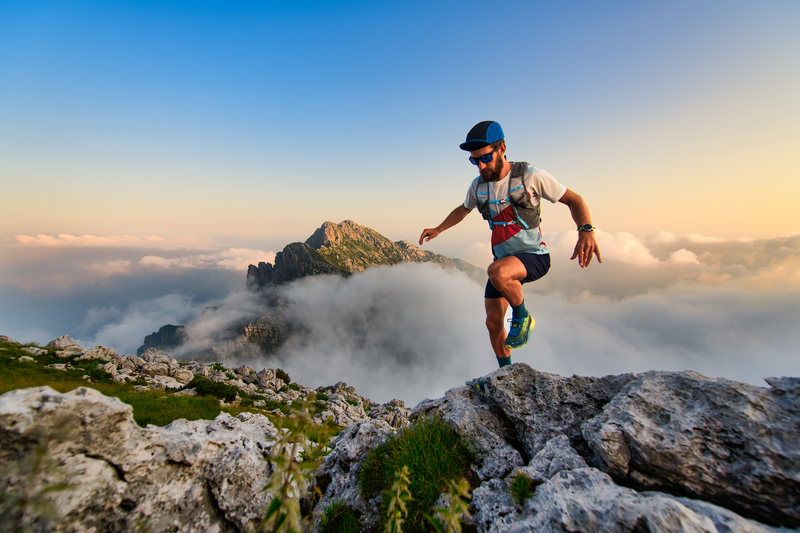
94% of researchers rate our articles as excellent or good
Learn more about the work of our research integrity team to safeguard the quality of each article we publish.
Find out more
ORIGINAL RESEARCH article
Front. Oncol. , 22 February 2024
Sec. Cancer Imaging and Image-directed Interventions
Volume 14 - 2024 | https://doi.org/10.3389/fonc.2024.1255438
This article is part of the Research Topic Precision Medical Imaging for Cancer Diagnosis and Treatment - Vol. II View all 35 articles
Objective: The aim of this study was to assess the ability of a multiparametric magnetic resonance imaging (MRI)-based radiomics signature model to predict disease-free survival (DFS) in patients with rectal cancer treated by surgery.
Materials and methods: We evaluated data of 194 patients with rectal cancer who had undergone radical surgery between April 2016 and September 2021. The mean age of all patients was 62.6 ± 9.7 years (range: 37–86 years). The study endpoint was DFS and 1132 radiomic features were extracted from preoperative MRIs, including contrast-enhanced T1- and T2-weighted imaging and apparent diffusion coefficient values. The study patients were randomly allocated to training (n=97) and validation cohorts (n=97) in a ratio of 5:5. A multivariable Cox regression model was used to generate a radiomics signature (rad score). The associations of rad score with DFS were evaluated using Kaplan–Meier analysis. Three models, namely a radiomics nomogram, radiomics signature, and clinical model, were compared using the Akaike information criterion.
Result: The rad score, which was composed of four MRI features, stratified rectal cancer patients into low- and high-risk groups and was associated with DFS in both the training (p = 0.0026) and validation sets (p = 0.036). Moreover, a radiomics nomogram model that combined rad score and independent clinical risk factors performed better (Harrell concordance index [C-index] =0.77) than a purely radiomics signature (C-index=0.73) or clinical model (C-index=0.70).
Conclusion: An MRI radiomics model that incorporates a radiomics signature and clinicopathological factors more accurately predicts DFS than does a clinical model in patients with rectal cancer.
Being one of the major causes of cancer-related death worldwide, rectal cancer is a global health problem (1, 2). About 70% of rectal cancer patients are successfully treated surgically. However, the prognosis is poor, distant metastases or local recurrence being detected in up to 30% of patients, often within a few years of surgery (3, 4). Identifying tumor characteristics that are associated with a high-risk of adverse outcomes is therefore extremely important in enabling devising risk-adapted personalized treatment strategies for rectal cancer patients.
Nowadays, the TNM classification is routinely used in clinical practice for preoperative risk stratification and treatment allocation (5, 6). However, this classification ignores tumoral spatial heterogeneity, which indicates hemorrhage, necrosis, and cell density and thus provides important guidance for decisions concerning administration of radio- and/or chemo-therapy. Radiomics involves extracting a large set of quantitative features from a series of radiological images. In particular, radiomics based on multiparametric MRI (mpMRI) has emerged as a reproducible, non-invasive means of characterizing intratumor features and assessing risk and treatment response (7).
