- 1Department of Hematology, Nicolae Testemitanu State University of Medicine and Pharmacy, Chisinau, Moldova
- 2Department of Hematology, Public Medical Sanitary Institution (PMSI) Institute of Oncology, Chisinau, Moldova
- 3University Hospital of Santiago de Compostela, Servizo Galego de Saude (SERGAS), Santiago de Compostela, Spain
- 4Health Research Institute of Santiago de Compostela, Santiago de Compostela, Spain
- 5Department of Forensic Sciences, Pathology, Ginecology and Obstetrics and Pediatrics, Faculty of Medicine, University of Santiago de Compostela, Santiago de Compostela, Spain
Next generation sequencing (NGS) is a technology that broadens the horizon of knowledge of several somatic pathologies, especially in oncological and oncohematological pathology. In the case of NHL, the understanding of the mechanisms of tumorigenesis, tumor proliferation and the identification of genetic markers specific to different lymphoma subtypes led to more accurate classification and diagnosis. Similarly, the data obtained through NGS allowed the identification of recurrent somatic mutations that can serve as therapeutic targets that can be inhibited and thus reducing the rate of resistant cases. The article’s purpose is to offer a comprehensive overview of the best ways of integrating of next-generation sequencing technologies for diagnosis, prognosis, classification, and selection of optimal therapy from the perspective of tailor-made medicine.
1 Introduction
Non-Hodgkin’s lymphomas (NHL) are hematopoietic tumors that develop from the malignant proliferation of the lymphatic tissue. NHLs are the most common hematological neoplasms, accounting for roughly 3% of cancer cases worldwide. According to the most recent GLOBOCAN data, 544,352 new cases of NHL were diagnosed worldwide in 2020 (1, 2).
Classifications of non-Hodgkin’s lymphomas have undergone numerous refinements and completions over time, ranging from classifications based on the histological and immunological profile of tumors (Rappoport classification (3), Kiel classification (4), Lukes and Collins classification (5), etc.) to current classifications systems (World Health Organization Classifications from 2016 and 2022 and The International Consensus Classification of Mature Lymphoid Neoplasms (6–8)) which divides non-Hodgkin’s lymphomas according to histological, immunohistochemical and gene expression profiles (GEP).
Healthcare practitioners prefer also to group NHL subtypes based on the speed of disease progression. For example, indolent B-cell lymphomas, such as follicular lymphomas, marginal zone lymphoma, small lymphocytic lymphoma, proceed as chronic incurable diseases, in which the clinical course is slow-progressing and oligosymptomatic for a long period of time but for the treatment of which, however, regular exposure to toxic cytostatic drugs and/or radiation therapy is required (9).
On the other hand, aggressive B-cell lymphomas represent a heterogeneous category of lymphomas that may involve precursor lymphoid neoplasms (B-lymphoblastic leukaemia/lymphoma NOS and B-lymphoblastic leukaemia/lymphoma with recurrent genetic abnormalities) as well as a variety of mature B-cell lymphomas, like Burkitt lymphoma, mantle cell lymphoma, primary effusion lymphoma, and diffuse large B-cell lymphoma. They have aggressive behavior, with frequent extranodal involvement and require immediate treatment, otherwise, resulting in patient’s rapid desmise. Although modern treatment regimens can increase survival in certain patients with aggressive large-cell lymphomas (approximately 60% in diffuse B-large-cell lymphoma, about 30% in peripheral T-cell lymphomas), disease progression remains the leading cause of death (1, 9–11).
The notable progress in recent years should be attributed to the advances in molecular genetics. These advancements have enabled a shift from analyzing individual genes and markers to conducting comprehensive studies on multiple genes or their expressed products concurrently, particularly in the context of cancer research (12–14). The emergence of high-tech genome-wide research methods and their integration into publicly available databases make it possible to obtain more detailed information about the mechanisms of oncogenesis, explain the division of tumors by histological types, differentiate gene networks that determine the main stages of tumor pathogenesis, and study the mechanisms of drug resistance (15–18).. The study of gene expression profiles in certain types and subtypes of tumors makes it possible to identify additional markers associated with the clinical course, the risk of invasion and metastasis, as well as to supplement and refine the existing classification or propose a new one based on the molecular characteristics of the tumor (19–22).
The beginnings of gene study date back to Sanger et al. who introduced his chain termination method for sequencing DNA in 1977 (23), that quickly gained great acceptance and popularity around the world, becoming in fact the first generation of the DNA sequencing technology (24, 25).
Next-generation sequencing(NGS) is a method of sequencing multiple DNA or RNA products in parallel. This technique is also known by other names (eg, short-read sequencing, deep sequencing, second-generation sequencing). In contrast to Sanger sequencing, the speed of sequencing and the amount of DNA sequence data generated by NGS are exponentially higher, and the cost of production is significantly lower (26). The most complete molecular assessment lymphoma genetics was obtained by using whole genome sequencing of all coding sequences (exome) by high-throughput next-generation parallel sequencing (WES). WES studies were performed for each of the major immunomorphological subtypes of lymphomas: DLBCL, Burkitt lymphoma, follicular lymphoma, mantle, splenic marginal zone lymphoma, and peripheral T-cell lymphomas (27).
The contemporary diagnosis of NHLs is based on morphological and immunophenotypic studies, as well as chromosomal and molecular analyses, which are indicated as diagnostic procedures to establish high-precision diagnoses (28). The current recommendations, however, do not provide clear stipulations for the conditions of sequencing techniques used for NHL diagnosis and prognosis. There is no uniform strategy at this time, and aspects such as gene selection, sequencing platform, read depth, and variant analysis may vary among laboratories. Therefore, standardization of the panels is needed especially taking into account the fact that the NGS panels of the lymphoid lineage are becoming more accessible for clinical practice.
The purpose of this article is to provide a comprehensive overview of the gene panels that are identified in different NHL types by the use of NGS techniques.
2 B-cell lymphomas
B-cell lymphomas represent the predominant type of NHL diagnosed globally. About 85-90% of NHL cases are derived from B cells, whereas the remaining lymphomas originate from T cells or NK cells (6, 29). This epidemiological circumstance likely explains the greater inclination for studying the genomic and transcriptomic features of these neoplasms by various research groups. In the following sections, we will describe the specifics of gene expression profiles in some of the most common types of NHL. Table 1 includes a summary of the most common GEP associated with different types of B-cell lymphomas.
2.1 Diffuse large B cell lymphoma
Diffuse large B cell lymphoma (DLBCL) is the most frequent type of non-Hodgkin lymphoma in the world, accounting for 30–40% of all occurrences depending on the geographical region (44).
Traditionally, DLBCL cases were classified according to cell-of-origin (COO), with two different subtypes described: germinal center B-cell like (GCB) and activated B-cell like (ABC), and with about 10–15 percent of cases remaining unclassifiable (45).
Patients who have the GCB subtype have a better prognosis than those who have the ABC subtype. Although COO can help predict the outcome, the GCB and ABC subtypes are still very heterogeneous raising the question of a more accurate prognostic stratification (6, 44, 46).
In 2017, Reddy et al. conducted a study that used whole-exome and transcriptome sequencing of tumors from 1,001 newly diagnosed DLBCL patients to determine genetic drivers of the disease and establish probable links to clinical outcomes (47).
As a result, the authors identified 150 genes that are directly involved in the pathogenesis of DLBCL. These genes can be classified into four main categories:
1) genes involved in signaling pathways (for example, MTOR, PIK3R1, PIM2, BTK);
2) genes associated with transcription and translation in the cell (for example, SF3B1, XPO1, HIST1H1E);
3) genes responsible for the stages of B cell differentiation (for example, EBF1, IRF4, PAX5, POU2F2, YY1);
4) genes responsible for cell growth and proliferation (for example, MYC, CHD8, BCL2).
Also, MYD88 was chosen as a critical mutation in the ABC subtype, whereas XPO1 was chosen as an essential mutation in GCB DLBCL. The publication is limited by the lack of explanation in case of DNA mutation-based disease clustering, focusing only on RNA-based or translocation-based classification with DNA mutations (47).
Chapuy et al. in 2018, proposed a DNA-based classification of DLBCL. In this study WES was performed on 304 patients samples. C1–C5 were the names given to these clusters, permitting the classification of ABC and GCB-DLBCL cases into two different groups with favorable and adverse outcomes. ABC subtypes were divided into two groups: a lower risk group with a putative marginal zone origin (C1) characterized by NOTCH2 mutations/BCL6 translocation, and one with a higher risk (C5) with chromosome 18q gain with BCL2 and MALT1 gene overexpression and CD79B and MYD88 mutations. The C2 subgroup was associated with biallelic loss or mutation of TP53 and widespread somatic copy number alterations. Additionally, C2 tumors frequently showed copy loss of 9p21.13/CDKN2A and 13q14.2/RB1. Two other subtypes of the GCB were identified (C3 and C4). C4, which was associated with low risk disease and revealed mutations impacting the BCR/PI3K, JAK/STAT, and BRAF pathways. Conversely, mutations impacting BCL2 translocation, PTEN, and epigenetic mediators such as KMT2D, CREBBP, and EZH2 were all linked to the poorer prognosis of the C3 subgroup (17).
