- 1Department of Urology, Xinhua Hospital Affiliated to Shanghai Jiao Tong University School of Medicine, Shanghai, China
- 2Shanghai Jiao Tong University School of Medicine, Shanghai, China
- 3Department of Burn Surgery, The First Affiliated Hospital of Naval Medical University, Shanghai, China
Background: Prostate cancer (PCa) is one of the most threatening health problems for the elderly males. However, our understanding of the disease has been limited by the research technology for a long time. Recently, the maturity of sequencing technology and omics studies has been accelerating the studies of PCa, establishing themselves as an essential impetus in this field.
Methods: We assessed Web of Science (WoS) database for publications of sequencing and omics studies in PCa on July 3rd, 2023. Bibliometrix was used to conduct ulterior bibliometric analysis of countries/affiliations, authors, sources, publications, and keywords. Subsequently, purposeful large amounts of literature reading were proceeded to analyze research hotspots in this field.
Results: 3325 publications were included in the study. Research associated with sequencing and omics studies in PCa had shown an obvious increase recently. The USA and China were the most productive countries, and harbored close collaboration. CHINNAIYAN AM was identified as the most influential author, and CANCER RESEARCH exhibited huge impact in this field. Highly cited publications and their co-citation relationships were used to filtrate literatures for subsequent literature reading. Based on keyword analysis and large amounts of literature reading, ‘the molecular pathogenesis of PCa’ and ‘the clinical application of sequencing and omics studies in PCa’ were summarized as two research hotspots in the field.
Conclusion: Sequencing technology had a deep impact on the studies of PCa. Sequencing and omics studies in PCa helped researchers reveal the molecular pathogenesis, and provided new possibilities for the clinical practice of PCa.
1 Introduction
Prostate cancer (PCa), is estimated to become the second most common cancer in males, accounting for 14.1% of all cancer cases according to the global cancer statistics of 2020 (1). Besides, the specific mortalities attributed to PCa reached 375,304 worldwide in 2020, ranking fifth in all cancers (1). PCa is still a threatening health problem in the face of elderly males. Nowadays, the diagnosis of PCa mainly relied on physical examination, prostate-specific antigen (PSA) screening, and prostate biopsy. As for the treatments of PCa, androgen deprivation therapy (ADT) as well as prostatectomy were the most common strategies for localized PCa. However, for non-metastatic castration resistant prostate cancer (nmCRPC) and metastatic castration resistant prostate cancer (mCRPC), some novel drugs including androgen pathway inhibitors (APIs) and inhibitors of Poly ADP Ribose Polymerase (PARP1) (PARPi) had shown their unique therapeutic effects in PCa (2). The challenges in the field of PCa mainly existed in the lack of understanding of its molecular pathogenesis, the short of effective biomarkers for the early diagnosis and prognosis, and the resistance to the current therapies. But for many years, our grasp of PCa was hindered by the limitation of technology and the biological heterogeneity of PCa. Nevertheless, in the past few decades, with the development of sequencing technology and the occurrence of corresponding omics studies, the research on PCa has gained a huge leap. A more comprehensive landscape of PCa is gradually coming into our view.
Sequencing technology refers to the technology detecting the sequence of biomacromolecules in the central dogma of molecular biology, which covers determining base sequences in a DNA or RNA molecule, and the order of amino acids in a protein. In 1977, Sanger sequencing was invented by Walter Gilbert and Frederick Sanger, and scientists were able to detect the sequence of nucleic acid for the first time (3). However, the length of Sanger sequencing was restricted to 700-1000 bp, which obviously could not satisfy the urgent demand of gene sequences in modern biology. With the completion of ‘human gene project’ in 2003, the sequencing technology was propelled onto an accelerated trajectory of development (4). Around 2005, high-throughput sequencing, also called next-generation sequencing (NGS), broke the restriction of measuring one sequence at one time, and could detect millions of sequences in a single run (5, 6). The giant breakthrough in the field of DNA sequencing consequently catalyzed an unprecedented surge in genomics studies. In the subsequent period, with the maturity of RNA sequencing and protein sequencing, transcriptomics and proteomics had gained remarkable advancement (7, 8). Notably, the first single-cell RNA sequencing method (scRNA-seq) was developed in 2009, and brought a huge leap for the field of sequencing. ScRNA-seq could detect the heterogeneity of each single cell, and analyze the biological process at a microscopic resolution, providing solutions to many puzzled problems in the past (9). More recently, in 2011, single-molecule sequencing was developed, overcoming the disadvantage of NGS in the necessary preliminary PCR process and could directly sequence a single molecule without prior amplification step, which took sequencing technology into the third revolution (10).
The development of sequencing technology and the prosperity of the corresponding omics studies have achieved great success in PCa, especially for the discovery of molecular pathogenesis, and the identification of biomarkers or targets for the susceptibility, diagnosis, therapy, and prognosis of PCa. The genomics and epigenomics studies identified many important genetic alterations, which provided new insights into the possible pathogenesis of PCa. RNA-seq, particularly scRNA-seq, further detected the transcriptional profile of PCa, and identified a series of differentially expressed genes (DEGs) between cancer tissues and normal tissues. Besides, the application of mass spectrometry (MS) had unveiled a repertoire of crucial proteins and metabolites. These biomacromolecules held immense potential as prognostic and diagnostic predictors, or as prospective therapeutic targets of PCa.
Bibliometrics refers to the interdisciplinary science of quantitative analysis of all knowledge carriers by mathematical and statistical methods, which originated in the early 20th century and became an independent discipline in 1969. Bibliometrics analyzes published information (such as journal articles, books) and its associated metadata (such as citations, abstracts, and keywords), in order to characterize or demonstrate correlations between published works (11). A number of bibliometric studies have been conducted in the field of rheumatism, spinal cord injury, COVID-19, etc. (12–14). However, there has not been a comprehensive bibliometric study concerned with sequencing and omics studies in PCa. After a preliminary retrieval and reading several associated literatures, we discerned the vital significance of sequencing and omics studies towards PCa, and obtained large amounts of related literatures. Thus, we intended to conduct an objective and quantitative analysis of these literatures using the methods of bibliometrics, and proceed purposeful literature reading on the basis of this, in order to comprehensively understand the historical trajectory and research hotspots in this field.
2 Materials and methods
2.1 Data sources and retrieval strategies
The WoS database includes over 13,000 of the world’s most influential academic journals, spanning various fields including natural sciences, engineering, biomedical sciences, etc. Besides, the WoS is the only database that could export full record and cited references, which is essential for bibliometric analysis. Thus, we chose the WoS database to conduct the bibliometric study. Our search term was as follows: ((TS=((prostate OR prostatic) NEAR/2 (cancer* OR tumor* OR tumor* OR cancer* OR carcinoma* OR adenocarcinoma OR oncology))) AND ((TI=transcriptomic) OR (TI=proteome) OR (TI=proteomics) OR (TI=metabolomics) OR (TI=bioinformatics) OR (TI=metagenomics) OR (TI=metatranscriptomics) OR (TI=omics) OR (TI=microarray) OR (TI=sequence) OR (TI=RNA-seq) OR (TI=sequencing) OR (TI=ATAC-seq) OR (TI=single cell sequencing) OR (TI= single cell sequence) OR (TI=single cell RNA sequencing) OR (TI= single cell RNA sequence) OR (TI= expression profile) OR (TI= bioinformatic*) OR (TI= high throughput))). Up to July 3rd, 2023, a total of 7743 publications were retrieved. Selecting research articles and reviews, only 3499 publications were left. Finally, we filtrated 3325 publications associated in the WoS database (core collection) for further analysis.
2.2 Bibliometric methodology
Full records and cited references of the 3325 publications retrieved were downloaded from WoS, and put into further analysis. Bibliometrix is an R package developed by Massimo Aria and Corrado Cuccurullo based on bibliometrics. Through the visualization of the retrieved results using Bibliometrix, we could quickly understand the classic publications and leading figures in the field, and analyze the futural developing trend as well (15). VOSviewer is a computer program for bibliometric mapping based on the data normalization approach of probability theory, and is capable of generating a visualized map of collaborative network analysis, keyword co-occurrence analysis, co-citation analysis, coupling analysis and so on (16). Citespace is a practical visualized analysis tool for bibliometric research developed by Professor Chaomei Chen, which uses the data normalization approach of aggregation theory to accomplish the similarity evaluation of knowledge units (17). VOSviewer and Citespace were used as supplementary analysis tools for our study.
Quantitative statistics were made by Bibliometrix. In the source analysis and author analysis, H index, G index, and M index are used to evaluate the local impact of certain source or author. H index is defined as the number of documents with citation number >= H, which is used to characterize a journal’s or scholar’s scientific output and local impact (18). G index is defined as follows: given a set of articles ranked in decreasing order of citations, the G index is the (unique) largest number such that the top G articles receive (together) at least g2 citations (19). The M index is an author’s H index/the years since the first publication (20). Besides, local citations refer to the citations in the retrieving field, while total citations refer to the citations in the global field, and the above two indexes could be used to evaluate the local and total impact, respectively. Bradford Law is used to describe the constructure of literatures in a field. The specific content is as follows: if journals are arranged in a descending order according to publications in a certain field, we could distinguish these journals into several parts with publications’ ratio as 1: α: α2: ⋯ (α > 1). The first part is the core area with the highest outputs (21). Lotka’s Law is stated as follows: the number of authors publishing n articles approximately accounts for 1/n2 of the number of authors publishing one article in a certain field (22). In the publication analysis, co-citation network is an effective way to evaluate the relationships between the topics of two publications as co-citation means the frequency of which the two publications are cited at the same time (23). In the keyword analysis, co-occurrence network measures the correlation of keywords co-occurred in the same publication (24).
3 Results
3.1 Overview
Our analysis flow chart was shown in Figure 1. Till July 3rd, 2023, a total of 3225 publications were retrieved from 957 journals, books, etc. in WoS (core collection). The publication average year was 10.1, accompanied by an annual growth rate of 10.28%. The total references were 110700, and average citations per publications were 44.47. Especially, since 2001, both the publications and citations had shown an obvious increase. An explosive growth of publications and citations was seen in 2021 with a peak in Figure 2. As for the authors, a total of 18371 authors contributed to these publications, and 70 of them were authors of single-authored publications. The percentage of international co-authorships was 25.67%. In the publications, there were 7202 keywords plus (ID) and 5620 author’s keywords (DE) in all.
3.2 Countries and affiliations
The top 20 most productive countries were listed in Table 1. The USA and China were the most productive countries in the world, but the majority of publications were restricted in single-country collaboration (Figure 3A). The USA, Europe, and China were three research centers of sequencing and omics studies in PCa in the world, and had close collaborations with each other (Figure 3B).
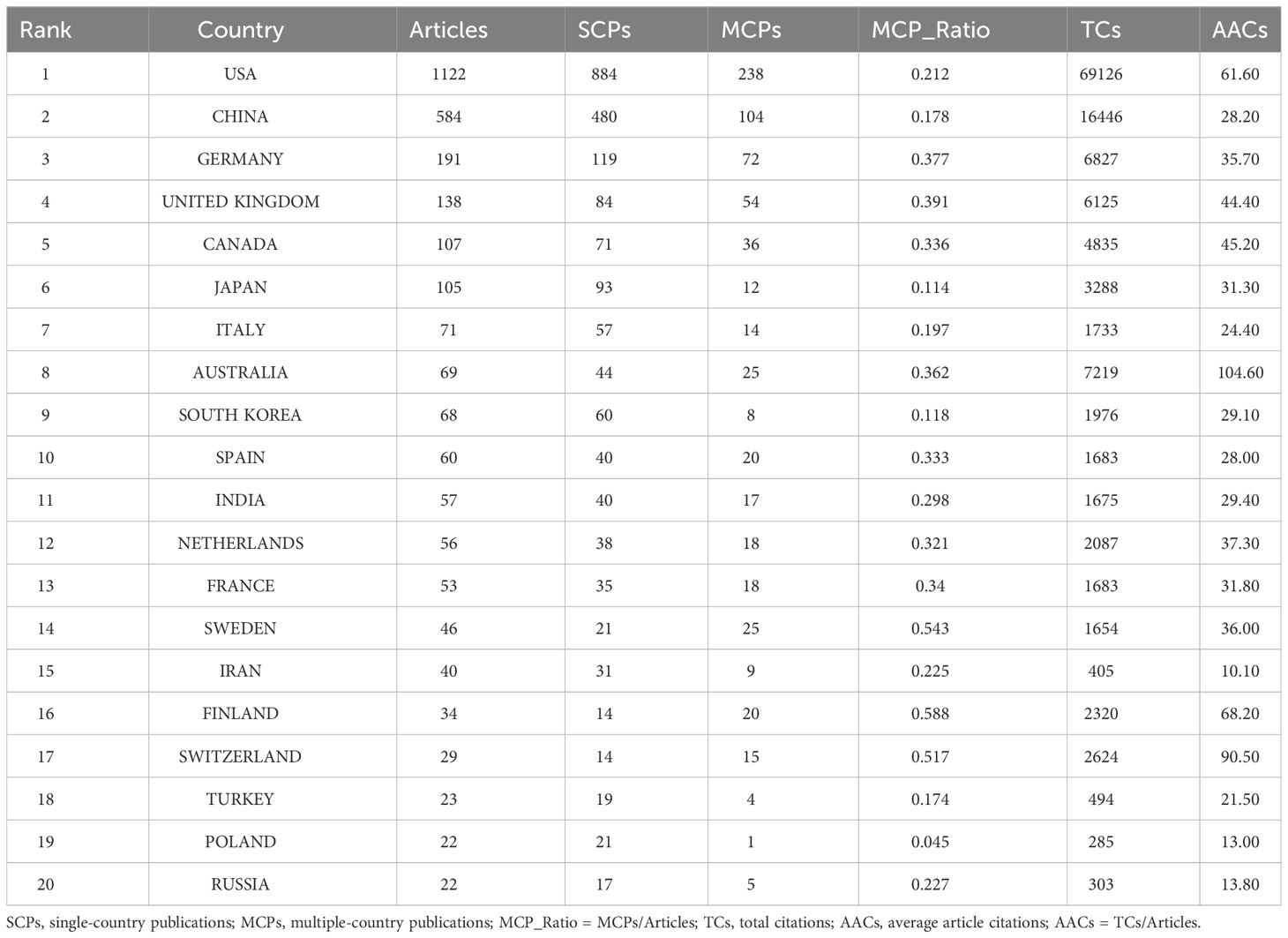
Table 1 The top 20 most relevant countries in the field of sequencing and omics studies in prostate cancer.
