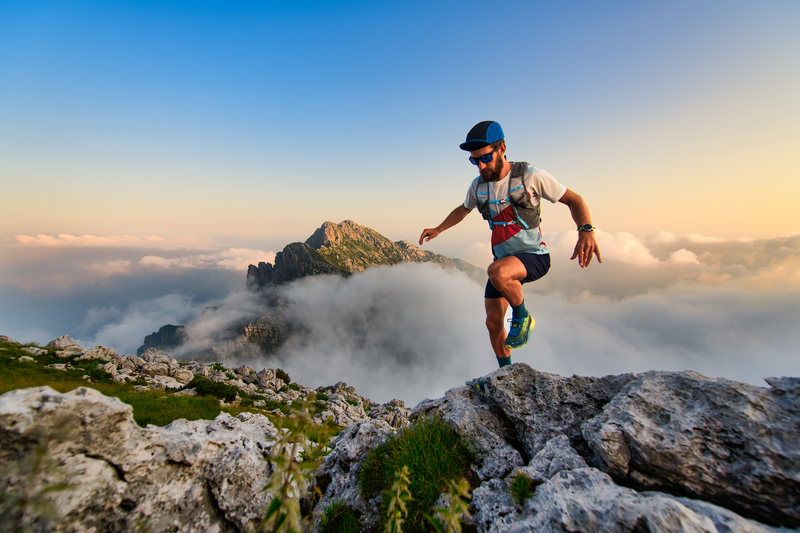
95% of researchers rate our articles as excellent or good
Learn more about the work of our research integrity team to safeguard the quality of each article we publish.
Find out more
REVIEW article
Front. Oncol. , 06 December 2023
Sec. Gastrointestinal Cancers: Gastric and Esophageal Cancers
Volume 13 - 2023 | https://doi.org/10.3389/fonc.2023.1239788
With an increasing number of patients with gastrointestinal cancer, effective and accurate early diagnostic clinical tools are required provide better health care for patients with gastrointestinal cancer. Recent studies have shown that artificial intelligence (AI) plays an important role in the diagnosis and treatment of patients with gastrointestinal tumors, which not only improves the efficiency of early tumor screening, but also significantly improves the survival rate of patients after treatment. With the aid of efficient learning and judgment abilities of AI, endoscopists can improve the accuracy of diagnosis and treatment through endoscopy and avoid incorrect descriptions or judgments of gastrointestinal lesions. The present article provides an overview of the application status of various artificial intelligence in gastric and colorectal cancers in recent years, and the direction of future research and clinical practice is clarified from a clinical perspective to provide a comprehensive theoretical basis for AI as a promising diagnostic and therapeutic tool for gastrointestinal cancer
Recently, artificial intelligence(AI) technology has been successfully adopted in health care diagnostics, which is the branch of computer science (1). It is used to attempt to learn and solve problems by emulating human-like mind and cognition (2).Machine learning (ML) and deep learning(DL) can be considered subsets of AI. ML-based approaches refer to the scientific studies of algorithms and statistical models that can perform complex tasks after manually extracting features (3). The algorithm can learn independently using multiple datasets without explicit instructions, which is at the forefront of AI and data science (4). In this statistical method of fitting models to data, the models are trained and learned using databases to make predictions based on new data (5). DL is a particular ML approach that developed through the advancement of artificial neural networks (ANN) and specialized in deep neural networks. A classification and recognition system for focal images can be constructed without complex image-processing algorithm (6).The algorithm can also learn and utilize interaction factors between data inputs to predict its target (4). Convolutional neural network (CNN) is the main DL algorithm used for image recognition and image processing (7, 8). After identifying and extracting specific features from the original images, the CNN uses mathematical convolution operations to perform endoscopic diagnosis, which has been reported as a successful image classification computing system (9, 10).(Figure 1).
In recent years, the image recognition ability of AI has become increasingly advanced (7), and DL has been widely applied in diagnostic imaging in various medical fields (11–13). Compared to traditional ANN, the DL algorithm significantly enhances the width and depth of the network. It consists of digitized inputs that can extract information from shallow, intermediate, and deep layers of images, and output layer, which is used for classification and processing lesion images at the backend (2, 14). Deep learning architecture has high detection, classification and segmentation capabilities. Therefore, it is particularly suitable for image quantization. With adequate learning, clinicians can achieve high accuracy and rapidity of AI when assessing gastrointestinal tumors, thereby improving clinical efficiency and reducing costs for patients and clinical teams (15).
Gastric cancer (GC) remains an important cancer worldwide and was responsible for over one million new cases in 2020 and an estimated 769,000 deaths, ranking fifth in incidence and fourth in mortality globally of all cancers (16). Statistically, the relative 5-year survival rate of patients with GC is <40% (17, 18), which is attributed to the late onset of symptoms and delayed diagnosis (19). Early gastric cancer (EGC) has a high endoscopic cure rate and the 5-year survival rate exceeds 90% (20, 21). Therefore, timely and accurate diagnosis of EGC through endoscopy is a key strategy for improving survival rates. The AI-based diagnosis system has high diagnostic accuracy, which can monitor and distinguish cancer from non-neoplastic lesions in a timely manner and predict the invasion depth through gastroscopy images. The application of AI in endoscopic gastric cancer is shown in Table 1.
EGC are often present in the background of gastric mucosal inflammation and are difficult to identify by endoscopists. The use of a CNN-based AI to detect GC in endoscopic images was first reported by Hirasawa et al (22).The model required a significantly shorter time for diagnosis than endoscopists and correctly diagnosed 71 of 77 gastric cancer lesions with an overall sensitivity of 92.2%, resulting in a positive predictive value(PPV) of 30.6%. Wu et al. (23)constructed a system using DCNN to detect EGC without blind spots. After validating the 200 endoscopic images, the sensitivity and specificity were comparable, and the accuracy was significantly higher. They also used it on unprocessed videos to proactively track suspicious cancerous lesions without blind spots. Luo et al. (24) developed and validated GRAIDS using a large cohort of more than one million images from different tiers of hospitals, with a diagnostic accuracy of 91.5% to 97.7%. GRAIDS can support non-expert endoscopists to a level similar to that of experts, suggesting the effectiveness of combining of AI and endoscopists. At that time, it was the largest study in the field of AI-guided cancer detection based on upper gastrointestinal endoscopic images worldwide.
Image-enhanced endoscopy (IEE) uses narrow-band spectrum or blue laser imaging to enhance micro-vessels patterns as well as color differences of gastric mucosa and structural features to improve diagnostic accuracy (37). Magnifying-IEE(M-IEE) has satisfies diagnostic ability for GC, however, its high cost of equipment and strict requirements for endoscopists limit its popularity (38).Weak magnifying-IEE(WM-IEE) has wide utility and relatively lower cost than M-IEE, providing a significant option for diagnosis of high-risk lesions (39). Since EGC shows only subtle mucosal changes, narrow-band imaging (NBI) has been reported to be a powerful tool for characterizing gastric mucosal lesions because it can use narrow light source to enhance visualization of the surface micro-vessels (40). In particular, magnifying NBI(ME-NBI) is a powerful optical technology with accurate real-time diagnostic performance in EGC. The application of CNN in ME-NBI diagnosis is a potential solution to improve the optical diagnosis (41–43). Li et al. (25) established a CNN model on 2088 images for analyzing gastric mucosal lesions observed by ME-NBI and achieved an accuracy of 90.91% in 341 still images. The diagnostic sensitivity of CNN was significantly higher than that of the experts. Similarly, Horiuchi et al. (26) verified the performance of AI for ME-NBI using 174 videos to enable real-time diagnosis of EGC and the system demonstrated an area under the curve (AUC) of 0.8684.The diagnostic performance was equivalent to or better than that of 11 endoscopic experts. A CNN computer-aided system was constructed by Ueyama et al. (27)based on ME-NBI images and achieved a high diagnostic accuracy of 98.7% among homogeneous systems and AUC of 99%. The most important difference was that the images processed by water immersion technique with maximal magnification in this study were optimal for AI-assisted diagnostics.