Schmitz et al. used whole-exome and transcriptome sequencing, DNA copy number analysis, and deep targeted amplicon sequencing to examine data from 574 DLBCL patients. As a result, four different subtypes of DLBCL were identified: MCD (based on the presence of MYD88L265P and CD79B mutations), BN2 (based on BCL6 fusions and NOTCH2 mutations), N1 (based on the presence of NOTCH1 mutations), and EZB (based on the presence of EZH2 mutations and BCL2 translocations) (30). There are numerous parallels between Chapuy’s and Schmitz’s subgroups, including the following: C1 resembles the BN2 group, C3 overalps EZB, and C5 is similar to MCD.
A follow-up to the findings of Schmitz et al. was the research done by Wright et al. examining the initially unclassified cases. The researchers identified two other subtypes, one with high levels of aneuploidy and mutation of TP53, and the second ST2 (SGK1 and TET2 mutations). These corresponded closely to the Chapuy subgroups, C2 and C4. Thus, each of the five Chapuy clusters could now be mapped to one of the Schmitz genetic subgroups. LymphGen is the name given to this classification at the moment (31). The correlation between these three molecular classifications is shown in Figure 1.
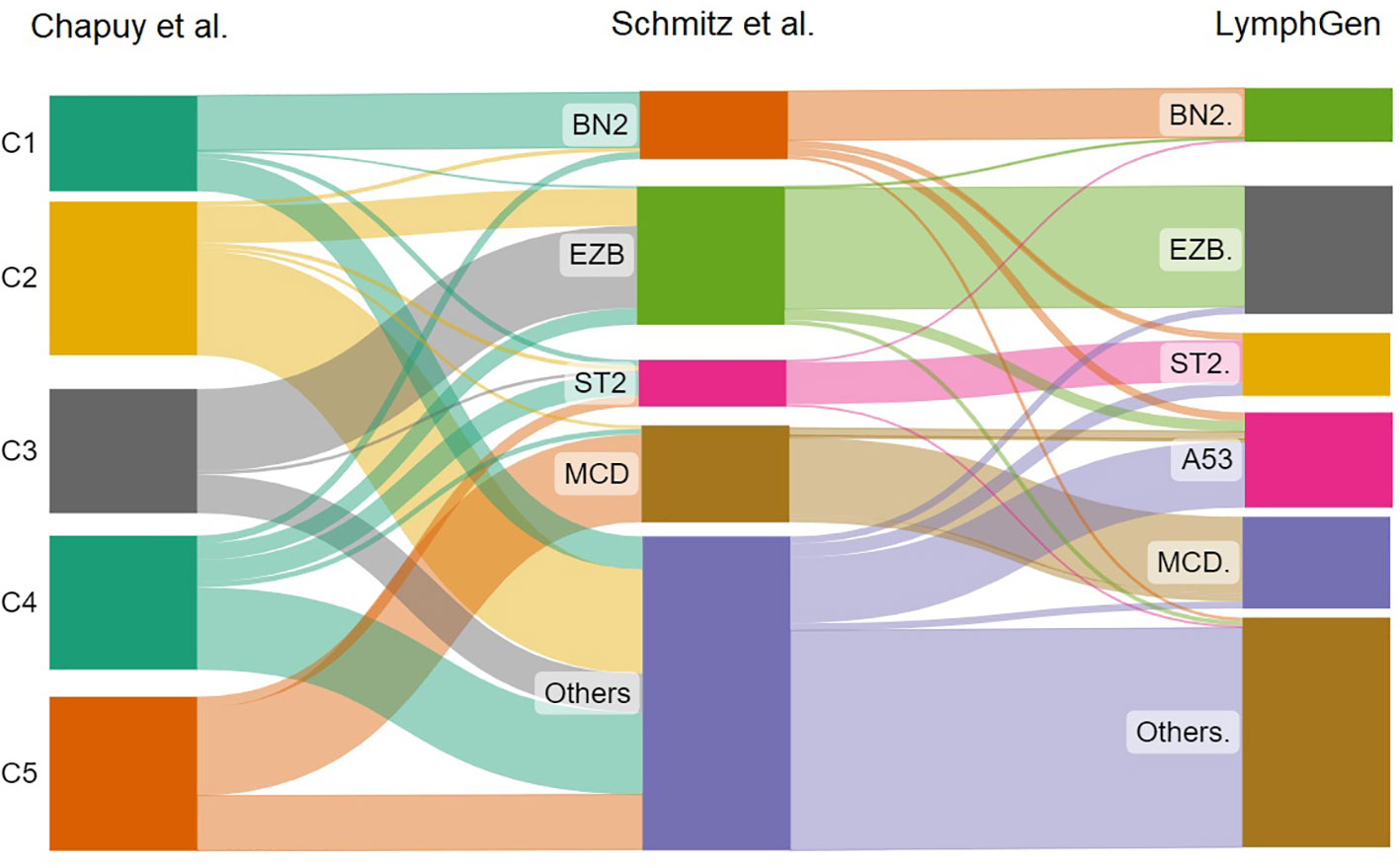
Figure 1 The sankey plot shows the relative proportion of cases from the Chapuy et al. classification that correlates with the Schmitz et al. molecular groups and LymphGen classification.
Recently, studies incorporating clinical, biochemical, and genetic data into multimodal machine learning models have yielded to the elaboration of a gene expression profiling tool that is offering encouraging results in terms of more accurate DLBCL prognostication (48).
2.2 Follicular lymphoma
Follicular lymphoma (FL) represents the second most common non-Hodgkin lymphoma and the most prevalent indolent lymphoma. The chromosomal translocation t(14;18)(q32:q21), in which the immunoglobulin heavy chain (IGH) enhancer region at 14q32 and the B-cell lymphoma 2 (BCL2) gene at 18q21 are juxtaposed, is the hallmark of FL, which is identified in about 90% of cases (49). NGS research has been useful not only in creating a list of genomic events that occur in addition to t(14;18), but also in identifying new potential genetic drivers. The high frequency of mutations affecting epigenetic control is the second distinguishing feature of FL.
Deregulation of such processes (e.g. aberrant DNA hypermethylation) has been recognized as a central feature of hematologic malignancies, and FL in particular, observed in 80% of cases (50). The histone methyltransferases KMT2D (90%) and EZH2 (25%) as well as the histone acetyltransferases CREBBP (30–60%) and EP300 (9%) are among the most commonly mutated genes (51). A clinical-genomic score was created using seven genes including those mentioned above, to predict Failure-Free Survival (FFS) and Overall Survival (OS) (33).
In the era of FL treatment with conventional chemotherapy, the scientists found that mutations in EP300, FOXO1, CREBBP, and CARD11 (providing poor prognosis) and MEF2B, ARID1A, and EZH2 (providing good prognosis) in association with clinical parameters of the FLIPI score, improved PFS and OS prediction. Furthermore, the m7-FLIPI was able to reclassify almost half of the high-risk FLIPI patients into a low-risk m7-FLIPI group, mainly through the discovery of EZH2 mutations (33, 34). An another research on the m7-FLIPI score across different populations with FL suggests that this molecular score has no impact on patients with FL, treated in the first line, with chemotherapy-free regimens (52, 53). In addition, another study has shown that four mutant genes in FL samples (NOTCH2, DTX1, UBE2A, and HIST1H1E) were linked to shorter transformation time to DLBCL (35).
In a recent study performed by Gao et al., for the first time, they studied the genomic and transcriptomic characteristics that could predict progression of disease within 24 months (POD24). As a result of this study, they identified genomic markers that are able to predict POD24 in patients with FL. So, HIST1H1D, known as a driver mutation, was significantly correlated with POD24. Furthermore, gains of 6q22.2 (HIST1H1D) and 18q21.33 (BCL2) and loss of 1p36.13 (NBPF1) predicted POD24 independent of FLIPI (54).
2.3 Mantle cell lymphoma
Mantle Cell lymphoma (MCL) is an incurable type of aggressive lymphoma with a median survival of approximately 5 years (38, 55). The revised World Health Organization classification from 2016 identified two molecular routes of MCL dividing them in cases of Nodal MCL and Leukemic non-nodal MCL (10-20% of cases, more indolent) (6, 39).
More than 30 years have passed since the first report of the well-known hallmark genetic alteration t (11,14) (q13; q32)/CCND1::IGH, which is seen in 95 percent of MCL cases. The result of juxtaposition of heavy-chain immunoglobulin (IGH) enhancer region (on 14q32) next to CCND1 (on 11q13), results in its overexpression of Cyclin D1 (56, 57).
