprised 38 consecutive CC patients who underwent MRI evaluation between July 2021 and October 2022.
The inclusion criteria were set as follows: (1) patients who were preoperatively diagnosed with CC by hysteroscopy, (2) patients who had MRI scans performed within 7 days before surgical resection, (3) patients who underwent hysterectomy with pelvic LN dissection, and (4) the LVSI status confirmed by pathological examination. Patients were excluded from these analyses if: (1) their clinical data were not complete, (2) the quality of images is poor, (3) they were pregnant patients, (4) they showed any other comorbid malignancies, or (5) they had undergone preoperative chemotherapy or radiotherapy.
The collected data from all participants encompassed baseline age, BMI, tumor differentiation, 2018 FIGO clinical staging, depth of invasion, and levels of serum tumor biomarkers (such as carcinoembryonic antigen [CEA], alpha-fetoprotein [AFP], carbohydrate antigen 199 [CA199], and squamous cell carcinoma antigen [SCC]).
MRI analyses
The MRI scanning procedure was conducted utilizing a 1.5T MRI apparatus manufactured by Philips, employing a body array coil known as Ingenia. Each patient underwent axial T2WI, fat suppression T2WI, axial diffusion-weighted imaging (DWI), sagittal T1WI, and sagittal T2WI sequence. The SPAIR sequences were employed to suppress fat. For further details concerning individual scanning protocols, see Supplementary Table 1.
Feature extraction
The axial ADC, axial T2WI-SPAIR, and Sagittal T2WI MRI results were analyzed with 3D Slicer (v 5.03) in which volumes of interest (VOIs) were defined by manually drawing the boundaries of target tumors (Figure 1). The VOIs were extracted by two radiologists, one with 10 years of experience and the other with 5 years of experience. The radiologists were uninformed about the pathological findings of the patients. Additionally, the 3D Slicer application was utilized for feature extraction, and the observer consistency was evaluated by considering both intra- and inter-class coefficient (ICC) values. Two radiologists independently segmented MRI images from a randomly selected training sample of 20 patients. In addition, one of these radiologists (Reader 1) repeated the segmentation of tumors from these same 20 patients 1-week after the initial segmentation. Features that demonstrated an ICC of at least ≥ 0.8 were deemed sufficiently reproducible and were kept for further examination. After that, Reader 1 segmented all remaining images.
Feature selection
A variance threshold method was first used to determine whether the characteristics had a variance > 0.75 to select features. After that, the Selec-K-Best approach was used for the retained characteristics, retaining those with a P-value of > 0.05. Lastly, factors associated with LVSI status were selected using a LASSO regression model.
Radiomics model formulation and testing
The selected characteristics were utilized to create a radiomics signature model, which allowed radiomics scores for each CC patient to be determined as follows:
Risk factors associated with the LVSI status of training cohort patients were selected via univariate and multivariate logistic regression approaches to enable the combined assessment of clinical features, serum biomarkers, and radiomics scores. These findings led to the development of a predictive nomogram, which was subsequently validated using the data from the testing cohort.
Statistical analyses
Data were analyzed with SPSS 25.0 and R 4.1.2, comparing categorical data with χ2 tests or Fisher’s exact test. Normally (non-normally) distributed continuous data were compared with Student’s t-tests (Mann-Whitney U tests). Several logistic regression analyses were used to determine risk factors associated with LVSI status. The area under the curve (AUC) values for generated receiver operating characteristic (ROC) curves were compared using the DeLong test.
Results
Experimental group
The experimental group consisted of 86 patients diagnosed with cervical cancer, with 62 testing negative and 24 testing positive for LVSI. The data from these patients are summarized in Table 1. The age, BMI, FIGO staging, pathological CC type, and serum tumor marker concentration distributions were similar across these groups. The differentiation level (P = 0.001) and cervical stromal invasion depth (P = 0.007) did, however, differ significantly between LVSI-positive and LVSI-negative patients.
Feature selection
A total of 851 radiomics characteristics were extracted for each sequence (T2WI, T2WI-SPAIR, ADC). The development of radiomics scores was then based on combining these three sequences. See Supplementary Table 2 for more information about the feature selection procedure. Ultimately, this strategy led to the selection of 17 features utilized for radiomics score calculations (Supplementary Figure 1). The corresponding coefficient values for these features and the combined sequence-based mean square errors are shown in Supplementary Figure 2.
