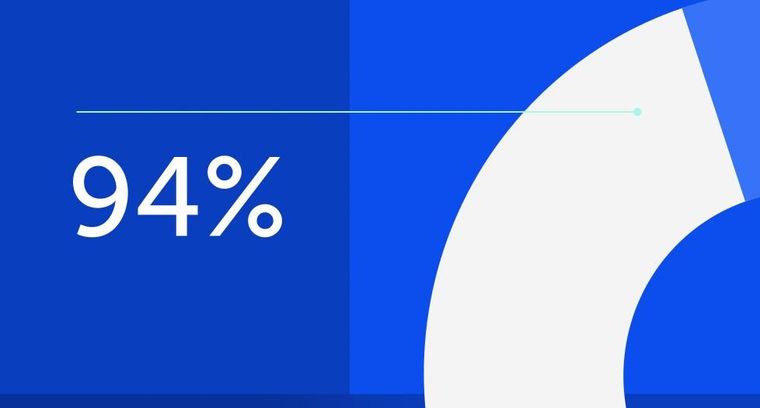
94% of researchers rate our articles as excellent or good
Learn more about the work of our research integrity team to safeguard the quality of each article we publish.
Find out more
REVIEW article
Front. Oncol., 21 February 2023
Sec. Breast Cancer
Volume 13 - 2023 | https://doi.org/10.3389/fonc.2023.993540
This article is part of the Research TopicPrecision Medical Imaging for Cancer Diagnosis and TreatmentView all 20 articles
Breast cancer is the leading cause of cancer in women with a huge medical, social and economic impact. Mammography (MMG) has been the gold standard method until now because it is relatively inexpensive and widely available. However, MMG suffers from certain limitations, such as exposure to X-rays and difficulty of interpretation in dense breasts. Among other imaging methods, MRI has clearly the highest sensitivity and specificity, and breast MRI is the gold standard for the investigation and management of suspicious lesions revealed by MMG. Despite this performance, MRI, which does not rely on X-rays, is not used for screening except for a well-defined category of women at risk, because of its high cost and limited availability. In addition, the standard approach to breast MRI relies on Dynamic Contrast Enhanced (DCE) MRI with the injection of Gadolinium based contrast agents (GBCA), which have their own contraindications and can lead to deposit of gadolinium in tissues, including the brain, when examinations are repeated. On the other hand, diffusion MRI of breast, which provides information on tissue microstructure and tumor perfusion without the use of contrast agents, has been shown to offer higher specificity than DCE MRI with similar sensitivity, superior to MMG. Diffusion MRI thus appears to be a promising alternative approach to breast cancer screening, with the primary goal of eliminating with a very high probability the existence of a life-threatening lesion. To achieve this goal, it is first necessary to standardize the protocols for acquisition and analysis of diffusion MRI data, which have been found to vary largely in the literature. Second, the accessibility and cost-effectiveness of MRI examinations must be significantly improved, which may become possible with the development of dedicated low-field MRI units for breast cancer screening. In this article, we will first review the principles and current status of diffusion MRI, comparing its clinical performance with MMG and DCE MRI. We will then look at how breast diffusion MRI could be implemented and standardized to optimize accuracy of results. Finally, we will discuss how a dedicated, low-cost prototype of breast MRI system could be implemented and introduced to the healthcare market.
Screening has been shown as an effective method to improve the outcome of breast cancer, the leading cause of cancer in women. Mammography is the preferred method due to its low cost and favorable benefit/risk ratio. However, mammography has some limitations, such as exposure to X-rays, difficulty of interpretation in dense breasts, and overdiagnosis. Among other imaging methods, MRI has clearly the highest sensitivity and specificity. Still, MRI is mainly used to manage suspicious lesions revealed by mammography and not for screening, except for a category of well-defined women at risk, due to a high cost and a limited availability. While the standard breast MRI approach relies on the injection of contrast agents, which have their own contraindications, diffusion MRI which delivers information on tissue microstructure and tumor perfusion without the need for contrast agents, has been shown to provide a similar specificity and sensitivity, emerging as a promising alternative approach to breast cancer screening. To achieve this goal, it is necessary to standardize protocols for acquisition and analysis of diffusion MRI data. Second, the accessibility and cost-effectiveness of MRI examinations need to improve significantly, which may become possible with the development of dedicated breast, low-cost units for breast cancer screening.
With the advent of widespread breast cancer screening by mammography (MMG) in the early to mid-1980s, detection of breast lesions has increased worldwide, and breast cancer is no longer a fatal disease when diagnosed and treated early. Approximately 60% of cancers diagnosed early have a 5-year survival of 99% after treatment and 31% have a 5-year survival of 85% (1). Breast cancer screening has therefore been shown to be an effective method of improving prognosis. In the absence of a reliable blood test, imaging is the primary approach available for screening. MMG has been the reference method until now because it is relatively inexpensive, widely available and has a favorable benefit/risk ratio with good sensitivity and specificity. Nevertheless, MMG suffers from certain limitations, such as exposure to X-rays given the recent discovery that breast tissue is more sensitive to the effects of radiation than most organs. In addition, with MMG, it is often not possible to predict on mammograms whether lesions are malignant, requiring active treatment, or not, so additional investigations must be performed, especially in dense breasts.
Of particular concern is the relatively high rate of overdiagnosis. Recent immunohistochemical studies have revealed that benign proliferative breast disease, most high-grade ductal carcinoma in situ (DCIS), and invasive carcinoma develop along distinct pathways, in contrast to colonic adenoma-carcinoma, which evolves along a single line (2). These findings suggest that different treatment approaches should be offered depending on the nature of the lesion, including therapeutic abstention for benign lesions. For example, while DCIS lesions often do not become invasive, patients diagnosed with DCIS are generally treated as if they were going to have invasive carcinoma. The rate of “overdiagnosis” is estimated to be between 21 and 66% (3). The social, ethical, and economic consequences of such management of DCIS lesions are enormous: more than 40% of women with DCIS undergo mastectomies, at a rate of some 10,000 per year, so much so that DCIS could be called a “mammographic disease” (4). Clearly, new approaches must be sought to better predict the grade and outcome of diagnosed breast lesions and to reduce burdensome, costly, and potentially unnecessary surgical procedures, such as mastectomy or axillary lymph node excision, whose morbidity is not negligible. It would also reduce surgical scars that could lead to pseudo-lesions on subsequent imaging. Conversely, the sensitivity of MMG for early detection of cancer in breast cancer screening is only 33% (40% for ultrasound) in patients with a high familial risk for breast cancer (lifetime risk ≧ 20%), missing some prognostically important diseases (5). Borderline lesions with uncertain malignant potential at biopsy [histologically classified as “B3”, (6)] most often result in a benign end result. However, these lesions are sometimes associated with the simultaneous presence of a malignant tumor with an enhancement rate of between 10 and 35%, and may also act as a risk factor or precursor to malignancy (7, 8). It is therefore necessary to obtain a more accurate classification of lesions at the time of initial diagnosis in order to personalize the therapeutic approach, avoid unnecessary procedures and reduce costs and social burden. With MMG, it is possible to suspect high-grade lesions from the morphology of microcalcifications, but grading is still difficult, with sparse biopsy sampling, because high-grade and low-grade components can coexist in the same patient or even in the same duct. Indeed, MMG may tend to detect slow-growing cancers.
Recently, breast MRI has been successfully introduced in the management of breast cancer. For example, in DCIS, the sensitivity of MRI for accurate assessment of the extent of DCIS is as high as 89%, much higher than MMG, tomosynthesis, or ultrasound (9). Increasing evidence suggests that, overall, breast MRI may be more sensitive, especially for the diagnosis of high-grade DCIS. Breast MRI is often performed by injection of gadolinium-based contrast agents (GBCA), but more recently, diffusion MRI, a completely noninvasive approach that is highly sensitive to changes in tissue microstructure, has been introduced for cancer imaging. Diffusion MRI has both very high sensitivity and specificity for the detection of breast malignancy (10). Diffusion MRI has been successfully used to differentiate between benign and malignant lesions of the breast, as well as tumor extension.
Yet MRI is exceptionally used for breast cancer screening, although supplemental MRI screening in women with extremely dense breast tissue and normal results on MMG has been recommended, as the addition of MRI leads to significantly fewer interval cancers than MMG alone during a 2-year screening period (11). Still, the main problem with breast MRI is that examinations are today performed using expensive general purpose MRI scanners. MRI is therefore performed as a second-line procedure, which adds to the cost of other imaging modalities (MMG and ultrasound), or in specific populations of women. In addition, there are concerns about the side effects of GBCA when performing dynamic contrast-enhanced (DCE) breast MRI (12). Blood tests may become available to screen for certain breast cancers, but they remain largely non-specific today with many false positives or negatives, and imaging will always remain mandatory to localize lesions and personalize treatment. If a dedicated, small-scale, inexpensive breast MRI scanner can be made available, it could be envisaged that one day MRI could be used as a screening imaging modality, instead of MMG, at least for a larger number of women at moderate to high risk based on personal history, genetic predisposition, or positivity to blood screening tests when these tests become reliable. This view was enthusiastically supported by an international (EU, USA, Asia) committee of breast imaging experts appointed by the European Society of Breast Imaging (EUSOBI) under the chairmanship of Profs. Denis Le Bihan and Julia Camps-Herrero (13). Breast cancer screening represents a huge market. In the United States alone, more than 60 million women over the age of 40 are responsible for 40 million mammograms per year, which corresponds to 65% of the population concerned (14). In contrast, MRI (using standard whole-body systems) accounted for only 0.4% of women aged 25-64 years in 2017 (15).
In this article, we will first review the principles and current status of diffusion MRI of the breast, and evaluate its clinical performance compared with MMG and DCE MRI. We will then discuss how diffusion MRI of the breast could be implemented and standardized to optimize accuracy of results. Finally, we will discuss how a dedicated, low-cost prototype breast MRI system could be implemented and introduced to the healthcare market.