MCL was divided into two categories by the WHO classification: classical MCL and indolent leukemic non-nodal MCL. Indolent leukemic non-nodal MCL is characterized by mutated IGHV and primarily SOX11 negativity, as well as peripheral blood, bone marrow, and occasionally splenic involvement but no major nodal involvement. Classical MCL is characterised by unmutated or minimally mutated IGHV and mostly SOX11 positivity (6, 58).
In recent years, genomic techniques have revealed mutations with prognostic implication for MCL. A recent meta-analysis summarized the most common mutations discovered using molecular methods in MCL patients. Among the most common mutant genes were: ATM (43.5%) followed by TP53 (26.8%), CDKN2A (23.9%), and CCND1 (20.2%). Aberrations in IGH (38.4%) and MYC (20.8%) were also discovered, mostly by cytogenetic techniques. Other prevalent baseline mutations included NSD2 (15%), KMT2A (8.9%), S1PR1 (8.6%), and CARD11 (8.5%). The authors propose that a panel of these genes shall be added to NGS panels (55). CDNK2A deletion, ATM, NOTCH1/2, NSD2 mutations were highlighted as markers of poor prognosis. Other mutations were described to have potential diagnostic, therapeutic and predictive role, such as those in BIRC3, BTK, PLCG2, SMARCA4 and MAP3K14 (40, 59, 60).
Agarwal et al. discovered genetic patterns that separates responders and nonresponders in a prospective study performed on patients with MCL. ATM mutations were found in the majority of patients who had a complete response, while chromosome 9p21.1-p24.3 loss and/or mutations in SWI-SNF chromatin-remodeling complex components were found in all patients with primary resistance and two-thirds of patients with relapsed disease (61).
TP53 mutation is another significant indicator of MCL prognosis. Patients with TP53 mutation were related to the blastoid morphology of MCL, elevated Ki-67, high-risk MIPI, and MIPI-c. When compared to TP53-unmutated cases, TP53 mutations lead to inferior results in terms of response following both induction and autologous stem cell transplantation, as well as shorter PFS (62, 63).
Recently, Yi et al. (37) conducted a WES study on 152 samples of MCL patients, classifying MCL molecularly into 4 distinct clusters (C1-C4). C1 had a 5-year OS of 100% and it was associated with mutant immunoglobulin heavy variable (IGHV), CCND1 mutation, amp(11q13), and active B cell receptor (BCR) signaling. C2 was linked with del(11q)/ATM mutations, activation of NF-kB and DNA repair pathways, and it was associated with a 5-year OS of 56.7%. C3 was characterized by mutations in SP140, NOTCH1, and NSD2, as well as downregulation of BCR signaling and MYC targets, and had a 5-year OS of 47%. C4 included patients with del(17p)/TP53 mutations, del(13q), and del(9p), as well as active MYC pathway and hyperproliferation signatures, and it was associated with a poor prognosis (5-year OS of only 14.2%) (37).
3 NK/T-cell lymphomas
Malignant T/NK lymphomas(TNKL) are a distinct group of non-Hodgkin’s lymphomas that account for an estimated 10-15% of the total NHL, with a higher incidence in certain geographic areas (Asia, South America) (64, 65). TNKL, like other malignant proliferative disorders, exhibit genetic instability and chromosomal abnormalities, which combined induce malignant transformation. Therefore, the use of NGS and GEP represent a chance to discover new patterns that can have real prognostic and theranostic impact on TNKL. Despite this, for various reasons, compared to B cell lymphomas, there are fewer reports of the use of NGS, WES, WGS in the case of TNKL. Next, we will attempt to compile the existing data which has genuine prognostic or therapeutic implications. Similarly, data on GEP within distinct TNKLs will be reported in Table 2 separately.
3.1 Angioimunoblastic T-cell lymphoma
AITL is a distinct clinicopathologic, and genetic subtype of peripheral T-cell lymphoma (PTCL). AITL is the second most prevalent PTCL subtype worldwide, accounting for 15% to 20% of all PTCL cases, and the most common subtype in the Western world, accounting for more than 30% of all PTCL cases (18, 84, 85).
In 2007, De Leval et al. identified the cell of origin being the T follicular helper cell (TFH) based on the use of gene expression profile studies (86). CD28 (9.4–11.3%), DNMT3A (20–30%), IDH2 (20–45%), TET2 (47–83%), and RHOA mutations (50–70%) are the most common genetic alterations detected in AITL.
The RHOA G17V is the result of a valine substitution for glycine at aminoacid 17, which causes the protein to lose its ability to bind GTP. Furthermore, patients with RHOA mutations are thought to have enhanced microvascular density and to exhibit a high number of follicular helper T-cell markers (87). In contrast to other mutations such as TET2 and DNMT3A, which can occur in both tumor and nontumor cells of AITL patients, RHOA mutations appear to be limited to tumor cells, indicating that they play an important role in AITL pathogenesis (67, 88).
TET2 encodes a 2-oxoglutarate/Fe2+–dependent oxygenase that participates in the epigenetic control of gene expression by catalyzing the oxidation of DNA 5-methylcytosine to 5-hydroxymethylcytosine. TET2 was first described as a tumor suppressor in myeloid neoplasms, but afterward, a high loss of function in TET2 was identified in PTCL and especially AITL (89–91). TET2 mutations are also found in hematopoietic cells in a borderline disease called clonal hematopoiesis of indeterminate potential (CHIP), and are associated with the risk of clonal malignancy over time. The fact that not all people with CHIP associated with a TET2 mutation can develop malignant haemopathy indicates that it is necessary to acquire secondary mutations for the malignant transformation to take place (92, 93). Loss-of-function mutations in DNMT3A, a DNA methyltransferase, are common in AITL and frequently co-occur with TET2 mutations (68). Cooperation between DNMT3A and TET2 mutations has been found to result in malignant transformation in mice models (94).
In the mitochondria, the isocitrate dehydrogenase 2 (IDH2) gene normally encodes enzymes that convert isocitrate to alpha-ketoglutarate (2-oxoglutarate, aKG). The neomorphic enzymatic activity of the mutant enzymes catalyzes the conversion of alpha ketoglutarate to 2-hydroxyglutarate (2-HG), an oncometabolite that inhibits the function of the TET family of enzymes (68). AITL is the only type of PTCL in which recurrent IDH2 mutations appear. Mutations in position R172 of IDH2 are specific for AITL and typically co-occur with TET2 mutations (68).
TET2, DNMT3A, and IDH2 mutations occur early in hematopoietic stem cell development, contributing to increased clonal hematopoiesis and greater hematopoietic stem cell self-renewal, but they do not impact T cell differentiation and are therefore considered non-lineage impact mutations, according to a recent review by Yu et al. (91) Late in the T-cell lineage differentiation, mutations in RHOA, VAV1, VAV::STAP2, CD28, CTLA::CD28, ITK::SYK, PLCy1, and TNFRSF21 induce malignant T-cell transformation (91). Considering hypermethylation as the fundamental pathogenetic mechanism of AITL, the use of hypomethylating drugs appears to be a reasonable therapeutic option, and is currently in the clinical trials phase (95, 96).
3.2 Mycosis fungoides/Sézary syndrome
The nosological entities known as Mycosis fungoides (MF) and Sézary Syndrome (SS) account for about 75% of all Cutaneous T-cell lymphomas (97). SS is a generalized form of the condition that manifests itself clinically with erythrodermic lesions along with lymph node and blood involvement at onset. MF is a disorder with limited expansion in the skin area, being associated with a good prognosis (77, 98).
The difference in COO can explain the clinical distinctions between MF and SS. MF and SS develop from different subtypes of CD4 + memory T cells; The source cell in the case of MF are T resident memory (Trm) cells exhibiting CCR4 +/CLA +/L-selectin-/CCR7– (TRM), which have a higher tropism to the skin and epithelial barriers, while in the case of SS the COO are T-cell central memory cells (Tcm) that express CCR4 +/Lselectin +/CCR7+, and these cells have the ability to migrate between skin, lymph nodes and blood (99).
Recent NGS research in MF/SS has found a high rate of C>T transitions (40–74%), a mutational signature linked to ultraviolet B (UVB) exposure that is uncommon to be seen in other hematological neoplasms (100–102).
Litvinov et al. described 17 genes (CCL18, CCL26, FYB, T3JAM, MMP12, LEF1, LCK, ITK, GNLY, IL2RA, IL-26, IL-22, CCR4, GTSF1, SYCP1, STAT5A, TOX) that identified those patients who are at risk of progression and differentiated MF/SS from benign dermatological diseases (103).
The accuracy of diagnosing SS using distinct gene panels has been demonstrated by Nebozhyn et al. and Michel et al. in two separate papers. Nebozhyn et al. used a panel of five genes (STAT4, GATA3, PLS3, CD1D, and TRAIL) that could correctly separate patient samples from controls with 90% accuracy. On the other hand, Michel et al. used a signature based on four genes (PLS3, Twist1, CD158k/KIR3DL2, and NKp46) with the ability to separate SS samples from control samples in 100% of cases. They noted that only the Twist1 gene has a diagnostic sensitivity of SS of 91% (104, 105).
