- Department of General Surgery, State Key Laboratory of Complex Severe and Rare Diseases, Peking Union Medical College Hospital, Peking Union Medical College and Chinese Academy of Medical Sciences, Beijing, China
Cholangiocarcinoma, classified as intrahepatic, perihilar, and extrahepatic, is considered a deadly malignancy of the hepatobiliary system. Most cases of cholangiocarcinoma are asymptomatic. Therefore, early detection of cholangiocarcinoma is significant but still challenging. The routine screening of a tumor lacks specificity and accuracy. With the application of AI, high-risk patients can be easily found by analyzing their clinical characteristics, serum biomarkers, and medical images. Moreover, AI can be used to predict the prognosis including recurrence risk and metastasis. Although they have some limitations, AI algorithms will still significantly improve many aspects of cholangiocarcinoma in the medical field with the development of computing power and technology.
Introduction
Cholangiocarcinoma (CCA) is an advanced and lethal malignancy with a rare incidence of the disease (1). It arises from the epithelial cells of the biliary ducts. According to the original anatomical site, cholangiocarcinoma is divided into intrahepatic, perihilar, or extrahepatic (2). The annual incidence is approximately 1.26 cases of cholangiocarcinoma per 100,000 people, and two-thirds of cases are intrahepatic type in the United States (3). The signs and symptoms associated with cholangiocarcinoma are non-specific. The location of the lesion is suggested by the patient’s clinical presentation and the initial radiographic findings (4). Extrahepatic cholangiocarcinoma becomes symptomatic when the biliary drainage system is obstructed, including jaundice, pruritus, and color change of the stools and urine. Intrahepatic cholangiocarcinoma is usually asymptomatic and less possibly presents jaundice (5). Tumor markers, including CEA, CA 19-9, and even the combined parameters of CA 19-9 and CEA, do not have enough specificity and accuracy to detect early-stage cholangiocarcinoma (6–8). Transabdominal ultrasound, as the first appropriate imaging study for patients with jaundice, has a high sensitivity to detect the main reason for the biliary tract dilation and evaluate potential vascular involvement. However, early-stage distal extrahepatic cancers may not be detected due to the limited visualization of the distal common bile duct, especially if the small lesion does not make the bile duct become visibly dilated (9, 10). CT, MRI, and PET/CT are not recommended for asymptomatic populations as routine examinations due to the low incidence of cholangiocarcinoma and the financial cost involved (2, 11–13). ERCP and other invasive procedures including percutaneous cholangiography, brush cytology, and endoscopic ultrasound are also not recommended (14, 15). Only a minority of patients present with early-stage disease and are considered candidates for resection, which is the only possible cure (1, 6, 16). Consequently, early diagnosis of cholangiocarcinoma is still challenging nowadays.
Artificial intelligence (AI) was first proposed by John McCarthy et al. in 1956, where it was widely considered as “thinking machines.” AI is defined as investigating and developing a digital computer or computer-controlled machine to simulate and perform the intellectual process characteristic of humans (17). AI has shown great success in a wide variety of medical studies, including radiology (18, 19), pathology (20), gastroenterology (21), and ophthalmology (22). AI has a great advantage in analyzing vast amounts of data and identifying patterns and trends of disease, such as histologic, cytologic, serum marker, and radiologic methods. In the early diagnosis of cholangiocarcinoma, AI can analyze the input histologic, cytologic, or radiologic images to extract features that are specific for cholangiocarcinoma but not fully recognized by humans, with the type of dimensionality reduction (23). Decision tree algorithms can be trained with serum markers to determine the better cutoff value and workflow for the diagnosis of cholangiocarcinoma. Moreover, AI efficiently automates the repetitive jobs for physicians, achieving more rapid identification of the suspected nodule (17, 24). With the continuous improvement of the computing power of computers, AI is the driving force in the development of new treatments and technologies in the medical field.
However, there are still some disadvantages to the application of AI in the medical field, particularly in building user trust in AI systems (25). Interpretability means that the cause and effect can be determined (26). Nowadays, some AI algorithms function as uninterpretable “black boxes” and are difficult to explain how cholangiocarcinoma is being predicted by the input information. Artificial intelligence itself cannot be the subject of a legal relationship so a framework for determining the responsibilities and legal liability for AI is also needed (27). Furthermore, medical data from different institutions may be varied in quality and complicated in feature labeling (28). It also raises our concerns about data privacy during the application of AI (29). Most AI applications currently approved by the FDA are studied in retrospective studies, while few prospective studies investigated the clinical application of AI algorithms (30).
In this review, “artificial intelligence,” “machine learning,” and “cholangiocarcinoma” were used as the keywords. Relevant literature published until September 2023 in PubMed, Embase, Web of Science, and other databases were reviewed. We highlighted the AI function in serum biomarkers, radiologic analysis, and pathological examination in the tumor diagnosis process. In prognosis prediction, recurrence risk, metastasis, and overall survival of cholangiocarcinoma are investigated by AI. Finally, we discussed the future challenges for cholangiocarcinoma management.
Machine learning
Machine learning (ML) is a branch of artificial intelligence. By making use of data and algorithms, it simulates the way how humans think and execute (31). Machine learning can handle large volumes of data and discover potential trends, especially in radiology. The number of images often exceeds the processing capacity of radiologists, while it can be rapidly studied by ML to localize the cholangiocarcinoma for radiologists (32). Also, it is widely used in the three-dimensional reconstruction of the biliary system from the images so that the area of malignancy can be automatically delineated for surgery and radiotherapy (33, 34).
The performance of an ML model is usually measured by the AUROC, which determines the tradeoff value between sensitivity and specificity (35). Other parameters, including accuracy, C-index, and PPV, are also commonly used to evaluate the predictive result of ML. The higher the values of the parameters are, the more accurate the prediction of cholangiocarcinoma can be achieved.
Supervised learning
Supervised learning is a training algorithm that uses labeled features to classify data and predict outcomes accurately (36). For cholangiocarcinoma, clinical physicians labeled the specific site of malignancy in radiology or abnormal serum biomarkers for malignancy. Then, the labeled data are fed into the model, and the cross-validation process is performed to adjust the weights appropriately for diagnosis. In this review, most of the models are established by supervised learning, typically in the differential diagnosis part of cholangiocarcinoma with clinical features and radiomic features (Figure 1).
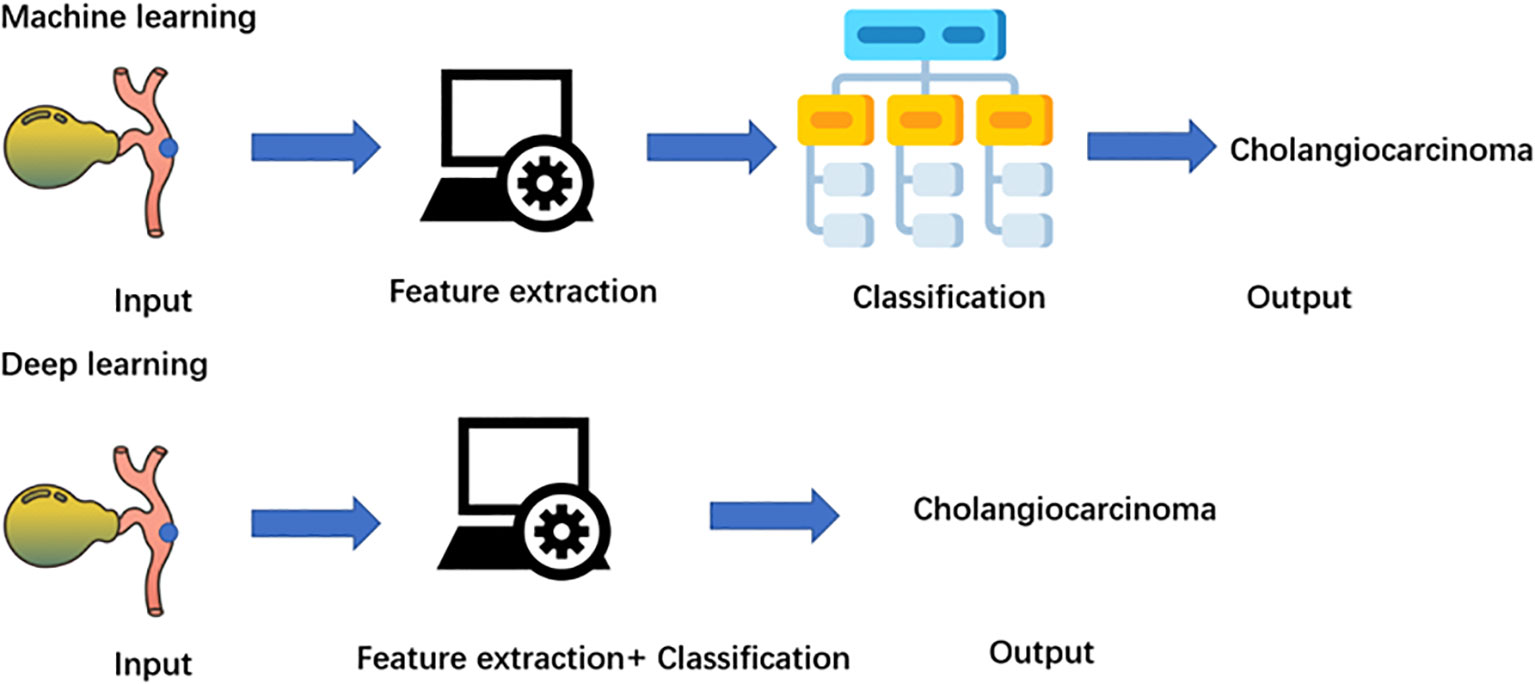
Figure 1 Machine learning can be classified as supervised, unsupervised, semi-supervised and reinforcement learning. All data is labeled In supervised learning, while unsupervised learning is unlabeled. Small amount of labeled data fed semi-supervised learning, and a large amount of unlabeled data can be handled. Reinforcement learning interact with rewards or punishments from the environment.
Unsupervised learning
Without the need for human intervention for images of cholangiocarcinoma, unsupervised learning analyzes unlabeled data and discovers similarities and differences (37). Potential patterns or data groupings can be identified by unsupervised learning. Also, it can be used to map the original high-dimensional data to achieve dimensionality reduction, typically in radiomic studies for cholangiocarcinoma. PCA, SVD, and k-means clustering are some common algorithms.
Semisupervised learning
In semisupervised learning, clinical physicians label smaller data for cholangiocarcinoma and feed the algorithms to perform learning tasks on a bigger unlabeled database for the diagnosis of cholangiocarcinoma (38). Semisupervised learning overcomes the disadvantage of supervised learning, which requires large enough labeled data. Moreover, using labeled data can prevent models from learning false correlations to improve the accuracy of the model.
Reinforcement learning
Reinforcement machine learning is an ML model that is not trained by sample data (39). This model learns by using trial and error and simulates the process to maximize the cumulative reward. For cholangiocarcinoma, the goal-oriented algorithm accumulates the successful treatment outcomes for cholangiocarcinoma to develop the best recommendation. Therefore, specific patients who may benefit from the surgery can be identified.
