- 1Department of Obstetrics and Gynecology, Peking University Shenzhen Hospital, Shenzhen, China
- 2Institute of Obstetrics and Gynecology, Shenzhen Peking University-The Hong Kong University of Science and Technology (PKU-HKUST) Medical Center, Shenzhen, China
- 3Shenzhen Key Laboratory on Technology for Early Diagnosis of Major Gynecologic Diseases, Shenzhen, China
- 4Department of Computer Science, City University of Hong Kong, Hong Kong, Hong Kong SAR, China
- 5Suzhou Ruiqian Technology Company Ltd., Suzhou, China
Given the shortage of cytologists, women in low-resource regions had inequitable access to cervical cytology which plays an pivotal role in cervical cancer screening. Emerging studies indicated the potential of AI-assisted system in promoting the implementation of cytology in resource-limited settings. However, there is a deficiency in evaluating the aid of AI in the improvement of cytologists’ work efficiency. This study aimed to evaluate the feasibility of AI in excluding cytology-negative slides and improve the efficiency of slide interpretation. Well-annotated slides were included to develop the classification model that was applied to classify slides in the validation group. Nearly 70% of validation slides were reported as negative by the AI system, and none of these slides were diagnosed as high-grade lesions by expert cytologists. With the aid of AI system, the average of interpretation time for each slide decreased from 3 minutes to 30 seconds. These findings suggested the potential of AI-assisted system in accelerating slide interpretation in the large-scale cervical cancer screening.
Introduction
Cervical cancer is threatening women’s health and caused 342,000 deaths worldwide in 2020 (1). Screening plays an important role in eliminating cervical cancer, such as diagnosing precancerous cervical intraepithelial neoplasia (CIN) that can be surgically eliminated to prevent the incidence of cervical cancer (2, 3). However, there are disparities of cervical cancer screening globally (4, 5). Self-sampling has dramatically ameliorated inequity of human papillomavirus (HPV) testing especially in resource-limited settings (6–9). In contrast, cervical cytology remains an issue in low-resource regions due to the shortage of cytologists (10, 11).
Emerging studies indicated the potential of artificial intelligence (AI) system for cervical cytology (12–16). For instance, Cheng et al. applied a recurrent neural network-based whole slide image (WSI) classification model to achieve high specificity and sensitivity for slide classification (14). Nevertheless, most of prior reports assessed the potential of AI system in classifying cervical lesions (12–15). Besides to the diagnosis of cervical lesions, it is important to lessen the dependence of cervical cytology on professionals in resource-limited settings. Given the indispensable role of cytologists in cervical cancer screening, it is imperative to reduce the number of cytologist-interpreted slides and to shorten the interpretation time for each slide.
Our study aimed to evaluate how AI-assisted system improved the work efficiency of cytologist-based cytology. To fulfill this goal, well-annotated cervical slides were applied to develop the model of slide classification. Then we assessed the feasibility of AI system in excluding NILM(Negative for Intraepithelial Lesion or Malignancy) slides. Subsequently, the efficiency of slide interpretation was evaluated for cytologists with and without the aid of AI system. These findings should have the potential to promote the implementation of cervical cytology in China.
Materials and methods
Automated staining and microscopic imaging of cervical exfoliated cells
We applied a liquid-based sedimentation cytology approach RQLCT1000 (Ruiqian co. ltd, Jiangsu) to achieve stained slides. Then, the automated slide scanner RQ1000 (Ruiqian co. ltd, Jiangsu) using continuous array scanning technology was applied to rapidly generate multi-depth images. The scanning process included two stages: 10X and 20X microscope scanning and multi-depth scanning as well as seamless layer fusion via Z-stack technology (17).
Image annotation
Three experimental clinicians and two expert pathologists from tertiary hospitals annotated scanned slides, adhering to TBS-2014 guidelines (18). In detail, digital images were divided into three non-repetitive sets for annotation by distinct medical professionals. Annotation cells, which results were agreed by two experts, were integrated into a standardized database for robust data management.
Cell detection and classification
A 10× image acquisition system was used for Pap test AI detection to identify single cells and cell clusters. Cell clusters were characterized by closely packed cells without easily distinguishable cytoplasm (Supplementary Figure 1). Meanwhile, each cell cluster should include more than three cells.