Breast MRI has been widely available after the introduction of the use of contrast agents (16). Almost all types of breast cancer show detectable patterns of neovascularization with GBCA, which can readily extravasate into the extravascular and extracellular space (17). Thus, the likelihood of breast cancer can be considered extremely low in the absence of contrast enhancement. In practice, contrast-enhanced T1-weighted MRI is the gold standard. Many malignant breast lesions show maximal contrast enhancement in the early phase after injection, with GBCA being removed from the tissue in the late stage (Figure 1). Conversely, benign lesions and normal fibroglandular tissue usually show maximal enhancement in the late stage and of lower amplitude than in malignant lesions, allowing differentiation of these lesions (19). Given the high sensitivity for detection of breast cancer compared to other modalities such as MMG and ultrasound, breast MRI is also used for preoperative evaluation and tumor staging prior to treatment planning, monitoring tumor response to neoadjuvant therapies, to sort scars from recurrences, or in the presence of implants (20, 21).
Figure 1 DCE-MRI in a 71-year-old Woman with grade 2 invasive ductal carcinoma in the right breast. (A) Axial contrast-enhanced T1w MRI image shows a 15-mm irregular mass (arrows). (B) Color axial maximum-intensity-projection MR image overlaid over the R1 breast mass. A computer-aided detection (CAD) algorithm displays areas in red, yellow, and blue indicating rapid washout-type delayed enhancement, plateau-type delayed enhancement, and persistent-type delayed enhancement patterns, respectively. (C) Graph of the contrast agent uptake shows a rapid initial enhancement and a rapid washout-type curve. The initial peak enhancement value was 119%. With respect to the delayed phase enhancement, 39% of the mass showed washout, 4% of the mass showed a persistent-type curve, and 58% showed a plateau-type curve. Adapted from (18).
However, despite its good clinical performance, MRI is usually performed in second intention. Breast cancer screening by MRI is therefore reserved for women with a moderate to high risk of breast cancer (personal history, genetic predisposition, follow-up after breast conserving surgery or contralateral breast screening, mediastinal irradiation, as for Hodgkin’s disease, suspicion of specific lesions, such as atypical ductal hyperplasia (ADH), atypical lobular hyperplasia (ALH), and lobular carcinoma in situ (LCIS) (22, 23). A first problem is the cost of MRI scans. Efforts have been made to shorten their duration [shortened DCE protocols, (24)]. However, there are also questions regarding GBCA-related side effects. The primary concern regarding nephrogenic systemic fibrosis (NSF) has almost disappeared, at least for DCE after assessment of renal function (25, 26), as it occurred only in patients with impaired renal dysfunction, and only seven of the 639 cases of patients with biopsy-confirmed NSF to date were discovered after 2008 (with the avoidance of the use of double and triple doses of GBCA that could trigger NSF) (27). The second concern is related to gadolinium retention in tissues, particularly the brain, after repeated exposure to GBCA (28). This risk is particularly important when considering the repeated annual injection of GBCA that would be required for screening (29). Various new approaches are being investigated to mitigate this risk, such as reducing the dose of GBCA. In a recent study, all breast cancers in 41 consecutive women with biopsy-proven breast cancer were detected as small as 0.4 cm with half (0.05 mmol/kg) a dose of gadobutrol on 3T DCE breast MRI (30).
Thus, there is a growing trend toward the use of new approaches based on unenhanced breast MRI for cancer detection (29). Although no consensus has yet been reached, these approaches could open up breast cancer screening to women at intermediate or even low risk for breast cancer. Given its high potential, diffusion MRI would be the obvious candidate for such an approach.
While the concept of diffusion MRI emerged in the mid-1980s, diffusion MRI has become a mainstay of modern clinical imaging. Diffusion MRI is both a powerful method and concept because diffusing water molecules provide unique information about the microscopic architecture of tissues. Water diffusion is significantly diminished in most malignant tissues, and diffusion MRI, which requires no tracer injection, is rapidly becoming the modality of choice for detecting, characterizing, or even classifying malignant lesions, especially in the breast (31). Diffusion MRI is deeply rooted in the concept that, during their diffusion-induced movements, molecules probe the structure of tissues at a microscopic scale, well beyond the usual millimeter resolution of images. During typical diffusion imaging times of about 50-100 ms, water molecules move through tissues on average over distances of about 1-15 μm, bouncing off, passing through, or interacting with many tissue components, such as cell membranes, fibers, or macromolecules. Due to the tortuous movement of water molecules around these obstacles (“hindered” diffusion), the actual diffusion distance is reduced compared to free water. Therefore, non-invasive observation of water diffusion-induced displacement distributions in vivo provides unique clues to the fine structural features and geometric organization of cells in tissues, as well as to changes in these features as a function of physiological or pathological states.
MRI signals can be sensitized to diffusion by applying a pair of sharp magnetic field gradient pulses, the duration and separation of which can be adjusted to achieve a specific level of diffusion sensitization defined as the “b-value.” By acquiring data with different gradient pulse amplitudes, images with different degrees of diffusion sensitivity are obtained. The overall effect of diffusion in the presence of these gradient pulses is a signal attenuation and the MRI signal becomes “diffusion weighted”, hence the term “Diffusion Weighted Imaging” (DWI). The signal attenuation is more pronounced when large values of b are used and when diffusion is fast (because molecules diffuse over larger distances) (Figure 2). It is important to note that only the displacement (diffusion) component in the direction of the gradient pulses is detectable, but the diffusion can be anisotropic.
Figure 2 Diffusion attenuation versus b value. (A) Signal attenuation as a function of b value (logarithmic scale). With free diffusion we expect a straight line, whose slope is the diffusion coefficient. In tissues, diffusion is not free (non-Gaussian), resulting in a curvature. Therefore, the ADC taken from b=0 and any b values will decrease when b increases. The effects of IVIM, which result in a curvature at very low b values (<200s/mm²), are not shown for clarity. (B) Example of a breast tumor showing that indeed the ADC value decreases when using larger b values (reprinted with permission from 32).
In DWI, qualitative contrast depends not only on diffusion, but also on other MRI parameters, such as T1 and T2 water relaxation times, which can lead to well-known artifacts, such as the “T2-shine-through” effect, as high T2 signal lesions (e.g., necrosis, cysts) can retain a relatively high signal level at high b values. Therefore, these images are often combined numerically to determine a quantitative estimate of the diffusion process in each image location, through an Apparent Diffusion Coefficient (ADC), “apparent” because diffusion is impeded by many processes (33):
where S(b0) and S(b1) are the signals (in a voxel or region of interest, ROI) acquired at the b values b0 and b1, respectively. This simple ADC is an incredibly robust and powerful parameter, which has been widely used in all clinical applications of diffusion MRI since its inception (34). The optimal value of b1 that provides the best contrast-to-noise ratio in breast tissue, i.e., sufficient attenuation of the signal by scattering while maintaining a sufficient signal level is about 800s/mm² (13).
Beyond molecular diffusion, blood microcirculation in capillary networks (perfusion) also contributes to the diffusion MRI signal. Indeed, the flow of blood water in pseudo-randomly oriented capillaries (at the voxel level) mimics a random walk (“pseudo-diffusion”) which leads to an attenuation of the signal in the presence of diffusion encoding gradient pulses. This effect has been named IntraVoxel Incoherent Motion (IVIM) (35). In the presence of blood microcirculation, the global attenuation of the MRI signal, S(b)/S(0), becomes the sum of two components, one for tissue diffusion and one for the blood compartment:
where fIVIM is the fraction of circulating blood, D* is the pseudo-diffusion coefficient attributed to the random microcirculation of blood, D is the diffusion coefficient of water in tissue, and Dblood is the diffusion coefficient of water in blood. The perfusion effect is observed only at low values of b, because the pseudo-diffusion coefficient, D*, associated with blood flow is higher than the water diffusion coefficient and decreases more rapidly with the b-value.
IVIM MRI has become an important modality for perfusion imaging, with applications throughout the body (31, 36), particularly in cancer imaging (detection of neovascularization and treatment efficacy). A key feature of IVIM diffusion MRI is that it does not involve contrast agents, and it may serve as an attractive alternative to perfusion MRI in some patients with contraindications to contrast agents, or in patients with renal insufficiency at risk for NSF (see above).
Another important feature of diffusion MRI, which should be considered, counter-intuitively, as an advantage and not as a limitation, is that the ADC value depends on the acquisition parameters, especially the b-value, because diffusion in tissues is not “free” but “hindered”. With free (or “Gaussian”) diffusion, as in a cyst, the ADC remains the same regardless of the set of b values used to measure it (only the accuracy of ADC estimates changes with b values). However, in most tissues, the ADC value decreases as the diffusion sensitivity is increased by the b value (32) (Figure 2).
The reason is that an increasing number of molecules slowed down by their interaction with microstructural tissue components (fibers, cell membranes) during their diffusion movements become visible in the highly diffusion-sensitized MRI signal. This non-Gaussian diffusion behavior is therefore more pronounced when high b values are used. In short, sticking to the “optimal” b-value (e.g. 800s/mm²) deprives one of the potentially valuable clinical information about tissue microstructure encoded in the “non-Gaussian diffusion” provided by higher b-values. To reveal this hidden information about tissue microstructure, one must rely on models other than the standard ADC. There are essentially two types of such models. Some approaches aim to model the diffusion MRI signal biophysically, based on the different tissue compartments present in the tissue, as with NODDI (Neurite Orientation Dispersion and Density Imaging) used in the brain (37). The other way is simply to model the decay of the scattering signal mathematically, empirically, without any assumptions about the underlying biophysical properties of the tissue. Although several models have been proposed (38), the most popular approach simply quantifies the deviation of the scattering signal behavior from an ideal Gaussian behavior. This is the so-called Kurtosis model (39), also called Diffusion Kurtosis Imaging, DKI (40). With the Kurtosis model, which also includes the IVIM effect, the signal is described as follows:
ADC0 is the extrapolated ADC value as b approaches 0 and K is the Kurtosis quantifying the deviation from Gaussian scattering (K=0 for Gaussian diffusion). Kurtosis has shown great potential for characterizing pathological or physiological conditions (41). A major drawback of DKI, however, is that it requires the acquisition of large data sets with multiple values of b to be fitted with equation (3), which significantly increases acquisition times, a premium in clinical practice.
