- 1Department of Radiology, People’s Hospital of Henan University, Zhengzhou, Henan, China
- 2Department of Radiology, Henan Provincial People’s Hospital, Zhengzhou, Henan, China
- 3Department of Radiology, People’s Hospital of Zhengzhou University, Zhengzhou, Henan, China
- 4Department of Radiology, Affiliated Cancer Hospital of Zhengzhou University &Henan Provincial Cancer Hospital, Zhengzhou, China
- 5Heart Center, People’s Hospital of Zhengzhou University & Henan Provincial People’s Hospital, Zhengzhou, China
- 6Beijing United Imaging Research Institute of Intelligent Imaging & United Imaging Intelligence Co., Ltd., Beijing, China
- 7School of Basic Medical Sciences, Henan University, Kaifeng, China
Objective: To investigate the value of predicting axillary lymph node (ALN) metastasis based on intratumoral and peritumoral dynamic contrast-enhanced MRI (DCE-MRI) radiomics and clinico-radiological characteristics in breast cancer.
Methods: A total of 473 breast cancer patients who underwent preoperative DCE-MRI from Jan 2017 to Dec 2020 were enrolled. These patients were randomly divided into training (n=378) and testing sets (n=95) at 8:2 ratio. Intratumoral regions (ITRs) of interest were manually delineated, and peritumoral regions of 3 mm (3 mmPTRs) were automatically obtained by morphologically dilating the ITR. Radiomics features were extracted, and ALN metastasis-related radiomics features were selected by the Mann-Whitney U test, Z score normalization, variance thresholding, K-best algorithm and least absolute shrinkage and selection operator (LASSO) algorithm. Clinico-radiological risk factors were selected by logistic regression and were also used to construct predictive models combined with radiomics features. Then, 5 models were constructed, including ITR, 3 mmPTR, ITR+3 mmPTR, clinico-radiological and combined (ITR+3 mmPTR+ clinico-radiological) models. The performance of models was assessed by sensitivity, specificity, accuracy, F1 score and area under the curve (AUC) of receiver operating characteristic (ROC), calibration curves and decision curve analysis (DCA).
Results: A total of 2264 radiomics features were extracted from each region of interest (ROI), 3 and 10 radiomics features were selected for the ITR and 3 mmPTR, respectively. 5 clinico-radiological risk factors were selected, including lesion size, human epidermal growth factor receptor 2 (HER2) expression, vascular cancer thrombus status, MR-reported ALN status, and time-signal intensity curve (TIC) type. In the testing set, the combined model showed the highest AUC (0.839), specificity (74.2%), accuracy (75.8%) and F1 Score (69.3%) among the 5 models. DCA showed that it had the greatest net clinical benefit compared to the other models.
Conclusion: The intra- and peritumoral radiomics models based on DCE-MRI could be used to predict ALN metastasis in breast cancer, especially for the combined model with clinico-radiological characteristics showing promising clinical application value.
1 Introduction
Breast cancer has become the leading cause of cancer-related death among women worldwide (1). It has been reported that the 5-year survival rate for breast cancer patients with axillary lymph node (ALN) metastasis is 14% lower than that without metastasis (2). Accurate assessment of ALN status is critical for the clinical staging, selection of appropriate management and prognosis evaluation of breast cancer patients (3). Axillary lymph node dissection (ALND) remains the definitive treatment for palpable axillary positive patients, but this invasive procedure may result in postoperative complications. Sentinel lymph node biopsy (SLNB) has replaced ALND as the standard ALN assessment procedure for palpable axillary negative patients. However, it has a high false-negative rate of 7.8-27.3%, potentially causing challenges in subsequent treatment and management (4–7).
Imaging examinations, such as mammography, ultrasound, and MRI, are commonly used for preoperative assessment in ALN metastasis clinically. However, the results of these examinations can be subjectively affected by the experience of radiologists, which could lead to relatively elevated rates of missed diagnoses (8). Preoperative ultrasound-guided needle biopsy is also one of the clinically utilized methods for the assessment of ALN metastasis (9). However, it is difficult to reflect the whole heterogeneity due to the limited tissue samples obtained, and patients with negative biopsy results still need to undergo surgery to confirm the ALN pathological staging. Hence, the major problem is how to precisely evaluate the ALN with a noninvasive method.
Radiomics involves extracting quantitative features from medical images. Its goal is to explore connections between radiomics features and clinical information for better diagnosis and prognosis (10). DCE-MRI could provide crucial tumor hemodynamic information based on the multisequence imaging, and radiomics based on DCE-MRI has been corroborated by multiple studies for predicting ALN metastasis (11, 12). Peritumoral radiomics can reflect the microenvironment closely related to tumor growth and invasion (13), while research exploring their relevance to ALN metastasis remains limited. Additionally, clinico-radiological characteristics of breast cancer have been shown to be correlated with ALN metastasis (14, 15). This study aims to construct and validate preoperative predictive models for ALN metastasis based on both intra- and peritumoral DCE-MRI radiomics features and clinico-radiological characteristics in breast cancer.
2 Methods
2.1 Patient population
The study was approved by the Ethics Committee of Henan Provincial People’s Hospital (No: 2022-124), and the participants informed consent requirement was waived. Breast cancer patients who underwent initial DCE-MRI examination in our hospital from Jan 2017 to Dec 2020 were retrospectively enrolled. The inclusion criteria were as follows: (1). Patients were initially confirmed with invasive breast cancer of nonspecific type (IBC-NST) by pathology; (2). Patients with complete and available clinicopathologic information; (3). All cases have ALN pathological results confirmed by ALND, puncture pathology or SLNB; (4). Image quality was satisfactory. The exclusion criteria were as follows: (1). Breast cancer patients who underwent biopsy, radiotherapy or chemotherapy before MRI examination; (2). Patients with a lesion diameter less than 1 cm; (3). Patients presenting non-mass enhancement on DCE-MRI (In consideration of the accuracy of ROI delineation, non-mass enhanced lesions were excluded because of their unclear boundaries and various distribution patterns). In this study, positive ALNs were determined by the results of ALND and puncture biopsy, while negative ALNs were mainly determined by the results of ALND and SLNB. Patients with negative SLNB were directly considered to have no ALN metastasis. According to this criterion, patients were divided into ALN-positive and ALN-negative groups. A total of 473 patients were enrolled based on the inclusion and exclusion criteria, including 162 positive and 311 negative ALN patients. Patients were randomly divided into training (n = 378, mean age 50.20 ± 10.21) and testing sets (n = 95, mean age 47.45 ± 9.15) at a ratio of 8:2. The study flow chart is shown in Figure 1.
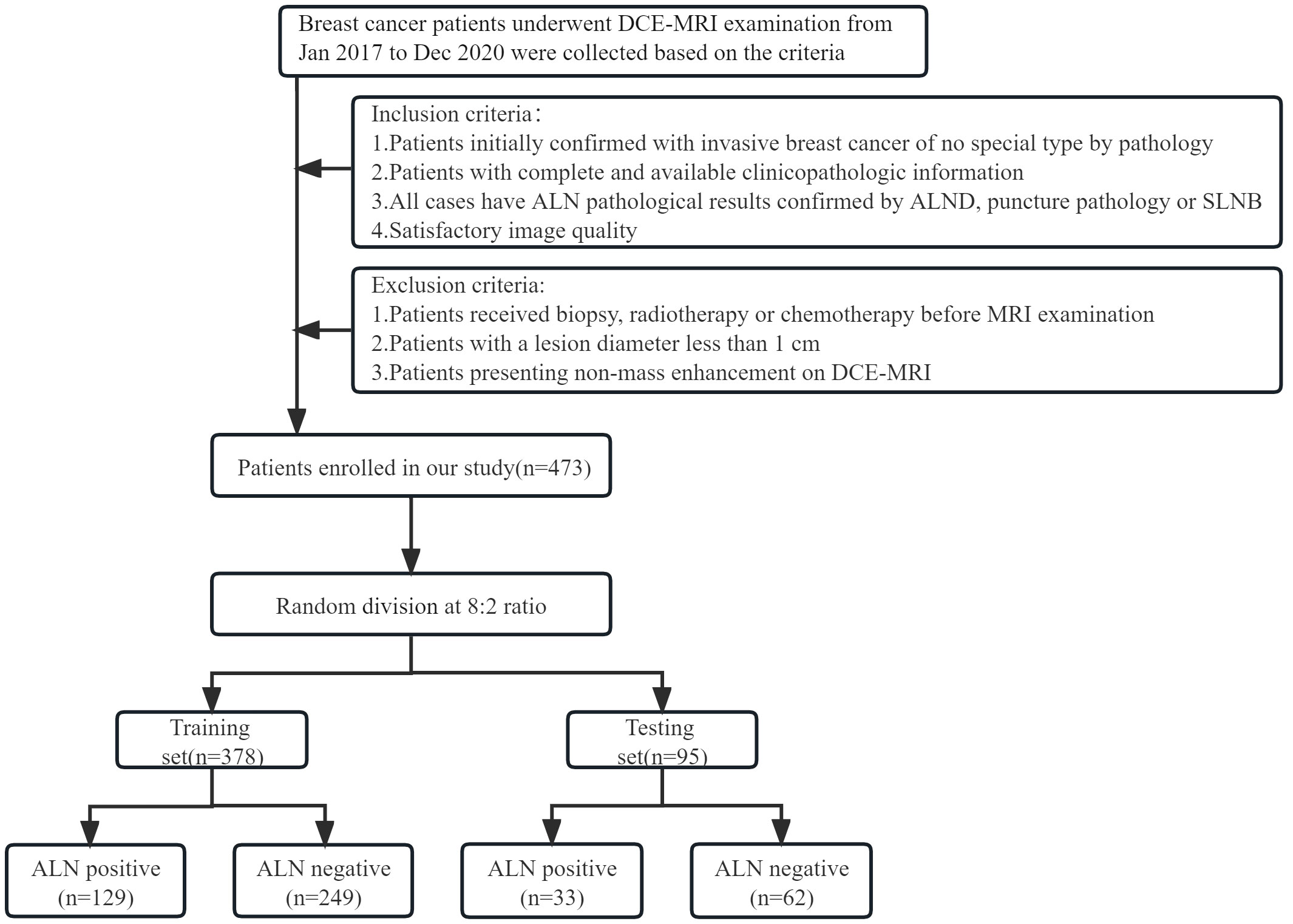
Figure 1 Flow chart for selection and grouping of populations according to inclusion and exclusion criteria.
