- The Fifth People’s Hospital of Jinan, Jinan, Shandong, China
Background: With the increasing use of radiomics in cancer diagnosis and treatment, it has been applied by some researchers to the preoperative risk assessment of endometrial cancer (EC) patients. However, comprehensive and systematic evidence is needed to assess its clinical value. Therefore, this study aims to investigate the application value of radiomics in the diagnosis and treatment of EC.
Methods: Pubmed, Cochrane, Embase, and Web of Science databases were retrieved up to March 2023. Preoperative risk assessment of EC included high-grade EC, lymph node metastasis, deep myometrial invasion status, and lymphovascular space invasion status. The quality of the included studies was appraised utilizing the RQS scale.
Results: A total of 33 primary studies were included in our systematic review, with an average RQS score of 7 (range: 5–12). ML models based on radiomics for the diagnosis of malignant lesions predominantly employed logistic regression. In the validation set, the pooled c-index of the ML models based on radiomics and clinical features for the preoperative diagnosis of endometrial malignancy, high-grade tumors, lymph node metastasis, lymphovascular space invasion, and deep myometrial invasion was 0.900 (95%CI: 0.871–0.929), 0.901 (95%CI: 0.877–0.926), 0.906 (95%CI: 0.882–0.929), 0.795 (95%CI: 0.693–0.897), and 0.819 (95%CI: 0.705–0.933), respectively.
Conclusions: Radiomics shows excellent accuracy in detecting endometrial malignancies and in identifying preoperative risk. However, the methodological diversity of radiomics results in significant heterogeneity among studies. Therefore, future research should establish guidelines for radiomics studies based on different imaging sources.
Systematic review registration: https://www.crd.york.ac.uk/PROSPERO/display_record.php?RecordID=364320 identifier CRD42022364320.
1 Introduction
Endometrial cancer (EC), also known as uterine corpus cancer, is the most prevalent gynecological cancer in high-income countries, with an increasing global incidence rate. This cancer mainly occurs in perimenopausal and postmenopausal women. Current lifestyle leads to the rising prevalence of obesity, thereby increasing the risk of EC (1–3). The annual mortality risk for EC patients exhibits an upward trend (4).
Surgical intervention remains the primary treatment approach for EC patients, with total hysterectomy combined with bilateral salpingo-oophorectomy being the standard procedure. Surgery can be performed through open or minimally invasive methods. Some EC patients may require adjuvant therapies (4). There is considerable variation in the postoperative prognosis of EC patients. Two reviews have indicated that tumor diameter, myometrial invasion, lymphovascular space invasion, and lymph node metastasis are significantly associated with poor prognosis (5, 6). However, in clinical practice, achieving an accurate preoperative diagnosis of high-grade tumors, myometrial invasion, lymph node metastasis, and lymphovascular space invasion remains a daunting challenge.
Radiomics, an emerging field based on quantitative imaging techniques, can extract high-throughput quantitative radiological features from medical images (7, 8). Radiomics is the process of extracting quantifiable features from large amounts of data that may be relevant to potential biological or clinical outcomes using advanced machine learning analysis techniques. It is carried out based on 2D, 3D or 4D medical images. There are two main branches of the field, namely, manual radiomics and deep learning radiomics (9). In manual radiomics, firstly, clinical staff use specialized software (commonly 3D-slicer (10) or ITK-snap (11)) to segment the region of interest (ROI) and extract texture features in the ROI region from established medical images. Secondly, in the process of feature filtering or dimensionality reduction, a large number of features will be generated in the process of extracting texture features of the ROI region, thus creating a “dimensionality disaster”. Therefore, it is necessary to combine with reasonable feature filtering methods or dimensionality reduction methods (e.g., principal component analysis). Thirdly, machine learning models (e.g., Random Forest, Support Vector Machines, Artificial Neural Networks) are then constructed based on the filtered features or dimensionality reduction results to make predictions about disease state or treatment outcomes. Fourthly, the constructed machine learning method is then validated. As for radiomics carried out by deep learning, researchers can directly construct deep learning models based on images (12, 13). Currently, manual radiomics is dominant in radiomics research.
Radiomics has gradually been used to help develop tumor treatment strategies (14). Against this backdrop, radiomics has been introduced into the detection of endometrial malignancies and the preoperative risk assessment of EC patients. However, comprehensive systematic evidence is required to explore its feasibility and accuracy. Therefore, the present study was carried out to investigate the application value of radiomics in the diagnosis and treatment of EC patients.
2 Methods
2.1 Study registration
The current study was conducted following the Preferred Reporting Items for Systematic Reviews and Meta-analyses (PRISMA) statement (15). The study protocol has been registered in the International prospective register of systematic reviews (ID: CRD42022364320).
2.2 Eligibility criteria
2.2.1 Inclusion criteria
1. Studies that had reasonable diagnostic criteria for (EC).
2. Studies that comprehensively constructed radiomics-based machine learning (ML) models for the detection of endometrial malignancy or risk assessment, including high-grade EC, lymph node metastasis, deep myometrial invasion, and lymphovascular space invasion.
3. Primary studies in which independent external validation was not performed.
4. Studies that used different ML approaches published on the same dataset.
5. Study designs: case-control studies, cohort studies, cross-sectional studies, or randomized controlled trials (RCTs).
6. Studies reported in English.
2.2.2 Exclusion criteria
1. Meta-analyses, reviews, expert opinions, guidelines, and similar types of studies.
2. Studies that only conduct differential factor analysis without constructing complete ML models.
3. Studies lacking the following outcome measures for evaluating the predictive accuracy of ML models: ROC curve, c-statistic, c-index, sensitivity, specificity, accuracy, recall, precision, confusion matrix, diagnostic fourfold table, F1 score, and calibration curve.
4. Studies with a small sample size (<20 cases).
5. Studies that solely focused on image segmentation or texture extraction without constructing complete ML models.
2.3 Data sources and search strategy
PubMed, Cochrane, Embase, and Web of Science databases were retrieved up to July 24, 2022. A combination of MeSH terms and free-text terms was used for the search, without restrictions on publication year or region. To mitigate the risk of missing newly published primary studies, we conducted additional searches in all databases in March 2023. Detailed search strategies are presented in Supplementary Table 1.
2.4 Study selection and data extraction
The retrieved articles were imported into EndNote software. Duplicate studies were identified and excluded using both automated and manual methods. Titles and abstracts were screened to select potentially eligible studies. Full texts of these articles were then downloaded and read to determine eligible primary studies.
Prior to data extraction, a standardized form was used to collect the following information: first author, country, year of publication, type of artificial intelligence model, sample size, mean/median age of patients, histological grading of EC, depth of myometrial invasion and cervical invasion, assessment of lymph node metastasis, source of imaging data, number of segmenters for ROI segmentation and software used, number of cases in the training set, generation method of validation set, number of cases in the validation set, feature selection method, model type, modeling variables, and outcome measures for model evaluation.