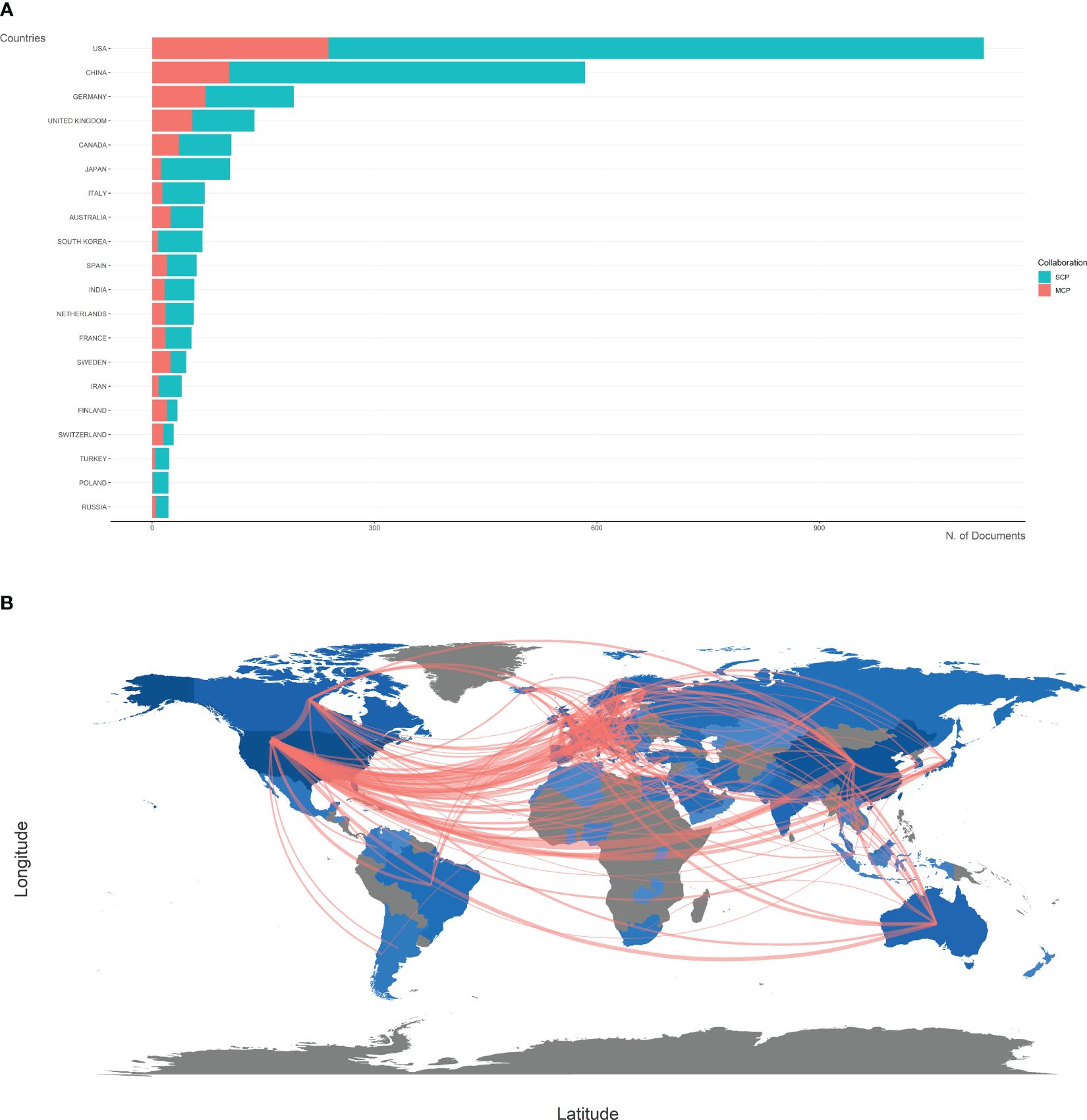
Figure 3 Country analysis. (A) The productions of top 20 countries in the field of sequencing and omics studies in prostate cancer were listed, with single-country publications and multiple-country publications shown separately. (B) The world map presented the productions of different countries and their collaboration with each other. SCP, single-country publication; MCP, multiple-country publication.
As for the corresponding authors’ affiliations, HARVARD UNIVERSITY (publications = 290), UNIVERSITY OF MICHIGAN (publications = 267), UNIVERSITY OF CALIFORNIA SYSTEM (publications = 240), NIH NATIONAL CANCER INSTITUTE (NCI) (publications = 213), and UNIVERSITY OF MICHIGAN SYSTEM (publications = 209) were the top five affiliations based on the number of publications (Supplementary Figure S1A). The above top five affiliations had close collaborations with each other (cluster red, cluster green, and cluster purple) (Supplementary Figure S1B).
3.3 Authors
Based on the number of publications, the top 20 most relevant authors were listed in Table 2 and Supplementary Figure S2A. Their scientific productions over time and the total citations per year were shown in Figure 4A. WANG Y (publications = 37), CHINNAIYAN AM (publications = 35), RUBIN MA (publications = 32), and SAUTER G (publications = 32) far surpassed the left authors in the aspect of production. Authors’ impact was evaluated by local citations, total citations, H index, G index, and M index. CHINNAIYAN AM (local citations = 330, total citations = 8222, H index = 28, G index = 35) was regarded as the most influential author in the field of sequencing and omics studies in PCa (Supplementary Figures S2B–D). All the authors could be divided into several groups, and authors inside each group had close collaboration with each other (Figure 4B). In the field of sequencing and omics studies in PCa, the distribution of authors roughly accorded with Lotka’s Law (Supplementary Figure S2E).
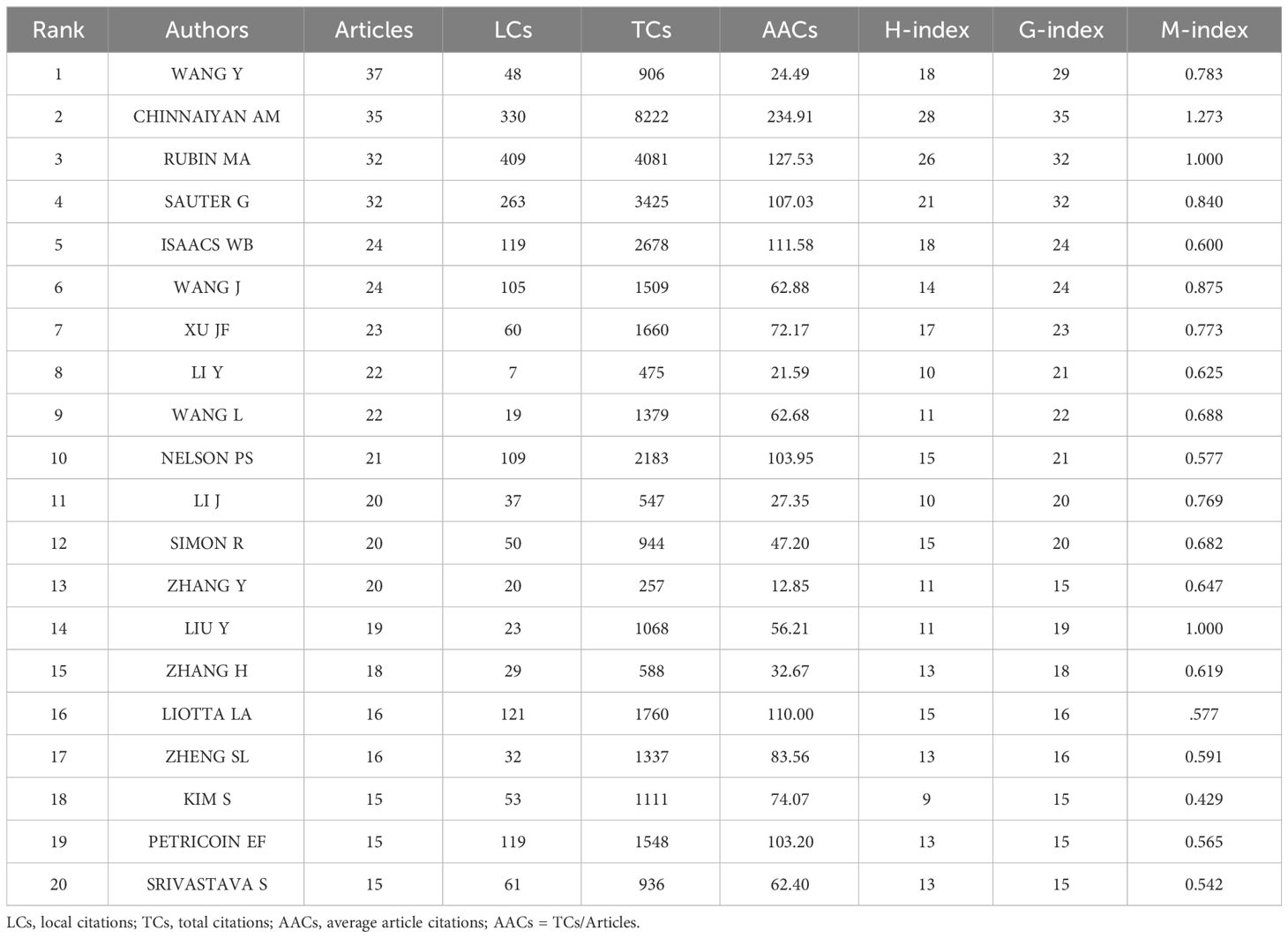
Table 2 The top 20 most relevant authors in the field of sequencing and omics studies in prostate cancer.
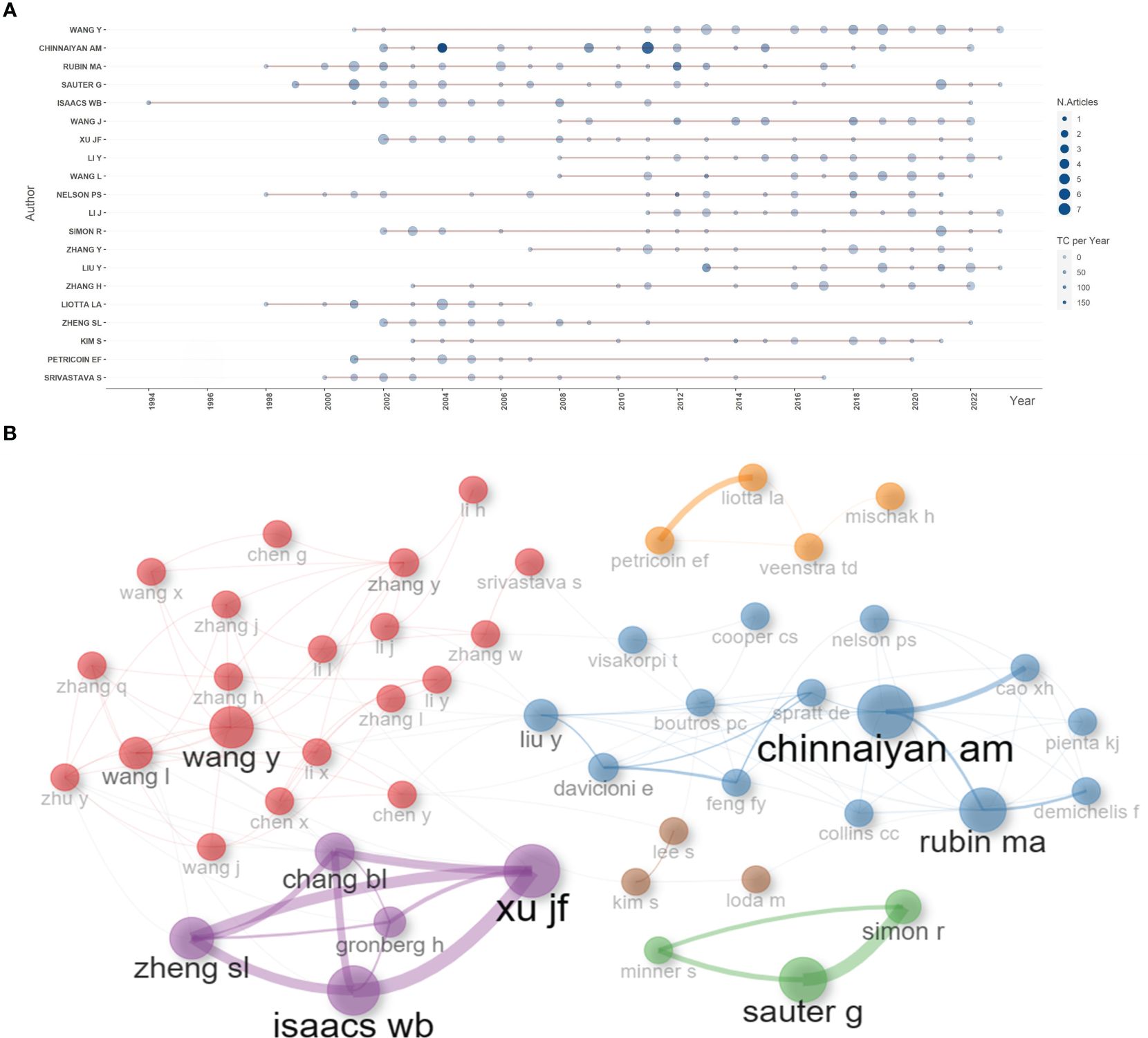
Figure 4 Author analysis. (A) The dynamic plot displayed the change of publications and total citations over time in the field of sequencing and omics studies in prostate cancer. (B) The collaboration network displayed the relationships among different authors in this field. TC, total citation.
3.4 Sources
Based on the number of publications, the top 20 most relevant sources were listed in Table 3. PLOS ONE ranked first, publishing 105 literatures, followed by PROSTATE, CANCER RESEARCH, and JOURNAL OF PROTEOME RESEARCH, with publications of 66, 56, 53, separately (Supplementary Figure S3A). Sources’ impact was evaluated by local citations, total citations, H index, G index, and M index. CANCER RESEARCH was regarded as one of the most influential sources in the field of sequencing and omics studies in PCa (local citations = 6378, total citations = 7983, H index = 44, G index = 56) (Supplementary Figures S3B-D). According to Bradford Law, the top 36 relevant journals were regarded as core sources in the field of sequencing and omics studies in PCa (Supplementary Figure S3E).
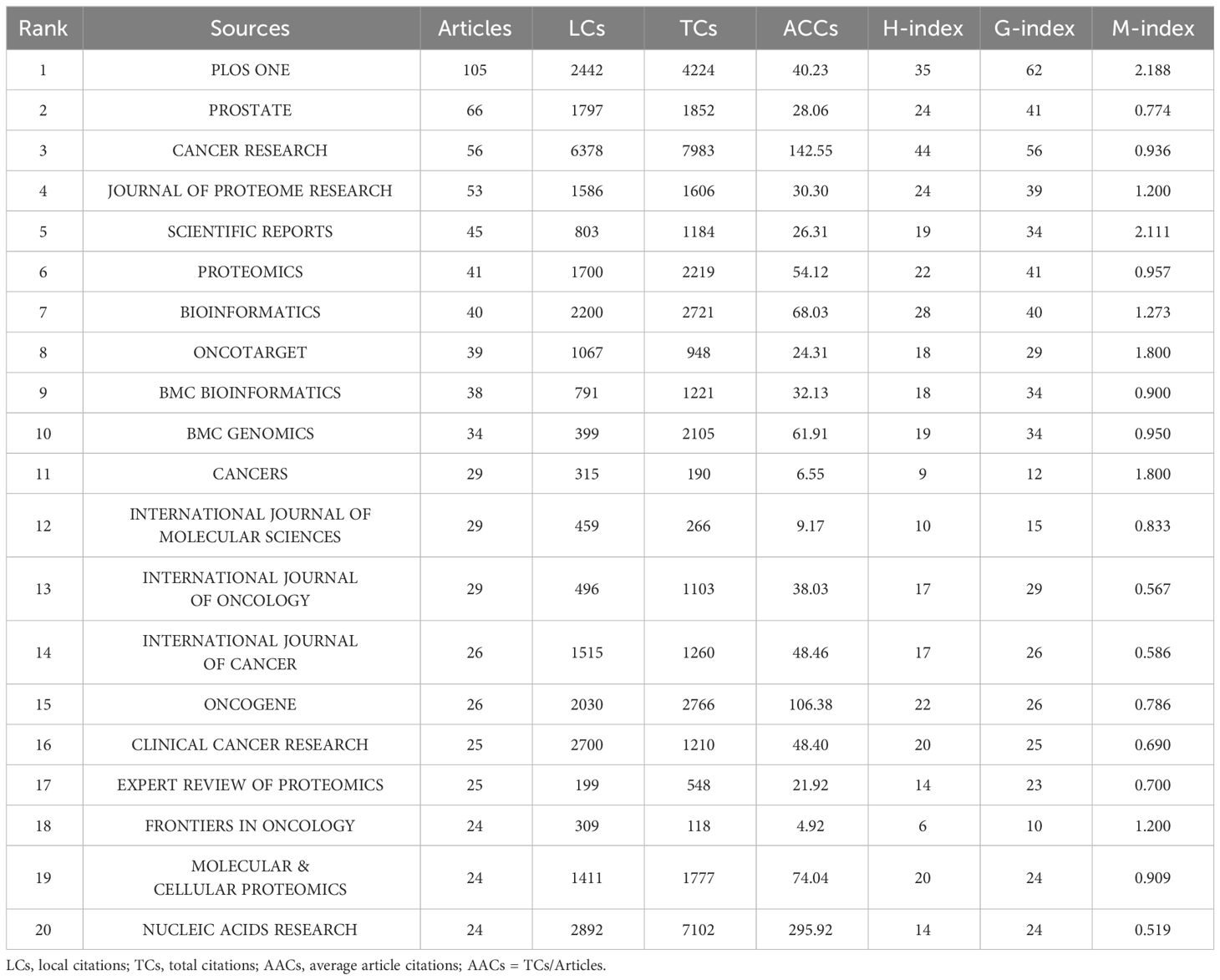
Table 3 The top 20 most relevant sources in the field of sequencing and omics studies in prostate cancer.