We then classified cells via two modules: cell primary screening and cell classification module. The cell primary screening module applied Yolov5 as the basic framework, and the cell classification module utilized ResNet as the basic framework (19–21). Among them, the cell primary screening module was used for detecting all suspicious lesioned cells in the image to ensure a high recall rate of detected diseased cells. The cell classification module further screened and classified diseased cells on the basis of the cell primary screening module to improve the accuracy of detecting diseased cells.
The dataset of the cell primary screening module was composed of annotated cells, that were classified into NILM(Negative for Intraepithelial Lesion or Malignancy), HSIL(High-Grade Squamous Intraepithelial Lesion), LSIL (Low-Grade Squamous Intraepithelial Lesion), and ASU(Atypical Squamous Cells of Undetermined Significance) types.
The dataset of the cell classification module was composed of the detected positive cell images of the cell primary screening module, wherein the ‘false’ diseased cells detected by the cell primary screening module were regarded as NILM, and the detected ‘true’ diseased cells were regarded as lesion-positive.
Slide classification
To determine if the slide was lesioned, we first ranked all single cells and cell clusters based on lesion probability. Afterwards, we calculated the average probability of top 10 single cells as well as the average probability of top 5 cell clusters. Then scatter plotting was conducted for all slides to determine the cutoff of probability, using the average of top 10 single cells as x-axis and the average of top 5 cell clusters as y-axis. And SVM classifier was applied to determine the degree of lesion, such as ASU and HSIL (22).
Statistic analysis
To assess the feasibility of AI system in excluding NILM slides, we calculated the percentage of AI-reported NILM slides in the validation group. Then we compared it to cytologist-interpreted results.
For slides in the validation group, the interpretation time of cytologists was recorded for two types of slide interpretation: with and without the aid of AI system. To compare the differences of consumed time in slide interpretation, the average of the interpretation time was calculated by the formula: the total of consumed time/slide number. In addition, cytologist-reported results were also compared between two interpretation models.
Results
Automatic AI system to perform cell staining and imaging
We devised an integrated approach for the automated diagnosis of cervical lesions, utilizing the cytology of cervical exfoliated cells (Figure 1). All experiments were seamlessly integrated into an automated and cohesive process, encompassing the staining of exfoliated cells and multi-depth scanning fusion imaging.
Within this automatic system, we totally scanned 43,057 cervical slides in two hospitals within 74 days (10 running hours/day), resulting in an average production rate of nearly 30 high-quality images per hour. Then we selected 5,000 high-quality slides for developing cell classification model and the remaining 31,753 high-quality slides were applied to assess the performance of developed models.
Performance of AI-system in classifying cervical cells
Based on 5,000 high-confidence slides, 98,212 high-quality 10× images of individual cells were chosen, being annotated as NILM (n=50,100), ASU (n=16,602), LSIL (n=13,968), and HSIL (n=17,542) (Table 1). Additionally, we selected 64,087 10× high-quality images for cell clusters, which were annotated as NILM (n=34,775), ASU (n=9,062), LSIL (n=9,552), and HSIL (n=10,698) (Table 1). The confusion matrix showed that the accuracy of NILM prediction from single cell and cell cluster was 81.40% and 88.85%, respectively. The highest accuracy were found for HSIL prediction (90.0% and 85.0% for single cell and cell cluster), compare to the ASU (42.54% and 37.17% for single cell and cell cluster) and LSIL(68.23% and 65.75% for single cell and cell cluster) (Table 2).
The same methods were applied in 20× microscope classification, but combination of cell clusters and single cells were used to train the classification model. As the accuracy of NILM remained at 81.49%, there was a reduction in misclassification ratio from ASU, LSIL and HSIL to NILM by 10.61%, 2.71% and 4.45% (Table 3).
To achieve the higher accuracy of NILM prediction, we calculated both the average probability of the top 10 single cells and the average probability of the top 5 cell clusters. By applying a cutoff of 0.6 for single cell scores and 0.65 for cell cluster scores (Figure 2), we achieved the highest accuracy in NILM prediction.