The largest retrospective WES evaluation of CTCL to date utilized publicly available sequencing data from nine studies, comprising 220 patients with CTCL, which included 186 SS patients and 25 MF patients (106). This study identified fifty-five putative driver genes and implicated seventeen gene mutations previously not described as being involved in CTCL. These novel mutations target pathways that are involved chromatin remodeling (BCOR, KDM6A, SMARCB1, TRRAP), immune surveillance (CD58, RFXAP), MAPK signaling (MAP2K1, NF1), NF-κB signaling (PRKCB, CSNK1A1), PI-3-kinase signaling (PIK3R1, VAV1), RHOA/cytoskeleton remodeling (ARHGEF3), RNA splicing (U2AF1), T-cell receptor signaling (PTPRN2, RLTPR), and T-cell differentiation (RARA) (106). The JAK/STAT pathway, which includes JAK1, JAK3, STAT3, and STAT5B, is frequently affected by gain-of-function mutations and amplifications in CTCL resulting in the hyperactivation of this signaling pathway (106). Nevertheless, genomic studies in MF/SS do not allow to have a complete picture as in B cell lymphomas on the prognostic stratification of cases or the establishment of molecular classification, this will most likely be the moment of interest for further investigations.
4 The use of liquid biopsy in non-Hodgkin lymphomas
Currently, the diagnosis of non-Hodgkin’s lymphoma is based on excisional biopsy of the tumoral tissue. Tissue biopsies, however, are invasive methods of diagnosis with a several disadvantages, such as the risks of tissue biopsy (bleeding, infection, functional disability, etc.), the difficulty of obtaining biopsy samples, and do not allow the dynamic heterogeneity of the case to be assessed (107).
The concept of liquid biopsy which is a non-invasive technique, and can be used to explore the entire mutational landscape of the lymphoma. Liquid biopsy allows for an evaluation of lymphoma at the stage of diagnosis, and prognostic stratification.
Both healthy cells and malignant cells release nucleic acids (DNA, mRNA, and miRNA) into body fluids like the cerebrospinal fluid, peripheral blood, and urine. The term “cell-free DNA” (cfDNA) refers to non-cell-bound DNA fragments discovered in the circulatory system. cfDNA often contains both normal DNA and circulating tumor DNA (ctDNA). The lysis of circulating tumor cells (CTCs), apoptosis, necrosis, or the release of DNA from tumor cells into the bloodstream are possible origins for the tumor-specific part of cfDNA. Because cfDNA can emerge from both malignant and non-malignant cells, assays for the detection of ctDNA are more specific for tumor identification in the case of non-Hodgkin’s lymphomas (108, 109).
Close monitoring of NHL cases by using ctDNA quantification of liquid biopsies can identify the genetic heterogeneities that appear between the primary tumor and the primary areas of metastasis, as well as between various locations of metastases. This information can then be used to find biomarkers indicative of spreading mechanisms and lymphomatous transformation. Multiple studies including a recent meta-analysis, have shown higher levels of cfDNA in cancer patients compared with healthy controls. Different subsets of lymphoma can be distinguished at the time of diagnosis with the help of NGS-based analysis of ctDNA. Furthermore, ctDNA load strongly reflects tumor burden, as it appears to correlate significantly with lactate dehydrogenase (LDH) and the International Prognostic Index (IPI), as observed in DLBCL, NKTCL and other types of lymphomas (110–113). In DLBCL, interim ctDNA monitoring during therapy directly evaluates tumor kinetics response and foretells early treatment failure. The determination of interim levels of ctDNA has a greater sensitivity than existing imaging methods, creating a so-called “window of opportunity” during which, the earlier initiation of salvage therapy prior to clinical relapse to be diagnosed, has the potential to improve outcomes (114).
In the case of DLBCL, initial levels of ctDNA are significantly associated with the International Prognostic Index (IPI), total metabolic tumor volume (TMTV), lactate dehydrogenase (LDH) concentrations, and the Ann Arbor stage. Pretreatment ctDNA concentrations have been demonstrated to be highly accurate predictors of clinical outcomes in univariate and multivariate analysis in those trials, and hence gain prognostic importance (43, 115, 116). ctDNA in DLBCL can also be used for the real-time assessment of treatment response, increases in ctDNA levels and changes in KMT2D mutation status have been found to be useful indicators of disease progression (117). The depth of response is an important predictor of outcomes in the post-treatment surveillance of NHL subtypes. Relapsed NHL likely originates from MRD below the current level of detection, and a recent systematic review demonstrated that between 7% and 20% of DLBCL patients in remission by PET scans will ultimately relapse (118). A recent MRD study on DLBCL patients treated with CAR-T cell therapy has shown better sensitivity and predictive value for progression to treatment than the PET scan (119). This study, among others, suggests that liquid biopsy and NGS would create an excellent platform for assessing the efficacy of treatments (116).
In the case of extranodal natural killer/T cell lymphoma (ENKTCL), a recent study explored the use of ctDNA methylation markers for diagnosing, continuously monitoring, and predicting the prognosis. This research has proposed a score formed by 7 ctDNA markers, namely HLX-AS1, MIR12123, CHST12, DLK1, LINC02115, MIR3973, and NCAM, which achieves over 90% accuracy in distinguishing ENKTCL from nasopharyngeal carcinoma, nasopharyngitis, and normal conditions (120).
However, despite the encouraging data of NHL evaluation by liquid biopsies, few validation studies have been published at the moment (121), with the vast majority of the data presented requiring validation in further research.
5 NGS use for a personalized approach and future perspective of use
NHL remains a condition treated primarily whit chemoimmunotherapy. The standard of care for years has been frontline R-CHOP, despite multiple attempts to investigate more aggressive regimens like R-DA-EPOCH or incorporate new therapies like obinutuzumab, bortezomib, or ibrutinib. Frontline R-CHOP cures around 60% of DLBCL cases. Nowadays, DA-EPOCH-R is utilized as the first-line therapy for double/triple hit lymphomas, primary mediastinal B cell lymphoma, and HIV-associated DLBCL.
The discovery by Wilson et al. that the co-occurrence of mutations in MYD88 and CD79B can predict response to ibrutinib is an illustration of possible clinical utility of genomic profile data in DLBCL, that may have a real impact in the practice (122).
In MCL, the data obtained through genome sequencing allowed the identification of a group of patients in whom there are inactivating mutations in the SWI-SNF chromatin-remodeling complex that lead to BCL-XL upregulation and subsequent resistance to the therapeutic combination with ibrutinib and venetoclax (61).
Many T-cell lymphomas harbor mutations in epigenetic regulatory genes, such as TET2, DNMT3A, and IDH2, but they are most frequently seen in AITL. Therefore, the use of drugs from the class of HDAC inhibitors or demethylating agents may have a potential beneficial role.
Recently Huang et al. have proposed the DrugComboExplorer, a computational systems biology tool that concurrently integrates pharmacogenomics profiles of 5585 drugs and bioactive compounds from the NIH LINCS program (Library of Integrated Network-based Cellular Signatures) and genomic profiles for specific cancer types (i.e., signaling pathways, interactome, and pharmacological data). This tool does large-scale medication combination prediction and integrates multi-omics data from cancer patients including non-Hodgkin lymphomas (123).
In conclusion, the knowledge provided by the genomic mapping of non-Hodgkin’s lymphomas in near future will allow the targeting of molecular pathways that cause treatment refractoriness or, on the contrary, the inhibition of which is vital in stopping uncontrolled tumor proliferation. Personalized medicine will not only select a single mutation that it will inhibit through the action of a drug, but by selecting molecular targets that have a synergistic costimulatory or inhibitory effect thus self-potentiating. The increased interest in this field confirms that the integration of genomic and transcriptomic data will allow a better understanding of the therapy of malignant lymphomas and of tumor resistance.
Author contributions
VT: Validation, Writing – original draft. AM: Validation, Writing – review & editing. CS: Validation, Writing – original draft. MR: Validation, Writing – original draft. SB: Validation, Writing – original draft. MF: Validation, Writing – review & editing.
Funding
The author(s) declare that no financial support was received for the research, authorship, and/or publication of this article.
Conflict of interest
The authors declare that the research was conducted in the absence of any commercial or financial relationships that could be construed as a potential conflict of interest.
Publisher’s note
All claims expressed in this article are solely those of the authors and do not necessarily represent those of their affiliated organizations, or those of the publisher, the editors and the reviewers. Any product that may be evaluated in this article, or claim that may be made by its manufacturer, is not guaranteed or endorsed by the publisher.
References
1. Thandra KC, Barsouk A, Saginala K, Padala SA, Barsouk A, Rawla P. Epidemiology of non-hodgkin’s lymphoma. Med Sci (2021) 9:5. doi: 10.3390/MEDSCI9010005
2. Sung H, Ferlay J, Siegel RL, Laversanne M, Soerjomataram I, Jemal A, et al. Global cancer statistics 2020: GLOBOCAN estimates of incidence and mortality worldwide for 36 cancers in 185 countries. CA Cancer J Clin (2021) 71:209–49. doi: 10.3322/CAAC.21660
3. Rappaport H. Tumors of the hematopoietic system. In: Atlas of tumor pathology sect. 3. Fasicle S. Washington: DC Armed Forces Institute of pathology (1966). p. 97–161.
