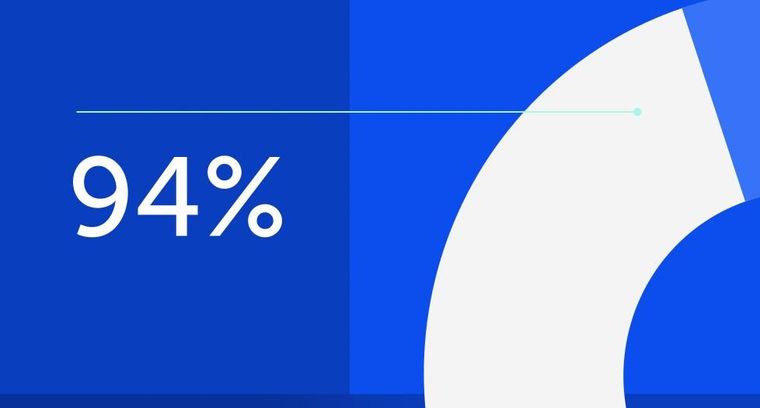
94% of researchers rate our articles as excellent or good
Learn more about the work of our research integrity team to safeguard the quality of each article we publish.
Find out more
ORIGINAL RESEARCH article
Front. Oncol., 25 September 2023
Sec. Cancer Molecular Targets and Therapeutics
Volume 13 - 2023 | https://doi.org/10.3389/fonc.2023.1228865
This article is part of the Research TopicDrug Repurposing for Cancer Treatment: Current and Future DirectionsView all 5 articles
Breast and lung cancer are two of the most lethal forms of cancer, responsible for a disproportionately high number of deaths worldwide. Both doctors and cancer patients express alarm about the rising incidence of the disease globally. Although targeted treatment has achieved enormous advancements, it is not without its drawbacks. Numerous medicines and chemotherapeutic drugs have been authorized by the FDA; nevertheless, they can be quite costly and often fall short of completely curing the condition. Therefore, this investigation has been conducted to identify a potential medication against breast and lung cancer through structural modification of genistein. Genistein is the active compound in Glycyrrhiza glabra (licorice), and it exhibits solid anticancer efficiency against various cancers, including breast cancer, lung cancer, and brain cancer. Hence, the design of its analogs with the interchange of five functional groups—COOH, NH2 and OCH3, Benzene, and NH-CH2-CH2-OH—have been employed to enhance affinities compared to primary genistein. Additionally, advanced computational studies such as PASS prediction, molecular docking, ADMET, and molecular dynamics simulation were conducted. Firstly, the PASS prediction spectrum was analyzed, revealing that the designed genistein analogs exhibit improved antineoplastic activity. In the prediction data, breast and lung cancer were selected as primary targets. Subsequently, other computational investigations were gradually conducted. The mentioned compounds have shown acceptable results for in silico ADME, AMES toxicity, and hepatotoxicity estimations, which are fundamental for their oral medication. It is noteworthy that the initial binding affinity was only −8.7 kcal/mol against the breast cancer targeted protein (PDB ID: 3HB5). However, after the modification of the functional group, when calculating the binding affinities, it becomes apparent that the binding affinities increase gradually, reaching a maximum of −11.0 and −10.0 kcal/mol. Similarly, the initial binding affinity was only −8.0 kcal/mol against lung cancer (PDB ID: 2P85), but after the addition of binding affinity, it reached −9.5 kcal/mol. Finally, a molecular dynamics simulation was conducted to study the molecular models over 100 ns and examine the stability of the docked complexes. The results indicate that the selected complexes remain highly stable throughout the 100-ns molecular dynamics simulation runs, displaying strong correlations with the binding of targeted ligands within the active site of the selected protein. It is important to further investigate and proceed to clinical or wet lab experiments to determine the practical value of the proposed compounds.
The human body is composed of countless tiny cells, and after a specific period, all the cells die and are replaced by new ones through a continuous process. When these cells develop out of control, they are known as tumors (1). Tumors can be classified into two types: benign and malignant. In detail, the uncontrolled growth of tissue is referred to as neoplasia or a tumor. Benign tumors are generally a result of regular cell function; furthermore, they do not become cancerous unless they spread to other parts of the body. When neoplasia extends beyond the vicinity of normal cells, it is termed a malignant tumor or cancer. In this case, cells continuously undergo uncontrolled differentiation, giving rise to what are known as cancer cells (2–4). Cancers initially grow similarly to benign tumors, and subsequently, the cells of the benign tumor may transform into malignant cells, giving rise to cancer. However, it is not guaranteed that all benign tumors will progress to cancer. Metastasis is a stage of cancer where malignant cells invade other areas and spread to neighboring tissues through the bloodstream and lymphatic system (5–8).