Predictive model development
In univariate and multivariate analyses, factors associated with LVSI included elevated combined sequence-based radiomics scores (P = 0.001), moderate differentiation (P = 0.005), and a poor differentiation (P = 0.001). The correlation between these parameters and the incidence of LVSI was validated by multivariate analyses (Table 2).
Next, a nomogram and predictive model were developed based on these results (Figure 2). The formula used to determine nomogram scores was as follows: score = 0.5202 - 1.9712×differentiation level (0: poor; 1: moderate; 2: well) ++ 5.6740 × combined sequence radiomics score.
Analyzing CC patients in the training cohort, the prediction model showed an AUC of 0.897 and corresponding sensitivity and specificity values of 87.5% and 82.3% (Figure 3A, Table 3). This AUC value was higher than corresponding values based solely on radiomics scores based upon the T2WI (0.739), T2WI-SPAIR (0.698), ADC (0.815), and combined sequence (0.839) features (P = 0.006, 0.004, 0.067, and 0.144).
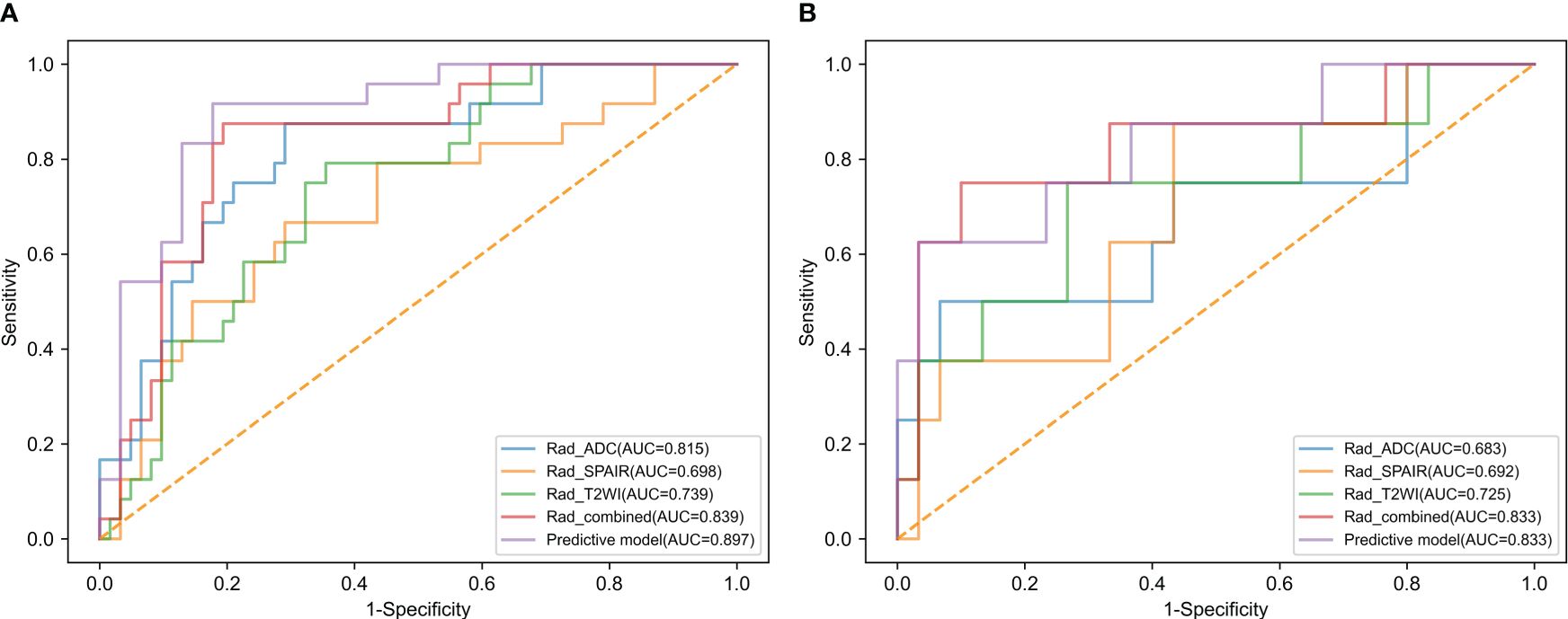
Figure 3 The ROC curves of radiomics score of T2WI, radiomics score of T2WI-SPAIR, radiomics score of ADC, radiomics score of combined sequences, and the predictive model in the (A) training and (B) test groups.