The aforementioned literature screening was conducted independently by two researchers, with cross-checking performed upon completion. Discrepancies, if any, were resolved by consulting a third researcher.
2.5 Assessment of study quality
The methodological quality of the included studies was appraised by two independent researchers using the Radiomics Quality Score (RQS) (8). After completion, a cross-check was carried out. Dissents, if any, were resolved by consulting a third researcher.
2.6 Outcomes
The primary outcome measure is the c-index, which reflects the overall accuracy of ML models. In many primary studies, only the c-index was reported. However, when the number of cases is severely imbalanced, it becomes challenging to interpret the specific accuracy of the model for positive and negative events based on the c-index alone. Therefore, our main outcome measures also include sensitivity and specificity at the optimal threshold value of the model.
2.7 Synthesis methods
A meta-analysis of c-index was carried out to assess the overall accuracy of the ML models. For primary studies where the 95% confidence interval and standard error were missing for the c-index, we estimated the standard error following the approach described by Debray et al. (16). A random-effects model was preferred for the meta-analysis of c-index, given the variations in the included variables and inconsistent parameters across different ML models.
Additionally, the meta-analysis of sensitivity and specificity was performed utilizing a bivariate mixed-effects model. The meta-analysis of sensitivity and specificity was based on the diagnostic fourfold table. However, as many primary studies did not report the diagnostic fourfold table, we constructed it using sensitivity, specificity, precision, and the number of cases, or using sensitivity and specificity derived from the best Youden’s index and the number of cases. R4.2.0 was employed for meta-analysis (R development Core Team, Vienna, http://www.R-project.org).
3 Results
3.1 Study selection
We retrieved a total of 290 articles (183 from the initial search and 107 from the supplementary search), out of which 172 were identified as duplicates (141 by automated software and 31 by manual identification). After screening titles and abstracts, 54 articles remained. After reading the full text, 33 studies were ultimately included in our systematic review (17–49) (Figure 1).
3.2 Study characteristics
This study includes 33 articles published within the past five years. These studies were conducted in countries such as Italy, France, Norway, China, Spain, Japan, and Canada. Among the 33 studies included, two studies (18, 32) were prospective cohort studies, while the other studies were case-control studies. Eleven studies (24, 30, 35, 36, 38, 41, 42, 44–46, 48) were multicenter studies, while the other 23 studies were conducted at a single center. Three studies (17, 21) utilized 18F-FDG PET/CT as the imaging modality, while the rest of the studies utilized MRI. The predominant models in the included studies were logistic regression (LR), with only a few studies utilizing artificial neural networks (ANN), support vector machines (SVM), and decision trees (DT). Detailed information on the included studies is provided in Supplementary Table 2.
3.3 Assessment of study quality
The included primary studies achieved no scores due to a lack of description of the differences between imaging scanners, vendor dependencies, imaging at multiple time points with collection of individual images at other time points, reducing overfitting by reducing functionality or multiple testing, prospective registration in trial databases, comparison with the “gold standard,” and open science and data—open code and data. The validation set was generated by random sampling. Overall, the average score for the 33 studies was 7 (range: 5–10) (Table 1).
3.4 Meta-analysis
3.4.1 Preoperative diagnosis of malignant lesions
ML models based on radiomics for the diagnosis of malignant lesions predominantly employed logistic regression. In the training dataset, the pooled c-index, sensitivity, and specificity of ML models based solely on clinical features were 0.733 (95%CI: 0.674–0.791), 0.55–0.73, and 0.67–0.77, respectively. The pooled c-index, sensitivity, and specificity of ML models constructed solely using radiomics features were 0.869 (95%CI: 0.809–0.928), 0.88 (95%CI: 0.81–0.93), and 0.73 (95%CI: 0.63–0.82), respectively. The pooled c-index, sensitivity, and specificity of ML models constructed based on radiomics and clinical features were 0.924 (95%CI: 0.910–0.937), 0.83 (95%CI: 0.80–0.87), and 0.88 (95%CI: 0.85–0.90), respectively (Tables 2, 3).
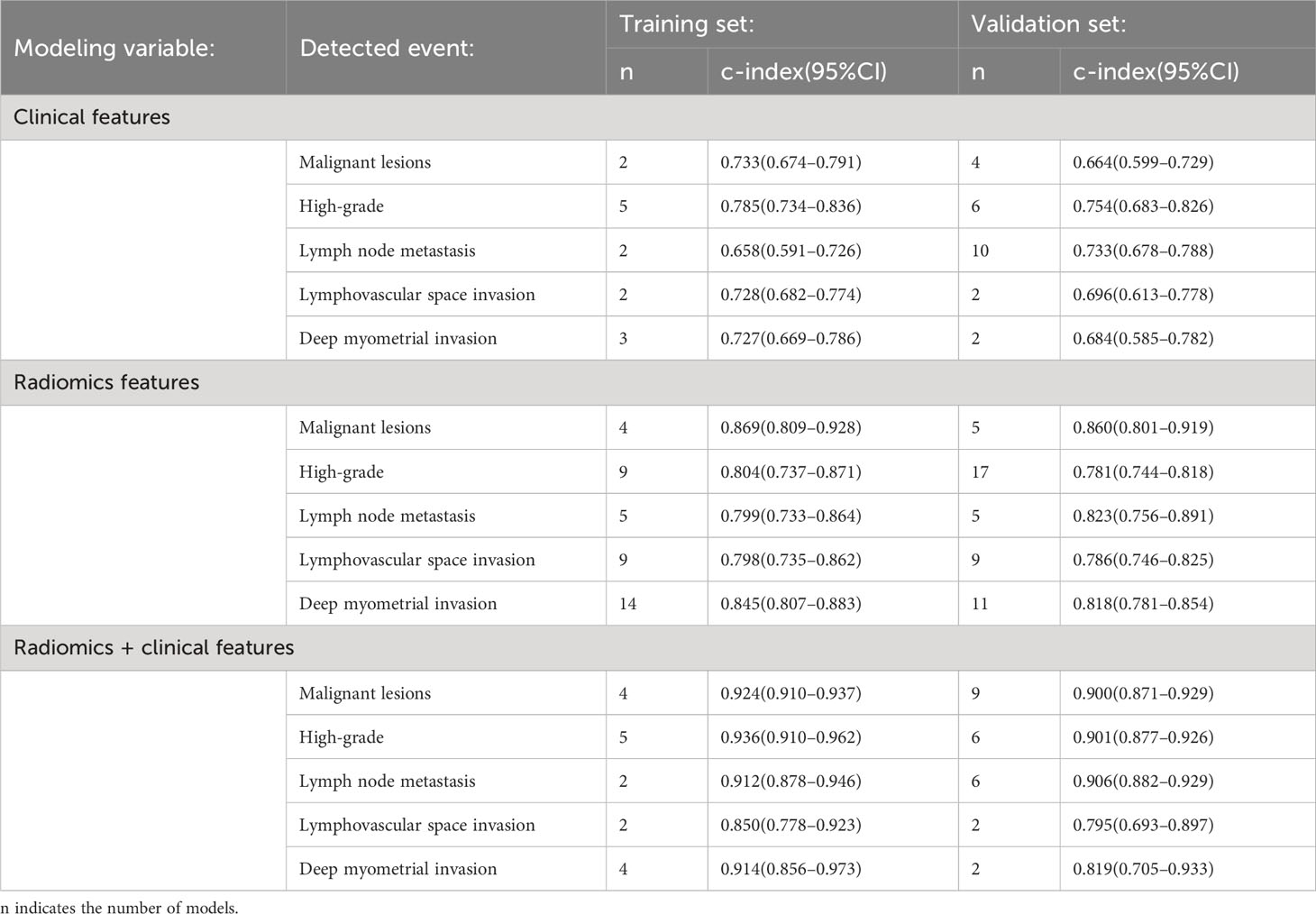
Table 2 Meta-analysis results of the c-index for radiomics-based models in the detection of malignant endometrial lesions and preoperative identification of risks in EC patients.