3.5 Publications
Based on total citations, the top 20 most total cited publications were listed in Table 4. ‘TANG ZF, 2017, NUCLEIC ACIDS RES’ (total citations = 5381), ‘YOUNG MD, 2010, GENOME BIOL’ (total citations = 4207), and ‘RHODES DR, 2004, NEOPLASIA’ (total citations = 2786) had the largest total impact (Figure 5A). Based on local citations, the top 20 most local cited publications were listed in Figure 5B. ‘LI JN, 2002, CLIN CHEM’ (local citations = 64), ‘BARBIERI CE, 2012, NAT GENET’ (local citations = 64), and ‘LUO J, 2001, CANCER RES’ (local citations = 62) had the largest local impact. In the co-citation network, the co-cited publications were distributed in the same cluster, thus different clusters probably represented different research hotspots in the field of sequencing and omics studies in PCa (Figure 5C).
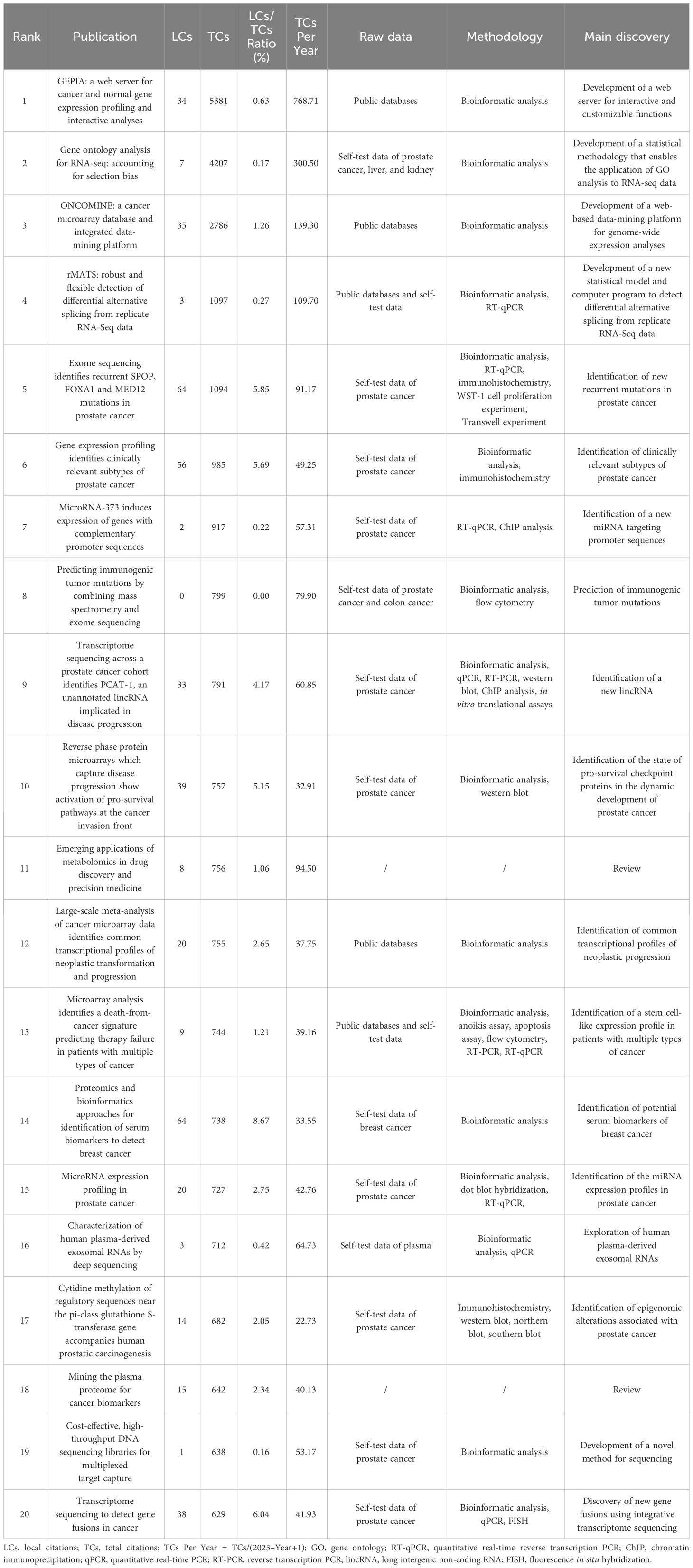
Table 4 The top 20 most total cited publications in the field of sequencing and omics studies in prostate cancer.
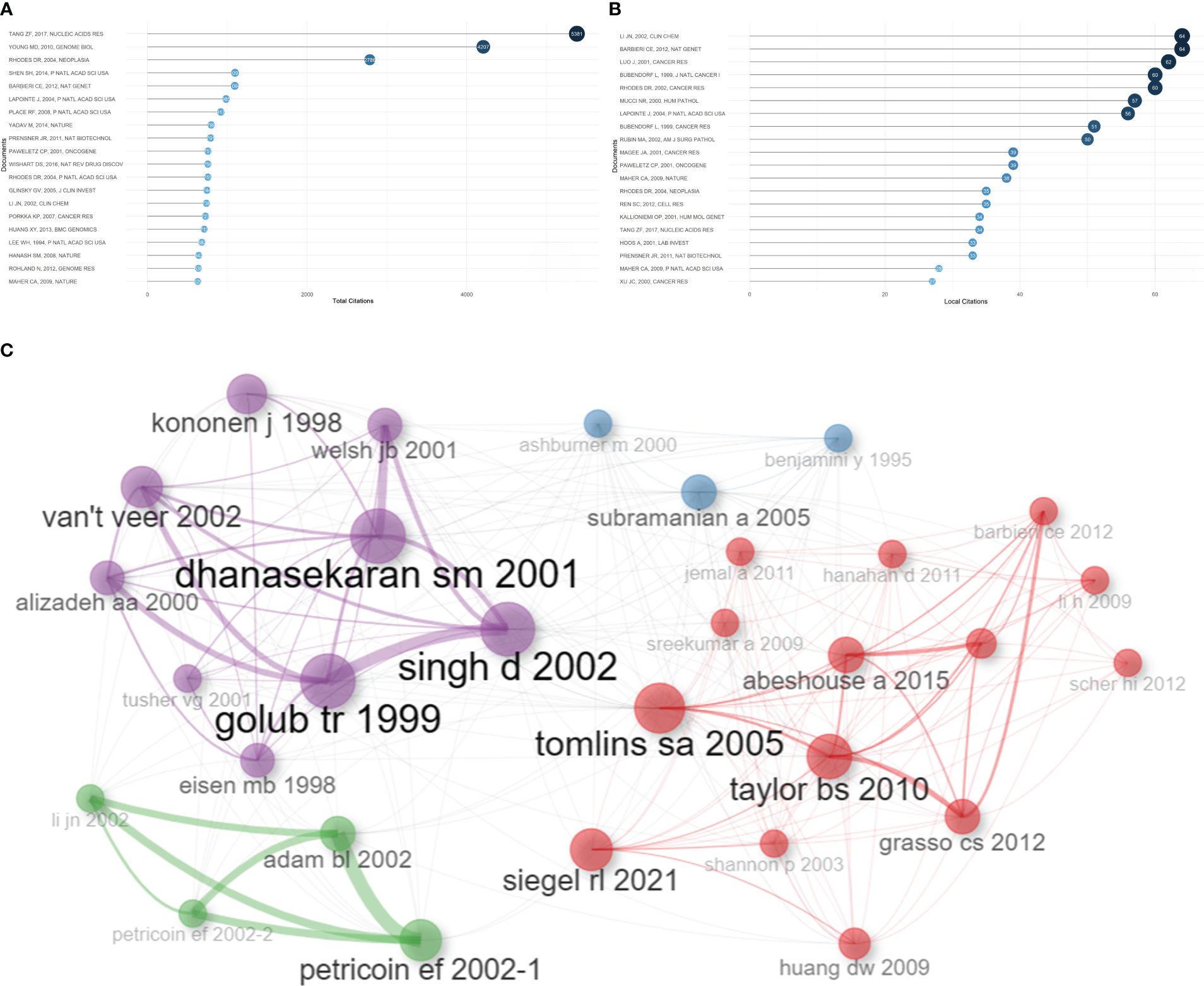
Figure 5 Publication analysis. (A) The top 20 most total cited publications in the field of sequencing and omics studies in prostate cancer were listed. (B) The top 20 most local cited publications were listed. (C) The co-cited publications were distributed in the same cluster in the co-citation network.
Besides, there was a disparity in quality among the included studies. Thus, we further listed the raw data, methodology, and major discoveries of the top 20 total cited publications, in order to analyze the common methods of these high-quality studies. Generally speaking, there were two main kinds of research methods in the field of sequencing and omics studies in PCa with high quality. The first one was developing a novel web platform through bioinformatic analysis of public databases. The other one was identifying new biomarkers of PCa through bioinformatic analysis together with the corresponding molecular, cell, and animal experiments based on self-test data. These results suggested that high-quality studies in this field not only required comprehensive bioinformatic analysis, but also needed experimental validation to bolster the discoveries (Table 4).
3.6 Keywords
Based on the occurrence of authors’ keywords, the most relevant keywords were listed in Figure 6A, and their evolution over time was shown in Figure 6B. The co-occurrence network exhibited three clusters of authors’ keywords in the field of sequencing and omics studies in PCa. Cluster 1 (red) included keywords like ‘microarrays’, ‘expression’, ‘gene expression profiling’, ‘apoptosis’, etc. and might represent the molecular pathogenesis of PCa. Cluster 2 (blue) covered keywords such as ‘diagnosis’, ‘biomarkers’, ‘biomarker discovery’, ‘proteomics’, ‘metabolomics’, etc. and possibly referred to the diagnosis of PCa. Cluster 3 (green) was consisted of keywords like ‘radical prostatectomy’, ‘immunotherapy’, ‘androgen receptor’, ‘prognosis’, ‘metastasis’, ‘biochemical recurrence’, etc. and indicated the therapy and prognosis of PCa (Figure 6C). Above all, there were two research hotspots in the field of sequencing and omics studies in PCa: the molecular pathogenesis of PCa and the clinical application of sequencing and omics studies in PCa.
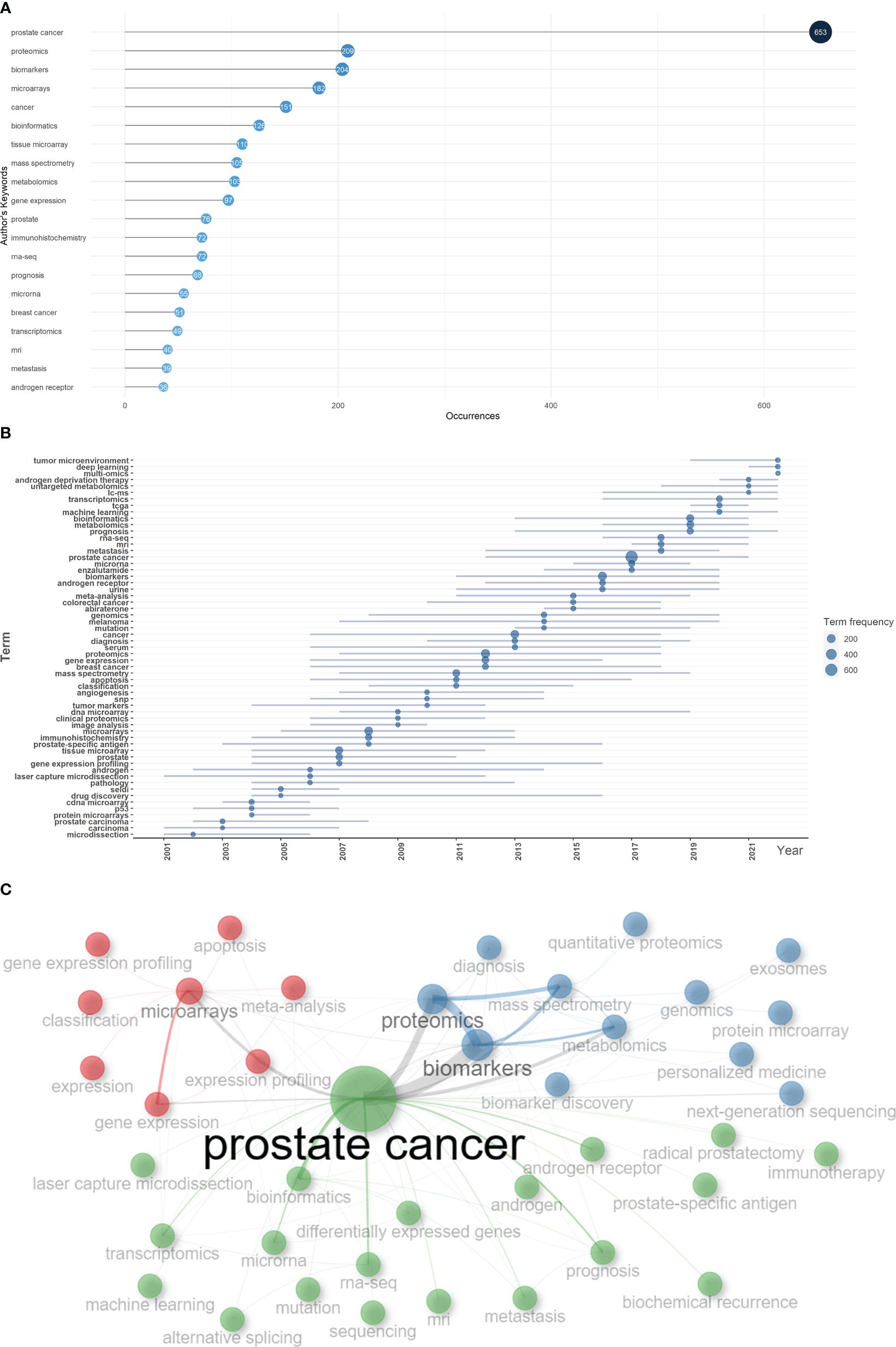
Figure 6 Keyword analysis. (A) The top 20 authors’ keywords in the field of sequencing and omics studies in prostate cancer were listed. (B) The dynamic plot displayed the change of keywords over time. (C) The keywords were distributed in different clusters in the co-occurrence map.
4 Discussion
PCa is the second most common cancer threatening the health of the elderly males worldwide (1). The major challenge in the field of PCa is the unclearness about the disease at a micro resolution, due to the limitation of technology. Nonetheless, in the past few decades, along with the rapid development of sequencing technology and the corresponding omics studies, we have witnessed a transformative evolution in the comprehension of PCa. This progress has effectively ushered researchers into the realm of the microworld of PCa, facilitating the gradual revelation of the intricate molecular biological properties characterizing the disease.
We conducted a bibliometric analysis in the field of sequencing and omics studies in PCa. Till July 3rd, 2023, a total of 3225 publications were retrieved from 957 journals, books, etc. in WoS (core collection). Since 2001, the publications had shown an obvious increase, especially for 2021. As for the most contributive countries in the world, the USA and China produced the majority of publications in this field. These two countries along with Europe harbored close collaborations with each other. WANG Y, CHINNAIYAN AM, RUBIN MA, and SAUTER G were the most productive authors in the field of sequencing and omics studies in PCa. Among them, CHINNAIYAN AM was regarded as the most influential author in the world. All the authors could be divided into several collaboration groups and maintained close relationships with each other. PROSTATE, CANCER RESEARCH, and JOURNAL OF PROTEOME RESEARCH were the top 3 journals which had the highest publications, and CANCER RESEARCH harbored the largest impact in this field. In the publications analysis, the literatures in this field were classified into different clusters in the co-citation network, possibly indicating different research hotspots. Most importantly, we listed the most frequent authors’ keywords along with their dynamic evolution over time, and ulteriorly classified these keywords into three clusters indicating different research topics. In conclusion, ‘the molecular pathogenesis of PCa’ and ‘the clinical application of sequencing and omics studies in PCa’ were two research hotspots in the field of sequencing and omics studies in PCa, and the latter one could be further classified into the susceptibility, diagnosis, therapy, and prognosis of PCa.
