- 1Department of Magnetic Resonance Imaging, the First Affiliated Hospital of Zhengzhou University, Zhengzhou, China
- 2Jiangsu Key Laboratory of Medical Optics, Suzhou Institute of Biomedical Engineering and Technology, Chinese Academy of Sciences, Suzhou, China
Objectives: To investigate the clinical value of hemodynamic parameters derived from dynamic contrast-enhanced MRI (DCE-MRI) in predicting glioma genotypes including isocitrate dehydrogenase (IDH) mutation, 1p/19q codeletion status and the tumor proliferation index (Ki-67) noninvasively. And to compare the diagnostic performance of parameters of distributed parameter (DP)model and extended Tofts (Ex-Tofts) model.
Materials and methods: Dynamic contrast-enhanced MRI (DCE-MRI) data of patients with glioma were prospectively enrolled from April 2021 to May 2023. The imaging data were analyzed using DP and Ex-Tofts model for evaluating the perfusion and permeability characteristics of glioma. Comparisons were performed according to IDH genotype in all glioma patients and 1p/19q codeletion in IDH mutation glioma patients. Receiver operating characteristic (ROC) curves were generated for DCE-MRI parameters. The Spearman rank correlation coefficients were calculated between DCE MRI parameters and Ki-67 index.
Results: In IDH-mutation gliomas, a higher blood flow (F) was found in 1p/19q codeletion gliomas than in 1p/19q intact gliomas. No parameter derived from Ex-Tofts model showed significant differences in predicting 1p/19q status. Fractional volume of interstitial space (Ve) derived from both the DP and Ex-Tofts models exhibited optimal performance in predicting IDH genotype (AUC = 0.818, 0.828, respectively). Ve also showed the highest correlations with Ki-67 LI within their respective models in all gliomas (ρ = 0.62, 0.61), indicating comparable moderate positive associations. Ki-67
Conclusion: DP model showed a clear advantage in predicting 1p/19q status compared to Ex-Tofts model. The DP and Ex-Tofts models performed similarly in predicting IDH mutation and Ki-67 index.
1 Introduction
Gliomas, being the most commonly occurring primary malignant brain tumors in adults (1), are classified by the 2021 version of the World Health Organization (WHO) into three groups based on two critical molecular markers: the isocitrate dehydrogenase (IDH) genotype and 1p/19q codeletion status. The groups include IDH wild-type, IDH mutation with 1p/19q intact, and IDH mutation with 1p/19q codeletion (2). This new classification system applies to the glioma subtype, thus establishing a link between the grade of glioma and not just its natural disease progression but also the impact of clinical treatment on the course and prognosis of the disease. Ki-67, a nuclear antigen involved in cellular proliferation, represents a valuable biomarker for the evaluation of cell proliferation. An elevation in Ki-67 labeling index (LI) indicates augmented tumor proliferation, which in turn correlates with inferior prognosis among glioma patients (3). Studies have demonstrated that certain genetic factors, including IDH mutation, 1p/19q codeletion, and o6-methylguanine-DNA-methyltransferase (MGMT) promoter methylation, can predict treatment response, particularly in the context of chemotherapy (4, 5). Moreover, in recent years, additional treatment modalities, such as targeted therapy and radioimmunotherapy, have emerged and are currently under investigation in clinical trials (6, 7). These innovative approaches rely on the identification of specific molecular targets within glioma cells, highlighting the significance of genetic molecular diagnosis in guiding treatment decisions and identifying suitable targets for these therapies.
Therefore, the histological diagnosis and gene molecular diagnosis of glioma play a pivotal role in developing personalized preoperative treatment strategies, and have substantial implications in improving patients’ quality of life and prognosis. Currently, histopathological analysis based on resection or biopsy is considered the most reliable means for molecular diagnosis of glioma genes (8). However, it is characterized by its high cost, demanding expertise, and the risk of sampling errors (9). Particularly in patients unsuitable for surgery, obtaining necessary pathological information without increasing patient burden and risk can maximize their benefits. Against this backdrop, many radiologists are actively exploring the relationship between imaging techniques and molecular biomarkers, aiming to predict molecular information non-invasively (10).
Dynamic contrast-enhanced magnetic resonance imaging (DCE-MRI) is a technique employed to assess blood-brain barrier (BBB) disruption and neovascularization in gliomas. These characteristics offer essential insights into the tumor microenvironment and metabolic properties of various glioma subtypes (11). Several recent reviews (12–14) have collectively concluded that while DCE imaging exhibits promising clinical application prospects in predicting IDH status, it lacks satisfactory performance in identifying 1p/19q codeletion, and further research is still needed to investigate the use of DCE imaging in predicting 1p/19q status. In DCE-MRI, mathematical models are employed to estimate pharmacokinetic parameters that provide insights into the perfusion and permeability of lesions. The accurate characterization of these parameters relies on an appropriate mathematical model. Presently, the extended Tofts (Ex-Tofts) model is widely used in DCE-MRI due to its relatively relaxed requirements for equipment and scan duration (15). However, the main parameter, transfer constant (Ktrans), in Ex-Tofts model does not accurately reflect vascular permeability since it does not differentiate between the intravascular transport of tracer molecules and the exchange process of tracer molecules between the intravascular and interstitial spaces (16). As technology and equipment continue to advance, the distributed parameter (DP) model was proposed to addresses such limitation by separately considering the intravascular transport and the exchange between the intravascular and interstitial compartments (17). DP model incorporates two key parameters: blood flow (F), which characterizes intravascular transport, and the permeability-surface area product (PS), which describes the exchange process.