Consequently, cancer cells develop and grow uncontrolled, leading to a patient’s death. Based on current estimates, metastasis is accountable for approximately 90% of cancer fatalities (9, 10). Breast cancer is the most prevalent malignant growth in women, representing a complex and life-threatening disorder, particularly for female patients. Furthermore, breast cancer is among the most feared and widespread forms of cancer in women. It arises when a woman’s breast cells undergo irregular growth, especially within the milk-producing glands of the body (11, 12). It is estimated that approximately 50% of breast cancers occur in women with no known risk factors for breast cancer (over 40 years) (13, 14). According to many research findings, sexual dysfunction contributes significantly to breast cancer. Eventually, this is linked to various physiological factors, such as anxiety over fertility (15, 16).
Lung cancer is caused by a variety of factors, with the most common being smoking. Some lung cancer cases among individuals with no smoking history may be attributed to exposure to radon (222Rn), which emanates from building materials. Clinically, only a small percentage of NSCLC (non-small cell lung cancer) patients are diagnosed at an early stage, while the majority present with advanced or even metastatic disease, resulting in a 5-year survival rate of less than 15%. Despite some therapeutic advancements in conventional treatments over the last several decades, the prognosis for lung cancer remains challenging due to its unique physiological characteristics and the frequent occurrence of tumor escape (17, 18). Nowadays, lung cancer is regarded as one of the deadliest oncological diseases globally. Literature reports that more than 80% to 85% of all lung cancers are categorized as NSCLC. In 2020, lung cancer accounted for more than 2.2 million new cases and approximately 1.8 million deaths (19, 20).
Currently, numerous chemotherapeutic agents are available in the market, which have dangerous side effects such as damage to lung tissue, heart problems, infertility, kidney problems, and nerve damage (peripheral neuropathy) (21). Evidence illustrates that effective and innovative medication is very much needed to treat this disease with lower side effects. Developing an effective and commercially available drug is time-consuming and requires a lot of human resources, time, and money.
Therefore, we attempted to identify an active compound within natural plant sources and subsequently modified it to enhance its efficiency compared to the original compound. Our focus was on G. glabra (licorice) as a potential source for identifying such a compound. G. glabra was a common ingredient in numerous Ancient Greek medicinal formulations, and it contains a variety of bioactive compounds, including genistein, daidzin, ononin, and formononetin (22). Among them, it has been revealed that genistein can prevent the development of different cancer cells. The interruption of the cell cycle, which brings about a stoppage to the proliferation of cells, could be the causative agent of the growth inhibition observed in cancer cells (23). Moreover, genistein has strong anticancer efficiency against numerous diseases such as breast cancer, lung cancer, and brain cancer. Thus, although licorice is composed of numerous bioactive compounds, we have chosen genistein as our targeted drug (24). Hence, to save time, money, and resources, the computational technique should be a helpful method, and this is why this investigation, via structural modification of genistein (C15H10O5) belonging to a group of chemicals classified as “isoflavones”, has been performed (25).
Breast cancer and lung cancer need to be well treated due to the lack of potential medicine. In this study, we investigated the natural compound genistein and its derivatives for breast and lung cancer. Computer-aided drug design (CADD) techniques were applied to the dataset, such as PASS predictions, protein–ligand interactions, molecular dynamics (MD) simulation analysis, and ADMET profile estimations. Therefore, our results will add knowledge to breast and lung cancer drug design and development.
Genistein (C15H10O5) is classified as an isoflavone, which places it among the flavonoid family of compounds. It is a phytoestrogen that comes predominantly from legumes as its source material. It is a naturally occurring chemical component. It is said that it may improve several aspects of one’s health, including protection against osteoporosis, a decrease in the likelihood of developing cardiovascular disease, relief from the symptoms of post-menopause, and anticancer capabilities (26). In the past, a wide variety of research projects, both in vitro and in vivo, have been carried out to examine the possible anti-inflammatory effects of genistein (27, 28). Hence, several studies have shown that exposure to genistein may cause cancer cells to commit suicide via a variety of pathways, including those involved in cell signaling. Evidence of the apoptotic nature of genistein on breast cancer cells is emphasized, demonstrating that genistein may play a promising function. These data come from both in vitro and in vivo studies (29).
Several studies have shown that early introduction to genistein might lower the risk of developing breast cancer (30). Mammary terminal end buds are ducts that may be detected in young animals. These ducts include a significant number of undifferentiated cells that are susceptible to damage from carcinogens. The administration of genistein to young rats resulted in a reduction in the number of terminal end buds and an expansion of the number of lobules. Scientists concluded that pre-pubertal and adult treatment of experimentally induced breast cancer in genistein-protected rats should take place between the time of birth and the pre-pubertal stage of mammary gland growth for there to be a beneficial factor of genistein (29, 31). Scientists concluded that genistein acts as a chemo-preventive medicine during the pre-pubertal phase, which they feel correlates to the adolescent phase in human life. As a result of this research, it has been shown that one of the cellular mechanisms of action of genistein is a rise in the proliferation and differentiation of the breast (29).