As the first study to evaluate AI using a multicenter validation cohort, Hu et al. (28) trained and tested a model using 1777 ME-NBI images from the database and achieved accuracies of 77% and 76% in the internal and external test cohorts, respectively. It can not only effectively improve the diagnostic performance of endoscopists of different levels, but also delineate lesion boundaries. He et al. (38) proposed a system to diagnose EGC by M-IEE and validated its effectiveness using multicenter static images from six hospitals, real-time videos, and a prospective clinical trial. This showed the great potential for the diagnosis of EGC in clinical practice. A deep CNN(DCNN) converts one level of representation into a more abstract level for prediction (7). A real-time DCNN system was developed to diagnose EGC using 21785 NBI images and 20 videos, with the largest sample size at the time (29). It showed a generalized diagnostic performance with an AUC of 0.947 on the internal validation dataset and 0.888–0.951 on the four external validation datasets. Notably, the system significantly enhanced the performance of senior (89.4%, 95% CI, 87.9–90.7%) and junior (84.9%, 95% CI, 83.4–86.3%) endoscopists. These experimental studies have promoted the development of AI technology, which has the potential for future clinical applications.
Randomized controlled studies of target population with appropriate inclusion and exclusion criteria are necessary to validate the diagnostic accuracy of AI. In addition to EGC, it is necessary to ensure the diagnosis of hard-to-detect GC, such as early undifferentiated cancers and gastritis-like cancers (44). Furthermore, most studies used DL to identify GC with WL or M-IEE, whereas few concentrated on WM-IEE (45, 46). In clinical practice, guidelines recommend the use of multimodal light sources with chromoendoscopy and white-light imaging (WLI) endoscopy, instead of a single light source (47), which underscores the importance of accurate diagnosis and risk stratification in these patients.
It is sometimes difficult to distinguish benign lesions from EGC, and the PPV of biopsy using conventional endoscopy with WLI is only 3.2-5.6%. Horiuchi et al. (30) applied a CNN system to differentiate EGC from gastritis with 151 EGC and 107 gastritis images based on ME-NBI. The accuracy, sensitivity, and specificity of the system were 85.3%, 95.4%, and 71.0%, respectively. To evaluate the applicability for the classification of GC and gastric ulcer, Namikawa et al. (31) developed an AI-based system by adding 4453 gastric ulcer images to the original AI. The overall accuracies of the advanced and original AI were 95.9% and 45.9%, respectively, indicating a high level of recognition and classification. A CNN model based on ultrasound endoscopic (EUS) images distinguished gastrointestinal stromal tumors (GIST) from non-GISTs with 83.0% sensitivity, 75.5% specificity, and 79.2% accuracy (32). Therefore, it complemented the clinical practice of EUS in the diagnosis of gastric mesenchymal tumors. The application of AI to differentiate cancer from non-cancerous changes could potentially reduce the number of unnecessary biopsies.
EGC refers to GC confined to the mucosa or submucosa, regardless of the presence of lymph node metastasis (48) and is classified into intramucosal cancer (T1a) and submucosal invasive cancer (T1b). Endoscopic resection has become the treatment of choice for EGC because of minimally invasive and superior cost-effectiveness (49–51). One of the most important preoperative criteria for curative endoscopic resection is tumor invasion depth. Absolute indication for endoscopic surgery is a differentiated-type adenocarcinoma without ulcerative findings (UL0), in which the invasion depth is clinically diagnosed as T1a and the diameter is ≤ 2 cm (49, 52, 53). As undifferentiated-type intramucosal adenocarcinoma of diameter < 2cm is also an absolute indication for endoscopic submucosal dissection (ESD) (54), accurate prediction of infiltration depth based on endoscopy images is a key to screen patients for endoscopic resection.
Conventional endoscopy is an effective method for T staging of EGC (55, 56), and EUS can distinguish between the different layers of the stomach wall and reveal the peri-gastric lymph nodes (57). However, influenced by endoscopists and images, EUS has no substantial effect on pretreatment T-staging of EGC patients (58, 59). Therefore, with increasing interest in the field of medical imaging, there is a requirement for a more detailed classification and higher accuracy AI system.The first investigation of the depth of GC invasion depth using a computer-aided system based on 902 images with 10-fold cross-validation method (60). The diagnostic accuracies were 77.2%, 49.1%, 51.0% and 55.3% for T1, T2, T3, and T4 stages, respectively, and the accuracy was 68.9% and 63.6% for T1a and T1b stages of EGC, respectively. Recent years, Zhu et al. (33) reported that their CNN system developed with 790 images could differentiate the depth of M, SM1, and SM2 from all GCs, with an accuracy of 89.16% and specificity of 95.56%. In a study simultaneously detected GC and invasion depth with AI, Yoon et al. (34) reported the sensitivity and specificity of tumor depth as 79.2% and 77.8%, respectively. They also analyzed factors that influence AI diagnosis, such as whether undifferentiated-type histology is correlated with low T-stage prediction accuracy. Using 16577 selected endoscopic images from different angles and distances for each lesion, the system developed by Nagao et al. (35) could diagnose invasion depth with an accuracy of 94.4%. Notably, the impact of WLI, NBI and Indigo on the ability to predict invasion depth was compared for the first time.
Zhu and Nagao reported that it is easier to diagnose the depth of invasion in advanced gastric cancer in clinical practice. Therefore, developing systems to improve the diagnostic ability for EGC would be more beneficial. Goto et al. (36) constructed an AI classifier for differentiating intramucosal and submucosal GC and devised a diagnostic method based on cooperation between AI and endoscopists. A total of test images showed that the accuracy, specificity, and F1 measure based on cooperation were 78.0%, 80.0%, and 0.776, respectively, and that the accuracy of using F1 measure was higher than that of using AI or endoscopists alone.
Colorectal cancer (CRC) is the third most frequently diagnosed cancer and the second most common cause of cancer-related deaths. More than 1.9 million new CRC cases and 935,000 deaths were estimated in 2020, accounting for approximately one in ten cancer cases and deaths (16). Colonoscopy plays an important role in screening and preventing CRC (61, 62). Adenomatous polyps are the most important precursor lesions and CRC usually develops from sporadic mutation-accumulating adenomatous polyps in a relatively predictable stepwise sequence (63). Colonoscopy can be used to detect and remove these lesions via polypectomy, thereby significantly reducing the incidence and mortality risk of CRC (64, 65). Evidence suggests that colonoscopy can reduce the risk of death from CRC by 67% (66) and the incidence of late-stage CRC by 70% (67).
Adenoma detection rate (ADR) is defined as the proportion of at least one histologically identified colorectal adenoma or adenocarcinoma when performing colonoscopy (68). CRCs detected after a prior colonoscopy or during the interval between surveillance colonoscopies are known as interval CRC or post-colonoscopy CRC (PCCRC). ADR is a proxy for colonoscopy quality indicator and has been inversely correlated with the risk of PCCRC (69–73). The incidence of PCCRC is estimated to be as high as 3.5 per 1,000 screened person (74). Each 1.0% increase in ADR correlated with a 3.0% decrease in the risk of PCCRC (69) and a 5% decrease in the risk of fatal interval CRC (75). It is also reported that 58% of PCCRC could be categorized as “possible missed lesion, prior examination inadequate,” which emphasized the importance of careful colonoscopy examination (76). In fact, the adenoma missing rate (AMR) of WLI colonoscopy ranges from 6% to 41% (77–79), depending on various polyps and surgical characteristics. For example, smaller polyps, flat polyps (77, 80), and left colonic location (79) may be associated with an increased AMR. The ability to examine the colorectal mucosa to the maximum extent possible and accurately identify neoplastic lesions depend mainly on the mastery of technical and cognitive skills (81). A potential solution to mitigate the variability in both endoscopic detection and histological prediction is to apply computerized image analysis to deliver computer decision-support solutions.
Moreover, accurate in vivo differentiation can reduce unnecessary endoscopic resections, complications, physician burden, and medical costs (82). Studies using full-spectrum colonoscopy (FUSE), which provides a 330° angle of view, showed an AMR between 7.0% (83) and 20.5% (78). AI can compensate for differences in endoscopists’ diagnostic ability due to limitations in experience, visual perception, and other human factors (84). Several computer-aided diagnostic systems have been developed and applied clinically to evaluate the benefits of improving ADR (85). The data suggest that application of CNN may lead to “resect and discard” and “detect and leave” strategies in real time, which will avoid unnecessary non-neoplastic polyp removal and improve colonoscopy efficiency and cost-effectiveness. The two significant roles of AI in CRC screening are computer-aided detection (CADe) and computer-aided diagnosis or differentiation (CADx). Using complex algorithms or CNN, CADe is used to detect lesions, whereas CADx characterizes lesions by performing optical biopsies, reducing the need for histopathological evaluation to some extent (86). Therefore, CADe can help endoscopists reduce missed polyps and augment performance, whereas CADx can interpret polyp histology more accurately (87). The application of AI in endoscopic colorectal cancer is shown in Table 2.