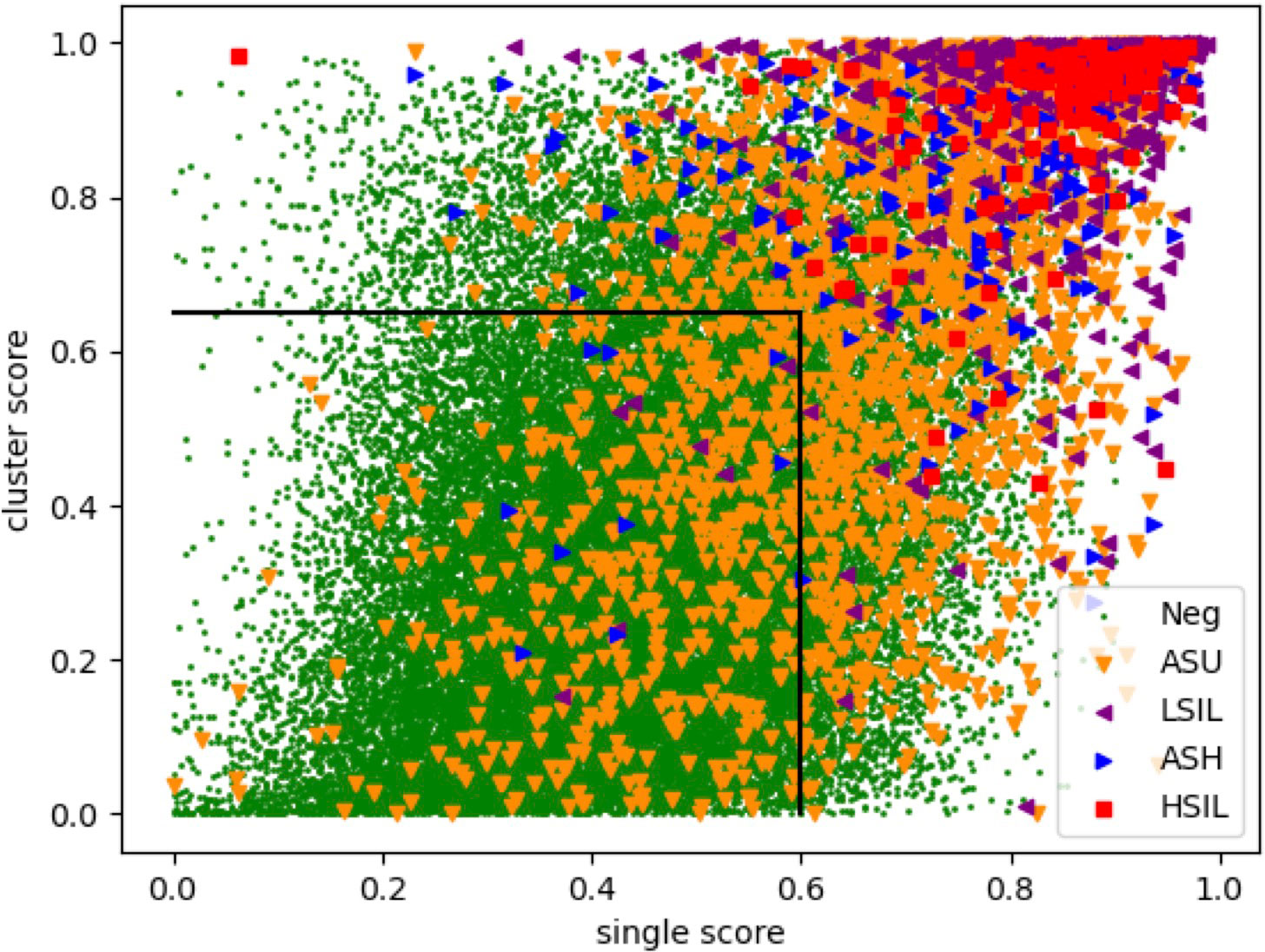
Figure 2 Scatter plot for slide assessment based on single cell and cell cluster scores. Each specific represents a slide and the colors represent expert-conducted diagnosis of the slide. X-axis and y-axis represent the average of probability for top 10 single cells and top 5 cell clusters, respectively.
Performance of AI system in excluding cytology-negative slides and improving the efficiency of the slide interpretation
We further applied our AI-system on 31,753 slides in the validation groups. AI system reported 29,625 slides with NILM among which 98.27% (29,113/29,625) were also diagnosed as NILM by two expert cytologists who reviewed slides together and provided a consensus result. For remaining 512 AI-reported NILM slides, 1.67% (496/29,625) and 0.05% (16/29,625) were diagnosed as ASU and LSIL by cytologists. None of AI-reported NILM slides was diagnosed as HSIL.