To the best of our knowledge, only one study on using mpMRI, diffusion kurtosis imaging in particular, to predict the response to therapy in patients with rectal cancer has been published (8). Because this is rarely performed in clinical practice, there are no established standard scan parameters; thus, its generalizability and usefulness need to be further verified. Diffusion weighted imaging (DWI), a functional MRI technique, has been widely used in clinical practice.
The apparent diffusion coefficient (ADC), which is derived from DWI sequencing, reflects heterogeneity at the microscopic level, such as cell density of tumors (9). The aim of our study was to establish a model for predicting the disease-free survival (DFS) of rectal cancer treated by surgery by combining contrast-enhanced (CE)-T1WI, T2WI, ADC radiomics features, and clinical factors.
This study was approved by our institution’s ethics review board, who waived the need for patient approval and informed consent because this was a retrospective study.
This study cohort comprised 194 patients (121 men and 73 women; mean age: 62.6 ± 9.7 years; age range: 37–86 years) with histologically confirmed rectal cancer who had undergone resection in our institution between April 2016 and September 2021. The inclusion criteria were as follows: (a) preoperative conventional MRI and DWI sequences performed within 3 weeks before resection; (b) pathologically confirmed rectal cancer; and (c) follow-up at our hospital. Exclusion criteria were: (a) administration of preoperative chemoradiotherapy; (b) another cancer in addition to rectal cancer; and (c) failure to attend for follow up.
All patients were randomly allocated to training (n=97) and validation groups (n=97) in a ratio of 5:5.
We set the DFS, defined as the time from CT examination until either the date of disease progression, including distant metastasis, local tumor recurrence, or death from any cause, or until the last date known to be free of relapse (censored) as the main endpoint. All disease progression was diagnosed on the basis of findings on imaging such as abdominal CT and MRI, clinical examination, or biopsy. Our institution’s follow-up protocol is every 3–6 months during the first 2 years after surgery, every 6–9 months for the following 2–3 years, and annually thereafter. The median follow-up period of the whole cohort was 32 months (range, 4–99 months).
Rectal MRI imaging was obtained on a 3T system (Verio, Siemens, Germany) equipped with a 12-channel body coil. The MRI scanning sequence included high-resolution axial T2-weighted, DWI, and enhanced T1-weighted sequences. Detailed information concerning the acquisition parameters is provided in Supplementary Materials.
Manual segmentation of each tumor was performed using open-source ITK-SNAP software (www.itksnap.org) on data obtained from axial T2WI, ADC, and CE-T1WI slices. Region of interest (ROI) segmentation was performed by two experienced radiologists who examined the whole tumor and avoided necrotic tissue and bleeding (Figure 1).
Figure 1 MR images of a 53-yearold man with rectal cancer. Examples of tumors with MRI (A, C) and 3D segmentation (B, D).
Radiomics feature extraction was preprocessed by a pyradiomics package (http://www.PyRadiomics.readthedocs.io/en/latest/) that comprises four groups of features. In total, 1132 radiomic features, including ROI shape, intensity, texture, and wavelet features, were then extracted from multiple image sequences from each patient. To obtain a standardized normal distribution of the MRI image intensities, multiple images were normalized by z-score after manual ROI segmentation of the tumor.
We used a four-step process for feature selection and to identify robust DFS-associated radiomic features. Intraclass correlation coefficients of >0.75 were considered to denote high inter-/intra-observer stability and kept for subsequent analyses. Univariate Cox regression analyses were then conducted to identify statistically significant DFS-related radiomic features (p ≤ 0.05). Next, Spearman’s correlation analysis with r≥0.90 was used to eliminate redundancy. Finally, multivariate Cox analysis was performed to develop independent predictors of DFS. Radiomics signatures (defined as rad scores) were computed through a linear combination of each selected feature with non-zero coefficients.