CADe systems have been developed to increase ADR and adenomas by providing real-time visual information on previously unrecognized polyps (91). Being a standardized second observer, the system can help avoid any missed diagnoses of visible lesions that briefly appear in the field of vision (106) and has been proven to increase polyp detection with high accuracy and consistency (107–109).
Misawa et al. (88) developed an original CNN-based CADe using 411 colonoscopy videos with a sensitivity and specificity of 90% and 63%, respectively, for 50 polyp and 85 non-polyp videos. This compensates for the shortcomings of static images and insufficient samples in previous systems. A subsequent study reported the first real-time application of CNN-based CADe to identify and locate polyps by Urban et al. (89), which showed 96% cross-validation accuracy and an AUC of 0.991 on 8641 images. Notably, four expert reviewers identified 17 additional polyps with CNN compared with eight additional polyps without assistance. Another real-time CAD system CAD EYE trained with linked color imaging technology focused on sessile serrated lesions and achieved the detection rate of 100% (90). The first prospective, randomized controlled trial was conducted by Wang et al. (91) to investigate its effect on ADR. The CADe system significantly increased the mean number of adenomas per patient (0.53 vs. 0.31, p<0.001) and ADR (29.1% vs. 20.3%, p<0.001) than standard colonoscopy, which was mainly attributed to the detection of a greater number of small polyps. In another prospective randomized controlled trial, Su et al. (92) designed a CADe that was able to not only detect colorectal polyps but also measure withdrawal time to improve the performance of endoscopists. The CAD-assisted group had a significantly higher ADR (29% vs. 17%, p < 0.001), a prolonged exposure time (7.03 minutes vs 5.68 minutes, P <0.001), and adequate bowel preparation(87.34% vs 80.63%, P =0.023).Yamada et al. (93) developed a system using a supervised DNN and validated it using a dataset of 705 still images of 752 lesions and 4135 still images of noncancerous tissue. The system achieved sensitivity and specificity of 97.3% and 99.0%, respectively, and speed of 21.9 ms/image on average. To overcome the operational bias in previous non-blinded trial, Wang et al. (94) used a double-blind design to evaluate the effectiveness of the system. The ADR was significantly greater in the CADe group than in the sham group, with 165 of 484 patients(34%) versus 132 of 478 patients(28%) having an adenoma detected.
Repici et al. (95) performed a multicenter, randomized trial to evaluate the safety and efficacy of CADe, known as GI Genius from Medtronic. With 685 subjects randomly assigned (1:1), the ADR was higher in the CADe group (54.8%) than in the control group (40.4%) without increasing withdrawal time. In a later study, a random trial was performed with colonoscopists (96). When the data from the above two studies were merged, the application of CADe and colonoscopy indication were correlated with the ADR, and experience seemed to play a secondary role. Because the AMR obtained from tandem colonoscopy is a better indicator than the ADR of how endoscopists performed, Wang et al. (97) compared the specific AMR of CADe colonoscopy with that of routine white-light colonoscopy. The overall AMR was lower with CADe colonoscopy (13.89% vs. 40.00%, P<0.0001), which mean that routine CADe may reduce the incidence of interval CRC. A prospective study conducted by Ishiyama et al. including 1836 patients (98) showed the ADR was higher in the CADe group than in the control group (26.4% vs. 19.9%, OR, 1.32; 95%CI, 1.12–1.57), but there was no significant increase in the advanced neoplasia detection rate (3.7% vs. 2.9%). Another multicenter prospective study firstly took user friendliness into account and presented data on the safety and user experience of Discovery system from Pentax (99). In order to yield better representations of polyp lesions, CADDIE system constructed with novel hybrid 2D/3D network can realize training with smaller static images (110). After testing on 95 videos and 1833 polyp images, it achieved an improvement across all performance metrics included temporal consistency, showing better generalization performance and increased suitability for clinical application.
With continuous improvement of the quality of endoscopic imaging systems, optical diagnosis is increasingly applied to the histological prediction of colorectal polyps. However, tissue biopsy remains the gold standard. The accuracy of AI for optical biopsy depends on the extent to which the surface structure reflects the histological characteristics of the lesion (91). CADx can analyze endoscopic images to make a qualitative diagnosis of colorectal tumors with low inter-observer variation. Generally, CAD for colonoscopy is designed to extract features from colonoscopy images or videos and the output includes predicted polyp location or pathology (111). The optical prediction of polyp histology helps guide subsequent treatment and is the key to the “resect and discard” and “detect and leave” strategy (112, 113). Previous studies have demonstrated that en bloc R0 ER of selected colorectal neoplasms confined to the mucosa or superficial submucosa (T1a, with <1000 μm of submucosal invasion and favorable histological features) may be considered a curative resection (114, 115). Therefore, the estimation of the invasion depth is of utmost importance for establishing treatment strategies for colorectal neoplasms (116). The diagnostic capability of the CAD system was evaluated by observing between invasive and less invasive lesions.
Kominami et al. (117) evaluated whether the real-time image recognition system could predict the histological diagnosis of colorectal lesions depicted on NBI. The concordance between the endoscopic diagnosis and CADx output was 97.5%(115/118). Owing to the need for the manual design of imaging features in support vector classifiers, subsequent models are mostly based on AI, especially DL. Similarly, Chen et al. (100) used a CAD model with deep neural network to predict the histopathology of 284 diminutive polyps diagnosed with NBI and achieved an NPV of 91.5% for adenomas. Byrne et al. (101) further demonstrated that the AI model based on DCNN could be used to classify diminutive colorectal polyps. The model achieved 94% accuracy, 98% sensitivity, 97% NPV, and 90% PPV for 106 diminutive polyps. The use of video images can effectively reduce selection bias and simplifies the steps of the clinical work. Kudo et al. (102) performed a retrospective comparative analysis to determine the diagnostic performance of EndoBRAIN, which can identify colon neoplasms by analyzing their microstructures. When the pathological results were used as the standard, the ability of NBI to distinguish neoplastic lesions was significantly higher than or comparable to that of the 30 endoscopists. If high-quality images are available, the system will be a powerful tool for endoscopists with quick response and reproducibility.
To distinguish between invasive cancer and adenomas, Takeda et al. (118) evaluated endoscopic CAD for the diagnosis of invasive CRC. They trained on 5843 images and tested on 200 images with specificity of 98.9% and accuracy of 94.1%. Another CAD system based on ME-NBI was further applied to classify hyperplastic polyps, adenoma/adenocarcinoma lesions, and deep sub-mucosal lesions (119). A single-center, large-scale prospective study (103) showed that endocytoscopy with CADx had an NPV for diminutive rectosigmoid adenomas of 93.7%–96.4% with the stained mode and 95.2%–96.5% with NBI. Considering the missing data, their model met the threshold of 90% recommended by ASGE PIVI. Instead of endoscopy, Zacharia et al. (104) created a CNN-based DL algorithm for real-time in situ diagnosis of colorectal polyps. With 5-fold cross validation, the NPV was 97% for diminutive polyps and the surveillance interval concordance achieved 94%. A novel CADx model capable of delineating polyp boundaries and providing localized histological predictions has been presented (105). The histology map increased the transparency and interpretability of the results, and the model was tested on 254 polyps with sensitivity, specificity and NPV of 96%, 84%, and 91%, respectively.
Given the high risk of CRC, to develop a real-time automated polyp detection system can significantly reduce missed diagnosis rates and guide management decisions regarding polyps (120). An ideal CAD system would support the simultaneous detection and classification of polyps to achieve optimal CRC prevention and treatment. In a previous study that evaluated real-time CADx with CADe, the model failed to generate sufficient confidence to predict 15% of the polyps in 125 videos (101). For the remaining 106 polyps, the model achieved an accuracy of 94%, sensitivity of 98%, NPV of 97%, and PPV of 90%. On the one hand, computer analysis of video may reduce differences among endoscopic observers and lead to widespread acceptance of “resect and discard.” On the other hand, AI systems are regarded as low-risk devices that can assist but not replace the work of endoscopists, making it impossible to guarantee the added value of AI in clinical practice (121).