Deep learning
Deep learning is a branch of machine learning that focuses on deep artificial neural networks (40). Compared with machine learning algorithms, deep learning eliminates some of the data preprocessing and ingests the unstructured raw data (Figure 2). The features for the classification of cholangiocarcinoma are automatically extracted and analyzed to determine the most important features to distinguish cholangiocarcinoma, such as the biliary tract infiltration and the tumor size. Furthermore, it adjusts and fits itself for accuracy through the processes of gradient descent and backpropagation, especially when handling high-dimensional data. Deep learning algorithms, such as CNN and RNN, have been widely used in radiomics for cholangiocarcinoma and have achieved great success in image classification in recent years (41–43).
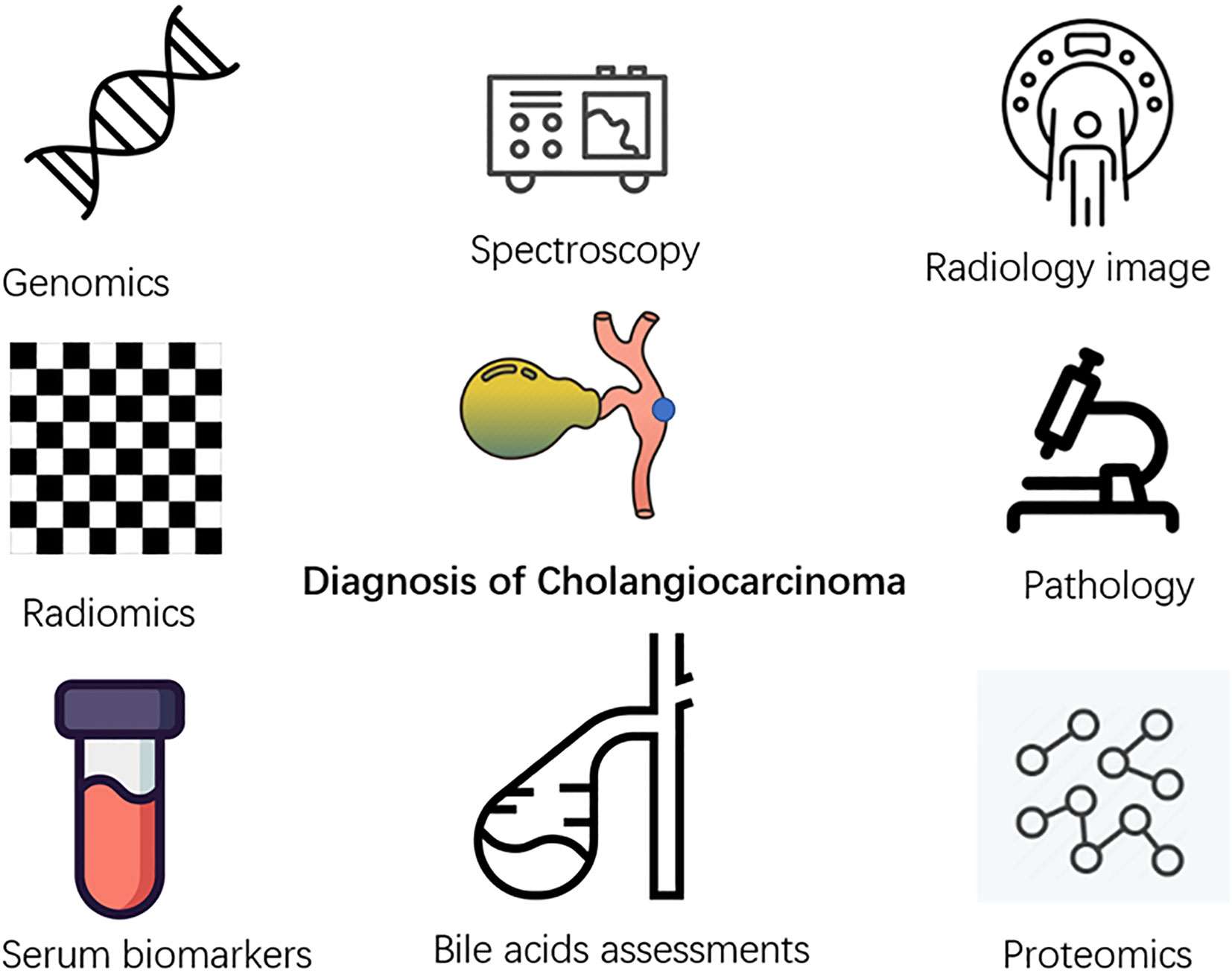
Figure 2 Application of artificial intelligence in multiple related fields of diagnosis of cholangiocarcinoma.
AI and diagnosis
Cholangiocarcinoma lacks accurate early diagnostic methods, which are important for patients at high risk of suffering from primary sclerosing cholangitis, polycystic liver disease, and chronic hepatolithiasis (44). Many researchers currently focus on applying AI to the early diagnosis of cholangiocarcinoma using different clinical information of the patients (Figure 3).
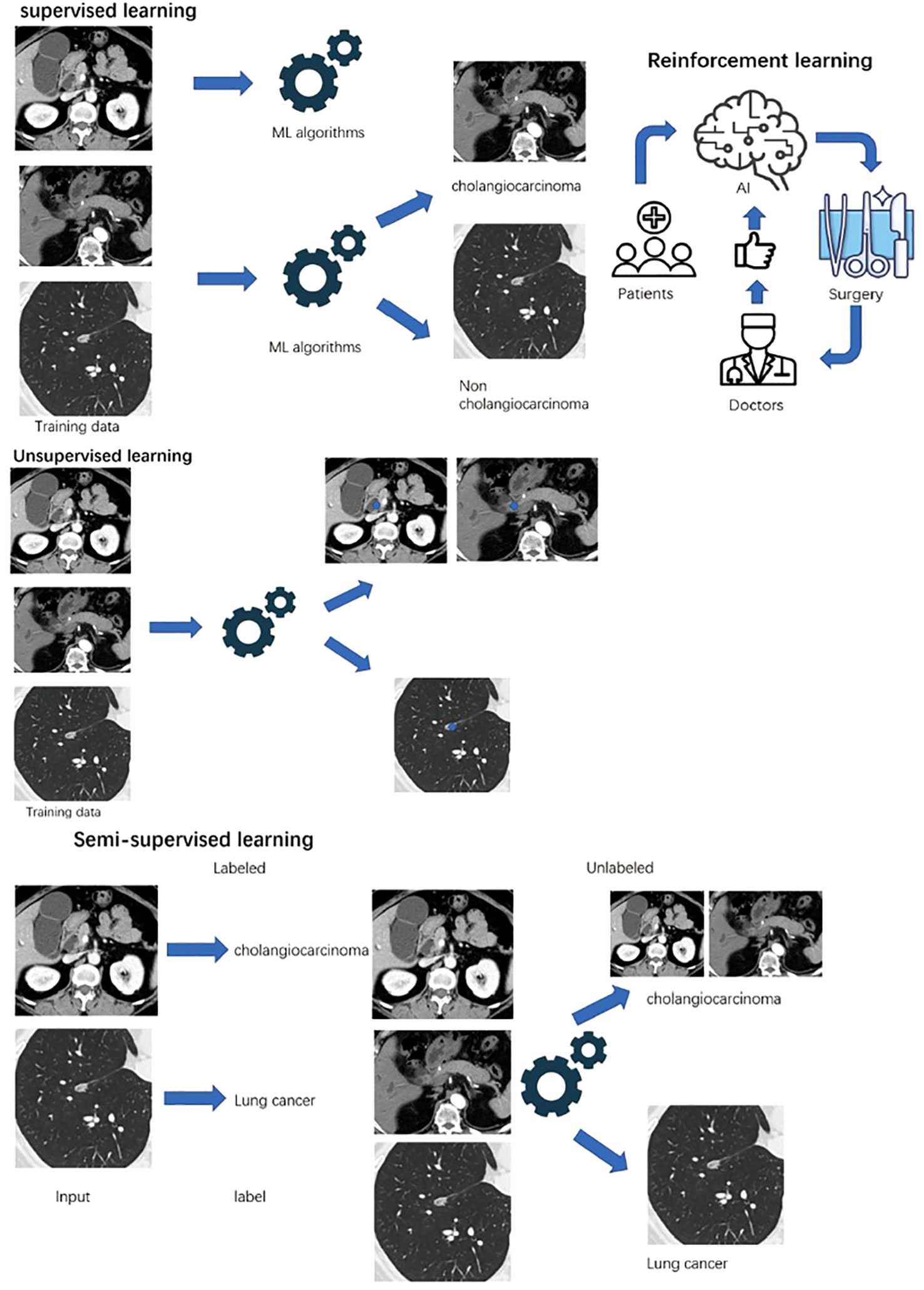
Figure 3 Diagram of machine learning and deep learning process. Machine learning needs four steps: input, feature extraction, classification, and output. Deep learning is a subgroup of a machine learning algorithm, which can automatically extract labels.
Table 1 summarized the application of AI in diagnosis of cholangiocarcinoma. Spectroscopy is considered a useful method for identifying subtle changes associated with cancer development in biological samples (45, 46). Making use of patients’ serum samples, Su et al. (47) applied Raman spectroscopy to identify typical biomolecular components in CCA and achieved good diagnostic accuracy combined with the SVM algorithm. Giordano et al. (48) also applied mass spectrometry with SVM and RF to permit cholangiocarcinoma identification, with an accuracy of 99.0%, sensitivity of 98%, and specificity of 100%. Furthermore, sera from CCA patients were detected by Chatchawal et al. to find out specific molecular vibrations of molecules and gain cancer-specific biomarkers using attenuated total reflectance-Fourier transform infrared (ATR-FTIR) spectroscopy (49). SVM, RF, and NN models were established. The variations of collagen molecules, phosphate groups, lipid ester carbonyls, and polysaccharides were found in CCA patients. Specific lipid metabolism in HCC and abnormal extracellular pathways in CCA were found by mass spectrometry (50). RF was used to select the features and establish the model with an area under the curve (AUC) value of 0.92 and an accuracy of 90%. To differentiate adenocarcinomas of the pancreas and biliary tree, Bollwein et al. (51) investigated the mass spectrometric peptide sequences of histone and collagen with GB, SVM, and KNN algorithms, achieving an AUC of 0.96 and accuracy of 0.91. Serum lipid and peptide detection was conducted by nano-assisted laser desorption ionization mass spectrometry to discriminate between benign biliary diseases and cholangiocarcinoma (52). Three algorithms (ANN, LASSO, SVM) were used to investigate the lipidomics panel, peptidomics panel, and multiomics panel, achieving sensitivity and specificity of 96.5% and 96.4% in the diagnosis.