2.2 DCE-MRI examination
Breast MRI examination was performed by 3.0T MR imaging devices and dedicated breast phased-array surface coils (GE Medical Systems Discovery MR750, Milwaukee). All enrolled patients lay in the prone position on the breast coil, with both breasts freely hanging pendulous. The main MRI sequence scanning parameters were as follows: unenhanced T1-weighted axial sequences (TR/TE=792/10 mms, FOV=340340 mm, matrix=512 512, slice thickness=5 mm, interval=2.5 mm, and number of slices=24). Dynamic contrast-enhanced scanning was performed using the T1-weighted volume imaging for breast assessment (VIBRANT) technique (TR/TE,3.8/1.6 mms; slice thickness, 1.1 mm; field of view, 751 340 mm; matrix scan, 512 512; phase,8). Intravenous access was established using a 12G intravenous indwelling needle before the examination. The contrast medium (Gado-linium-DTPA; Magnevist, Schering, Germany, 0.2 mmol/kg) was intravenously administered as a bolus injection to the patients undergoing contrast-enhanced MRI, followed by a 20 ml saline flush. A total of 8 phases were scanned, with 124 scanning layers for each phase.
2.3 Clinico-radiological characteristics
Clinical characteristics were obtained from the electronic medical records, including age, pathological grade, expression of estrogen receptor (ER), progesterone-receptor (PR), human epidermal growth factor receptor 2 (HER2), antigen Ki67, and vascular cancer thrombus status. ER expression was categorized into low (≤10%) and high (> 10%) groups. PR and Ki67 expression were classified as low (PR ≤ 20%, Ki67 <20%) and high (PR>20%, Ki67≥20%) groups based on a cut-off of 20% (16, 17). HER2 status was confirmed by immunohistochemistry (IHC) and fluorescence in situ hybridization (FISH). Radiological characteristics were analyzed by 2 radiologists with more than 5 years of experience in breast imaging. They were blinded to the pathological results, and a consensus decision was made in cases of discrepancy. All the original images acquired after scanning were transmitted to the AW4.6 postprocessing workstation. The ROIs were selected at the obvious lesion enhancement site, avoiding areas with hemorrhage, necrosis and calcification to analyze the time-signal intensity curve (TIC), which were classified into 3 classical types: type I – inflow type, type II – platform type, and type III – outflow type. The assessment was mainly based on the second edition of the Breast Imaging Reporting and Data System (BI-RADS) for breast MRI (18). Background parenchymal enhancement (BPE) type, lesion size (longest diameter), MR-reported ALN status and TIC type were recorded as the radiological characteristics.
2.4 Image segmentation
The workflow of the radiomics analysis is illustrated in Figure 2. The third phase of DCE-MRI images, in which lesions achieved sufficient enhancement (19), were anonymously exported from the picture archiving and communication systems (PACS) and saved in DICOM format. Using ITK-SNAP software (Version 3.8.0, http://www.itk-snap.org), a breast radiologist with more than 5 years of experience manually delineated the intratumoral region (ITR) slice-by-slice, avoiding necrotic areas. The ITRs were verified by an associate chief physician with over 10 years of experience in breast radiology. The peritumoral region (PTR) was generated by morphologically dilating the ITR outwards by 3 mm using the uRP platform (uAI research portal, https://www.uii-ai.com/en/uai/scientifific-research), which is a clinical research platform integrating AI module algorithms (20). PTR portions extending beyond the breast parenchyma were removed manually. Each lesion obtained 2 ROIs, including ITR and 3 mm PTR (Figure 3).
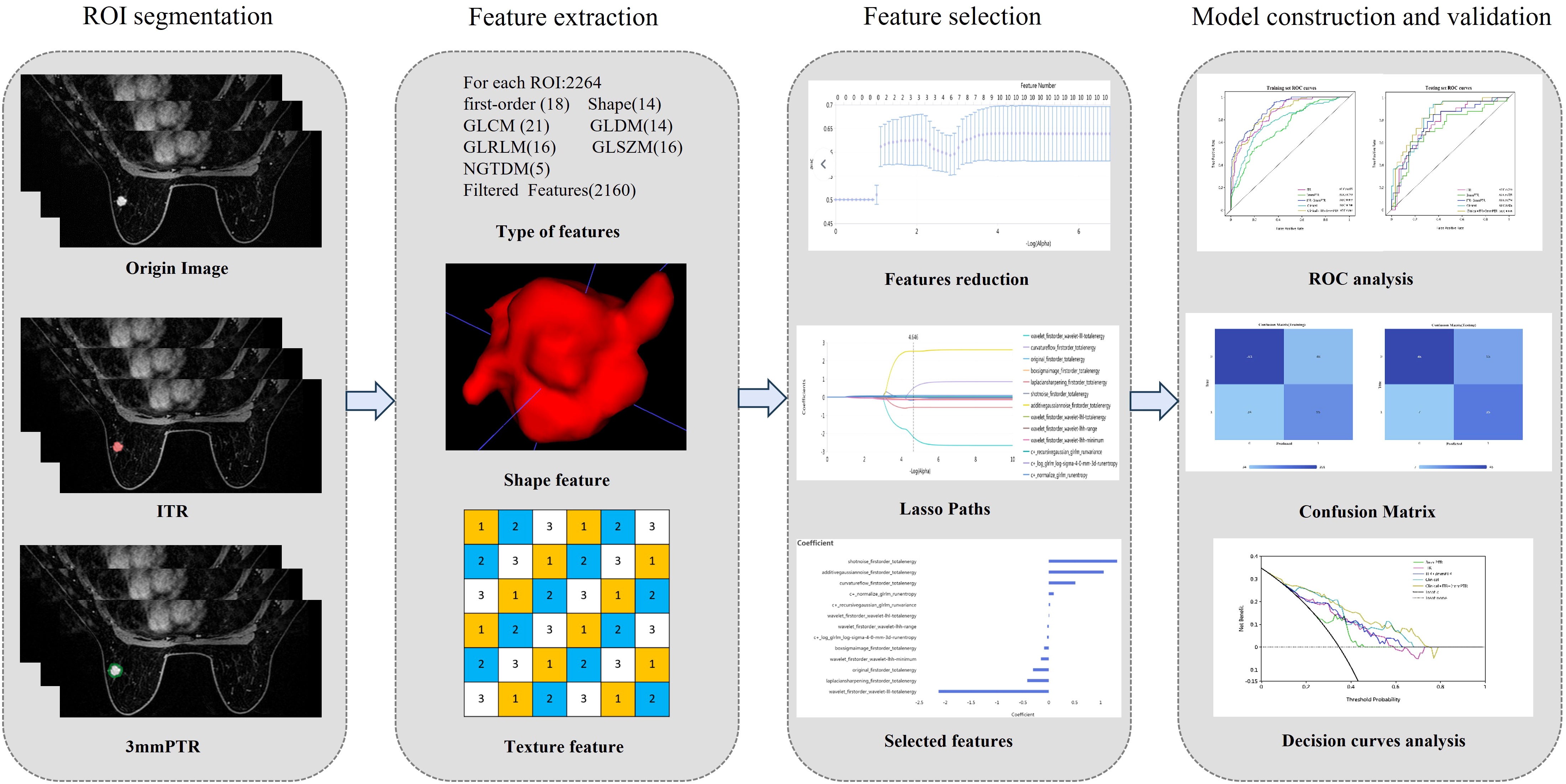
Figure 2 The workflow of radiomics. (1) Outlining ROI by manual joint automatic algorithm (2) Extraction of high-throughput features based on 2 different ROIs respectively (3) Feature selection (4) Model construction and validation. ITR: intratumoral region; 3mmPTR: peritumoral region of 3 mm.