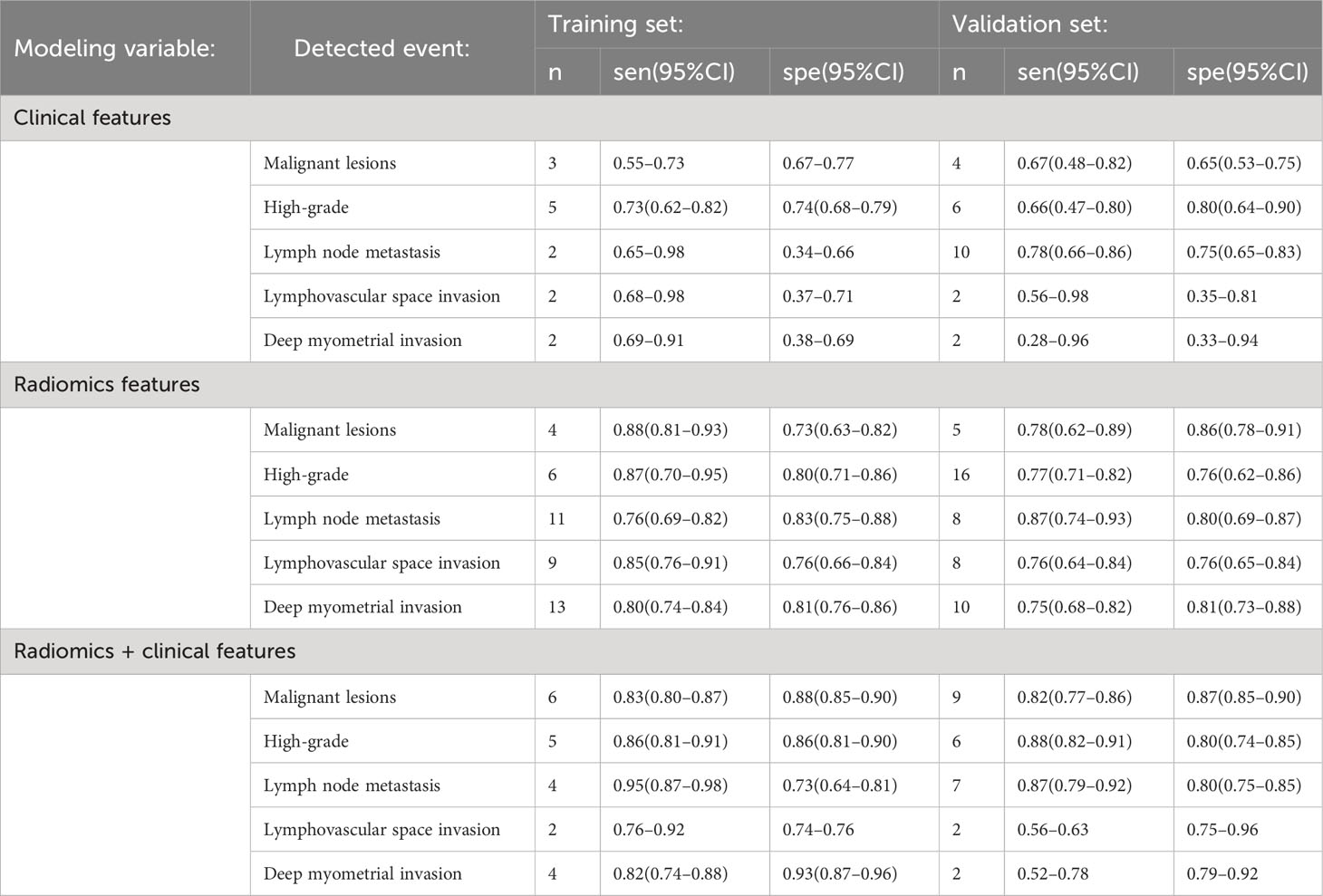
Table 3 Meta-analysis results of sensitivity and specificity for radiomics-based models in the detection of malignant endometrial lesions and preoperative identification of risks in EC patients.
In the validation dataset, the pooled c-index, sensitivity, and specificity for ML models based solely on clinical features were 0.664 (95%CI: 0.599–0.729), 0.67 (95%CI: 0.48–0.82) and 0.65 (95%CI: 0.53–0.75), respectively. The pooled c-index, sensitivity, and specificity of ML models constructed solely using radiomics features were 0.860 (95%CI: 0.801–0.919), 0.78 (95%CI: 0.62–0.89) and 0.86 (95%CI: 0.78–0.91), respectively. The pooled c-index, sensitivity, and specificity of ML models constructed based on radiomics and clinical features were 0.900 (95%CI: 0.871–0.929), 0.82 (95%CI: 0.77–0.86), and 0.87 (95%CI: 0.85–0.90), respectively (Tables 2, 3).
3.4.2 Diagnosis of high-grade tumors
ML models based on radiomics for the diagnosis of high-grade ECs predominantly employed logistic regression. In the training dataset, the pooled c-index, sensitivity, and specificity of ML models based solely on clinical features were 0.785 (95%CI: 0.734–0.836), 0.73 (95%CI: 0.62–0.82), and 0.74(95%CI: 0.68–0.79), respectively. The pooled c-index, sensitivity, and specificity of ML models constructed solely using radiomics features were 0.804 (95%CI: 0.737–0.871), 0.87 (95%CI: 0.70–0.95), and 0.80 (95%CI: 0.71–0.86), respectively. The pooled c-index, sensitivity, and specificity of ML models constructed based on radiomics and clinical features were 0.936 (95%CI: 0.910–0.962), 0.86 (95%CI: 0.81–0.91) and 0.86 (95%CI: 0.81–0.90), respectively (Tables 2, 3).