Testing cohort validation
The testing cohort consisted of 38 patients with CC, with 30 patients with LVSI-negative disease and 8 with LVSI-positive disease. Among the baseline parameters examined, the only significant difference observed between these two groups of patients was in the depth of cervical stromal invasion (Table 1). When the data from these patients were introduced into the developed predictive model, the respective AUC, sensitivity, and specificity values were 0.833, 75.0%, and 76.7% (Figure 3B, Table 3). While the predictive model-derived AUC value was higher than corresponding values for T2WI (0.725), T2WI-SPAIR (0.692), ADC (0.683), and combined sequence (0.833) based radiomics scores, these differences were not statistically significant (P = 0.293, 0.069, 0.137, and 1.000, respectively).
Evaluation of predictive model clinical utility
The calibration curve analysis demonstrated a high level of agreement between the model-predicted LVSI status of CC patients and their actual LVSI status in both the testing and training cohorts (Figure 4). Upon doing a decision curve analysis for this predictive model, it was found that the model produced significant net benefits in both the experimental and control groups. The risk thresholds for these groups were determined to be 0–0.84 and 0–0.36, respectively (Figure 5).
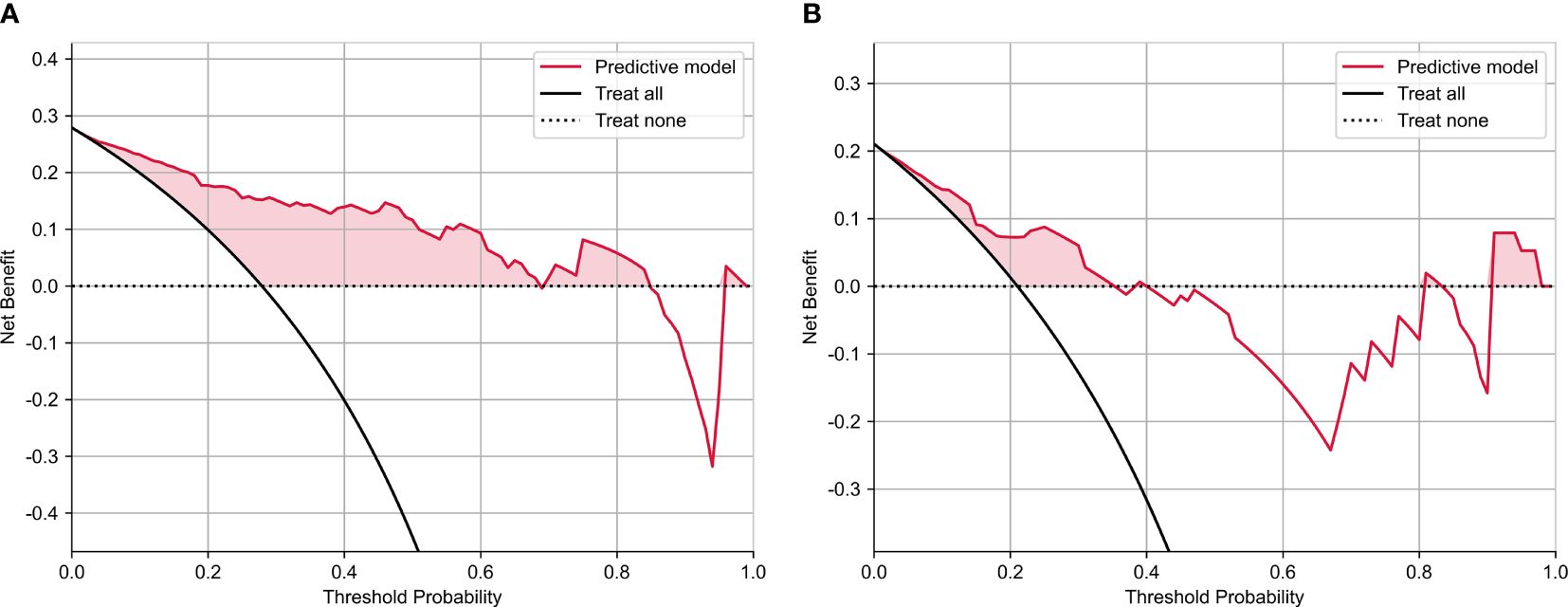
Figure 5 The decision curve analysis of nomograms of predictive model for (A) training and (B) test groups.