Based on the above bibliometric analysis, we selected the top 100 local cited publications, the top 100 total cited publications, as well as the publications in the historialgraph, co-citation network, etc. for purposeful literature reading, and summarized the achievements of different omics studies. As for genomics studies, large amounts of gene mutations in PCa were discovered, especially for the rearrangements of ETS family. Additionally, genome itself could be used as a diagnostic tool. Epigenomics studies mainly focused on epigenetic alterations occurred in PCa, like DNA methylation, and analyzed their impacts on gene expression. Transcriptomics was most frequently applied to detect DEGs and gene fusions across diverse tumor samples. Besides, some non-coding RNAs like miRNAs were also analyzed, and their special functions in PCa gradually emerged. Proteomics had advantages in detecting differentially expressed proteins as potential biomarkers for the diagnosis, prognosis of PCa. Besides, some metabolites were also identified as biomarkers in metabolomics studies. However, the profound significance of metabolomics existed in describing special metabolic phenotype of PCa. Multi-omics, which integrated genomics, epigenomics, transcriptomics, proteomics, metabolomics, etc. altogether, had unique advantages in the studies of PCa. Multi-omics studies were instrumental in constructing regulation networks in PCa, and identifying potential biomolecular mechanisms beneath the disease. Besides, multi-omics studies compensated for the limitations of single omics and harbored an advantage in accurately detecting biomarkers. Last but not least, multi-omics studies included the data of DNAs, RNAs, proteins, etc., thus providing a comprehensive model for the classification and prognosis evaluation of PCa. Above all, the research outputs of omics studies, summarized through large amounts of literature reading, could be classified into several themes encompassing the pathogenesis, susceptibility, diagnosis, therapy, and prognosis of PCa, which perfectly corresponded with the two hotspots we identified in the bibliometric analysis. In the following discussion part, we will further picture the historical trajectory of these two research hotspots in the field of sequencing and omics studies in PCa (Figure 7).
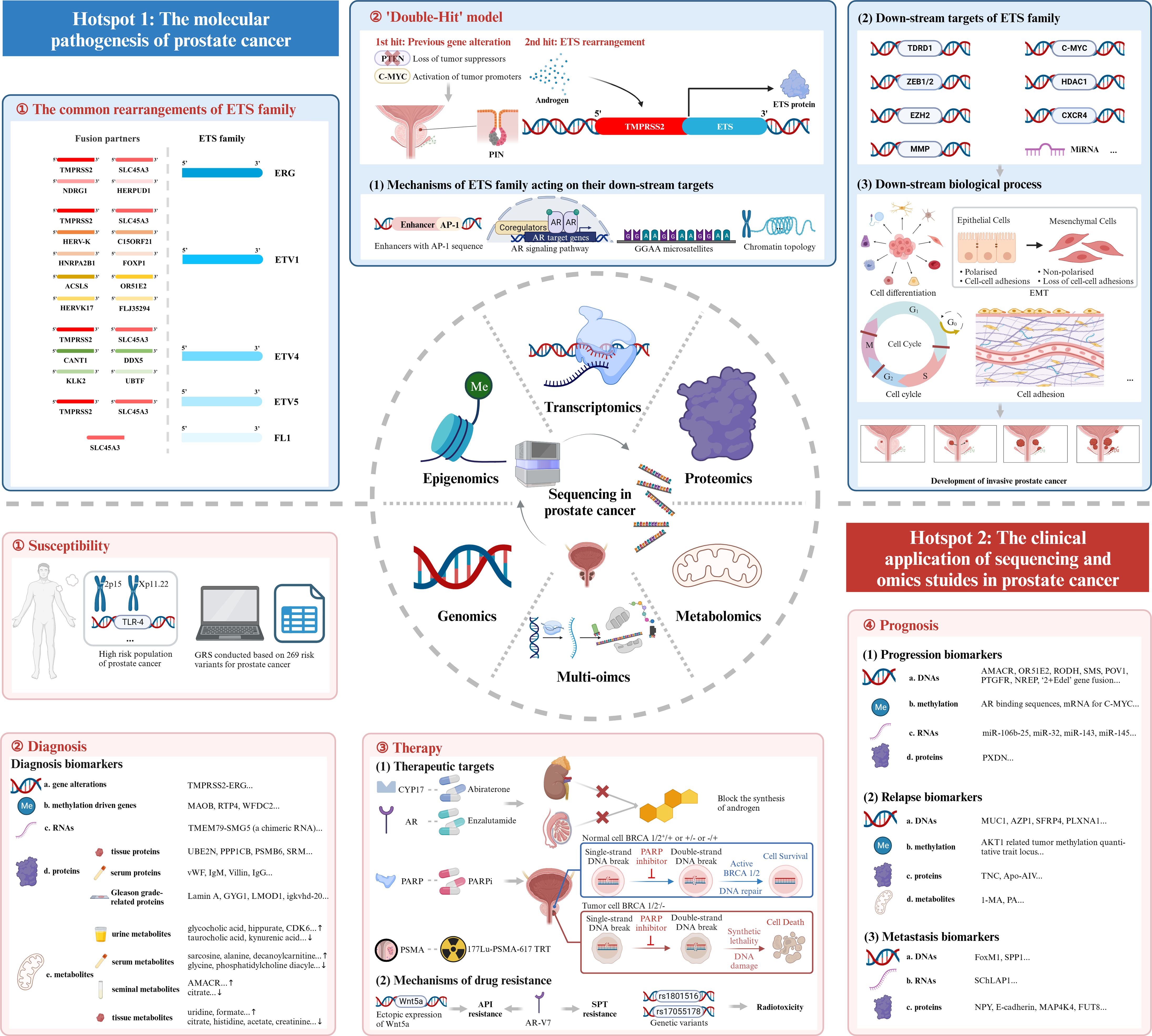
Figure 7 Mechanism diagram. ETS family, E26 transformation-specific family; AR, androgen receptor; EMT, epithelial-mesenchymal transition; GRS, genetic risk score; PARP, Poly ADP Ribose Polymerase; PARPi, inhibitor of PARP; PSMA, prostate-specific membrane antigen; TRT, targeted radionuclide therapy; API, androgen pathway inhibitor; AR-V7, androgen receptor splice variant 7; SPT, supraphysiological testosterone.
4.1 The molecular pathogenesis of prostate cancer
4.1.1 The discovery of the E26 transformation-specific family rearrangements in PCa
The first research hotspot in the field of sequencing and omics studies in PCa was the molecular pathogenesis of PCa. Through bibliometric analysis and large amounts of literature reading, we found that the rearrangement of ETS family genes was regarded as the most important pathogenesis of PCa. ETS family proteins were a large family of 28 transcription factors, which had long been implicated in tumorigenesis (25). Five members of them, ERG, ETV1, ETV4, ETV5 and FL1, were seriatim identified by researchers, illustrating their oncogenic function in PCa. Tomlins et al. initially identified the rearrangements of ERG and ETV1 in 50% and 5-10% of PCa (26–28), followed by the discovery of the rearrangements of ETV4 and ETV5 (29–32). FL1, fused to SLC45A3 gene, was the fifth rearranged ETS family gene identified in PCa (33). TMPRSS2, a prostate-specific androgen reactive gene, was the most frequent gene fused to ETS family genes to form the gene rearrangements. Apart from this, other genes sharing analogous functions like SLC45A3, HERV-K_22q11.23 also took part in the rearrangements of ETS family genes. Here, we intended to elaborate the pathogenesis of TMPRSS2-ETS (+) PCa, in which the 5’ untranslated region of TMPRSS2 was fused to ETS, due to its highest occurrence in PCa (26). Other rearrangements of ETS family genes may harbor similar oncogenic mechanisms.
4.1.2 The down-stream targets of ETS family genes
TMPRSS2 was activated by androgen, and launched the expression of ETS family genes. Then, the overexpressed ETS proteins combined with ETS targets and induced the down-stream signaling pathways. It was of great importance to explore the ETS target genes and the corresponding down-stream mechanisms so as to figure out the complicated oncogenic function of ETS rearrangements. Most of the studies were conducted to discover the target genes of ERG. Manuel R Teixeira et al. filtrated seven tumor-associated ERG target genes (CACNA1D, PLA1A, HLA-DMB, ATP8A2, PDE3B, TDRD1, and TMBIM1) in PCa (34). Among them, TDRD1 was the first confirmed gene that was directly regulated by ERG, and encoded the tudor domain-containing protein 1, involved in male germ cell differentiation and in the small RNAs pathway. But its special function in PCa had not been uncovered (34). Olli Kallioniemi et al. identified the up-regulation of histone deacetylase 1 (HDAC1) as the most common characteristic in PCa with TMPRSS2-ERG gene fusion (35). HDAC1 could induce the activation of urokinase-type plasminogen activator (uPA), matrix metalloprotease-1 (MMP-1) and MMP-2, but suppressed E-cadherin in order to accelerate the invasion and migration of prostate cancer cells (36–38).
Cell dedifferentiation was an important characteristic of tumorigenesis, and the differentiation degree of malignant cells usually represented the malignant grade of PCa. As for MMPs, ERG had been discovered to activate the transcription of MMP-1, resulting in loss of cell adhesion, which led to the dedifferentiation of prostate cells (39). Besides, C-MYC and polycomb group protein EZH2, a stemness-related gene, were also found to be down-stream factors of ERG influencing the dedifferentiation process (40, 41). EZH2 could also catalyze the methylation of ERG at the lysine 263 residue, and reversely enhance the oncogenic activity of ERG (42, 43).
Epithelial-mesenchymal transition (EMT) referred to the biological process of the transformation from epithelial cells to mesenchymal cells, characterized by less cell polarity and weaker cell adhesion, which played a role in oncogenesis (44). Wnt/β-catenin pathway was activated by ERG and resulted in EMT to increase the survival and invasive properties of prostate cells (45). TMPRSS2-ERG could also directly bind to Zinc finger e-box binding homeobox 1 (ZEB1) promoter and ZEB2 modulators and increase the expression of ZEB1 and ZEB2, which were key regulators in the EMT (46, 47).
Cell adhesion might affect the migration of cancer cells, and lead to the progression of cancers. Chemokine receptor type 4 (CXCR4) was activated by TMPRSS2-ERG. Combined with its ligand CXCL12, CXCR4/CXCL12 axis increased the aggressiveness of PCa and enhanced the ability of prostate cancer cells to adhere to the extracellular matrix. The axis could also indirectly accelerate the tumorigenesis via increasing the expression of MMPs (48–51).
Several other down-stream targets of ERG were also identified. MicroRNAs (MiRNAs) were another target of ERG. It had been revealed that ERG inhibited the expression of miR-200c, which down-regulated EMT-related genes and acted as a tumor suppressor (52). MiR-221, a cell cycle-related miRNA regulating the phase transition from G0 to S, showed a decreased expression in patients with TMPRSS2-ERG gene fusion (53, 54). As for cell cycle, ETS-E2F fusion oncoproteins destroyed the normal cell cycle control and took part in the tumorigenesis of PCa (55). Changmeng Cai et al. discovered that soluble guanylyl cyclase (sGC) might be a novel transcription target of TMPRSS2-ERG. The NO-cGMP pathway mediated by sGC played an important role in accelerating the proliferation of cancer cells (56).
ETV1, another important member of ETS family, also harbored unique function in PCa, even sometimes opposite to the function of ERG. Although phosphatase and tensin homolog gene (PTEN), MMPs, urokinase type plasminogen activator/urokinase type plasminogen activator receptor (uPA/uPAR) systems had been described as ETV1-related genes (33, 57), we still lacked large amounts of studies to describe the global landscape of gene expressions regulated by ETV1. We intended not to illustrate the down-stream biological process of other ETS family genes in detail, due to their relatively low occurrence of rearrangements in PCa.
4.1.3 The mechanisms of oncogenic ETS family acting on their targets
Subsequent studies elaborated the complicated mechanisms of oncogenic ETS family acting on their targets. A category of enhancers combined with ETS family had been identified in PCa. These enhancers had a special ETS-binding sequence named AP-1, which was composed of dimers of JUN and FOS family transcription factors (58). ETS/AP-1 enhancer elements were found to be located near the genes associated with cell migration, cell morphogenesis, and cell development, like PLAU, VIM, etc., and might affect the expression of these genes (59). In addition, ETS family was associated with androgen receptor (AR) signaling pathways. Whole-genome ChIP-seq had shown the overlap between the ERG-binding area and AR-binding area. Thus, the overexpression of ERG may have an influence on AR-binding genes in PCa. Arul M Chinnaiyan et al. had raised a model in which the overexpression of ERG inhibited the AR-regulated differentiation but induced the dedifferentiation mediated by the H3K27 methyltransferase EZH2 (60). However, ERG showed an opposite function of enhancing AR-related pathways when PTEN, a phosphatase inhibiting the development of tumor by antagonizing the activity of phosphorylases, was lost (61). ETV1 was found to cooperate with the AR signaling pathways in LNCaP cells (hormone-dependent human PCa cell lines) and PTEN deficient mouse models of PCa. Additionally, ETV1 could also directly up-regulated genes associated with steroid hormone synthesis and androgen metabolism to increase the expression of testosterone, followed by an up-regulated transcriptional activity of AR (62, 63). Besides, Peter C. Hollenhorst et al. found that ETS family could bind an unusual regulatory sequence consisting of repeats of the sequence GGAA, called GGAA microsatellites, and activate the down-stream target genes (64). Apart from this, ETS family genes functioned as transcriptional activators or suppressors when they were combined with different molecules. CBP/p300, MED25 could combine with ETS family to perform a transcriptional activated function, while EZH2, HDAC1, HDAC2, and FOXO1 inhibited ETS-induced gene transcription (65). Last but not least, ETS family could significantly impact the topology of chromatin to influence the gene transcription, since the interaction of ETS family with many chromatin modifying enzymes like p300, HDAC1, EZH2, PRMT5, KDM4A, SETPB1, etc. (66–70).
The overall pathogenesis of TMPRSS2-ETS gene fusion in PCa could be concluded as a ‘Double-Hit’ model. Previous genetic alterations like gene mutations, inhibition of tumor suppressors and activation of tumor promoters might accelerate the progression of prostatic intraepithelial neoplasia (PIN), and the subsequent molecular biological change mediated by ETS rearrangements promoted the development of invasive PCa. The combination of the biological effects of ETS rearrangements and existed genetic alterations contributed to the tumorigenesis of PCa together (28).
4.2 The clinical application of sequencing and omics studies in prostate cancer
4.2.1 Susceptibility
The application of sequencing and omics studies in PCa had revealed certain population that were more susceptible to PCa. In a Genome Wide Association Study (GWAS) in 2008, common sequence variants on 2p15 and Xp11.22 were found to be associated with susceptibility to PCa (71). Another study revealed toll-like receptor 4 (TLR-4), a candidate inflammatory gene, was associated to high risk of PCa when its 3’-untranslated region suffered mutations (72). Through a large multiancestry GWAS meta-analysis, 86 novel risk variants associated with the susceptibility to PCa were identified. Integrated with the known risk loci for prostate cancer, ‘genetic risk score (GRS)’ was conducted on the basis of 269 risk variants for PCa. GRS could effectively stratify the risk of PCa across populations, and had been verified in two independent cohorts of men of European and African ancestry (73).
4.2.2 Diagnosis
The diagnosis of PCa was currently based on the microscopic evaluation of prostate tissue obtained via needle biopsy. A pathologist examined these tissues and reported the Gleason grade of the predominant histological pattern and the secondary histological pattern. Then, clinicians classified PCa into low-, intermediate-, and high-risk groups according to the sum of Gleason patterns, PSA, and clinical stage. However, the biomarker of PSA harbored a certain probability of false positives and false negatives, and the biopsy was an invasive procedure that may induce discomfort and pain in patients (74). We urgently demanded more specific and sensitive biomarkers to improve the diagnosis effectiveness of PCa.