Bile acid assessments are potentially involved in the development and progression of CCA (53). Using plasma bile acid profiles, the performance of six ML algorithms was evaluated by Negrini et al. (54). Naive Bayes, using direct bilirubin concentration as the normalization process, achieved the best performance, with an AUC of 0.95, specificity of 1.00, PPV of 1.00, and accuracy of 86.4%, which was significantly better than the other five algorithms (LR, KNN, RBF SVM, RF, XGBoost). CLU protein was upregulated in bile proteomics in CCA, proven by immunoblotting analysis, qRT-PCR, and immunohistochemistry staining. Combined with serum CA 19-9, indirect bilirubin, GGT, TG, LDLC, and TBA levels, the seven-panel models with LASSO and RF methods had an AUC of 0.947, sensitivity of 90.3%, specificity of 84.9%, and accuracy of 87.0% (55). Urman et al. (56) further analyzed the metabolomics and proteomics of human bile with spectrometry. The NN algorithm was performed to extract useful metabolomic data. A total of 15 molecular features were identified with an AUC of 0.984, sensitivity of 94.1%, and specificity of 92.3% in differentiating CCA from benign biliary stenoses.
Differentially expressed genes are also available for CCA diagnosis (57). Human gene expression profiles of the ICC GEO database were investigated to screen out the differentially expressed genes with three algorithms (LASSO, SVM-RFE, RF). MMP14 was finally determined with high accuracy and an AUC of 0.999, which affects the infiltration of monocytes and the activation of memory CD4 T cells (58). Six hundred seventy-two mutations in 45 different CCA genes were also detected by Ali Shah et al. (59). With three long short-term models, gated recurrent units, and bidirectional LSTM algorithms based on RNN, the deep learning model was established with a sensitivity of 100%, specificity of 98%, and accuracy of 99%. Liao et al. (60) screened out 166 CCA disease signature genes by RF algorithms and built an ANN prediction model for CCA, with an AUC of 0.980. Genomic profiles were used to identify the gene alteration of HCC, CCA, and cHCC-CCA. Murugesan et al. (61) built an RF model to classify cHCC-CCA by integrating genomic-derived data. TP53, TERT, and PTEN were the most common in gene alterations. Combined with the DNA methylation data from public data and in-house patients, RF, SVM, and NN were developed. Further improving by anomaly detection, the accuracies were continually enhanced, and the best balance was reached by NN concerning accuracy and the number of samples (62). With the single-cell RNA-sequencing datasets, Swain et al. (63) used KNN and SNN to cluster the cell group. Moreover, UMAP and t-SNE identified the top 2 components to classify HCC and ICC. Further analysis indicated the interaction between smooth muscle cells and epithelial cells and cell adhesion pathway alteration in ICC. Transcriptomic data from public datasets were used to identify the classification between ICC and ECC. Differentially expressed genes in EMT, DNA repair, and the EGFR pathway were identified. KNN, SVM, and fast unified RF were used to build the prediction model (64). The translational proteomic approach was also used to discover specific CCA biomarkers. Seven candidate proteins and four significantly increased proteins proven by enzyme-linked immunosorbent assays with SVM showed strong predictive performances for CCA, with an AUC of 0.96 (28).
Imaging technology plays an important part in the diagnosis of CCA. Current technology includes ultrasound, CT, MRI, and 18F-FDG PET/CT. Due to similar invasive features of hepatic alveolar echinococcosis with malignancy, Wa et al. (65) utilized ultrasound and CEUS features with LASSO regression to establish the US scoring system for differentiation.
The first study with the application of the AI algorithm in CCA CT images was in 2006 (66). HCC is a liver tumor due to the abnormal proliferation of parenchyma, whereas dysregulation of epithelioid cells of the biliary tract results in ICC. ANN is designed based on the 24 CT image features and 9 clinical findings. The AUC was 0.961 and the radiologist’s performance with the ANN model significantly improved (p < 0.02). With multiphase CT scan images, SVM was utilized by Ponnoprat et al. to develop a method and achieve an accuracy of 88% in the classification of liver cancer (67). Portal venous phase images from CT scans analyzed by the modified Inception v3 network algorithms obtained an accuracy of 96% and sensitivity of 94% in ICC classification (68). Combining CT images with the tumor markers, the CNN model was developed to categorize ICC from HCC, achieving better accuracy and specificity than the radiologist (43).
Different from the previous study concerning gene expression profiles, several phases of the CE-CT were enrolled in the study to investigate the different radiological features in different CCA-related gene expression levels (58, 59). The IDH gene, which translated key enzymes in the tricarboxylic acid cycle, frequently mutated and participated in the carcinogenesis of ICC. Venous phase images showed great accuracy of 86.3% and specificity of 88.5% in predicting the IDH mutations with the SVM algorithm (69).
MRI has become the better choice for the staging and differentiation of CCA because of the high sensitivity of the infiltration and high resolution. Shen et al. (70) developed a nomogram based on LASSO and RF to identify ICC among intrahepatic lithiasis patients, with an accuracy of 82.6%. Hu et al. (71) made use of a tree-based pipeline optimization tool. After manual segmentation of the tumors, different thresholds of feature selections and classifiers were evaluated. The best combinations were established with an accuracy of 73%–75%. To differentiate the cHCC-CCA, the LASSO model, composed of clinical features (tumor size, age, etc.) and MRI images, was built with an AUC of 0.863 and specificity of 91.8% (72). CNN with multiphasic MRI features achieved a specificity of 98% and AUC of 0.992 in classifying six hepatic tumors. Further study about the radiologic imaging features for each hepatic tumor reached a PPV of 83.3% in differentiating the ICC from liver lesions (41, 42). The strided feature fusion residual network, a deep learning workflow composed of MFF, SRB, and CBAM, was also proposed to automatically utilize the MRI features to distinguish ICC, with an AUC of 0.9680 (73). Compared with the deep learning workflow, Gd‐EOB‐DTPA-enhanced MRI features with RF, SGD, and SVM algorithms achieved an AUC of 0.90 in differentiating atypical intrahepatic mass-forming CCA from poorly differentiated HCC.
Radiomics is defined as the extraction and analysis of advanced quantitative features from medical images of ultrasound, CT, MRI, and 18F-FDG PET/CT. Radiomics features can be extracted after the region of interest is delineated. Several algorithms were used to establish the predictive model and had favorable value to predict CCA from infected focal liver lesions (74). Ren et al. (75) performed SVM based on ultrasonics to distinguish ICC from HCC, with an AUC of 0.936 and a sensitivity of 0.900. Also, the ultrasound-based radiomics with LASSO algorithm analysis achieved an AUC of 0.920 (76). Xu et al. (77) employed 45 models based on the combination of 5 selection methods and 9 classification methods to study radiomics in CE-CT. The RF algorithm combined with LDA achieved the highest AUC and accuracy (0.997 and 0.969, respectively) to differentiate between ICC and hepatic lymphoma. Combined CT radiomics features and clinical features can detect early malignant lesions and predict the tumor prognosis. Utilized by Mahmoudi et al. (19), four independent ML models were established and LR achieved the best AUC of 0.82. A better AUC of 0.855 was achieved by Xu et al. with LASSO and SVM algorithms to classify ICC with CT radiomics features (78). Moreover, SVM was conducted by Liu et al. to differentiate cHCC-CCA by using MRI radiomics features, with an AUC of 0.81, while CT radiomics was of limited value (79). Meanwhile, LASSO and SVM may provide a better AUC of 0.91 and an accuracy of 89% with MRI radiomics to distinguish different pathological types of liver cancer, including cHCC-CCA, HCC, and ICC. The workflow for optimal radiomics classification toolbox, involving eight machine learning approaches, analyzed T2-weighted MRI radiomics to distinguish malignant and benign primary solid liver lesions, with an AUC of 0.78. Furthermore, the CSAM-Net model was established with better AUC values and accuracy than those of the conventional radiomics models (80).
Microscopic hyperspectral (HSI) pathological images of CCA are collected, typically with more pixel information than the traditional RGB images. Exploration was conducted using deep CNN and further RF algorithms to predict CCA on a pathological patch size of 299, with an accuracy of 88.2% (81). Optical coherence tomography is available for scanning the resection specimens to determine the resection margins intraoperatively. Trained with CNN, the sensitivity and specificity reached 94% and 93%, respectively (82). To identify the impact of AI algorithms in assisting pathologists to differentiate between HCC and CCA, CNN was trained to classify hematoxylin and eosin-stained whole-slide images, with an accuracy of 0.885. The algorithm improved the accuracy of the well-experienced pathologists (p = 0.045). Meanwhile, the accuracy of the algorithm had a great impact on the decision-making of the pathologists (83).
AI and prognosis
Accurately predicting the prognosis of CCA is important for individual treatment strategy. For potentially curative cholangiocarcinoma, the long-term prognosis depends on various factors, including the location and stage of the primary lesion, surgery-associated complications, and treatment-related complications (1, 2, 84, 85). The main prognostic factors are margin status and lymph node involvement (86, 87). For advanced cholangiocarcinoma, the prognosis is mainly predicted by the M stage, primary lesion site, and elevated serum alkaline phosphatase levels (88). Chemotherapy and clinical trials may not significantly improve the prognosis (89).
Table 2 summarized the application of AI in prognosis of cholangiocarcinoma. Clinical features and TNM stage are frequently used in predicting the survival and prognosis of CCA patients. Classification algorithms can easily identify the important factors concerning the prognosis. To detect the burden of the ICC and predict the prognosis, the CART algorithm was used to find out the best estimator. Lymph node metastasis and tumor size had a great effect on long-term prognosis (90). Also, the CART algorithm was used by Kaibori to make risk classification in ICC. Preoperative biomarkers, including CA 19-9, CRP, and CAR (ratio between CRP and albumin), were used for predicting OS and RFS (91). Tsilimigras et al. (92) divided the ICC into three phenotypes by tumor size, CA 19-9, and NLR. The classification tree algorithm assigned patients to different clusters and indicated different OS rates. Zhou et al. (93) established three RF models and developed an online tool, with an AUC of 0.7478. Serum albumin-to-fibrinogen ratio and CA 19-9 were used to develop the DeepSurv model to predict the prognosis of ECC and guide individualized postoperative chemotherapy as shown in the study by Wang et al. (87). The prognostic scoring system of ICC was constructed based on XGBoost, RF, and GBDT to evaluate the prognosis by biomarkers, with a C-index of 0.693 (94). The psoas muscle index, defined as the area of the psoas muscle at the L3 vertebra level divided by the squared body height, combined with the features of tumor burden and hepatic reserve in ANN, with an AUC of 0.89 in the 1-year survival prediction in ICC, was significantly higher than the Fudan score (95, 96). Clinical risk factors and CT radiomics, selected by the LASSO algorithm, were also used to build a nomogram to preoperatively predict the prognosis of patients with ICC, with an AUC of 0.783 in a 3-year OS (97). To identify patients who will benefit the most from surgery, Tsilimigras et al. (98) further utilized preoperative estimated tumor number and size, albumin–bilirubin (ALBI) grade, and preoperative lymph node by the CART algorithm to generate four different groups, achieving different R0 resection rates, microvascular invasion rates, 5-year OS, and 5-year DFS. Clinical features and serum biomarkers were conveniently obtained and trained with the algorithms. Tools established by those features can be easily found online, but they may not provide a more accurate prediction of prognosis.
