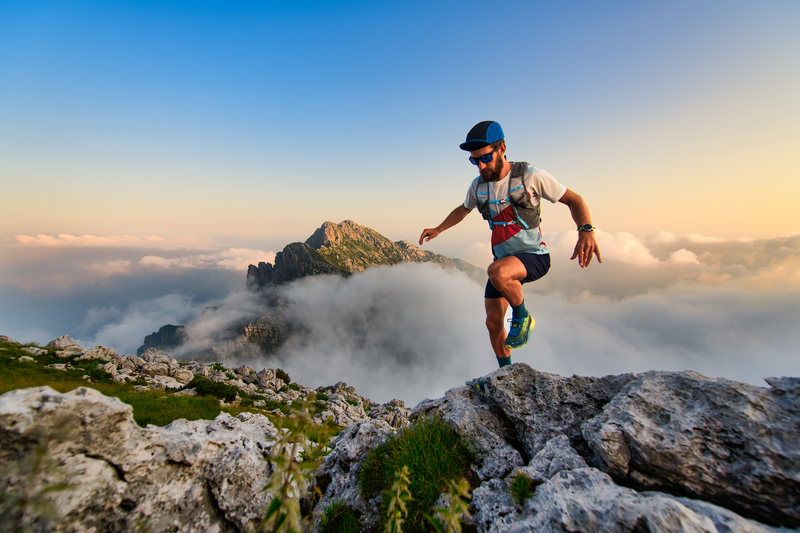
94% of researchers rate our articles as excellent or good
Learn more about the work of our research integrity team to safeguard the quality of each article we publish.
Find out more
REVIEW article
Front. Oncol. , 08 May 2024
Sec. Radiation Oncology
Volume 14 - 2024 | https://doi.org/10.3389/fonc.2024.1338225
This article is part of the Research Topic Radiotherapy for Brain Metastases View all 10 articles
Brain metastases (BMs) are the most prevalent intracranial malignant tumors in adults and are the leading cause of mortality attributed to malignant brain diseases. Radiotherapy (RT) plays a critical role in the treatment of BMs, with local RT techniques such as stereotactic radiosurgery (SRS)/stereotactic body radiotherapy (SBRT) showing remarkable therapeutic effectiveness. The precise determination of gross tumor target volume (GTV) is crucial for ensuring the effectiveness of SRS/SBRT. Multimodal imaging techniques such as CT, MRI, and PET are extensively used for the diagnosis of BMs and GTV determination. With the development of functional imaging and artificial intelligence (AI) technology, there are more innovative ways to determine GTV for BMs, which significantly improve the accuracy and efficiency of the determination. This article provides an overview of the progress in GTV determination for RT in BMs.
Brain metastases (BMs) are the most frequent intracranial malignant tumors in adults; among patients with malignant tumors, the incidence rate of BMs in adults is 10%–30%, and that in children is 6%–10% (1). The leading tumor type resulting in BMs is lung cancer, accounting for approximately 20%, followed by melanoma, breast cancer, etc. (1). Notably, the incidence of BMs surpasses that of primary brain tumors, making them the primary cause of mortality from malignant brain diseases (2).
The treatment methods for BMs include whole-brain radiotherapy (WBRT), stereotactic radiosurgery (SRS)/stereotactic body radiotherapy (SBRT), surgery, and systemic therapy (3). In recent years, an increasing amount of clinical evidence supports the application of local RT techniques such as SRS/SBRT in BMs (4). Accurate determination of the gross tumor target volume (GTV) is crucial for ensuring the efficacy of SRS/SBRT. With the development of advanced imaging technology and information science and technology, the use of multimodal imaging technology and artificial intelligence (AI) to improve the accuracy of GTV determination in BMs has become a popular research direction.
Radiotherapy (RT) serves as the primary treatment method for BMs. Objective studies have shown that patients with symptomatic BMs typically have a median overall survival (OS) of just 1 month without treatment. However, treatment with glucocorticosteroids alone, such as dexamethasone, can extend the median OS to 2 months (5). Lagerwaard et al. (6) reported that patients who received different doses of WBRT experienced an extended median OS of 3–6 months and a 1-year survival rate of 10%.
WBRT is the standard treatment for multiple BMs (7). However, WBRT often results in delayed adverse events including leukoencephalopathy, associated cognitive dysfunction, cerebral atrophy, and radionecrosis. Cognitive dysfunction is reported in approximately 10%–20% of patients undergoing WBRT (8). To avoid radiation damage to normal brain tissue, local RT methods such as SRS/SBRT have been developed. Multiple international clinical trials support SRS as the preferred treatment for BM patients with one to four metastases (9–12). The JLGK0901 (4) study revealed that patients with five to 10 BMs achieved comparable treatment results with patients with two to four BMs by receiving SRS treatment; the median OS all reached 10.8 months. Therefore, SRS could be considered an alternative treatment for multiple BMs instead of WBRT.
SRS/SBRT requires precise GTV determination and accurate dose delivery, which are directly related to the therapeutic efficacy and prognosis. The small size of BMs and their unclear enhancement affect lesion detection and visibility of tumor boundaries, which are the key challenges that need to be addressed in GTV determination for BMs.