However, it is possible to obtain quantitative information about non-Gaussian diffusion with data sets acquired with a limited range of b values. For example, using data acquired for only 2 b values, one can calculate a shifted ADC (sADC). The concept of sADC (31) is based on the use of shifted key b-values (200 and 1500s/mm² for the breast, instead of 0 and 800s/mm²) providing an interesting balance between Gaussian and non-Gaussian diffusion effects. This approach has been evaluated for the breast (42). Another approach, S-index, provides a direct classification of tissue types by calculating a distance between the acquired signals and a library of reference (“signature”) signals from known or simulated tissues (e.g., benign, malignant, etc.) by intrinsically accounting for Gaussian and non-Gaussian diffusion effects, without the need for any mathematical or biophysical modeling (43). This approach has also been shown to provide the immunohistochemical status and molecular subtypes of invasive breast carcinomas (44) (Figure 3).
Figure 3 S-index. (A) Invasive ductal carcinoma of luminal A type in 50-year-old woman. The axial early-phase DCE-MRI image shows a mass with an irregular margin (top, arrow). The three-dimensional rendering voxel-by-voxel S-index image (bottom) shows the entire tumor in reddish color, corresponding to an average signature index (S-index) of this mass was 90.4. (B) Human epidermal growth factor receptor 2 enriched invasive ductal carcinoma in 73-year-old woman. The axial early-phase DCE-MRI image shows non-mass enhancement with a heterogeneous internal pattern in the right breast (top, arrow). The three-dimensional S-index rendering map of the entire tumor exhibits a yellow-green color (mean S-index of 55.8). [Adapted from (44)].
It is also possible to estimate the main parameters of the IVIM/Kurtosis model described by equation [3], fIVIM, ADCo and K, without fitting, using a limited set of 4 b values (b0, b1, b2 and b3 in ascending order), providing the signal:noise ratio is not too low. The proposed algorithm assumes that IVIM effects become negligible in signals acquired above b1 and that non-Gaussian diffusion effects appear visible in b2 and b3 signals. According to this 4b algorithm the model parameters estimates can be calculated as:
where D1=ln[S(0)/S(b1)]/(b1-b0); D2=ln[S(0)/S(b2)]/(b2-b0); D3=ln(S(0)/S(b3)/(b3-b0); H=(D2-D3)/(b3-b2)+ln(1-F)/(b3.b2); F= 1-exp[-(D1-D2)(b1.b2)/(b2-b1)]; A= (D2-D3)/(b3-b2)+ln(1- fIVIM)/(b3.b2).
In the absence of IVIM and non-Gaussian diffusion effects one obviously has D1=D2=D3=sADC, ADCo=sADC and K=0.
If non-Gaussian diffusion is present without IVIM effects (fIVIM=0) ADCo and K are obtained exactly as:
A graphical interpretation of this set of equations can be given by plotting the (log) of the (curved) signal attenuation versus the b value and the straight lines corresponding to D1, D2, D3 and sADC (Figure 4). In the absence of IVIM and non-Gaussian diffusion effects the signal attenuation follows a straight line with a slope D1=D2=D3=sADC=ADCo. In the presence of IVIM effects only the curvature at low b values creates an angle between the D1 and D2 lines. From this angle fIVIM can be estimated (Eq. 4) while ADCo remains very close to the sADC (Eq. 6). In the presence of non-Gaussian diffusion only the curvature at high b values forms an angle between the D2 and D3 lines, from which K can be estimated (Eq. 7). However, one can see that fIVIM and non-Gaussian diffusion slightly contribute also to the angle between the D2 and D3 lines, and the angle between the D1 and D2 lines, respectively. Hence, estimated fIVIM and K values must be corrected (variables H and A in Eq. 4, 6). Note that the sADC now includes ADCo, fIVIM and K effects, so that the ADCo values derived from sADC must be corrected (A variable). Also, with this algorithm D* cannot be estimated, however, a review of the literature shows that D* is a parameter difficult to estimate even with the full fitting approach, resulting in extremely variable clinical relevance.
Figure 4 Graphical representation of the 4b-diffusion MRI abbreviated protocol. The plots show the signal attenuation and the straight lines associated to the intermediate calculation parameters (slopes) D1, D2, D3 used to estimate fIVIM, ADCo and K, as well as sADC. In the presence of free diffusion (K=0) and in the absence of IVIM effects (top left) the signal attenuation follows a straight line whose slope is ADCo. D1, D2, D3 and sADC are all equal to ADCo. When IVIM effects appear (top right) the D1 line starts to deviate from the signal attenuation curve with an angle with D2 reflecting fIVIM. With non-Gaussian diffusion effects only, both D1 and D3 deviate from D2 with an angle reflecting K (bottom left). The sADC line depends on ADCo and K. When both IVIM and non-Gaussian diffusion effects are present one can see that the angle between D1 and D2 primarily reflects fIVIM while the D2D3 angle mainly reflects K (bottom right). The sADC now reflects ADCo, K and fIVIM. By combining sADC with D1, D2 and D3 one can get accurate estimates of ADCo, K and fIVIM using equation (4-7).
It is expected that those abbreviated quantitative DWI protocols will play a major role, in addition to qualitative DWI, such as DWIBS (see below), in the context of breast cancer screening with diffusion MRI.
Molecular mobility in biological tissues may not be the same in all directions, which is referred to as diffusion anisotropy. In the breast diffusion anisotropy can arise from the geometric organization of the glandular tissue around ducts. To characterize the effects of anisotropy, diffusion-weighted images must be sensitized to diffusion along multiple directions (at least 6) within the Diffusion Tensor Imaging (DTI) (45) With DTI one gets information on the tissue mean diffusivity, MD, which is equivalent to an orientation invariant ADC, and lambda values (λ1, λ2, and λ3) which give diffusivity along the main diffusivity directions (so-called eigenvectors ϵ1, ϵ2, and ϵ3). The eigenvector ϵ1, associated with the highest λ value, λ1, is aligned along the main orientation of aligned structures (e.g. ducts), allowing to produce maps showing their orientation in space. Some vendors propose to estimate the MD from a set of 3 orthogonal directions, but this is an approximation that should not be used in the presence of strong anisotropy effects. The genuine mean diffusivity is simply the average of the 3 λ values. The other important parameter, called Fractional Anisotropy (FA), quantifies the degree of anisotropy (FA = 0 indicates that diffusion is isotropic). It is calculated from the λ values. Whereas the existence of diffusion anisotropy in fibroglandular breast tissue has been claimed by many groups (46–49), the nature of the anatomical features which might cause this anisotropy remains somewhat controversial. Some studies have shown that breast cancer lesions could be associated with significantly lower FA values relative to normal breast tissue, and that λ1 or (λ1–λ3) could overperform the ADC (or MD) for lesion detection and classification (50, 51). However, one has to keep in mind that λ1 and λ3 (and FA which depends on them) are, by principle, highly sensitive to noise because of the strongly non-linear nature of the DTI calculation algorithm. The mere fact that MD values are lower in malignant lesions than in normal tissue might lead to reduced FA values, which should not necessarily be interpreted as “reduced anisotropy” (32).
There is an extensive literature on breast diffusion MRI. We give below a brief summary of the highlights. Many more details can be found in (10). In addition, a survey of the implementation of breast DWI in clinical practice from the EUSOBI has recently been published (52).
Most often diffusion MRI is used qualitatively for lesion detection. Lesion detection can be achieved from DWI acquired with high b values, which have a higher contrast between breast lesions (which appear bright) and normal parenchyma (dark background). Breast cancer detection using DWI has been shown to be more sensitive than MMG, with the DWI screening approach allowing to detect mammographically occult cancers (53–55) and DWI has been shown to detect significantly more contralateral breast cancers in women with unilateral breast cancer than MMG (56). High b-values are also useful in decreasing false-positive breast cancer cases (57).
A variant of the DWI techniques for qualitative lesion detection is DWIBS (Diffusion-weighted Whole-body Imaging with Background body signal). A previous study in 280 patients has shown that the diagnostic performance using non-contrast technique including DWIBS for breast lesion detection (sensitivity, specificity, diagnostic accuracy, Positive Predictive Value (PPV) and Negative Predictive Value (NPV) values of 94%, 79%, 86%, 79% and 94%, respectively) was comparable to that of DCE-MRI (sensitivity, specificity, diagnostic accuracy, PPV and NPV values of 98%, 83%, 90%, 84% and 98%, respectively) (58). DWIBS performed with Maximum Intensity Projection (MIP) mapping also has a comparable diagnostic performance (sensitivity, specificity, PPV and NPV values of 92%, 94%, 93%, and 92%, respectively) to that of DCE-MRI performed with MIP (sensitivity, specificity, PPV and NPV values of 85%, 90%, 89%, and 87%, respectively). MIP-DWIBS has been shown to rule out previously suggested malignancy on screening MMGs in 50 participants with carcinoma in 24 patients (59).
Nevertheless, a unique feature of breast DWI is its quantitative assessment capability. As the most popular quantitative marker, the ADC can be used as a threshold to sort out benign from malignant lesions (13, 60, 61), but also to build a lexicon to describe and classify lesions, for instance to distinguish breast cancers from benign lesions (13). Many groups have also found significant differences of ADC values between benign and metastatic breast lymph nodes (62–64), however, their diagnostic performance in differentiating these lymph nodes still need further investigation (64) compared to simpler markers such as the lymph node size. Quantitative DWI in addition to DCE‐MRI and other plain MRI such as T1WI and T2WI also leads to improved diagnostic performance, in terms of specificity for BI-RADS (Breast Imaging-Reporting And Data System) 3 and 4 lesions, or evaluating malignancies with BI-RADS 4 lesions (65, 66).
DWI is often used in multiparametric protocols in combination with other MRI modalities, such as DCE-MRI, contributing to improve overall diagnostic specificity and accuracy over DCE-MRI alone (67), especially when examining non-Gaussian diffusion (42) (Figure 5). The combination of DCE-MRI and DWI could increase diagnostic accuracy in characterization of non-mass-like enhancement lesions (68). It has also been reported that DWI combined with T2WI improved the diagnostic specificity of enhancing lesions incidentally detected in breast DCE-MRI (69), and that multiparametric DWI outcome parameters have associations with molecular prognostic factors or subtypes (70, 71).