Besides to accurately exclude cytology-negative slides, our AI system shortened the interpretation time for cytologists. Based on AI-assisted system, cytologists only need to interpret the top 20 cells which were ranked by the lesion probability. Therefore, the average interpretation time for each slide can decrease from 3 minutes to 30 seconds. And we observed no differences of interpretation results for 979 AI-reported lesion-positive slides between whole slide image and AI-provided top 20 cells, including 129 HSIL, 137 ASH and 713 LSIL slides.
Discussion
Cervical cancer screening play an important role in eliminating cervical cancer (2, 3). Despite the potential of AI-assisted system in facilitating cervical cytology, professionals play essential roles in near future. Nevertheless, there is a deficiency in assessing the improved work efficiency of cytologists and the lessened dependence on professionals based on AI-assisted system. Our study evaluated the exclusion of cytology-negative slides and shortened interpretation time for cytologists with the aid of AI system.
Our large-scale cervical cancer screening projects indicated that nearly 80% of cervical slides were cytology-negative (23–26). Therefore, it can dramatically decrease the workload of cytologists if the AI-system can exclude these cytology-negative slides with high accuracy. In this study, we identified the potential of AI-assisted system in excluding cytology-negative slides, thus decreasing the number of slides to be interpreted by cytologists. Nearly 70% of analyzed slides were reported as NILM by AI-assisted system. Among 29,625 AI-reported NILM slides, 98.27% were diagnosed as NILM. The remaining 1.72% were diagnosed as ASU as well as LSIL by cytologists. Thus, the risk of missing HSIL slides is low when applying the AI system to exclude cytology-negative slides.
Besides to excluding about 70% cytology-negative slides, AI system can shorten the interpretation time of AI-reported positive slides (27). In prior, it took about 3 minutes for to interpret a slide. With the aid of the AI-assisted system, cytologists only need to interpret the top 20 cells which were ranked by the lesion probability. Therefore, the average interpretation time for each slide can decrease from 3 minutes to 30 seconds.
The limitations of our study included the retrospective design. And it was not tested in real resource-limited settings. In addition, it was completed with two steps: preparing slides then conducting slide scanning and AI-assisted cytology. Thus, it would bring additional burden and decrease work efficiency in resource-limited settings. However, our team and other researchers are testing one-stop machine, which can automatically perform high-throughput slide preparation, staining, imaging and AI-assisted cytology. Then it can further improve the feasibility and efficacy of cervical cytology in large-scale cervical cancer screening projects. And this should ensure the equity of cervical cancer screening and precancer/cancer treatment for underserved communities in China.
In summary, AI-assisted system can improve the work efficiency of cytologists, such as decreasing the number of slides to be interpreted and shortened the time of slide interpretation. Additionally, automatic sample processing and AI-assisted cytology can lessen the dependence of cytology on medical resources. And this should increase the coverage of cervical cancer screening in low-resource regions.
Data availability statement
The original contributions presented in the study are included in the article/Supplementary Material. Further inquiries can be directed to the corresponding authors.
Ethics statement
This study was approved by the Ethics Committee of Peking University Shenzhen Hospital (registration number: 2023-116).
Author contributions
HD: Conceptualization, Funding acquisition, Resources, Writing – original draft. WD: Methodology, Visualization, Writing – original draft. QZ: Methodology, Writing – original draft. CL: Methodology, Software, Writing – original draft. SL: Methodology, Writing – original draft. CW: Data curation, Resources, Validation, Writing – original draft. JT: Data curation, Validation, Writing – original draft. XW: Conceptualization, Supervision, Writing – review & editing. RW: Conceptualization, Resources, Supervision, Writing – review & editing.
Funding
The author(s) declare financial support was received for the research, authorship, and/or publication of this article. This study was supported by Shenzhen Science and Technology Project (GJHZ20210705142543018).
Acknowledgments
We thank team members of CW for the aid of slide preparation and interpretation.
Conflict of interest
Authors XW and CL is employed by Suzhou Ruiqian Technology Company Ltd.
The remaining authors declare that the research was conducted in the absence of any commercial or financial relationships that could be construed as a potential conflict of interest.
The author(s) declared that they were an editorial board member of Frontiers, at the time of submission. This had no impact on the peer review process and the final decision.
Publisher’s note
All claims expressed in this article are solely those of the authors and do not necessarily represent those of their affiliated organizations, or those of the publisher, the editors and the reviewers. Any product that may be evaluated in this article, or claim that may be made by its manufacturer, is not guaranteed or endorsed by the publisher.