All statistical analyses were analyzed with R software, version 3.6.3 (http://www.R-project.org). Continuous and categorical variables were compared between the training and validation sets by using an independent samples t-test, Mann–Whitney U-test, or χ2 test as appropriate. DFS probabilities were assessed by Kaplan–Meier analysis and the differences between high- and low-risk groups were compared with the log-rank test. The optimal cutoff value according to the rad score and X-tile was used to divide patients into low- and high-risk groups. The Harrell concordance index (C-index) was calculated to quantify the model’s ability to discriminate. Decision curve analysis was used to evaluate the clinical usefulness of various models. A two-sided p<0.05 was regarded as denoting statistical significance.
Selected clinico-radiological characteristics of the 194 study patients are shown in Table 1.
Table 1 Clinical characteristics of patients with rectal cancer in the training data set and validation data set.
The median follow-up period of the whole cohort was 32 months (range, 4–99 months). The relationships between survival time, age, and rad-score are shown in Figure 2.
Four potential predictors including one, one, and two features were selected from the T2WI, ADC and CE-T1WI to build a radiomics signature based on a formula for calculating radiomics score (Supplementary Materials).
According to the rad score optimum cut-off point generated by X-tile plot, we further classified patients into high- (rad score ≥ −0.7) and low-risk groups (rad-score < −0.7), and performed Kaplan–Meier analysis in the training and validation sets to determine the ability of the rad score to predict prognosis. The distributions of the high- and low-risk rad scores of the included four features are shown in Figure 3.
Figure 3 Plots (A–D) illustrate the distributions of the high- and low-risk rad scores of the included four features.
Lower rad scores were associated with better DFS in both the training (p = 0.0026) and validation sets (p = 0.036) (Figure 4).
Figure 4 Kaplan–Meier survival analysis based on high risk and low risk rad scores in training set (A) and validation set (B).
Three models (radiomics nomogram, clinical, radiomics signature) were assessed in the training and validation sets. The ability of each model to discriminate was then evaluated by the C-index in the validation set. Only three clinical features, namely age, CA199, and pN, were used in the clinical model. The abilities of the radiomics nomogram and clinical models to predict DFS are shown in Figure 5. The clinical and radiomics signature models alone had similar discriminatory capability (C-index: 0.70 vs. 0.73). However, a radiomics nomogram model incorporating the radiomics signature and clinical model was better able to discriminate DFS in the validation cohort (C-index, 0.77) than was either the radiomic signature or clinical model alone (Table 2).
Figure 5 Plots illustrate radiomics nomogram (A) and clinical model nomogram (B) for the prediction of predicting DFS.
Decision curve analysis also revealed that the radiomics nomogram model achieved a higher net benefit than did the clinical model or radiomics signature in predicting DFS (Figure 6).
The cumulative 3-year DFS rates were 42.7% versus 63.6%, respectively, (p < 0.05), for high- versus low-risk patients in the training set, and 48.1% versus 60.0%, respectively, (p < 0.05) in the validation set. Subsequently, the cumulative 5-year DFS rates were 10.7% versus 36.4%, respectively, (p < 0.05), for high- versus low-risk patients in the training set and 13.0% versus 45.0%, respectively, (p < 0.05) in the validation set.
Radiomics features, which are noninvasively acquired high-dimension features from radiological images, are closely associated with treatment response (10), prognosis (11), and molecular phenotypes (12). Previous studies have found that radiomics can predict individual responses to neoadjuvant therapy for rectal cancer (13–15). Several studies have shown that radiomics models have promising prognostic value in patients with rectal cancer (8, 16). However, because diffusion kurtosis imaging is rarely used in clinical practice, there is a lack of standard scan parameters. This means that the generalizability and clinical relevance of using radiomics models based on features identified by this modality to predict responses to therapy require further verification. Furthermore, the above-cited studies did not examine all stages of rectal cancer treated by surgery.
Giving this background, we constructed a multi-feature-based radiomics signature extracted from mpMRI to evaluate the prognostic value of radiomics in patients with rectal cancer. Our clinical and radiomics signature models had similar discriminatory capability (C-index: 0.70 vs. 0.73). However, a combination of the radiomics nomogram model incorporating the radiomics signature and our clinical model improved the discriminating power for DFS in the validation cohort, as evidenced by a higher C-index (0.77), lower Akaike information criterion (781.5), and improvement in reclassification. This is in line with previous research (13, 17, 18) and demonstrates that the radiomics signature has an incremental value in risk stratification for predicting DFS.