Advanced diffusion markers (IVIM, Kurtosis, DTI) can further increase diagnostic performance, although there are not yet used in routine clinical practice (Figures 3, 5). IVIM parameters have been shown to provide a high diagnostic performance in differentiating benign and malignant breast tumors (sensitivity = 86%, specificity = 86%, AUC = 0.91 for D, sensitivity = 80%, specificity = 76%, AUC = 0.85 for f, and sensitivity = 84%, specificity = 59%, AUC = 0.71 for D*) (72), especially in combination with DCE-MRI (73), and IVIM parameters are known to be correlated with DCE-MRI parameters (74). IVIM histogram parameters have been shown to be associated with molecular prognostic factors (75, 76). Regarding DKI higher K and lower MD values are usually observed in malignant compared to benign lesions (41, 77), DKI was found to be useful in the differentiation of additional suspicious lesions at preoperative breast MRI (78). In 2 recent meta-analyses of DKI studies (79, 80) the sensitivity and specificity of K and MD to differentiate malignant from brewing breast lesions were found to be around 89-90% and 86-88% for K, and 84-86% and 83-88% for MD. The utility of DKI in differentiating molecular prognostic factors (81) or predicting treatment response (82) has also been reported.
Figure 5 Example of non-Gaussian diffusion MRI maps in breast lesions. Images in a 72-year-old woman with invasive ductal carcinoma. (A) Dynamic contrast-enhanced axial MR image, (B) fIVIM map, (C) ADC0 map, and (D) K map. The white rectangle on (A) shows the area covered by the parametric maps. (B) fIVIM distribution is heterogeneous inside the tumor. The peripheral area of the tumor exhibits low ADC0 (C) and high K values (D), suggesting high cellularity diffusion hindrance effect (likely from cellular membranes) corresponding to the viable malignant component (also high fIVIM values), whereas the central part shows high ADC0 and low K, suggesting lower cellularity (possible necrosis with fluid motion at the center resulting in high fIVIM values). [Adapted from (42)].
As for DTI parameters, malignant breast lesions have significantly lower MD and λ1 values compared to benign lesions (46). Indeed, λ1 and MD are known to have a high diagnostic performance in differentiating malignant and benign breast lesions (AUC 0.97, sensitivity 93%, specificity 92% for λ1 and AUC 0.92, sensitivity 87%, specificity 83% for D (50), although this trend might not be related to diffusion anisotropy [see above (32)]. Similarly, the use of FA to differentiate between malignant and benign breast tumors remains controversial, suggesting that caution should be exercised in the use of this parameter, although several studies have suggested its usefulness to sort out malignant and benign lesions (14, 50). Yamaguchi et al. (83) also reported higher FA in lesions with more favorable prognostic factors, such as positive estrogen receptor status, lower nuclear grade and cancer intrinsic subtype, and reduced DTI metrics had association with poor prognostic factors of breast cancer (84). A correlation between DTI parameters and molecular prognostic factors (estrogen receptor status or Ki-67) has been shown (49) and DTI has been investigated to differentiate recurrent breast cancer from post operative changes with breast-conserving surgery in patients (85).
In reviewing the literature, the overall sensitivity of DWI alone is very high, approaching 90% for detection of breast malignancy (with a specificity of approximately 82%, superior to any other imaging modality, including GBCA MRI) (51).
Many investigators have studied the potential of DWI alone for non-contrast cancer detection. The performance of DWI imaging for cancer detection is variable across studies, with a mean sensitivity of 81% (range 44-97%) and a mean specificity of 88% (range 73-96%) (86). This variation could be due to the diversity of the study population as well as the image acquisition protocols, highlighting the need for standardization (see below). Nonetheless, DWI based primarily on qualitative assessment is less sensitive than DCE MRI (mean sensitivity of 80 vs. 90s % for DWI vs. DCE MRI in studies (54, 58, 59, 67, 87–93). This situation is entertaining the idea that diffusion MRI would be difficult as a stand-alone modality compared to DCE-MRI and multiparametric MRI (67). However, in reviewing the literature, the overall sensitivity of quantitative ADC alone is very high, approaching 90% for detection of breast malignancy (with a specificity of approximately 82%, superior to any other imaging modality, including GBCA-MRI) (51). In any case, with respect to screening, diffusion MRI offers much better clinical performance than MMG or ultrasound (94). This is an important point, considering that repeated use of GBCA would be a problem for screening. Not only does DWI remain more sensitive than MMG across studies (52, 92, 95, 96), but mammography-occluded breast cancers are better represented with DWI than with ultrasound (94).
Despite this good clinical performance, it may seem surprising that DWI has not yet been recommended to be used as a stand-alone modality for breast cancer evaluation, let alone for breast cancer screening. Diffusion MRI is not even included in the BI-RADS lexicon used to assess breast lesions from GBCA MRI (97), although it is considered useful (52). The main reason is likely the high variability of the results found in the literature, especially with regard to ADC values (13, 98). The EUSOBI international committee on breast DWI has provided guidelines for obtaining optimized and consistent results (13). This report, along with the EUSOBI survey (52) have pointed out to an urgent need for standardization of DWI acquisition and processing protocols to achieve consistent results among breast DWI users.
Technical advances in MRI scanners, particularly for gradient hardware and fast imaging, facilitate the exploration of new features beyond ADC by allowing perfusion-driven IVIM to become more reliable (99), providing access to non-Gaussian diffusion through high b-values, and investigating diffusion time effects. This increasing flexibility of diffusion MRI acquisitions is supporting the expansion of more complex models, allowing for a better understanding of the relationship between diffusion MRI parameters and the microscopic characteristics of the underlying tissue. This is particularly true in the field of breast imaging, where a wide variety of diffusion MRI techniques have great potential for clinical applications in the breast field. However, this flexibility implies that some normalization must be implemented in order to compare quantitative results obtained at multiple sites. Not only are ADC values strongly dependent on b-values (100–102), but they are also influenced by TE, due to differences in T2 values between tissue components. Hidden parameters, such as diffusion time (set by the duration and intervals of the gradient pulses) also have important effects. For example, while high performance gradient hardware can achieve high b-values with shorter TEs, increasing the signal-to-noise ratio, diffusion contrast may be partially lost, as diffusion hindrance decreases with short diffusion time (103). Thus, there is a clear need for standardization of acquisition protocols. Validation of these protocols in different clinical sites would benefit from calibrated phantoms, as suggested by EUSOBI (the European Society of Breast Imaging) (13), QIBA (Quantitative Imaging Biomarkers Alliance) organized by the Radiological Society of North America (RSNA) (104). Clearly, additional efforts are needed in collaboration with vendors if consensus is to be reached on optimal acquisition parameters for diffusion MRI of the breast (10).
Single-shot echo-planar imaging (EPI) is currently the method of choice for in vivo diffusion imaging, as it allows efficient and ultrafast acquisition of multiple diffusion-weighted images (different b-values) without in-plane motion artifacts, to which diffusion MRI is notoriously sensitive. Nevertheless, EPI has several limitations related to spatial resolution, artifacts, and signal-to-noise ratio. In particular, small breast lesions (<2 mm) may be undetectable. In addition, EPI requires a very homogeneous magnetic field. For breast imaging, field inhomogeneities may be more pronounced at the air/tissue interface in the anterior part of the breast, resulting in local image distortion or signal loss. Another source of geometric distortion comes from eddy currents induced by the switching of strong diffusion encoding gradient pulses. Therefore, the degree of geometric distortion increases with the b-value. This geometric distortion must be corrected before performing any quantitative analysis involving multiple values of b to avoid artifacts around small lesions, especially at high spatial resolution. Segmented EPI acquisitions (e.g., ‘RESOLVE’ (Readout Segmentation of Long Variable Echo-trains) (105) can overcome these limitations at the cost of longer diffusion times and a sensitivity to motion between acquired segments that must be corrected using ad-hoc approaches during image reconstruction. Parallel acquisition techniques, which allow simultaneous signal collection using an array of multiple RF coils, can also address these limitations. Incorrect fat suppression can also lead to misinterpretation of diffusion MRI, as residual fat present in breast tumors results in low diffusion values, mimicking malignancy, visually and quantitatively (ADC values). The Spectrally Adiabatic Inversion Recovery (SPAIR) method has been recommended for breast imaging (13).
Efforts are also needed on the image processing side. Diffusion-weighted images are often noisy, especially for high b-values, because the signal is strongly attenuated by the diffusion effect. Noise is a vicious enemy because it is not always visible, while having a profound impact on the values of the parameters estimated with the various models available including ADC. For high b-values, due to the nature of the MRI signal (a “magnitude” signal that cannot be negative), there always remains a background noise signal and the diffusion signal remains above a threshold, the “noise floor”, instead of asymptotically approaching 0, resulting in underestimated ADC values. If one classifies lesions (e.g., benign or malignant) on the basis of ADC threshold values, it is easy to see that this trap of underestimated ADC could lead to a significant bias toward the “malignant” nature of lesions. Therefore, an adequate signal-to-noise ratio must be ensured, e.g., by increasing the voxel size (at the expense of spatial resolution) or by repeating image acquisitions at high b-values for signal averaging before amplitude reconstruction (which unfortunately increases acquisition time). Finally, background noise effects must also be removed from the signals before analysis, especially in images acquired at high b-values (106). Noise effects may partly explain the discrepancies in the literature on the different reported values of diffusion MRI and IVIM parameters. Image preprocessing could also include steps to correct for motion artifacts and geometric distortion before the signals can be processed to calculate ADC values or estimate parameters for advanced DWI models. Another problem with clinical diffusion MRI is that quantitative analysis is often performed remotely on workstations and not on the acquisition console, which is cumbersome. Efforts are underway by vendors to provide dedicated tools for breast DWI [see the final chapters of the book (10)].
DWI data analysis would also benefit from recent developments in artificial intelligence (AI). Various approaches are being investigated for breast MRI, as well as remarkably increasing applications of convolutional neural network models (107) and machine learning (108). For example, a recent study showed that DWI radiomic classifiers for differentiating suspicious lesions in 50 asymptomatic women screened with MMG outperformed the average ADC, with an area under the curve (AUC) of receiver operating characteristics (ROC) of 84.2%/85.1% for unconstrained/constrained radiomic classifiers compared with 77.4% for the average ADC (109). The AI-based multiparametric MRI approach, including DCE, T2WI, and DWI, had better diagnostic performance (AUC ROC area of 0.852) than ultrafast DCE alone (0.811) (110). Machine learning with multiparametric MRI (DCE, DWI, and T2WI) also found that several features, including those of DWI (minimum ADC), were relevant features for predicting residual cancer burden (111). Whole breast segmentation on DWI data from different institutions and scanner types was also found to be effective using deep learning methods, which could facilitate computer-assisted quantitative analyses of DWI images of the breast (112).