Supplementary material
The Supplementary Material for this article can be found online at: https://www.frontiersin.org/articles/10.3389/fonc.2023.1290112/full#supplementary-material
References
1. Singh D, Vignat J, Lorenzoni V, Eslahi M, Ginsburg O, Lauby-Secretan B, et al. Global estimates of incidence and mortality of cervical cancer in 2020: a baseline analysis of the WHO Global Cervical Cancer Elimination Initiative. Lancet Glob Health (2023) 11(2):e197–206. doi: 10.1016/S2214-109X(22)00501-0
2. He WQ, Li C. Recent global burden of cervical cancer incidence and mortality, predictors, and temporal trends. Gynecol Oncol (2021) 163(3):583–92. doi: 10.1016/j.ygyno.2021.10.075
3. Brisson M, Kim JJ, Canfell K, Drolet M, Gingras G, Burger EA, et al. Impact of HPV vaccination and cervical screening on cervical cancer elimination: a comparative modelling analysis in 78 low-income and lower-middle-income countries. Lancet (2020) 395(10224):575–90. doi: 10.1016/S0140-6736(20)30068-4
4. Buskwofie A, David-West G, Clare CA. A review of cervical cancer: incidence and disparities. J Natl Med Assoc (2020) 112(2):229–32. doi: 10.1016/j.jnma.2020.03.002
5. Bruni L, Serrano B, Roura E, Alemany L, Cowan M, Herrero R, et al. Cervical cancer screening programmes and age-specific coverage estimates for 202 countries and territories worldwide: a review and synthetic analysis. Lancet Glob Health (2022) 10(8):e1115–27. doi: 10.1016/S2214-109X(22)00241-8
6. Serrano B, Ibáñez R, Robles C, Peremiquel-Trillas P, de Sanjosé S, Bruni L. Worldwide use of HPV self-sampling for cervical cancer screening. Prev Med (2022) 154:106900. doi: 10.1016/j.ypmed.2021.106900
7. Nishimura H, Yeh PT, Oguntade H, Kennedy CE, Narasimhan M. HPV self-sampling for cervical cancer screening: a systematic review of values and preferences. BMJ Glob Health (2021) 6(5):e003743. doi: 10.1136/bmjgh-2020-003743
8. Li J, Wu R, Qu X, Huang X, Li L, Lin Z, et al. Effectiveness and feasibility of self-sampling for human papillomavirus testing for internet-based cervical cancer screening. Front Public Health (2022) 10:938272. doi: 10.3389/fpubh.2022.938272
9. Belinson JL, Wang G, Qu X, Du H, Shen J, Xu J, et al. The development and evaluation of a community based model for cervical cancer screening based on self-sampling. Gynecol Oncol (2014) 132(3):636–42. doi: 10.1016/j.ygyno.2014.01.006
10. Olubodun T, Balogun MR, Odeyemi AK, Odukoya OO, Ogunyemi AO, Kanma-Okafor OJ, et al. Barriers and recommendations for a cervical cancer screening program among women in low-resource settings in Lagos Nigeria: a qualitative study. BMC Public Health (2022) 22(1):1906. doi: 10.1186/s12889-022-14314-2
11. Adewumi K, Nishimura H, Oketch SY, Adsul P, Huchko M. Barriers and facilitators to cervical cancer screening in western Kenya: a qualitative study. J Cancer Educ (2022) 37(4):1122–8. doi: 10.1007/s13187-020-01928-6
12. Nambu Y, Mariya T, Shinkai S, Umemoto M, Asanuma H, Sato I, et al. A screening assistance system for cervical cytology of squamous cell atypia based on a two-step combined CNN algorithm with label smoothing. Cancer Med (2022) 11:520–9. doi: 10.1002/cam4.4460
13. Sanyal P, Barui S, Deb P, Sharma HC. Performance of a convolu- tional neural network in screening liquid based cervical cytology smears. J Cytol (2019) 36:146–51. doi: 10.4103/JOC.JOC_201_18
14. Cheng S, Liu S, Yu J, Rao G, Xiao Y, Han W, et al. Robust whole slide image analysis for cervical cancer screening using deep learning. Nat Commun (2021) 12(1):5639. doi: 10.1038/s41467-021-25296-x
15. Zhu X, Li X, Ong K, Zhang W, Li W, Li L, et al. Hybrid AI-assistive diagnostic model permits rapid TBS classification of cervical liquid-based thin-layer cell smears. Nat Commun (2021) 12(1):3541. doi: 10.1038/s41467-021-23913-3