Computed tomography (CT) simulation images serve as the primary foundation for GTV determination in BMs. CT offers high spatial resolution, minimal distortion, and sensitivity to features such as bleeding, calcification, and structural changes in the surrounding skull of BMs. The linear relationship between the CT value and tissue electron density is the basis for dose calculations in RT planning (12) (Figure 1).
Figure 1 CT imaging manifestations of BMs. [(A) CT scan without contrast; (B) CT contrast scan in arterial phase; (C) CT contrast scan in vein phase].
However, single-energy CT (SECT) is susceptible to bone artifacts, peri-tumor edematous areas, and fibrosis, limiting its use in GTV determination in BMs. Emerging CT technologies provide a reliable means to improve the precision of GTV determination.
Dual-energy computed tomography (DECT) improves the signal-noise ratio (SNR) and contrast-noise ratio (CNR) of intracranial abnormal metal deposits, iodine contrast, normal brain tissue, and abnormal lesions, resulting in superior image quality (13). DECT reduces the beam hardening artifacts caused by the skull through a special reconstruction algorithm, improving the image quality of posterior fossa tumors and improving BM imaging (14).
DECT shows greater image enhancement at low tube voltages (15). Karino et al. (16) analyzed energy spectral images of virtual monochromatic images (VMI) with energy levels ranging from 40 to 140 KeV in increments of 1 keV gradient and found that a VMI of 63 KeV significantly improved the overall image quality and BM boundary display. Kraft et al. (17) further compared the differences between 63 keV reconstructed VMI and 120 kV CT in BM imaging and confirmed that the image quality of VMI was significantly better than that of conventional CT, which could improve the reliability and accuracy of GTV determination in BMs.
Compared with CT, magnetic resonance (MR) provides high soft tissue resolution, clear differentiation between tumors and tissue edema, and avoids radiation injury. MR is thus indispensable for diagnosing, planning treatment, and posttreatment monitoring of brain tumors (18).
Contrast-enhanced MRI (CE-MRI) with gadolinium (Gd)-based contrast serves as the gold standard for identifying BMs (19). However, the contrast of the enhanced region of BMs in CE-MRI is affected by various factors, including the characteristics of the blood–brain barrier (BBB), magnetic field strength, concentration of Gd-based contrast agent (GBCA), relaxivity properties, time elapsed since injection, and the MR imaging technique (20). Derks et al. (12) found that 3T MR was more sensitive than 1.5 T MR in the diagnosis of small-volume BMs with diameters < 5 mm.
Cheng et al. (21) found that 7T MR improved visualization of small structures and subtle brain tumor lesions compared to 3T MR, which had significant potential for the diagnosis, treatment, and monitoring of BMs. However, the increase in magnetic field strength also leads to greater magnetization artifacts, which affects the GTV determination of BMs.
CE-T1WI and T2/Fluid-attenuated inversion recovery (FLAIR) are the most commonly used sequences in MR simulations of BMs (22).
There are differences in the imaging of BMs on T1WI with different imaging bases. A comparative analysis of the detection rates of BMs using enhanced spin-echo (SE) and gradient-echo (GRE) sequences by Suh et al. (23) revealed that, with a layer thickness of 1 mm, 3D SE images had a 20.6% higher detection rate than 3D GRE images. Additionally, for lesions with diameters less than 5 mm, 3D SE images exhibited a 30.1% higher detection rate than 3D GRE images. For the detection of BMs, especially lesions less than 5 mm in size, 3D SE-enhanced images with a 1-mm-layer slice thickness are more suitable.
Whether delayed enhancement MRI can improve the detection rate of BMs has been controversial (Figure 2). Cohen et al. (24) concluded that the detection rate of BMs by delayed CE-MRI is related to lesion volume. For larger BMs, delayed CE-MRI is not more advantageous than immediate postcontrast-enhanced imaging; however, for lesions with diameters ≤ 5 mm, delayed CE-MRI improves the detection rate of lesions and increases the display of tumor boundaries, especially when the delay time is more than 10 min (25–28).
Figure 2 The effect of time-delayed contrast-enhanced T1WI on the visualization of BMs. [(A–F) The BM manifest on contrast-enhanced T1WI at 1, 3, 5, 10, 18, and 20 min after Gd-DTPA injection].
Chen et al. (29) investigated the effect of CE-MRI with different delay times on the generation of small-volume BMs, and the results showed that compared with 10 min after contrast agent injection, the metastatic volumes at 1, 3, and 5 min decreased by 31.6%, 18.5%, and 10.1%, respectively, and the metastatic volumes at 18 and 20 min increased by only 8% and 10%, respectively. Therefore, CE-MRI with a delay time of more than 10 min should be a routine modality for the detection and border display of small-volume BMs.
Several studies have demonstrated that high-dose Gd-based contrast agents (GBCA) improve the demonstration of BMs, and the use of triple-dose contrast agents increases the detection of BMs < 5 mm by 65.6%; however, it also increases the risk of patient complications and the cost of the examination (26, 30–33).