We employed the PASS online web tool (http://way2drug.com/PassOnline/predict.phpp) to assess the antibacterial, antifungal, antiviral, and antineoplastic activity PASS prediction spectrum of the reported drug molecules. To begin, the structures of the drug molecules were drawn using ChemBioDraw and then converted into their SMILES formats with the assistance of the SwissADME free online tool (http://www.swissadme.ch).
Subsequently, the SMILES forms were uploaded to the PASS website to determine the antifungal, antiviral, and antineoplastic activity PASS prediction spectrum. This web tool incorporates more than 4,000 types of features, encompassing both drug and non-drug activities, enabling the identification of potential bioactive compounds with 90% authenticity (32, 33).
The measurement values provided by PASS are described by Pa (probability for active molecule) and Pi (probability for inactive molecule). For a molecule to be considered potential, the Pa and Pi values should fall within the range of 0.00 to 1.00, with Pa + Pi ≠ 1. In this context, biological actions with Pa > Pi are deemed probable for the selected drug molecule. PASS measurement values are described by Pa (probability for active molecule) and Pi (probability for inactive molecule). To be a potential molecule, the Pa and Pi values should range from 0.00 to 1.00, and generally, Pa + Pi ≠ 1. The biological actions with Pa > Pi are thought probable for a selected drug molecule (34, 35).
Firstly, genistein was prepared and drawn with ChemBioDraw (32). In this case, five activated functional groups have been chosen, namely, —COOH, NH2, OCH3, Benzene, and NH-CH2-CH2-OH. The parent molecule was genistein, which consists of a hydroxyl group in different positions of the aromatic ring. Thus, while designing analogs of genistein, the hydroxyl group of genistein was modified, and substitution of —COOH, NH2, OCH3, Benzene, and NH-CH2-CH2-OH functional groups. After that, these modified structures of genistein were optimized with the help of material studio software (36) and saved as a PDB file type for further computational studies such as molecular docking, ADMET, protein–ligand interaction, MD simulation, and Lipinski rule. In Figure 1, optimized structures are displayed.
To determine the pharmacokinetics and toxicity of the drugs as mentioned above, we used the web-based servers pkCSM (http://biosig.unimelb.edu.au/pkcsm/prediction) (37). This online database has been used to assess the pharmacokinetics parameters related to drug absorption, metabolism, and toxicity features. This machine learning method predicts the pharmacokinetics parameter based on the structural similarity of the chemical associated with known ADMET features. Moreover, this is the most trusted and well-known machine learning-based tool for forecasting ADMET (38). The essential data include water solubility Log S, human intestinal absorption, Caco-2 permeability, VDss (human), blood–brain barrier (BBB) permeability, CYP450 1A2 inhibitor, CYP450 2C9 substrate, total clearance (ml/min/kg), and renal OCT2 substrate. Then, toxicity data including AMES toxicity, max. tolerated dose, oral rat acute toxicity (LD50), oral rat chronic toxicity, hepatotoxicity, skin sensitization, and Tetrahymena pyriformis toxicity were analyzed. Lipinski’s rule of five is often used to assess the drug-likeness of chemical or bioactive molecules. Any chemical compound should be an oral drug if it follows Lipinski’s rule of five, which includes molecular mass and the number of hydrogen bond acceptors, the number of hydrogen bond donors, the number of rotatable bonds, molar refractivity, and bioavailability score. Lipinski’s rule of five, topological polar surface area (TPSA), and solubility were estimated using this method. Additionally, to determine this feature, the SwissADME (http://www.swissadme.ch/index.php) online application was used, and the required data were listed (39).
The three-dimensional (3D) protein of breast cancer (PDB ID: 3HV5) and lung cancer (PDB ID: 2P85) was selected and downloaded from the Protein Data Bank (PDB) as a PDB file type (Table 1) (40, 41). Gradually, all hetero atoms, excess water molecules, and other impurities were cleaned away by implementing Pymol application (42). Finally, the optimized selected compounds were picked up for molecular docking study with breast cancer (PDB ID: 3HV5) and lung cancer (PDB ID: 2P85). In the end, the PyRx software was used to create the molecular docking interaction with AutoDock Vina (43). The protein information and tertiary structure are displayed in Figure 2.
Figure 2 Three-dimensional structure of selected breast cancer and lung cancer target proteins used in this study.
The MD simulations of the targeted breast cancer protein with the selected ligand complex have been conducted to establish the binding accuracy of the five newly developed drug candidates (L02, L03, L08, L09, and epirubicin hydrochloride) to the targeted protein breast cancer (PDB ID 3HB5). The “Desmond v3.6 program” in Schrödinger (https://www.schrodinger.com/) has been implemented for MD simulation of the macromolecular protein and ligand complex in a high configuration desktop of Linux mode to determine the thermodynamic stability (44). For this methodology, a pre-determined TIP3P water technique was introduced to ensure a certain capacity with the rhombohedral periodic bounding box geometry at a value of 10. This strategy was designed to keep the volume constant. Suitable ions, such as 0+ and 0.15 M salt, were selected to neutralize the foundation electronically, and these ions have been arbitrarily distributed throughout the suitable solvent. After developing the solvency protein system with a ligand complex, the system framework was decreased and loosened via the predefined protocol created by merely making use of the Desmond module’s OPLS3e parameters for the force field and the implementation details (45). There were 50 PS capture intervals of 1.2 kcal/mol each in NPT components using the temperature amalgamation of Nose-Hoover and the isotropic method, which were held at 300 K and one atmospheric pressure.