In recent years, artificial intelligence (AI) has made remarkable progress in medical image recognition and has shown promise in the diagnosis and treatment of gastrointestinal tumors. Medical and engineering institutions are actively conducting a great deal of researches. However, it is critical to overcome the following limitations before it become part of the clinical workflow.
Firstly, most current studies rely on retrospective datasets, especially validation sets, which may be affected by selection bias. On the one hand, due to selection bias, the results obtained in retrospective studies are often better than those obtained in clinical practice. However, since low-quality endoscopic images are often excluded from retrospective studies, they cannot usually determine how to manage low-quality images in clinical practice. To overcome this limitation, multi-center prospective studies, which are necessary for clinical validation, should be prioritized. Secondly, false-positive or false-negative results can be found in some models. The main reason may be the limited quantity and quality of learning materials, which limit the clinical applicability. Therefore, further accumulation of various endoscopic images could reduce these inaccurate results. Video images can be used as learning materials (88, 101)to realize the real-time diagnosis of lesions, and the number and type of images can be further increased. Thirdly, the results lack external validity. Due to differences in genetics, diet, and lifestyle between Chinese and Western populations, the results from one region may not be generalizable to parts of the world with different incidence rates, so adaptability and effectiveness in other areas need to be further explored. In this regard, multicenter studies have been widely conducted in other areas of medicine to evaluate DL systems. Similarly, specific colonoscopy devices were used in most studies, and the adaptability of the model to equipment manufactured by other companies should be further explored to ensure the same performance level. Finally, to date, there is no effective method to verify the status of tumor resection; therefore, future efforts may facilitate AI utilization to distinguish between normal mucosa, adenoma, and submucosal tissue, enabling endoscopists to evaluate resection status (122).
This study reviewed the research and development of AI for gastrointestinal tumors endoscopy. Owing to the insidious clinical symptoms of early gastrointestinal tumors and large variations among endoscopists, the use of AI for lesion detection is not influenced by factors known to influence the size and shape of human observers. AI-assisted systems have evolved from traditional ML algorithms to DL based on neural networks, from still image analysis to real-time video processing. More importantly, it can promote the development of telemedicine since the system is automated and online. With the processing power and high performance of algorithms such as DL, the use of a new era of AI-based assisted endoscopy systems can help endoscopists perform basic tasks such as the early detection and classification of gastrointestinal tumors, and more development and validation is undergoing. Most previous diagnosis process are difficult to be understood by humans, known as “black box”, so it is very necessary to further explore the construction of AI endoscopic systems with man–machine interaction capability. In the training process, a sufficient number of training datasets is essential, and in-depth analysis of as many variables as possible should be carried out. In the validation process, the risk of overtreatment should be taken into account if the specificity is reduced. The selection of included variables may be inappropriate when the sensitivity is reduced. The objectivity and reproducibility of AI technology will enable its further application in the treatment of gastrointestinal tumors, including early detection, pathological identification, risk assessment, treatment guidance, and outcome prediction. AI will likely be introduced into the composition of endoscopic equipment for diagnosis and treatment to improve clinical outcomes. More and more patients and physicians will benefit from the progress of endoscopic AI-assisted systems.
YX, XLiu, and BL reviewed literature and originally drafted the manuscript. QZ and TM contributed to editing and embellished the manuscript. XLi approved the final version of the manuscript. All authors contributed to the article and approved the submitted version.
The author(s) declare financial support was received for the research, authorship, and/or publication of this article. The study was supported by the National Natural Science Foundation of China (Grant No. 82270676) and 2023 Qingdao Technology benefiting Demonstration Project(Grant No.23-2-8-smjk-8-nsh).
The authors declare that the research was conducted in the absence of any commercial or financial relationships that could be construed as a potential conflict of interest.
All claims expressed in this article are solely those of the authors and do not necessarily represent those of their affiliated organizations, or those of the publisher, the editors and the reviewers. Any product that may be evaluated in this article, or claim that may be made by its manufacturer, is not guaranteed or endorsed by the publisher.
1. Killock D. AI outperforms radiologists in mammographic screening. Nat Rev Clin Oncol (2020) 17(3):134. doi: 10.1038/s41571-020-0329-7
2. Topol EJ. High-performance medicine: the convergence of human and artificial intelligence. Nat Med (2019) 25(1):44–56. doi: 10.1038/s41591-018-0300-7
3. Jordan MI, Mitchell TM. Machine learning: Trends, perspectives, and prospects. Science (2015) 349(6245):255–60. doi: 10.1126/science.aaa8415
4. Ruffle JK, Farmer AD, Aziz Q. Artificial intelligence-assisted gastroenterology- promises and pitfalls. Am J Gastroenterol (2019) 114(3):422–8. doi: 10.1038/s41395-018-0268-4
5. Davenport T, Kalakota R. The potential for artificial intelligence in healthcare. Future Healthc J (2019) 6(2):94–8. doi: 10.7861/futurehosp.6-2-94
6. Litjens G, Kooi T, Bejnordi BE, Setio AAA, Ciompi F, Ghafoorian M, et al. A survey on deep learning in medical image analysis. Med Image Anal (2017) 42:60–88. doi: 10.1016/j.media.2017.07.005
7. LeCun Y, Bengio Y, Hinton G. Deep learning. Nature (2015) 521(7553):436–44. doi: 10.1038/nature14539
8. Shin HC, Roth HR, Gao M, Lu L, Xu Z, Nogues I, et al. Deep convolutional neural networks for computer-aided detection: CNN architectures, dataset characteristics and transfer learning. IEEE Trans Med Imaging (2016) 35(5):1285–98. doi: 10.1109/TMI.2016.2528162
9. Hsiao Y-J, Wen Y-C, Lai W-Y, Lin Y-Y, Yang Y-P, Chien Y, et al. Application of artificial intelligence-driven endoscopic screening and diagnosis of gastric cancer. World J Gastroenterol (2021) 27(22):2979–93. doi: 10.3748/wjg.v27.i22.2979
10. Bera K, Schalper KA, Rimm DL, Velcheti V, Madabhushi A, Bejnordi Ehteshami B, et al. Artificial intelligence in digital pathology - new tools for diagnosis and precision oncology. Nat Rev Clin Oncol (2019) 16(11):703–15. doi: 10.1038/s41571-019-0252-y
11. Ehteshami Bejnordi B, Veta M, Johannes van Diest P, van Ginneken B, Karssemeijer N, Litjens G, et al. Diagnostic assessment of deep learning algorithms for detection of lymph node metastases in women with breast cancer. Jama (2017) 318(22):2199–210. doi: 10.1001/jama.2017.14585
12. Esteva A, Kuprel B, Novoa RA, Ko J, Swetter SM, Blau HM, et al. Dermatologist-level classification of skin cancer with deep neural networks. Nature (2017) 542(7639):115–8. doi: 10.1038/nature21056
13. Gulshan V, Peng L, Coram M, Stumpe MC, Wu D, Narayanaswamy A, et al. Development and validation of a deep learning algorithm for detection of diabetic retinopathy in retinal fundus photographs. Jama (2016) 316(22):2402–10. doi: 10.1001/jama.2016.17216
14. Wang L, Song H, Wang M, Wang H, Ge R, Shen Y, et al. Utilization of ultrasonic image characteristics combined with endoscopic detection on the basis of artificial intelligence algorithm in diagnosis of early upper gastrointestinal cancer. J Healthc Eng 2021 (2021) p:2773022. doi: 10.1155/2021/2773022
15. Matheny ME, Whicher D, Thadaney Israni S. Artificial intelligence in health care: A report from the national academy of medicine. Jama (2020) 323(6):509–10. doi: 10.1001/jama.2019.21579
16. Sung H, Ferlay J, Siegel RL, Laversanne M, Soerjomataram I, Jemal A, et al. Global cancer statistics 2020: GLOBOCAN estimates of incidence and mortality worldwide for 36 cancers in 185 countries. CA Cancer J Clin (2021) 71(3):209–49. doi: 10.3322/caac.21660