Unlike CE-T1WI, T2/FLAIR uses a low concentration of GBCA to enhance the lesion, and the contrast agent required to enhance the lesion is only one-fourth of that required for CE-T1WI (34, 35). Several studies have demonstrated that CE-T2/FLAIR is superior in detecting leptomeningeal metastases, small-volume lesions, and lesions located in superficial areas of the brain (36, 37) (Figure 3).
Figure 3 The display differences of BMs among T1WI, T1WI+C, and T2/FLAIR. [(A) T1WI without contrast; (B) CE-T1WI; (C) CE-T2/FLAIR].
There were differences in the detection rate and enhancement degree of BMs between CE-T2/FLAIR and CE-T1WI. Jin et al. (38) suggested that this was related to the vascular permeability and microvascular density around the lesions of BMs. In lesions with higher density or greater damage to the BBB, the venous leakage of the contrast medium increased, resulting in a large accumulation of GBCA in the extracellular space. The enhancement effect of T2 reduced the degree of enhancement of the lesions and even led to negative enhancement. Thus, the degree of CE-T2/FLAIR enhancement was negatively associated with vascular permeability and demonstrated superior enhancement in BMs with low vascular permeability. CE-T2/FLAIR is an effective supplement to CE-T1WI, and the combined application of the two improves the detection and determination accuracy of BMs (39).
Positron emission tomography (PET) is a noninvasive imaging technique used to assess the biological function and metabolism of tumors. 18F fluorodeoxyglucose (18F-FDG) is the most widely used PET tracer, but normal brain tissue also shows high glucose uptake, which affects the GTV determination of BMs (40). Compared to 18F-FDG, the uptake of radiolabeled amino acids is lower, allowing them to cross the intact BBB, revealing BMs beyond CE MRI, and providing new insights for delineating biological targets in BMs (41).
PET/CT is used to obtain information on tumor biology and metabolism, which is valuable for determining biological GTVs. However, it has limitations in detecting BMs, especially for small-volume BMs. Factors such as lower FDG uptake by small BMs and the lower spatial resolution of PET/CT affect image quality, while the high uptake of inflammatory tissue reduces diagnostic specificity (42). In a study of more than 900 patients with BMs from lung cancer, Li et al. (43) found that CE-MRI had a higher sensitivity than FDG PET/CT for diagnosing BMs in patients (77% vs. 21%).
PET/CT is primarily used to detect extracranial lesions and is not highly sensitive for BMs, especially those with small volumes. Therefore, PET/CT is often complemented by the combination of enhanced MRI or CT (Figure 4).
PET/MRI has the advantage of simultaneously capturing both structural and functional aspects of tumors, which enhances RT planning and the assessment of treatment response (44). By combining high-resolution soft tissue PET with MRI, PET/MR provides a reliable basis for precise GTV determination in BMs.
Singnurkar et al. (45) compared the clinical performance of FDG PET/MR with FDG PET/CT in tumor imaging. FDG PET/MR was found to be similar to FDG PET/CT in detecting local lymph nodes and distant metastases but superior in assessing the local extent of tumors. Similarly, another study by Sekine et al. (46) demonstrated a 6.7% improvement in PET/MR imaging compared to PET/CT for occult tumors, including brain tumors.
CT provides crucial anatomical information and electron density data for RT planning and dose calculation, but it lacks sufficient soft tissue contrast, making it unreliable to determine GTV only based on CT alone (47). MRI provides high soft tissue resolution, enabling differentiation between active tumors and edematous tissue. The fusion of CT and MRI images combines the advantages of both modalities. However, differences in the underlying principles of CT and MRI imaging modalities, poor reproducibility of body fixation, poor accuracy of patient positioning, interlayer differences in layer thicknesses of images from different modalities, and tumor regression due to treatment all contribute to the poor quality of CT/MRI fusion images, which affects the visualization of the BMs and the accuracy of the GTV determination.
Few controlled studies of the volume and dosimetric effects of CT/MRI fusion in BMs’ GTVs have been reported. However, in general, CT/MRI fusion can improve the determination accuracy of GTV in BMs, allowing for personalized treatment options, organ preservation, or functional avoidance. Moreover, it facilitates intensification and dose escalation strategies (Figure 5).
Figure 5 The boundary determination of BMs applying CT/MRI fusion. [(A) CE-T1WI images; (B) Enhanced CT images; (C) CT/MRI fusion].
AI is trained and validated on large datasets to provide automated tools to assist physicians in accurately and quickly detecting BMs in large-scale medical imaging datasets. Manually determining BMs’ GTVs is time-consuming and challenging, and autosegmentation significantly enhances efficiency and precision. Deep learning models, such as convolutional neural networks (CNN), have shown promising results in BM image segmentation. Since 2018, AI-based contouring has evolved from classical machine learning (cML) to deep learning (DL). Cho et al. (48) conducted a systematic review of the literature on BM detection based on machine learning. The detection rates of BMs in the cML and DL groups were 88.7% and 90.1%, respectively. The DL group had a lower false-positive rate per patient than the cML group (10 vs. 135), indicating a clear advantage for DL. Deep learning models are adaptable and resilient in handling complex lesion shapes and indistinct boundaries through substantial labeled image data assimilation.