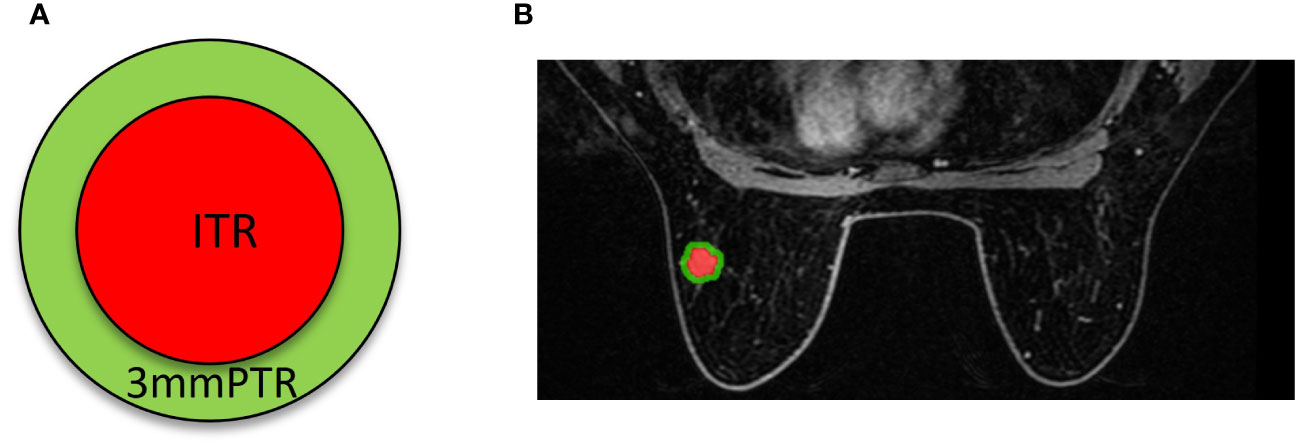
Figure 3 Schematics (A) and examples (B) of different ROI segmentation schemes. The red region represents the ITR, and the green region represents the 3 mm PTR. ITR: intratumoral region; 3mmPTR: peritumoral region of 3 mm.
2.5 Radiomics feature extraction and selection
A total of 2264 radiomics features were extracted from each ROI through the uRP platform. Feature selection was performed to avoid overfitting the models, and this process was conducted in the training set. Firstly, Mann-Whitney U test was performed to select ALN metastasis -related features. Subsequently, Z score normalization was used to reduce feature dimensionality differences, features with variance over threshold (0.1) were retained by variance threshold, and high p value features were filtered using K-Best (i.e., F value method). Finally, least absolute shrinkage and selection operator (LASSO) regression was applied to remove features with high collinearity, thereby obtaining the optimal feature subset based on the 2 ROIs. The detailed features and their respective coefficients are shown in the Supplementary Data.
2.6 Model construction and performance assessment
First, univariate analysis and binary logistic regression were used to select clinico-radiological independent factors of ALN metastasis. Second, the optimal radiomics features and clinico-radiological independent factors were standardized using preprocessing methods to unify dimensions respectively. Bagging decision tree was applied to construct predictive models. Detailed preprocessing methods are shown in the Supplementary Data. Finally, based on the model performance assessment indices, 3 optimal models were selected, including ITR, 3 mm PTR and clinico-radiological models. Then, 2 additional models were constructed based on the optimal radiomics features from intra- and peritumoral regions and clinico-radiological independent factors, including the ITR + 3 mm PTR model and the combined (ITR+3 mmPTR+ clinico-radiological) model.
The performance of the models was assessed by sensitivity, specificity, accuracy, F1 score and area under the curve (AUC) of receiver operating characteristic (ROC). The calibration of the models was assessed using the Hosmer-Lemeshow goodness-of-fit test and calibration curves. Decision curve analysis (DCA) compared the net clinical benefits of the models across a range of threshold probabilities.
2.7 Statistical analysis
Statistical analysis was performed using SPSS software (V.26.0) and R software (V. 4.3.1). Quantitative variables were compared using the t test or Mann-Whitney U test, while qualitative variables were compared using the χ2 test or Fisher’s test, if one of the theoretical frequencies was less than 1, the likelihood ratio chi-square was adopted. The ordered classified variables were compared with the rank sum test. Binary logistic regression analysis was performed to select clinico-radiological independent factors, which were used to construct predictive models combined with radiomics features. The AUCs of different models were compared by the DeLong test, The correction for multiple comparisons was conducted using Bonferroni correction. The value of sensitivity, specificity, accuracy, and F1Score were obtained based on the cut-off 0.5. P <0.05 was considered statistically significant.
3 Results
3.1 Clinico-radiological characteristics
Comparing the clinico-radiological characteristics between the training and testing sets, there were no significant differences except for age and MR-reported ALN status (both p<0.05, Table 1). In the ALN-positive group, the percentages of positive HER2 expression, positive vascular cancer thrombus, MR reported-positive ALN and type II~III TIC were higher than those in the negative group, lesion size was also larger in the positive group (all p<0.05, Table 2), and they were used to select independent risk factors for ALN metastasis by binary logistic regression. The results showed that positive HER2 expression (OR = 1.979, P = 0.011), positive vascular cancer thrombus (OR = 3.183, P < 0.001), larger lesion size (OR = 1.036, P = 0.004), MR-reported positive ALN (OR = 1.862, P = 0.010), and TIC types (II: OR = 3.363, P = 0.027; III: OR = 3.811, P = 0.014) were independent risk factors for ALN metastasis (Figure 4).
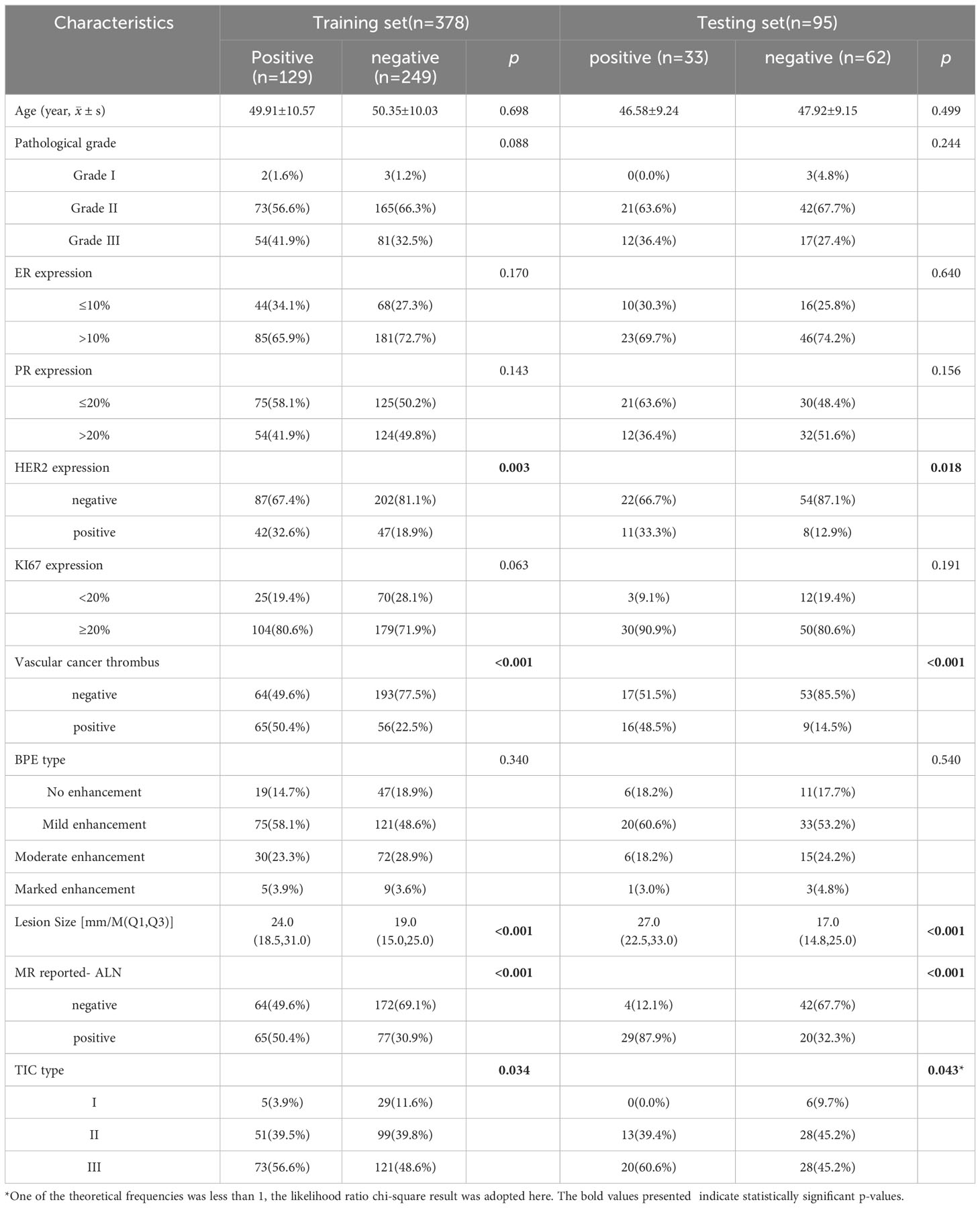
Table 2 Comparison of clinico-radiological characteristics between different ALN groups in the training and testing sets.