The Schrodinger Maestro program version 9.5 was used to create every MD simulation illustration. The macromolecular Interaction Schematic of the Desmond mode in the Schrodinger application was utilized to examine the simulation activity; correspondingly, this analysis was performed so that the accuracy of the MD could be verified. RMSD, RMSF, intramolecular hydrogen bonds, protein–ligand contacts (P-L), solvent-accessible surface area (SASA), the radius of gyration (Rg) score, MolSA, and polar surface area (PSA) were used to evaluate the stability of the protein–ligand complex structure.
Root mean square deviation (RMSD) is a measure utilized in MD simulations that measures how far a single atom has moved over a certain amount of time compared to a reference point (46). First, the RMSD of macromolecular structures such as side chains, backbone, and heavier particles is computed. Next, the RMSD of protein fit ligand atoms from all time frames is generated, and then they are adjusted and evaluated in comparison to the required time (100 ns). The following equation may be used to calculate the RMSD of an MD simulation with period x (Eq. 1).
Here, N shows the number of atoms chosen; tref is the reference time, and r’ conveys the placement of the bit selected in the system x after superimposing the point of the reference system.
The root mean square fluctuation (RMSF) has been mainly used to identify and monitor variations in dynamic structure within the binding protein (47). The RMSF value of an MD simulation of a protein with a particular number of residues may be determined with the help of the following equation (Eq. 2), which can be found in the previous sentence (Eq. 1).
Here, T generally denotes the trajectory time; tref denotes the given time; r’ denotes the location of the reported molecules in a framework I after transposing on the given frame, and (<>) suggests the average square distances covered over residues.
The PASS prediction parameter is used to assess the probability to be active (Pa) and Pi probability to be inactive in the potential bioactive compounds (48). The values of Pa and Pi range might be 0.00 to 1.0, and importantly, Pa + Pi ≠ 1, indicating that a compound cannot be fully active and inactive simultaneously (49). In the above discussion, our reported chemical compounds (Table 1) have carried the most potent antineoplastic activity, which is above Pa value 0.650+ by most of the molecules and owing to Ligand no 01, 05, 06, 07, 08, 10 and 11 showed the highest Pa value (Pa > 0.750, Pa > 0.771, Pa>735, Pa>0.712, Pa> 0.776, Pa> 0.742, and Pa > 0.783), where the antiviral, antibacterial, and antifungal values are reported as 0.096–0.339, 0.265–0.442, and 0.241–0.502. Such high Pa values underscore their potential as effective antineoplastic agents. The primary concern is to determine the antineoplastic properties. We also calculated the antiviral, antibacterial, and antifungal features to assess the potential Pa and Pi parameters and compare them with the antineoplastic activity. It became evident that the probability of being active (Pa) and inactive (Pi) is more favorable for antineoplastic activity compared to antiviral, antibacterial, and antifungal activities. Hence, based on these features, breast cancer and lung cancer target proteins have been chosen from the PDB for further investigation.
The drug (ADMET) profiles of biologically active compounds, such as membrane permeability, gastrointestinal absorption and bioavailability, partition coefficient (log P), molecular weight (MW), and the number of hydrogen bond acceptors/donors, represent overall features of chemical acceptances and drug-likeness in accordance with the Lipinski rule, among other things (50). It has been determined that all the newly developed molecules completely satisfied all the criteria and follow the Lipinski rule after studying the Lipinski rule, pharmacokinetics, and drug-likeness (Table 2). Whereas, the features of the G.I. absorption rate are very high according to the obtained result, and only drug molecule 09 has a lower G.I. absorption rate. Finally, it has been reported that all the mentioned ligands have better oral bioavailability scores according to the finding from the SwissADME (http://www.swissadme.ch/index.php) online application. Consequently, it could be summarized that these mentioned drugs should be used as an oral medication against life-threatening breast cancer.
Table 2 Summary of ligand calculated results for Lipinski rule, pharmacokinetics, and drug-likeness activities.