Discussion
In this study, a model based on MRI radiomics was effectively created and validated as a predictive tool for the occurrence of lymphovascular space invasion (LVSI) in cervical cancer patients. A nomogram was ultimately established by combining MRI-based radiomics scores and relevant clinical features, and the resultant model exhibited high AUC values in training and testing cohorts consisting of individuals with CC (0.897 and 0.833, respectively). The data reported in this study suggest that the examined model can effectively guide healthcare decisions and treatment planning for patients with CC.
The ability to preoperatively assess LVSI status is critical in patients with early-stage CC because the presence or absence of LVSI determines the primary therapeutic strategy for individuals with clinical-stage IA disease (16–21). According to the National Comprehensive Cancer Network guidelines, surgery is often the main treatment for the early-stage CC (20). For the stage IA patients with LVSI(-), only cervical conization can be performed, thus avoiding radical hysterectomy, with the preservation of fertility (20). Furthermore, during the surgery for CC, LN dissection should be usually performed in order to test the LNM status (20, 21). LVSI was found to be a risk factor for LNM (19), Therefore, preoperative assessment of LVSI status may help to make the treatment decision. In addition, LVSI status was also found to be the risk factor for parametrial involvement and tumor recurrence (19). Therefore, preoperative evaluations of LVSI status is also important in the aspect of prognosis assessment.
Efficient evaluation of the appearance, anatomy, and molecular mobility of tumors can be achieved using conventional MRI scanning (18). However, since these scans cannot assess microscopic tumor pathology features, they are not well adapted for LVSI detection (17). Moreover, no direct MRI characteristic related to LVSI has been found.
Conventional pictures can be examined to derive radiomics features, which provide a means of evaluating tumors in greater depth and that correspond to a wide range of biological properties (17). Li et al. (19) previously developed an MRI radiomics-based model to predict CC patient LSVI status, but the AUC values for their training and testing cohorts were just 0.754 and 0.727. The AUC values in the prior study were likely lower than those in the present analysis since they only used T1WI sequences for feature extraction (19).
This study used a combination of many MRI sequences to extract radiomics features. The constructed predictive model yielded an AUC value of 0.897 when assessing patients in the training group. In addition, the sensitivity and specificity values were calculated to be 87.5% and 82.3%, respectively. The present study demonstrates the significance of a combined predictive radiomics scoring model, which surpasses the performance of radiomics scores produced from separate MRI sequences, as indicated by a more excellent AUC value. Utilizing multimodal MRI radiomics features can provide a more comprehensive understanding of the biological condition of a specific tumor compared to any single MRI sequence. The ultimate predictive model also included the differentiation level as the clinical characteristic strongly associated with the extent of CC malignancy. The model showed improved accuracy in predicting the LVSI status of CC patients by including these variables. The experimental group did not demonstrate a statistically significant increase in the AUC for the prediction model compared to those obtained from radiomics scores derived from the concatenated sequences, specifically ADC, T2WI, SPAIR, or T2WI. This can be attributed to the reduced sample size in this testing group.
This study has some limitations. Firstly, utilizing a retrospective patient group carries a significant potential for bias, underscoring the importance of doing prospective validation. Secondly, the limited number of patients recruited in the study restricts the statistical strength of these results. Because of this limitation, the detailed FIGO stages could not be divided and the FIGO stage was not associated with the LVSI status. Thirdly, the developed predictive model was based on a logistic regression analysis, and it may not be the most optimal predictive model given that machine learning models such as k-nearest neighbors, support vector machine, XG boost, random forest, or light GBM approaches were not employed. Lastly, LVSI status can be divided into the focal and diffused LVSI (19). However, we did not conduct any subgroup analysis for differentiating the focal and diffused LVSI due to the limited sample size. Further studies should be conducted to predict the diffused LVSI in CC patients.
Conclusions
Overall, a radiomics-based model using MRI was successfully developed and utilized to predict the condition of LVSI in operable CC patients, showing a high level of diagnostic accuracy in all participants.
Data availability statement
The original contributions presented in the study are included in the article/Supplementary Material. Further inquiries can be directed to the corresponding author.