17. Dicken BJ, Bigam DL, Cass C, Mackey JR, Joy AA, Hamilton SM, et al. Gastric adenocarcinoma: review and considerations for future directions. Ann Surg (2005) 241(1):27–39. doi: 10.1097/01.sla.0000149300.28588.23
18. Rawla P, Barsouk A. Epidemiology of gastric cancer: global trends, risk factors and prevention. Prz Gastroenterol (2019) 14(1):26–38. doi: 10.5114/pg.2018.80001
19. Smyth EC, Nilsson M, Grabsch HI, Ct van Grieken N, Lordick F. Gastric cancer. Lancet (2020) 396(10251):635–48. doi: 10.1016/S0140-6736(20)31288-5
20. Katai H, Ishikawa T, Akazawa K, Isobe Y, Miyashiro I, Oda I, et al. Five-year survival analysis of surgically resected gastric cancer cases in Japan: a retrospective analysis of more than 100,000 patients from the nationwide registry of the Japanese Gastric Cancer Association (2001-2007). Gastric Cancer (2018) 21(1):144–54. doi: 10.1007/s10120-017-0716-7
21. Sano T, Coit DG, Kim HH, Roviello F, Kassab P, Wittekind C, et al. Proposal of a new stage grouping of gastric cancer for TNM classification: International Gastric Cancer Association staging project. Gastric Cancer (2017) 20(2):217–25. doi: 10.1007/s10120-016-0601-9
22. Hirasawa T, Aoyama K, Tanimoto T, Ishihara S, Shichijo S, Ozawa T, et al. Application of artificial intelligence using a convolutional neural network for detecting gastric cancer in endoscopic images. Gastric Cancer (2018) 21(4):653–60. doi: 10.1007/s10120-018-0793-2
23. Wu L, Zhou W, Wan X, Zhang J, Shen L, Hu S, et al. A deep neural network improves endoscopic detection of early gastric cancer without blind spots. Endoscopy (2019) 51(6):522–31. doi: 10.1055/a-0855-3532
24. Luo H, Xu G, Li C, He L, Luo L, Wang Z, et al. Real-time artificial intelligence for detection of upper gastrointestinal cancer by endoscopy: a multicentre, case-control, diagnostic study. Lancet Oncol (2019) 20(12):1645–54. doi: 10.1016/S1470-2045(19)30637-0
25. Li L, Chen Y, Shen Z, Zhang X, Sang J, Ding Y, et al. Convolutional neural network for the diagnosis of early gastric cancer based on magnifying narrow band imaging. Gastric Cancer (2020) 23(1):126–32. doi: 10.1007/s10120-019-00992-2
26. Horiuchi Y, Hirasawa T, Ishizuka N, Tokai Y, Namikawa K, Yoshimizu S, et al. Performance of a computer-aided diagnosis system in diagnosing early gastric cancer using magnifying endoscopy videos with narrow-band imaging (with videos). Gastrointest Endosc (2020) 92(4):856–865.e1. doi: 10.1016/j.gie.2020.04.079
27. Ueyama H, Kato Y, Akazawa Y, Yatagai N, Komori H, Takeda T, et al. Application of artificial intelligence using a convolutional neural network for diagnosis of early gastric cancer based on magnifying endoscopy with narrow-band imaging. J Gastroenterol Hepatol (2021) 36(2):482–9. doi: 10.1111/jgh.15190
28. Hu H, Gong L, Dong D, Zhu L, Wang M, He J, et al. Identifying early gastric cancer under magnifying narrow-band images with deep learning: a multicenter study. Gastrointest Endosc (2021) 93(6):1333–1341.e3. doi: 10.1016/j.gie.2020.11.014
29. Tang D, Ni M, Zheng C, Ding X, Zhang N, Yang T, et al. A deep learning-based model improves diagnosis of early gastric cancer under narrow band imaging endoscopy. Surg Endosc (2022) 36(10):7800–10. doi: 10.1007/s00464-022-09319-2
30. Horiuchi Y, Aoyama K, Tokai Y, Hirasawa T, Yoshimizu S, Ishiyama A, et al. Convolutional neural network for differentiating gastric cancer from gastritis using magnified endoscopy with narrow band imaging. Dig Dis Sci (2020) 65(5):1355–63. doi: 10.1007/s10620-019-05862-6
31. Namikawa K, Hirasawa T, Nakano K, Ikenoyama Y, Ishioka M, Shiroma S, et al. Artificial intelligence-based diagnostic system classifying gastric cancers and ulcers: comparison between the original and newly developed systems. Endoscopy (2020) 52(12):1077–83. doi: 10.1055/a-1194-8771
32. Kim YH, Kim GH, Kim KB, Lee MW, Lee BE, Baek DH, et al. Application of A convolutional neural network in the diagnosis of gastric mesenchymal tumors on endoscopic ultrasonography images. J Clin Med (2020) 9(10):3162–72. doi: 10.3390/jcm9103162
33. Zhu Y, Wang QC, Xu MD, Zhang Z, Cheng J, Zhong YS, et al. Application of convolutional neural network in the diagnosis of the invasion depth of gastric cancer based on conventional endoscopy. Gastrointest Endosc (2019) 89(4):806–815.e1. doi: 10.1016/j.gie.2018.11.011
34. Yoon HJ, Kim S, Kim J-H, Keum JS, Oh S-I, Jo J, et al. A lesion-based convolutional neural network improves endoscopic detection and depth prediction of early gastric cancer. J Clin Med (2019) 8(9):1310–9. doi: 10.3390/jcm8091310
35. Nagao S, Tsuji Y, Sakaguchi Y, Takahashi Y, Minatsuki C, Niimi K, et al. Highly accurate artificial intelligence systems to predict the invasion depth of gastric cancer: efficacy of conventional white-light imaging, nonmagnifying narrow-band imaging, and indigo-carmine dye contrast imaging. Gastrointest Endosc (2020) 92(4):866–873.e1. doi: 10.1016/j.gie.2020.06.047
36. Goto A, Kubota N, Nishikawa J, Ogawa R, Hamabe K, Hashimoto S, et al. Cooperation between artificial intelligence and endoscopists for diagnosing invasion depth of early gastric cancer. Gastric Cancer (2023) 26(1):116–22. doi: 10.1007/s10120-022-01330-9
37. Yoshida N, Dohi O, Inoue K. Necessity of image-enhanced endoscopy for detecting colorectal lesions. Dig Endosc (2022) 34(5):960–2. doi: 10.1111/den.14311
38. He X, Wu L, Dong Z, Gong D, Jiang X, Zhang H, et al. Real-time use of artificial intelligence for diagnosing early gastric cancer by magnifying image-enhanced endoscopy: a multicenter diagnostic study (with videos). Gastrointest Endosc (2022) 95(4):671–678.e4. doi: 10.1016/j.gie.2021.11.040
39. Kakushima N, Yoshida N, Doyama H, Yano T, Horimatsu T, Uedo N, et al. Near-focus magnification and second-generation narrow-band imaging for early gastric cancer in a randomized trial. J Gastroenterol (2020) 55(12):1127–37. doi: 10.1007/s00535-020-01734-3
40. Kaltenbach T, Sano Y, Friedland S, Soetikno R. American Gastroenterological Association (AGA) Institute technology assessment on image-enhanced endoscopy. Gastroenterology (2008) 134(1):327–40. doi: 10.1053/j.gastro.2007.10.062
41. Ezoe Y, Muto M, Uedo N, Doyama H, Yao K, Oda I, et al. Magnifying narrowband imaging is more accurate than conventional white-light imaging in diagnosis of gastric mucosal cancer. Gastroenterology (2011) 141(6):2017–2025.e3. doi: 10.1053/j.gastro.2011.08.007
42. Pimentel-Nunes P, Libânio D, Lage J, Abrantes D, Coimbra M, Esposito G, et al. A multicenter prospective study of the real-time use of narrow-band imaging in the diagnosis of premalignant gastric conditions and lesions. Endoscopy (2016) 48(8):723–30. doi: 10.1055/s-0042-108435
43. Yamada S, Doyama H, Yao K, Uedo N, Ezoe Y, Oda I, et al. An efficient diagnostic strategy for small, depressed early gastric cancer with magnifying narrow-band imaging: a post-hoc analysis of a prospective randomized controlled trial. Gastrointest Endosc (2014) 79(1):55–63. doi: 10.1016/j.gie.2013.07.008
44. Hirasawa T, Ikenoyama Y, Ishioka M, Namikawa K, Horiuchi Y, Nakashima H, et al. Current status and future perspective of artificial intelligence applications in endoscopic diagnosis and management of gastric cancer. Dig Endosc (2021) 33(2):263–72. doi: 10.1111/den.13890
45. Ling T, Wu L, Fu Y, Xu Q, An P, Zhang J, et al. A deep learning-based system for identifying differentiation status and delineating the margins of early gastric cancer in magnifying narrow-band imaging endoscopy. Endoscopy (2021) 53(5):469–77. doi: 10.1055/a-1229-0920
46. Wu L, He X, Liu M, Xie H, An P, Zhang J, et al. Evaluation of the effects of an artificial intelligence system on endoscopy quality and preliminary testing of its performance in detecting early gastric cancer: a randomized controlled trial. Endoscopy (2021) 53(12):1199–207. doi: 10.1055/a-1350-5583
47. Pimentel-Nunes P, Libânio D, Marcos-Pinto R, Areia M, Leja M, Esposito G, et al. Management of epithelial precancerous conditions and lesions in the stomach (MAPS II): European Society of Gastrointestinal Endoscopy (ESGE), European Helicobacter and Microbiota Study Group (EHMSG), European Society of Pathology (ESP), and Sociedade Portuguesa de Endoscopia Digestiva (SPED) guideline update 2019. Endoscopy (2019) 51(4):365–88. doi: 10.1055/a-0859-1883
48. Liu Q, Ding L, Qiu X, Meng F, Japanese Gastric Cancer Association. Updated evaluation of endoscopic submucosal dissection versus surgery for early gastric cancer: A systematic review and meta-analysis. Int J Surg (2020) 73:28–41. doi: 10.1016/j.ijsu.2019.11.027
49. Japanese Gastric Cancer Association. Japanese gastric cancer treatment guidelines 2018 (5th edition). Gastric Cancer (2021) 24(1):1–21.