In this study, our objective was to evaluate the potential of DCE-MRI using the DP model in predicting the IDH genotype, chromosome 1p/19q codeletion status, and Ki-67 LI in adult diffuse gliomas, and to assess whether the DP model offers advantages in the molecular diagnosis of glioma, which may enhance their clinical management.
2 Materials and methods
This retrospective study was approved by our hospital’s institutional review board, and informed consent was waived.
2.1 Study participants
Patients with glioma who underwent DCE examination between April 2021 and May 2023 were retrospectively collected. The inclusion criteria were as follows: DCE-MRI performed within two weeks prior to surgery and before the initiation of antitumor therapy, and a diagnosis of gliomas of grade 2-4 based on the 2021 WHO guideline on brain tumor classification following tumor resection and pathology examination. The exclusion criteria were: a diagnosis of WHO grade 1 glioma; inadequate MRI quality. The IDH1/2 mutations in the hotspot codons R132 and R172 on the excised surgical specimens were determined by Sanger sequencing or immunohistochemical staining. A mutation in any one of them was diagnosed as an IDH mutation. The 1p/19q deletions were detected through fluorescence in situ hybridization analysis. The Ki-67 labeling index was determined by using immunohistochemistry.
2.2 MR imaging acquisition
All scans were conducted using a 3.0 T MRI scanner from Siemens Healthcare (Magnetom Prisma). The DCE scan employed an axial fast-spoiled gradient (SPGR) echo sequence. This sequence included a pre-contrast and a post-contrast phase with the following parameters: TR/TE (3.03 ms/1.06 ms), FOV (230 × 230mm2), matrix (192×134.4), slice thickness (5 mm), flip angles for the pre-contrast scan (3°, 6°, and 9°), and for the post-contrast scan (9°). For each flip angle, ten dynamic pre-contrast scans were acquired, while the post-contrast sequence consisted of 180 dynamic scans, with a temporal resolution of 2 seconds. The contrast agent used was Gadovist (Magnevist; Bayer Schering Pharma AG), administered at an injection rate of 3.5 mL/sec (followed by a 20 mL normal saline flush), with a dose of 0.1 mmol/kg body weight.
2.3 Image processing
DCE images were processed using a commercially software (MItalytics, FITPU Healthcare, Singapore). Two experienced neuroradiologists (K.Z. and X.M., with 3 and 11 years of experience, respectively) manually delineated the tumor region of interest (ROI) in reference to the late-phase dynamic T1-enhanced image (with obvious enhanced lesions) or the T2-FLAIR sequence images (without obvious enhanced lesions). The delineation includes the solid components of the tumor and avoids areas of necrosis, hemorrhage, calcification, large vessels, and cystic regions. Voxels in ROI were aggregated, and the median values of following kinetic parameters were calculated for each patient: Ex-Tofts model derived transfer constant Ktrans (min−1), fractional volume of extravascular extracellular space Ve (mL/100 mL), plasma fractional volume Vp (mL/100 mL), efflux rate constant Kep (min−1). DP model derived blood flow F (mL/min/100 mL), permeability-surface area product PS (mL/min/100 mL), extraction ratio of first pass E (%), Ve and Vp (same as in the Ex-Tofts model). To ensure completeness, the operational equations of these models, which specify the relationship between tissue tracer concentration Ctiss(t) (as a function of time t) and AIF as well as relevant physiological parameters, are presented below:
Ex-Tofts model:
DP model:
2.4 Statistical analysis
Statistical analysis was performed using R software (version 4.3.1; https://www.R-project.org/). Normality of data and homogeneity of variance were assessed using Shapiro-Wilk and Levene’s tests, respectively. Differences in parameters and mean age were evaluated between IDH-mutation and IDH-wild-type gliomas, as well as IDH mutation&1p/19q intact and IDH mutation&1p/19q codeletion gliomas using independent t-test or Mann–Whitney U test according to the results of test for normality and homoscedasticity. Benjamini-Hochberg correction was applied to adjust the P values of DCE parameters for multiple comparisons. The receiver operating characteristic (ROC) curves were utilized for assessing the performance of kinetic parameters in predicting IDH mutation and 1p/19q status. The diagnostic performance was quantified using the area under the ROC curve (AUC). The DeLong test was conducted to compare the diagnostic performance of the Ex-Tofts model and the DP model by comparing their respective parameters with the largest AUC values in each model. The method of Youden index was utilized to determine the optimal threshold for classification and compute the corresponding sensitivity, specificity, and accuracy. Relationship between Ex-Tofts parameters, DP parameters and Ki-67 LI was assessed using the Spearman correlation test. Statistical significance was set at P< 0.05.
3 Results
3.1 Patient characteristics
48 glioma patients were finally included in the study. Table 1 summarizes the clinical, demographic, and pathological characteristics of the patients. Based on the 2021 WHO classification of CNS tumors, the tumors were classified into IDH-mutation and 1p/19q intact glioma (WHO grade 2 astrocytoma, n=3; WHO grade 3 astrocytoma, n=3; WHO grade 4 astrocytoma, n=3), IDH-mutation and 1p/19q codeletion glioma (WHO grade 2 oligodendroglioma, n=7; WHO grade 3 oligodendroglioma, n=8), and IDH-wild-type glioma (WHO grade 4 glioblastoma, n=24). Patients with IDH wild-type glioma were found to be older than those with IDH-mutation glioma. There was no significant difference between glioma subtypes in terms of sex distribution.