4. Lennert K, Stein H, Kaiserling E. Cytological and functional criteria for the classification of Malignant lymphomata. Br J Cancer (1975) 31:29–43.
5. Lukes RJ, Collins RD. Immunologic characterization of human Malignant lymphomas. Cancer (1974) 34:1488–503. doi: 10.1002/1097-0142(197410)34:8+<1488::AID-CNCR2820340822>3.0.CO;2-C
6. Swerdlow SH, Campo E, Pileri SA, Lee Harris N, Stein H, Siebert R, et al. The 2016 revision of the World Health Organization classification of lymphoid neoplasms. Blood (2016) 127:2375–90. doi: 10.1182/BLOOD-2016-01-643569
7. Alaggio R, Amador C, Anagnostopoulos I, Attygalle AD, Araujo IB de O, Berti E, et al. The 5th edition of the world health organization classification of haematolymphoid tumours: lymphoid neoplasms. Leuk (2022) 36(7):1720–48. doi: 10.1038/s41375-022-01620-2
8. Campo E, Jaffe ES, Cook JR, Quintanilla-Martinez L, Swerdlow SH, Anderson KC, et al. The international consensus classification of mature lymphoid neoplasms: a report from the clinical advisory committee. Blood (2022) 140:1229–53. doi: 10.1182/blood.2022015851
9. Binder AF, Brody JD. Non-hodgkin lymphoma. Oncology (2020) 342–53. doi: 10.1002/9781119189596.ch30
10. Beham-Schmid C. Aggressive lymphoma 2016: revision of the WHO classification. Memo - Mag Eur Med Oncol (2017) 10:248–54. doi: 10.1007/S12254-017-0367-8/FIGURES/5
11. Tomacinschii V, Robu M, Buruiana S, Finciuc V, Grecu A, Dudnic C, et al. Impact of targeted treatment in non-Hodgkin’s lymphoma with primary lymph node involvement. Mold Med J (2021) 64:56–61. doi: 10.52418/MOLDOVAN-MED-J.64-5.21.11
12. Stratton MR, Campbell PJ, Futreal PA. The cancer genome. Nature (2009) 458:719–24. doi: 10.1038/NATURE07943
13. Forbes SA, Beare D, Boutselakis H, Bamford S, Bindal N, Tate J, et al. COSMIC: somatic cancer genetics at high-resolution. Nucleic Acids Res (2017) 45:D777. doi: 10.1093/NAR/GKW1121
14. Ding Z, Wang N, Ji N, Chen ZS. Proteomics technologies for cancer liquid biopsies. Mol Cancer (2022) 21:1–11. doi: 10.1186/S12943-022-01526-8/FIGURES/2
15. Papaemmanuil E, Gerstung M, Bullinger L, Gaidzik VI, Paschka P, Roberts ND, et al. Genomic classification and prognosis in acute myeloid leukemia. N Engl J Med (2016) 374:2209–21. doi: 10.1056/NEJMOA1516192
16. Nadeu F, Delgado J, Royo C, Baumann T, Stankovic T, Pinyol M, et al. Clinical impact of clonal and subclonal TP53, SF3B1, BIRC3, NOTCH1, and ATM mutations in chronic lymphocytic leukemia. Blood (2016) 127:2122–30. doi: 10.1182/BLOOD-2015-07-659144
17. Chapuy B, Stewart C, Dunford AJ, Kim J, Kamburov A, Redd RA, et al. Molecular subtypes of diffuse large B cell lymphoma are associated with distinct pathogenic mechanisms and outcomes. Nat Med (2018) 24:679–90. doi: 10.1038/S41591-018-0016-8
18. Vose JM, Neumann M, Harris ME. International peripheral T-cell and natural killer/T-cell lymphoma study: pathology findings and clinical outcomes. J Clin Oncol (2008) 26:4124–30. doi: 10.1200/JCO.2008.16.4558
19. Visco C, Li Y, Xu-Monette ZY, Miranda RN, Green TM, Li Y, et al. Comprehensive gene expression profiling and immunohistochemical studies support application of immunophenotypic algorithm for molecular subtype classification in diffuse large B-cell lymphoma: a report from the International DLBCL Rituximab-CHOP Consortium Program Study. Leukemia (2012) 26:2103–13. doi: 10.1038/LEU.2012.83
20. Hoppe MM, Jaynes P, Shuangyi F, Peng Y, Sridhar S, Hoang PM, et al. Patterns of oncogene coexpression at single-cell resolution influence survival in lymphoma. Cancer Discovery (2023) 13:1144–63. doi: 10.1158/2159-8290.CD-22-0998
21. Ramis-Zaldivar JE, Gonzalez-Farré B, Balagué O, Celis V, Nadeu F, Salmerón-Villalobos J, et al. Distinct molecular profile of IRF4-rearranged large B-cell lymphoma. Blood (2020) 135:274–86. doi: 10.1182/BLOOD.2019002699
22. Sukswai N, Lyapichev K, Khoury JD, Medeiros LJ. Diffuse large B-cell lymphoma variants: an update. Pathology (2020) 52:53–67. doi: 10.1016/J.PATHOL.2019.08.013
23. Sanger F, Nicklen S, Coulson AR. DNA sequencing with chain-terminating inhibitors. Proc Natl Acad Sci U.S.A. (1977) 74:5463–7. doi: 10.1073/PNAS.74.12.5463
24. Lander ES, Linton LM, Birren B, Nusbaum C, Zody MC, Baldwin J, et al. Initial sequencing and analysis of the human genome. Nature (2001) 409:860–921. doi: 10.1038/35057062
25. Abdellah Z, Ahmadi A, Ahmed S, Aimable M, Ainscough R, Almeida J, et al. Finishing the euchromatic sequence of the human genome. Nat (2004) 431:931–45. doi: 10.1038/nature03001
26. Linnarsson S. Recent advances in DNA sequencing methods – general principles of sample preparation. Exp Cell Res (2010) 316:1339–43. doi: 10.1016/J.YEXCR.2010.02.036
27. Intlekofer AM, Younes A. Precision therapy for lymphoma–current state and future directions. Nat Rev Clin Oncol (2014) 11:585–96. doi: 10.1038/NRCLINONC.2014.137
28. Zelenetz AD, Gordon LI, Chang JE, Christian B, Abramson JS, Advani RH, et al. NCCN guidelines® Insights: B-cell lymphomas, version 5.2021: featured updates to the NCCN guidelines. J Natl Compr Cancer Netw (2021) 19:1218–30. doi: 10.6004/JNCCN.2021.0054
29. Armitage JO, Gascoyne RD, Lunning MA, Cavalli F. Non-hodgkin lymphoma. Lancet (2017) 390:298–310. doi: 10.1016/S0140-6736(16)32407-2
30. Schmitz R, Wright GW, Huang DW, Johnson CA, Phelan JD, Wang JQ, et al. Genetics and pathogenesis of diffuse large B-cell lymphoma. N Engl J Med (2018) 378:1396–407. doi: 10.1056/NEJMOA1801445/SUPPL_FILE/NEJMOA1801445_DISCLOSURES.PDF
31. Wright GW, Huang DW, Phelan JD, Coulibaly ZA, Roulland S, Young RM, et al. A probabilistic classification tool for genetic subtypes of diffuse large B cell lymphoma with therapeutic implications. Cancer Cell (2020) 37:551–568.e14. doi: 10.1016/J.CCELL.2020.03.015
32. Sujobert P, Le Bris Y, De Leval L, Gros A, Merlio JP, Pastoret C, et al. The need for a consensus next-generation sequencing panel for mature lymphoid Malignancies. HemaSphere (2019) 3. doi: 10.1097/HS9.0000000000000169
33. Pastore A, Jurinovic V, Kridel R, Hoster E, Staiger AM, Szczepanowski M, et al. Integration of gene mutations in risk prognostication for patients receiving first-line immunochemotherapy for follicular lymphoma: A retrospective analysis of a prospective clinical trial and validation in a population-based registry. Lancet Oncol (2015) 16:1111–22. doi: 10.1016/S1470-2045(15)00169-2
34. Jurinovic V, Kridel R, Staiger AM, Szczepanowski M, Horn H, Dreyling MH, et al. Clinicogenetic risk models predict early progression of follicular lymphoma after first-line immunochemotherapy. Blood (2016) 128:1112–20. doi: 10.1182/BLOOD-2016-05-717355