50. Bourke MJ, Neuhaus H, Bergman JJ. Endoscopic submucosal dissection: indications and application in western endoscopy practice. Gastroenterology (2018) 154(7):1887–1900.e5. doi: 10.1053/j.gastro.2018.01.068
51. Hatta W, Gotoda T, Koike T, Masamune A. History and future perspectives in Japanese guidelines for endoscopic resection of early gastric cancer. Dig Endosc (2020) 32(2):180–90. doi: 10.1111/den.13531
52. Hasuike N, Ono H, Boku N, Mizusawa J, Takizawa K, Fukuda H, et al. A non-randomized confirmatory trial of an expanded indication for endoscopic submucosal dissection for intestinal-type gastric cancer (cT1a): the Japan Clinical Oncology Group study (JCOG0607). Gastric Cancer (2018) 21(1):114–23. doi: 10.1007/s10120-017-0704-y
53. Takizawa K, Ono H, Hasuike N, Takashima A, Minashi K, Boku N, et al. A nonrandomized, single-arm confirmatory trial of expanded endoscopic submucosal dissection indication for undifferentiated early gastric cancer: Japan Clinical Oncology Group study (JCOG1009/1010). Gastric Cancer (2021) 24(2):479–91. doi: 10.1007/s10120-020-01134-9
54. Japanese Gastric Cancer Association. Japanese gastric cancer treatment guidelines 2021 (6th edition). Gastric Cancer (2023) 26(1):1–25. doi: 10.1007/s10120-022-01331-8
55. Abe S, Oda I, Shimazu T, Kinjo T, Tada K, Sakamoto T, et al. Depth-predicting score for differentiated early gastric cancer. Gastric Cancer (2011) 14(1):35–40. doi: 10.1007/s10120-011-0002-z
56. Choi J, Kim SG, Im JP, Kim JS, Jung HC, Song IS, et al. Endoscopic prediction of tumor invasion depth in early gastric cancer. Gastrointest Endosc (2011) 73(5):917–27. doi: 10.1016/j.gie.2010.11.053
57. Mocellin S, Marchet A, Nitti D. EUS for the staging of gastric cancer: a meta-analysis. Gastrointest Endosc (2011) 73(6):1122–34. doi: 10.1016/j.gie.2011.01.030
58. Choi J, Kim SG, Im JP, Kim JS, Jung HC, Song IS, et al. Comparison of endoscopic ultrasonography and conventional endoscopy for prediction of depth of tumor invasion in early gastric cancer. Endoscopy (2010) 42(9):705–13. doi: 10.1055/s-0030-1255617
59. Kim J-H, Song KS, Youn YH, Lee YC, Cheon JH, Song SY, et al. Clinicopathologic factors influence accurate endosonographic assessment for early gastric cancer. Gastrointest Endosc (2007) 66(5):901–8. doi: 10.1016/j.gie.2007.06.012
60. Kubota K, Kuroda J, Yoshida M, Ohta K, Kitajima M. Medical image analysis: computer-aided diagnosis of gastric cancer invasion on endoscopic images. Surg Endosc (2012) 26(5):1485–9. doi: 10.1007/s00464-011-2036-z
61. Lin JS, Perdue LA, Henrikson NB, Bean SI, Blasi PR, et al. Screening for colorectal cancer: updated evidence report and systematic review for the US preventive services task force. Jama (2021) 325(19):1978–98. doi: 10.1001/jama.2021.4417
62. Qaseem A, Harrod CS, Crandall CJ, Wilt TJ, Clinical Guidelines Committee of the American College of Physicians, Balk EM, et al. Screening for colorectal cancer in asymptomatic average-risk adults: A guidance statement from the American college of physicians. Ann Intern Med (2019) 171(9):643–54. doi: 10.7326/M19-0642
63. Song M, Emilsson L, Bozorg SR, Nguyen LH, Joshi AD, Staller K, et al. Risk of colorectal cancer incidence and mortality after polypectomy: a Swedish record-linkage study. Lancet Gastroenterol Hepatol (2020) 5(6):537–47. doi: 10.1016/S2468-1253(20)30009-1
64. Winawer SJ, Zauber AG, Ho MN, O’Brien MJ, Gottlieb LS, Sternberg SS, et al. Prevention of colorectal cancer by colonoscopic polypectomy. The National Polyp Study Workgroup. N Engl J Med (1993) 329(27):1977–81. doi: 10.1056/NEJM199312303292701
65. Zauber AG, Winawer SJ, MJ, Lansdorp-Vogelaar I, Ballegooijen van M, Hankey BF, et al. Colonoscopic polypectomy and long-term prevention of colorectal-cancer deaths. N Engl J Med (2012) 366(8):687–96. doi: 10.1056/NEJMoa1100370
66. Doubeni CA, Corley DA, Quinn VP, Jensen CD, Zauber AG, Goodman M, et al. Effectiveness of screening colonoscopy in reducing the risk of death from right and left colon cancer: a large community-based study. Gut (2018) 67(2):291–8. doi: 10.1136/gutjnl-2016-312712
67. Doubeni CA, Weinmann S, Adams K, Kamineni A, Buist DSM, Ash AS, et al. Screening colonoscopy and risk for incident late-stage colorectal cancer diagnosis in average-risk adults: a nested case-control study. Ann Intern Med (2013) 158(5 Pt 1):312–20. doi: 10.7326/0003-4819-158-5-201303050-00003
68. Rex DK, Petrini JL, Baron TH, Chak A, Cohen J, Deal SE, et al. Quality indicators for colonoscopy. Gastrointest Endosc (2006) 63(4 Suppl):S16–28. doi: 10.1016/j.gie.2006.02.021
69. Corley DA, Levin TR, Doubeni CA. Adenoma detection rate and risk of colorectal cancer and death. N Engl J Med (2014) 370(26):2541. doi: 10.1056/NEJMoa1309086
70. Cross AJ, Robbins EC, Saunders BP, Duffy SW, Wooldrage K. Higher adenoma detection rates at screening associated with lower long-term colorectal cancer incidence and mortality. Clin Gastroenterol Hepatol (2022) 20(2):e148–67. doi: 10.1016/j.cgh.2020.09.020
71. Kaminski MF, Regula J, Kraszewska E, Polkowski M, Wojciechowska U, Didkowska J, et al. Quality indicators for colonoscopy and the risk of interval cancer. N Engl J Med (2010) 362(19):1795–803. doi: 10.1056/NEJMoa0907667
72. Kaminski MF, Thomas-Gibson S, Bugajski M, Bretthauer M, Rees CJ, Dekker E, et al. Performance measures for lower gastrointestinal endoscopy: a European Society of Gastrointestinal Endoscopy (ESGE) Quality Improvement Initiative. Endoscopy (2017) 49(4):378–97. doi: 10.1055/s-0043-103411
73. Wisse PHA, Erler NS, Boer SY, Hartog den B, Pool MO, Droste Sive Terhaar JS, et al. Adenoma detection rate and risk for interval postcolonoscopy colorectal cancer in fecal immunochemical test-based screening: A population-based cohort study. Ann Intern Med (2022) 175(10):1366–73. doi: 10.7326/M22-0301
74. Pohl H, Robertson DJ. Colorectal cancers detected after colonoscopy frequently result from missed lesions. Clin Gastroenterol Hepatol (2010) 8(10):858–64. doi: 10.1016/j.cgh.2010.06.028
75. Corley DA, Levin TR, Doubeni CA. Adenoma detection rate and risk of colorectal cancer and death. N Engl J Med (2014) 370(14):1298–306. doi: 10.1056/NEJMoa1309086
76. Anderson R, Burr NE, Valori R. Causes of post-colonoscopy colorectal cancers based on world endoscopy organization system of analysis. Gastroenterology (2020) 158(5):1287–1299.e2. doi: 10.1053/j.gastro.2019.12.031