CNN-based AI has gained widespread acceptance for the screening and identification of BMs. Grovik et al. (49) conducted a study evaluating a CNN deep learning method for the automatic detection and segmentation of BMs using multisequence MRI. The results showed an average sensitivity of 83% for detecting BMs, indicating remarkable accuracy. However, the network’s ability to detect BMs was related to lesion size. It was shown that by using the optimal probability threshold (average sensitivity = 83%), the network showed an average false-positive rate of 8.3 (no size limit) and 3.4 (10 mm3 size limit) lesions per case, with the highest sensitivity and lowest numbers of false positives in patients with few metastases.
Zhou et al. (50) developed a DL single-shot detector (SSD) algorithm based on CE T1WI to detect BMs. For the test group, the sensitivity of the baseline SSD was 81%; the sensitivity was 98% for metastases ≥ 6 mm in diameter. The combined algorithm of feature fusion (FF) and SSD developed by Amemiya et al. (51) revealed that the FF and baseline SSDs showed an overall sensitivity of 86.0% and 83.8% and a positive predictive value (PPV) of 46.8% and 45.2%, respectively. Thus, FF SSD significantly improved the small lesion detection without reducing the overall PPV. Li et al. (52) introduced a two-stage deep learning model designed for automatic BM detection and segmentation, achieving a detection sensitivity rate of 91%.
With more data availability and technological advancements, AI is expected to become a crucial tool for diagnosing and treating BMs. However, there are still challenges in brain tumor imaging research related to AI technology. Modeling requires large datasets from multiple centers. However, subjectivity can be introduced during the preprocessing stage when physicians manually segment the images. This necessitates high requirements for consistency in image data quality. Therefore, most AI models for BMs are still in the research stage, and their clinical application requires thorough validation.
With the development of functional imaging, biology-guided RT has gradually become part of clinical practice. Biologic target volumes refer to regions within the target volume with varying radiosensitivities, determined by various tumor biological factors, including hypoxia, blood supply, proliferation, apoptosis, cell cycle regulation, infiltration, and metastatic properties. Tumor hypoxia is common in RT due to abnormalities in the tumor vasculature. Hypoxic cells are highly resistant to RT, resulting in a relative lack of local tumor doses. Therefore, identifying and quantifying tumor hypoxia is crucial for improving the effectiveness of RT.
Functional MRI, including dynamic contrast-enhanced MRI (DCE-MRI) and dynamic susceptibility contrast-perfusion-weighted imaging (DSC-PWI), can identify, quantify, and spatially map areas of hypoxia before treatment and track hypoxia changes during radiation (53, 54). It can help improve the radiation dose to the hypoxic RT-resistance area through dose engraving and protect the organ at risk (OAR), and it should be incorporated into the practice of radiotherapy in BMs (55, 56).
Functional MR imaging techniques, such as PWI, diffusion-weighted imaging (DWI), and magnetic resonance spectroscopy, provide clinical insight into tumor metabolism, pathophysiology, and microcirculatory status, and they are increasingly used for GTV determination in BMs.
PWI can be categorized into two main types: those that use Gd contrast agents and those that do not. DSC and DCE, both based on Gd injections, efficiently evaluate vascular infiltration and neovascularization in the enhanced regions of BMs. DSC quantifies tumor vascular supply and is the most frequently employed PWI technique for brain tumor evaluation (57).
Arterial spin labeling (ASL) is a noninvasive method for examining blood flow changes in BMs without the use of paramagnetic contrast agents and without being influenced by the BBB. This imaging technique uses hydrogen protons present in arterial blood as endogenous tracers to provide accurate results. Soni et al. (58) conducted a quantitative comparison of perfusion values acquired through ASL and DSC in brain tumors, demonstrating a favorable degree of agreement. As a result, ASL, as a noninvasive test, exhibits greater potential for broad utilization.
Hou et al. (54) used MR-3D-ASL to map cerebral blood flow to determine the high-perfused GTV (GTVH) versus the low-perfused GTV (GTVL). Their findings revealed an uneven distribution of perfusion within BMs and variations in intratumoral and intertumoral perfusion between tumors with and without necrosis. Dose escalation to low-perfusion areas with RT resistance should be performed by reducing the dose boost volume so that the dose to the target area is targeted and distributed according to blood perfusion. Hou et al. (59) further designed three RT plans based on CBF measurements. When compared to the conventional plan, the dose painting plan exhibited that the D2%, D98% (doses to 2% and 98% volume of the PTV), and the mean dose increased by 20.50%, 19.32%, and 19.60% in the low-perfusion region, respectively. Therefore, 3D-ASL-guided dose painting effectively increases the radiation dose to the low-perfusion subregion without increasing the dose to the OAR, and subregion identification and segmentation based on the differences in blood perfusion in the GTV are of great clinical significance (Figure 6).
Figure 6 The cerebral blood volume variation of BMs before and after radiotherapy. [(A, C, E) T1WI+C images; (B, D, F) 3D-ASL cerebral blood volume images; (A, B) before radiotherapy; (C, D) 4 weeks after radiotherapy; (E, F) 1 year after radiotherapy].