3.2 Kinetic parameters in identification of molecular subtypes
As the distribution of all data did not meet the criteria for normality according to the Shapiro-Wilk test at a significance level of 5%, the Mann-Whitney U test was used to assess the differences between parameters. Kep derived from Ex-Tofts model was found significantly higher in IDH mutation gliomas than in IDH wild-type gliomas. Ve, Vp derived from Ex-Tofts model and Ve, Vp, PS, E derived from DP model were found significantly lower in IDH mutation gliomas compared to IDH wild-type gliomas (Table 2). Only the F derived from DP model exhibited a significant difference between 1p/19q codeleted glioma and 1p/19q intact glioma, and the 1p/19q codeleted glioma had a higher F value compared to the 1p/19q intact glioma. No parameters in Ex-Tofts showed significant differences in predicting 1p/19q status (Table 3). Representative cases of three different subtypes glioma are shown in Figure 1. Figure 2 shows the boxplots of Ex-Tofts and DP parameters, illustrating the intergroup differences in the distribution of kinetic parameters.
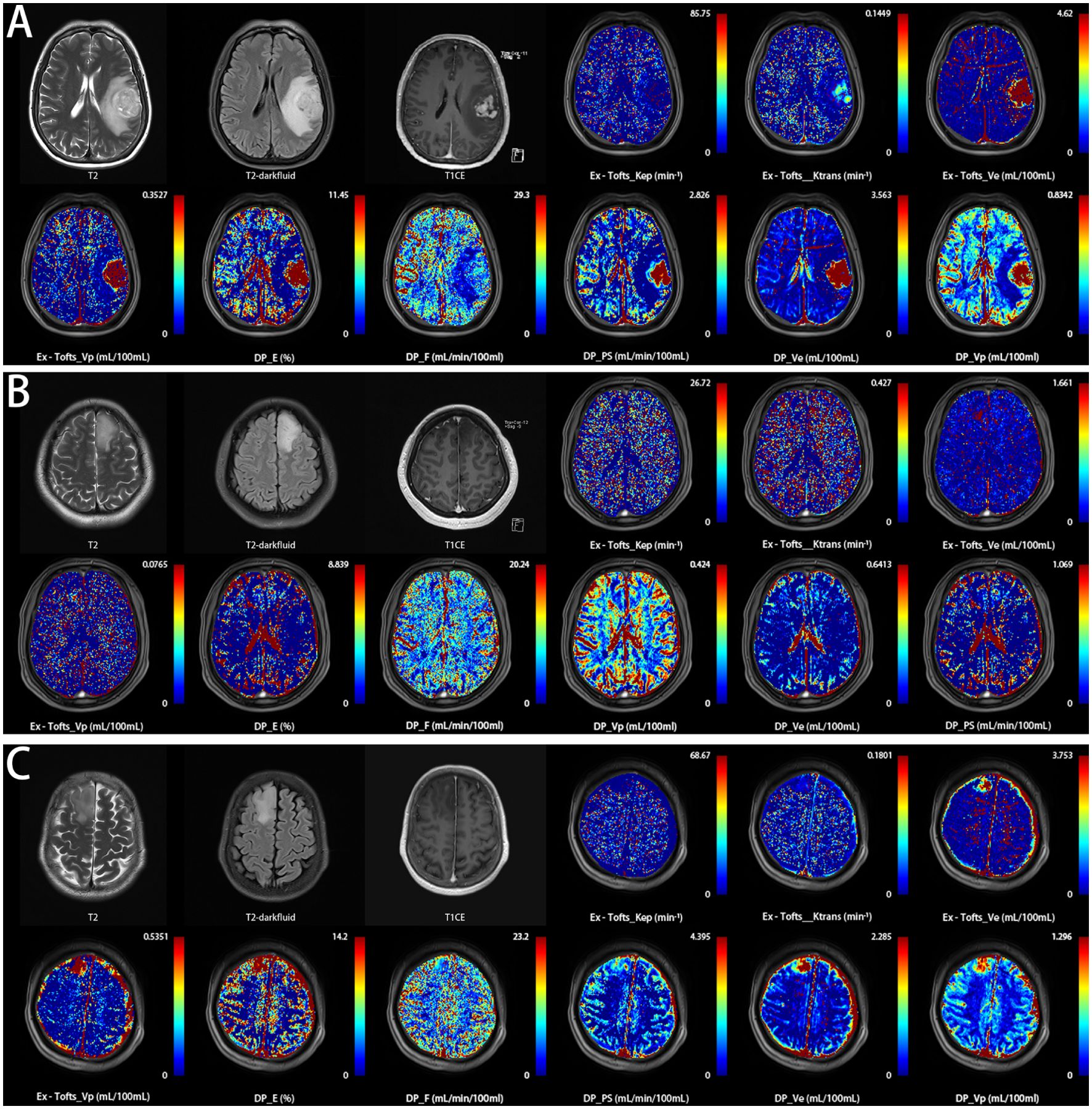
Figure 1. Three representative patients with glioma were correctly classified into their respective subtypes based on the threshold values of DCE parameters in this study, using pathological examination results as the gold standard. (A) a 59-year-old female with histologically proven glioblastoma IDH wild-type (Ex-Tofts_Ve = 16.08; DP_F = 9.21). (B) a 46-year-old male with histologically proven astrocytoma IDH mutation&1p/19q intact (Ex-Tofts_Ve = 0.08; DP_F = 7.00). (C) a 47-year-old female with histologically proven oligodendroglioma IDH mutation&1p/19q codeleted (Ex-Tofts_Ve = 1.34; DP_F = 8.82).