77. Heresbach D, Barrioz T, Lapalus MG, Coumaros D, Bauret P, Potier P, et al. Miss rate for colorectal neoplastic polyps: a prospective multicenter study of back-to-back video colonoscopies. Endoscopy (2008) 40(4):284–90. doi: 10.1055/s-2007-995618
78. Kudo T, Saito Y, Ikematsu H, Hotta K, Takeuchi Y, Shimatani M, et al. New-generation full-spectrum endoscopy versus standard forward-viewing colonoscopy: a multicenter, randomized, tandem colonoscopy trial (J-FUSE Study). Gastrointest Endosc (2018) 88(5):854–64. doi: 10.1016/j.gie.2018.06.011
79. Leufkens AM, van Oijen MGH, Vleggaar FP, Siersema PD. Factors influencing the miss rate of polyps in a back-to-back colonoscopy study. Endoscopy (2012) 44(5):470–5. doi: 10.1055/s-0031-1291666
80. Rex DK, Cutler CS, Lemmel GT, Rahmani EY, Clark DW, Helper DJ, et al. Colonoscopic miss rates of adenomas determined by back-to-back colonoscopies. Gastroenterology (1997) 112(1):24–8. doi: 10.1016/S0016-5085(97)70214-2
81. Forbes N, Boyne DJ, Mazurek MS, Hilsden RJ, Sutherland RL, Pader J, et al. Association between endoscopist annual procedure volume and colonoscopy quality: systematic review and meta-analysis. Clin Gastroenterol Hepatol (2020) 18(10):2192–2208.e12. doi: 10.1016/j.cgh.2020.03.046
82. Hassan C, Pickhardt PJ, Rex DK. A resect and discard strategy would improve cost-effectiveness of colorectal cancer screening. Clin Gastroenterol Hepatol (2010) 8(10):865–9, 869.e1-3. doi: 10.1016/j.cgh.2010.05.018
83. Gralnek IM, Siersema PD, Halpern Z, Segol O, Melhem A, Suissa A, et al. Standard forward-viewing colonoscopy versus full-spectrum endoscopy: an international, multicentre, randomised, tandem colonoscopy trial. Lancet Oncol (2014) 15(3):353–60. doi: 10.1016/S1470-2045(14)70020-8
84. Bisschops R, East JE, Hassan C, Hazewinkel Y, Kamiński MF, Neumann H. Advanced imaging for detection and differentiation of colorectal neoplasia: European Society of Gastrointestinal Endoscopy (ESGE) Guideline - Update 2019. Endoscopy (2019) 51(12):1155–79. doi: 10.1055/a-1031-7657
85. Hassan C, Spadaccini M, Iannone A, Maselli R, Jovani M, Chandrasekar VT, et al. Performance of artificial intelligence in colonoscopy for adenoma and polyp detection: a systematic review and meta-analysis. Gastrointest Endosc (2021) 93(1):77–85.e6. doi: 10.1016/j.gie.2020.06.059
86. Parsa N, Byrne MF. Artificial intelligence for identification and characterization of colonic polyps. Ther Adv Gastrointest Endosc (2021) 14:26317745211014698. doi: 10.1177/26317745211014698
87. Hann A, Troya J, Fitting D. Current status and limitations of artificial intelligence in colonoscopy. United Eur Gastroenterol J (2021) 9(5):527–33. doi: 10.1002/ueg2.12108
88. Misawa M, Kudo S-E, Mori Y, Cho T, Kataoka S, Yamauchi A, et al. Artificial intelligence-assisted polyp detection for colonoscopy: initial experience. Gastroenterology (2018) 154(8):2027–2029.e3. doi: 10.1053/j.gastro.2018.04.003
89. Urban G, Tripathi P, Alkayali T, Mittal M, Jalali F, Karnes W, et al. Deep learning localizes and identifies polyps in real time with 96% Accuracy in screening colonoscopy. Gastroenterology (2018) 155(4):1069–1078.e8. doi: 10.1053/j.gastro.2018.06.037
90. Neumann H, Kreft A, Sivanathan V, Rahman F, Galle PR. Evaluation of novel LCI CAD EYE system for real time detection of colon polyps. PloS One (2021) 16(8):e0255955. doi: 10.1371/journal.pone.0255955
91. Wang P, Berzin TM, Brown JRG, Bharadwaj S, Becq A, Xiao X, et al. Real-time automatic detection system increases colonoscopic polyp and adenoma detection rates: a prospective randomised controlled study. Gut (2019) 68(10):1813–9. doi: 10.1136/gutjnl-2018-317500
92. Su JR, Li Z, Shao XJ, Ji CR, Ji R, Zhou RC, et al. Impact of a real-time automatic quality control system on colorectal polyp and adenoma detection: a prospective randomized controlled study (with videos). Gastrointest Endosc (2020) 91(2):415–424.e4. doi: 10.1016/j.gie.2019.08.026
93. Yamada M, Saito Y, Imaoka H, Saiko M, Yamada S, Kondo H, et al. Development of a real-time endoscopic image diagnosis support system using deep learning technology in colonoscopy. Sci Rep (2019) 9(1):14465. doi: 10.1038/s41598-019-50567-5
94. Wang P, Liu X, Berzin TM, Brown JRG, Liu P, Zhou C, et al. Effect of a deep-learning computer-aided detection system on adenoma detection during colonoscopy (CADe-DB trial): a double-blind randomised study. Lancet Gastroenterol Hepatol (2020) 5(4):343–51. doi: 10.1016/S2468-1253(19)30411-X
95. Repici A, Badalamenti M, Maselli R, Correale L, Radaelli F, Rondonotti E, et al. Efficacy of real-time computer-aided detection of colorectal neoplasia in a randomized trial. Gastroenterology (2020) 159(2):512–520.e7. doi: 10.1053/j.gastro.2020.04.062
96. Repici A, Spadaccini M, Antonelli G, Correale L, Maselli R, Galtieri PA, et al. Artificial intelligence and colonoscopy experience: lessons from two randomised trials. Gut (2022) 71(4):757–65. doi: 10.1136/gutjnl-2021-324471
97. Wang P, Liu P, Brown JRG, Berzin TM, Zhou G, Lei S, et al. Lower adenoma miss rate of computer-aided detection-assisted colonoscopy vs routine white-light colonoscopy in a prospective tandem study. Gastroenterology (2020) 159(4):1252–1261.e5. doi: 10.1053/j.gastro.2020.06.023
98. Ishiyama M, Kudo SE, Misawa M, Mori Y, Maeda Y, Ichimasa K, et al. Impact of the clinical use of artificial intelligence-assisted neoplasia detection for colonoscopy: a large-scale prospective, propensity score-matched study (with video). Gastrointest Endosc (2022) 95(1):155–63. doi: 10.1016/j.gie.2021.07.022
99. Soons E, Rath T, Hazewinkel Y, van Dop WA, Esposito D, Testoni PA, et al. Real-time colorectal polyp detection using a novel computer-aided detection system (CADe): a feasibility study. Int J Colorectal Dis (2022) 37(10):2219–28. doi: 10.1007/s00384-022-04258-9
100. Chen PJ, Lin MC, Lai MJ, Lin JC, Lu HHS, Tseng VS, et al. Accurate classification of diminutive colorectal polyps using computer-aided analysis. Gastroenterology (2018) 154(3):568–75. doi: 10.1053/j.gastro.2017.10.010
101. Byrne MF, Chapados N, Soudan F, Oertel C, Pérez ML, Kelly R, et al. Real-time differentiation of adenomatous and hyperplastic diminutive colorectal polyps during analysis of unaltered videos of standard colonoscopy using a deep learning model. Gut (2019) 68(1):94–100. doi: 10.1136/gutjnl-2017-314547