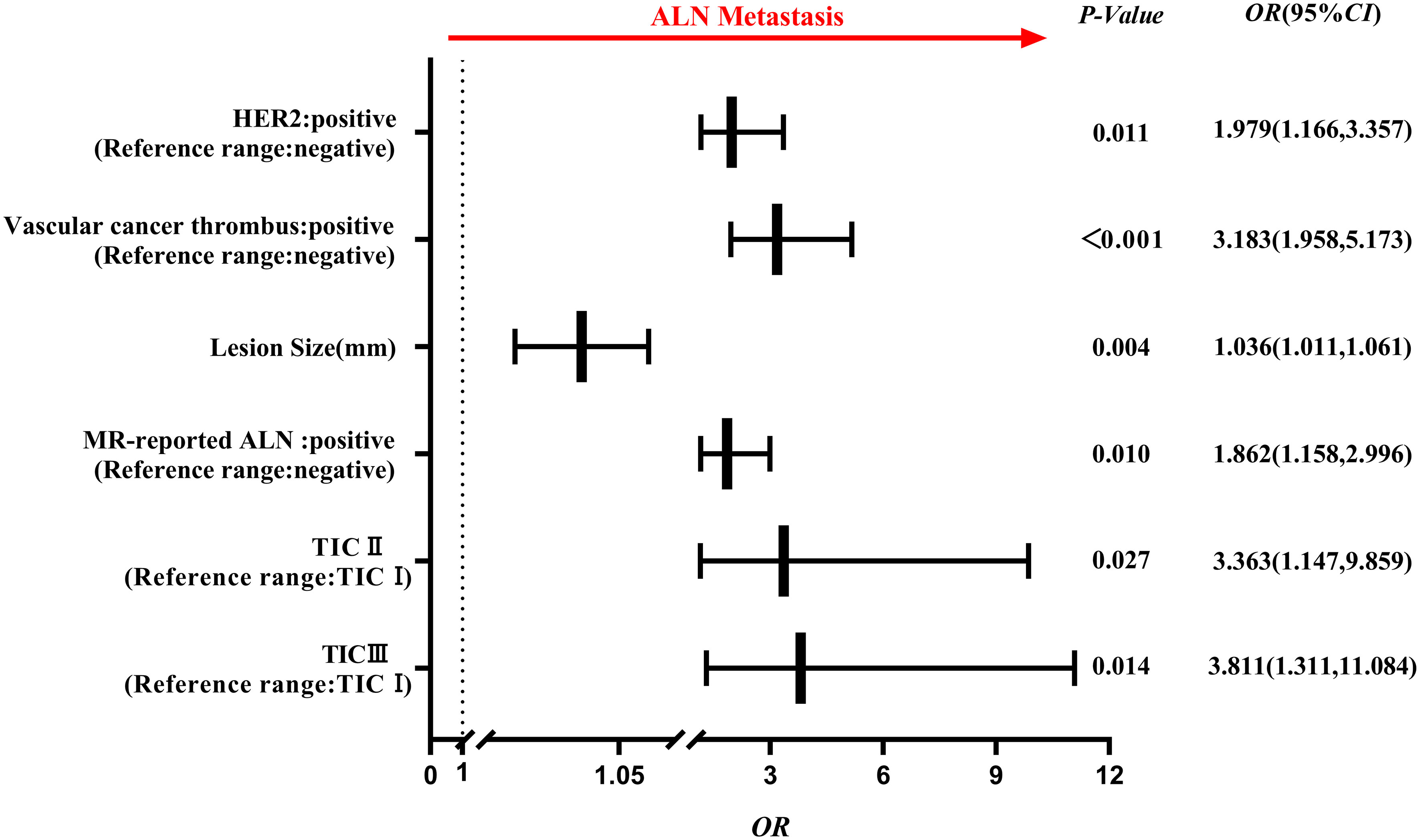
Figure 4 Forest plot of independent factors for predicting ALN metastasis based on clinico-radiological characteristics of breast cancer. The results revealed that positive HER2 expression, positive vascular cancer thrombus, lesion size, MR-reported positive ALN and TIC (II, III) type were independent risk factors for ALN metastasis.
3.2 Feature extraction and selection
A total of 2264 radiomics features were extracted from each ROI, including 18 first-order statistical features, 14 shape features, 72 texture features and 2160 filtered features (i.e., high-order statistical features). 3 and 10 features were finally selected as the optimal features based on ITR and 3 mm PTR using the LASSO method. The ITR contained 3 high-order statistical features, while the 3 mm PTR contained 1 first-order statistical feature and 9 high-order statistical features (20).
3.3 Construction and validation of models
The performances of the models based on ITR, PTR and clinico-radiological characteristics are shown in Table 3. The AUC of the combined model (ITR+3 mmPTR+clinico-radiological) in the testing set was 0.839, which was the highest compared to the other 4 models (ITR, 3 mm PTR, ITR + 3 mm PTR, clinico-radiological, Figure 5). The combined model also achieved the highest specificity (74.2%), accuracy (75.8%) and F1 Score (69.3%). The DeLong test showed that the AUCs of the 3 mm PTR and ITR models were significantly different from that of the combined model in the testing set (P=0.047 and 0.036, respectively), and the results are shown in Table 4.