In the validation dataset, the pooled c-index, sensitivity, and specificity of ML models based solely on clinical features were 0.754 (95%CI: 0.683–0.826), 0.66 (95%CI: 0.47–0.80) and 0.80 (95%CI: 0.64–0.90), respectively. The pooled c-index, sensitivity, and specificity of ML models constructed solely using radiomics features were 0.781 (95%CI: 0.744–0.818), 0.77 (95%CI: 0.71–0.82), and 0.76 (95%CI: 0.62–0.86), respectively. The pooled c-index, sensitivity, and specificity of ML models constructed based on radiomics and clinical features were 0.901 (95%CI: 0.877–0.926), 0.88 (95%CI: 0.82–0.91) and 0.86 (95%CI: 0.74–0.85), respectively (Tables 2, 3).
3.4.3 Preoperative diagnosis of lymph node metastasis
ML models utilizing radiomics for the diagnosis of lymph node metastasis predominantly employed logistic regression. In the training dataset, the pooled c-index, sensitivity, and specificity of ML models based solely on clinical features were 0.658 (95%CI: 0.591–0.726), 0.65–0.98 and 0.34–0.66, respectively. The pooled c-index, sensitivity, and specificity of ML models constructed solely using radiomics features were 0.799 (95%CI: 0.733–0.864), 0.77 (95%CI: 0.63–0.86), and 0.87 (95%CI: 0.81–0.92), respectively. The pooled c-index, sensitivity, and specificity of ML models constructed based on radiomics and clinical features were 0.912 (95%CI: 0.878–0.946), 0.92–0.95 and 0.64–0.84, respectively (Tables 2, 3).
In the validation dataset, the pooled c-index, sensitivity, and specificity of ML models based solely on clinical features were 0.733 (95%CI: 0.678–0.788), 0.78 (95%CI: 0.66–0.86) and 0.75 (95%CI: 0.65–0.83), respectively. The pooled c-index, sensitivity, and specificity of ML models constructed solely using radiomics features were 0.823 (95%CI: 0.756–0.891), 0.86 (95%CI: 0.73–0.94), and 0.80 (95%CI: 0.67–0.88), respectively. The pooled c-index, sensitivity, and specificity of ML models constructed based on radiomics and clinical features were 0.906 (95%CI: 0.882–0.929), 0.87 (95%CI: 0.79–0.92) and 0.81 (95%CI: 0.75–0.85), respectively (Tables 2, 3).
3.4.4 Preoperative diagnosis of lymphovascular space invasion
ML models utilizing radiomics for the diagnosis of lymphovascular space invasion predominantly employed logistic regression. In the training dataset, the pooled c-index, sensitivity, and specificity of ML models based solely on clinical features were 0.728 (95%CI: 0.682–0.774), 0.68–0.98 and 0.37–0.71, respectively. The pooled c-index, sensitivity, and specificity of ML models constructed solely using radiomics features were 0.798 (95%CI: 0.735–0.862), 0.85 (95%CI: 0.76–0.91), and 0.76 (95%CI: 0.66–0.84), respectively. The pooled c-index, sensitivity, and specificity of ML models constructed based on radiomics and clinical features were 0.850 (95%CI: 0.778–0.923), 0.76–0.92 and 0.74–0.76, respectively (Tables 2, 3).
In the validation dataset, the pooled c-index, sensitivity, and specificity of ML models based solely on clinical features were 0.696 (95%CI: 0.613–0.778), 0.56–0.98 and 0.35–0.81, respectively. The pooled c-index, sensitivity, and specificity of ML models constructed solely using radiomics features were 0.786 (95%CI: 0.746–0.825), 0.76 (95%CI: 0.64–0.84) and 0.76 (95%CI: 0.65–0.84), respectively. The pooled c-index, sensitivity, and specificity of ML models constructed based on radiomics and clinical features were 0.795 (95%CI: 0.693–0.897), 0.56–0.63 and 0.75–0.96, respectively (Tables 2, 3).
3.4.5 Preoperative diagnosis of deep myometrial invasion
ML models utilizing radiomics for the diagnosis of deep myometrial invasion predominantly employed logistic regression. In the training dataset, the pooled c-index, sensitivity, and specificity of ML models based solely on clinical features were 0.727 (95%CI: 0.669–0.786), 0.69–0.91 and 0.38–0.69, respectively. For ML models constructed solely using radiomics features, the pooled c-index, sensitivity, and specificity were 0.845 (95%CI: 0.807–0.883), 0.80 (95%CI: 0.74–0.84), and 0.81 (95%CI: 0.76–0.86), respectively. For ML models constructed based on radiomics and clinical features, the pooled c-index, sensitivity, and specificity were 0.914 (95%CI: 0.856–0.973), 0.82 (95%CI: 0.74–0.88) and 0.93 (95%CI: 0.87–0.96), respectively (Tables 2, 3).
In the validation dataset, the pooled c-index, sensitivity, and specificity of ML models based solely on clinical features were 0.684 (95%CI: 0.585–0.782), 0.28–0.96 and 0.33–0.94, respectively. For ML models constructed solely using radiomics features, the pooled c-index, sensitivity, and specificity were 0.818 (95%CI: 0.781–0.854), 0.75 (95%CI: 0.68–0.82), and 0.81 (95%CI: 0.73–0.88), respectively. For ML models constructed based on radiomics and clinical features, the pooled c-index, sensitivity, and specificity were 0.819 (95%CI: 0.705–0.933), 0.52–0.78 and 0.79–0.92, respectively (Tables 2, 3).
4 Discussion
4.1 Summary of the main findings
This work examined the application value of radiomics-based methods in the preoperative detection of malignant endometrial lesions, high-grade tumors, lymph node metastasis, lymphovascular space invasion, and deep myometrial invasion in EC patients. Additionally, we meta-analyzed the c-index values of ML models constructed using clinical features alone, radiomics features alone, and a combination of radiomics and clinical features, and the sensitivity and specificity at the optimal cut-off values were also meta-analyzed. Our findings demonstrate that radiomics features have shown promising accuracy in the diagnosis of malignant endometrial lesions, high-grade ECs, lymph node metastasis in EC patients, lymphovascular space invasion, and deep myometrial invasion. In particular, radiomic features combined with clinical features show a more favorable performance, yielding the best results. Importantly, no overfitting phenomenon was observed in our analysis.