Molecular alterations of cancer cells were also useful as the prevalence of sequencing. Ruan et al. (99) combined LASSO and Cox regression to establish the final risk scoring of 21 gene-pair signatures to predict the prognosis, with an AUC of 0.88. Angiogenesis is a factor of rapid development and metastasis of malignancy, including CCA. Liu et al. (100) developed and validated a model based on several ML algorithms to predict vascular endothelial growth factor (VEGF) expression and microvessel density of ECC, with an AUC of 0.912. For further classifications and survival analysis of hepatobiliary cancers, ANN algorithms with whole-exome sequencing in the TCGA database revealed that IDH or METH-2 molecular subtypes of CCA may have a worse prognosis, with a C-index of 0.71 (90). Target therapy toward the PD-1/PD-L1 pathway has shown capability in improving CCA patients’ survival. The expression status of PD-1/PD-L1 was commonly assessed by immunohistochemical staining. Zhang et al. (101) first selected radiomics features from AP and PVP MR images to construct PD-1 and PD-L1 predictive models based on LASSO, with an AUC of 0.897. Xie et al. (102) focused on the tumor microenvironment of ICC and explored the lymphocyte number and segment features in the H&E-stained whole-slide images. After feature selection by the mRMR algorithm, three algorithms, namely, LDA, QDA, and DT, were selected to predict the survival of ICC, achieving the best AUC of 0.74 ± 0.06 by DT. Molecular subtyping of CCA with different prognoses now can be identified by several methods, which also provide patients with precision therapy options. However, such methods involve high costs.
A treatment strategy should be determined by surgeons to improve the long-term outcomes of CCA. For operable CCA, Alaimo et al. (103) set up an optimal policy tree model based on the RF algorithm to help surgeons determine margin width in hepatectomy, with an AUC of 0.81. Liver failure after hemihepatectomy for liver malignancy is the leading fatal reason for postoperative complications. For preoperative prediction, a dense neural network block was used to detect the radical information from CE-CT, with an accuracy of 84.15% (104). For inoperable hilar CCA, bilateral plastic stent placement is commonly used for maintaining biliary drainage. To predict the early occlusion of bilateral plastic stent placement, the ANN model analyzed the cancer stage and the Bismuth stage, achieving a larger AUC than the logistic regression model (0.9648 vs. 0.8763) (105). Radiation therapy and radioembolization with isotopes are available adjuvant therapies for CCA. Ibragimov et al. (106) combined CT images and delivered dose plans with the CNN algorithm to detect the specific anatomical regions with high hepatobiliary toxicity risk after radiation therapy. Consequently, the individual-delivered dose was determined with an accuracy of 0.73. Furthermore, the authors used the CNN + SVM+ RF + FcNN algorithms to predict the potential radiation toxicity of healthy organs and reach an AUC of 0.85 (34). Radioisotope 90Y microspheres in radioembolization were used in CCA treatment. The biodistribution of the isotopes used to be predicted with difficulty, but generative adversarial network algorithms with PET/CT scans and dose volume input have accurately predicted the biodistribution and are suitable for personalized pretreatment planning (107). AI now can provide better accuracy and simulation of the determination of the treatment strategy.
Recurrence
Although surgery may offer the potential cure for localized and resectable CCA, the prognosis still remains low, with a 5-year survival of 25%–35%, and 50%–70% of patients experience recurrence (2). The most common type of recurrence for eCCA is local recurrence. Distant metastasis of cholangiocarcinoma is relatively common in hilar cholangiocarcinoma. A study showed that 41% of patients with hilar cholangiocarcinoma experienced initial recurrence involving distant areas (108). Other studies have reported that 60% of patients experienced distant metastasis after complete (R0) resection of the hilar cholangiocarcinoma under a microscope (109). On the other hand, the types of iCCA recurrence are intrahepatic recurrence, lymph node recurrence, and distant extrahepatic recurrence (usually in the peritoneum) (110). Consequently, identifying patients who may experience recurrence is important. Alaimo et al. (111) used 14 clinical features to conduct three ML algorithms. The RF algorithm shows better achievement than SVM and LR, with an AUC of 0.904 in the training set. Ji et al. (112) analyzed age, TMN stage, histological type, and surgery strategy to yield the GBM model to predict the prognosis of the ICC after liver resection. The model had improved prognostic discrimination (C-statistic, 0.723) compared with the AJCC staging system and MEGNA prognostic score system developed by Raoof et al. (86). Moreover, Jolissaint et al. (113) constructed the predictive model based on RF classification by the four tumor features and future liver remnant in CT, with an AUC of 0.84. Combining 15 radiomic features with 3 clinical features, Song et al. (18) constructed the LightGBM model with an AUC of 0.974.
Radiology also provides a methodological approach to predict the recurrence of cholangiocarcinoma. Qin et al. (114) combined clinical and molecular features with radiomic features from the CE-CT to make a model with good discrimination and sensitivity (AUC of 0.883 and accuracy of 0.826), which was superior to the 8th AJCC, MSKCC, and Gazzaniga staging systems in predicting the early recurrence of patients after curative resection in PHC (115–117). This model provided a comprehensive analysis of recurrence although some features may not be a high risk. To predict the early recurrence of ICC, Bo et al. (118) developed ML radiomics models with seven algorithms, with a mean AUC of 0.87 ± 0.02, and RF, NN, and SVM achieved the best performance, with an AUC of 0.89. With the same purpose, the arterial-phase image features of contrast-enhanced MRI, extracted by the LASSO algorithm, were used to establish a nomogram, with an AUC of 0.90, as shown by the study of Liang et al. (119).
Metastasis
Several tumor cells in the bloodstream are considered early metastatic biomarkers for malignant tumors. To more efficiently detect circulating tumor cells, Piansaddhayanon et al. (120) established a large-scale microscopic imaging dataset of tumor and normal cells from organoid-derived cells of CCA. The model based on the deep NN algorithm provided a foundation for circulating tumor cell detection, with an AUC of 0.78, and may need further investigation.
Lymph node metastasis is an important factor for CCA prognosis. The 8th AJCC guideline demonstrated the stage of metastatic lymph nodes as a factor of poor prognosis. Recently, the radiomic models have gained great accuracy in predicting the lymph node status of CCA. Wang et al. (121) developed a model integrating SVM with clinical features to predict the lymph node stage of CCA patients, with an AUC of 0.870. To predict ICC lymph node metastasis preoperatively, serum CEA, CA 19-9, and lymphadenopathy on CT/MR image were enrolled in the RF training model. Compared with the nomogram based on logistic regression, the RF model has better sensitivity and accuracy (122). Xu et al. (123) investigated the image features from T1WI contrast-enhanced MRI. After extracting related features by the mRMR algorithm, the SVM model was built. Combined with clinical features, the nomogram was established with an AUC of 0.870. Tang et al. (124) focused on the differentiation degree and lymphatic node metastasis of ECC predicted by MRI radiomics. Four MRI sequence features and six clinical features were selected by five methods, including JMI, mRMR, SKB, and Wilcoxon. Then, 10 machine learning algorithms were conducted. The bagging classifier gained the best performance for differentiation degree, and XGB gained the best performance for lymphatic node metastasis, both significantly different from the other eight models (ADB, DT, Gaussian naive Bayesian, etc.). To preoperatively predict the microvascular invasion in ICC, several parametric MRI images were fused by the CNN algorithms. Gradient-weighted class activation mapping is used for visualizing the network and highlighting the important factors in estimation, with a sensitivity of 85.7% and accuracy of 86.8% (125). Consequently, lymph node metastasis nowadays can be predicted with great sensitivity and accuracy, while other characteristics including microvascular invasion are less studied.
Gene and protein expression profiling acts as a potential approach to malignant diagnosis and prognosis, but not in the metastasis prediction due to the batch effects. Therefore, genome-wide integrative proteome and transcriptome analysis was necessary. Ruan et al. (99) assembled models by using their own proteome and transcriptome datasets, validated by the TCGA dataset and the Ahn dataset. Developed from the k-TSP method, the authors employed a weighted voting procedure for the gene pairs to finally predict the metastasis possibilities. The EMLI algorithm achieved an AUC of 0.958 and an accuracy of 97.1% in ICC patients. However, detection of the proteome and transcriptome still involves a high cost and may not be widely accepted in medical practice.
AI and other aspects
Fibroblast growth factor receptors bind fibroblast growth factor and mediate cellular functions. With a dataset of 2,356 chemical compounds, Charan et al. utilized four ML algorithms (SVM, RF, k-NN, and ANN) to screen potential FGFR1 inhibitors for various cancers, including CCA (126). Furthermore, to predict the efficacy of anticancer drugs in individual patients, Gerdes et al. (127) used the proteomics and phosphoproteomics data from various cell lines to train and verify drug ranking using the ML algorithm, which was based on the DL, NNET, BGLM, RF, PLS, PCR, SVM, and cubist ML models. This algorithm has achieved quite a low error, with a mean-squared error <0.1. In CCA patients, inhibitors of histone deacetylase and the PI3K pathway were selected as high-ranking therapies (128). The TensorFlow deep learning algorithm was used to investigate the adjuvant treatment risk for ICC patients. A total of 8 features including tumor burden, CA 19-9, and CEA were selected as significant prognostic factors with the TensorFlow deep learning algorithm, reaching an AUC of 0.78, which was higher than the AJCC stage prediction (129).
The latent Dirichlet allocation algorithm, a machine learning method to identify research topics, was used to summarize scientific publications in the field of CCA. The results demonstrated that survival and differential diagnosis were the highly concerned research topics of CCA, which were in accordance with the conclusion of this review. Although several studies are concerned about microRNA expression, high-quality clinical trials and basic studies are still urgently needed (130).
Discussion
Several studies have investigated the application of AI on malignancy in several aspects of diagnosis, prognosis, recurrence, and metastasis with some extent of automation. Moreover, it is obvious that AI is already better than people in some areas as a consequence of technological development. However, not a single algorithm can solve all problems as different algorithms are suitable for different scenarios. Artificial intelligence algorithms are difficult to completely replace human decision-making and judgment.
Although AI algorithms have many involvements and gain great results in the daily medical work of CCA, many challenges still remain (30, 40, 131, 132). AI requires a large amount of data for training and optimization, but some data are difficult to obtain because the equipment needed may be quite expensive so they may not be available in a single center trial. Moreover, many medical slides may contain millions of pixels and are too large to be fed into the algorithms. Once the whole image is split into small slides to input, some potential trends may be ignored by the algorithms. On the other hand, some medical data lack labels necessary for supervised learning, and the quality of input data may be varied due to the different experiences of the experts. This may significantly affect the accuracy of the model, and handling large datasets becomes difficult.