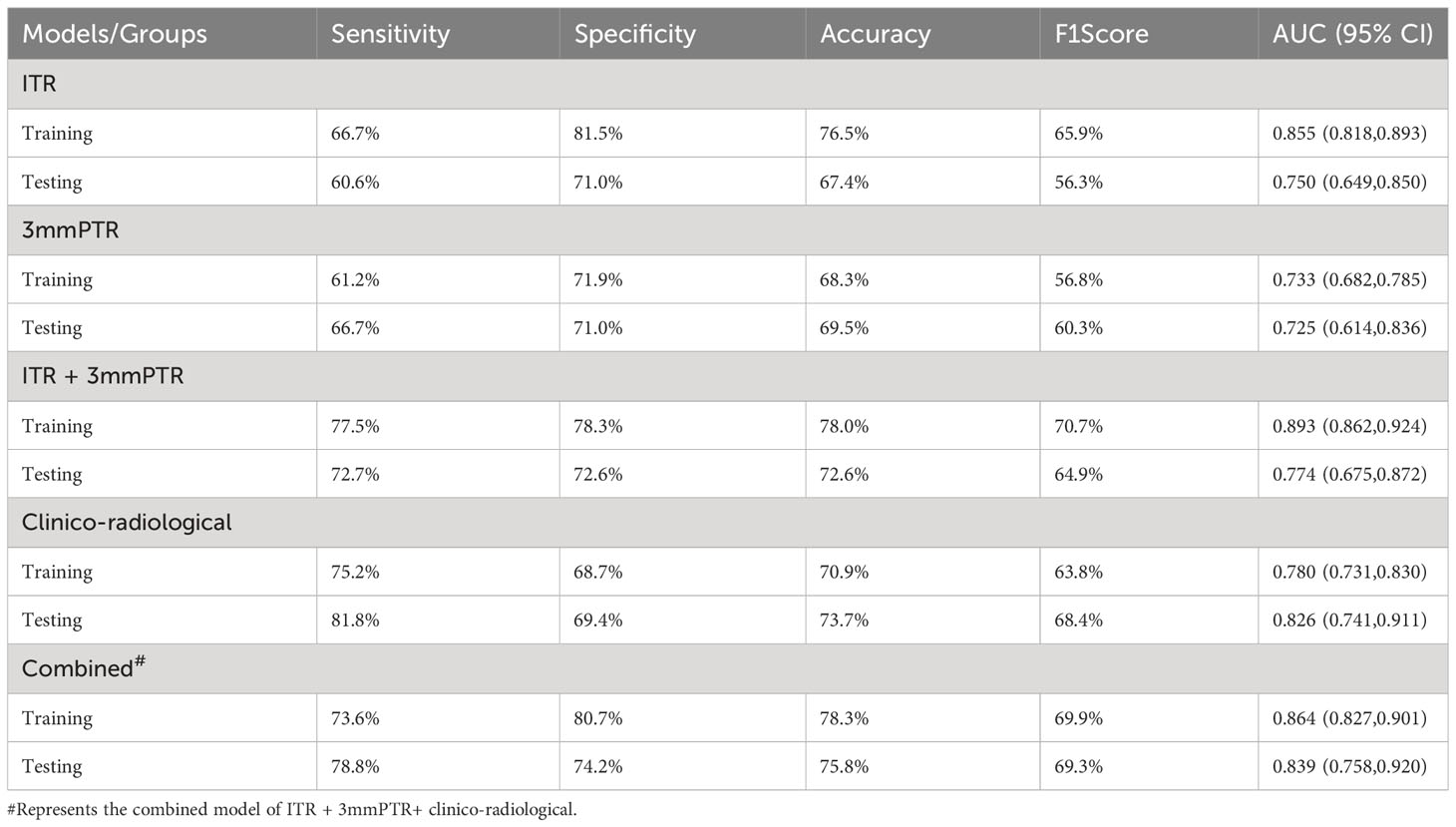
Table 3 Performances of the models based on radiomics features and clinico-radiological characteristics.
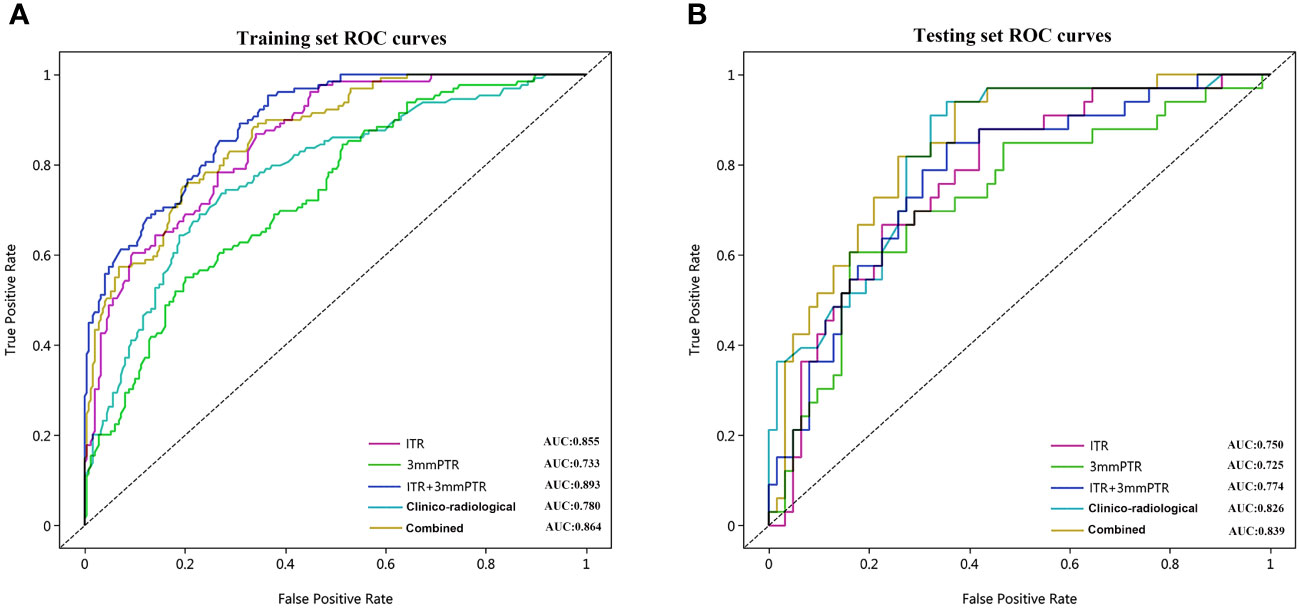
Figure 5 The ROC curves of the 5 models used to predict ALN metastasis in breast cancer patients in the training (A) and testing (B) sets. ROC, receiver operating characteristic; AUC, area under the curve of ROC; Combined, means the ITR+3 mmPTR+ clinico-radiological model.
The calibration curves demonstrated good consistency between predicted risks and observed probabilities across the 5 datasets. (Figure 6). The DCA showed that the combined model had the best clinical net benefit across threshold probabilities of 0.04-0.76 and widest applicable range compared to other models (Figure 7).
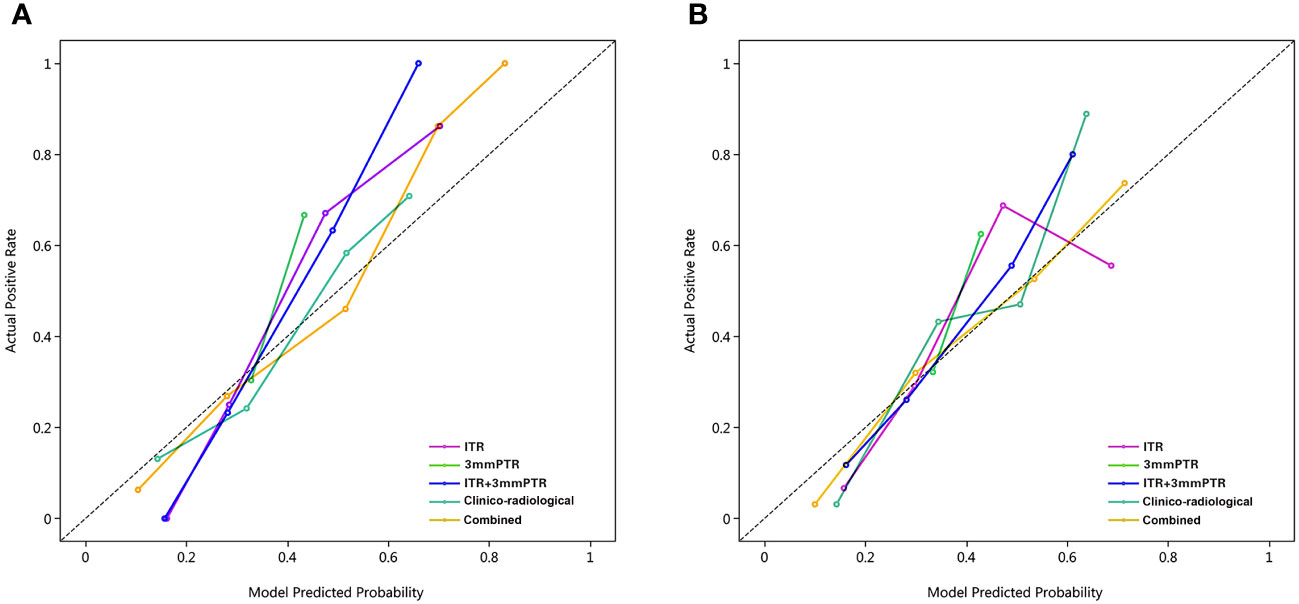
Figure 6 Calibration curves of the 5 models in the training and testing sets. The calibration curves show the agreement between the predicted probability of ALN metastasis and the actual metastasis outcomes. The y-axis represents the actual metastasis rate. The x-axis represents the predicted metastasis probability. The diagonal line represents ideal prediction. (A) Training set; (B) Testing set; Combined, means the ITR+3 mmPTR+ clinico-radiological model.