4.2 Comparison with previous studies (other reviews)
Current non-invasive preoperative diagnosis of endometrial malignancies mainly involves ultrasound, which appears to rely on different cut-off values for endometrial thickness. A systematic review by Breijer et al. (50) focusing on the detection of endometrial malignancies in asymptomatic postmenopausal women reported a sensitivity of 0.83 (95%CI: 0.19–1.00) when using a threshold of 5 mm for endometrial thickness. However, with a threshold of 6mm, the sensitivity dropped to only 0.33 (95%CI: 0.04–0.85). Similarly, Vitale et al. (51), in their systematic review on the detection of endometrial malignancies in asymptomatic postmenopausal women, did not recommend a specific cut-off value for endometrial thickness. Instead, they suggested a range of 3.0–5.9 mm, which seemed to have higher sensitivity and specificity. Furthermore, Long et al. (52), in their systematic review focusing on endometrial malignancy detection in postmenopausal women with bleeding, had excellent sensitivity of 0.96 (95%CI: 0.92–0.98) but sacrificed specificity (0.52 (95%CI: 0.42–0.61)). These findings indicate that endometrial thickness remains an important diagnostic criterion in the ultrasound-based diagnosis of endometrial malignancies. Moreover, we also observed that certain clinical features hold significant diagnostic value in detecting EC. For example, Li et al. (53) reported in their systematic review that human epididymis protein 4 demonstrated a sensitivity of 0.71 (95%CI: 0.56–0.82) and specificity of 0.87 (95%CI: 0.80–0.92). Our systematic review demonstrated that ML models solely based on radiomics for detecting endometrial malignancies achieved a sensitivity of 0.78 (95%CI: 0.62–0.89) and specificity of 0.86 (95%CI: 0.78–0.91) in the validation set. For models constructed using radiomics and clinical features, there was a modest improvement in sensitivity (0.82, 95%CI: 0.77–0.86) and specificity (0.87, 95%CI: 0.85–0.90) in the validation set.
For preoperative risk assessment of EC, three-dimensional vaginal ultrasound, MRI, and 18F-FDG PET/CT are the main imaging modalities commonly used. They primarily contribute to the preoperative diagnosis of lymph node metastasis (54, 55), lymphovascular space invasion (56), deep myometrial invasion (56), and cervical stromal invasion (57, 58). In our study, the majority of included imaging data were derived from MRI, with only two studies utilizing 18F-FDG PET/CT for identifying lymph node metastasis. A recent systematic review by Di Donato et al. (59) on MRI in EC focused on the diagnosis of high-grade tumors, deep myometrial invasion, lymph node metastasis, and lymphovascular space invasion. There are notable differences between our findings and those reported by Di Donato et al., mainly due to a broader scope of systematic search and comprehensive consideration of the importance of clinical variables in radiomics research.
Clinical features play a significant role in existing radiomics models. Reijnen et al. (60) highlighted in their systematic review that CA125 and other clinical variables can assist in identifying lymph node metastasis in EC patients. In our study, the accuracy of models solely based on clinical features was limited in the diagnosis and risk assessment of endometrial malignancies. However, models constructed based on radiomic and clinical features showed improved diagnostic performance compared to those based solely on radiomics. This finding underscores that effective modeling variables still serve as a key factor in enhancing the accuracy of ML models. In future research, exploring efficient predictive factors remains an important direction for advancing automation diagnosis of diseases.
Additionally, in our study, the models were primarily predictive nomograms based on logistic regression, with only a limited number of ANN, SVM, and DT models. The nomograms and decision trees are highly interpretable in clinical practice. The interpretability of models is significant in clinical practice (61), particularly those constructed based on clinical features. This is because in some opaque ML models such as SVM, random forest (RF), ANNs, and deep learning (DL), it becomes challenging to assess the impact of different levels of a variable on outcome events. This poses significant challenges in developing simplified risk scoring tools. The application of deep learning models based on imaging data for automatic disease diagnosis remains a daunting challenge in radiomics research, and its scope is still limited. In this context, in addition to ensuring accuracy, better interpretability seems to be an important assessment factor in model selection in clinical practice.
4.3 Advantages and limitations of the study
Our study explored the value of radiomics methods for the detection of endometrial malignancies and preoperative risk assessment from a systematic review perspective for the first time. However, our study also has the following limitations. (1) Despite a systematic search, the included studies were limited in quantity for different risk outcome events, which may have somewhat restricted the interpretation of our results; (2) The quality assessment of the included studies revealed concerns about the overall quality. However, we found that RQS is a stringent radiomics evaluation tool, with some items being challenging to meet in the primary studies and not applicable to certain ML models such as RFs, ANNs, SVMs, and DL (62). This resulted in relatively lower RQS scores in previously published radiomics-related systematic reviews (63, 64); (3) In the primary studies, validation methods for the models mainly were random sampling or k-fold cross-validation, with rare external validation; (4) In the primary studies, effective measures to mitigate the risk of overfitting were rarely employed when using radiomics.
5 Conclusions
Radiomics-based models appear to have promising diagnostic performance in the identification of endometrial malignancies and preoperative risk assessment, but the value of clinical features should not be overlooked. However, we also observed significant biases and concerns regarding the implementation of radiomics, particularly in terms of mitigating the risk of overfitting during the research process.
Data availability statement
The original contributions presented in the study are included in the article/Supplementary Material. Further inquiries can be directed to the corresponding author.
Author contributions
JH: Conceptualization, Formal analysis, Investigation, Methodology, Writing – original draft, Writing – review & editing. YL: Conceptualization, Formal analysis, Investigation, Writing – original draft, Writing – review & editing. JL: Conceptualization, Formal analysis, Investigation, Writing – review & editing. SL: Conceptualization, Methodology, Supervision, Writing – review & editing.
Funding
The author(s) declare that no financial support was received for the research, authorship, and/or publication of this article.
Conflict of interest
The authors declare that the research was conducted in the absence of any commercial or financial relationships that could be construed as a potential conflict of interest.
Publisher’s note
All claims expressed in this article are solely those of the authors and do not necessarily represent those of their affiliated organizations, or those of the publisher, the editors and the reviewers. Any product that may be evaluated in this article, or claim that may be made by its manufacturer, is not guaranteed or endorsed by the publisher.