Conventional MRI sequences have limitations in distinguishing between infiltrating tumors and vasogenic edema. Apparent diffusion coefficient (ADC) values can measure the extent to which water molecules are restricted in healthy brain tissue and central nervous system lesions. On high b-valued DWI, BMs appear as high-intensity signals with low ADC values, which can distinguish tumor and edema.
A study conducted by Zhong et al. (60) identified differences in the ADC values between patients with and without BMs. An ADC value of 0.837 × 10−3 mm/s was found to be critical for distinguishing BMs from nonbrain metastases, yielding a sensitivity of 83.7% and a specificity of 69.2%, resulting in a high BM detection rate. However, DWI has low resolution and is prone to magnetization artifacts, and its clinical application in BMs is still mainly qualitative. The study of GTV determination of BMs based on DWI is still under investigation.
Accurate target determination is the primary premise for ensuring the efficacy of local RT for BMs. How to improve the accuracy of GTV determination is one of the key issues in improving the efficacy of SRS/SBRT. With the progression of functional imaging technology and AI, methods such as MRI and PET provide more comprehensive data analysis concerning tumor size, infiltration depth, internal microenvironment, and chemical composition within BMs, which provides a feasible method for further improving the detection of BMs and the precision of GTV determination and provides a broad perspective for the clinical application of individualized RT.
SD: Writing – original draft. GG: Writing – review & editing. RL: Writing – original draft. KM: Writing – original draft. YY: Writing – review & editing.
The author(s) declare financial support was received for the research, authorship, and/or publication of this article. This work was supported in part by the Taishan Scholars Project of Shandong Province (Grant No. ts201712098).
The authors acknowledge the assistance of Shandong Cancer Hospital and Institute (Shandong Cancer Hospital) in the preparation of the manuscript.
The authors declare that the research was conducted in the absence of any commercial or financial relationships that could be construed as a potential conflict of interest.
All claims expressed in this article are solely those of the authors and do not necessarily represent those of their affiliated organizations, or those of the publisher, the editors and the reviewers. Any product that may be evaluated in this article, or claim that may be made by its manufacturer, is not guaranteed or endorsed by the publisher.
1. Noh T, Walbert T. Brain metastasis: clinical manifestations, symptom management, and palliative care. Handb Clin Neurol. (2018) 149:75–88. doi: 10.1016/B978-0-12-811161-1.00006-2
2. Deshpande K, Buchanan I, Martirosian V, Neman J. Clinical perspectives in brain metastasis. Cold Spring Harb Perspect Med. (2020) 10:a037051. doi: 10.1101/cshperspect.a037051
3. Garsa A, Jang JK, Baxi S, Chen C, Akinniranye O, Hall O, et al. Radiation therapy for brain metastases: A systematic review. Pract Radiat Oncol. (2021) 11:354–65. doi: 10.1016/j.prro.2021.04.002
4. Yamamoto M, Serizawa T, Shuto T, Akabane A, Higuchi Y, Kawagishi J, et al. Stereotactic radiosurgery for patients with multiple brain metastases (JLGK0901): a multi-institutional prospective observational study. Lancet Oncol. (2014) 15:387–95. doi: 10.1016/S1470-2045(14)70061-0
5. Den RB, Andrews DW. Radiotherapy for brain metastases. Neurosurg Clin N Am. (2011) 22:37–44, vi. doi: 10.1016/j.nec.2010.08.001
6. Lagerwaard FJ, Levendag PC, Nowak PJ, Eijkenboom WM, Hanssens PE, Schmitz PI. Identification of prognostic factors in patients with brain metastases: a review of 1292 patients. Int J Radiat Oncol Biol Physics. (1999) 43:795–803. doi: 10.1016/s0360-3016(98)00442-8
7. Brown PD, Ahluwalia MS, Khan OH, Asher AL, Wefel JS, Gondi V. Whole-brain radiotherapy for brain metastases: evolution or revolution? J Clin Oncol. (2018) 36:483–+. doi: 10.1200/jco.2017.75.9589
8. Narita Y, Sato S, Kayama T. Review of the diagnosis and treatment of brain metastases. Jpn J Clin Oncol. (2022) 52:3–7. doi: 10.1093/jjco/hyab182
9. Kayama T, Sato S, Sakurada K, Mizusawa J, Nishikawa R, Narita Y, et al. Effects of surgery with salvage stereotactic radiosurgery versus surgery with whole-brain radiation therapy in patients with one to four brain metastases (JCOG0504): A phase III, noninferiority, randomized controlled trial. J Clin Oncol. (2018) 36:3282–+. doi: 10.1200/jco.2018.78.6186
10. Mahajan A, Ahmed S, McAleer MF, Weinberg JS, Li J, Brown P, et al. Post-operative stereotactic radiosurgery versus observation for completely resected brain metastases: a single-centre, randomised, controlled, phase 3 trial. Lancet Oncol. (2017) 18:1040–8. doi: 10.1016/s1470-2045(17)30414-x