35. González-Rincón J, Méndez M, Gómez S, García JF, Martín P, Bellas C, et al. Unraveling transformation of follicular lymphoma to diffuse large B-cell lymphoma. PloS One (2019) 14:e0212813. doi: 10.1371/JOURNAL.PONE.0212813
36. Nakamura S, Ponzoni M. Marginal zone B-cell lymphoma: lessons from Western and Eastern diagnostic approaches. Pathology (2020) 52:15–29. doi: 10.1016/J.PATHOL.2019.08.012
37. Yi S, Yan Y, Jin M, Bhattacharya S, Wang Y, Wu Y, et al. Genomic and transcriptomic profiling reveals distinct molecular subsets associated with outcomes in mantle cell lymphoma. J Clin Invest (2022) 132. doi: 10.1172/JCI153283
38. Maddocks K. Update on mantle cell lymphoma. Blood (2018) 132:1647–56. doi: 10.1182/BLOOD-2018-03-791392
39. Li S, Xu J, James You M. The pathologic diagnosis of mantle cell lymphoma. Histol Histopathol (2021) 36:1037–51. doi: 10.14670/HH-18-351
40. Jain P, Wang ML. Mantle cell lymphoma in 2022—A comprehensive update on molecular pathogenesis, risk stratification, clinical approach, and current and novel treatments. Am J Hematol (2022) 97:638–56. doi: 10.1002/AJH.26523
41. Tausch E, Beck P, Schlenk RF, Jebaraj BMC, Dolnik A, Yosifov DY, et al. Prognostic and predictive role of gene mutations in chronic lymphocytic leukemia: results from the pivotal phase III study COMPLEMENT1. Haematologica (2020) 105:2440–7. doi: 10.3324/HAEMATOL.2019.229161
42. Cohen JA, Bomben R, Pozzo F, Tissino E, Härzschel A, Hartmann TN, et al. An updated perspective on current prognostic and predictive biomarkers in chronic lymphocytic leukemia in the context of chemoimmunotherapy and novel targeted therapy. Cancers (Basel) (2020) 12. doi: 10.3390/CANCERS12040894
43. Scherer F, Kurtz DM, Newman AM, Stehr H, Craig AFM, Esfahani MS, et al. Distinct biological subtypes and patterns of genome evolution in lymphoma revealed by circulating tumor DNA. Sci Transl Med (2016) 8:364ra155. doi: 10.1126/SCITRANSLMED.AAI8545
44. Li S, Young KH, Medeiros LJ. Diffuse large B-cell lymphoma. Pathology (2018) 50:74–87. doi: 10.1016/J.PATHOL.2017.09.006
45. Alizadeh AA, Elsen MB, Davis RE, Ma CL, Lossos IS, Rosenwald A, et al. Distinct types of diffuse large B-cell lymphoma identified by gene expression profiling. Nature (2000) 403:503–11. doi: 10.1038/35000501
46. Susanibar-Adaniya S, Barta SK. 2021 Update on Diffuse large B cell lymphoma: A review of current data and potential applications on risk stratification and management. Am J Hematol (2021) 96:617–29. doi: 10.1002/AJH.26151
47. Reddy A, Zhang J, Davis NS, Moffitt AB, Love CL, Waldrop A, et al. Genetic and functional drivers of diffuse large B cell lymphoma. Cell (2017) 171:481–494.e15. doi: 10.1016/J.CELL.2017.09.027/ATTACHMENT/10836D88-A738-4F08-83E5-08ACDD907DEE/MMC5.XLSX
48. Mosquera Orgueira A, Diaz Arias JA, Cid Lopez M, Peleteiro Raindo A, Lopez Garcia A, Abal Garcia R, et al. Prognostic stratification of diffuse large B-cell lymphoma using clinico-genomic models: validation and improvement of the lymForest-25 model. HemaSphere (2022) 6. doi: 10.1097/HS9.0000000000000706
49. Morin RD, Mendez-Lago M, Mungall AJ, Goya R, Mungall KL, Corbett RD, et al. Frequent mutation of histone modifying genes in non-Hodgkin lymphoma. Nature (2011) 476:298. doi: 10.1038/NATURE10351
50. Pasqualucci L, Dominguez-Sola D, Chiarenza A, Fabbri G, Grunn A, Trifonov V, et al. Inactivating mutations of acetyltransferase genes in B-cell lymphoma. Nature (2011) 471:189–96. doi: 10.1038/NATURE09730
51. Li H, Kaminski MS, Li Y, Yildiz M, Ouillette P, Jones S, et al. Mutations in linker histone genes HIST1H1 B, C, D, and E; OCT2 (POU2F2); IRF8; and ARID1A underlying the pathogenesis of follicular lymphoma. Blood (2014) 123:1487–98. doi: 10.1182/BLOOD-2013-05-500264
52. Lockmer S, Ren W, Brodtkorb M, Østenstad B, Wahlin BE, Pan-Hammarström Q, et al. M7-FLIPI is not prognostic in follicular lymphoma patients with first-line rituximab chemo-free therapy. Br J Haematol (2020) 188:259–67. doi: 10.1111/BJH.16159
53. Lockmer S, Ren W, Ostenstad B, Brodtkorb M, Wahlin BE, Pan-Hammarstrom Q, et al. M7-FLIPI not valid in follicular lymphoma patients with first-line rituximab chemo-free therapy. Blood (2018) 132:4154. doi: 10.1182/BLOOD-2018-99-117949
54. Gao F, Liu H, Meng X, Liu J, Wang J, Yu J, et al. Integrative genomic and transcriptomic analysis reveals genetic alterations associated with the early progression of follicular lymphoma. Br J Haematol (2023) 1151–64. doi: 10.1111/bjh.18974
55. Hill HA, Qi X, Jain P, Nomie K, Wang Y, Zhou S, et al. Genetic mutations and features of mantle cell lymphoma: a systematic review and meta-analysis. Blood Adv (2020) 4:2927–38. doi: 10.1182/BLOODADVANCES.2019001350
56. Rimokh R, Berger F, Cornillet P, Wahbi K, Rouault J -P, Ffrench M, et al. Break in the BCL1 locus is closely associated with intermediate lymphocytic lymphoma subtype. Genes Chromosom Cancer (1990) 2:223–6. doi: 10.1002/GCC.2870020310
57. Leroux D, Le Marc’hadour F, Gressin R, Jacob M -C, Keddari E, Monteil M, et al. Non-Hodgkin’s lymphomas with t(11;14)(q13;q32): a subset of mantle zone/intermediate lymphocytic lymphoma? Br J Haematol (1991) 77:346–53. doi: 10.1111/J.1365-2141.1991.TB08582.X
58. Jiang P, Desai A, Ye H. Progress in molecular feature of smoldering mantle cell lymphoma. Exp Hematol Oncol (2021) 10:41. doi: 10.1186/s40164-021-00232-3
59. Clot G, Jares P, Giné E, Navarro A, Royo C, Pinyol M, et al. A gene signature that distinguishes conventional and leukemic nonnodal mantle cell lymphoma helps predict outcome. Blood (2018) 132:413–22. doi: 10.1182/BLOOD-2018-03-838136
60. Sethi S, Epstein-Peterson Z, Kumar A, Ho C. Current knowledge in genetics, molecular diagnostic tools, and treatments for mantle cell lymphomas. Front Oncol (2021) 11:739441/BIBTEX. doi: 10.3389/FONC.2021.739441/BIBTEX
61. Agarwal R, Chan YC, Tam CS, Hunter T, Vassiliadis D, Teh CE, et al. Dynamic molecular monitoring reveals that SWI-SNF mutations mediate resistance to ibrutinib plus venetoclax in mantle cell lymphoma. Nat Med (2019) 25:119–29. doi: 10.1038/S41591-018-0243-Z
62. Eskelund CW, Dahl C, Hansen JW, Westman M, Kolstad A, Pedersen LB, et al. TP53 mutations identify younger mantle cell lymphoma patients who do not benefit from intensive chemoimmunotherapy. Blood (2017) 130:1903–10. doi: 10.1182/BLOOD-2017-04-779736