The interpretability of the algorithms, the degree to which machine learning algorithms can be understood by humans, is still the biggest challenge for their wide usage in the field of medicine. There are several ethical challenges related to AI in the medical field. The most obvious part is distrusting the accuracy of AI prediction as the replicability of specific AI is hard to achieve without publicly released data. Furthermore, the continual learning capability of AI also raises challenges in its regulation. In 2019, the FDA proposed a framework to evaluate AI products from premarket performance to postmarket performance (https://www.fda.gov/medical-device). In addition, it is unclear whether developers or doctors are to be blamed when AI models make mistakes (133).
Nowadays, three approaches have attempted to explain the interpretability of AI models: 1) surrogate models, which use a simple and interpretable model to approximate the original model. When the accuracy is close enough, a surrogate model can be used to explain the original “black-box” model; 2) intrinsic interpretability, which shows the workflow of the algorithms and compares with the mind of humans; and 3) data visualization, which helps us to quickly and comprehensively understand the characteristics of data distribution, thereby assisting us in choosing the most reasonable model to approximate the optimal solution that the problem can achieve. These three methods may provide us with the way to test the safety and efficacy of the AI algorithms before application in the medical field and avoid patient harm (134, 135).
With further development of large databases and improvement of computing power, AI will be trained with clinical features based on multicenter and multiomics data to achieve better clinical decision-making capability. Despite the challenges of AI, it will eventually be integrated into the diagnosis and treatment of cholangiocarcinoma due to its great advantage in clinical practice. Apart from radiology and serum biomarkers, cell-free tumor DNA in whole genome sequencing can be studied by AI to identify early cholangiocarcinoma and predict patient outcomes (136). It can be assumed that early detection of cholangiocarcinoma may be achieved with the corporative training of physicians and the use of AI. AI also creates the need for new capabilities in data processing and machine learning.
Conclusions
We concluded the applications of AI on CCA. Early detection can be achieved by the combination of medical data and AI. Moreover, AI assists physicians in making accurate diagnoses and proper treatment options. Several studies focused on the diagnosis of and survival from cholangiocarcinoma, and high-quality clinical trials are still urgently needed for cholangiocarcinoma patients. Despite some limitations of current AI applications, AI will still significantly improve many aspects of cholangiocarcinoma in the medical field.
Author contributions
JH: Conceptualization, Data curation, Formal Analysis, Investigation, Methodology, Project administration, Resources, Software, Visualization, Writing – original draft. YQ: Conceptualization, Data curation, Formal Analysis, Investigation, Methodology, Project administration, Resources, Software, Visualization, Writing – original draft. XB: Conceptualization, Data curation, Formal Analysis, Investigation, Methodology, Project administration, Resources, Software, Visualization, Writing – original draft. XH: Conceptualization, Funding acquisition, Validation, Writing – review & editing.
Funding
The author(s) declare financial support was received for the research, authorship, and/or publication of this article. This work was supported by the Chinese Academy of Medical Sciences Innovation Fund for Medical Sciences (CAMS) Initiative for Innovative Medicine (Grant No. 2021-I2M-1-022) and National High Level Hospital Clinical Research Funding (Grant No. 2022-PUMCHB-003).
Conflict of interest
The authors declare that the research was conducted in the absence of any commercial or financial relationships that could be construed as a potential conflict of interest.
Publisher’s note
All claims expressed in this article are solely those of the authors and do not necessarily represent those of their affiliated organizations, or those of the publisher, the editors and the reviewers. Any product that may be evaluated in this article, or claim that may be made by its manufacturer, is not guaranteed or endorsed by the publisher.
Glossary
References
1. Rizvi S, Khan SA, Hallemeier CL, Kelley RK, Gores GJ. Cholangiocarcinoma - evolving concepts and therapeutic strategies. Nat Rev Clin Oncol (2018) 15(2):95–111. doi: 10.1038/nrclinonc.2017.157
2. Razumilava N, Gores GJ. Cholangiocarcinoma. Lancet (London England) (2014) 83(9935):2168–79. doi: 10.1016/S0140-6736(13)61903-0
3. Patel N, Benipal B. Incidence of cholangiocarcinoma in the USA from 2001 to 2015: A US cancer statistics analysis of 50 states. Cureus (2019) 11(1):e3962. doi: 10.7759/cureus.3962
4. Rizvi S, Gores GJ. Pathogenesis, diagnosis, and management of cholangiocarcinoma. Gastroenterology (2013) 145(6):1215–29. doi: 10.1053/j.gastro.2013.10.013
5. Blechacz B, Komuta M, Roskams T, Gores GJ. Clinical diagnosis and staging of cholangiocarcinoma. Nat Rev Gastroenterol hepatology (2011) 8(9):512–22. doi: 10.1038/nrgastro.2011.131
6. Izquierdo-Sanchez L, Lamarca A, La Casta A, Buettner S, Utpatel K, Klümpen HJ, et al. Cholangiocarcinoma landscape in Europe: Diagnostic, prognostic and therapeutic insights from the ENSCCA Registry. J hepatology (2022) 76(5):1109–21. doi: 10.1016/j.jhep.2021.12.010
7. Liang B, Zhong L, He Q, Wang S, Pan Z, Wang T, et al. Diagnostic accuracy of serum CA19-9 in patients with cholangiocarcinoma: A systematic review and meta-analysis. Med Sci monitor (2015) 21:3555–63. doi: 10.12659/MSM.895040
8. Venkatesh PG, Navaneethan U, Shen B, McCullough AJ. Increased serum levels of carbohydrate antigen 19-9 and outcomes in primary sclerosing cholangitis patients without cholangiocarcinoma. Digestive Dis Sci (2013) 58(3):850–7. doi: 10.1007/s10620-012-2401-3
9. Kawashima H, Ohno E, Ishikawa T, Mizutani Y, Iida T, Yamamura T, et al. Endoscopic management of perihilar cholangiocarcinoma. Digestive endoscopy (2022) 34(6):1147–56. doi: 10.1111/den.14317
10. Eaton JE, Welle CL, Bakhshi Z, Sheedy SP, Idilman IS, Gores GJ, et al. Early cholangiocarcinoma detection with magnetic resonance imaging versus ultrasound in primary sclerosing cholangitis. Hepatol (Baltimore Md) (2021) 73(5):1868–81. doi: 10.1002/hep.31575
11. Manfredi R, Barbaro B, Masselli G, Vecchioli A, Marano P. Magnetic resonance imaging of cholangiocarcinoma. Semin liver disease (2004) 24(2):155–64. doi: 10.1055/s-2004-828892
12. Potretzke TA, Tan BR, Doyle MB, Brunt EM, Heiken JP, Fowler KJ. Imaging features of biphenotypic primary liver carcinoma (Hepatocholangiocarcinoma) and the potential to mimic hepatocellular carcinoma: LI-RADS analysis of CT and MRI features in 61 cases. AJR Am J roentgenology (2016) 207(1):25–31. doi: 10.2214/AJR.15.14997
13. Fiz F, Masci C, Costa G, Sollini M, Chiti A, Ieva F, et al. PET/CT-based radiomics of mass-forming intrahepatic cholangiocarcinoma improves prediction of pathology data and survival. Eur J Nucl Med Mol imaging (2022) 49(10):3387–400. doi: 10.1007/s00259-022-05765-1
14. Njei B, McCarty TR, Varadarajulu S, Navaneethan U. Cost utility of ERCP-based modalities for the diagnosis of cholangiocarcinoma in primary sclerosing cholangitis. Gastrointestinal endoscopy (2017) 85(4):773–81.e10. doi: 10.1016/j.gie.2016.08.020
15. Parodi A, Fisher D, Giovannini M, Baron T, Conio M. Endoscopic management of hilar cholangiocarcinoma. Nat Rev Gastroenterol hepatology (2012) 9(2):105–12. doi: 10.1038/nrgastro.2011.271
16. Cillo U, Fondevila C, Donadon M, Gringeri E, Mocchegiani F, Schlitt HJ, et al. Surgery for cholangiocarcinoma. Liver Int (2019) 39 Suppl 1(Suppl Suppl 1):143–55. doi: 10.1111/liv.14089
17. Hamet P, Tremblay J. Artificial intelligence in medicine. Metabolism: Clin Exp (2017) 69s:S36–s40. doi: 10.1016/j.metabol.2017.01.011
18. Song Y, Zhou G, Zhou Y, Xu Y, Zhang J, Zhang K, et al. Artificial intelligence CT radiomics to predict early recurrence of intrahepatic cholangiocarcinoma: a multicenter study. Hepatol Int (2023) 17(4):1016–27. doi: 10.1007/s12072-023-10487-z
19. Mahmoudi S, Bernatz S, Ackermann J, Koch V, Dos Santos DP, Grünewald LD, et al. Computed tomography radiomics to differentiate intrahepatic cholangiocarcinoma and hepatocellular carcinoma. Clin Oncol (Royal Coll Radiologists (Great Britain)) (2023) 35(5):e312–e8. doi: 10.1016/j.clon.2023.01.018
20. Niazi MKK, Parwani AV, Gurcan MN. Digital pathology and artificial intelligence. Lancet Oncol (2019) 20(5):e253–e61. doi: 10.1016/S1470-2045(19)30154-8
21. Le Berre C, Sandborn WJ, Aridhi S, Devignes MD, Fournier L, Smaïl-Tabbone M, et al. Application of artificial intelligence to gastroenterology and hepatology. Gastroenterology (2020) 158(1):76–94.e2. doi: 10.1053/j.gastro.2019.08.058
22. Li JO, Liu H, Ting DSJ, Jeon S, Chan RVP, Kim JE, et al. Digital technology, tele-medicine and artificial intelligence in ophthalmology: A global perspective. Prog retinal eye Res (2021) 82:100900. doi: 10.1016/j.preteyeres.2020.100900