Through the application of sequencing and omics studies to PCa, a series of genetic alterations had been discovered, which was significant to the diagnosis of PCa. Since the rearrangements of ETS family was the most important pathogenesis in PCa, these genetic alternations might serve as potential diagnosis markers of PCa. Mao X et al. and Arul M Chinnaiyan et al. had detected the gene fusion of TMPRSS2-ERG in circulating tumor cells and urine sediments from PCa patients, respectively (75, 76). And the combined detection of TMPRSS2-ERG and PCA3 significantly improved the sensitivity of diagnosis (77). However, TMPRSS2-ERG, as a diagnosis biomarker, had obvious limitations due to the existence of ETS gene fusion negative PCa (28). Besides, the epigenetic modifications could also perform as diagnosis biomarkers of PCa. Xue−Yi Xue et al. demonstrated key DNA methylation-driven genes like MAOB and RTP4, as novel biomarkers for the accurate prognosis of PCa (78). In 2011, Arul M. Chinnaiyan et al. painted a DNA methylation profile of PCa. CpG islands (CGI) referred to a certain area of DNA that contained large amounts of connected cytosine and guanine. CGI usually located in the transcription regulation domain, and remained non-methylated. However, in PCa, Arul M. Chinnaiyan et al. found that the methylation level was significantly up-regulated, especially for WFDC2. The promoter of WFDC2 showed recurrent methylation in PCa, but remained non-methylated in normal tissues, which might harbor diagnostic value in PCa (79). TMEM79-SMG5, one chimeric RNA, was another potential diagnostic biomarker of PCa. In comparison with TMPRSS2-ERG, which could be found in about 50% of the PCa patients, TMEM79-SMG5 was detected by RT-PCR in approximately 90% of PCa samples tested (80). Proteins were the most common biomarkers for the diagnosis of PCa. A number of differentially expressed proteins were observed in PCa tissues, like UBE2N, PPP1CB, PSMB6, metalloproteinase inhibitor-1, PCa-24, SRM, NOLC1, and PTGIS (81–84). These proteins might offer valuable insights for the diagnosis of PCa. Besides, Brian B Haab et al. identified five serum biomarkers (von Willebrand Factor, immunoglobulinM, Alpha1-antichymotrypsin, Villin, and immunoglobulinG) exhibiting significant differential expression levels between PCa samples and control samples, which may also serve as potential diagnosis biomarkers (85). Notably, several proteins were found to be in accordance to the Gleason grade of PCa. Lukas Alfons Huber et al. discovered that the expression level of Lamin A corresponded with the Gleason grade, qualifying itself as a potential biomarker for tumor differentiation and prognosis (86). Likewise, in 2019, GYG1, LMOD1, igkvhd -20, and RNASET2 were also identified as four proteins correlated with the Gleason grade of PCa (87). Metabolites well reflected the physiological process in an individual. Metabolome, located in the downstream of central dogma, was more directly associated to the phenotype and harbored more direct clinical significance, compared to genome, transcriptome, etc. The study of metabolomics provided a useful way to identify potential biomarkers in biofluid, which may offer non-invasive diagnosis indexes for PCa. The most reported biomarkers in PCa situated in urine, serum, and seminal fluid. In urine, several metabolites exhibited upregulation, including glycocholic acid, hippurate, 7-methylguanine, FABP5, BCAAs, AMBP, CDK6, etc., while taurocholic acid, kynurenic acid, glucoronate, glycine, etc. showed obvious down-regulation (81, 88–94). In serum samples, an elevated expression was observed for sarcosine, alanine, pyruvate, decanoylcarnitine, etc., but glycine, citrate, phosphatidylcholine diacyl exhibited the opposite trend (95–97). Concerning seminal fluid, citrate was down-regulated in 2 reported studies, but AMACR was up-regulated (98–100). However, the metabolomic biomarkers identified in tissues may be more significantly changed than those in biofluid. Citrate, histidine, acetate, creatinine, valine, glycine, lysine, leucine, glutamine, and choline were decreased in PCa tissues, however, uridine and formate were oppositely increased (101, 102). The number of biomarkers that had been identified was so huge to list, but hardly any of them were applied to clinical practice. Multi-omics studies might provide a novel method of diagnosis based on the integrated data. In 2018, epigenomics, transcriptomics, proteomics, and metabolomics studies were applied to a set of patients receiving radical prostatectomy. The prediction model merging the data of DNA methylation, transcripts, proteins, and glycosylation biomarkers successfully increased prognosis predicting accuracy of PCa (103).
4.2.3 Therapy
The application of sequencing and omics studies in PCa helped us to discover the novel potential therapeutic targets of PCa. A series of studies had shown that the increased copy numbers and the point mutation of AR were the most frequent genetic alterations in castration-resistant prostate cancer (CRPC) (104–106). The complicated rearrangements of AR included highly recurrent tandem duplications concerned an up-stream enhancer of AR (107). More recent studies had illustrated the heterogeneity of AR-activity in primary PCa as well. The subtype of primary PCa with low AR-activity harbored similar biological characteristics to mCRPC, and was more likely to develop the resistance to ADT, docetaxel, etc. (108). Since the AR-related pathways were of great importance in PCa, several target drugs had been developed. Abiraterone, which targeted CYP17, the key enzyme in the synthesis of androgen, and enzalutamide, the second-generation AR inhibitors had been widely applied in the therapy of CRPC (109). DNA damage repair (DDR) deficiency was another frequent genetic alteration in PCa. It had been discovered that the inactivation of core homologous recombination (HR) and HR-associated genes, such as BRCA1, BRCA2, CHEK2, PALB2, CDK12, CHD1, ATM, RAD54L, RAD51B, or PTEN, occurred in a considerable proportion of localized PCa, even more frequently in metastatic PCa (110). This particular subtype of PCa was sensitive to PARPis via the mechanism of synthetic lethality, as PARPi blocked the other DDR pathway, base excision repair, as well (111). Several PARPi including olaparib, rucaparib had been studied in phase 3 trial to treat PCa (112, 113). Last but not least, prostate-specific membrane antigen (PSMA), a type II transmembrane glycoprotein, was fairly lowly expressed in normal prostate tissues, but showed a massive expression in PCa tissues, offering another therapeutic target for PCa (114). As a result, a series of PSMA target therapies, including PSMA-targeted radionuclide therapy (PSMA-TRT), PSMA-antibody-drug conjugates (PSMA-ADC), PSMA-based chimeric antigen receptor (CAR)-T cells therapy had been developed, especially for 177Lu-PSMA-617 TRT (115). Several relatively less reported therapeutic targets were also revealed by the application of sequencing and omics studies in PCa. Felix Y Feng et al. conducted a systematic study of mCRPC integrating whole-genome, whole-methylome, and whole-transcriptome sequencing. A novel epigenetic CpG Methylator Phenotype (CMP) subtype of mCRPC was identified, with characteristics of hyper-methylation both within and outside of CpG islands, shelves, and shores. This subtype harbored potential therapeutic significance as methylation inhibitors were FDA-approved anti-tumor drugs (116). Another transcriptomics-proteomics-metabolomics combined study revealed the CDK9 (cyclin-dependent kinase 9)-inhibition responses of PCa cells. Acute metabolic stress was induced by using pan-CDK-inhibitor AT7519. AT7519 treatment caused the accumulation of acyl-carnitines, metabolic intermediates in fatty acid oxidation (FAO) produced by carnitine palmitoyltransferase (CPT) enzymes 1 and 2. Co-inhibition of CDK9 and CPT1/CPT2 was lethal to PCa cells (117).
Apart from the potential of discovering therapeutic targets, the application of sequencing and omics studies in PCa could also help evaluate the therapeutic effect and reveal the mechanisms of drug resistance. AR-inhibitor was one of the most effective therapies in PCa, but therapeutic effects varied among patients. Transcriptomic heterogeneity of AR activity well explained the interesting phenomenon (108). Besides, patients receiving AR-inhibitor therapy had shown an activation of atypical Wnt pathway, and the ectopic expression of Wnt5a reduced the anti-proliferation function of AR inhibitors (118). Moreover, the mutations of AR also contributed to the failure of API treatment. With the assistance of sequencing technology, androgen receptor splice variant 7 (AR-V7), characterized by a truncated ligand-binding domain (LBD), was found to be associated to the drug resistance of API, as LBD was the direct target of enzalutamide and indirect target of abiraterone (119). Supraphysiological testosterone (SPT)-based therapy to some extent improved life quality of some CRPC patients, but the resistance of SPT was still a severe problem in front of us. Through transcriptomics study, Eva Corey et al. discovered that SPT durable response was associated with the sustained suppression of E2F and AR-V7 transcriptional output and the impairment of DDR. The combination therapy of SPT and AR-V7/DDR targeted drugs may well improve the response of SPT (120). Last but not least, as for radiotherapy, in 2020, a genome-wide association meta-analysis confirmed several common genetic variants associated with the susceptibility to radiotoxicity of PCa therapy, including rs1801516, rs17055178, etc. (121).
4.2.4 Prognosis
4.2.4.1 Progression
Omics studies had identified plenty of prognostic predictors, associated with the progression of PCa. DEGs might contribute to the progression of PCa. Compared to normal epithelial cells, 21 up-regulated genes and 63 down-regulated genes including AMACR, OR51E2, RODH, SMS, etc. were found in PIN and PCa, which may mediate the early onset of PCa. Besides, other DEGs were also observed in the transformation from PIN to PCa, including POV1, CDKN2C, EPHA4, FASN, LAMB2, etc., which contributed to the malignant progression (122). Based on machine learning, Luis Rueda et al. identified DOCK9, PTGFR, NREP, SCARNA22, FLVCR2, CLASP1, IK2F3, and USP13 as potential biomarkers predicting the progression of PCa, especially between stage II and subsequent stages (123). Besides, a special type of gene fusion could also well predict the progression of PCa. Based on the studies of ERG rearrangements in PCa, researchers had identified a novel category of PCa, characterized by ‘2+Edel’, which was defined as duplication of the fusion of TMPRSS2 to ERG sequences together with interstitial deletion of sequences 5’ to ERG. ‘2+Edel’ was found to be correlated with aggressiveness and usually resulted in worse clinical outcomes. Notably, ‘2+Edel’ might be a more reliable prognostic marker than Gleason grade, due to its stability independent of observers or time (124). As an important component of epigenetic modification, methylation could activate or silence the expression of target genes to influence the progression of PCa. Gerhardt Attard et al. described the plasma methylation profile of PCa. The methylation of AR binding sequences was related to more aggressive clinical course (125). Moreover, methyltransferase-like 3 (METTL3) increased the expression of C-MYC via up-regulating the N6-methyladenosine (m(6)A) level of mRNA for C-MYC, and resulted in the progression of PCa (126). MiRNAs were a category of small non-coding RNAs encoded in the genomes, that cleaved messages of protein-coding genes or repressed translation (127). Located in the upstream of gene expression, miRNAs mediated the differential expression of genes, and served as progression predictors as well. Carlo M. Croce et al. discovered that the expression of miR-106b-25 (regulating E2F1 and p21/WAF1), miR-32 (regulating Bim) increased along with the progression of PCa (128). In addition, miR-143 and miR-145 combined with mRNA for myosin VI (MYO6) and regulated its expression. The binding cite mutation located in the 3’-untranslated region (UTR) of the mRNA caused the loss of inhibition of miR-143 and miR-145. As a result, the expression level of MYO6 was up-regulated, which might play a role in the development of PCa (129). Proteins also played a role in the progression of PCa. Valerie A. Odero-Marah et al. used the method of proteomics-metabolomics and identified peroxidasin (PXDN) as a protector against metabolic and oxidative stress in PCa. They firstly found the increased expression of PXDN along with the progression of PCa by immunohistochemistry. The metabolomics analysis subsequently revealed increased oxidative stress, mitochondrial dysfunction, and gluconeogenesis pathways with PXDN knockdown, and metabolic reprogramming associated with decreased nucleotide biosynthesis and increased oxidative stress was induced. Further studies verified that PXDN knockdown led to a reactive oxygen species (ROS) increase associated with decreased cell viability and increased apoptosis. Overall, PXDN down-regulated cell apoptosis by inhibiting oxidative stress, leading to the progression of PCa (130). Specifically, CRPC represented an advanced stage of PCa, usually associated with clinical progression. The special molecular biological characteristics of CRPC may well explain its worse clinical outcomes. Through single cell analysis, a certain type of endothelial (activated endothelial cells, aECs) was enriched in CRPC, harboring active communications with malignant cells, and promoting the aggressiveness of CRPC (131). Mark A Rubin et al. discovered that 44% of CRPCs harbored the genomic alterations of AR, including the AR copy number increase and the AR point mutations. Other recurrent alterations included the loss of PTEN, retinoblastoma gene (RB), the mutation of tumor protein p53 gene (TP53), phosphatidylinositol-4,5-bisphosphate 3-kinase, catalytic subunit α gene (PIK3CA), etc. (104).
4.2.4.2 Relapse
Relapse was another important factor leading to poor clinical outcomes of PCa. Previous studies had identified several potential relapse predictors of PCa. Jonathan R Pollack et al. applied immunohistochemistry on tissue microarrays and identified MUC1 and AZP1 as significant predictors of the relapse of PCa, independent of tumor grade, stage, and preoperative PSA levels (132). In addition, Lars Dyrskjøt et al. found that SFRP4 was an independent predictor of the relapse after prostatectomy (133). In 2019, Paul C. Boutros et al. identified one tumor methylation quantitative trait locus related to the expression of AKT1, which could be used to predict the relapse after local therapy (134). Moreover, the increased expression of PLXNA1 independently predicted the biochemical relapse of PCa (135). Based on a multi-omics discovery platform, several serum prognostic biomarkers were identified. Two proteins-Tenascin C (TNC) and Apolipoprotein A1V (Apo-AIV), one metabolite-1-Methyladenosine (1-MA) and one phospholipid molecular species phosphatidic acid (PA) showed fairly high cumulative prediction effectiveness for the biochemical relapse of PCa, which had been confirmed in the validation set. The prediction sensitivity was ulteriorly improved when combined with pTstage and Gleason grade (136).
4.2.4.3 Metastasis
Metastasis was the most common adverse prognostic event in PCa, especially for bone metastasis. Large amounts of studies had been applied to find potential predictors of metastasis. In a 2007 study, hundreds of DEGs were detected in metastatic PCa, like Forkhead Box M1 (FoxM1), Osteopontin (SPP1), etc., which covered the androgen ablation related pathways as well as other metastatic pathways such as cell adhesion, bone remodeling, and so on (137). SChLAP1, a long non-coding RNA, was identified to be the highest expressed associated with the progression of metastatic PCa. Validation in three independent cohorts confirmed the unique value of SChLAP1 in the metastasis of PCa (138). As for proteins, low expression of neuropeptide Y (NPY) was considered as a risk factor that correlated with metastasis (139), and pro-NPY was identified as a specific prognosis-related biomarker, which correlated with the increase of mortality risk (140). M L Day et al. illustrated that E-cadherin was transiently down-regulated in localized PCa, but showed a fairly high expression level in metastatic PCa (141). Additionally, mitogen-activated protein kinase kinase kinase kinase 4 (MAP4K4) was up-regulated driven by MYC, and activated the process of metastasis (142). Interestingly, the increased expression of fucosyltranferase (FUT8) could reduce the number of extracellular vesicles (EVs) secreted by malignant cells in PCa and increase the protein abundance associated with metastasis (143).