Supplementary material
The Supplementary Material for this article can be found online at: https://www.frontiersin.org/articles/10.3389/fonc.2024.1334546/full#supplementary-material
References
1. Morice P, Leary A, Creutzberg C, Abu-Rustum N, Darai E. Endometrial cancer. Lancet (2016) 387(10023):1094–108. doi: 10.1016/S0140-6736(15)00130-0
2. Sung H, Ferlay J, Siegel RL, Laversanne M, Soerjomataram I, Jemal A, et al. Global cancer statistics 2020: GLOBOCAN estimates of incidence and mortality worldwide for 36 cancers in 185 countries. CA Cancer J Clin (2021) 71(3):209–49. doi: 10.3322/caac.21660
3. Crosbie EJ, Kitson SJ, McAlpine JN, Mukhopadhyay A, Powell ME, Singh N. Endometrial cancer. Lancet (2022) 399(10333):1412–28. doi: 10.1016/S0140-6736(22)00323-3
4. Makker V, MacKay H, Ray-Coquard I, Levine DA, Westin SN, Aoki D, et al. Endometrial cancer. Nat Rev Dis Primers (2021) 7(1):88. doi: 10.1038/s41572-021-00324-8
5. Jin X, Shen C, Yang X, Yu Y, Wang J, Che X. Association of tumor size with myometrial invasion, lymphovascular space invasion, lymph node metastasis, and recurrence in endometrial cancer: A meta-analysis of 40 studies with 53,276 patients. Front Oncol (2022) 12:881850. doi: 10.3389/fonc.2022.881850
6. Wang J, Xu P, Yang X, Yu Q, Xu X, Zou G, et al. Association of myometrial invasion with lymphovascular space invasion, lymph node metastasis, recurrence, and overall survival in endometrial cancer: A meta-analysis of 79 studies with 68,870 patients. Front Oncol (2021) 11:762329. doi: 10.3389/fonc.2021.762329
7. Gillies RJ, Kinahan PE, Hricak H. Radiomics: images are more than pictures, they are data. Radiology (2016) 278(2):563–77. doi: 10.1148/radiol.2015151169
8. Lambin P, Leijenaar RTH, Deist TM, Peerlings J, de Jong EEC, van Timmeren J, et al. et al. Radiomics: the bridge between medical imaging and personalized medicine. Nat Rev Clin Oncol (2017) 14(12):749–62. doi: 10.1038/nrclinonc.2017.141
9. Lambin P, Rios-Velazquez E, Leijenaar R, Carvalho S, van Stiphout RG, Granton P, et al. Radiomics: extracting more information from medical images using advanced feature analysis. Eur J Cancer (2012) 48(4):441–6. doi: 10.1016/j.ejca.2011.11.036
10. Kapur T, Pieper S, Fedorov A, Fillion-Robin JC, Halle M, O'Donnell L, et al. Increasing the impact of medical image computing using community-based open-access hackathons: The NA-MIC and 3D Slicer experience. Med Image Anal (2016) 33:176–80. doi: 10.1016/j.media.2016.06.035
11. Besson FL, Henry T, Meyer C, Chevance V, Roblot V, Blanchet E, et al. Rapid contour-based segmentation for 18F-FDG PET imaging of lung tumors by using ITK-SNAP: comparison to expert-based segmentation. Radiology (2018) 288(1):277–84. doi: 10.1148/radiol.2018171756
12. Mayerhoefer ME, Materka A, Langs G, Häggström I, Szczypiński P, Gibbs P, et al. Introduction to radiomics. J Nucl Med (2020) 61(4):488–95. doi: 10.2967/jnumed.118.222893
13. Huang EP, O'Connor JPB, McShane LM, Giger ML, Lambin P, Kinahan PE, et al. Criteria for the translation of radiomics into clinically useful tests. Nat Rev Clin Oncol (2023) 20(2):69–82. doi: 10.1038/s41571-022-00707-0
14. Bera K, Braman N, Gupta A, Velcheti V, Madabhushi A. Predicting cancer outcomes with radiomics and artificial intelligence in radiology. Nat Rev Clin Oncol (2022) 19(2):132–46. doi: 10.1038/s41571-021-00560-7
15. Page MJ, McKenzie JE, Bossuyt PM, Boutron I, Hoffmann TC, Mulrow CD, et al. The PRISMA 2020 statement: an updated guideline for reporting systematic reviews. Bmj (2021) 372:n71. doi: 10.1136/bmj.n71
16. Debray TP, Damen JA, Riley RD, Snell K, Reitsma JB, Hooft L, et al. A framework for meta-analysis of prediction model studies with binary and time-to-event outcomes. Stat Methods Med Res (2019) 28(9):2768–86. doi: 10.1177/0962280218785504
17. De Bernardi E, Buda A, Guerra L, Vicini D, Elisei F, Landoni C, et al. Radiomics of the primary tumour as a tool to improve (18)F-FDG-PET sensitivity in detecting nodal metastases in endometrial cancer. EJNMMI Res (2018) 8(1):86. doi: 10.1186/s13550-018-0441-1
18. Ytre-Hauge S, Dybvik JA, Lundervold A, Salvesen ØO, Krakstad C, Fasmer KE, et al. Preoperative tumor texture analysis on MRI predicts high-risk disease and reduced survival in endometrial cancer. J Magn Reson Imaging (2018) 48(6):1637–47. doi: 10.1002/jmri.26184
19. Bereby-Kahane M, Dautry R, Matzner-Lober E, Cornelis F, Sebbag-Sfez D, Place V, et al. Prediction of tumor grade and lymphovascular space invasion in endometrial adenocarcinoma with MR imaging-based radiomic analysis. Diagn Interv Imaging (2020) 101(6):401–11. doi: 10.1016/j.diii.2020.01.003
20. Chen J, Gu H, Fan W, Wang Y, Chen S, Chen X, et al. MRI-based radiomic model for preoperative risk stratification in stage I endometrial cancer. J Cancer (2021) 12(3):726–34. doi: 10.7150/jca.50872
21. Crivellaro C, Landoni C, Elisei F, Buda A, Bonacina M, Grassi T, et al. Combining positron emission tomography/computed tomography, radiomics, and sentinel lymph node mapping for nodal staging of endometrial cancer patients. Int J Gynecol Cancer (2020) 30(3):378–82. doi: 10.1136/ijgc-2019-000945
22. Han Y, Xu H, Ming Y, Liu Q, Huang C, Xu J, et al. Predicting myometrial invasion in endometrial cancer based on whole-uterine magnetic resonance radiomics. J Cancer Res Ther (2020) 16(7):1648–55. doi: 10.4103/jcrt.JCRT_1393_20
23. Luo Y, Mei D, Gong J, Zuo M, Guo X. Multiparametric MRI-based radiomics nomogram for predicting lymphovascular space invasion in endometrial carcinoma. J Magn Reson Imaging (2020) 52(4):1257–62. doi: 10.1002/jmri.27142
24. Yan BC, Li Y, Ma FH, Feng F, Sun MH, Lin GW, et al. Preoperative assessment for high-risk endometrial cancer by developing an MRI- and clinical-based radiomics nomogram: A multicenter study. J Magn Reson Imaging (2020) 52(6):1872–82. doi: 10.1002/jmri.27289