23. Yamamoto Y, Tsuzuki T, Akatsuka J, Ueki M, Morikawa H, Numata Y, et al. Automated acquisition of explainable knowledge from unannotated histopathology images. Nat Commun (2019) 10(1):5642. doi: 10.1038/s41467-019-13647-8
24. Bhinder B, Gilvary C, Madhukar NS, Elemento O. Artificial intelligence in cancer research and precision medicine. Cancer discovery (2021) 11(4):900–15. doi: 10.1158/2159-8290.CD-21-0090
25. Yu KH, Beam AL, Kohane IS. Artificial intelligence in healthcare. Nat Biomed engineering (2018) 2(10):719–31. doi: 10.1038/s41551-018-0305-z
26. Yoon CH, Torrance R, Scheinerman N. Machine learning in medicine: should the pursuit of enhanced interpretability be abandoned? J Med ethics (2022) 48(9):581–5. doi: 10.1136/medethics-2020-107102
27. Reed C. How should we regulate artificial intelligence? Philos Trans Ser A Mathematical physical Eng Sci (2128) 2018:376. doi: 10.1098/rsta.2017.0360
28. Watcharatanyatip K, Chutipongtanate S, Chokchaichamnankit D, Weeraphan C, Mingkwan K, Luevisadpibul V, et al. Translational proteomic approach for cholangiocarcinoma biomarker discovery, validation, and multiplex assay development: A pilot study. Molecules (Basel Switzerland) (2022) 27(18):5904. doi: 10.3390/molecules27185904
29. Raimundo R, Rosário A. The impact of artificial intelligence on data system security: A literature review. Sensors (Basel Switzerland) (2021) 21(21):7029. doi: 10.3390/s21217029
30. Rajpurkar P, Chen E, Banerjee O, Topol EJ. AI in health and medicine. Nat Med (2022) 28(1):31–8. doi: 10.1038/s41591-021-01614-0
31. Jordan MI, Mitchell TM. Machine learning: Trends, perspectives, and prospects. Sci (New York NY) (2015) 349(6245):255–60. doi: 10.1126/science.aaa8415
32. Obermeyer Z, Emanuel EJ. Predicting the future - big data, machine learning, and clinical medicine. New Engl J Med (2016) 375(13):1216–9. doi: 10.1056/NEJMp1606181
33. Deo RC. Machine learning in medicine. Circulation (2015) 132(20):1920–30. doi: 10.1161/CIRCULATIONAHA.115.001593
34. Ibragimov B, Toesca DAS, Chang DT, Yuan Y, Koong AC, Xing L, et al. Deep learning for identification of critical regions associated with toxicities after liver stereotactic body radiation therapy. Med physics (2020) 47(8):3721–31. doi: 10.1002/mp.14235
35. Obuchowski NA, Bullen JA. Receiver operating characteristic (ROC) curves: review of methods with applications in diagnostic medicine. Phys Med Biol (2018) 63(7):07tr1. doi: 10.1088/1361-6560/aab4b1
36. Jiang T, Gradus JL, Rosellini AJ. Supervised machine learning: A brief primer. Behav Ther (2020) 51(5):675–87. doi: 10.1016/j.beth.2020.05.002
37. Jafari M, Wang Y, Amiryousefi A, Tang J. Unsupervised learning and multipartite network models: A promising approach for understanding traditional medicine. Front Pharmacol (2020) 11:1319. doi: 10.3389/fphar.2020.01319
38. Uddin S, Khan A, Hossain ME, Moni MA. Comparing different supervised machine learning algorithms for disease prediction. BMC Med Inf decision making (2019) 19(1):281. doi: 10.1186/s12911-019-1004-8
39. Nath S, Korot E, Fu DJ, Zhang G, Mishra K, Lee AY, et al. Reinforcement learning in ophthalmology: potential applications and challenges to implementation. Lancet Digital Health (2022) 4(9):e692–e7. doi: 10.1016/S2589-7500(22)00128-5
40. Tran KA, Kondrashova O, Bradley A, Williams ED, Pearson JV, Waddell N. Deep learning in cancer diagnosis, prognosis and treatment selection. Genome Med (2021) 13(1):152. doi: 10.1186/s13073-021-00968-x
41. Hamm CA, Wang CJ, Savic LJ, Ferrante M, Schobert I, Schlachter T, et al. Deep learning for liver tumor diagnosis part I: development of a convolutional neural network classifier for multi-phasic MRI. Eur radiology (2019) 29(7):3338–47. doi: 10.1007/s00330-019-06205-9
42. Wang CJ, Hamm CA, Savic LJ, Ferrante M, Schobert I, Schlachter T, et al. Deep learning for liver tumor diagnosis part II: convolutional neural network interpretation using radiologic imaging features. Eur radiology (2019) 29(7):3348–57. doi: 10.1007/s00330-019-06214-8
43. Nakai H, Fujimoto K, Yamashita R, Sato T, Someya Y, Taura K, et al. Convolutional neural network for classifying primary liver cancer based on triple-phase CT and tumor marker information: a pilot study. Japanese J radiology (2021) 39(7):690–702. doi: 10.1007/s11604-021-01106-8
44. Chapman RW. Risk factors for biliary tract carcinogenesis. Ann oncology: Off J Eur Soc Med Oncol (1999) 10 Suppl 4:308–11. doi: 10.1093/annonc/10.suppl_4.S308
45. Li S, Guo Z, Liu Z. Surface-enhanced Raman spectroscopy + support vector machine: a new noninvasive method for prostate cancer screening? Expert Rev Anticancer Ther (2015) 15(1):5–7. doi: 10.1586/14737140.2015.992419
46. Li SX, Zeng QY, Li LF, Zhang YJ, Wan MM, Liu ZM, et al. Study of support vector machine and serum surface-enhanced Raman spectroscopy for noninvasive esophageal cancer detection. J Biomed optics (2013) 18(2):27008. doi: 10.1117/1.JBO.18.2.027008
47. Su N, Dawuti W, Hu Y, Zhao H. Noninvasive cholangitis and cholangiocarcinoma screening based on serum Raman spectroscopy and support vector machine. Photodiagnosis Photodyn Ther (2022) 40:103156. doi: 10.1016/j.pdpdt.2022.103156
48. Giordano S, Takeda S, Donadon M, Saiki H, Brunelli L, Pastorelli R, et al. Rapid automated diagnosis of primary hepatic tumour by mass spectrometry and artificial intelligence. Liver Int (2020) 40(12):3117–24. doi: 10.1111/liv.14604
49. Chatchawal P, Wongwattanakul M, Tippayawat P, Kochan K, Jearanaikoon N, Wood BR, et al. Detection of human cholangiocarcinoma markers in serum using infrared spectroscopy. Cancers (2021) 13(20):5109. doi: 10.3390/cancers13205109
50. Yi X, Zhu J, Liu W, Peng L, Lu C, Sun P, et al. Proteome landscapes of human hepatocellular carcinoma and intrahepatic cholangiocarcinoma. Mol Cell proteomics: MCP (2023) 22(8):100604. doi: 10.1016/j.mcpro.2023.100604
51. Bollwein C, Gonçalves CPL, Utpatel K, Weichert W, Schwamborn K. MALDI mass spectrometry imaging for the distinction of adenocarcinomas of the pancreas and biliary tree. Molecules (Basel Switzerland) (2022) 27(11):3464. doi: 10.3390/molecules27113464
52. Qu X, He B, Li Z, Jiang X, Liu X, Chen X, et al. Accurate discrimination of benign biliary diseases and cholangiocarcinoma with serum multiomics revealed by high-throughput nanoassisted laser desorption ionization mass spectrometry. J Proteome Res (2023) 22(6):1855–67. doi: 10.1021/acs.jproteome.2c00846
53. Danese E, Salvagno GL, Negrini D, Brocco G, Montagnana M, Lippi G. Analytical evaluation of three enzymatic assays for measuring total bile acids in plasma using a fully-automated clinical chemistry platform. PloS One (2017) 12(6):e0179200. doi: 10.1371/journal.pone.0179200
54. Negrini D, Zecchin P, Ruzzenente A, Bagante F, De Nitto S, Gelati M, et al. Machine learning model comparison in the screening of cholangiocarcinoma using plasma bile acids profiles. Diagnostics (Basel Switzerland) (2020) 10(8):551. doi: 10.3390/diagnostics10080551
55. Gao L, Lin Y, Yue P, Li S, Zhang Y, Mi N, et al. Identification of a novel bile marker clusterin and a public online prediction platform based on deep learning for cholangiocarcinoma. BMC Med (2023) 21(1):294. doi: 10.1186/s12916-023-02990-9
56. Urman JM, Herranz JM, Uriarte I, Rullán M, Oyón D, González B, et al. Pilot multi-omic analysis of human bile from benign and Malignant biliary strictures: A machine-learning approach. Cancers (2020) 12(6):1644. doi: 10.3390/cancers12061644
57. Kendall T, Verheij J, Gaudio E, Evert M, Guido M, Goeppert B, et al. Anatomical, histomorphological and molecular classification of cholangiocarcinoma. Liver Int (2019) 39 Suppl 1:7–18. doi: 10.1111/liv.14093
58. Wu J, Guo Y, Zuo ZF, Zhu ZW, Han L. MMP14 is a diagnostic gene of intrahepatic cholangiocarcinoma associated with immune cell infiltration. World J gastroenterology (2023) 29(19):2961–78. doi: 10.3748/wjg.v29.i19.2961
59. Shah AA, Alturise F, Alkhalifah T, Faisal A, Khan YD. EDLM: ensemble deep learning model to detect mutation for the early detection of cholangiocarcinoma. Genes (2023) 14(5):1104. doi: 10.3390/genes14051104
60. Liao J, Meng C, Liu B, Zheng M, Qin J. A cholangiocarcinoma prediction model based on random forest and artificial neural network algorithm. J Coll Physicians Surgeons–Pakistan: JCPSP (2023) 33(5):578–86. doi: 10.29271/jcpsp.2023.05.578
61. Murugesan K, Sharaf R, Montesion M, Moore JA, Pao J, Pavlick DC, et al. Genomic profiling of combined hepatocellular cholangiocarcinoma reveals genomics similar to either hepatocellular carcinoma or cholangiocarcinoma. JCO Precis Oncol (2021) 5:PO.20.00397. doi: 10.1200/PO.20.00397
62. Dragomir MP, Calina TG, Perez E, Schallenberg S, Chen M, Albrecht T, et al. DNA methylation-based classifier differentiates intrahepatic pancreato-biliary tumours. EBioMedicine (2023) 93:104657. doi: 10.1016/j.ebiom.2023.104657
63. Swain AK, Pandey P, Sera R, Yadav P. Single-cell transcriptome analysis identifies novel biomarkers involved in major liver cancer subtypes. Funct Integr Genomics (2023) 23(3):235. doi: 10.1007/s10142-023-01156-3
64. Silvestri M, Nghia Vu T, Nichetti F, Niger M, Di Cosimo S, De Braud F, et al. Comprehensive transcriptomic analysis to identify biological and clinical differences in cholangiocarcinoma. Cancer Med (2023) 12(8):10156–68. doi: 10.1002/cam4.5719
65. Wa ZC, Du T, Li XF, Xu HQ, Suo-Ang QC, Chen LD, et al. Differential diagnosis between hepatic alveolar echinococcosis and intrahepatic cholangiocarcinoma with conventional ultrasound and contrast-enhanced ultrasound. BMC Med imaging (2020) 20(1):101. doi: 10.1186/s12880-020-00499-8