The advancements in sequencing and omics studies had greatly accelerated the development of studies in PCa, especially for the exploration of the molecular pathogenesis of PCa and discovering novel biomarkers for clinical practice. However, in the past decade, a number of novel techniques had emerged and contributed to the studies of PCa. As mentioned above, with the evolution from bulk RNA-seq to scRNA-seq, the sequencing precision had stepped into a new era, providing researchers with a useful tool to study tumor heterogeneity and molecular mechanisms (144–146). The past decades had witnessed the prosperity of the application of scRNA-seq in PCa studies. Chang Zou et al. used the method of scRNA-seq, and identified three luminal clusters in the early stage of PCa (147). Daniel A Haber et al. applied scRNA-seq to 77 circulating tumor cells from 13 patients, and found that the activation of noncanonical Wnt signaling was involved in the antiandrogen resistance of PCa (118). In spite of this, due to the limitations of technology, scRNA-seq was not able to reveal the spatial locations of gene expressions. The occurrence of spatial RNA sequencing (spRNA-seq) compensated for this limitation and reserved the original appearance of tissues during the sequencing process (148). However, on account of the relatively lower sequencing resolutions, spRNA-seq was usually employed in combination with scRNA-seq. In 2020, Itai Yanai et al. integrated scRNA-seq and spRNA-seq together, and revealed the tissue architecture in pancreatic ductal adenocarcinoma (149). Up to now, there have been no relevant studies using the method of scRNA-seq and spRNA-seq in PCa. In fact, the integration of scRNA-seq and spRNA-seq did not really reach in situ sequencing at a single-cell resolution. The novel technique of spatial enhanced resolution omics-sequencing (stereo-seq) could in situ identify the gene expression profiles with a nanometer resolution and a centimeter panoramic view (150, 151). In the research field of tumors, stereo-seq could be a useful tool to study the natural development process of tumors, including local growth, nearby invasion, distant metastasis, and recurrence after treatment, and reveal the molecular mechanisms beneath them. Recently, Jia Fan et al. utilized the method of stereo-seq and successfully portrayed the spatiotemporal evolution of metastatic hepatocellular carcinoma (152). However, there were no relevant studies in PCa. More stereo-seq-based studies aiming at a comprehensive atlas of the progression and metastasis of PCa might be a promising direction in the future.
Although our study comprehensively summarized the research landscape of sequencing and omics studies in PCa, there still existed some limitations. First of all, as our retrieval timespan was set till July 3rd, 2023, publications after our retrieval were not included, due to the continuous update of the database. These excluded studies might cause possible bias to the bibliometric analysis. In addition, some latest research has not gained enough citations due to the limited publication time. However, bibliometric analysis could not recognize these situations and might classify these works into those less important studies. To overcome these limitations, we proceeded large amounts of purposeful literature reading in this field, and conducted our discussion mainly based on the literatures themselves, rather than simply on the bibliometric results.
5 Conclusion
In this article, through bibliometric analysis and large amounts of purposeful literature reading, we comprehensively understand the research landscape of sequencing and omics studies in PCa. The advancement of sequencing technology alongside its associated omics studies has led to a revelation of the molecular pathogenesis of PCa, especially for the rearrangements of ETS family. Beyond this, our analysis has also unearthed a suite of pivotal biomarkers that hold significant relevance for the susceptibility, diagnosis, therapy, and prognosis of PCa, and might provide new possibilities for the clinical practice of PCa. Our bibliometric study offered the knowledge constructures and research trends of sequencing and omics studies in PCa, for those who are interested in PCa studies and might inspire innovative explorations in this field.
Data availability statement
The original contributions presented in the study are included in the article/Supplementary Material. Further inquiries can be directed to the corresponding author.
Author contributions
BL: Conceptualization, Data curation, Formal analysis, Investigation, Methodology, Visualization, Writing – original draft, Writing – review & editing. YL: Conceptualization, Data curation, Formal analysis, Investigation, Methodology, Visualization, Writing – original draft, Writing – review & editing. YY: Conceptualization, Data curation, Formal analysis, Investigation, Methodology, Visualization, Writing – original draft, Writing – review & editing. TY: Conceptualization, Formal analysis, Methodology, Writing – review & editing. HZ: Conceptualization, Formal analysis, Methodology, Writing – review & editing. XY: Conceptualization, Formal analysis, Methodology, Writing – review & editing. RH: Conceptualization, Funding acquisition, Methodology, Writing – review & editing. WZ: Conceptualization, Methodology, Supervision, Writing – review & editing. XP: Conceptualization, Funding acquisition, Methodology, Supervision, Writing – review & editing. XC: Conceptualization, Funding acquisition, Methodology, Supervision, Writing – review & editing.
Funding
The author(s) declare financial support was received for the research, authorship, and/or publication of this article. This work was sponsored by the National Natural Science Foundation of China (No. 82330094, 82173265, 82072806); Leading health talents of Shanghai Municipal Health Commission (2022LJ002); Shanghai Rising-Star Program (23QC1401400); Shanghai Rising-Star Program (Sailing Special Program) (23YF1458400); the Natural Science Foundation of Shanghai (23ZR1441300); Hospital Funded Clinical Research, Xinhua Hospital Affiliated to Shanghai Jiao Tong University School of Medicine (21XHDB06). The funders had no role in study design, data collection and analysis, decision to publish, or preparation of the manuscript.
Acknowledgments
We thank Web of Science (WoS) for the use of their data.
Conflict of interest
The authors declare that the research was conducted in the absence of any commercial or financial relationships that could be construed as a potential conflict of interest.
The reviewer RC declared a shared affiliation with the author RH to the handling editor at the time of review.
Publisher’s note
All claims expressed in this article are solely those of the authors and do not necessarily represent those of their affiliated organizations, or those of the publisher, the editors and the reviewers. Any product that may be evaluated in this article, or claim that may be made by its manufacturer, is not guaranteed or endorsed by the publisher.
Supplementary material
The Supplementary Material for this article can be found online at: https://www.frontiersin.org/articles/10.3389/fonc.2024.1355551/full#supplementary-material
Glossary
References
1. Sung H, Ferlay J, Siegel RL, Laversanne M, Soerjomataram I, Jemal A, et al. Global cancer statistics 2020: GLOBOCAN estimates of incidence and mortality worldwide for 36 cancers in 185 countrie. CA: A Cancer J Clin. (2021) 71:209–49. doi: 10.3322/caac.21660
2. Schaeffer EM, Srinivas S, Adra N, An Y, Barocas D, Bitting R, et al. Prostate cancer, version 4.2023, NCCN clinical practice guidelines in oncology. J Natl Compr Canc Netw. (2023) 21:1067–96. doi: 10.6004/jnccn.2023.0050
3. Sanger F, Nicklen S, Coulson AR. DNA sequencing with chain-terminating inhibitors. Proc Natl Acad Sci USA. (1977) 74:5463–7. doi: 10.1073/pnas.74.12.5463
4. Finishing the euchromatic sequence of the human genome. Nature. (2004) 431:931–45. doi: 10.1038/nature03001
5. Mardis ER. Next-generation DNA sequencing methods. Annu Rev Genomics Hum Genet. (2008) 9:387–402. doi: 10.1146/annurev.genom.9.081307.164359
6. Shendure J, Ji H. Next-generation DNA sequencing. Nat Biotechnol. (2008) 26:1135–45. doi: 10.1038/nbt1486
7. Zhong H, Zhang Y, Wen Z, Li L. Protein sequencing by mass analysis of polypeptide ladders after controlled protein hydrolysis. Nat Biotechnol. (2004) 22:1291–6. doi: 10.1038/nbt1011
8. Stark R, Grzelak M, Hadfield J. RNA sequencing: the teenage years. Nat Rev Genet. (2019) 20:631–56. doi: 10.1038/s41576-019-0150-2
9. Hedlund E, Deng Q. Single-cell RNA sequencing: Technical advancements and biological applications. Mol Aspects Med. (2018) 59:36–46. doi: 10.1016/j.mam.2017.07.003
10. Braslavsky I, Hebert B, Kartalov E, Quake SR. Sequence information can be obtained from single DNA molecules. Proc Natl Acad Sci USA. (2003) 100:3960–4. doi: 10.1073/pnas.0230489100
11. Ninkov A, Frank JR, Maggio LA. Bibliometrics: Methods for studying academic publishing. Perspect Med Educ. (2022) 11:173–6. doi: 10.1007/S40037-021-00695-4
12. Huang R, Tang J, Wang S, Liu Y, Zhang M, Jin M, et al. Sequencing technology as a major impetus in the advancement of studies into rheumatism: A bibliometric study. Front Immunol. (2023) 14:1067830. doi: 10.3389/fimmu.2023.1067830
13. Wang S, Qian W, Chen S, Xian S, Jin M, Liu Y, et al. Bibliometric analysis of research on gene expression in spinal cord injury. Front Mol Neurosci. (2022) 15:1023692. doi: 10.3389/fnmol.2022.1023692
14. Putman MS, Ruderman EM, Niforatos JD. Publication Rate and Journal Review Time of COVID-19-Related Research. Mayo Clin Proc. (2020) 95:2290–1. doi: 10.1016/j.mayocp.2020.08.017
15. Aria M, Cuccurullo C. bibliometrix: An R-tool for comprehensive science mapping analysis. J Informetrics. (2017) 11:959–75. doi: 10.1016/j.joi.2017.08.007
16. van Eck NJ, Waltman L. Software survey: VOSviewer, a computer program for bibliometric mapping. Scientometrics. (2010) 84:523–38. doi: 10.1007/s11192-009-0146-3
17. Chen CM. CiteSpace II: Detecting and visualizing emerging trends and transient patterns in scientific literature. J Am Soc Inf Sci Technol. (2006) 57:359–77. doi: 10.1002/asi.20317
18. Hirsch JE. An index to quantify an individual's scientific research output. Proc Natl Acad Sci USA. (2005) 102:16569–72. doi: 10.1073/pnas.0507655102
19. Egghe L. Theory and practise of the g-index. Scientometrics. (2006) 69:131–52. doi: 10.1007/s11192-006-0144-7
20. Musbahi A, Rao CB, Immanuel A. A bibliometric analysis of robotic surgery from 2001 to 2021. World J Surg. (2022) 46:1314–24. doi: 10.1007/s00268-022-06492-2
21. Venable GT, Shepherd BA, Loftis CM, McClatchy SG, Roberts ML, Fillinger ME, et al. Bradford's law: identification of the core journals for neurosurgery and its subspecialties. J Neurosurg. (2016) 124:569–79. doi: 10.3171/2015.3.JNS15149
22. Coile RC. Lotkas frequency-distribution of scientific productivity. J Am Soc Inf Sci. (1977) 28:366–70. doi: 10.1002/asi.4630280610
23. Small H. Cocitation in scientific literature - new measure of relationship between 2 documents. J Am Soc Inf Sci. (1973) 24:265–9. doi: 10.1002/asi.4630240406
24. Cambrosio A, Limoges C, Courtial JP, Laville F. Historical scientometrics - mapping over 70 years of biological safety research with co-word analysis. Scientometrics. (1993) 27:119–43. doi: 10.1007/BF02016546
25. Qian C, Li D, Chen Y. ETS factors in prostate cancer. Cancer Lett. (2022) 530:181–9. doi: 10.1016/j.canlet.2022.01.009
26. Tomlins SA, Rhodes DR, Perner S, Dhanasekaran SM, Mehra R, Sun XW, et al. Recurrent fusion of TMPRSS2 and ETS transcription factor genes in prostate cancer. Science. (2005) 310:644–8. doi: 10.1126/science.1117679
27. Clark JP, Cooper CS. ETS gene fusions in prostate cancer. Nat Rev Urol. (2009) 6:429–39. doi: 10.1038/nrurol.2009.127
28. Kumar-Sinha C, Tomlins SA, Chinnaiyan AM. Recurrent gene fusions in prostate cancer. Nat Rev Cancer. (2008) 8:497–511. doi: 10.1038/nrc2402
29. Han B, Mehra R, Dhanasekaran SM, Yu J, Menon A, Lonigro RJ, et al. A fluorescence in situ hybridization screen for E26 transformation-specific aberrations: identification of DDX5-ETV4 fusion protein in prostate cancer. Cancer Res. (2008) 68:7629–37. doi: 10.1158/0008-5472.CAN-08-2014
30. Helgeson BE, Tomlins SA, Shah N, Laxman B, Cao Q, Prensner JR, et al. Characterization of TMPRSS2:ETV5 and SLC45A3:ETV5 gene fusions in prostate cancer. Cancer Res. (2008) 68:73–80. doi: 10.1158/0008-5472.CAN-07-5352
31. Hermans KG, Bressers AA, van der Korput HA, Dits NF, Jenster G, Trapman J. Two unique novel prostate-specific and androgen-regulated fusion partners of ETV4 in prostate cancer. Cancer Res. (2008) 68:3094–8. doi: 10.1158/0008-5472.CAN-08-0198
32. Tomlins SA, Mehra R, Rhodes DR, Smith LR, Roulston D, Helgeson BE, et al. TMPRSS2:ETV4 gene fusions define a third molecular subtype of prostate cancer. Cancer Res. (2006) 66:3396–400. doi: 10.1158/0008-5472.CAN-06-0168
33. Paulo P, Barros-Silva JD, Ribeiro FR, Ramalho-Carvalho J, Jerónimo C, Henrique R, et al. FLI1 is a novel ETS transcription factor involved in gene fusions in prostate cancer. Genes Chromosomes Cancer. (2012) 51:240–9. doi: 10.1002/gcc.20948
34. Paulo P, Ribeiro FR, Santos J, Mesquita D, Almeida M, Barros-Silva JD, et al. Molecular subtyping of primary prostate cancer reveals specific and shared target genes of different ETS rearrangements. Neoplasia. (2012) 14:600–11. doi: 10.1593/neo.12600
35. Iljin K, Wolf M, Edgren H, Gupta S, Kilpinen S, Skotheim RI, et al. TMPRSS2 fusions with oncogenic ETS factors in prostate cancer involve unbalanced genomic rearrangements and are associated with HDAC1 and epigenetic reprogramming. Cancer Res. (2006) 66:10242–6. doi: 10.1158/0008-5472.CAN-06-1986
36. Zou Z, Zeng F, Xu W, Wang C, Ke Z, Wang QJ, et al. PKD2 and PKD3 promote prostate cancer cell invasion by modulating NF-κB- and HDAC1-mediated expression and activation of uPA. J Cell Sci. (2012) 125:4800–11. doi: 10.1242/jcs.106542
37. De U, Kundu S, Patra N, Ahn MY, Ahn JH, Son JY, et al. A new histone deacetylase inhibitor, MHY219, inhibits the migration of human prostate cancer cells via HDAC1. Biomol Ther (Seoul). (2015) 23:434–41. doi: 10.4062/biomolther.2015.026
38. Kim NH, Kim SN, Kim YK. Involvement of HDAC1 in E-cadherin expression in prostate cancer cells; its implication for cell motility and invasion. Biochem Biophys Res Commun. (2011) 404:915–21. doi: 10.1016/j.bbrc.2010.12.081
39. Adamo P, Ladomery MR. The oncogene ERG: a key factor in prostate cancer. Oncogene. (2016) 35:403–14. doi: 10.1038/onc.2015.109
40. Kunderfranco P, Mello-Grand M, Cangemi R, Pellini S, Mensah A, Albertini V, et al. ETS transcription factors control transcription of EZH2 and epigenetic silencing of the tumor suppressor gene Nkx3.1 in Prostate Cancer. PloS One. (2010) 5. doi: 10.1371/journal.pone.0010547
41. Boldrini L, Bartoletti R, Giordano M, Manassero F, Selli C, Panichi M, et al. C-MYC, HIF-1α, ERG, TKT, and GSTP1: an axis in prostate cancer? Pathol Oncol Res. (2019) 25:1423–9. doi: 10.1007/s12253-018-0479-4
42. Zoma M, Curti L, Shinde D, Albino D, Mitra A, Sgrignani J, et al. EZH2-induced lysine K362 methylation enhances TMPRSS2-ERG oncogenic activity in prostate cancer. Nat Commun. (2021) 12:4147. doi: 10.1038/s41467-021-24380-6
43. Margueron R, Reinberg D. The Polycomb complex PRC2 and its mark in life. Nature. (2011) 469:343–9. doi: 10.1038/nature09784
44. Dongre A, Weinberg RA. New insights into the mechanisms of epithelial-mesenchymal transition and implications for cancer. Nat Rev Mol Cell Biol. (2019) 20:69–84. doi: 10.1038/s41580-018-0080-4
45. Wu L, Zhao JC, Kim J, Jin HJ, Wang CY, Yu J. ERG is a critical regulator of Wnt/LEF1 signaling in prostate cancer. Cancer Res. (2013) 73:6068–79. doi: 10.1158/0008-5472.CAN-13-0882
46. Soleymani L, Zarrabi A, Hashemi F, Hashemi F, Zabolian A, Banihashemi SM, et al. Role of ZEB family members in proliferation, metastasis, and chemoresistance of prostate cancer cells: revealing signaling networks. Curr Cancer Drug Targets. (2021) 21:749–67. doi: 10.2174/1568009621666210601114631
47. Wang J, Farkas C, Benyoucef A, Carmichael C, Haigh K, Wong N, et al. Interplay between the EMT transcription factors ZEB1 and ZEB2 regulates hematopoietic stem and progenitor cell differentiation and hematopoietic lineage fidelity. PloS Biol. (2021) 19:e3001394. doi: 10.1371/journal.pbio.3001394
48. Cai J, Kandagatla P, Singareddy R, Kropinski A, Sheng S, Cher ML, et al. Androgens induce functional CXCR4 through ERG factor expression in TMPRSS2-ERG fusion-positive prostate cancer cells. Transl Oncol. (2010) 3:195–203. doi: 10.1593/tlo.09328
49. Clézardin P, Coleman R, Puppo M, Ottewell P, Bonnelye E, Paycha F, et al. Bone metastasis: mechanisms, therapies, and biomarkers. Physiol Rev. (2021) 101:797–855. doi: 10.1152/physrev.00012.2019
50. Sun YX, Fang M, Wang J, Cooper CR, Pienta KJ, Taichman RS. Expression and activation of alpha v beta 3 integrins by SDF-1/CXC12 increases the aggressiveness of prostate cancer cells. Prostate. (2007) 67:61–73. doi: 10.1002/pros.20500
51. Singareddy R, Semaan L, Conley-Lacomb MK, St John J, Powell K, Iyer M, et al. Transcriptional regulation of CXCR4 in prostate cancer: significance of TMPRSS2-ERG fusions. Mol Cancer Res. (2013) 11:1349–61. doi: 10.1158/1541-7786.MCR-12-0705
52. Kim J, Wu L, Zhao JC, Jin HJ, Yu J. TMPRSS2-ERG gene fusions induce prostate tumorigenesis by modulating microRNA miR-200c. Oncogene. (2014) 33:5183–92. doi: 10.1038/onc.2013.461
53. Gordanpour A, Stanimirovic A, Nam RK, Moreno CS, Sherman C, Sugar L, et al. miR-221 Is down-regulated in TMPRSS2:ERG fusion-positive prostate cancer. Anticancer Res. (2011) 31:403–10.