Ethics statement
The studies involving humans were approved by Ethics Committee of Xuzhou Central Hospital. The studies were conducted in accordance with the local legislation and institutional requirements. The ethics committee/institutional review board waived the requirement of written informed consent for participation from the participants or the participants’ legal guardians/next of kin because this is a retrospective study.
Author contributions
N-NM: Data curation, Formal analysis, Writing – original draft. TW: Methodology, Software, Writing – review & editing. Y-NL: Methodology, Software, Writing – review & editing. S-DL: Conceptualization, Supervision, Writing – review & editing.
Funding
The author(s) declare that no financial support was received for the research, authorship, and/or publication of this article.
Conflict of interest
The authors declare that the research was conducted in the absence of any commercial or financial relationships that could be construed as a potential conflict of interest.
Publisher’s note
All claims expressed in this article are solely those of the authors and do not necessarily represent those of their affiliated organizations, or those of the publisher, the editors and the reviewers. Any product that may be evaluated in this article, or claim that may be made by its manufacturer, is not guaranteed or endorsed by the publisher.
Supplementary material
The Supplementary Material for this article can be found online at: https://www.frontiersin.org/articles/10.3389/fonc.2024.1394427/full#supplementary-material
Supplementary Figure 1 | Radiomics features and their coefficients.
Supplementary Figure 2 | The (A) coefficient of each feature and (B) mean square error of the combined sequences.
Abbreviations
ADC, apparent diffusion coefficient; AFP, alpha-fetoprotein; AUC, area under curve; CA199, carbohydrate antigen 199; CC, cervical cancer; CEA, carcinoembryonic antigen; DWI, diffused weighted imaging; FIGO, International Federation of Gynecology and Obstetrics; LASSO, least absolute shrinkage and selection operator; LNM, lymph node metastasis; LVSI, lymphovascular space invasion; MRI, magnetic resonance imaging; ROC, receiver operator characteristic; SCC, squamous cell carcinoma antigen; VOI, volume of interest.
References
1. Siegel RL, Miller KD, Jemal A. Cancer statistics, 2019. CA Cancer J Clin. (2019) 69:7–34. doi: 10.3322/caac.21551
2. Bray F, Ferlay J, Soerjomataram I, Siegel RL, Torre LA, Jemal A Global cancer statistics 2018: GLOBOCAN estimates of incidence and mortality worldwide for 36 cancers in 185 countries. CA Cancer J Clin. (2018) 68:394–424. doi: 10.3322/caac.21492
3. Togami S, Kamio M, Kobayashi H, Douchi T. Is it possible to perform less radical surgery for invasive uterine cervical cancer? Gynecol Obstet Invest. (2016) 81:251–5. doi: 10.1159/000439528
4. Xiao M, Ma F, Li Y, Li Y, Li M, Zhang G, et al. Multiparametric MRI-based radiomics nomogram for predicting lymph node metastasis in early-stage cervical cancer. J Magn Reson Imaging. (2020) 52:885–96. doi: 10.1002/jmri.27101
5. Kan Y, Dong D, Zhang Y, Jiang W, Zhao N, Han L, et al. Radiomic signature as a predictive factor for lymph node metastasis in early-stage cervical cancer. J Magn Reson Imaging. (2019) 49:304–10. doi: 10.1002/jmri.26209
6. Deng YR, Chen XJ, Xu CQ, Wu QZ, Zhang W, Guo SQ, et al. A preoperative nomogram predicting risk of lymph node metastasis for early-stage cervical cancer. BMC Women's Health. (2023) 23:568. doi: 10.1186/s12905-023-02726-0
7. Wu Y, Wang S, Chen Y, Liao Y, Yin X, Li T, et al. A multicenter study on preoperative assessment of lymphovascular space invasion in early-stage cervical cancer based on multimodal MR radiomics. J Magn Reson Imaging. (2023) 58:1638–48. doi: 10.1002/jmri.28676