Additionally, rad scores can stratify patients into high- and low-risk groups. Lower rad scores (< −0.7) in patients with rectal cancer were generally associated with better DFS, suggesting that some high-risk patients should receive risk-adapted personalized treatment strategies such as neoadjuvant chemoradiotherapy or adjuvant chemoradiotherapy. Moreover, rad scores were significantly associated with DFS (p<0.05), showing that they can be a useful tool for individualized estimation of survival of patients with rectal cancer.
Intriguingly, the four radiomics features selected for the integrated radiomics model were all wavelet-based, which is consistent with recent studies (19–21). This shows that almost all high-dimensional features are wavelet-based. Wavelet transformation enables quantification of high-dimensional tumor information, which is difficult to explain intuitively (21, 22). In one study (7), the researchers constructed two types of radiomics signatures: with and without wavelet features. However, there was no evidence that the model with wavelet features improved the accuracy of prediction; this may be attributable to redundant radiomics features (23). Most high-dimensional features are not perceptible visually; however, they have been used successfully in radiomics-based prediction of survival (24, 25), recurrence (26), and gene expression (27).
The present study had several limitations. First, the sample size was relatively small. Second, because this was a retrospective, single-center study, our findings need to be further validated by drawing prospectively on a large-scale multicenter database. Finally, stratification of other risk factors derived from staging and pathologic type may provide more accurate estimation of risk of survival and recurrence.
In conclusion, mpMRI radiomics improves prediction of prognosis in patients with rectal cancer. Our comprehensive model including clinical features provides additional prognostic information beyond a clinical model alone. Further prospective studies and clinical validation are required.
The original contributions presented in the study are included in the article/Supplementary Material. Further inquiries can be directed to the corresponding author.
The studies involving humans were approved by Academic Committee of Shaoxing People’s Hospital. The studies were conducted in accordance with the local legislation and institutional requirements. The participants provided their written informed consent to participate in this study. Written informed consent was obtained from the individual(s) for the publication of any potentially identifiable images or data included in this article.
JM: Writing – original draft. WY: Writing – original draft. WM: Methodology, Writing – original draft. JL: Software, Writing – original draft. WZ: Software, Writing – original draft. HY: Visualization, Writing – original draft. TL: Visualization, Writing – original draft. LG: Writing – original draft. DW: Writing – original draft.
The author(s) declare financial support was received for the research, authorship, and/or publication of this article. This study was supported by grants from the Medical and Health Science and Technology Plan Project of Zhejiang Province (#2022KY1296, 2022KY1287) and Clinical Research Fund Project of Zhejiang Medical Association (#2020ZYC-B17).
The authors declare that the research was conducted in the absence of any commercial or financial relationships that could be construed as a potential conflict of interest.
All claims expressed in this article are solely those of the authors and do not necessarily represent those of their affiliated organizations, or those of the publisher, the editors and the reviewers. Any product that may be evaluated in this article, or claim that may be made by its manufacturer, is not guaranteed or endorsed by the publisher.
The Supplementary Material for this article can be found online at: https://www.frontiersin.org/articles/10.3389/fonc.2024.1255438/full#supplementary-material
1. Hotta M, Minamimoto R, Gohda Y, Miwa K, Otani K, Kiyomatsu T, et al. Prognostic value of (18)F-FDG PET/CT with texture analysis in patients with rectal cancer treated by surgery. Ann Nucl Med. (2021) 35:843–52. doi: 10.1007/s12149-021-01622-7.
2. Jemal A, Siegel RL, Miller KD. Cancer statistics, 2007. CA Cancer J Clin. (2007) 57:43–66. doi: 10.3322/canjclin.57.1.43.
3. Sargent DJ, Wieand HS, Haller DG, Gray R, Benedetti JK, Buyse M, et al. Disease-free survival versus overall survival as a primary end point for adjuvant colon cancer studies: individual patient data from 20,898 patients on 18 randomized trials. J Clin Oncol. (2005) 23:8664–70. doi: 10.1200/JCO.2005.01.6071.
4. Yang ZF, Wu DQ, Wang JJ, Lv ZJ, Li Y. Short- and long-term outcomes following laparoscopic vs open surgery for pathological T4 colorectal cancer: 10 years of experience in a single center. World J Gastroenterol. (2018) 24:76–86. doi: 10.3748/wjg.v24.i1.76.