Structure-based drug design, such as the molecular docking technique, plays a fundamental role. This technique’s principal purpose is to find probable binding geometries of a suspected receptor of a specified 3D structure with a biological target using molecular docking simulations (51). Using the PyRx AutoDock Vina tool, probable binding affinities and interaction sites with the active site of breast and lung cancer-targeted proteins have been determined (52). The potential binding energies of the targeted site of the breast and lung cancer targeted proteins are summarized in Table 3 for all the reported compounds. The standard docking score has been regarded as −6.0 kcal/mol to be active molecules (53, 54). The range of docking score is −8.4 kcal/mol to −11.00 kcal/mol for breast cancer (PDB ID: 3HB5), while the binding affinity range for lung cancer is −7.2 kcal/mol to −9.5 kcal/mol. According to the finding obtained from docking analysis, the maximum score has been reported as −11.0 kcal/mol and −10.0 kcal/mol in ligand nos. 08 and 09 against PDB ID: 3HB5, while the maximum docking score of −9.5 kcal/mol was obtained against lung cancer (PDB ID: 2P85). Finally, it could be summarized that the designing derivatives of genistein (C15H10O5) are much better compared with the FDA-approved epirubicin hydrochloride. Thus, they should be suggested as treatment against both breast cancer and lung cancer and might be potential drug candidates.
Table 3 Binding affinities of docked ligand calculated against breast and lung cancer targeted proteins.
The interactions between the inhibitor and the targeted protein active side, docking pocket ligand–protein, are graphically represented in Figure 3. In the three-dimensional configuration, the active side of the amino acid residue has been analyzed. In most cases, the number of hydrophobic bond residues is higher than the others, such as hydrogen as electrostatic, van der Waals interactions, and halogen bonds. This protein–ligand interaction has been designed by importing the protein–ligand complex file into the Discovery Studio Visualizer and UCSF Chimera (53). The reported active sites and binding pocket of breast cancer complex with ligand 08 and ligand 09 and lung cancer complex with ligand 09 are displayed in Figures 3A–C.
Figure 3 Molecular docking pocket and active site analysis. (A) Breast cancer complex with ligand 08. (B) Breast cancer complex with ligand 09. (C) Lung cancer complex with ligand 09.
The pkCSM methodology approach was applied to investigate the pharmacokinetic and ADMET physicochemical features of the reported drugs, such as water solubility Log S, Caco-2 permeability, VDss (human) BBB permeability, CYP450 1A2 inhibitor, CYP450 2C9 inhibitor, total clearance, and renal OCT2 substrate, while all these features influence the absorption, distribution, metabolism, and excretion of drug molecules. This feature is essential for a drug to be physiologically active and reach its target organ in the physiological system by a sample concentration (55).
The investigation has shown that each compound’s water solubility range is different. The solubility range is −2.892 to −5.035, which indicates that most of the compounds are highly soluble in physiological conditions; in comparison, only ligand 08 is poorly soluble. Noted that the solubility feature of the compounds is categorized as insoluble if the value is more negative than −10. The solubility ranges from poorly soluble to highly soluble, with values ranging from −10 to greater than zero, respectively. Compounds with values between −10 and −6 are considered poorly soluble, while those higher than −6 and less than −4 are classified as moderately soluble; soluble compounds might be between −4 to −2, and when the value is between −2 and 0, compounds are very soluble (56). The membrane permeability is represented by the colon cancer cell line (Caco-2)] in our investigated drugs, and the maximum has been observed in ligand 07 (1.058 × 10−6). The VDss (human) level varies from −0.495 to 0.277. Another critical parameter is BBB, and these data were collected from SwissADME online tools, which indicate that only ligand 07 can cross the BBB, and the other 10 ligands cannot pass the BBB. Since metabolism is investigated based on the cytochrome P450 enzyme for substrate (CYP1A2 and CYP 2C9), the ADME predicted table revealed that maximum ligand can be metabolized or inhibited in CYP450 1A2 inhibitor and CYP450 1A2 inhibitor 2C9. The pkCSM model has been implemented to measure the pharmacokinetics of the total clearance log (CLtot) of a mentioned compound in the log(ml/min/kg). The higher the CLtot ranges of the ligands, the faster the excretion rate, and it is revealed that ligand 02 has maximum clearance rates (0.492 ml/min/kg). The different biochemical and ADME features of the ligands demonstrated that all candidates have excellent pharmacokinetic properties (Table 4).
Numerous drugs or potent molecules fail after development or during development stages due to aquatic and non-aquatic toxicity, which impacts the ecosystem and phycological system. Thus, aquatic and non-aquatic toxicity parameters have a significant rule to be established and made commercially available in the market. AMES toxicity, max. tolerated dose, oral rat acute toxicity, oral rat chronic toxicity, hepatotoxicity, skin sensitization, and T. pyriformis toxicity were listed in the table to evaluate the toxicity level. In AMES toxicity level, ligands 01, 02, 04, 06, 07, and 11 have no effects, while the remaining ligands positively respond to AMES toxicity. It signifies that the chemical (03, 05, 08, 09, and 10) is carcinogenic and, as a result, can potentially cause cancer. The tolerated dose (human) is approximately 1.068 mg/kg/day in ligand 11, indicating that if a person should take higher than 1.068 mg/kg/day, this drug should produce toxicity. The oral rat acute toxicity (LD50) level (1–2.678 mol/kg) and the oral rat chronic toxicity level (1–50.324 mg/kg/day) indicate that the compounds may be lethal if given at very high doses. No drugs affect skin sensitization while only ligands 07 and 11 may produce hepatotoxicity (Table 5).