25. Long L, Sun J, Jiang L, Hu Y, Li L, Tan Y, et al. MRI-based traditional radiomics and computer-vision nomogram for predicting lymphovascular space invasion in endometrial carcinoma. Diagn Interv Imaging (2021) 102(7-8):455–62. doi: 10.1016/j.diii.2021.02.008
26. Rodríguez-Ortega A, Alegre A, Lago V, Carot-Sierra JM, Ten-Esteve A, Montoliu G, et al. Machine learning-based integration of prognostic magnetic resonance imaging biomarkers for myometrial invasion stratification in endometrial cancer. J Magn Reson Imaging (2021) 54(3):987–95. doi: 10.1002/jmri.27625
27. Soydal Ç, Varlı B, Araz M, Bakırarar B, Taşkın S, Ortaç UF. Radiomics analysis of uterine tumors in 18F-fluorodeoxyglucose positron emission tomography for prediction of lymph node metastases in endometrial carcinoma. Turk J Med Sci (2022) 52(3):762–9. doi: 10.55730/1300-0144.5371
28. Stanzione A, Cuocolo R, Del Grosso R, Nardiello A, Romeo V, Travaglino A, et al. Deep myometrial infiltration of endometrial cancer on MRI: A radiomics-Powered machine learning pilot study. Acad Radiol (2021) 28(5):737–44. doi: 10.1016/j.acra.2020.02.028
29. Xu Y, Zhao R. A prediction model of endometrial cancer lesion metastasis under region of interest target detection algorithm. Sci Programming (2021). doi: 10.1155/2021/9928842
30. Yan BC, Li Y, Ma FH, Zhang GF, Feng F, Sun MH, et al. Radiologists with MRI-based radiomics aids to predict the pelvic lymph node metastasis in endometrial cancer: a multicenter study. Eur Radiol (2021) 31(1):411–22. doi: 10.1007/s00330-020-07099-8
31. Yan BC, Ma XL, Li Y, Duan SF, Zhang GF, Qiang JW. MRI-based radiomics nomogram for selecting ovarian preservation treatment in patients with early-stage endometrial cancer. Front Oncol (2021) 11:730281. doi: 10.3389/fonc.2021.730281
32. Yang LY, Siow TY, Lin YC, Wu RC, Lu HY, Chiang HJ, et al. Computer-Aided segmentation and machine learning of integrated clinical and diffusion-weighted imaging parameters for predicting lymph node metastasis in endometrial cancer. Cancers (Basel) (2021) 13(6):1406–21. doi: 10.3390/cancers13061406
33. Zhang K, Zhang Y, Fang X, Fang M, Shi B, Dong J, et al. Nomograms of combining apparent diffusion coefficient value and radiomics for preoperative risk evaluation in endometrial carcinoma. Front Oncol (2021) 11:705456. doi: 10.3389/fonc.2021.705456
34. Zheng T, Yang L, Du J, Dong Y, Wu S, Shi Q, et al. Combination analysis of a radiomics-based predictive model with clinical indicators for the preoperative assessment of histological grade in endometrial carcinoma. Front Oncol (2021) 11:582495. doi: 10.3389/fonc.2021.582495
35. Chen X, Wang X, Gan M, Li L, Chen F, Pan J, et al. MRI-based radiomics model for distinguishing endometrial carcinoma from benign mimics: A multicenter study. Eur J Radiol (2022) 146:110072. doi: 10.1016/j.ejrad.2021.110072
36. Lefebvre TL, Ueno Y, Dohan A, Chatterjee A, Vallières M, Winter-Reinhold E, et al. Development and validation of multiparametric MRI-based radiomics models for preoperative risk stratification of endometrial cancer. Radiology (2022) 305(2):375–86. doi: 10.1148/radiol.212873
37. Liu XF, Yan BC, Li Y, Ma FH, Qiang JW. Radiomics nomogram in assisting lymphadenectomy decisions by predicting lymph node metastasis in early-stage endometrial cancer. Front Oncol (2022) 12:894918. doi: 10.3389/fonc.2022.894918
38. Mainenti PP, Stanzione A, Cuocolo R, Del Grosso R, Danzi R, Romeo V, et al. MRI radiomics: A machine learning approach for the risk stratification of endometrial cancer patients. Eur J Radiol (2022) 149:110226. doi: 10.1016/j.ejrad.2022.110226
39. Otani S, Himoto Y, Nishio M, Fujimoto K, Moribata Y, Yakami M, et al. Radiomic machine learning for pretreatment assessment of prognostic risk factors for endometrial cancer and its effects on radiologists' decisions of deep myometrial invasion. Magn Reson Imaging (2022) 85:161–7. doi: 10.1016/j.mri.2021.10.024
40. Wang Y, Bi Q, Deng Y, Yang Z, Song Y, Wu Y, et al. Development and validation of an MRI-based radiomics nomogram for assessing deep myometrial invasion in early stage endometrial adenocarcinoma. Acad Radiol (2023) 30(4):668–79. doi: 10.1016/j.acra.2022.05.017
41. Zhao M, Wen F, Shi J, Song J, Zhao J, Song Q, et al. MRI-based radiomics nomogram for the preoperative prediction of deep myometrial invasion of FIGO stage I endometrial carcinoma. Med Phys (2022) 49(10):6505–16. doi: 10.1002/mp.15835
42. Liu XF, Yan BC, Li Y, Ma FH, Qiang JW. Radiomics feature as a preoperative predictive of lymphovascular invasion in early-stage endometrial cancer: A multicenter study. Front Oncol (2022) 12:966529. doi: 10.3389/fonc.2022.966529
43. Bo J, Jia H, Zhang Y, Fu B, Jiang X, Chen Y, et al. Preoperative prediction value of pelvic lymph node metastasis of endometrial cancer: combining of ADC value and radiomics features of the primary lesion and clinical parameters. J Oncol (2022) 2022:3335048. doi: 10.1155/2022/3335048
44. Bi Q, Wang Y, Deng Y, Liu Y, Pan Y, Song Y, et al. Different multiparametric MRI-based radiomics models for differentiating stage IA endometrial cancer from benign endometrial lesions: A multicenter study. Front Oncol (2022) 12:939930. doi: 10.3389/fonc.2022.939930
45. Lefebvre TL, Ciga O, Bhatnagar SR, Ueno Y, Saif S, Winter-Reinhold E, et al. Predicting histopathology markers of endometrial carcinoma with a quantitative image analysis approach based on spherical harmonics in multiparametric MRI. Diagn Interv Imaging (2023) 104(3):142–52. doi: 10.1016/j.diii.2022.10.007
46. Celli V, Guerreri M, Pernazza A, Cuccu I, Palaia I, Tomao F, et al. MRI- and histologic-molecular-based radio-genomics nomogram for preoperative assessment of risk classes in endometrial cancer. Cancers (Basel) (2022) 14(23):5881–901. doi: 10.3390/cancers14235881
47. Zhang J, Zhang Q, Wang T, Song Y, Yu X, Xie L, et al. Multimodal MRI-based radiomics-clinical model for preoperatively differentiating concurrent endometrial carcinoma from atypical endometrial hyperplasia. Front Oncol (2022) 12:887546. doi: 10.3389/fonc.2022.887546