102. Kudo SE, Misawa M, Mori Y, Hotta K, Ohtsuka K, Ikematsu H, et al. Artificial intelligence-assisted system improves endoscopic identification of colorectal neoplasms. Clin Gastroenterol Hepatol (2020) 18(8):1874–1881.e2. doi: 10.1016/j.cgh.2019.09.009
103. Mori Y, Kudo SE, Misawa M, Saito Y, Ikematsu H, Hotta K, et al. Real-time use of artificial intelligence in identification of diminutive polyps during colonoscopy: A prospective study. Ann Intern Med (2018) 169(6):357–66. doi: 10.7326/M18-0249
104. Zachariah R, Samarasena J, Luba D, Duh E, Dao T, Requa J, et al. Prediction of polyp pathology using convolutional neural networks achieves “Resect and discard” Thresholds. Am J Gastroenterol (2020) 115(1):138–44. doi: 10.14309/ajg.0000000000000429
105. Rodriguez-Diaz E, Baffy G, Lo WK, Mashimo H, Vidyarthi G, Mohapatra SS, et al. Real-time artificial intelligence-based histologic classification of colorectal polyps with augmented visualization. Gastrointest Endosc (2021) 93(3):662–70. doi: 10.1016/j.gie.2020.09.018
106. Wang P, Xiao X, Brown JRG, Berzin TM, Tu M, Xiong F, et al. Development and validation of a deep-learning algorithm for the detection of polyps during colonoscopy. Nat BioMed Eng (2018) 2(10):741–8. doi: 10.1038/s41551-018-0301-3
107. Aslanian HR, Shieh FK, Chan FW, Ciarleglio MM, Deng Y, Rogart JN, et al. Nurse observation during colonoscopy increases polyp detection: a randomized prospective study. Am J Gastroenterol (2013) 108(2):166–72. doi: 10.1038/ajg.2012.237
108. Buchner AM, Shahid MW, Heckman MG, Diehl NN, McNeil RB, Cleveland P, et al. Trainee participation is associated with increased small adenoma detection. Gastrointest Endosc (2011) 73(6):1223–31. doi: 10.1016/j.gie.2011.01.060
109. Lee CK, Park DI, Lee S-H, Hwangbo Y, Eun CS, Han DS, et al. Participation by experienced endoscopy nurses increases the detection rate of colon polyps during a screening colonoscopy: a multicenter, prospective, randomized study. Gastrointest Endosc (2011) 74(5):1094–102. doi: 10.1016/j.gie.2011.06.033
110. González-Bueno Puyal J, Brandao P, Ahmad OF, Bhatia KK, Toth D, Kader R, et al. Polyp detection on video colonoscopy using a hybrid 2D/3D CNN. Med Image Anal (2022) 82:102625. doi: 10.1016/j.media.2022.102625
111. Mori Y, Kudo SE, Berzin TM, Misawa M, Takeda K. Computer-aided diagnosis for colonoscopy. Endoscopy (2017) 49(8):813–9. doi: 10.1055/s-0043-109430
112. von Renteln D, Kaltenbach T, Rastogi A, Anderson JC, Rösch T, Soetikno R, et al. Simplifying resect and discard strategies for real-time assessment of diminutive colorectal polyps. Clin Gastroenterol Hepatol (2018) 16(5):706–14. doi: 10.1016/j.cgh.2017.11.036
113. Neumann H, Sen HN, Vieth M, Bisschops R, Thieringer F, Rahman KF, et al. Leaving colorectal polyps in place can be achieved with high accuracy using blue light imaging (BLI). United Eur Gastroenterol J (2018) 6(7):1099–105. doi: 10.1177/2050640618769731
114. Niimi K, Fujishiro M, Kodashima S, Goto O, Ono S, Hirano K, et al. Long-term outcomes of endoscopic submucosal dissection for colorectal epithelial neoplasms. Endoscopy (2010) 42(9):723–9. doi: 10.1055/s-0030-1255675
115. Saito Y, Uraoka T, Yamaguchi Y, Hotta K, Sakamoto N, Ikematsu H, et al. A prospective, multicenter study of 1111 colorectal endoscopic submucosal dissections (with video). Gastrointest Endosc (2010) 72(6):1217–25. doi: 10.1016/j.gie.2010.08.004
116. Yoshida N, Naito Y, Yagi N, Yanagisawa A. Importance of histological evaluation in endoscopic resection of early colorectal cancer. World J Gastrointest Pathophysiol (2012) 3(2):51–9. doi: 10.4291/wjgp.v3.i2.51
117. Kominami Y, Yoshida S, Tanaka S, Sanomura Y, Hirakawa T, Raytchev B, et al. Computer-aided diagnosis of colorectal polyp histology by using a real-time image recognition system and narrow-band imaging magnifying colonoscopy. Gastrointest Endosc (2016) 83(3):643–9. doi: 10.1016/j.gie.2015.08.004
118. Takeda K, Kudo SE, Mori Y, Misawa M, Kudo T, Wakamura K, et al. Accuracy of diagnosing invasive colorectal cancer using computer-aided endocytoscopy. Endoscopy (2017) 49(8):798–802. doi: 10.1055/s-0043-105486
119. Tamai N, Saito Y, Sakamoto T, Nakajima T, Matsuda T, Sumiyama K, et al. Effectiveness of computer-aided diagnosis of colorectal lesions using novel software for magnifying narrow-band imaging: a pilot study. Endosc Int Open (2017) 5(8):E690–e694. doi: 10.1055/s-0043-105490
120. Mitsala A, Tsalikidis C, Pitiakoudis M, Simopoulos C, Tsaroucha AK. Artificial intelligence in colorectal cancer screening, diagnosis and treatment. A new era. Curr Oncol (2021) 28(3):1581–607. doi: 10.3390/curroncol28030149
121. Sharma P, Hassan C. Artificial intelligence and deep learning for upper gastrointestinal neoplasia. Gastroenterology (2022) 162(4):1056–66. doi: 10.1053/j.gastro.2021.11.040
Keywords: artificial intelligence, deep learning, gastric cancer, colorectal cancer, adenoma detection rate
Citation: Xin Y, Zhang Q, Liu X, Li B, Mao T and Li X (2023) Application of artificial intelligence in endoscopic gastrointestinal tumors. Front. Oncol. 13:1239788. doi: 10.3389/fonc.2023.1239788
Received: 14 June 2023; Accepted: 17 November 2023;
Published: 06 December 2023.
Edited by:
Cornelis F. M. Sier, Leiden University, NetherlandsReviewed by:
Albino Eccher, Integrated University Hospital Verona, ItalyCopyright © 2023 Xin, Zhang, Liu, Li, Mao and Li. This is an open-access article distributed under the terms of the Creative Commons Attribution License (CC BY). The use, distribution or reproduction in other forums is permitted, provided the original author(s) and the copyright owner(s) are credited and that the original publication in this journal is cited, in accordance with accepted academic practice. No use, distribution or reproduction is permitted which does not comply with these terms.
*Correspondence: Xiaoyu Li, bGl4aWFveXUwNUAxNjMuY29t
Disclaimer: All claims expressed in this article are solely those of the authors and do not necessarily represent those of their affiliated organizations, or those of the publisher, the editors and the reviewers. Any product that may be evaluated in this article or claim that may be made by its manufacturer is not guaranteed or endorsed by the publisher.
Research integrity at Frontiers
Learn more about the work of our research integrity team to safeguard the quality of each article we publish.