63. Condoluci A, Rossi D, Zucca E, Cavalli F. Toward a risk-tailored therapeutic policy in mantle cell lymphoma. Curr Oncol Rep (2018) 20. doi: 10.1007/S11912-018-0728-4
64. Jiang M, Bennani NN, Feldman AL. Lymphoma classification update: T-cell lymphomas, Hodgkin lymphomas, and histiocytic/dendritic cell neoplasms. Expert Rev Hematol (2017) 10:239. doi: 10.1080/17474086.2017.1281122
65. Wang H, Bi-bo Fu, Gale RP, Liang Y. NK-/T-cell lymphomas. Leukemia (2021) 35:2460. doi: 10.1038/S41375-021-01313-2
66. Rohr J, Guo S, Huo J, Bouska A, Lachel C, Li Y, et al. Recurrent activating mutations of CD28 in peripheral T-cell lymphomas. Leukemia (2016) 30:1062–70. doi: 10.1038/LEU.2015.357
67. Fujisawa M, Sakata-Yanagimoto M, Nishizawa S, Komori D, Gershon P, Kiryu M, et al. Activation of RHOA–VAV1 signaling in angioimmunoblastic T-cell lymphoma. Leuk (2018) 32:694–702. doi: 10.1038/leu.2017.273
68. Cortés JR, Palomero T. The curious origins of Angioimmunoblastic T-Cell Lymphoma. Curr Opin Hematol (2016) 23:434. doi: 10.1097/MOH.0000000000000261
69. Kataoka K, Iwanaga M, Yasunaga JI, Nagata Y, Kitanaka A, Kameda T, et al. Prognostic relevance of integrated genetic profiling in adult T-cell leukemia/lymphoma. Blood (2018) 131:215–25. doi: 10.1182/BLOOD-2017-01-761874
70. Bonn BR, Huge A, Rohde M, Oschlies I, Klapper W, Voss R, et al. Whole exome sequencing hints at a unique mutational profile of paediatric T-cell lymphoblastic lymphoma. Br J Haematol (2015) 168:308–13. doi: 10.1111/BJH.13105
71. Laurent C, Nicolae A, Laurent C, Le Bras F, Haioun C, Fataccioli V, et al. Gene alterations in epigenetic modifiers and JAK-STAT signaling are frequent in breast implant-associated ALCL. Blood (2020) 135:360–70. doi: 10.1182/BLOOD.2019001904
72. Zhang Y, Li C, Xue W, Zhang M, Li Z. Frequent mutations in natural killer/T cell lymphoma. Cell Physiol Biochem (2018) 49:1–16. doi: 10.1159/000492835
73. Montes-Mojarro IA, Chen BJ, Ramirez-Ibarguen AF, Quezada-Fiallos CM, Pérez-Báez WB, Dueñas D, et al. Mutational profile and EBV strains of extranodal NK/T-cell lymphoma, nasal type in Latin America. Mod Pathol (2020) 33:781–91. doi: 10.1038/S41379-019-0415-5
74. Kataoka K, Miyoshi H, Sakata S, Dobashi A, Couronné L, Kogure Y, et al. Frequent structural variations involving programmed death ligands in Epstein-Barr virus-associated lymphomas. Leukemia (2019) 33:1687–99. doi: 10.1038/S41375-019-0380-5
75. Moffitt AB, Ondrejka SL, McKinney M, Rempel RE, Goodlad JR, Teh CH, et al. Enteropathy-associated T cell lymphoma subtypes are characterized by loss of function of SETD2. J Exp Med (2017) 214:1371–86. doi: 10.1084/JEM.20160894
76. Nairismägi ML, Tan J, Lim JQ, Nagarajan S, Ng CCY, Rajasegaran V, et al. JAK-STAT and G-protein-coupled receptor signaling pathways are frequently altered in epitheliotropic intestinal T-cell lymphoma. Leukemia (2016) 30:1311–9. doi: 10.1038/LEU.2016.13
77. Kamijo H, Miyagaki T. Mycosis fungoides and sézary syndrome: updates and review of current therapy. Curr Treat Options Oncol (2021) 22. doi: 10.1007/S11864-020-00809-W
78. Iżykowska K, Przybylski GK, Gand C, Braun FC, Grabarczyk P, Kuss AW, et al. Genetic rearrangements result in altered gene expression and novel fusion transcripts in Sézary syndrome. Oncotarget (2017) 8:39627–39. doi: 10.18632/ONCOTARGET.17383
79. Hamrouni A, Fogh H, Zak Z, Dum N, Gniadecki R. Clonotypic diversity of the T-cell receptor corroborates the immature precursor origin of cutaneous T-cell lymphoma. Clin Cancer Res (2019) 25:3104–14. doi: 10.1158/1078-0432.CCR-18-4099
80. Koh J, Jang I, Mun S, Lee C, Jeong Cha H, Oh YH, et al. Genetic profiles of subcutaneous panniculitis-like T-cell lymphoma and clinicopathological impact of HAVCR2 mutations. Blood Adv (2021) 5:3919–30. doi: 10.1182/BLOODADVANCES.2021004562
81. Andersson EI, Brück O, Braun T, Mannisto S, Saikko L, Lagström S, et al. STAT3 mutation is associated with STAT3 activation in CD30 + ALK - ALCL. Cancers (Basel) (2020) 12. doi: 10.3390/CANCERS12030702
82. Blombery P, Thompson E, Ryland GL, Joyce R, Byrne DJ, Khoo C, et al. Frequent activating STAT3 mutations and novel recurrent genomic abnormalities detected in breast implant-associated anaplastic large cell lymphoma. Oncotarget (2018) 9:36126. doi: 10.18632/ONCOTARGET.26308
83. Jaffe ES, Ashar BS, Clemens MW, Feldman AL, Gaulard P, Miranda RN, et al. Best practices guideline for the pathologic diagnosis of breast implant-associated anaplastic large-cell lymphoma. J Clin Oncol (2020) 38:1102–11. doi: 10.1200/JCO.19.02778
84. de Leval L, Parrens M, Le Bras F, Jais JP, Fataccioli V, Martin A, et al. Angioimmunoblastic T-cell lymphoma is the most common T-cell lymphoma in two distinct French information data sets. Haematologica (2015) 100:e361–4. doi: 10.3324/HAEMATOL.2015.126300
85. Federico M, Rudiger T, Bellei M, Nathwani BN, Luminari S, Coiffier B, et al. Clinicopathologic characteristics of angioimmunoblastic T-cell lymphoma: analysis of the international peripheral T-cell lymphoma project. J Clin Oncol (2013) 31:240–6. doi: 10.1200/JCO.2011.37.3647
86. De Leval L, Rickman DS, Thielen C, De Reynies A, Huang YL, Delsol G, et al. The gene expression profile of nodal peripheral T-cell lymphoma demonstrates a molecular link between angioimmunoblastic T-cell lymphoma (AITL) and follicular helper T (TFH) cells. Blood (2007) 109:4952–63. doi: 10.1182/BLOOD-2006-10-055145
87. Ondrejka SL, Grzywacz B, Bodo J, Makishima H, Polprasert C, Said JW, et al. Angioimmunoblastic T-cell lymphomas with the RHOA p.Gly17Val mutation have classic clinical and pathologic features. Am J Surg Pathol (2016) 40:335–41. doi: 10.1097/PAS.0000000000000555
88. Nguyen TB, Sakata-Yanagimoto M, Asabe Y, Matsubara D, Kano J, Yoshida K, et al. Identification of cell-type-specific mutations in nodal T-cell lymphomas. Blood Cancer J (2017) 7:e516–6. doi: 10.1038/bcj.2016.122
89. Abdel-Wahab O, Mullally A, Hedvat C, Garcia-Manero G, Patel J, Wadleigh M, et al. Genetic characterization of TET1, TET2, and TET3 alterations in myeloid Malignancies. Blood (2009) 114:144–7. doi: 10.1182/BLOOD-2009-03-210039
90. Palomero T, Couronné L, Khiabanian H, Kim MY, Ambesi-Impiombato A, Perez-Garcia A, et al. Recurrent mutations in epigenetic regulators, RHOAand FYN kinase in peripheral T cell lymphomas. Nat Genet (2014) 46:166. doi: 10.1038/NG.2873
91. Yu DD, Zhang J. Update on recurrent mutations in angioimmunoblastic T-cell lymphoma. Int J Clin Exp Pathol (2021) 14:1108–18.