8. Haesen J, Salihi R, Van Gorp T, Van Nieuwenhuysen E, Han SN, Christiaens M, et al. Radical hysterectomy without adjuvant radiotherapy in patients with cervix carcinoma FIGO 2009 IB1, with or without positive Sedlis criteria. Gynecol Oncol. (2021) 162:539–45. doi: 10.1016/j.ygyno.2021.06.026
9. Merz J, Bossart M, Bamberg F, Eisenblaetter M. Revised FIGO staging for cervical cancer - A new role for MRI. Rofo. (2020) 192:937–44. doi: 10.1055/a-1198-5729
10. Balcacer P, Shergill A, Litkouhi B. MRI of cervical cancer with a surgical perspective: staging, prognostic implications and pitfalls. Abdom Radiol (NY). (2019) 44:2557–71. doi: 10.1007/s00261-019-01984-7
11. Re GL, Cucinella G, Zaccaria G, Crapanzano A, Salerno S, Pinto A, et al. Role of MRI in the assessment of cervical cancer. Semin Ultrasound CT MR. (2023) 44:228–37. doi: 10.1053/j.sult.2023.03.010
12. Mayerhoefer ME, Materka A, Langs G, Häggström I, Szczypiński P, Gibbs P, et al. Introduction to radiomics. J Nucl Med. (2020) 61:488–95. doi: 10.2967/jnumed.118.222893
13. Zhao X, Wang X, Zhang B, Liu X, Xuan D, Xia Y, et al. Classifying early stages of cervical cancer with MRI-based radiomics. Magn Reson Imaging. (2022) 89:70–6. doi: 10.1016/j.mri.2022.03.002
14. Shi J, Dong Y, Jiang W, Qin F, Wang X, Cui L, et al. MRI-based peritumoral radiomics analysis for preoperative prediction of lymph node metastasis in early-stage cervical cancer: A multi-center study. Magn Reson Imaging. (2022) 88:1–888. doi: 10.1016/j.mri.2021.12.008
15. Zhang Y, Zhang K, Jia H, Xia B, Zang C, Liu Y, et al. IVIM-DWI and MRI-based radiomics in cervical cancer: Prediction of concurrent chemoradiotherapy sensitivity in combination with clinical prognostic factors. Magn Reson Imaging. (2022) 91:37–44. doi: 10.1016/j.mri.2022.05.005
16. Bhatla N, Aoki D, Sharma DN, Sankaranarayanan R. Cancer of the cervix uteri. Int J Gynaecol Obstet. (2018) 143:22–36. doi: 10.1002/ijgo.12611
17. Luo Y, Mei D, Gong J, Zuo M, Guo X. Multiparametric MRI-based radiomics nomogram for predicting lymphovascular space invasion in endometrial carcinoma. J Magn Reson Imaging. (2020) 52:1257–62. doi: 10.1002/jmri.27142
18. Zhang A, Song J, Ma Z, Chen T. Application of apparent diffusion coefficient values derived from diffusion-weighted imaging for assessing different sized metastatic lymph nodes in cervical cancers. Acta Radiol. (2020) 61:848–55. doi: 10.1177/0284185119879686
19. Ronsini C, Anchora LP, Restaino S, Fedele C, Arciuolo D, Teodorico E, et al. The role of semiquantitative evaluation of lympho-vascular space invasion in early stage cervical cancer patients. Gynecol Oncol. (2021) 162:299–307. doi: 10.1016/j.ygyno.2021.06.002
20. Cervical cancer. National Comprehensive Cancer Network (US) NCCN. (2024). Available at: https://www.nccn.org/guidelines/guidelines-detail?category=1&id=1426. clinical practice guideline in oncology. Version 3.2024.
Keywords: MRI, radiomics, lymphovascular space invasion, cervical cancer, prediction, model
Citation: Ma N-N, Wang T, Lv Y-N and Li S-D (2024) An MRI radiomics-based model for the prediction of invasion of the lymphovascular space in patients with cervical cancer. Front. Oncol. 14:1394427. doi: 10.3389/fonc.2024.1394427
Received: 01 March 2024; Accepted: 24 June 2024;
Published: 05 July 2024.
Edited by:
Stefano Restaino, Ospedale Santa Maria della Misericordia di Udine, ItalyReviewed by:
Carlo Ronsini, Università degli Studi della Campania “Luigi Vanvitelli”, ItalyFederica Perelli, Azienda USL Toscana Centro, Italy
Copyright © 2024 Ma, Wang, Lv and Li. This is an open-access article distributed under the terms of the Creative Commons Attribution License (CC BY). The use, distribution or reproduction in other forums is permitted, provided the original author(s) and the copyright owner(s) are credited and that the original publication in this journal is cited, in accordance with accepted academic practice. No use, distribution or reproduction is permitted which does not comply with these terms.
*Correspondence: Shao-Dong Li, MTM4NTIwMDMzNzhAMTYzLmNvbQ==