16. Gupta R, Kumar N, Bansal S, Singh S, Sood N, Gupta S. Artificial intelligence-driven digital cytology-based cervical cancer screening: is the time ripe to adopt this disruptive technology in resource-constrained settings? A literature review. J Digit Imaging (2023) 36(4):1643–52. doi: 10.1007/s10278-023-00821-0
17. Lugagne JB, Jain S, Ivanovitch P, Meriem ZB, Vulin C, Fracassi C, et al. Identification of individual cells from z-stacks of bright-field microscopy images. Sci Rep (2018) 8(1):11455. doi: 10.1038/s41598-018-29647-5
18. Wang T, Zhang H, Liu Y, Zhao C. Updates in cervical cancer screening guidelines, the Bethesda system for reporting cervical cytology, and clinical management recommendations. J Clin Transl Pathol (2023) 3(2):75–83. doi: 10.14218/jctp.2023.00004
19. Karras T, Laine S, Aila T. A style-based generator architecture for generative adversarial networks. IEEE Trans Pattern Anal Mach Intell (2021) 43(12):4217–28. doi: 10.1109/TPAMI.2020.2970919
20. ZainEldin H, Gamel SA, El-Kenawy EM, Alharbi AH, Khafaga DS, Ibrahim A, et al. Brain tumor detection and classification using deep learning and sine-cosine fitness grey wolf optimization. Bioengineering (Basel) (2022) 10(1):18. doi: 10.3390/bioengineering10010018
21. He K, Zhang X, Ren S, Sun J. Deep residual learning for image recognition. IEEE Xplore (2016) 16541111:770–8. doi: 10.1109/CVPR.2016.90
22. Zhang J, Li Z, Yang J. A parallel SVM training algorithm on large-scale classification problems. IEEE Xplore (2005) 8723897:1637–41. doi: 10.1109/ICMLC.2005.1527207
23. Song F, Belinson JL, Yan P, Huang X, Wang C, Du H, et al. Evaluation of p16INK4a immunocytology and human papillomavirus (HPV) genotyping triage after primary HPV cervical cancer screening on self-samples in China. Gynecol Oncol (2021) 162(2):322–30. doi: 10.1016/j.ygyno.2021.05.014
24. Du H, Luo H, Wang C, Qu X, Belinson JL, Wu R. The prevalence of HR-HPV infection based on self-sampling among women in China exhibited some unique epidemiologic features. J Clin Epidemiol (2021) 139:319–29. doi: 10.1016/j.jclinepi.2021.06.009
25. Guo C, Du H, Belinson JL, Wang C, Huang X, Qu X, et al. The prevalence and distribution of human papillomavirus among 10,867 Chinese Han women. Infect Agent Cancer (2021) 16(1):21. doi: 10.1186/s13027-021-00360-9
26. Du H, Duan X, Liu Y, Shi B, Zhang W, Wang C, et al. Evaluation of cobas HPV and seqHPV assays in the Chinese multicenter screening trial. J Low Genit Tract Dis (2021) 25(1):22–6. doi: 10.1097/LGT.0000000000000577
Keywords: HPV, cervical cancer screening, artificial intelligence, slide interpretation, low-resource areas
Citation: Du H, Dai W, Zhou Q, Li C, Li SC, Wang C, Tang J, Wu X and Wu R (2023) AI-assisted system improves the work efficiency of cytologists via excluding cytology-negative slides and accelerating the slide interpretation. Front. Oncol. 13:1290112. doi: 10.3389/fonc.2023.1290112
Received: 07 September 2023; Accepted: 09 November 2023;
Published: 23 November 2023.
Edited by:
Chengquan Zhao, University of Pittsburgh, United StatesReviewed by:
Jianyu Rao, University of California, Los Angeles, United StatesLakshmi Harinath, University of Pittsburgh Medical Center, United States
Copyright © 2023 Du, Dai, Zhou, Li, Li, Wang, Tang, Wu and Wu. This is an open-access article distributed under the terms of the Creative Commons Attribution License (CC BY). The use, distribution or reproduction in other forums is permitted, provided the original author(s) and the copyright owner(s) are credited and that the original publication in this journal is cited, in accordance with accepted academic practice. No use, distribution or reproduction is permitted which does not comply with these terms.
*Correspondence: Ruifang Wu, wurfpush@126.com; Xiangchen Wu, xc.wu@ruiqian-tech.com
†These authors have contributed equally to this work