Table 5 Summary of aquatic and non-aquatic toxicity results for 11 selected derivatives of genistein.
The goal of MD simulation is to get a real-time understanding of the stabilization and intermolecular connection of a protein–ligand complex. This method may also be used to quantify the structural change that occurs in a complex system after it has been subjected to an enclosed environment (57).
In this study, a 100-ns MD simulation of the protein in contact with a particular ligand was performed to comprehend the protein’s conformational changes. The terminal illustration of MD simulations results at 100 ns, and movements of the molecule were stabilized for evaluation of the intermolecular dynamics.
When the RMSD range of 1–3 Å is present during the simulation, it has been counted that the ligand–protein is bound with each other perfectly, and smaller deviations suggest that the complicated structure is more stable than it seems. This shows that the protein’s structure has changed significantly if the RMSD value is larger than 1–3 Å. In light of this investigation, the MD simulations were carried out for a period of 100 ns in order to ascertain the conformational change that occurred in the target protein since it was in the presence of the four ligand substances L02, L03, L08, and L09, as well as epirubicin hydrochloride. The blue color represents L02, orange represents L03, gray represents L08, yellow represents L09, and sky blue represents epirubicin hydrochloride. For the bioactive compound L02, the average RMSD was between 1 and 2 Å; L03, 1–1.8 Å; L08, 1–1.8 Å; L09, 1–3 Å; and epirubicin hydrochloride, 1–2.5 Å. In this investigation, the RMSD score changed with the change of the period, and finally, fell in the 1–3 Å range at 100 ns, which indicates that they are perfectly bonded with the targeted protein (Figure 4).
Figure 4 Comparison of RMSD values among the top 5 compounds: 02, 03, 08, 09, and epirubicin hydrochloride.
The RMSF may help characterize and determine the specific changes occurring in the protein chain when specific ligand molecules bind with distinct positions, and it is useful for obtaining details on the fluctuations that occur per residue in protein–ligand complexes (58). As seen in Figure 5, the RMSF values of compounds L02, L03, L08, and L09 in association with the breast cancer protein (PDB ID: 3HB5) were determined to examine the modification in protein structural plasticity induced by the binding of designated ligand molecules to a certain residual location. Figure 5 represents the RMSF values measured for the complex of breast cancer protein (PDB ID: 3HB5) in the docked protein–ligand complex. Since this study has been performed based on higher binding energy, it is clearly understood that the RMSF score is also much better in a stable configuration.
Figure 5 Comparison of RMSF values among the top 5 compounds: 02, 03, 08, 09, and Epirubicin hydrochloride.
The average RMSF values are presented at 4.2 Å. Each colored peak presented the RMSF fluctuation values among five docked complexes.
The radius of gyration, often known as Rg, was calculated so that investigators could evaluate how efficient the protein was both in the absence and the presence of simulated impacts, and the distribution of a protein–ligand interaction system’s atoms along its axis is one way to describe the properties. The computation of Rg is one of the most critical signs to look for when attempting to forecast the structural functioning of a macromolecule. This is because it exposes variations in the complex efficiency over time, which is among the most important things to determine. The Rg investigation provides a comprehensive view of the bending and expansion of the protein structure as a consequence of the interaction of simulation hits.
According to the finding in Figure 6, the average Rg values have been computed for breast cancer protein (PDB ID: 3HB5); L02, L08, L09, and standard epirubicin hydrochloride obtained a value of 4.5–4.70, while L03 obtained an Rg score of 4.0 from the beginning to the endpoint. According to the findings, the binding of compounds L02, L03, L08, and L09 resulted in a considerable enhancement of the stability.
Figure 6 Comparison of the radius of gyration values among the top 5 compound docked complexes: 02, 03, 08, 09, and epirubicin hydrochloride.
Protein or biological macromolecule folding and stability investigation have historically relied heavily on the amount of solvent-accessible surface area (SASA) of the protein. It is described as the surface that is circumscribed around a protein by a theoretical center of a solvent spherical that is in touch with the van der Waals contact surface of the biological macromolecules. It has been regarded as amino acid residues on a protein’s surface that act as potential binding sites and/or engagement with the potential drug candidate. This feature enhances the characteristics of biological macromolecules including hydrophilicity or hydrophobicity and also protein–ligand binding relation.
Figure 7 shows that protein–ligand engagement was utilized to derive the SASA of the substances involved in the interaction. The reported bioactive molecules L02, L03, L08, L09, and standard epirubicin hydrochloride have been displayed in blue, sky blue, orange, yellow, and gray, respectively. The bioactive molecules had an average SASA value of 0 to 410 A2, suggesting that a high proportion of the designated ligand molecules was present in a complicated medium.