54. Bauer S, Ratz L, Heckmann-Nötzel D, Kaczorowski A, Hohenfellner M, Kristiansen G, et al. miR-449a repression leads to enhanced NOTCH Signaling in TMPRSS2:ERG fusion positive prostate cancer cells. Cancers (Basel). (2021) 13. doi: 10.3390/cancers13050964
55. Bilke S, Schwentner R, Yang F, Kauer M, Jug G, Walker RL, et al. Oncogenic ETS fusions deregulate E2F3 target genes in Ewing sarcoma and prostate cancer. Genome Res. (2013) 23:1797–809. doi: 10.1101/gr.151340.112
56. Zhou F, Gao S, Han D, Han W, Chen S, Patalano S, et al. TMPRSS2-ERG activates NO-cGMP signaling in prostate cancer cells. Oncogene. (2019) 38:4397–411. doi: 10.1038/s41388-019-0730-9
57. de Launoit Y, Baert JL, Chotteau-Lelievre A, Monte D, Coutte L, Mauen S, et al. The Ets transcription factors of the PEA3 group: transcriptional regulators in metastasis. Biochim Biophys Acta. (2006) 1766:79–87. doi: 10.1016/j.bbcan.2006.02.002
58. Selvaraj N, Budka JA, Ferris MW, Plotnik JP, Hollenhorst PC. Extracellular signal-regulated kinase signaling regulates the opposing roles of JUN family transcription factors at ETS/AP-1 sites and in cell migration. Mol Cell Biol. (2015) 35:88–100. doi: 10.1128/MCB.00982-14
59. Hollenhorst PC, Ferris MW, Hull MA, Chae H, Kim S, Graves BJ. Oncogenic ETS proteins mimic activated RAS/MAPK signaling in prostate cells. Genes Dev. (2011) 25:2147–57. doi: 10.1101/gad.17546311
60. Yu J, Yu J, Mani RS, Cao Q, Brenner CJ, Cao X, et al. An integrated network of androgen receptor, polycomb, and TMPRSS2-ERG gene fusions in prostate cancer progression. Cancer Cell. (2010) 17:443–54. doi: 10.1016/j.ccr.2010.03.018
61. Chen Y, Chi P, Rockowitz S, Iaquinta PJ, Shamu T, Shukla S, et al. ETS factors reprogram the androgen receptor cistrome and prime prostate tumorigenesis in response to PTEN loss. Nat Med. (2013) 19:1023–9. doi: 10.1038/nm.3216
62. Baena E, Shao Z, Linn DE, Glass K, Hamblen MJ, Fujiwara Y, et al. ETV1 directs androgen metabolism and confers aggressive prostate cancer in targeted mice and patients. Genes Dev. (2013) 27:683–98. doi: 10.1101/gad.211011.112
63. Cai C, Hsieh CL, Omwancha J, Zheng Z, Chen SY, Baert JL, et al. ETV1 is a novel androgen receptor-regulated gene that mediates prostate cancer cell invasion. Mol Endocrinol. (2007) 21:1835–46. doi: 10.1210/me.2006-0480
64. Kedage V, Selvaraj N, Nicholas TR, Budka JA, Plotnik JP, Jerde TJ, et al. An interaction with Ewing's sarcoma breakpoint protein EWS defines a specific oncogenic mechanism of ETS factors rearranged in prostate cancer. Cell Rep. (2016) 17:1289–301. doi: 10.1016/j.celrep.2016.10.001
65. Nicholas TR, Strittmatter BG, Hollenhorst PC. Oncogenic ETS factors in prostate cancer. Adv Exp Med Biol 2019. (1210) p:409–36.
66. Rickman DS, Strittmatter BG, Hollenhorst PC. Oncogene-mediated alterations in chromatin conformation. Proc Natl Acad Sci U.S.A. (2012) 109:9083–8. doi: 10.1073/pnas.1112570109
67. Chng KR, Chang CW, Tan SK, Yang C, Hong SZ, Sng NY, et al. A transcriptional repressor co-regulatory network governing androgen response in prostate cancers. EMBO J. (2012) 31:2810–23. doi: 10.1038/emboj.2012.112
68. Roe JS, Mercan F, Rivera K, Pappin DJ, Vakoc CR. BET bromodomain inhibition suppresses the function of hematopoietic transcription factors in acute myeloid leukemia. Mol Cell. (2015) 58:1028–39. doi: 10.1016/j.molcel.2015.04.011
69. Kim TD, Shin S, Janknecht R. ETS transcription factor ERG cooperates with histone demethylase KDM4A. Oncol Rep. (2016) 35:3679–88. doi: 10.3892/or.2016.4747
70. Mounir Z, Korn JM, Westerling T, Lin F, Kirby CA, Schirle M, et al. ERG signaling in prostate cancer is driven through PRMT5-dependent methylation of the Androgen Receptor. Elife. (2016) 5. doi: 10.7554/eLife.13964
71. Gudmundsson J, Sulem P, Rafnar T, Bergthorsson JT, Manolescu A, Gudbjartsson D, et al. Common sequence variants on 2p15 and Xp11.22 confer susceptibility to prostate cancer. Nat Genet. (2008) 40:281–3. doi: 10.1038/ng.89
72. Zheng SL, Augustsson-Bälter K, Chang B, Hedelin M, Li L, Adami HO, et al. Sequence variants of toll-like receptor 4 are associated with prostate cancer risk: results from the CAncer Prostate in Sweden Study. Cancer Res. (2004) 64:2918–22. doi: 10.1158/0008-5472.CAN-03-3280
73. Conti DV, Darst BF, Moss LC, Saunders EJ, Sheng X, Chou A, et al. Trans-ancestry genome-wide association meta-analysis of prostate cancer identifies new susceptibility loci and informs genetic risk prediction. Nat Genet. (2021) 53:11–5. doi: 10.1038/s41588-020-00748-0
74. Litwin MS, Tan HJ. The diagnosis and treatment of prostate cancer: a review. Jama. (2017) 317:2532–42. doi: 10.1001/jama.2017.7248
75. Mao X, Shaw G, James SY, Purkis P, Kudahetti SC, Tsigani T, et al. Detection of TMPRSS2:ERG fusion gene in circulating prostate cancer cells. Asian J Androl. (2008) 10:467–73. doi: 10.1111/j.1745-7262.2008.00401.x
76. Laxman B, Tomlins SA, Mehra R, Morris DS, Wang L, Helgeson BE, et al. Noninvasive detection of TMPRSS2:ERG fusion transcripts in the urine of men with prostate cancer. Neoplasia. (2006) 8:885–8. doi: 10.1593/neo.06625
77. Hessels D, Smit FP, Verhaegh GW, Witjes JA, Cornel EB, Schalken JA. Detection of TMPRSS2-ERG fusion transcripts and prostate cancer antigen 3 in urinary sediments may improve diagnosis of prostate cancer. Clin Cancer Res. (2007) 13:5103–8. doi: 10.1158/1078-0432.CCR-07-0700
78. Xu N, Wu Y-P, Ke Z-B, Liang Y-C, Cai H, Su W-T, et al. Identification of key DNA methylation-driven genes in prostate adenocarcinoma: an integrative analysis of TCGA methylation data. J Trans Med. (2019) 17(1). doi: 10.1186/s12967-019-2065-2
79. Kim JH, Dhanasekaran SM, Prensner JR, Cao X, Robinson D, Kalyana-Sundaram S, et al. Deep sequencing reveals distinct patterns of DNA methylation in prostate cancer. Genome Res. (2011) 21:1028–41. doi: 10.1101/gr.119347.110
80. Kannan K, Wang L, Wang J, Ittmann MM, Li W, Yen L. Recurrent chimeric RNAs enriched in human prostate cancer identified by deep sequencing. Proc Natl Acad Sci U.S.A. (2011) 108:9172–7. doi: 10.1073/pnas.1100489108
81. Davalieva K, Kostovska IM, Kiprijanovska S, Markoska K, Kubelka-Sabit K, Filipovski V, et al. Proteomics analysis of malignant and benign prostate tissue by 2D DIGE/MS reveals new insights into proteins involved in prostate cancer. Prostate. (2015) 75:1586–600. doi: 10.1002/pros.v75.14
82. Liu AY, Zhang H, Sorensen CM, Diamond DL. Analysis of prostate cancer by proteomics using tissue specimens. J Urol. (2005) 173:73–8. doi: 10.1097/01.ju.0000146543.33543.a3
83. Zheng Y, Xu Y, Ye B, Lei J, Weinstein MH, O'Leary MP, et al. Prostate carcinoma tissue proteomics for biomarker discovery. Cancer. (2003) 98:2576–82. doi: 10.1002/cncr.11849
84. Kwon OK, Ha Y-S, Na A-Y, Chun SY, Kwon TG, Lee JN, et al. Identification of novel prognosis and prediction markers in advanced prostate cancer tissues based on quantitative proteomics. Cancer Genomics Proteomics. (2020) 17:195–208. doi: 10.21873/cgp.20180
85. Miller JC, Zhou H, Kwekel J, Cavallo R, Burke J, Butler EB, et al. Antibody microarray profiling of human prostate cancer sera: antibody screening and identification of potential biomarkers. Proteomics. (2003) 3:56–63. doi: 10.1002/pmic.200390009
86. Skvortsov S, Schäfer G, Stasyk T, Fuchsberger C, Bonn GK, Bartsch G, et al. Proteomics profiling of microdissected low- and high-grade prostate tumors identifies Lamin A as a discriminatory biomarker. J Proteome Res. (2011) 10:259–68. doi: 10.1021/pr100921j
87. Kawahara R, Recuero S, Nogueira FCS, Domont GB, Leite KRM, Srougi M, et al. Tissue proteome signatures associated with five grades of prostate cancer and benign prostatic hyperplasia. Proteomics. (2019) 19. doi: 10.1002/pmic.201900174
88. Heger Z, Michalek P, Guran R, Cernei N, Duskova K, Vesely S, et al. Differences in urinary proteins related to surgical margin status after radical prostatectomy. Oncol Rep. (2015) 34:3247–55. doi: 10.3892/or.2015.4322
89. Pérez-Rambla C, Puchades-Carrasco L, García-Flores M, Rubio-Briones J, López-Guerrero JA, Pineda-Lucena A. Non-invasive urinary metabolomic profiling discriminates prostate cancer from benign prostatic hyperplasia. Metabolomics. (2017) 13:52. doi: 10.1007/s11306-017-1194-y
90. Fujita K, Kume H, Matsuzaki K, Kawashima A, Ujike T, Nagahara A, et al. Proteomic analysis of urinary extracellular vesicles from high Gleason score prostate cancer. Sci Rep. (2017) 7:42961. doi: 10.1038/srep42961
91. Gkotsos G, Virgiliou C, Lagoudaki I, Sardeli C, Raikos N, Theodoridis G, et al. The role of sarcosine, uracil, and kynurenic acid metabolism in urine for diagnosis and progression monitoring of prostate cancer. Metabolites. (2017) 7. doi: 10.3390/metabo7010009
92. Puhka M, Takatalo M, Nordberg ME, Valkonen S, Nandania J, Aatonen M, et al. Metabolomic profiling of extracellular vesicles and alternative normalization methods reveal enriched metabolites and strategies to study prostate cancer-related changes. Theranostics. (2017) 7:3824–41. doi: 10.7150/thno.19890
93. Fernández-Peralbo MA, Gómez-Gómez E, Calderón-Santiago M, Carrasco-Valiente J, Ruiz-García J, Requena-Tapia MJ, et al. Prostate cancer patients-negative biopsy controls discrimination by untargeted metabolomics analysis of urine by LC-QTOF: upstream information on other omics. Sci Rep. (2016) 6:38243. doi: 10.1038/srep38243
94. Liang Q, Liu H, Xie L-X, Li X, Zhang A-H. High-throughput metabolomics enables biomarker discovery in prostate cancer. Rsc Adv. (2017) 7:2587–93. doi: 10.1039/C6RA25007F
95. Kumar D, Gupta A, Mandhani A, Sankhwar SN. Metabolomics-derived prostate cancer biomarkers: fact or fiction? J Proteome Res. (2015) 14:1455–64. doi: 10.1021/pr5011108
96. Kumar D, Gupta A, Mandhani A, Sankhwar SN. NMR spectroscopy of filtered serum of prostate cancer: A new frontier in metabolomics. Prostate. (2016) 76:1106–19. doi: 10.1002/pros.v76.12
97. Giskeødegård GF, Hansen AF, Bertilsson H, Gonzalez SV, Kristiansen KA, Bruheim P, et al. Metabolic markers in blood can separate prostate cancer from benign prostatic hyperplasia. Br J Cancer. (2015) 113:1712–9. doi: 10.1038/bjc.2015.411
98. Etheridge T, Straus J, Ritter MA, Jarrard DF, Huang W. Semen AMACR protein as a novel method for detecting prostate cancer. Urol Oncol. (2018) 36:532.e1–7. doi: 10.1016/j.urolonc.2018.09.010
99. Kline EE, Treat EG, Averna TA, Davis MS, Smith AY, Sillerud LO. Citrate concentrations in human seminal fluid and expressed prostatic fluid determined via 1H nuclear magnetic resonance spectroscopy outperform prostate specific antigen in prostate cancer detection. J Urol. (2006) 176:2274–9. doi: 10.1016/j.juro.2006.07.054
100. Averna TA, Kline EE, Smith AY, Sillerud LO. A decrease in 1H nuclear magnetic resonance spectroscopically determined citrate in human seminal fluid accompanies the development of prostate adenocarcinoma. J Urol. (2005) 173:433–8. doi: 10.1097/01.ju.0000148949.72314.d7
101. Zheng H, Dong B, Ning J, Shao X, Zhao L, Jiang Q, et al. NMR-based metabolomics analysis identifies discriminatory metabolic disturbances in tissue and biofluid samples for progressive prostate cancer. Clinica Chimica Acta. (2020) 501:241–51. doi: 10.1016/j.cca.2019.10.046
102. Lima AR, Pinto J, Amaro F, Bastos MDL, Carvalho M, de Pinho PG, et al. Advances and perspectives in prostate cancer biomarker discovery in the last 5 years through tissue and urine metabolomics. Metabolites. (2021) 11. doi: 10.3390/metabo11030181
103. Murphy K, Murphy BT, Boyce S, Flynn L, Gilgunn S, O'Rourke CJ, et al. Integrating biomarkers across omic platforms: an approach to improve stratification of patients with indolent and aggressive prostate cancer. Mol Oncol. (2018) 12:1513–25. doi: 10.1002/1878-0261.12348
104. Beltran H, Yelensky R, Frampton GM, Park K, Downing SR, MacDonald TY, et al. Targeted next-generation sequencing of advanced prostate cancer identifies potential therapeutic targets and disease heterogeneity. Eur Urol. (2013) 63:920–6. doi: 10.1016/j.eururo.2012.08.053
105. Bubendorf L, Kononen J, Koivisto P, Schraml P, Moch H, Gasser TC, et al. Survey of gene amplifications during prostate cancer progression by high throughput fluorescence in situ hybridization on tissue microarrays. Cancer Res. (1999) 59:803–6.