Given the outstanding clinical performance of breast MRI, which has much higher sensitivity and specificity than MMG and does not rely on x-rays, it should ideally be the screening modality of choice for many women. Unfortunately, breast MRI remains expensive when performed using general-purpose body MRI scanners operating at 1.5T or even 3T. The cost (and limited availability) of these scanners prohibits the use of MRI as a screening modality (the cost today is approximately $1000 for a 40-minute exam). Breast cancer screening with MRI is therefore reserved for women with moderate to high risk of breast cancer, as detailed above. However, if a small-scale, inexpensive, dedicated breast MRI scanner were available, MRI could be used as a screening imaging modality, rather than MMG, for more women, such as women with dense breasts or a family history of breast cancer.
One issue that comes to mind when considering breast cancer screening with MRI is the use of GBCA, as examinations will need to be repeated over many years, knowing that an accumulation of gadolinium deposits in the brain or other organs in patients who have received multiple injections of contrast agents has been demonstrated. For this reason, several groups have considered the possibility of using diffusion MRI as a stand-alone imaging modality for breast cancer screening (55, 59). As detailed above, diffusion MRI, which is completely noninvasive, has been successfully used to differentiate between benign and malignant breast lesions and tumor extension. Diffusion MRI also has the potential to detect many occult mammographic and clinical carcinomas of the breast, making it a preferred modality for cancer screening. Contrast agents could then still be used, but as a second line if necessary.
A major technical implication of using diffusion MRI instead of GBCA MRI is that only one breast can be scanned at a time, as with MMG, making the design of a dedicated breast MRI scanner much easier, smaller, and therefore available at much lower cost. Here we propose some specifications that might be kept in mind when designing such a dedicated imaging system. Ideally, the device should be small to be mobile and affordable. In total, the footprint of the system should also be small compared to the 5-gauss line. Patients could be in a standing position, as a bed structure would increase space and cost (Figure 6). This will also shorten the examination time and therefore reduce imaging costs. The disadvantage is that breast motion (which is already a problem with conventional MRI) will have to be controlled mechanically (motion sensors) and/or using ad hoc post-processing algorithms. Field homogeneity should be < 1ppm/20cm peak-to-peak (0.05 ppm after shimming). This is a very important requirement because breast MRI requires “fat suppression” techniques that rely on the differential frequencies between fast and water resonance frequencies. In addition, thoracic bones and air contained in the lungs are responsible for local magnetic susceptibility effects that distort the magnetic field. As with general MRI, the field stability must be better than 0.05ppm/h (10-4ppm/10 minutes). An open design will also allow image-guided biopsy or therapy (113). The field strength should be low to keep construction and maintenance costs as low as possible, ideally using helium-free magnets. This means that several technical improvements must be implemented to maintain sufficient signal-to-noise ratios, especially when using high diffusion weighting (large b values). Efficient and powerful gradient hardware must be implemented to achieve high b-values while maintaining a short TE. Innovative radio frequency systems will need to be designed for both transmission and reception. For example, receive coil arrays could be tailored to different breast sizes to maximize fill factor, such as “bra coils.” AI algorithms that have been developed for acquisition (sparse sampling) and signal processing (114) will help maintain adequate signal-to-noise levels while achieving spatial resolution greater than 2 mm. To exploit the full content of the diffusion MRI signal, one can even envision that processing will be performed not on the reconstructed images (which are only for the eyes of radiologists and clinicians), but on the denoised raw signals using AI algorithms trained and optimized to detect disease signatures. The images will then be reconstructed by focusing on these anomalies when they are detected. Assuming that no suspicious lesions will be found in the vast majority of cases, radiologists will be able to focus on the remaining cases that the AI system will identify as difficult to classify.
Figure 6 Prototype of a MRI magnet dedicated to breast cancer screening. The superconducting magnet consists in 2 halves. The patient stands between the 2 halves. To reduce size and cost, this magnet can be tailored for scanning one breast at a time (courtesy T. Schild, Irfu/CEA).
Clearly, designing such a prototype is a team effort. Clinicians must work closely with physicists, engineers and technicians, not only to design the most patient-friendly system, but also with market attention. The price of the overall system should be similar to that of high-end mammography systems, around 400 k€. In addition to the cost of building a proof-of-concept prototype, costs for patenting, multi-center trials and market research, calibration and quality control, FDA (US Food and Drug Administration) and CE (Conformité Européenne) marking, etc., must be considered. We sincerely hope that some vendors will be interested in this challenge, invest and bring such a breast MRI screening device to the market for the benefit of patients worldwide.
Non-contrast breast diffusion MRI has emerged as a potential alternative for breast cancer screening and lesion characterization. Without GBCA injections and with higher sensitivity and specificity than MMG, breast diffusion MRI is emerging as an ideal imaging modality for cancer screening. Consensus is needed to define the population categories that could benefit from this approach, such as women at moderate to high risk for cancer. Efforts are still needed to standardize acquisition and processing protocols and to decrease the cost of breast MRI examinations. To this end, the development of a low-cost MRI system dedicated to DWI for breast cancer screening is an option that should be seriously considered.
Conception or design of the work. MI, DB. Materials collection. MI, DB. Materials analysis and interpretation. MI, DB. Drafting the article. MI, DB. Critical revision of the article. MI, DB. Final approval of the version to be published. MI, DB. All authors contributed to the article and approved the submitted version.
This study was supported in-part by the Program for the Development of Next-generation Leading Scientists with Global Insight (L-INSIGHT), sponsored by the Ministry of Education, Culture, Sports, Science and Technology (MEXT), Japan.
We would like to thank Ms. Rena Nakayama for contributing to edit this manuscript.
The authors declare that the research was conducted in the absence of any commercial or financial relationships that could be construed as a potential conflict of interest.
All claims expressed in this article are solely those of the authors and do not necessarily represent those of their affiliated organizations, or those of the publisher, the editors and the reviewers. Any product that may be evaluated in this article, or claim that may be made by its manufacturer, is not guaranteed or endorsed by the publisher.
1. Siegel RL, Miller KD, Fuchs HE, Jemal A. Cancer statistics, 2022. CA Cancer J Clin (2022) 72:7–33. doi: 10.3322/caac.21708
2. Casasent AK, Edgerton M, Navin NE. Genome evolution in ductal carcinoma in situ: Invasion of the clones. J Pathol (2017) 241:208–18. doi: 10.1002/path.4840
3. van Luijt PA, Heijnsdijk EAM, Fracheboud J, Overbeek LIH, Broeders MJM, Wesseling J, et al. The distribution of ductal carcinoma in situ (DCIS) grade in 4232 women and its impact on overdiagnosis in breast cancer screening. Breast Cancer Res (2016) 18:47. doi: 10.1186/s13058-016-0705-5
4. Park HL, Chang J, Lal G, Lal K, Ziogas A, Anton-Culver H. Trends in treatment patterns and clinical outcomes in young women diagnosed with ductal carcinoma in situ. Clin Breast Cancer (2018) 18:e179–85. doi: 10.1016/j.clbc.2017.08.001
5. Kuhl CK, Schrading S, Leutner CC, Morakkabati-Spitz N, Wardelmann E, Fimmers R, et al. Mammography, breast ultrasound, and magnetic resonance imaging for surveillance of women at high familial risk for breast cancer. J Clin Oncol (2005) 23:8469–76. doi: 10.1200/JCO.2004.00.4960
6. Pinder SE, Shaaban A, Deb R, Desai A, Gandhi A, Lee AHS, et al. NHS Breast screening multidisciplinary working group guidelines for the diagnosis and management of breast lesions of uncertain malignant potential on core biopsy (B3 lesions). Clin Radiol (2018) 73:682–92. doi: 10.1016/j.crad.2018.04.004
7. Giannotti E, James JJ, Chen Y, Sun R, Karuppiah A, Yemm J, et al. Effectiveness of percutaneous vacuum-assisted excision (VAE) of breast lesions of uncertain malignant potential (B3 lesions) as an alternative to open surgical biopsy. Eur Radiol (2021) 31:9540–7. doi: 10.1007/s00330-021-08060-z
8. Bianchi S, Caini S, Renne G, Cassano E, Ambrogetti D, Cattani MG, et al. Positive predictive value for malignancy on surgical excision of breast lesions of uncertain malignant potential (B3) diagnosed by stereotactic vacuum-assisted needle core biopsy (VANCB): A large multi-institutional study in Italy. Breast (2011) 20:264–70. doi: 10.1016/j.breast.2010.12.003
9. Lehman CD. Magnetic resonance imaging in the evaluation of ductal carcinoma in situ. J Natl Cancer Inst Monogr (2010) 2010:150–1. doi: 10.1093/jncimonographs/lgq030
10. Iima M, Partridge S, Le Bihan D. Diffusion MRI of the Breast. Philadelphia elsevier health sciences. Philadelphia (2022). p. 260.