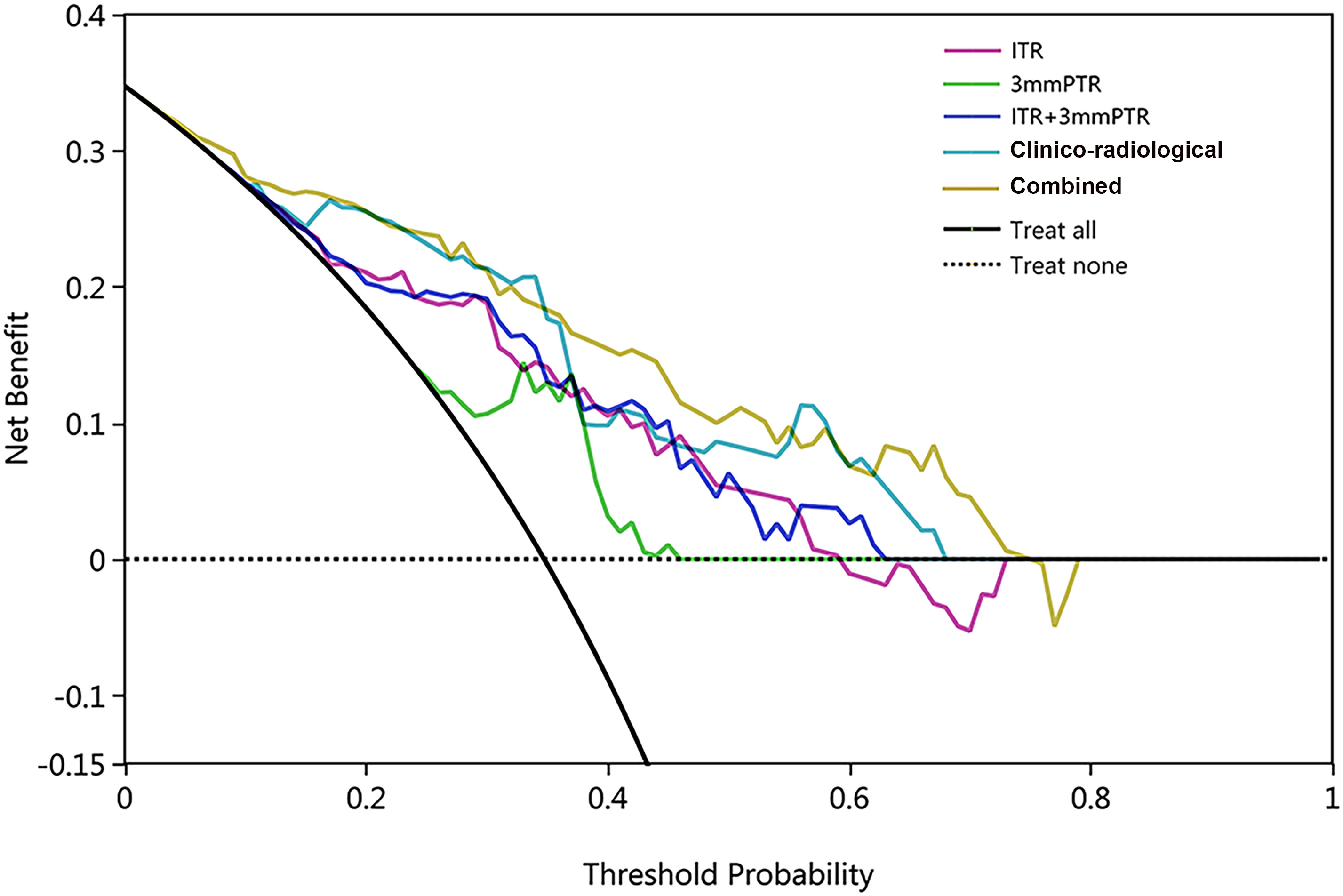
Figure 7 The DCA of 5 models in the testing set, with threshold probability on the x-axis and net benefit on the y-axis. The black solid and dashed lines represent the ‘treat-all’ and ‘treat-none’ strategies. The combined model (ITR+3mmPTR+clinico-radiological) showed the highest net benefit within the threshold probability range of 0.04-0.76, with the widest applicable range.
4 Discussion
As an essential prognostic factor of breast cancer, ALN status is critical for therapy decision- making. However, accurate preoperative assessment of ALN metastasis remains challenging for radiologists relying on medical imaging, for the high false-negative rate of morphological features on images. Radiomics is a method of data mining to extract crucial information from images, enabling tumor diagnosis, prognosis prediction, and other clinical applications (21). DCE-MRI radiomics has been reported to be useful for assessing ALN metastasis (22–24). Additionally, peritumoral radiomics can capture the heterogeneity of the tumor microenvironment, but few studies have utilized it to predict ALN metastasis. In this study, we constructed and validated a radiomics model based on features extracted from intratumoral and peritumoral regions, and the capability of the model for predicting ALN metastasis is impressive. Integrated with clinico-radiological characteristics, the combined model showed excellent performance in predicting ALN metastasis, exhibiting greater net benefits, which to some extent reduced unnecessary pathological surgeries.
Our results confirmed that previously reported clinico-radiological predictors, such as HER2 expression, lesion size and MR-reported ALN status, could be related to ALN metastasis (25, 26). The results showed that positive vascular tumor thrombus was a moderate intensity risk factor (OR = 3.183), which could be explained by the widespread lymphatic vessels in the breast. It make it easy for cancer cells draining to the ALN through lymphatic vessels infiltrating the breast lobules (27). Interestingly, type II and III TICs were also strongly correlated with ALN metastasis (II: OR = 3.363, III: OR = 3.811), although they were primarily used to differentiate benign and malignant breast lesions. And MR-reported ALN status can also contributes to enhance the predictive performance of the radiomics model in this study (28). In the testing set, the clinico-radiological model outperformed the radiomics models with an AUC of 0.826. However, it performed slightly inferior in the training set, which was probably caused by the instability of the characteristics or imbalanced grouping. However, when the ITR+3 mmPTR model was integrated with clinico-radiological characteristics, the AUC improved from 0.774 to 0.839 in the testing set. Therefore, clinico-radiological characteristics were valuable for assessing ALN metastasis. For the selected features in our study, we found the subclass of the optimal high-order statistical features were first-order statistics and gray level run length matrix (GLRLM) that belongs to a type of textural features. The first-order statistics features, such as the mean and median, reflect the distribution of voxel intensity for images. The results of our study are consistent with Yan et al (29), they demonstrated the first-order features were related to LN metastasis in endometrial cancer. The GLRLM features, commonly utilized to quantify regional heterogeneity differences, have been validated for their predictive value in ALN metastasis (30), consistent with our study findings.
Intratumoral radiomics was one of the most important components in predicting ALN metastasis. Notably, regarding the choice of the DCE phase, there is no consensus in defining which phase of the radiomics offers the best prediction performance. We chose the third phase of post-contrast DCE-MRI for radiomics analysis because it offers sufficient tumor enhancement, facilitating clear visualization of lesion boundaries and providing valuable hemodynamic and heterogeneity information (31), and the radiomics model based on the third phase exhibited good performance in this study. Chai et al (23). constructed a radiomics model based on the second phase of postcontrast imaging, obtaining an AUC of 0.850. Liu et al. (31)used radiomics features of the obvious enhancement phase according to the TIC, but the predictive performance was similar to that of Chai et al. Consequently, the selection of the optimal DCE phase needs further exploration.
Previous studies in predicting ALN metastasis have focused solely on the primary tumor but have seldom taken peritumoral radiomics into account for analysis (32, 33). Peritumoral radiomics is related to the proliferation and metastasis of malignant cells, infiltration lymphocytes, angiogenesis, and stromal reactions (34, 35). Studies have shown that models incorporating peritumoral radiomics can better identify the molecular subtypes of breast cancer and evaluate neoadjuvant chemotherapy efficacy (36, 37). In this study, we analyzed the potential radiomics features of both the primary tumor and peritumoral region for the assessment of ALN metastasis. Our results demonstrated that peritumoral radiomics had good performance in the training (AUC: 0.733) and testing sets (AUC: 0.725). When combined with intratumoral features, the AUC reached 0.893 (training set) and 0.774 (testing set). Although the AUC did not improve much in the testing set, the ITR + 3 mmPTR model showed improved sensitivity and accuracy by 12.1% and 5.2%, respectively, compared to the ITR model. The improved sensitivity helped avoid missing ALN metastasis to some extent, underscoring the optimization of the model through peritumoral radiomics integration. Though the size selection of peritumoral region is inconclusive currently, it is reported the performance of radiomics model will reduce when expanding peritumoral regions from 5 mm to 10 mm (38, 39). Zhou et al. also found higher accuracy with the proximal peritumoral region compared to the larger region (13).This maybe have some relationship with the localization between the tumor and peritumoral tissue. Our study assessed a 3 mm proximal peritumoral radiomics model with the AUC value of 0.725, which performed better than previous studies (38). Comparisons of radiomics models with different sizes of peritumoral regions were not conducted but will be explored using different sequences in the future.