106. Tamura K, Furihata M, Tsunoda T, Ashida S, Takata R, Mara W, et al. Molecular features of hormone-refractory prostate cancer cells by genome-wide gene expression profiles. Cancer Res. (2007) 67:5117–25. doi: 10.1158/0008-5472.CAN-06-4040
107. Viswanathan SR, Ha G, Hoff AM, Wala JA, Carrot-Zhang J, Whelan CW, et al. Structural alterations driving castration-resistant prostate cancer revealed by linked-read genome sequencing. Cell. (2018) 174:433–+. doi: 10.1016/j.cell.2018.05.036
108. Spratt DE, Alshalalfa M, Fishbane N, Weiner AB, Mehra R, Mahal BA, et al. Transcriptomic heterogeneity of androgen receptor activity defines a de novo low AR-active subclass in treatment naive primary prostate cancer. Clin Cancer Res. (2019) 25:6721–30. doi: 10.1158/1078-0432.CCR-19-1587
109. de Wit R, de Bono J, Sternberg CN, Fizazi K, Tombal B, Wülfing C, et al. Cabazitaxel versus abiraterone or enzalutamide in metastatic prostate cancer. N Engl J Med. (2019) 381:2506–18. doi: 10.1056/NEJMoa1911206
110. Marshall CH, Fu W, Wang H, Baras AS, Lotan TL, Antonarakis ES. Prevalence of DNA repair gene mutations in localized prostate cancer according to clinical and pathologic features: association of Gleason score and tumor stage. Prostate Cancer Prostatic Dis. (2019) 22:59–65. doi: 10.1038/s41391-018-0086-1
111. D'Andrea AD. Mechanisms of PARP inhibitor sensitivity and resistance. DNA Repair (Amst). (2018) 71:172–6. doi: 10.1016/j.dnarep.2018.08.021
112. de Bono J, Mateo J, Fizazi K, Saad F, Shore N, Sandhu S, et al. Olaparib for metastatic castration-resistant prostate cancer. N Engl J Med. (2020) 382:2091–102. doi: 10.1056/NEJMoa1911440
113. Fizazi K, Piulats JM, Reaume MN, Ostler P, McDermott R, Gingerich JR, et al. Rucaparib or physician's choice in metastatic prostate cancer. N Engl J Med. (2023) 388:719–32. doi: 10.1056/NEJMoa2214676
114. Kinoshita Y, Kuratsukuri K, Landas S, Imaida K, Rovito PM Jr, Wang CY, et al. Expression of prostate-specific membrane antigen in normal and malignant human tissues. World J Surg. (2006) 30:628–36. doi: 10.1007/s00268-005-0544-5
115. He Y, Xu W, Xiao YT, Huang H, Gu D, Ren S. Targeting signaling pathways in prostate cancer: mechanisms and clinical trials. Signal Transduct Target Ther. (2022) 7:198. doi: 10.1038/s41392-022-01042-7
116. Zhao SG, Chen WS, Li H, Foye A, Zhang M, Sjostrom M, et al. The DNA methylation landscape of advanced prostate cancer. Nat Genet. (2020) 52:778–+. doi: 10.1038/s41588-020-0648-8
117. Itkonen HM, Poulose N, Walker S, Mills IG. CDK9 inhibition induces a metabolic switch that renders prostate cancer cells dependent on fatty acid oxidation. Neoplasia. (2019) 21:713–20. doi: 10.1016/j.neo.2019.05.001
118. Miyamoto DT, Zheng Y, Wittner BS, Lee RJ, Zhu H, Broderick KT, et al. RNA-Seq of single prostate CTCs implicates noncanonical Wnt signaling in antiandrogen resistance. Science. (2015) 349:1351–6. doi: 10.1126/science.aab0917
119. Sobhani N, Neeli PK, D'Angelo A, Pittacolo M, Sirico M, Galli IC, et al. AR-V7 in metastatic prostate cancer: a strategy beyond redemption. Int J Mol Sci. (2021) 22. doi: 10.3390/ijms22115515
120. Lam H-M, Nguyen HM, Labrecque MP, Brown LG, Coleman IM, Gulati R, et al. Durable response of enzalutamide-resistant prostate cancer to supraphysiological testosterone is associated with a multifaceted growth suppression and impaired dna damage response transcriptomic program in patient-derived xenografts. Eur Urol. (2020) 77:144–55. doi: 10.1016/j.eururo.2019.05.042
121. Kerns SL, Fachal L, Dorling L, Barnett GC, Baran A, Peterson DR, et al. Radiogenomics consortium genome-wide association study meta-analysis of late toxicity after prostate cancer radiotherapy. Jnci-Journal Natl Cancer Institute. (2020) 112:179–90. doi: 10.1093/jnci/djz07
122. Ashida S, Nakagawa H, Katagiri T, Furihata M, Iiizumi M, Anazawa Y, et al. Molecular features of the transition from prostatic intraepithelial neoplasia (PIN) to prostate cancer: genome-wide gene-expression profiles of prostate cancers and PINs. Cancer Res. (2004) 64:5963–72. doi: 10.1158/0008-5472.CAN-04-0020
123. Alkhateeb A, Rezaeian I, Singireddy S, Cavallo-Medved D, Porter LA, Rueda L. Transcriptomics signature from next-generation sequencing data reveals new transcriptomic biomarkers related to prostate cancer. Cancer Inf. (2019) 18. doi: 10.1177/1176935119835522
124. Attard G, Clark J, Ambroisine L, Fisher G, Kovacs G, Flohr P, et al. Duplication of the fusion of TMPRSS2 to ERG sequences identifies fatal human prostate cancer. Oncogene. (2008) 27:253–63. doi: 10.1038/sj.onc.1210640
125. Wu A, Cremaschi P, Wetterskog D, Conteduca V, Franceschini GM, Kleftogiannis D, et al. Genome-wide plasma DNA methylation features of metastatic prostate cancer. J Clin Invest. (2020) 130:1991–2000. doi: 10.1172/JCI130887
126. Yuan Y, Du Y, Wang L, Liu X. The M6A methyltransferase METTL3 promotes the development and progression of prostate carcinoma via mediating MYC methylation. J Cancer. (2020) 11:3588–95. doi: 10.7150/jca.42338
127. Ho PTB, Clark IM, Le LTT. MicroRNA-based diagnosis and therapy. Int J Mol Sci. (2022) 23. doi: 10.3390/ijms23137167
128. Ambs S, Prueitt RL, Yi M, Hudson RS, Howe TM, Petrocca F, et al. Genomic profiling of microRNA and messenger RNA reveals deregulated microRNA expression in prostate cancer. Cancer Res. (2008) 68:6162–70. doi: 10.1158/0008-5472.CAN-08-0144
129. Szczyrba J, Löprich E, Wach S, Jung V, Unteregger G, Barth S, et al. The microRNA profile of prostate carcinoma obtained by deep sequencing. Mol Cancer Res. (2010) 8:529–38. doi: 10.1158/1541-7786.MCR-09-0443
130. Dougan J, Hawsawi O, Burton LJ, Edwards G, Jones K, Zou J, et al. Proteomics-metabolomics combined approach identifies peroxidasin as a protector against metabolic and oxidative stress in prostate cancer. Int J Mol Sci. (2019) 20. doi: 10.3390/ijms20123046
131. Chen S, Zhu G, Yang Y, Wang F, Xiao Y-T, Zhang N, et al. Single-cell analysis reveals transcriptomic remodellings in distinct cell types that contribute to human prostate cancer progression. Nat Cell Biol. (2021) 23. doi: 10.1038/s41556-020-00613-6
132. Lapointe J, Li C, Higgins JP, van de Rijn M, Bair E, Montgomery K, et al. Gene expression profiling identifies clinically relevant subtypes of prostate cancer. Proc Natl Acad Sci U.S.A. (2004) 101:811–6. doi: 10.1073/pnas.0304146101
133. Mortensen MM, Høyer S, Lynnerup AS, Ørntoft TF, Sørensen KD, Borre M, et al. Expression profiling of prostate cancer tissue delineates genes associated with recurrence after prostatectomy. Sci Rep. (2015) 5:16018. doi: 10.1038/srep16018
134. Houlahan KE, Shiah Y-J, Gusev A, Yuan J, Ahmed M, Shetty A, et al. Genome-wide germline correlates of the epigenetic landscape of prostate cancer. Nat Med. (2019) 25:1615–+. doi: 10.1038/s41591-019-0579-z
135. Ren S, Wei GH, Liu D, Wang L, Hou Y, Zhu S, et al. Whole-genome and transcriptome sequencing of prostate cancer identify new genetic alterations driving disease progression. Eur Urol. (2018) 73:322–39. doi: 10.1016/j.eururo.2017.08.027
136. Kiebish MA, Cullen J, Mishra P, Ali A, Milliman E, Rodrigues LO, et al. Multi-omic serum biomarkers for prognosis of disease progression in prostate cancer. J Transl Med. (2020) 18:10. doi: 10.1186/s12967-019-02185-y
137. Chandran UR, Ma C, Dhir R, Bisceglia M, Lyons-Weiler M, Liang W, et al. Gene expression profiles of prostate cancer reveal involvement of multiple molecular pathways in the metastatic process. BMC Cancer. (2007) 7:64. doi: 10.1186/1471-2407-7-64
138. Prensner JR, Zhao S, Erho N, Schipper M, Iyer MK, Dhanasekaran SM, et al. RNA biomarkers associated with metastatic progression in prostate cancer: a multi-institutional high-throughput analysis of SChLAP1. Lancet Oncol. (2014) 15:1469–80. doi: 10.1016/S1470-2045(14)71113-1
139. Alshalalfa M, Nguyen PL, Beltran H, Chen WS, Davicioni E, Zhao SG, et al. Transcriptomic and clinical characterization of neuropeptide y expression in localized and metastatic prostate cancer: identification of novel prostate cancer subtype with clinical implications. Eur Urol Oncol. (2019) 2:405–12. doi: 10.1016/j.euo.2019.05.001
140. Iglesias-Gato D, Wikström P, Tyanova S, Lavallee C, Thysell E, Carlsson J, et al. The proteome of primary prostate cancer. Eur Urol. (2016) 69:942–52. doi: 10.1016/j.eururo.2015.10.053
141. Rubin MA, Mucci NR, Figurski J, Fecko A, Pienta KJ, Day ML. E-cadherin expression in prostate cancer: a broad survey using high-density tissue microarray technology. Hum Pathol. (2001) 32:690–7. doi: 10.1053/hupa.2001.25902
142. Miao W, Yuan J, Li L, Wang Y. Parallel-reaction-monitoring-based proteome-wide profiling of differential kinase protein expression during prostate cancer metastasis in vitro. Analytical Chem. (2019) 91:9893–900. doi: 10.1021/acs.analchem.9b01561
143. Clark DJ, Schnaubelt M, Hoti N, Hu Y, Zhou Y, Gooya M, et al. Impact of increased FUT8 expression on the extracellular vesicle proteome in prostate cancer cells. J Proteome Res. (2020) 19:2195–205. doi: 10.1021/acs.jproteome.9b00578
144. Butler A, Hoffman P, Smibert P, Papalexi E, Satija R. Integrating single-cell transcriptomic data across different conditions, technologies, and species. Nat Biotechnol. (2018) 36:411–20. doi: 10.1038/nbt.4096
145. Lei Y, Tang R, Xu J, Wang W, Zhang B, Liu J, et al. Applications of single-cell sequencing in cancer research: progress and perspectives. J Hematol Oncol. (2021) 14:91. doi: 10.1186/s13045-021-01105-2
146. Zhang Y, Wang D, Peng M, Tang L, Ouyang J, Xiong F, et al. Single-cell RNA sequencing in cancer research. J Exp Clin Cancer Res. (2021) 40:81. doi: 10.1186/s13046-021-01874-1
147. Ma X, Guo J, Liu K, Chen L, Liu D, Dong S, et al. Identification of a distinct luminal subgroup diagnosing and stratifying early stage prostate cancer by tissue-based single-cell RNA sequencing. Mol Cancer. (2020) 19:147. doi: 10.1186/s12943-020-01264-9
148. Li X, Wang CY. From bulk, single-cell to spatial RNA sequencing. Int J Oral Sci. (2021) 13:36. doi: 10.1038/s41368-021-00146-0
149. Moncada R, Barkley D, Wagner F, Chiodin M, Devlin JC, Baron M, et al. Integrating microarray-based spatial transcriptomics and single-cell RNA-seq reveals tissue architecture in pancreatic ductal adenocarcinomas. Nat Biotechnol. (2020) 38:333–42. doi: 10.1038/s41587-019-0392-8
150. Chen A, Liao S, Cheng M, Ma K, Wu L, Lai Y, et al. Spatiotemporal transcriptomic atlas of mouse organogenesis using DNA nanoball-patterned arrays. Cell. (2022) 185:1777–1792.e21. doi: 10.1016/j.cell.2022.04.003
151. Cheng M, Jiang Y, Xu J, Mentis AA, Wang S, Zheng H, et al. Spatially resolved transcriptomics: a comprehensive review of their technological advances, applications, and challenges. J Genet Genomics. (2023) 50:625–40. doi: 10.1016/j.jgg.2023.03.011
Keywords: prostate cancer, sequencing, omics, molecular pathogenesis, clinical application
Citation: Lu B, Liu Y, Yao Y, Yang T, Zhang H, Yang X, Huang R, Zhou W, Pan X and Cui X (2024) Advances in sequencing and omics studies in prostate cancer: unveiling molecular pathogenesis and clinical applications. Front. Oncol. 14:1355551. doi: 10.3389/fonc.2024.1355551
Received: 14 December 2023; Accepted: 16 April 2024;
Published: 10 May 2024.
Edited by:
Benyi Li, University of Kansas Medical Center, United StatesReviewed by:
Rui Chen, Second Military Medical University, ChinaJiejie Xu, Fudan University, China
Wang Liu, University of Kansas Medical Center, United States
Copyright © 2024 Lu, Liu, Yao, Yang, Zhang, Yang, Huang, Zhou, Pan and Cui. This is an open-access article distributed under the terms of the Creative Commons Attribution License (CC BY). The use, distribution or reproduction in other forums is permitted, provided the original author(s) and the copyright owner(s) are credited and that the original publication in this journal is cited, in accordance with accepted academic practice. No use, distribution or reproduction is permitted which does not comply with these terms.
*Correspondence: Runzhi Huang, runzhihuang2022@163.com; Wang Zhou, brilliant212@163.com; Xiuwu Pan, panxiuwu@126.com; Xingang Cui, cuixingang@xinhuamed.com.cn
†These authors have contributed equally to this work and share first authorship