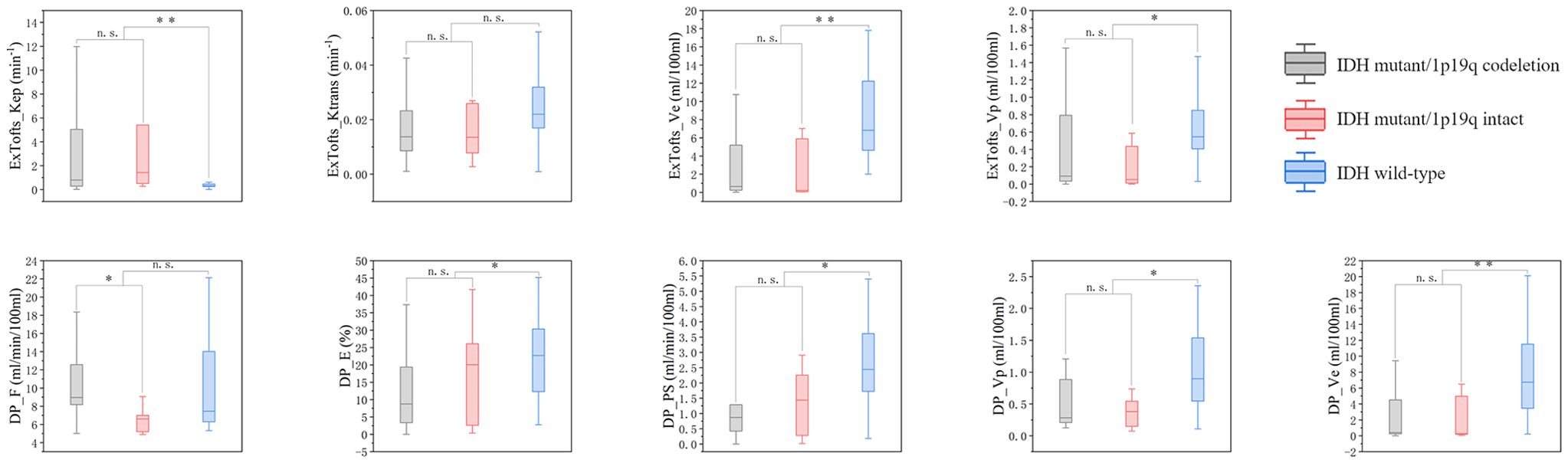
Figure 2. Boxplots of kinetic parameters in differentiating three types of gliomas, n.s. stands for not significant, *P< 0.05, **P< 0.01, ***P< 0.001.
3.3 ROC curve analysis
Tables 4 and 5 respectively summarizes the results of ROC curve analysis in differentiating IDH mutation (mutation vs. wild-type) and 1p/19q codeletion status in IDH mutation glioma (intact vs. codeleted). Ve attained the best performance in discriminating IDH-mutation from IDH-wild-type gliomas in both Ex-Tofts and DP model (AUC = 0.828 and 0.818, respectively). Delong test showed no significant difference between the AUCs of above two parameters (z = 0.509, P = 0.611). Among DP-derived parameters, F showed a good performance in predicting 1p/19q status with AUC = 0.793. The plots of ROC curves are shown in Figure 3.
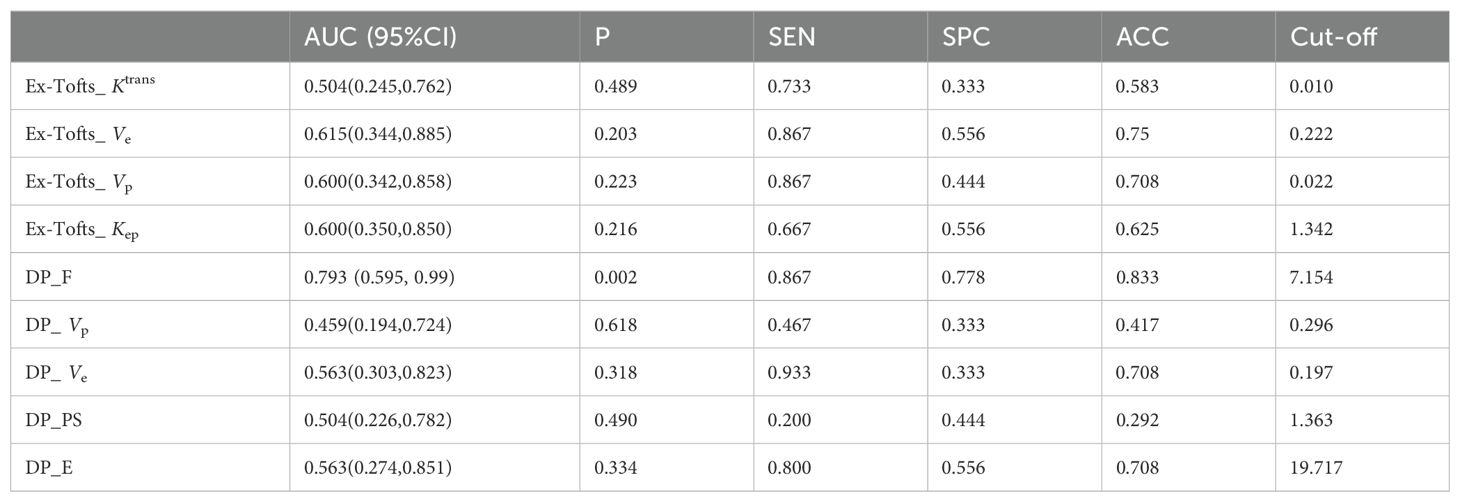
Table 5. ROC Analysis of kinetic parameters with significant difference in predicting 1p/19q status.