The combined model was established by incorporating intra- and peritumoral radiomics features with clinico-radiological risk factors in our study, which showed higher predictive efficacy than that of the independent model of radiomics and clinico-radiological characteristics. The combined model achieved the highest AUC (0.839), specificity (74.2%), accuracy (75.8%) and F1 Score (69.3%) among the 5 models in the testing set. DCA also showed that the combined model had the best clinical net benefit and widest applicable range compared to the other models, which indicates that the combined model has promising clinical application value.
There are some strengths in our study. We performed radiomics feature selection using various preprocessing methods, an approach that often yields models with stable performance. Additionally, the larger sample size in the present study compared to most previous studies may enhance the reliability of the results. However, there are also some limitations. First, as a single-center retrospective study, there may be selection bias. And the results need external validation, which would be conducted using datasets from multiple institutions in the subsequent studies. Second, all pathological types were IBC-NST and relatively homogeneous, and future studies could expand to more diverse cancer types. Finally, the peritumoral region was obtained by dilating the tumor 3 mm in this study, but whether this is the optimal peritumoral region requires further refinement of the expanded range and validation.
5 Conclusion
In summary, peritumoral radiomics based on DCE-MRI is helpful for accurately predicting ALN metastasis preoperatively. The combined model utilizing clinico-radiological, intratumoral and peritumoral information has higher predictive performance than each individual approach. It is expected to be a reliable tool for predicting ALN metastasis in clinical practice.
Data availability statement
The raw data supporting the conclusions of this article will be made available by the authors, without undue reservation.
Ethics statement
The studies involving humans were approved by Ethics Committee of Henan Provincial People’s Hospital (No: 2022-124). The studies were conducted in accordance with the local legislation and institutional requirements. Due to the retrospective nature of this study, the requirement for informed consent from patients was waived.
Author contributions
YXW: Conceptualization, Data curation, Formal Analysis, Investigation, Methodology, Software, Validation, Visualization, Writing – original draft. YS: Data curation, Investigation, Writing – original draft. YXG: Data curation, Investigation, Writing – original draft. MH: Writing – original draft. YG: Writing – original draft. QW: Software, Writing – review & editing. SL: Writing – original draft. JL: Writing – original draft. XS: Funding acquisition, Writing – review & editing. YPW: Software, Writing – review & editing. MW: Conceptualization, Writing – review & editing. HT: Conceptualization, Funding acquisition, Project administration, Supervision, Writing – review & editing.
Funding
The author(s) declare that financial support was received for the research, authorship, and/or publication of this article. This study was supported by Medical Science and Technological Project of Henan Province (No. LHGJ20220055) and Natural Science Foundation of Henan Province (No. 202300410330).
Acknowledgments
We thank the patients participating in this study and the technical staff for their support. We also acknowledge the pathologists who provided the final ALN results.
Conflict of interest
Author QW was employed by the company Beijing United Imaging Research Institute of Intelligent Imaging & United Imaging Intelligence Co., Ltd.
The remaining authors declare that the research was conducted in the absence of any commercial or financial relationships that could be construed as a potential conflict of interest.
Publisher’s note
All claims expressed in this article are solely those of the authors and do not necessarily represent those of their affiliated organizations, or those of the publisher, the editors and the reviewers. Any product that may be evaluated in this article, or claim that may be made by its manufacturer, is not guaranteed or endorsed by the publisher.
Supplementary material
The Supplementary Material for this article can be found online at: https://www.frontiersin.org/articles/10.3389/fonc.2024.1357145/full#supplementary-material
Abbreviations
DCE-MRI, dynamic contrast-enhanced MRI; ALN, axillary lymph node; ROI, regions of interest; ITR, intratumoral region; 3mmPTR, peritumoral region of 3 mm; LASSO, least absolute shrinkage and selection operator; ROC, receiver operating characteristic; AUC, area under the curve; DCA, decision curve analysis; IBC-NST, Invasive Breast Cancer of Nonspecific Type; ER, Estrogen receptor; PR, Progesterone receptor; HER2, Human epidermal growth factor receptor 2; IHC, immunohistochemistry; FISH, fluorescence in situ hybridization; TIC, time-signal intensity curve; PACS, picture archiving and communication systems.
References
1. Sung H, Ferlay J, Siegel RL, Laversanne M, Soerjomataram I, Jemal A, et al. Global cancer statistics 2020: GLOBOCAN estimates of incidence and mortality worldwide for 36 cancers in 185 countries. CA: Cancer J Clin. (2021) 71:209–49. doi: 10.3322/caac.21660
2. Samiei S, Granzier RWY, Ibrahim A, Primakov S, Lobbes MBI, Beets-Tan RGH, et al. Dedicated axillary MRI-based radiomics analysis for the prediction of axillary lymph node metastasis in breast cancer. Cancers. (2021) 13(4):757. doi: 10.3390/cancers13040757
3. Ahmed M, Purushotham AD, Douek M. Novel techniques for sentinel lymph node biopsy in breast cancer: a systematic review. Lancet Oncol. (2014) 15:e351–62. doi: 10.1016/S1470-2045(13)70590-4
4. Veronesi P, Corso G. Standard and controversies in sentinel node in breast cancer patients. Breast (Edinburgh Scotland). (2019) 48:Suppl 1:S53–s6. doi: 10.1016/S0960-9776(19)31124-5
5. Giuliano AE, Ballman KV, McCall L, Beitsch PD, Brennan MB, Kelemen PR, et al. Effect of axillary dissection vs no axillary dissection on 10-year overall survival among women with invasive breast cancer and sentinel node metastasis: the ACOSOG Z0011 (Alliance) randomized clinical trial. Jama. (2017) 318:918–26. doi: 10.1001/jama.2017.11470
6. Pesek S, Ashikaga T, Krag LE, Krag D. The false-negative rate of sentinel node biopsy in patients with breast cancer: a meta-analysis. World J surgery. (2012) 36:2239–51. doi: 10.1007/s00268-012-1623-z
7. Belmonte R, Messaggi-Sartor M, Ferrer M, Pont A, Escalada F. Prospective study of shoulder strength, shoulder range of motion, and lymphedema in breast cancer patients from pre-surgery to 5 years after ALND or SLNB. Supportive Care Cancer. (2018) 26:3277–87. doi: 10.1007/s00520-018-4186-1
8. Valente SA, Levine GM, Silverstein MJ, Rayhanabad JA, Weng-Grumley JG, Ji L, et al. Accuracy of predicting axillary lymph node positivity by physical examination, mammography, ultrasonography, and magnetic resonance imaging. Ann Surg Oncol. (2012) 19:1825–30. doi: 10.1245/s10434-011-2200-7
9. Balasubramanian I, Fleming CA, Corrigan MA, Redmond HP, Kerin MJ, Lowery AJ. Meta-analysis of the diagnostic accuracy of ultrasound-guided fine-needle aspiration and core needle biopsy in diagnosing axillary lymph node metastasis. Br J surgery. (2018) 105:1244–53. doi: 10.1002/bjs.10920
10. Lambin P, Rios-Velazquez E, Leijenaar R, Carvalho S, van Stiphout RG, Granton P, et al. Radiomics: extracting more information from medical images using advanced feature analysis. Eur J Cancer (Oxford England: 1990). (2012) 48:441–6. doi: 10.1016/j.ejca.2011.11.036
11. Yu Y, He Z, Ouyang J, Tan Y, Chen Y, Gu Y, et al. Magnetic resonance imaging radiomics predicts preoperative axillary lymph node metastasis to support surgical decisions and is associated with tumor microenvironment in invasive breast cancer: A machine learning, multicenter study. EBioMedicine. (2021) 69:103460. doi: 10.1016/j.ebiom.2021.103460