48. Miccò M, Gui B, Russo L, Boldrini L, Lenkowicz J, Cicogna S, et al. Preoperative tumor texture analysis on MRI for high-risk disease prediction in endometrial cancer: A hypothesis-generating study. J Pers Med (2022) 12(11):1854–69. doi: 10.3390/jpm12111854
49. Liu XF, Yan BC, Li Y, Ma FH, Qiang JW. Radiomics nomogram in aiding preoperatively dilatation and curettage in differentiating type II and type I endometrial cancer. Clin Radiol (2023) 78(2):e29–36. doi: 10.1016/j.crad.2022.08.139
50. Breijer MC, Peeters JA, Opmeer BC, Clark TJ, Verheijen RH, Mol BW, et al. Capacity of endometrial thickness measurement to diagnose endometrial carcinoma in asymptomatic postmenopausal women: a systematic review and meta-analysis. Ultrasound Obstet Gynecol (2012) 40(6):621–9. doi: 10.1002/uog.12306
51. Vitale SG, Riemma G, Haimovich S, Carugno J, Alonso Pacheco L, Perez-Medina T, et al. Risk of endometrial cancer in asymptomatic postmenopausal women in relation to ultrasonographic endometrial thickness: systematic review and diagnostic test accuracy meta-analysis. Am J Obstet Gynecol (2023) 228(1):22–35.e22. doi: 10.1016/j.ajog.2022.07.043
52. Long B, Clarke MA, Morillo ADM, Wentzensen N, Bakkum-Gamez JN. Ultrasound detection of endometrial cancer in women with postmenopausal bleeding: Systematic review and meta-analysis. Gynecol Oncol (2020) 157(3):624–33. doi: 10.1016/j.ygyno.2020.01.032
53. Li J, Wang X, Qu W, Wang J, Jiang SW. Comparison of serum human epididymis protein 4 and CA125 on endometrial cancer detection: A meta-analysis. Clin Chim Acta (2019) 488:215–20. doi: 10.1016/j.cca.2018.11.011
54. Bi Q, Chen Y, Wu K, Wang J, Zhao Y, Wang B, et al. The diagnostic value of MRI for preoperative staging in patients with endometrial cancer: A meta-analysis. Acad Radiol (2020) 27(7):960–8. doi: 10.1016/j.acra.2019.09.018
55. Hu J, Zhang K, Yan Y, Zang Y, Wang Y, Xue F. Diagnostic accuracy of preoperative (18)F-FDG PET or PET/CT in detecting pelvic and para-aortic lymph node metastasis in patients with endometrial cancer: a systematic review and meta-analysis. Arch Gynecol Obstet (2019) 300(3):519–29. doi: 10.1007/s00404-019-05207-8
56. Wang LJ, Tseng YJ, Wee NK, Low JJH, Tan CH. Diffusion-weighted imaging versus dynamic contrast-enhanced imaging for pre-operative diagnosis of deep myometrial invasion in endometrial cancer: A meta-analysis. Clin Imaging (2021) 80:36–42. doi: 10.1016/j.clinimag.2021.06.027
57. Lin G, Huang YT, Chao A, Lin YC, Yang LY, Wu RC, et al. Endometrial cancer with cervical stromal invasion: diagnostic accuracy of diffusion-weighted and dynamic contrast enhanced MR imaging at 3T. Eur Radiol (2017) 27(5):1867–76. doi: 10.1007/s00330-016-4583-0
58. Spagnol G, Noventa M, Bonaldo G, Marchetti M, Vitagliano A, Laganà AS, et al. Three-dimensional transvaginal ultrasound vs magnetic resonance imaging for preoperative staging of deep myometrial and cervical invasion in patients with endometrial cancer: systematic review and meta-analysis. Ultrasound Obstet Gynecol (2022) 60(5):604–11. doi: 10.1002/uog.24967
59. Di Donato V, Kontopantelis E, Cuccu I, Sgamba L, Golia D'Augè T, Pernazza A, et al. Magnetic resonance imaging-radiomics in endometrial cancer: a systematic review and meta-analysis. Int J Gynecol Cancer (2023) 33(7):1070–6. doi: 10.1136/ijgc-2023-004313
60. Reijnen C, IntHout J, Massuger L, Strobbe F, Küsters-Vandevelde HVN, Haldorsen IS, et al. Diagnostic accuracy of clinical biomarkers for preoperative prediction of lymph node metastasis in endometrial carcinoma: A systematic review and meta-analysis. Oncologist (2019) 24(9):e880–90. doi: 10.1634/theoncologist.2019-0117
61. Gunning D, Stefik M, Choi J, Miller T, Stumpf S, Yang GZ. XAI-Explainable artificial intelligence. Sci Robot (2019) 4(37):7120–2. doi: 10.1126/scirobotics.aay7120
62. Lecointre L, Dana J, Lodi M, Akladios C, Gallix B. Artificial intelligence-based radiomics models in endometrial cancer: A systematic review. Eur J Surg Oncol (2021) 47(11):2734–41. doi: 10.1016/j.ejso.2021.06.023
63. Chóliz M. Crisis, what crisis? The effect of economic crises on spending on online and offline gambling in Spain: implications for preventing gambling disorder. Int J Environ Res Public Health (2023) 20(4):2909–23. doi: 10.3390/ijerph20042909
64. Chen Q, Zhang L, Mo X, You J, Chen L, Fang J, et al. Current status and quality of radiomic studies for predicting immunotherapy response and outcome in patients with non-small cell lung cancer: a systematic review and meta-analysis. Eur J Nucl Med Mol Imaging (2021) 49(1):345–60. doi: 10.1007/s00259-021-05509-7
Keywords: radiomics, endometrial cancer, endometrial malignancy, lymph node metastasis, systematic review
Citation: He J, Liu Y, Li J and Liu S (2024) Accuracy of radiomics in the diagnosis and preoperative high-risk assessment of endometrial cancer: a systematic review and meta-analysis. Front. Oncol. 14:1334546. doi: 10.3389/fonc.2024.1334546
Received: 07 November 2023; Accepted: 02 January 2024;
Published: 25 January 2024.
Edited by:
Mohamed Otify, Liverpool Women’s Hospital, United KingdomReviewed by:
Dilyara Kaidarova, Kazakh Institute of Oncology and Radiology, KazakhstanJoao Siufi Neto, Beneficência Portuguesa de São Paulo, Brazil
Copyright © 2024 He, Liu, Li and Liu. This is an open-access article distributed under the terms of the Creative Commons Attribution License (CC BY). The use, distribution or reproduction in other forums is permitted, provided the original author(s) and the copyright owner(s) are credited and that the original publication in this journal is cited, in accordance with accepted academic practice. No use, distribution or reproduction is permitted which does not comply with these terms.
*Correspondence: Shuang Liu, 2645514730@qq.com
†These authors have contributed equally to this work