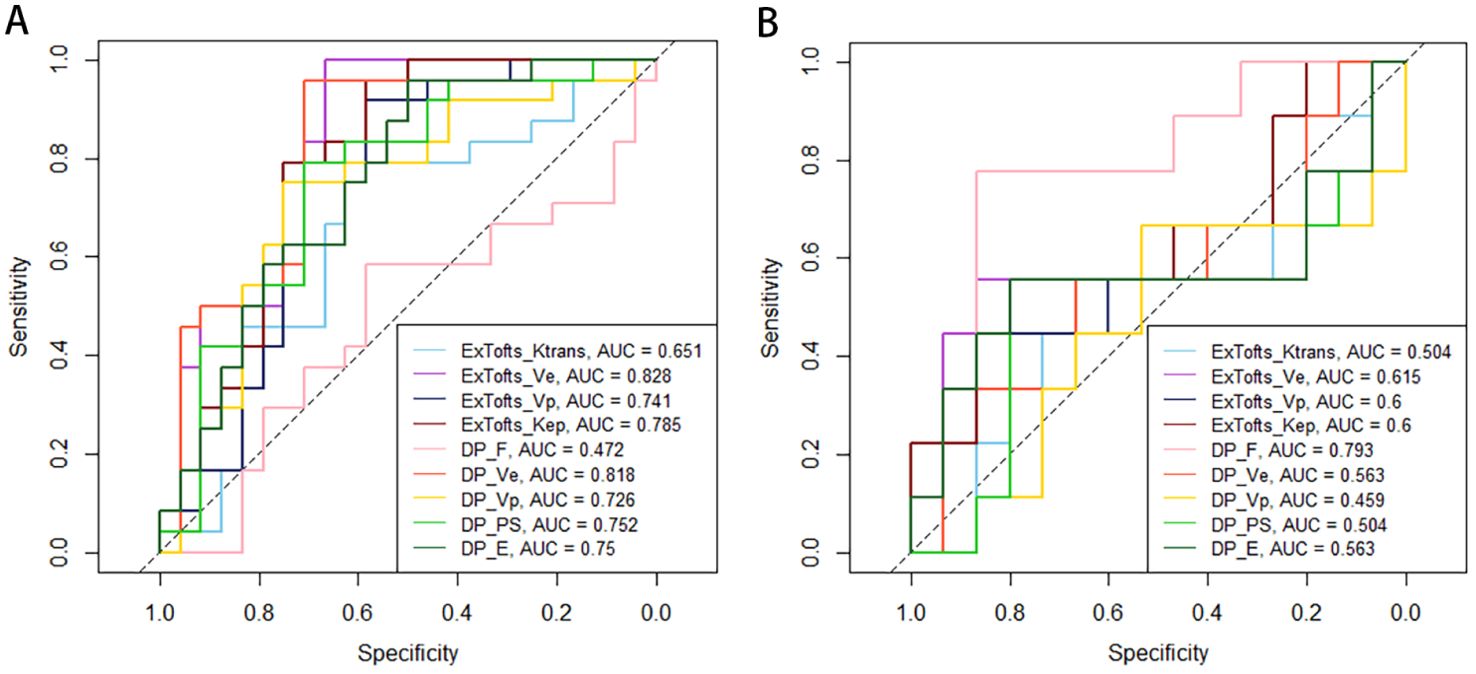
Figure 3. Receiver operating characteristic (ROC) plots and areas under ROC curve (AUCs) of Ex-Tofts and DP model parameters in differentiating of IDH mutation status (A) and 1p/19q codeletion status (B).
3.4 Correlation of kinetic parameters with the Ki−67 LI
The correlation results between the DCE parameters and Ki-67 LI are shown in Figure 4. The corresponding P values are shown in the supplementary materials. Ve derived from DP model and the Ex-Tofts model was correlated best with Ki-67 LI within their respective models in all gliomas with similar moderate positive correlations (ρ = 0.62, 0.61).
4 Discussion
This study aimed to investigate the potential of pharmacokinetic parameters derived from the Ex-Tofts model and the DP model as biomarkers for identifying IDH mutation, 1p/19q codeletion status, and tumor cell proliferation (Ki-67 LI) in gliomas. The results of this study revealed that there was no significant difference in the diagnostic efficacy between the two models for predicting IDH mutation status and Ki-67 expression. In predicting the 1p/19q status, the DP model demonstrated a substantial increase in the parameter F and exhibited favorable diagnostic performance (AUC = 0.793), while the Ex-Tofts model did not effectively predict the 1p/19q status. This suggests that the DP model holds greater potential than the Ex-Tofts model in predicting the 1p/19q status with the exclusive perfusion parameter F.
The measurement of F in predicting the 1p/19q status was made possible by the DP model, which separately describe intravascular perfusion and exchange between the intravascular and extravascular spaces. These processes are characterized by two distinct parameters, namely F and PS. Conversely, the Ex-Tofts model combines these two processes into a single parameter, Ktrans (15). The use of appropriate pharmacokinetic models is crucial for the analysis of DCE-MRI data. Developing advanced pharmacokinetic models may be an important avenue to address the limitations of DCE in predicting 1p/19q status. Higher F values observed in 2021 WHO oligodendrogliomas compared to astrocytomas may be related to their higher perfusion characteristics (18). An arterial spin labeling (ASL) study (19) has revealed that the cerebral blood flow (CBF) is significantly higher in oligodendrogliomas than astrocytomas, attributed to higher vascular density and gray matter involvement in oligodendrogliomas. Although CBF in ASL and F in DCE are not completely comparable, changes in this hemodynamic parameter indicate that the high perfusion characteristics of oligodendrogliomas can be used to predict the 1p/19q status, which corroborates our results. Another study (20) as also highlighted the higher perfusion characteristics of oligodendrogliomas compared to astrocytomas, utilizing dynamic susceptibility contrast-enhanced (DSC) MRI. This study indicated that oligodendrogliomas revealed significantly higher cerebral blood volume (CBV) when compared to astrocytomas. In DCE, the parameter Vp exhibits physiological similarity to CBV. Vp is a perfusion parameter that measures the fractional volume of the intravascular space and may be correlated with tissue microvascular density. Correlation analysis demonstrated that there was a relatively weak positive correlation between Vp and F (ρ = 0.56). This indicates that while both parameters represent tissue perfusion, they also possess a certain degree of independence from each other, suggesting that they characterize different aspects of tumor perfusion. Our results failed to found any significant difference in Vp between astrocytomas and oligodendrogliomas, which is consistent with Gupta’s (21) conclusion. However, Lee et al. (22) have found a significant increase in Vp in oligodendrogliomas. Currently, there is limited literature on the use of perfusion imaging for identifying 1p/19q codeletion status in gliomas, and most studies focus on DSC-MRI (12). The role of DCE in predicting 1p/19q codeletion status remains controversial, and selecting appropriate pharmacokinetic models may be crucial for improving its clinical utility. Our study suggested one of the limitations of the Ex-Tofts model in characterizing perfusion is its inability to describe tissue blood flow velocity, thus necessitating the development of advanced pharmacokinetic models that factor in the transport of contrast agent molecules within the vasculature.