12. Yu Y, Tan Y, Xie C, Hu Q, Ouyang J, Chen Y, et al. Development and validation of a preoperative magnetic resonance imaging radiomics-based signature to predict axillary lymph node metastasis and disease-free survival in patients with early-stage breast cancer. JAMA network Open. (2020) 3:e2028086. doi: 10.1001/jamanetworkopen.2020.28086
13. Zhou J, Zhang Y, Chang KT, Lee KE, Wang O, Li J, et al. Diagnosis of benign and Malignant breast lesions on DCE-MRI by using radiomics and deep learning with consideration of peritumor tissue. J magnetic resonance imaging: JMRI. (2020) 51:798–809. doi: 10.1002/jmri.26981
14. Ding Q, Huo L, Peng Y, Yoon EC, Li Z, Sahin AA. Immunohistochemical markers for distinguishing metastatic breast carcinoma from other common Malignancies: update and revisit. Semin Diagn pathology. (2022) 39:313–21. doi: 10.1053/j.semdp.2022.04.002
15. Xue M, Che S, Tian Y, Xie L, Huang L, Zhao L, et al. Nomogram based on breast MRI and clinicopathologic features for predicting axillary lymph node metastasis in patients with early-stage invasive breast cancer: A retrospective study. Clin Breast cancer. (2022) 22:e428–e37. doi: 10.1016/j.clbc.2021.10.014
16. Prat A, Cheang MC, Martín M, Parker JS, Carrasco E, Caballero R, et al. Prognostic significance of progesterone receptor-positive tumor cells within immunohistochemically defined luminal A breast cancer. J Clin Oncol. (2013) 31:203–9. doi: 10.1200/JCO.2012.43.4134
17. Allison KH, Hammond MEH, Dowsett M, McKernin SE, Carey LA, Fitzgibbons PL, et al. Estrogen and progesterone receptor testing in breast cancer: ASCO/CAP guideline update. J Clin Oncol. (2020) 38:1346–66. doi: 10.1200/JCO.19.02309
18. Vinnicombe S. How I report breast magnetic resonance imaging studies for breast cancer staging and screening. Cancer Imaging. (2016) 16:17. doi: 10.1186/s40644-016-0078-0
19. Ya G, Wen F, Xing-Ru L, Zhuan-Zhuan G, Jun-Qiang L. Difference of DCE-MRI parameters at different time points and their predictive value for axillary lymph node metastasis of breast cancer. Acad Radiol. (2022) 29 Suppl 1:S79–s86. doi: 10.1016/j.acra.2021.01.013
20. Wu J, Xia Y, Wang X, Wei Y, Liu A, Innanje A, et al. uRP: An integrated research platform for one-stop analysis of medical images. Front radiology. (2023) 3:1153784. doi: 10.3389/fradi.2023.1153784
21. Gillies RJ, Kinahan PE, Hricak H. Radiomics: images are more than pictures, they are data. Radiology. (2016) 278:563–77. doi: 10.1148/radiol.2015151169
22. Chen Y, Wang L, Dong X, Luo R, Ge Y, Liu H, et al. Deep learning radiomics of preoperative breast MRI for prediction of axillary lymph node metastasis in breast cancer. J digital imaging. (2023) 36:1323–31. doi: 10.1007/s10278-023-00818-9
23. Chai R, Ma H, Xu M, Arefan D, Cui X, Liu Y, et al. Differentiating axillary lymph node metastasis in invasive breast cancer patients: A comparison of radiomic signatures from multiparametric breast MR sequences. J magnetic resonance imaging: JMRI. (2019) 50:1125–32. doi: 10.1002/jmri.26701
24. Zhan C, Hu Y, Wang X, Liu H, Xia L, Ai T. Prediction of Axillary Lymph Node Metastasis in Breast Cancer using Intra-peritumoral Textural Transition Analysis based on Dynamic Contrast-enhanced Magnetic Resonance Imaging. Acad Radiol. (2022) 29 Suppl 1:S107–s15. doi: 10.1016/j.acra.2021.02.008
25. He ZY, Wu SG, Yang Q, Sun JY, Li FY, Lin Q, et al. Breast cancer subtype is associated with axillary lymph node metastasis: A retrospective cohort study. Medicine. (2015) 94:e2213. doi: 10.1097/MD.0000000000002213
26. Mao N, Dai Y, Lin F, Ma H, Duan S, Xie H, et al. Radiomics nomogram of DCE-MRI for the prediction of axillary lymph node metastasis in breast cancer. Front Oncol. (2020) 10:541849. doi: 10.3389/fonc.2020.541849
27. Lale A, Yur M, Özgül H, Alkurt EG, Yıldırım N, Aygen E, et al. Predictors of non-sentinel lymph node metastasis in clinical early stage (cT1-2N0) breast cancer patients with 1-2 metastatic sentinel lymph nodes. Asian J surgery. (2020) 43:538–49. doi: 10.1016/j.asjsur.2019.07.019
28. Kim EJ, Kim SH, Kang BJ, Choi BG, Song BJ, Choi JJ. Diagnostic value of breast MRI for predicting metastatic axillary lymph nodes in breast cancer patients: diffusion-weighted MRI and conventional MRI. Magnetic resonance imaging. (2014) 32:1230–6. doi: 10.1016/j.mri.2014.07.001
29. Yan BC, Li Y, Ma FH, Zhang GF, Feng F, Sun MH, et al. Radiologists with MRI-based radiomics aids to predict the pelvic lymph node metastasis in endometrial cancer: a multicenter study. Eur radiology. (2021) 31:411–22. doi: 10.1007/s00330-020-07099-8
30. Song D, Yang F, Zhang Y, Guo Y, Qu Y, Zhang X, et al. Dynamic contrast-enhanced MRI radiomics nomogram for predicting axillary lymph node metastasis in breast cancer. Cancer Imaging. (2022) 22:17. doi: 10.1186/s40644-022-00450-w
31. Liu J, Sun D, Chen L, Fang Z, Song W, Guo D, et al. Radiomics analysis of dynamic contrast-enhanced magnetic resonance imaging for the prediction of sentinel lymph node metastasis in breast cancer. Front Oncol. (2019) 9:980. doi: 10.3389/fonc.2019.00980
32. Huang YQ, Liang CH, He L, Tian J, Liang CS, Chen X, et al. Development and validation of a radiomics nomogram for preoperative prediction of lymph node metastasis in colorectal cancer. J Clin Oncol. (2016) 34:2157–64. doi: 10.1200/JCO.2015.65.9128
33. Yu J, Deng Y, Liu T, Zhou J, Jia X, Xiao T, et al. Lymph node metastasis prediction of papillary thyroid carcinoma based on transfer learning radiomics. Nat Commun. (2020) 11:4807. doi: 10.1038/s41467-020-18497-3
34. Schoppmann SF, Bayer G, Aumayr K, Taucher S, Geleff S, Rudas M, et al. Prognostic value of lymphangiogenesis and lymphovascular invasion in invasive breast cancer. Ann surgery. (2004) 240:306–12. doi: 10.1097/01.sla.0000133355.48672.22
35. Ejlertsen B, Jensen MB, Rank F, Rasmussen BB, Christiansen P, Kroman N, et al. Population-based study of peritumoral lymphovascular invasion and outcome among patients with operable breast cancer. J Natl Cancer Institute. (2009) 101:729–35. doi: 10.1093/jnci/djp090
36. Braman N, Prasanna P, Whitney J, Singh S, Beig N, Etesami M, et al. Association of peritumoral radiomics with tumor biology and pathologic response to preoperative targeted therapy for HER2 (ERBB2)-positive breast cancer. JAMA network Open. (2019) 2:e192561. doi: 10.1001/jamanetworkopen.2019.2561
37. Braman NM, Etesami M, Prasanna P, Dubchuk C, Gilmore H, Tiwari P, et al. Intratumoral and peritumoral radiomics for the pretreatment prediction of pathological complete response to neoadjuvant chemotherapy based on breast DCE-MRI. Breast Cancer research: BCR. (2017) 19:57. doi: 10.1186/s13058-017-0846-1
38. Wang Z, Zhang H, Lin F, Zhang R, Ma H, Shi Y, et al. Intra- and peritumoral radiomics of contrast-enhanced mammography predicts axillary lymph node metastasis in patients with breast cancer: A multicenter study. Acad Radiol. (2023) 30 Suppl 2:S133–s42. doi: 10.1016/j.acra.2023.02.005
Keywords: breast cancer, DCE-MRI, axillary lymph node, metastasis, radiomics
Citation: Wang Y, Shang Y, Guo Y, Hai M, Gao Y, Wu Q, Li S, Liao J, Sun X, Wu Y, Wang M and Tan H (2024) Clinical study on the prediction of ALN metastasis based on intratumoral and peritumoral DCE-MRI radiomics and clinico-radiological characteristics in breast cancer. Front. Oncol. 14:1357145. doi: 10.3389/fonc.2024.1357145
Received: 17 December 2023; Accepted: 04 March 2024;
Published: 19 March 2024.
Edited by:
Mariana Segovia, National Autonomous University of Mexico, MexicoCopyright © 2024 Wang, Shang, Guo, Hai, Gao, Wu, Li, Liao, Sun, Wu, Wang and Tan. This is an open-access article distributed under the terms of the Creative Commons Attribution License (CC BY). The use, distribution or reproduction in other forums is permitted, provided the original author(s) and the copyright owner(s) are credited and that the original publication in this journal is cited, in accordance with accepted academic practice. No use, distribution or reproduction is permitted which does not comply with these terms.
*Correspondence: Hongna Tan, bmF0YW4yMDAwQDEyNi5jb20=
†These authors have contributed equally to this work