Figure 7 Comparison of solvent accessible surface area (SASA) values among the top 5 compounds: 02, 03, 08, 09, and epirubicin hydrochloride.
The molecular surface area, also known as the MolSA, is comparable to the van der Waals surface area, and it has been computed using a probe radius of 1.4 Å. In the investigation of the current research, ligands L02, L08, and L09 have obtained a better van der Waals surface area (Figure 8).
Figure 8 Comparison of solvent molecular surface area (MolSA) values among the top 5 compounds: 02, 03, 08, 09, and epirubicin hydrochloride.
Furthermore, primarily oxygen and nitrogen atoms participate in a substance’s PSA while all three ligands (L02, L08, and L09) had high PSA values when tested with the specific biological macromolecules or proteins (Figure 9). PSA has been demonstrated to help with cellular efficiency, intestinal permeability, and BBB penetration or limitation to peripheral circulation (59). The protein–ligand engagement schematic was used to derive the calculations to determine the required PSA of the bioactive reported molecules involved in the formation of the protein–ligand complex. The finding of this investigation has been reported from 100 Å to 310 Å, which could be described as outstanding to be a potent medication.
Figure 9 Comparison of polar surface area (PSA) values among the top 5 compounds: 02, 03, 08, 09, and epirubicin hydrochloride.
The combination of the complex structure of a protein with the ligands consisted of different intermolecular interactions during the simulation of 100 ns using a tool called the simulation interactions diagram (SID) (60). The dependencies between macromolecular proteins and bioactive ligands that were found during the 100-ns simulation are displayed as stacked bar charts in Figure 10. The four bioactive molecules and standard epirubicin hydrochloride have been analyzed and graphically represented in this portion, such as L02, L03 L08, and L09 and standard epirubicin hydrochloride engaging in or binding with selected breast cancer macromolecules. Several different bonds have been seen during the formation of the protein–ligand complex including the non-covalent bond (hydrophobic bond), the hydrogen bond, the ionic bond, and the water bridge bond. During the 100-ns simulation, it is revealed that all molecules developed and produced several molecular couplings with the particular protein involving hydrogen, hydrophobic, ionic, and water bridge bonds, which finally help them form a stable configuration.
Figure 10 Comparison of intramolecular bond analysis values among the top 5 compounds: 02 (A), 03 (B), 08 (C), 09 (D), and epirubicin hydrochloride (E). Each colored bar presents a specific type of binding interaction among the docked complexes of the five compounds.
The engagement schematic (Figure 10) from the 100-ns simulation was used to compute the molecular surface area, abbreviated as “MolSA,” of the protein–ligand engagement complexes. The colors blue, orange, gray, and yellow were used to represent the documented bioactive compounds L02, L03, L08, and L09, respectively. The standard epirubicin hydrochloride is also included, shown in sky blue.
Through the use of MD modeling, it should be verified that a protein can maintain its stability while bound to ligands and can form a stable configuration, which also verifies the molecular docking simulation (61). It has also been able to obtain tightness, which described protein–ligand complexes remaining stable in a certain environment after reaching a psychological system. The maximal consistency of a biomolecule is reflected by its RMSD, while the mean fluctuations that characterize the tightness of the protein–ligand complex are defined by its RSMF measurements (62). The protein–ligand complex’s activities were used to compute the system’s RMSD, which confirmed the protein’s low degree of variability or minimum fluctuation. The RMSF measurement was utilized to determine the fluctuation of the protein, which indicated a decreased fluctuation overall, thus confirming the compound’s consistency regarding the target protein. According to the finding, the selected breast cancer protein and all four of its ligands (L02, L03, L08, and L09) have shown significant RMSD and RMSF values.
For Rg, the center of mass from the C and N terminals of the protein has been estimated, which investigated the protein reinforcement and offered a more comprehensive evaluation of protein folding properties (63). The elevated Rg quantity, on the other hand, implies that the chemicals have been disassociated from the protein, while the lower the Rg score, the greater the tightness. The structure has been considered less stable when the SASA value is larger, and the complex of water molecules and amino acid residues is more densely compacted when the SASA value is greater. According to our findings, all of the ligand-tested compounds had optimal Rg, SASA, MolSA, and PSA scores to be potent drug candidates.
Breast and lung cancer are major global health concerns, prompting the need for effective treatments. Although targeted therapy has progressed, side effects and acquired resistance remain challenges. Existing FDA-approved drugs and chemotherapies are costly and often ineffective. Thus, novel agents like genistein, derived from Glycyrrhiza glabra (licorice), offer hope in combating breast and lung cancers, according to our in silico findings. In our present CADD study, we designed 11 genistein derivatives through modification of side chains and functional groups. This investigation revealed significant protein–ligand docking interactions, stable conformations during MD simulations, favorable drug-like properties, and reliable PASS prediction for all derivatives. As a result, these compounds demonstrate promise as bioavailable oral drugs for breast and lung cancer treatment.