66. Matake K, Yoshimitsu K, Kumazawa S, Higashida Y, Irie H, Asayama Y, et al. Usefulness of artificial neural network for differential diagnosis of hepatic masses on CT images. Acad radiology (2006) 13(8):951–62. doi: 10.1016/j.acra.2006.04.009
67. Ponnoprat D, Inkeaw P, Chaijaruwanich J, Traisathit P, Sripan P, Inmutto N, et al. Classification of hepatocellular carcinoma and intrahepatic cholangiocarcinoma based on multi-phase CT scans. Med Biol Eng computing (2020) 58(10):2497–515. doi: 10.1007/s11517-020-02229-2
68. Midya A, Chakraborty J, Srouji R, Narayan RR, Boerner T, Zheng J, et al. Computerized diagnosis of liver tumors from CT scans using a deep neural network approach. IEEE J Biomed Health informatics (2023) 27(5):2456–64. doi: 10.1109/JBHI.2023.3248489
69. Zhu Y, Mao Y, Chen J, Qiu Y, Guan Y, Wang Z, et al. Value of contrast-enhanced CT texture analysis in predicting IDH mutation status of intrahepatic cholangiocarcinoma. Sci Rep (2021) 11(1):6933. doi: 10.1038/s41598-021-86497-4
70. Shen X, Zhao H, Jin X, Chen J, Yu Z, Ramen K, et al. Development and validation of a machine learning-based nomogram for prediction of intrahepatic cholangiocarcinoma in patients with intrahepatic lithiasis. Hepatobiliary Surg Nutr (2021) 10(6):749–65. doi: 10.21037/hbsn-20-332
71. Hu R, Li H, Horng H, Thomasian NM, Jiao Z, Zhu C, et al. Automated machine learning for differentiation of hepatocellular carcinoma from intrahepatic cholangiocarcinoma on multiphasic MRI. Sci Rep (2022) 12(1):7924. doi: 10.1038/s41598-022-11997-w
72. Guo L, Li X, Zhang C, Xu Y, Han L, Zhang L. Radiomics based on dynamic contrast-enhanced magnetic resonance imaging in preoperative differentiation of combined hepatocellular-cholangiocarcinoma from hepatocellular carcinoma: A multi-center study. J hepatocellular carcinoma (2023) 10:795–806. doi: 10.2147/JHC.S406648
73. Liu Y, Wang B, Mo X, Tang K, He J, Hao J. A deep learning workflow for mass-forming intrahepatic cholangiocarcinoma and hepatocellular carcinoma classification based on MRI. Curr Oncol (Toronto Ont) (2022) 30(1):529–44. doi: 10.3390/curroncol30010042
74. Peng JB, Peng YT, Lin P, Wan D, Qin H, Li X, et al. Differentiating infected focal liver lesions from Malignant mimickers: value of ultrasound-based radiomics. Clin radiology (2022) 77(2):104–13. doi: 10.1016/j.crad.2021.10.009
75. Ren S, Li Q, Liu S, Qi Q, Duan S, Mao B, et al. Clinical value of machine learning-based ultrasomics in preoperative differentiation between hepatocellular carcinoma and intrahepatic cholangiocarcinoma: A multicenter study. Front Oncol (2021) 11:749137. doi: 10.3389/fonc.2021.749137
76. Peng Y, Lin P, Wu L, Wan D, Zhao Y, Liang L, et al. Ultrasound-based radiomics analysis for preoperatively predicting different histopathological subtypes of primary liver cancer. Front Oncol (2020) 10:1646. doi: 10.3389/fonc.2020.01646
77. Xu H, Zou X, Zhao Y, Zhang T, Tang Y, Zheng A, et al. Differentiation of intrahepatic cholangiocarcinoma and hepatic lymphoma based on radiomics and machine learning in contrast-enhanced computer tomography. Technol Cancer Res Treat (2021) 20:15330338211039125. doi: 10.1177/15330338211039125
78. Xu X, Mao Y, Tang Y, Liu Y, Xue C, Yue Q, et al. Classification of hepatocellular carcinoma and intrahepatic cholangiocarcinoma based on radiomic analysis. Comput Math Methods Med (2022) 2022:5334095. doi: 10.1155/2022/5334095
79. Liu X, Khalvati F, Namdar K, Fischer S, Lewis S, Taouli B, et al. Can machine learning radiomics provide pre-operative differentiation of combined hepatocellular cholangiocarcinoma from hepatocellular carcinoma and cholangiocarcinoma to inform optimal treatment planning? Eur Radiol (2021) 31(1):244–55. doi: 10.1007/s00330-020-07119-7
80. Huang JL, Sun Y, Wu ZH, Zhu HJ, Xia GJ, Zhu XS, et al. Differential diagnosis of hepatocellular carcinoma and intrahepatic cholangiocarcinoma based on spatial and channel attention mechanisms. J Cancer Res Clin Oncol (2023) 149(12):10161–8. doi: 10.1007/s00432-023-04935-4
81. Sun L, Zhou M, Li Q, Hu M, Wen Y, Zhang J, et al. Diagnosis of cholangiocarcinoma from microscopic hyperspectral pathological dataset by deep convolution neural networks. Methods (San Diego Calif) (2022) 202:22–30. doi: 10.1016/j.ymeth.2021.04.005
82. Wolff LI, Hachgenei E, Goßmann P, Druzenko M, Frye M, König N, et al. Optical coherence tomography combined with convolutional neural networks can differentiate between intrahepatic cholangiocarcinoma and liver parenchyma ex vivo. J Cancer Res Clin Oncol (2023) 149(10):7877–85. doi: 10.1007/s00432-023-04742-x
83. Kiani A, Uyumazturk B, Rajpurkar P, Wang A, Gao R, Jones E, et al. Impact of a deep learning assistant on the histopathologic classification of liver cancer. NPJ digital Med (2020) 3:23. doi: 10.1038/s41746-020-0232-8
84. Mavros MN, Economopoulos KP, Alexiou VG, Pawlik TM. Treatment and prognosis for patients with intrahepatic cholangiocarcinoma: systematic review and meta-analysis. JAMA surgery (2014) 149(6):565–74. doi: 10.1001/jamasurg.2013.5137
85. Bai Y, Liu ZS, Xiong JP, Xu WY, Lin JZ, Long JY, et al. Nomogram to predict overall survival after gallbladder cancer resection in China. World J gastroenterology (2018) 24(45):5167–78. doi: 10.3748/wjg.v24.i45.5167
86. Raoof M, Dumitra S, Ituarte PHG, Melstrom L, Warner SG, Fong Y, et al. Development and validation of a prognostic score for intrahepatic cholangiocarcinoma. JAMA surgery (2017) 152(5):e170117. doi: 10.1001/jamasurg.2017.0117
87. Wang D, Pan B, Huang JC, Chen Q, Cui SP, Lang R, et al. Development and validation of machine learning models for predicting prognosis and guiding individualized postoperative chemotherapy: A real-world study of distal cholangiocarcinoma. Front Oncol (2023) 13:1106029. doi: 10.3389/fonc.2023.1106029
88. Palmieri LJ, Lavolé J, Dermine S, Brezault C, Dhooge M, Barré A, et al. The choice for the optimal therapy in advanced biliary tract cancers: Chemotherapy, targeted therapies or immunotherapy. Pharmacol Ther (2020) 210:107517. doi: 10.1016/j.pharmthera.2020.107517
89. Lamarca A, Ross P, Wasan HS, Hubner RA, McNamara MG, Lopes A, et al. Advanced intrahepatic cholangiocarcinoma: post hoc analysis of the ABC-01, -02, and -03 clinical trials. J Natl Cancer Institute (2020) 112(2):200–10. doi: 10.1093/jnci/djz071
90. Bagante F, Spolverato G, Merath K, Weiss M, Alexandrescu S, Marques HP, et al. Intrahepatic cholangiocarcinoma tumor burden: A classification and regression tree model to define prognostic groups after resection. Surgery (2019) 166(6):983–90. doi: 10.1016/j.surg.2019.06.005
91. Kaibori M, Yoshii K, Kosaka H, Ota M, Komeda K, Ueno M, et al. Preoperative serum markers and risk classification in intrahepatic cholangiocarcinoma: A multicenter retrospective study. Cancers (2022) 14(21):5459. doi: 10.3390/cancers14215459
92. Tsilimigras DI, Hyer JM, Paredes AZ, Diaz A, Moris D, Guglielmi A, et al. A novel classification of intrahepatic cholangiocarcinoma phenotypes using machine learning techniques: an international multi-institutional analysis. Ann Surg Oncol (2020) 27(13):5224–32. doi: 10.1245/s10434-020-08696-z
93. Zhou SN, Jv DW, Meng XF, Zhang JJ, Liu C, Wu ZY, et al. Feasibility of machine learning-based modeling and prediction using multiple centers data to assess intrahepatic cholangiocarcinoma outcomes. Ann Med (2023) 55(1):215–23. doi: 10.1080/07853890.2022.2160008
94. Li Z, Yuan L, Zhang C, Sun J, Wang Z, Wang Y, et al. A novel prognostic scoring system of intrahepatic cholangiocarcinoma with machine learning basing on real-world data. Front Oncol (2020) 10:576901. doi: 10.3389/fonc.2020.576901
95. Müller L, Mähringer-Kunz A, Gairing SJ, Foerster F, Weinmann A, Bartsch F, et al. Survival prediction in intrahepatic cholangiocarcinoma: A proof of concept study using artificial intelligence for risk assessment. J Clin Med (2021) 10(10):2071. doi: 10.3390/jcm10102071
96. Jiang W, Zeng ZC, Tang ZY, Fan J, Sun HC, Zhou J, et al. A prognostic scoring system based on clinical features of intrahepatic cholangiocarcinoma: the Fudan score. Ann oncology: Off J Eur Soc Med Oncol (2011) 22(7):1644–52. doi: 10.1093/annonc/mdq650
97. Tang Y, Zhang T, Zhou X, Zhao Y, Xu H, Liu Y, et al. The preoperative prognostic value of the radiomics nomogram based on CT combined with machine learning in patients with intrahepatic cholangiocarcinoma. World J Surg Oncol (2021) 19(1):45. doi: 10.1186/s12957-021-02162-0
98. Tsilimigras DI, Mehta R, Moris D, Sahara K, Bagante F, Paredes AZ, et al. A machine-based approach to preoperatively identify patients with the most and least benefit associated with resection for intrahepatic cholangiocarcinoma: an international multi-institutional analysis of 1146 patients. Ann Surg Oncol (2020) 27(4):1110–9. doi: 10.1245/s10434-019-08067-3
99. Ruan J, Xu S, Chen R, Qu W, Li Q, Ye C, et al. EMLI-ICC: an ensemble machine learning-based integration algorithm for metastasis prediction and risk stratification in intrahepatic cholangiocarcinoma. Briefings Bioinf (2022) 23(6):bbac450. doi: 10.1093/bib/bbac450
100. Liu J, Liu M, Gong Y, Su S, Li M, Shu J. Prediction of angiogenesis in extrahepatic cholangiocarcinoma using MRI-based machine learning. Front Oncol (2023) 13:1048311. doi: 10.3389/fonc.2023.1048311