5. Horvat N, Carlos Tavares Rocha C, Clemente Oliveira B, Petkovska I, Gollub MJ. MRI of rectal cancer: tumor staging, imaging techniques, and management. Radiographics. (2019) 39:367–87. doi: 10.1148/rg.2019180114.
6. Sun Y, Lin H, Lu X, Huang Y, Xu Z, Huang S, et al. A nomogram to predict distant metastasis after neoadjuvant chemoradiotherapy and radical surgery in patients with locally advanced rectal cancer. J Surg Oncol. (2017) 115:462–9. doi: 10.1002/jso.24522.
7. Choe J, Lee SM, Do KH, Kim S, Choi S, Lee JG, et al. Outcome prediction in resectable lung adenocarcinoma patients: value of CT radiomics. Eur Radiol. (2020) 30:4952–63. doi: 10.1007/s00330-020-06872-z.
8. Cui Y, Wang G, Ren J, Hou L, Li D, Wen Q, et al. Radiomics features at multiparametric MRI predict disease-free survival in patients with locally advanced rectal cancer. Acad Radiol. (2022) 29:128–38. doi: 10.1016/j.acra.2021.11.024.
9. Bo J, Jia H, Zhang Y, Fu B, Jiang X, Chen Y, et al. Preoperative prediction value of pelvic lymph node metastasis of endometrial cancer: combining of ADC value and radiomics features of the primary lesion and clinical parameters. J Oncol. (2022) 2022:3335048. doi: 10.1155/2022/3335048.
10. Liu Z, Zhang XY, Shi YJ, Wang L, Zhu HT, Tang Z, et al. Radiomics analysis for evaluation of pathological complete response to neoadjuvant chemoradiotherapy in locally advanced rectal cancer. Clin Cancer Res. (2017) 23:7253–62. doi: 10.1158/1078-0432.CCR-17-1038.
11. Kickingereder P, Götz M, Muschelli J, Wick A, Neuberger U, Shinohara RT, et al. Large-scale radiomic profiling of recurrent glioblastoma identifies an imaging predictor for stratifying anti-angiogenic treatment response. Clin Cancer Res. (2016) 22:5765–71. doi: 10.1158/1078-0432.CCR-16-0702.
12. Huang C, Cintra M, Brennan K, Zhou M, Colevas AD, Fischbein N, et al. Development and validation of radiomic signatures of head and neck squamous cell carcinoma molecular features and subtypes. EBioMedicine. (2019) 45:70–80. doi: 10.1016/j.ebiom.2019.06.034.
13. Liu Z, Meng X, Zhang H, Li Z, Liu J, Sun K, et al. Predicting distant metastasis and chemotherapy benefit in locally advanced rectal cancer. Nat Commun. (2020) 11:4308. doi: 10.1038/s41467-020-18162-9.
14. Cui Y, Yang W, Ren J, Li D, Du X, Zhang J, et al. Prognostic value of multiparametric MRI-based radiomics model: Potential role for chemotherapeutic benefits in locally advanced rectal cancer. Radiother Oncol. (2021) 154:161–9. doi: 10.1016/j.radonc.2020.09.039.
15. Zhang Z, Jiang X, Zhang R, Yu T, Liu S, Luo Y, et al. Radiomics signature as a new biomarker for preoperative prediction of neoadjuvant chemoradiotherapy response in locally advanced rectal cancer. Diagn Interv Radiol. (2021) 27:308–14. doi: 10.5152/dir.
16. Lovinfosse P, Polus M, Van Daele D, Martinive P, Daenen F, Hatt M, et al. FDG PET/CT radiomics for predicting the outcome of locally advanced rectal cancer. Eur J Nucl Med Mol Imaging. (2018) 45:365–75. doi: 10.1007/s00259-017-3855-5.
17. Huang Y, Liu Z, He L, Chen X, Pan D, Ma Z, et al. Radiomics signature: A potential biomarker for the prediction of disease-free survival in early-stage (I or II) non-small cell lung cancer. Radiology. (2016) 281:947–57. doi: 10.1148/radiol.2016152234.
18. Zhuang Z, Liu Z, Li J, Wang X, Xie P, Xiong F, et al. Radiomic signature of the FOWARC trial predicts pathological response to neoadjuvant treatment in rectal cancer. J Transl Med. (2021) 19:256. doi: 10.1186/s12967-021-02919-x.