In predicting the IDH genotype, both Ex-Tofts and DP models have existing research (23, 24), and our findings regarding the comparison of parameter magnitudes align with previous studies. We identified Ve as the most distinguishing feature in discriminating between IDH-mutation and IDH-wild-type gliomas. Ve refers to the fractional volume of the extravascular extracellular space. As tumor cells proliferate excessively, the interstitial space decreases, resulting in a smaller Ve. Compared to IDH wild-type, IDH mutation could inhibit proliferation in glioma (25). However, unlike other solid tumors (16), a decrease in Ve suggests elevated vessel permeability rather than higher cell proliferation. The blood-brain barrier restricts the leakage of contrast agent molecules from the vasculature, leading to smaller measured Ve values. In IDH wild-type gliomas, we observed a significant increase in Ve, indicating a greater tendency for contrast agent molecules to leak out. This can be attributed to the presence of newly formed immature blood vessels in IDH wild-type gliomas, along with the irregular arrangement of endothelial cells and detachment of pericytes and astrocytes from microvascular walls (26), which increase the permeability of the blood-brain barrier and promote microvascular leakage. Conversely, IDH-mutation gliomas have been shown to exhibit decreased activation of hypoxia-inducible factor 1α (HIF-1α), leading to a reduction in hypoxia-induced angiogenesis (27). DCE-MRI can indirectly predict these genetic alterations by describing changes in tissue permeability.
Ki-67 LI showed the highest correlation coefficient with Ve of DP model among the DCE parameters with a moderate positive correlation observed (ρ = 0.62). The positive correlation between Ve and Ki-67 may be related to the compromised integrity of the blood-brain barrier. The elevated proliferative activity of tumor cells requires a substantial amount of energy to sustain their rapid growth and division. In response to this increased energy demand, tumors activate various adaptive mechanisms, including the upregulation of HIF-1α, leading to an increase in tumor angiogenesis and a more abundant tumor microcirculation (28). The presence of newly formed and immature blood vessels increases tumor vascular permeability, facilitating the extravasation of contrast agents and subsequently resulting in elevated Ve values. This finding is consistent with Jiang et al. (29). However, we were unable to confirm a significant correlation between Ktrans and Ki-67, as they did. This discrepancy may be due to the fact that Jiang et al. measured the maximum values of tumor hemodynamic parameters, while we focused on the median values within the ROI. In future studies, we may consider employing histogram analysis of DCE data to further explore this correlation.
Several limitations should be acknowledged in our study. Firstly, the sample size was relatively small, potentially introducing chance correlations when predicting 1p/19q status, and the single-center design mean that the thresholds we identified may not be generalizable to other centers, limiting their applicability. Therefore, a prospective study with a larger sample size and multi-center is warranted to validate these findings. Secondly, the ROI delineation in our study was manually performed, and the adoption of machine learning algorithms for automated delineation holds promise in improving the objectivity of our research. Lastly, due to the update of the 2021 WHO CNS glioma classification, glioma grading is now categorized within pathological subtypes. The sample size in our study cohort was insufficient to conduct predictive research on glioma grading. We plan to further expand the sample size to explore the role of various DCE models in predicting glioma grading in future research.
5 Conclusion
DP model provided additional information on blood flow rate compared to the Ex-Tofts model, and it demonstrated a clear advantage in predicting 1p/19q status. However, it did not show a significant difference in predicting IDH and Ki-67 compared to the Ex-Tofts model.
Data availability statement
The original contributions presented in the study are included in the article/supplementary material. Further inquiries can be addressed to the corresponding author.
Ethics statement
The studies involving humans were approved by Ethics Committee of the First Affiliated Hospital of Zhengzhou University. The studies were conducted in accordance with the local legislation and institutional requirements. The participants provided their written informed consent to participate in this study.
Author contributions
KZ: Writing – original draft, Writing – review & editing. HH: Writing – original draft. EG: Writing – original draft. JQ: Writing – original draft. TC: Writing – original draft. GYZ: Writing – original draft. GZ: Writing – original draft. YuZ: Writing – original draft. PW: Writing – original draft. JB: Writing – review & editing. YoZ: Writing – review & editing. ZH: Writing – review & editing. JC: Writing – review & editing. XM: Writing – review & editing.
Funding
The author(s) declare financial support was received for the research, authorship, and/or publication of this article. This study has received funding from the Youth Project of Henan Medical Science and Technology Research Project (grant numbers SBGJ202103078).