11. Bakker MF, de Lange SV, Pijnappel RM, Mann RM, Peeters PHM, Monninkhof EM, et al. Supplemental MRI screening for women with extremely dense breast tissue. N Engl J Med (2019) 381:2091–102. doi: 10.1056/NEJMoa1903986
12. Sardanelli F, Schiaffino S, Cozzi A, Carbonaro LA. “Gadolinium-based contrast agents for breast MRI and uncertainties about brain gadolinium retention.,”. Breast MRI High-risk Screening. (2020) p:63–82. doi: 10.1007/978-3-030-41207-4_5
13. Baltzer P, Mann RM, Iima M, Sigmund EE, Clauser P, Gilbert FJ, et al. Diffusion-weighted imaging of the breast-a consensus and mission statement from the EUSOBI international breast diffusion-weighted imaging working group. Eur Radiol (2020) 30:1436–50. doi: 10.1007/s00330-019-06510-3
14. Lotter W, Diab AR, Haslam B, Kim JG, Grisot G, Wu E, et al. Robust breast cancer detection in mammography and digital breast tomosynthesis using an annotation-efficient deep learning approach. Nat Med (2021) 27:244–9. doi: 10.1038/s41591-020-01174-9
15. Lee MV, Aharon S, Kim K, Sunn Konstantinoff K, Appleton CM, Stwalley D, et al. Recent trends in screening breast MRI. J Breast Imaging (2022) 4:39–47. doi: 10.1093/jbi/wbab088
16. Kaiser W. MRI Of the female breast. First Clin results. Arch Int Physiol Biochim (1985) 93:67–76.
17. Knopp MV, Weiss E, Sinn HP, Mattern J, Junkermann H, Radeleff J, et al. Pathophysiologic basis of contrast enhancement in breast tumors. J Magn Reson Imaging (1999) 10:260–6. doi: 10.1002/(SICI)1522-2586(199909)10:3<260::AID-JMRI6>3.0.CO;2-7
18. Nam SY, Ko ES, Lim Y, Han B-K, Ko EY, Choi JS, et al. Preoperative dynamic breast magnetic resonance imaging kinetic features using computer-aided diagnosis: Association with survival outcome and tumor aggressiveness in patients with invasive breast cancer. PloS One (2018) 13:e0195756. doi: 10.1371/journal.pone.0195756
19. Kuhl CK, Mielcareck P, Klaschik S, Leutner C, Wardelmann E, Gieseke J, et al. Dynamic breast MR imaging: Are signal intensity time course data useful for differential diagnosis of enhancing lesions? Radiology (1999) 211:101–10. doi: 10.1148/radiology.211.1.r99ap38101
20. Leithner D, Wengert GJ, Helbich TH, Thakur S, Ochoa-Albiztegui RE, Morris EA, et al. Clinical role of breast MRI now and going forward. Clin Radiol (2018) 73:700–14. doi: 10.1016/j.crad.2017.10.021
21. Clauser P, Mann R, Athanasiou A, Prosch H, Pinker K, Dietzel M, et al. A survey by the European society of breast imaging on the utilisation of breast MRI in clinical practice. Eur Radiol (2018) 28:1909–18. doi: 10.1007/s00330-017-5121-4
22. Weinstein SP, Slanetz PJ, Lewin AA, Battaglia T, Chagpar AB, Dayaratna S, et al. ACR appropriateness criteria® supplemental breast cancer screening based on breast density. J Am Coll Radiol (2021) 18:S456–73. doi: 10.1016/j.jacr.2021.09.002
23. Rahman WT, Helvie MA. Breast cancer screening in average and high-risk women. Best Pract Res Clin Obstet Gynaecol (2022) 83:3–14. doi: 10.1016/j.bpobgyn.2021.11.007
24. Kuhl CK, Schrading S, Strobel K, Schild HH, Hilgers R-D, Bieling HB. Abbreviated breast magnetic resonance imaging (MRI): first postcontrast subtracted images and maximum-intensity projection-a novel approach to breast cancer screening with MRI. J Clin Oncol (2014) 32:2304–10. doi: 10.1200/JCO.2013.52.5386
25. High WA, Ayers RA, Cowper SE. Gadolinium is quantifiable within the tissue of patients with nephrogenic systemic fibrosis. J Am Acad Dermatol (2007) 56:710–2. doi: 10.1016/j.jaad.2007.01.022
26. ACR Manual on Contrast Media. (2013). Available at: http://www.acr.org//media/ACR/Documents/PDF/QualitySafety/Resources/Contrast%20Manual/2013_Contrast_Media.pdf.
27. Attari H, Cao Y, Elmholdt TR, Zhao Y, Prince MR. A systematic review of 639 patients with biopsy-confirmed nephrogenic systemic fibrosis. Radiology (2019) 292:376–86. doi: 10.1148/radiol.2019182916
28. Kanda T, Ishii K, Kawaguchi H, Kitajima K, Takenaka D. High signal intensity in the dentate nucleus and globus pallidus on unenhanced T1-weighted MR images: relationship with increasing cumulative dose of a gadolinium-based contrast material. Radiology (2014) 270:834–41. doi: 10.1148/radiol.13131669
29. Sardanelli F, Cozzi A, Trimboli RM, Schiaffino S. Gadolinium retention and breast MRI screening: More harm than good? Am J Roentgenology (2020) 214:324–7. doi: 10.2214/AJR.19.21988
30. Melsaether AN, Kim E, Mema E, Babb J, Kim SG. Preliminary study: Breast cancers can be well seen on 3T breast MRI with a half-dose of gadobutrol. Clin Imaging (2019) 58:84–9. doi: 10.1016/j.clinimag.2019.06.014
31. Iima M, Le Bihan D. Clinical intravoxel incoherent motion and diffusion MRI imaging: Past, present, and future. Radiology (2016) 278:13–32. doi: 10.1148/radiol.2015150244
32. Iima M, Partridge SC, Le Bihan D. Six DWI questions you always wanted to know but were afraid to ask: Clinical relevance for breast diffusion MRI. Eur Radiol (2020) 30:2561–70. doi: 10.1007/s00330-019-06648-0
33. Le Bihan D. Apparent diffusion coefficient and beyond: What diffusion MR imaging can tell us about tissue structure. Radiology (2013) 268:318–22. doi: 10.1148/radiol.13130420
34. Le Bihan D, Breton E, Lallemand D, Grenier P, Cabanis E, Laval-Jeantet M. MR imaging of intravoxel incoherent motions: Application to diffusion and perfusion in neurologic disorders. Radiology (1986) 161:401–7. doi: 10.1148/radiology.161.2.3763909
35. Le Bihan D, Breton E, Lallemand D, Aubin ML, Vignaud J, Laval-Jeantet M. Separation of diffusion and perfusion in intravoxel incoherent motion MR imaging. Radiology (1988) 168:497–505. doi: 10.1148/radiology.168.2.3393671
36. Le Bihan D, Iima M, Federau C. Sigmund EE eds. (2018) intravoxel incoherent motion (IVIM) MRI: Pan Stanford publishing. Singapore. p. 534.
37. Zhang H, Schneider T, Wheeler-Kingshott CA, Alexander DC. NODDI: practical in vivo neurite orientation dispersion and density imaging of the human brain. Neuroimage (2012) 61:1000–16. doi: 10.1016/j.neuroimage.2012.03.072
38. Tang L, Zhou XJ. Diffusion MRI of cancer: From low to high b-values. J Magn Reson Imaging (2019) 49:23–40. doi: 10.1002/jmri.26293
39. Chabert S, Meca C, Le Bihan D. Relevance of the information about the diffusion distribution in vivo given by kurtosis in q-space imaging. Proc 12th Annu Meeting ISMRM (2004) 1238.
40. Jensen JH, Helpern JA, Ramani A, Lu H, Kaczynski K. Diffusional kurtosis imaging: The quantification of non-gaussian water diffusion by means of magnetic resonance imaging. Magn Reson Med (2005) 53:1432–40. doi: 10.1002/mrm.20508
41. Wu D, Li G, Zhang J, Chang S, Hu J, Dai Y. Characterization of breast tumors using diffusion kurtosis imaging (DKI). PloS One (2014) 9:e113240. doi: 10.1371/journal.pone.0113240
42. Iima M, Kataoka M, Kanao S, Onishi N, Kawai M, Ohashi A, et al. Intravoxel incoherent motion and quantitative non-Gaussian diffusion MR imaging: Evaluation of the diagnostic and prognostic value of several markers of malignant and benign breast lesions. Radiology (2018) 287:432–41. doi: 10.1148/radiol.2017162853
43. Goto M, Le Bihan D, Yoshida M, Sakai K, Yamada K. Adding a model-free diffusion MRI marker to BI-RADS assessment improves specificity for diagnosing breast lesions. Radiology (2019) 292:84–93. doi: 10.1148/radiol.2019181780
44. Goto M, Le Bihan D, Sakai K, Yamada K. The diffusion MRI signature index is highly correlated with immunohistochemical status and molecular subtype of invasive breast carcinoma. Eur Radiol (2022) 32:4879–88. doi: 10.1007/s00330-022-08562-4
45. Furman-Haran E, Grobgeld D, Nissan N, Shapiro-Feinberg M, Degani H. Can diffusion tensor anisotropy indices assist in breast cancer detection? J Magn Reson Imaging (2016) 44:1624–32. doi: 10.1002/jmri.25292
46. Luo J, Hippe DS, Rahbar H, Parsian S, Rendi MH, Partridge SC. Diffusion tensor imaging for characterizing tumor microstructure and improving diagnostic performance on breast MRI: A prospective observational study. Breast Cancer Res (2019) 21:102. doi: 10.1186/s13058-019-1183-3
47. Eyal E, Shapiro-Feinberg M, Furman-Haran E, Grobgeld D, Golan T, Itzchak Y, et al. Parametric diffusion tensor imaging of the breast. Invest Radiol (2012) 47:284–91. doi: 10.1097/RLI.0b013e3182438e5d
48. Baltzer PAT, Schäfer A, Dietzel M, Grässel D, Gajda M, Camara O, et al. Diffusion tensor magnetic resonance imaging of the breast: A pilot study. Eur Radiol (2011) 21:1–10. doi: 10.1007/s00330-010-1901-9
49. Onaygil C, Kaya H, Ugurlu MU, Aribal E. Diagnostic performance of diffusion tensor imaging parameters in breast cancer and correlation with the prognostic factors. J Magn Reson Imaging (2017) 45:660–72. doi: 10.1002/jmri.25481
50. Wang K, Li Z, Wu Z, Zheng Y, Zeng S, Linning E, et al. Diagnostic performance of diffusion tensor imaging for characterizing breast tumors: A comprehensive meta-analysis. Front Oncol (2019) 9:1229. doi: 10.3389/fonc.2019.01229
51. Baxter GC, Graves MJ, Gilbert FJ, Patterson AJ. A meta-analysis of the diagnostic performance of diffusion MRI for breast lesion characterization. Radiology (2019) 291:632–41. doi: 10.1148/radiol.2019182510
52. Lo Gullo R, Sevilimedu V, Baltzer P, Le Bihan D, Camps-Herrero J, Clauser P, et al. A survey by the European society of breast imaging on the implementation of breast diffusion-weighted imaging in clinical practice. Eur Radiol (2022) 32:6588–97. doi: 10.1007/s00330-022-08833-0
53. Partridge SC, Demartini WB, Kurland BF, Eby PR, White SW, Lehman CD. Differential diagnosis of mammographically and clinically occult breast lesions on diffusion-weighted MRI. J Magn Reson Imaging (2010) 31:562–70. doi: 10.1002/jmri.22078
54. Yabuuchi H, Matsuo Y, Sunami S, Kamitani T, Kawanami S, Setoguchi T, et al. Detection of non-palpable breast cancer in asymptomatic women by using unenhanced diffusion-weighted and T2-weighted MR imaging: Comparison with mammography and dynamic contrast-enhanced MR imaging. Eur Radiol (2011) 21:11–7. doi: 10.1007/s00330-010-1890-8
55. McDonald ES, Hammersley JA, Chou SHS, Rahbar H, Scheel JR, Lee CI, et al. “Performance of DWI as a rapid unenhanced technique for detecting mammographically occult breast cancer in elevated-risk women with dense breasts. ” AJR Am J Roentgenol (2016) 207(1):205–16. doi: 10.2214/AJR.15.15873
56. Ha SM, Chang JM, Lee SH, Kim ES, Kim SY, Kim YS, et al. Detection of contralateral breast cancer using diffusion-weighted magnetic resonance imaging in women with newly diagnosed breast cancer: Comparison with combined mammography and whole-breast ultrasound. Korean J Radiol (2021) 22:867–79. doi: 10.3348/kjr.2020.1183
57. Ochi M, Kuroiwa T, Sunami S, Murakami J, Miyahara S, Nagaie T, et al. Diffusion-weighted imaging (b value = 1500 s/mm(2)) is useful to decrease false-positive breast cancer cases due to fibrocystic changes. Breast Cancer (2013) 20:137–44. doi: 10.1007/s12282-011-0319-9
58. Telegrafo M, Rella L, Stabile Ianora AA, Angelelli G, Moschetta M. Unenhanced breast MRI (STIR, T2-weighted TSE, DWIBS): An accurate and alternative strategy for detecting and differentiating breast lesions. Magn Reson Imaging (2015) 33:951–5. doi: 10.1016/j.mri.2015.06.002
59. Bickelhaupt S, Laun FB, Tesdorff J, Lederer W, Daniel H, Stieber A, et al. Fast and noninvasive characterization of suspicious lesions detected at breast cancer X-ray screening: Capability of diffusion-weighted MR imaging with MIPs. Radiology (2016) 278:689–97. doi: 10.1148/radiol.2015150425
60. Iima M, Honda M, Sigmund EE, Ohno Kishimoto A, Kataoka M, Togashi K, et al. Diffusion MRI of the breast: Current status and future directions. J Magn Reson Imaging (2020) 52:70–90.