11. Brown PD, Ballman KV, Cerhan JH, Anderson SK, Carrero XW, Whitton AC, et al. Postoperative stereotactic radiosurgery compared with whole brain radiotherapy for resected metastatic brain disease (NCCTG N107C/CEC·3): a multicentre, randomised, controlled, phase 3 trial. Lancet Oncol. (2017) 18:1049–60. doi: 10.1016/s1470-2045(17)30441-2
12. Derks S, van der Veldt AAM, Smits M. Brain metastases: the role of clinical imaging. Br J Radiol. (2022) 95:20210944. doi: 10.1259/bjr.20210944
13. Chiro GD, Brooks RA, Kessler RM, Johnston GS, Jones AE, Herdt JR, et al. Tissue signatures with dual-energy computed tomography. Radiol. (1979) 131:521–3. doi: 10.1148/131.2.521
14. Hwang WD, Mossa-Basha M, Andre JB, Hippe DS, Culbertson S, Anzai Y. Qualitative comparison of noncontrast head dual-energy computed tomography using rapid voltage switching technique and conventional computed tomography. J Comput Assist Tomogr. (2016) 40:320–5. doi: 10.1097/RCT.0000000000000350
15. Johnson TR, Krauss B, Sedlmair M, Grasruck M, Bruder H, Morhard D, et al. Material differentiation by dual energy CT: initial experience. Eur Radiol. (2007) 17:1510–7. doi: 10.1007/s00330-006-0517-6
16. Karino T, Ohira S, Kanayama N, Wada K, Ikawa T, Nitta Y, et al. Determination of optimal virtual monochromatic energy level for target delineation of brain metastases in radiosurgery using dual-energy CT. Br J Radiol. (2020) 93:20180850. doi: 10.1259/bjr.20180850
17. Kraft J, Lutyj P, Grabenbauer F, Strohle SP, Tamihardja J, Razinskas G, et al. Assessment of dual-energy computed tomography derived virtual monoenergetic imaging for target volume delineation of brain metastases. Radiother Oncol. (2023) 187:109840. doi: 10.1016/j.radonc.2023.109840
18. Fink JR, Muzi M, Peck M, Krohn KA. Multimodality brain tumor imaging: MR imaging, PET, and PET/MR imaging. J Nucl Med. (2015) 56:1554–61. doi: 10.2967/jnumed.113.131516
19. Essig M, Anzalone N, Combs SE, Dorfler A, Lee SK, Picozzi P, et al. MR imaging of neoplastic central nervous system lesions: review and recommendations for current practice. AJNR Am J Neuroradiol. (2012) 33:803–17. doi: 10.3174/ajnr.A2640
20. Danieli L, Riccitelli GC, Distefano D, Prodi E, Ventura E, Cianfoni A, et al. Brain tumor-enhancement visualization and morphometric assessment: A comparison of MPRAGE, SPACE, and VIBE MRI techniques. AJNR Am J Neuroradiol. (2019) 40:1140–8. doi: 10.3174/ajnr.A6096
21. Cheng K, Duan Q, Hu J, Li C, Ma X, Bian X, et al. Evaluation of postcontrast images of intracranial tumors at 7T and 3T MRI: An intra-individual comparison study. CNS Neurosci Ther. (2023) 29:559–65. doi: 10.1111/cns.14036
22. Tong E, McCullagh KL, Iv M. Advanced imaging of brain metastases: from augmenting visualization and improving diagnosis to evaluating treatment response. Front Neurol. (2020) 11:270. doi: 10.3389/fneur.2020.00270
23. Suh CH, Jung SC, Kim KW, Pyo J. The detectability of brain metastases using contrast-enhanced spin-echo or gradient-echo images: a systematic review and meta-analysis. J Neurooncol. (2016) 129:363–71. doi: 10.1007/s11060-016-2185-y
24. Cohen-Inbar O, Xu Z, Dodson B, Rizvi T, Durst CR, Mukherjee S, et al. Time-delayed contrast-enhanced MRI improves detection of brain metastases: a prospective validation of diagnostic yield. J Neurooncol. (2016) 130:485–94. doi: 10.1007/s11060-016-2242-6
25. Kang KM, Choi SH, Hwang M, Yoo RE, Yun TJ, Kim JH, et al. Application of Synthetic MRI for Direct Measurement of Magnetic Resonance Relaxation Time and Tumor Volume at Multiple Time Points after Contrast Administration: Preliminary Results in Patients with Brain Metastasis. Korean J Radiol. (2018) 19:783–91. doi: 10.3348/kjr.2018.19.4.783
26. Kushnirsky M, Nguyen V, Katz JS, Steinklein J, Rosen L, Warshall C, et al. Time-delayed contrast-enhanced MRI improves detection of brain metastases and apparent treatment volumes. J Neurosurg. (2016) 124:489–95. doi: 10.3171/2015.2.JNS141993
27. Jeon JY, Choi JW, Roh HG, Moon WJ. Effect of imaging time in the magnetic resonance detection of intracerebral metastases using single dose gadobutrol. Korean J Radiol. (2014) 15:145–50. doi: 10.3348/kjr.2014.15.1.145
28. Russell EJ, Geremia GK, Johnson CE, Huckman MS, Ramsey RG, Washburn-Bleck J, et al. Multiple cerebral metastases: detectability with Gd-DTPA-enhanced MR imaging. Radiol. (1987) 165:609–17. doi: 10.1148/radiology.165.3.3317495
29. Chen M, Wang P, Guo Y, Yin Y, Wang L, Su Y, et al. The effect of time delay for magnetic resonance contrast-enhanced scan on imaging for small-volume brain metastases. NeuroImage Clin. (2022) 36:103223. doi: 10.1016/j.nicl.2022.103223
30. Runge VM, Wells JW, Williams NM. Magnetic resonance imaging of an experimental model of intracranial metastatic disease. A study of lesion detectability. Invest Radiol. (1994) 29:1050–6. doi: 10.1097/00004424-199412000-00007
31. Yuh WT, Fisher DJ, Runge VM, Atlas SW, Harms SE, Maravilla KR, et al. Phase III multicenter trial of high-dose gadoteridol in MR evaluation of brain metastases. AJNR Am J Neuroradiol. (1994) 15:1037–51.