101. Zhang J, Wu Z, Zhang X, Liu S, Zhao J, Yuan F, et al. Machine learning: an approach to preoperatively predict PD-1/PD-L1 expression and outcome in intrahepatic cholangiocarcinoma using MRI biomarkers. ESMO Open (2020) 5(6):e000910. doi: 10.1136/esmoopen-2020-000910
102. Xie J, Pu X, He J, Qiu Y, Lu C, Gao W, et al. Survival prediction on intrahepatic cholangiocarcinoma with histomorphological analysis on the whole slide images. Comput Biol Med (2022) 146:105520. doi: 10.1016/j.compbiomed.2022.105520
103. Alaimo L, Moazzam Z, Endo Y, Lima HA, Butey SP, Ruzzenente A, et al. The application of artificial intelligence to investigate long-term outcomes and assess optimal margin width in hepatectomy for intrahepatic cholangiocarcinoma. Ann Surg Oncol (2023) 30(7):4292–301. doi: 10.1245/s10434-023-13349-y
104. Xu X, Xing Z, Xu Z, Tong Y, Wang S, Liu X, et al. A deep learning model for prediction of post hepatectomy liver failure after hemihepatectomy using preoperative contrast-enhanced computed tomography: a retrospective study. Front Med (2023) 10:1154314. doi: 10.3389/fmed.2023.1154314
105. Shao F, Huang Q, Wang C, Qiu L, Hu YG, Zha SY. Artificial neural networking model for the prediction of early occlusion of bilateral plastic stent placement for inoperable hilar cholangiocarcinoma. Surg laparoscopy endoscopy percutaneous techniques (2018) 28(2):e54–e8. doi: 10.1097/SLE.0000000000000502
106. Ibragimov B, Toesca D, Chang D, Yuan Y, Koong A, Xing L. Development of deep neural network for individualized hepatobiliary toxicity prediction after liver SBRT. Med physics (2018) 45(10):4763–74. doi: 10.1002/mp.13122
107. Plachouris D, Tzolas I, Gatos I, Papadimitroulas P, Spyridonidis T, Apostolopoulos D, et al. A deep-learning-based prediction model for the biodistribution of (90) Y microspheres in liver radioembolization. Med physics (2021) 48(11):7427–38. doi: 10.1002/mp.15270
108. Choi WJ, Williams PJ, Claasen M, Ivanics T, Englesakis M, Gallinger S, et al. Systematic review and meta-analysis of prognostic factors for early recurrence in intrahepatic cholangiocarcinoma after curative-intent resection. Ann Surg Oncol (2022). doi: 10.1016/j.ijsu.2022.106335
109. Hu HJ, Jin YW, Shrestha A, Ma WJ, Wang JK, Liu F, et al. Predictive factors of early recurrence after R0 resection of hilar cholangiocarcinoma: A single institution experience in China. Cancer Med (2019) 8(4):1567–75. doi: 10.1002/cam4.2052
110. Zhang XF, Beal EW, Bagante F, Chakedis J, Weiss M, Popescu I, et al. Early versus late recurrence of intrahepatic cholangiocarcinoma after resection with curative intent. Br J surgery (2018) 105(7):848–56. doi: 10.1002/bjs.10676
111. Alaimo L, Lima HA, Moazzam Z, Endo Y, Yang J, Ruzzenente A, et al. Development and validation of a machine-learning model to predict early recurrence of intrahepatic cholangiocarcinoma. Ann Surg Oncol (2023) 30:5406–5415. doi: 10.1245/s10434-023-13636-8
112. Ji GW, Jiao CY, Xu ZG, Li XC, Wang K, Wang XH. Development and validation of a gradient boosting machine to predict prognosis after liver resection for intrahepatic cholangiocarcinoma. BMC cancer (2022) 22(1):258. doi: 10.1186/s12885-022-09352-3
113. Jolissaint JS, Wang T, Soares KC, Chou JF, Gönen M, Pak LM, et al. Machine learning radiomics can predict early liver recurrence after resection of intrahepatic cholangiocarcinoma. HPB: Off J Int Hepato Pancreato Biliary Assoc (2022) 24(8):1341–50. doi: 10.1016/j.hpb.2022.02.004
114. Qin H, Hu X, Zhang J, Dai H, He Y, Zhao Z, et al. Machine-learning radiomics to predict early recurrence in perihilar cholangiocarcinoma after curative resection. Liver Int (2021) 41(4):837–50. doi: 10.1111/liv.14763
115. Groot Koerkamp B, Wiggers JK, Allen PJ, Busch OR, D’Angelica MI, DeMatteo RP, et al. American Joint Committee on Cancer staging for resected perihilar cholangiocarcinoma: a comparison of the 6th and 7th editions. HPB: Off J Int Hepato Pancreato Biliary Assoc (2014) 16(12):1074–82. doi: 10.1111/hpb.12320
116. Gazzaniga GM, Faggioni A, Filauro M. Surgical treatment of proximal bile duct tumors. Int surgery (1985) 70(1):45–8.
117. Jarnagin WR, Fong Y, DeMatteo RP, Gonen M, Burke EC, Bodniewicz BJ, et al. Staging, resectability, and outcome in 225 patients with hilar cholangiocarcinoma. Ann surgery (2001) 234(4):507–17. doi: 10.1097/00000658-200110000-00010
118. Bo Z, Chen B, Yang Y, Yao F, Mao Y, Yao J, et al. Machine learning radiomics to predict the early recurrence of intrahepatic cholangiocarcinoma after curative resection: A multicentre cohort study. Eur J Nucl Med Mol imaging (2023) 50(8):2501–13. doi: 10.1007/s00259-023-06184-6
119. Liang W, Xu L, Yang P, Zhang L, Wan D, Huang Q, et al. Novel nomogram for preoperative prediction of early recurrence in intrahepatic cholangiocarcinoma. Front Oncol (2018) 8:360. doi: 10.3389/fonc.2018.00360
120. Piansaddhayanon C, Koracharkornradt C, Laosaengpha N, Tao Q, Ingrungruanglert P, Israsena N, et al. Label-free tumor cells classification using deep learning and high-content imaging. Sci data (2023) 10(1):570. doi: 10.1038/s41597-023-02482-8
121. Wang Y, Shao J, Wang P, Chen L, Ying M, Chai S, et al. Deep learning radiomics to predict regional lymph node staging for hilar cholangiocarcinoma. Front Oncol (2021) 11:721460. doi: 10.3389/fonc.2021.721460
122. Huang T, Liu H, Lin Z, Kong J, Lin K, Lin Z, et al. Preoperative prediction of intrahepatic cholangiocarcinoma lymph node metastasis by means of machine learning: a multicenter study in China. BMC cancer (2022) 22(1):931. doi: 10.1186/s12885-022-10025-4
123. Xu L, Yang P, Liang W, Liu W, Wang W, Luo C, et al. A radiomics approach based on support vector machine using MR images for preoperative lymph node status evaluation in intrahepatic cholangiocarcinoma. Theranostics (2019) 9(18):5374–85. doi: 10.7150/thno.34149
124. Tang Y, Yang CM, Su S, Wang WJ, Fan LP, Shu J. Machine learning-based Radiomics analysis for differentiation degree and lymphatic node metastasis of extrahepatic cholangiocarcinoma. BMC cancer (2021) 21(1):1268. doi: 10.1186/s12885-021-08947-6
125. Gao W, Wang W, Song D, Wang K, Lian D, Yang C, et al. A multiparametric fusion deep learning model based on DCE-MRI for preoperative prediction of microvascular invasion in intrahepatic cholangiocarcinoma. J magnetic resonance imaging: JMRI (2022) 56(4):1029–39. doi: 10.1002/jmri.28126
126. Charan ES, Sharma A, Sandhu H, Garg P. FGFR1Pred: an artificial intelligence-based model for predicting fibroblast growth factor receptor 1 inhibitor. Mol Diversity (2023). doi: 10.1007/s11030-023-10714-7
127. Gerdes H, Casado P, Dokal A, Hijazi M, Akhtar N, Osuntola R, et al. Drug ranking using machine learning systematically predicts the efficacy of anti-cancer drugs. Nat Commun (2021) 12(1):1850. doi: 10.1038/s41467-021-22170-8
128. Khorsandi SE, Dokal AD, Rajeeve V, Britton DJ, Illingworth MS, Heaton N, et al. Computational analysis of cholangiocarcinoma phosphoproteomes identifies patient-specific drug targets. Cancer Res (2021) 81(22):5765–76. doi: 10.1158/0008-5472.CAN-21-0955
129. Jeong S, Ge Y, Chen J, Gao Q, Luo G, Zheng B, et al. Latent risk intrahepatic cholangiocarcinoma susceptible to adjuvant treatment after resection: A clinical deep learning approach. Front Oncol (2020) 10:143. doi: 10.3389/fonc.2020.00143
130. Zhang Z, Wang Z, Huang Y. A bibliometric analysis of 8,276 publications during the past 25 years on cholangiocarcinoma by machine learning. Front Oncol (2021) 11:687904. doi: 10.3389/fonc.2021.687904
131. Shimizu H, Nakayama KI. Artificial intelligence in oncology. Cancer science (2020) 111(5):1452–60. doi: 10.1111/cas.14377
132. Topol EJ. High-performance medicine: the convergence of human and artificial intelligence. Nat Med (2019) 25(1):44–56. doi: 10.1038/s41591-018-0300-7
133. Price WN 2nd, Gerke S, Cohen IG. Potential liability for physicians using artificial intelligence. Jama (2019) 322(18):1765–6. doi: 10.1001/jama.2019.15064
134. Nussberger AM, Luo L, Celis LE, Crockett MJ. Public attitudes value interpretability but prioritize accuracy in Artificial Intelligence. Nat Commun (2022) 13(1):5821. doi: 10.1038/s41467-022-33417-3
135. Linardatos P, Papastefanopoulos V, Kotsiantis S. Explainable AI: A review of machine learning interpretability methods. Entropy (Basel Switzerland) (2020) 23(1):18. doi: 10.3390/e23010018
Keywords: cholangiocarcinoma (CCA), machine learning (ML), artificial intelligence, prognosis, diagnosis
Citation: Huang J, Bai X, Qiu Y and He X (2024) Application of AI on cholangiocarcinoma. Front. Oncol. 14:1324222. doi: 10.3389/fonc.2024.1324222
Received: 19 October 2023; Accepted: 08 January 2024;
Published: 29 January 2024.
Edited by:
Wenqing Cao, New York University, United StatesReviewed by:
Valeria Merz, University of Verona, ItalyShilpi Minocha, Indian Institute of Technology Delhi, India
Copyright © 2024 Huang, Bai, Qiu and He. This is an open-access article distributed under the terms of the Creative Commons Attribution License (CC BY). The use, distribution or reproduction in other forums is permitted, provided the original author(s) and the copyright owner(s) are credited and that the original publication in this journal is cited, in accordance with accepted academic practice. No use, distribution or reproduction is permitted which does not comply with these terms.
*Correspondence: Xiaodong He, hxdpumch@163.com
†These authors have contributed equally to this work and share first authorship