61. He M, Ruan H, Ma M, Zhang Z. Application of diffusion weighted imaging techniques for differentiating benign and malignant breast lesions. Front Oncol (2021) 11:694634. doi: 10.3389/fonc.2021.694634
62. Fardanesh R, Thakur SB, Sevilimedu V, Horvat JV, Gullo RL, Reiner JS, et al. Differentiation between benign and metastatic breast lymph nodes using apparent diffusion coefficients. Front Oncol (2022) 12:795265. doi: 10.3389/fonc.2022.795265
63. De Cataldo C, Bruno F, Palumbo P, Di Sibio A, Arrigoni F, Clemente A, et al. Apparent diffusion coefficient magnetic resonance imaging (ADC-MRI) in the axillary breast cancer lymph node metastasis detection: A narrative review. Gland Surg (2020) 9:2225–34. doi: 10.21037/gs-20-546
64. Iima M, Kataoka M, Okumura R, Togashi K. Detection of axillary lymph node metastasis with diffusion-weighted MR imaging. Clin Imaging (2014) 38:633–6. doi: 10.1016/j.clinimag.2014.04.016
65. Dijkstra H, Dorrius MD, Wielema M, Pijnappel RM, Oudkerk M, Sijens PE. Quantitative DWI implemented after DCE-MRI yields increased specificity for BI-RADS 3 and 4 breast lesions. J Magn Reson Imaging (2016) 44:1642–9. doi: 10.1002/jmri.25331
66. Sun SY, Ding Y, Li Z, Nie L, Liao C, Liu Y, et al. Multiparameter MRI model with DCE-MRI, DWI, and synthetic MRI improves the diagnostic performance of BI-RADS 4 lesions. Front Oncol (2021) 11:699127. doi: 10.3389/fonc.2021.699127
67. Pinker K, Moy L, Sutton EJ, Mann RM, Weber M, Thakur SB, et al. Diffusion-weighted imaging with apparent diffusion coefficient mapping for breast cancer detection as a stand-alone parameter: Comparison with dynamic contrast-enhanced and multiparametric magnetic resonance imaging. Invest Radiol (2018) 53:587–95. doi: 10.1097/RLI.0000000000000465
68. Yabuuchi H, Matsuo Y, Kamitani T, Setoguchi T, Okafuji T, Soeda H, et al. Non-mass-like enhancement on contrast-enhanced breast MR imaging: Lesion characterization using combination of dynamic contrast-enhanced and diffusion-weighted MR images. Eur J Radiol (2010) 75:e126–32. doi: 10.1016/j.ejrad.2009.09.013
69. Arponen O, Masarwah A, Sutela A, Taina M, Könönen M, Sironen R, et al. Incidentally detected enhancing lesions found in breast MRI: Analysis of apparent diffusion coefficient and T2 signal intensity significantly improves specificity. Eur Radiol (2016) 26:4361–70. doi: 10.1007/s00330-016-4326-2
70. Suo S, Cheng F, Cao M, Kang J, Wang M, Hua J, et al. Multiparametric diffusion-weighted imaging in breast lesions: Association with pathologic diagnosis and prognostic factors. J Magn Reson Imaging (2017) 46:740–50. doi: 10.1002/jmri.25612
71. Sharma U, Sah RG, Agarwal K, Parshad R, Seenu V, Mathur SR, et al. Potential of diffusion-weighted imaging in the characterization of malignant, benign, and healthy breast tissues and molecular subtypes of breast cancer. Front Oncol (2016) 6:126. doi: 10.3389/fonc.2016.00126
72. Liang J, Zeng S, Li Z, Kong Y, Meng T, Zhou C, et al. Intravoxel incoherent motion diffusion-weighted imaging for quantitative differentiation of breast tumors: A meta-analysis. Front Oncol (2020) 10:585486. doi: 10.3389/fonc.2020.585486
73. Wang Q, Guo Y, Zhang J, Wang Z, Huang M, Zhang Y. Contribution of IVIM to conventional dynamic contrast-enhanced and diffusion-weighted MRI in differentiating benign from malignant breast masses. Breast Care (2016) 11:254–8. doi: 10.1159/000447765
74. Liu C, Wang K, Chan Q, Liu Z, Zhang J, He H, et al. Intravoxel incoherent motion MR imaging for breast lesions: comparison and correlation with pharmacokinetic evaluation from dynamic contrast-enhanced MR imaging. Eur Radiol (2016) 26:3888–98. doi: 10.1007/s00330-016-4241-6
75. Cho GY, Moy L, Kim SG, Baete SH, Moccaldi M, Babb JS, et al. Evaluation of breast cancer using intravoxel incoherent motion (IVIM) histogram analysis: Comparison with malignant status, histological subtype, and molecular prognostic factors. Eur Radiol (2016) 26:2547–58. doi: 10.1007/s00330-015-4087-3
76. Lee YJ, Kim SH, Kang BJ, Kang YJ, Yoo H, Yoo J, et al. Intravoxel incoherent motion (IVIM)-derived parameters in diffusion-weighted MRI: Associations with prognostic factors in invasive ductal carcinoma. J Magn Reson Imaging (2017) 45:1394–406. doi: 10.1002/jmri.25514
77. Sun K, Chen X, Chai W, Fei X, Fu C, Yan X, et al. Breast cancer: Diffusion kurtosis MR imaging-diagnostic accuracy and correlation with clinical-pathologic factors. Radiology (2015) 277:46–55. doi: 10.1148/radiol.15141625
78. Park VY, Kim SG, Kim E-K, Moon HJ, Yoon JH, Kim MJ. Diffusional kurtosis imaging for differentiation of additional suspicious lesions on preoperative breast MRI of patients with known breast cancer. Magn Reson Imaging (2019) 62:199–208. doi: 10.1016/j.mri.2019.07.011
79. Li Z, Li X, Peng C, Dai W, Huang H, Li X, et al. The diagnostic performance of diffusion kurtosis imaging in the characterization of breast tumors: A meta-analysis. Front Oncol (2020) 10:575272. doi: 10.3389/fonc.2020.575272
80. Gu H, Cui W, Luo S, Deng X. Diagnostic performance of diffusion kurtosis imaging for benign and malignant breast lesions: A systematic review and meta-analysis. Appl Bionics Biomech (2022) 2022:2042736. doi: 10.1155/2022/2042736
81. Yang ZL, Li Y, Zhan CA, Hu YQ, Guo YH, Xia LM, et al. Evaluation of suspicious breast lesions with diffusion kurtosis MR imaging and connection with prognostic factors. Eur J Radiol (2021) 145:110014. doi: 10.1016/j.ejrad.2021.110014
82. Zhang D, Geng X, Suo S, Zhuang Z, Gu Y, Hua J. The predictive value of DKI in breast cancer: Does tumour subtype affect pathological response evaluations? Magn Reson Imaging (2022) 85:28–34. doi: 10.1016/j.mri.2021.10.013
83. Yamaguchi K, Nakazono T, Egashira R, Komori Y, Nakamura J, Noguchi T, et al. Diagnostic performance of diffusion tensor imaging with readout-segmented echo-planar imaging for invasive breast cancer: Correlation of ADC and FA with pathological prognostic markers. Magn Reson Med Sci (2017) 16:245–52. doi: 10.2463/mrms.mp.2016-0037
84. Kim JY, Kim JJ, Kim S, Choo KS, Kim A, Kang T, et al. Diffusion tensor magnetic resonance imaging of breast cancer: Associations between diffusion metrics and histological prognostic factors. Eur Radiol (2018) 28:3185–93. doi: 10.1007/s00330-018-5429-8
85. Abdel Razek AAK, Zaky M, Bayoumi D, Taman S, Abdelwahab K, Alghandour R. Diffusion tensor imaging parameters in differentiation recurrent breast cancer from post-operative changes in patients with breast-conserving surgery. Eur J Radiol (2019) 111:76–80. doi: 10.1016/j.ejrad.2018.12.022
86. Shin HJ, Moon WK, Amornsiripanitch N, Partridge SC. DIFFUSION MRI OF THE BREAST. In: Iima M, Partridge S, Le Bihan D, editors. Diffusion MRI as a stand-alone unenhanced approach for breast imaging and screening. Philadelphia: Elsevier Health Sciences (2022). p. 260.