32. Runge VM, Wells JW, Nelson KL, Linville PM. MR imaging detection of cerebral metastases with a single injection of high-dose gadoteridol. J Magnetic Resonance Imaging: JMRI. (1994) 4:669–73. doi: 10.1002/jmri.1880040509
33. Yuh WT, Tali ET, Nguyen HD, Simonson TM, Mayr NA, Fisher DJ. The effect of contrast dose, imaging time, and lesion size in the MR detection of intracerebral metastasis. AJNR Am J Neuroradiol. (1995) 16:373–80.
34. Jin T, Ge M, Huang R, Yang Y, Liu T, Zhan Q, et al. Utility of contrast-enhanced T2 FLAIR for imaging brain metastases using a half-dose high-relaxivity contrast agent. AJNR Am J Neuroradiol. (2021) 42:457–63. doi: 10.3174/ajnr.A6931
35. Oguz KK, Cila A. Rim enhancement of meningiomas on fast FLAIR imaging. Neuroradiology. (2003) 45:78–81. doi: 10.1007/s00234-002-0914-8
36. Fukuoka H, Hirai T, Okuda T, Shigematsu Y, Sasao A, Kimura E, et al. Comparison of the added value of contrast-enhanced 3D fluid-attenuated inversion recovery and magnetization-prepared rapid acquisition of gradient echo sequences in relation to conventional postcontrast T1-weighted images for the evaluation of leptomeningeal diseases at 3T. AJNR Am J Neuroradiol. (2010) 31:868–73. doi: 10.3174/ajnr.A1937
37. Seong M, Park S, Kim ST, Park SG, Kim YK, Kim HJ, et al. Diagnostic accuracy of MR imaging of patients with leptomeningeal seeding from lung adenocarcinoma based on 2017 RANO proposal: added value of contrast-enhanced 2D axial T2 FLAIR. J Neurooncol. (2020) 149:367–72. doi: 10.1007/s11060-020-03617-2
38. Jin T, Zhang H, Liu X, Kong X, Makamure J, Chen Z, et al. Enhancement degree of brain metastases: correlation analysis between enhanced T2 FLAIR and vascular permeability parameters of dynamic contrast-enhanced MRI. Eur Radiol. (2021) 31:5595–604. doi: 10.1007/s00330-020-07625-8
39. Ahn SJ, Chung TS, Chang JH, Lee SK. The added value of double dose gadolinium enhanced 3D T2 fluid-attenuated inversion recovery for evaluating small brain metastases. Yonsei Med J. (2014) 55:1231–7. doi: 10.3349/ymj.2014.55.5.1231
40. Chen W. Clinical applications of PET in brain tumors. J Nucl Med. (2007) 48:1468–81. doi: 10.2967/jnumed.106.037689
41. Langen KJ, Galldiks N. Update on amino acid PET of brain tumours. Curr Opin Neurol. (2018) 31:354–61. doi: 10.1097/WCO.0000000000000574
42. Galldiks N, Langen KJ, Albert NL, Chamberlain M, Soffietti R, Kim MM, et al. PET imaging in patients with brain metastasis-report of the RANO/PET group. Neuro Oncol. (2019) 21:585–95. doi: 10.1093/neuonc/noz003
43. Li Y, Jin G, Su D. Comparison of Gadolinium-enhanced MRI and 18FDG PET/PET-CT for the diagnosis of brain metastases in lung cancer patients: A meta-analysis of 5 prospective studies. Oncotarget. (2017) 8:35743–9. doi: 10.18632/oncotarget.16182
44. Guo L, Wang G, Feng Y, Yu T, Guo Y, Bai X, et al. Diffusion and perfusion weighted magnetic resonance imaging for tumor volume definition in radiotherapy of brain tumors. Radiat Oncol. (2016) 11:123. doi: 10.1186/s13014-016-0702-y
45. Singnurkar A, Poon R, Metser U. Comparison of 18F-FDG-PET/CT and 18F-FDG-PET/MR imaging in oncology: a systematic review. Ann Nucl Med. (2017) 31:366–78. doi: 10.1007/s12149-017-1164-5
46. Sekine T, Barbosa FG, Sah BR, Mader CE, Delso G, Burger IA, et al. PET/MR outperforms PET/CT in suspected occult tumors. Clin Nucl Med. (2017) 42:e88–95. doi: 10.1097/RLU.0000000000001461
47. Popp I, Weber WA, Combs SE, Yuh WTC, Grosu AL. Neuroimaging for radiation therapy of brain tumors. Topics Magnetic Resonance Imaging: TMRI. (2019) 28:63–71. doi: 10.1097/rmr.0000000000000198
48. Cho SJ, Sunwoo L, Baik SH, Bae YJ, Choi BS, Kim JH. Brain metastasis detection using machine learning: a systematic review and meta-analysis. Neuro Oncol. (2021) 23:214–25. doi: 10.1093/neuonc/noaa232
49. Grovik E, Yi D, Iv M, Tong E, Rubin D, Zaharchuk G. Deep learning enables automatic detection and segmentation of brain metastases on multisequence MRI. J Magn Reson Imaging. (2020) 51:175–82. doi: 10.1002/jmri.26766