92. Steensma DP. Clinical consequences of clonal hematopoiesis of indeterminate potential. Blood Adv (2018) 2:3404–10. doi: 10.1182/BLOODADVANCES.2018020222
93. Steensma DP, Ebert BL. Clonal hematopoiesis as a model for premalignant changes during aging. Exp Hematol (2020) 83:48–56. doi: 10.1016/J.EXPHEM.2019.12.001
94. Scourzic L, Couronné L, Pedersen MT, Della Valle V, Diop M, Mylonas E, et al. DNMT3AR882H mutant and Tet2 inactivation cooperate in the deregulation of DNA methylation control to induce lymphoid Malignancies in mice. Leukemia (2016) 30:1388. doi: 10.1038/LEU.2016.29
95. Lemonnier F, Dupuis J, Sujobert P, Tournillhac O, Cheminant M, Sarkozy C, et al. Treatment with 5-azacytidine induces a sustained response in patients with angioimmunoblastic T-cell lymphoma. Blood (2018) 132:2305–9. doi: 10.1182/BLOOD-2018-04-840538
96. Ruan J, Moskowitz AJ, Mehta-Shah N, Sokol L, Chen Z, Rahim R, et al. Multi-center phase II study of oral azacitidine (CC-486) plus CHOP as initial treatment for peripheral T-cell lymphoma (PTCL). Blood (2020) 136:33–4. doi: 10.1182/BLOOD-2020-136023
97. Devata S, Wilcox RA. Cutaneous T-cell lymphoma: A review with a focus on targeted agents. Am J Clin Dermatol (2016) 17:225–37. doi: 10.1007/S40257-016-0177-5
98. Larocca C, Kupper T. Mycosis fungoides and sézary syndrome: an update. Hematol Oncol Clin North Am (2019) 33:103–20. doi: 10.1016/J.HOC.2018.09.001
99. Campbell JJ, Clark RA, Watanabe R, Kupper TS. Sézary syndrome and mycosis fungoides arise from distinct T-cell subsets: a biologic rationale for their distinct clinical behaviors. Blood (2010) 116:767. doi: 10.1182/BLOOD-2009-11-251926
100. Elenitoba-Johnson KSJ, Wilcox R. A new molecular paradigm in mycosis fungoides and Sézary syndrome. Semin Diagn Pathol (2017) 34:15–21. doi: 10.1053/J.SEMDP.2016.11.002
101. McGirt LY, Jia P, Baerenwald DA, Duszynski RJ, Dahlman KB, Zic JA, et al. Whole-genome sequencing reveals oncogenic mutations in mycosis fungoides. Blood (2015) 126:508–19. doi: 10.1182/BLOOD-2014-11-611194
102. Prasad A, Rabionet R, Espinet B, Zapata L, Puiggros A, Melero C, et al. Identification of gene mutations and fusion genes in patients with sézary syndrome. J Invest Dermatol (2016) 136:1490–9. doi: 10.1016/j.jid.2016.03.024
103. Litvinov IV, Netchiporouk E, Cordeiro B, Dore MA, Moreau L, Pehr K, et al. The use of transcriptional profiling to improve personalized diagnosis and management of Cutaneous T-Cell Lymphoma (CTCL). Clin Cancer Res (2015) 21:2820. doi: 10.1158/1078-0432.CCR-14-3322
104. Nebozhyn M, Loboda A, Kari L, Rook AH, Vonderheid EC, Lessin S, et al. Quantitative PCR on 5 genes reliably identifies CTCL patients with 5% to 99% circulating tumor cells with 90% accuracy. Blood (2006) 107:3189. doi: 10.1182/BLOOD-2005-07-2813
105. Michel L, Jean-Louis F, Begue E, Bensussan A, Bagot M. Use of PLS3, Twist, CD158k/KIR3DL2, and NKp46 gene expression combination for reliable Sézary syndrome diagnosis. Blood (2013) 121:1477–8. doi: 10.1182/BLOOD-2012-10-460535
106. Park J, Yang J, Wenzel AT, Ramachandran A, Lee WJ, Daniels JC, et al. Genomic analysis of 220 CTCLs identifies a novel recurrent gain-of-function alteration in RLTPR (p.Q575E). Blood (2017) 130:1430–40. doi: 10.1182/BLOOD-2017-02-768234
107. Alizadeh AA, Aranda V, Bardelli A, Blanpain C, Bock C, Borowski C, et al. Toward understanding and exploiting tumor heterogeneity. Nat Med (2015) 21:846–53. doi: 10.1038/NM.3915
108. Ray SK, Mukherjee S. Cell free DNA as an evolving liquid biopsy biomarker for initial diagnosis and therapeutic nursing in cancer- an evolving aspect in medical biotechnology. Curr Pharm Biotechnol (2022) 23:112–22. doi: 10.2174/1389201021666201211102710
109. Hiemcke-Jiwa LS, Leguit RJ, Snijders TJ, Jiwa NM, Kuiper JJW, de Weger RA, et al. Molecular analysis in liquid biopsies for diagnostics of primary central nervous system lymphoma: Review of literature and future opportunities. Crit Rev Oncol Hematol (2018) 127:56–65. doi: 10.1016/J.CRITREVONC.2018.05.010
110. Sun P, Chen C, Xia Y, Wang Y, Liu PP, Bi XW, et al. Mutation profiling of Malignant lymphoma by next-generation sequencing of circulating cell-free DNA. J Cancer (2019) 10:323–31. doi: 10.7150/JCA.27615
111. Qi F, Cao Z, Chen B, Chai Y, Lin J, Ye J, et al. Liquid biopsy in extranodal NK/T-cell lymphoma: a prospective analysis of cell-free DNA genotyping and monitoring. Blood Adv (2021) 5:2505–14. doi: 10.1182/BLOODADVANCES.2020001637
112. Yao L, Xu H, Wo J, Zhao M, Liu Z, Dong T, et al. Prognostic value of circulating tumor DNA in lymphoma: a meta-analysis. Clin Exp Med (2021) 22:1–7. doi: 10.1007/S10238-021-00718-8
113. Herrera AF, Tracy S, Croft B, Opat S, Ray J, Lovejoy AF, et al. Risk profiling of patients with relapsed/refractory diffuse large B-cell lymphoma by measuring circulating tumor DNA. Blood Adv (2022) 6:1651. doi: 10.1182/BLOODADVANCES.2021006415
114. Melani C, Wilson WH, Roschewski M. Liquid biopsy in non-Hodgkin’s lymphoma. Hematol Oncol (2019) 37 Suppl 1:70–4. doi: 10.1002/HON.2587
115. Kurtz DM, Scherer F, Jin MC, Soo J, Craig AFM, Esfahani MS, et al. Circulating tumor DNA measurements as early outcome predictors in diffuse large B-cell lymphoma. J Clin Oncol (2018) 36:2845. doi: 10.1200/JCO.2018.78.5246
116. Roschewski M, Dunleavy K, Pittaluga S, Moorhead M, Pepin F, Kong K, et al. Circulating tumour DNA and CT monitoring in patients with untreated diffuse large B-cell lymphoma: a correlative biomarker study. Lancet Oncol (2015) 16:541–9. doi: 10.1016/S1470-2045(15)70106-3
117. Zhang S, Zhang T, Liu H, Zhao J, Zhou H, Su X, et al. Tracking the evolution of untreated high-intermediate/high-risk diffuse large B-cell lymphoma by circulating tumour DNA. Br J Haematol (2022) 196:617–28. doi: 10.1111/BJH.17894
118. Arzuaga-Mendez J, Prieto-Fernández E, Lopez-Lopez E, Martin-Guerrero I, García-Ruiz JC, García-Orad A. Cell-free DNA as a biomarker in diffuse large B-cell lymphoma: A systematic review. Crit Rev Oncol Hematol (2019) 139:7–15. doi: 10.1016/J.CRITREVONC.2019.04.013
119. Hossain NM, Dahiya S, Le R, Abramian AM, Kong KA, Muffly LS, et al. Circulating tumor DNA assessment in patients with diffuse large B-cell lymphoma following CAR T-cell therapy. Leuk Lymphoma (2019) 60:503–6. doi: 10.1080/10428194.2018.1474463
120. Tian XP, Zhang YC, Lin NJ, Wang L, Li ZH, Guo HG, et al. Diagnostic performance and prognostic value of circulating tumor DNA methylation marker in extranodal natural killer/T cell lymphoma. Cell Rep Med (2023) 4. doi: 10.1016/J.XCRM.2022.100859
121. Bastos-Oreiro M, Suárez-González J, Andrés-Zayas C, Carrión NC, Moreno S, Carbonell D, et al. Incorporation of next-generation sequencing in clinical practice using solid and liquid biopsy for patients with non-Hodgkin’s lymphoma. Sci Rep (2021) 11:1–20. doi: 10.1038/s41598-021-02362-4
122. Wilson WH, Young RM, Schmitz R, Yang Y, Pittaluga S, Wright G, et al. Targeting B cell receptor signaling with ibrutinib in diffuse large B cell lymphoma. Nat Med (2015) 21(8):922–6. doi: 10.1038/nm.3884
Keywords: lymphomas, non-Hodgkin lymphomas, genomics, diagnosis, next-generation sequencing
Citation: Tomacinschii V, Mosquera Orgueira A, Santos CA, Robu M, Buruiana S and Fraga Rodriguez MF (2023) The implication of next-generation sequencing in the diagnosis and clinical management of non-Hodgkin lymphomas. Front. Oncol. 13:1275327. doi: 10.3389/fonc.2023.1275327
Received: 09 August 2023; Accepted: 27 October 2023;
Published: 08 November 2023.
Edited by:
Sophia Yohe, University of Minnesota Twin Cities, United StatesReviewed by:
Zhiming Li, Sun Yat-sen University Cancer Center (SYSUCC), ChinaXianhuo Wang, Tianjin Medical University Cancer Institute and Hospital, China
Copyright © 2023 Tomacinschii, Mosquera Orgueira, Santos, Robu, Buruiana and Fraga Rodriguez. This is an open-access article distributed under the terms of the Creative Commons Attribution License (CC BY). The use, distribution or reproduction in other forums is permitted, provided the original author(s) and the copyright owner(s) are credited and that the original publication in this journal is cited, in accordance with accepted academic practice. No use, distribution or reproduction is permitted which does not comply with these terms.
*Correspondence: Victor Tomacinschii, dmljdG9yLnRvbWFjaW5zY2hpaUB1c21mLm1k; Adrian Mosquera Orgueira, QWRyaWFuLk1vc3F1ZXJhLk9yZ2VpcmFAc2VyZ2FzLmVz