Conflict of interest
The authors declare that the research was conducted in the absence of any commercial or financial relationships that could be construed as a potential conflict of interest.
Publisher’s note
All claims expressed in this article are solely those of the authors and do not necessarily represent those of their affiliated organizations, or those of the publisher, the editors and the reviewers. Any product that may be evaluated in this article, or claim that may be made by its manufacturer, is not guaranteed or endorsed by the publisher.
Supplementary material
The Supplementary Material for this article can be found online at: https://www.frontiersin.org/articles/10.3389/fonc.2024.1333798/full#supplementary-material
References
1. Weller M, van den Bent M, Preusser M, Le Rhun E, Tonn JC, Minniti G, et al. EANO guidelines on the diagnosis and treatment of diffuse gliomas of adulthood. Nat Rev Clin Oncol. (2021) 18:170–86. doi: 10.1038/s41571-020-00447-z
2. Louis DN, Perry A, Wesseling P, Brat DJ, Cree IA, Figarella-Branger D, et al. The 2021 WHO classification of tumors of the central nervous system: a summary. Neuro Oncol. (2021) 23:1231–51. doi: 10.1093/neuonc/noab106
3. Xing Z, Huang W, Su Y, Yang X, Zhou X, Cao D. Non-invasive prediction of p53 and Ki-67 labelling indices and O-6-methylguanine-DNA methyltransferase promoter methylation status in adult patients with isocitrate dehydrogenase wild-type glioblastomas using diffusion-weighted imaging and dynamic susceptibility contrast-enhanced perfusion-weighted imaging combined with conventional MRI. Clin Radiol. (2022) 77:e576–e84. doi: 10.1016/j.crad.2022.03.015
4. Weller M, Tabatabai G, Kastner B, Felsberg J, Steinbach JP, Wick A, et al. MGMT promoter methylation is a strong prognostic biomarker for benefit from dose-intensified temozolomide rechallenge in progressive glioblastoma: the DIRECTOR trial. Clin Cancer Res. (2015) 21:2057–64. doi: 10.1158/1078-0432.CCR-14-2737
5. Cairncross G, Wang M, Shaw E, Jenkins R, Brachman D, Buckner J, et al. Phase III trial of chemoradiotherapy for anaplastic oligodendroglioma: long-term results of RTOG 9402. J Clin Oncol. (2013) 31:337–43. doi: 10.1200/JCO.2012.43.2674
6. Mellinghoff IK, Ellingson BM, Touat M, Maher E, de la Fuente MI, Holdhoff M, et al. Ivosidenib in isocitrate dehydrogenase 1-mutated advanced glioma. J Clin Oncol. (2020) 38:3398–406. doi: 10.1200/JCO.19.03327
7. Karpel-Massler G, Nguyen TTT, Shang E, Siegelin MD. Novel IDH1-targeted glioma therapies. CNS Drugs. (2019) 33:1155–66. doi: 10.1007/s40263-019-00684-6
8. Tanboon J, Williams EA, Louis DN. The diagnostic use of immunohistochemical surrogates for signature molecular genetic alterations in gliomas. J Neuropathol Exp Neurol. (2016) 75:4–18. doi: 10.1093/jnen/nlv009
9. Jackson RJ, Fuller GN, Abi-Said D, Lang FF, Gokaslan ZL, Shi WM, et al. Limitations of stereotactic biopsy in the initial management of gliomas. Neuro Oncol. (2001) 3:193–200. doi: 10.1093/neuonc/3.3.193
10. Lu J, Li X, Li H. Perfusion parameters derived from MRI for preoperative prediction of IDH mutation and MGMT promoter methylation status in glioblastomas. Magn Reson Imaging. (2021) 83:189–95. doi: 10.1016/j.mri.2021.09.005
11. Arzanforoosh F, van der Voort SR, Incekara F, Vincent A, Van den Bent M, Kros JM, et al. Microvasculature features derived from hybrid EPI MRI in non-enhancing adult-type diffuse glioma subtypes. Cancers (Basel). (2023) 15(7):2135. doi: 10.3390/cancers15072135
12. Siakallis L, Topriceanu CC, Panovska-Griffiths J, Bisdas S. The role of DSC MR perfusion in predicting IDH mutation and 1p19q codeletion status in gliomas: meta-analysis and technical considerations. Neuroradiology. (2023) 65:1111–26. doi: 10.1007/s00234-023-03154-5
13. Stumpo V, Guida L, Bellomo J, Van Niftrik CHB, Sebok M, Berhouma M, et al. Hemodynamic imaging in cerebral diffuse glioma-part B: molecular correlates, treatment effect monitoring, prognosis, and future directions. Cancers (Basel). (2022) 14(5):1342. doi: 10.3390/cancers14051342
14. van Santwijk L, Kouwenberg V, Meijer F, Smits M, Henssen D. A systematic review and meta-analysis on the differentiation of glioma grade and mutational status by use of perfusion-based magnetic resonance imaging. Insights Imaging. (2022) 13:102. doi: 10.1186/s13244-022-01230-7
15. Sourbron SP, Buckley DL. Classic models for dynamic contrast-enhanced MRI. NMR Biomed. (2013) 26:1004–27. doi: 10.1002/nbm.2940
16. Wang X, Li S, Lin X, Lu Y, Mao C, Ye Z, et al. Evaluation of tracer kinetic parameters in cervical cancer using dynamic contrast-enhanced MRI as biomarkers in terms of biological relevance, diagnostic performance and inter-center variability. Front Oncol. (2022) 12:958219. doi: 10.3389/fonc.2022.958219