50. Zhou Z, Sanders JW, Johnson JM, Gule-Monroe MK, Chen MM, Briere TM, et al. Computer-aided detection of brain metastases in T1-weighted MRI for stereotactic radiosurgery using deep learning single-shot detectors. Radiol. (2020) 295:407–15. doi: 10.1148/radiol.2020191479
51. Amemiya S, Takao H, Kato S, Yamashita H, Sakamoto N, Abe O. Feature-fusion improves MRI single-shot deep learning detection of small brain metastases. J Neuroimaging. (2022) 32:111–9. doi: 10.1111/jon.12916
52. Li R, Guo Y, Zhao Z, Chen M, Liu X, Gong G, et al. MRI-based two-stage deep learning model for automatic detection and segmentation of brain metastases. Eur Radiol. (2023) 33:3521–31. doi: 10.1007/s00330-023-09420-7
53. Jensen R, Salzman K, Schabel M. Preoperative dynamic contrast-enhanced mri correlates with molecular markers of hypoxia and vascularity in specific areas of intratumoral microenvironment and is predictive of patient outcome. Neuro-Oncology. (2014) 16:iii13. doi: 10.1093/neuonc/nou206.47
54. Hou C, Gong G, Wang L, Su Y, Lu J, Yin Y. Study of sub-region segmentation of brain metastases based on magnetic resonance perfusion imaging. Chin J Radiat Oncol. (2021) 30:1047–53. doi: 10.3760/cma.j.cn113030-20200613-00306
55. Li M, Zhang Q, Yang K. Role of MRI-based functional imaging in improving the therapeutic index of radiotherapy in cancer treatment. Front Oncol. (2021) 11:645177. doi: 10.3389/fonc.2021.645177
56. Djan I, Petrovic B, Erak M, Nikolic I, Lucic S. Radiotherapy treatment planning: benefits of CT-MR image registration and fusion in tumor volume delineation. Vojnosanit Pregl. (2013) 70:735–9. doi: 10.2298/vsp110404001d
57. Scola E, Desideri I, Bianchi A, Gadda D, Busto G, Fiorenza A, et al. Assessment of brain tumors by magnetic resonance dynamic susceptibility contrast perfusion-weighted imaging and computed tomography perfusion: a comparison study. Radiol Med. (2022) 127:664–72. doi: 10.1007/s11547-022-01470-z
58. Soni N, Dhanota DPS, Kumar S, Jaiswal AK, Srivastava AK. Perfusion MR imaging of enhancing brain tumors: Comparison of arterial spin labeling technique with dynamic susceptibility contrast technique. Neurol India. (2017) 65:1046–52. doi: 10.4103/neuroIndia.NI_871_16
59. Hou C, Yin H, Gong G, Wang L, Su Y, Lu J, et al. A novel approach for dose painting radiotherapy of brain metastases guided by mr perfusion images. Front Oncol. (2022) 12:828312. doi: 10.3389/fonc.2022.828312
Keywords: brain metastases, MRI, GTV, delineation, artificial intelligence
Citation: Du S, Gong G, Liu R, Meng K and Yin Y (2024) Advances in determining the gross tumor target volume for radiotherapy of brain metastases. Front. Oncol. 14:1338225. doi: 10.3389/fonc.2024.1338225
Received: 14 November 2023; Accepted: 19 April 2024;
Published: 08 May 2024.
Edited by:
Yirui Zhai, Chinese Academy of Medical Sciences and Peking Union Medical College, ChinaReviewed by:
Mirek Fatyga, Mayo Clinic Arizona, United StatesCopyright © 2024 Du, Gong, Liu, Meng and Yin. This is an open-access article distributed under the terms of the Creative Commons Attribution License (CC BY). The use, distribution or reproduction in other forums is permitted, provided the original author(s) and the copyright owner(s) are credited and that the original publication in this journal is cited, in accordance with accepted academic practice. No use, distribution or reproduction is permitted which does not comply with these terms.
*Correspondence: Yong Yin, eWlueW9uZ3NkQDEyNi5jb20=
Disclaimer: All claims expressed in this article are solely those of the authors and do not necessarily represent those of their affiliated organizations, or those of the publisher, the editors and the reviewers. Any product that may be evaluated in this article or claim that may be made by its manufacturer is not guaranteed or endorsed by the publisher.
Research integrity at Frontiers
Learn more about the work of our research integrity team to safeguard the quality of each article we publish.