87. Baltzer PAT, Benndorf M, Dietzel M, Gajda M, Runnebaum IB, Kaiser WA. False-positive findings at contrast-enhanced breast MRI: A BI-RADS descriptor study. AJR Am J Roentgenol (2010) 194(6):1658–63. doi: 10.2214/AJR.09.3486
88. Zhang L, Tang M, Min Z, Lu J, Lei X, Zhang X. Accuracy of combined dynamic contrast-enhanced magnetic resonance imaging and diffusion-weighted imaging for breast cancer detection: A meta-analysis. Acta Radiol (2016) 57:651–60. doi: 10.1177/0284185115597265
89. O’Flynn EAM, Blackledge M, Collins D, Downey K, Doran S, Patel H, et al. Evaluating the diagnostic sensitivity of computed diffusion-weighted MR imaging in the detection of breast cancer. J Magn Reson Imaging (2016) 44:130–7. doi: 10.1002/jmri.25131
90. Kang JW, Shin HJ, Shin KC, Chae EY, Choi WJ, Cha JH, et al. Unenhanced magnetic resonance screening using fused diffusion-weighted imaging and maximum-intensity projection in patients with a personal history of breast cancer: Role of fused DWI for postoperative screening. Breast Cancer Res Treat (2017) 165:119–28. doi: 10.1007/s10549-017-4322-5
91. Baltzer PAT, Bickel H, Spick C, Wengert G, Woitek R, Kapetas P, et al. Potential of noncontrast magnetic resonance imaging with diffusion-weighted imaging in characterization of breast lesions. Invest Radiol (2018) 53:229–35. doi: 10.1097/RLI.0000000000000433
92. Bu Y, Xia J, Joseph B, Zhao X, Xu M, Yu Y, et al. Non-contrast MRI for breast screening: preliminary study on detectability of benign and malignant lesions in women with dense breasts. Breast Cancer Res Treat (2019) 177:629–39. doi: 10.1007/s10549-019-05342-5
93. Ha SM, Chang JM, Lee SH, Kim ES, Kim S-Y, Cho N, et al. Diffusion-weighted MRI at 3.0 T for detection of occult disease in the contralateral breast in women with newly diagnosed breast cancer. Breast Cancer Res Treat (2020) 182:283–97. doi: 10.1007/s10549-020-05697-0
94. Amornsiripanitch N, Rahbar H, Kitsch AE, Lam DL, Weitzel B, Partridge SC. Visibility of mammographically occult breast cancer on diffusion-weighted MRI versus ultrasound. Clin Imaging (2018) 49:37–43. doi: 10.1016/j.clinimag.2017.10.017
95. Yoshikawa MI, Ohsumi S, Sugata S, Kataoka M, Takashima S, Kikuchi K, et al. Comparison of breast cancer detection by diffusion-weighted magnetic resonance imaging and mammography. Radiat Med (2007) 25:218–23. doi: 10.1007/s11604-007-0128-4
96. Kazama T, Kuroki Y, Kikuchi M, Sato Y, Nagashima T, Miyazawa Y, et al. Diffusion-weighted MRI as an adjunct to mammography in women under 50 years of age: an initial study. J Magn Reson Imaging (2012) 36:139–44. doi: 10.1002/jmri.23626
97. Breast imaging reporting and data system. In: ACR BI-RADS® atlas 5th edition. American College ofRadiology.
98. Newitt DC, Zhang Z, Gibbs JE, Partridge SC, Chenevert TL, Rosen MA, et al. Test-retest repeatability and reproducibility of ADC measures by breast DWI: Results from the ACRIN 6698 trial. J Magn Reson Imaging (2019) 49:1617–28. doi: 10.1002/jmri.26539
99. Chen W, Zhang J, Long D, Wang Z, Zhu JM. Optimization of intra-voxel incoherent motion measurement in diffusion-weighted imaging of breast cancer. J Appl Clin Med Phys (2017) 18:191–9. doi: 10.1002/acm2.12065
100. Pereira FPA, Martins G, Figueiredo E, Domingues MNA, Domingues RC, da Fonseca LMB, et al. Assessment of breast lesions with diffusion-weighted MRI: Comparing the use of different b values. AJR Am J Roentgenol (2009) 193:1030–5. doi: 10.2214/AJR.09.2522
101. Peters NHGM, Vincken KL, van den Bosch MAAJ, Luijten PR, Mali WPTM, Bartels LW. Quantitative diffusion weighted imaging for differentiation of benign and malignant breast lesions: The influence of the choice of b-values. J Magn Reson Imaging (2010) 31:1100–5. doi: 10.1002/jmri.22152
102. Eghtedari M, Ma J, Fox P, Guvenc I, Yang WT, Dogan BE. Effects of magnetic field strength and b value on the sensitivity and specificity of quantitative breast diffusion-weighted MRI. Quant Imaging Med Surg (2016) 6:374–80. doi: 10.21037/qims.2016.07.06
103. Iima M, Kataoka M, Honda M, Ohashi A, Ohno Kishimoto A, Ota R, et al. Rate of apparent diffusion coefficient change with diffusion time on breast diffusion-weighted imaging depends on breast tumor types and molecular prognostic biomarker expression. Invest Radiol (2021) 56:501–8. doi: 10.1097/RLI.0000000000000766
104. QIBA Profile. Diffusion-weighted magnetic resonanceimaging (DWI). Available at: http://qibawiki.rsna.org/images/1/1d/QIBADWIProfilev1.45_20170427_v5_accepted.pdf.
105. Porter DA, Heidemann RM. High resolution diffusion-weighted imaging using readout-segmented echo-planar imaging, parallel imaging and a two-dimensional navigator-based reacquisition. Magn Reson Med (2009) 62:468–75. doi: 10.1002/mrm.22024
106. Iima M, Yano K, Kataoka M, Umehana M, Murata K, Kanao S, et al. Quantitative non-Gaussian diffusion and intravoxel incoherent motion magnetic resonance imaging: differentiation of malignant and benign breast lesions. Invest Radiol (2015) 50:205–11. doi: 10.1097/RLI.0000000000000094
107. Yin H, Jiang Y, Xu Z, Huang W, Chen T, Lin G. Apparent diffusion coefficient-based convolutional neural network model can be better than sole diffusion-weighted magnetic resonance imaging to improve the differentiation of invasive breast cancer from breast ductal carcinoma in situ. Front Oncol (2021) 11:805911. doi: 10.3389/fonc.2021.805911
108. Reig B, Heacock L, Geras KJ, Moy L. Machine learning in breast MRI. J Magn Reson Imaging (2020) 52:998–1018. doi: 10.1002/jmri.26852
109. Bickelhaupt S, Paech D, Kickingereder P, Steudle F, Lederer W, Daniel H, et al. Prediction of malignancy by a radiomic signature from contrast agent-free diffusion MRI in suspicious breast lesions found on screening mammography. J Magn Reson Imaging (2017) 46:604–16. doi: 10.1002/jmri.25606
110. Dalmis MU, Gubern-Mérida A, Vreemann S, Bult P, Karssemeijer N, Mann R, et al. Artificial intelligence–based classification of breast lesions imaged with a multiparametric breast MRI protocol with ultrafast DCE-MRI, T2, and DWI. Invest Radiol (2019) 54:325. doi: 10.1097/RLI.0000000000000544
111. Tahmassebi A, Wengert GJ, Helbich TH, Bago-Horvath Z, Alaei S, Bartsch R, et al. Impact of machine learning with multiparametric magnetic resonance imaging of the breast for early prediction of response to neoadjuvant chemotherapy and survival outcomes in breast cancer patients. Invest Radiol (2019) 54:110–7. doi: 10.1097/RLI.0000000000000518
112. Zhang L, Mohamed AA, Chai R, Guo Y, Zheng B, Wu S. Automated deep learning method for whole-breast segmentation in diffusion-weighted breast MRI. J Magn Reson Imaging (2020) 51:635–43. doi: 10.1002/jmri.26860
113. Sattarov A, McIntyre P, Motowidlo L. High-field open MRI for breast cancer screening. IEEE Trans Appl Supercond (2015) 25:1–5. doi: 10.1109/TASC.2014.2377049
Keywords: breast cancer, screening, breast imaging, diffusion MRI, standardization, screening techniques, cost, dedicated units
Citation: Iima M and Le Bihan D (2023) The road to breast cancer screening with diffusion MRI. Front. Oncol. 13:993540. doi: 10.3389/fonc.2023.993540
Received: 13 July 2022; Accepted: 10 January 2023;
Published: 21 February 2023.
Edited by:
Mitchell Albert, Lakehead University, CanadaReviewed by:
Federica Pediconi, Sapienza University of Rome, ItalyCopyright © 2023 Iima and Le Bihan. This is an open-access article distributed under the terms of the Creative Commons Attribution License (CC BY). The use, distribution or reproduction in other forums is permitted, provided the original author(s) and the copyright owner(s) are credited and that the original publication in this journal is cited, in accordance with accepted academic practice. No use, distribution or reproduction is permitted which does not comply with these terms.
*Correspondence: Denis Le Bihan, ZGVuaXMubGViaWhhbkBnbWFpbC5jb20=
Disclaimer: All claims expressed in this article are solely those of the authors and do not necessarily represent those of their affiliated organizations, or those of the publisher, the editors and the reviewers. Any product that may be evaluated in this article or claim that may be made by its manufacturer is not guaranteed or endorsed by the publisher.
Research integrity at Frontiers
Learn more about the work of our research integrity team to safeguard the quality of each article we publish.