17. Koh TS, Cheong LH, Tan CK, Lim CC. A distributed parameter model of cerebral blood-tissue exchange with account of capillary transit time distribution. Neuroimage. (2006) 30:426–35. doi: 10.1016/j.neuroimage.2005.09.032
18. Yamashita K, Togao O, Kikuchi K, Kuga D, Sangatsuda Y, Fujioka Y, et al. The cortical high-flow sign of oligodendroglioma, IDH-mutant and 1p/19q-codeleted: comparison between arterial spin labeling and dynamic susceptibility contrast methods. Neuroradiology. (2024) 66:187–92. doi: 10.1007/s00234-023-03267-x
19. Brendle C, Hempel JM, Schittenhelm J, Skardelly M, Tabatabai G, Bender B, et al. Glioma grading and determination of IDH mutation status and ATRX loss by DCE and ASL perfusion. Clin Neuroradiol. (2018) 28:421–8. doi: 10.1007/s00062-017-0590-z
20. Latysheva A, Emblem KE, Brandal P, Vik-Mo EO, Pahnke J, Roysland K, et al. Dynamic susceptibility contrast and diffusion MR imaging identify oligodendroglioma as defined by the 2016 WHO classification for brain tumors: histogram analysis approach. Neuroradiology. (2019) 61:545–55. doi: 10.1007/s00234-019-02173-5
21. Gupta M, Gupta A, Yadav V, Parvaze SP, Singh A, Saini J, et al. Comparative evaluation of intracranial oligodendroglioma and astrocytoma of similar grades using conventional and T1-weighted DCE-MRI. Neuroradiology. (2021) 63:1227–39. doi: 10.1007/s00234-021-02636-8
22. Lee JY, Ahn KJ, Lee YS, Jang JH, Jung SL, Kim BS. Differentiation of grade II and III oligodendrogliomas from grade II and III astrocytomas: a histogram analysis of perfusion parameters derived from dynamic contrast-enhanced (DCE) and dynamic susceptibility contrast (DSC) MRI. Acta Radiol. (2018) 59:723–31. doi: 10.1177/0284185117728981
23. Li Z, Zhao W, He B, Koh TS, Li Y, Zeng Y, et al. Application of distributed parameter model to assessment of glioma IDH mutation status by dynamic contrast-enhanced magnetic resonance imaging. Contrast Media Mol Imaging. (2020) 2020:8843084. doi: 10.1155/2020/8843084
24. Zhang HW, Lyu GW, He WJ, Lei Y, Lin F, Wang MZ, et al. DSC and DCE histogram analyses of glioma biomarkers, including IDH, MGMT, and TERT, on differentiation and survival. Acad Radiol. (2020) 27:e263–e71. doi: 10.1016/j.acra.2019.12.010
25. Gao A, Zhang H, Yan X, Wang S, Chen Q, Gao E, et al. Whole-tumor histogram analysis of multiple diffusion metrics for glioma genotyping. Radiology. (2022) 302:652–61. doi: 10.1148/radiol.210820
26. Guo H, Kang H, Tong H, Du X, Liu H, Tan Y, et al. Microvascular characteristics of lower-grade diffuse gliomas: investigating vessel size imaging for differentiating grades and subtypes. Eur Radiol. (2019) 29:1893–902. doi: 10.1007/s00330-018-5738-y
27. Kickingereder P, Sahm F, Radbruch A, Wick W, Heiland S, Deimling A, et al. IDH mutation status is associated with a distinct hypoxia/angiogenesis transcriptome signature which is non-invasively predictable with rCBV imaging in human glioma. Sci Rep. (2015) 5:16238. doi: 10.1038/srep16238
28. Shin JK, Kim JY. Dynamic contrast-enhanced and diffusion-weighted MRI of estrogen receptor-positive invasive breast cancers: Associations between quantitative MR parameters and Ki-67 proliferation status. J Magn Reson Imaging. (2017) 45:94–102. doi: 10.1002/jmri.25348
Keywords: glioma, dynamic contrast-enhanced MRI, distributed parameter model, IDH mutation, 1p/19q codeletion, Ki-67
Citation: Zhao K, Huang H, Gao E, Qi J, Chen T, Zhao G, Zhao G, Zhang Y, Wang P, Bai J, Zhang Y, Hou Z, Cheng J and Ma X (2024) Distributed parameter model of dynamic contrast-enhanced MRI in the identification of IDH mutation, 1p19q codeletion, and tumor cell proliferation in glioma patients. Front. Oncol. 14:1333798. doi: 10.3389/fonc.2024.1333798
Received: 08 January 2024; Accepted: 30 September 2024;
Published: 25 October 2024.
Edited by:
Joubert Banjop Kharlyngdoh, Tulane University, United StatesReviewed by:
Jianping Chu, The First Affiliated Hospital of Sun Yat-sen University, ChinaNghi C. D. Truong, University of Texas Southwestern Medical Center, United States
Copyright © 2024 Zhao, Huang, Gao, Qi, Chen, Zhao, Zhao, Zhang, Wang, Bai, Zhang, Hou, Cheng and Ma. This is an open-access article distributed under the terms of the Creative Commons Attribution License (CC BY). The use, distribution or reproduction in other forums is permitted, provided the original author(s) and the copyright owner(s) are credited and that the original publication in this journal is cited, in accordance with accepted academic practice. No use, distribution or reproduction is permitted which does not comply with these terms.
*Correspondence: Xiaoyue Ma, bWF4aWFveXVlMDgyMkAxNjMuY29t; Jingliang Cheng, ZmNjY2hlbmdqbEB6enUuZWR1LmNu; Zujun Hou, aG91empAc2liZXQuYWMuY24=
†These authors share first authorship