19. Cui Y, Zhang J, Li Z, Wei K, Lei Y, Ren J, et al. A CT-based deep learning radiomics nomogram for predicting the response to neoadjuvant chemotherapy in patients with locally advanced gastric cancer: A multicenter cohort study. EClinicalMedicine. (2022) 46:101348. doi: 10.1016/j.eclinm.2022.101348.
20. Fang J, Zhang B, Wang S, Jin Y, Wang F, Ding Y, et al. Association of MRI-derived radiomic biomarker with disease-free survival in patients with early-stage cervical cancer. Theranostics. (2020) 10:2284–92. doi: 10.7150/thno.37429.
21. Zhang J, Wang G, Ren J, Yang Z, Li D, Cui Y, et al. Multiparametric MRI-based radiomics nomogram for preoperative prediction of lymphovascular invasion and clinical outcomes in patients with breast invasive ductal carcinoma. Eur Radiol. (2022) 32:4079–89. doi: 10.1007/s00330-021-08504-6.
22. Wu Q, Wang S, Chen X, Wang Y, Dong L, Liu Z, et al. Radiomics analysis of magnetic resonance imaging improves diagnostic performance of lymph node metastasis in patients with cervical cancer. Radiother Oncol. (2019) 138:141–8. doi: 10.1016/j.radonc.2019.04.035.
23. Berenguer R, Pastor-Juan MDR, Canales-Vázquez J, Castro-García M, Villas MV, Mansilla Legorburo F, et al. Radiomics of CT features may be nonreproducible and redundant: influence of CT acquisition parameters. Radiology. (2018) 288:407–15. doi: 10.1148/radiol.2018172361.
24. Liu Z, Cao Y, Diao W, Cheng Y, Jia Z, Peng X. Radiomics-based prediction of survival in patients with head and neck squamous cell carcinoma based on pre- and post-treatment (18)F-PET/CT. Aging (Albany NY). (2020) 12:14593–619. doi: 10.18632/aging.v12i14.
25. Wang H, Li X, Yuan Y, Tong Y, Zhu S, Huang R, et al. Association of machine learning ultrasound radiomics and disease outcome in triple negative breast cancer. Am J Cancer Res. (2022) 12:152–64.
26. Lucia F, Visvikis D, Vallières M, Desseroit MC, Miranda O, Robin P, et al. External validation of a combined PET and MRI radiomics model for prediction of recurrence in cervical cancer patients treated with chemoradiotherapy. Eur J Nucl Med Mol Imaging. (2019) 46:864–77. doi: 10.1007/s00259-018-4231-9.
Keywords: rectal cancer, radiomics, prognosis, disease-free survival, MRI
Citation: Mao J, Ye W, Ma W, Liu J, Zhong W, Yuan H, Li T, Guan L and Wu D (2024) Prediction by a multiparametric magnetic resonance imaging-based radiomics signature model of disease-free survival in patients with rectal cancer treated by surgery. Front. Oncol. 14:1255438. doi: 10.3389/fonc.2024.1255438
Received: 08 July 2023; Accepted: 31 January 2024;
Published: 22 February 2024.
Edited by:
Alla Reznik, Lakehead University, CanadaReviewed by:
Shreya Shukla, Tata Memorial Hospital, IndiaCopyright © 2024 Mao, Ye, Ma, Liu, Zhong, Yuan, Li, Guan and Wu. This is an open-access article distributed under the terms of the Creative Commons Attribution License (CC BY). The use, distribution or reproduction in other forums is permitted, provided the original author(s) and the copyright owner(s) are credited and that the original publication in this journal is cited, in accordance with accepted academic practice. No use, distribution or reproduction is permitted which does not comply with these terms.
*Correspondence: Dongping Wu, d3VkcDY2NjZAMTYzLmNvbQ==
†These authors have contributed equally to this work
Disclaimer: All claims expressed in this article are solely those of the authors and do not necessarily represent those of their affiliated organizations, or those of the publisher, the editors and the reviewers. Any product that may be evaluated in this article or claim that may be made by its manufacturer is not guaranteed or endorsed by the publisher.
Research integrity at Frontiers
Learn more about the work of our research integrity team to safeguard the quality of each article we publish.