Notably, compounds 08 and 09 showed particularly strong binding affinities of −11.0 kcal/mol and −10.0 kcal/mol against breast cancer (PDB ID: 3HB5). Ligands 03 and 09 also displayed notable binding energies of −8.8 kcal/mol and −9.5 kcal/mol against lung cancer, with minimal concerns regarding AMES or hepatotoxicity. The majority of the drugs met ADMET profile parameters, exhibiting good water solubility and high gastrointestinal absorption. Additionally, drug 07 showed potential in crossing the BBB.
Further validation through 100-ns MD simulations of selected complexes (L02, L03, L08, and L09) confirmed their stability in suppressing targeted proteins, with minor fluctuations in RMSD and RMSF measurements. Moreover, these ligands demonstrated promising outcomes in SASA, Rg, MolSA, and PSA evaluations. Finally, it should be summarized that these findings suggest that the designed compounds hold great promise as alternative and improved treatment options for breast and lung cancer, and further experimental work should be carried out.
The raw data supporting the conclusions of this article will be made available by the authors, without undue reservation.
Conceptualization: SA and SB; methodology: SA, PB, and NS; software and visualizations: SA, SB, PB, DK, MNH, and MEH.; validation: SB, SA, PB, and H-AN; data curation: SA, PB, DK, MB, and YJ; writing—original draft preparation: SA, SB, PB; writing—review and editing: H-AN, YJ, and NS; investigation and supervision: SB and MB. All authors have read and agreed to the published version of the manuscript.
The authors would like to extend their sincere appreciation to the Researchers Supporting Project, King Saud University, Riyadh, Saudi Arabia for funding this work through project number RSP2023R457.
The authors declare that the research was conducted in the absence of any commercial or financial relationships that could be construed as a potential conflict of interest.
All claims expressed in this article are solely those of the authors and do not necessarily represent those of their affiliated organizations, or those of the publisher, the editors and the reviewers. Any product that may be evaluated in this article, or claim that may be made by its manufacturer, is not guaranteed or endorsed by the publisher.
1. Warburg O. On the origin of cancer cells. Science (1956) 123:309–14. doi: 10.1126/science.123.3191.309
2. Samah AA, Fauzi MFA, Mansor S. (2017). Classification of benign and Malignant tumors in histopathology images, in: Proceedings of the 2017 IEEE International Conference on Signal and Image Processing Applications (ICSIPA), pp. 102–6.
3. Franks LM, Teich NM. Introduction to the cellular and molecular biology of cancer. USA: Oxford University Press (1997).
4. Chaplain MAJ, Sleeman BD. Modelling the growth of solid tumours and incorporating a method for their classification using nonlinear elasticity theory. J Math Biol (1993) 31:431–73. doi: 10.1007/BF00173886
5. Pierce GB, Wallace C. Differentiation of Malignant to benign cells. Cancer Res (1971) 31:127–34. Available at: https://aacrjournals.org/cancerres/article/31/2/127/478292/Differentiation-of-Malignant-to-Benign-Cells1.
6. Rusch V, Baselga J, Cordon-Cardo C, Orazem J, Zaman M, Hoda S, et al. Differential expression of the epidermal growth factor receptor and its ligands in primary non-small cell lung cancers and adjacent benign lung. Cancer Res (1993) 53:2379–85. Available at: https://aacrjournals.org/cancerres/article/53/10/2379/498817/Differential-Expression-of-the-Epidermal-Growth.
7. Ramos JR, Pabijan J, Garcia R, Lekka M. The softening of human bladder cancer cells happens at an early stage of the Malignancy process. Beilstein J nanotechnol (2014) 5:447–57. doi: 10.3762/bjnano.5.52
8. Alibert C, Goud B, Manneville JB. Are cancer cells really softer than normal cells? Biol Cell (2017) 109:167–89. doi: 10.1111/boc.201600078
9. Carcangiu ML, Zampi G, Pupi A, Castagnoli A, Rosai J. Papillary carcinoma of the thyroid. A clinicopathologic study of 241 cases treated at the University of Florence, Italy. Cancer (1985) 55:805–28. doi: 10.1002/1097-0142(19850215)55:4<805::AID-CNCR2820550419>3.0.CO;2-Z
10. Franssen LC, Lorenzi T, Burgess AE, Chaplain MA. A mathematical framework for modelling the metastatic spread of cancer. Bull Math Biol (2019) 81:1965–2010. doi: 10.1007/s11538-019-00597-x
11. Kelsey JL, Bernstein L. Epidemiology and prevention of breast cancer. Annu Rev Public Health (1996) 17:47–67. doi: 10.1146/annurev.pu.17.050196.000403
12. Ghoncheh M, Pournamdar Z, Salehiniya H. Incidence and mortality and epidemiology of breast cancer in the world. Asian Pacific J Cancer Prev (2016) 17:43–6. doi: 10.7314/APJCP.2016.17.S3.43
13. Fregene A, Newman LA. Breast cancer in sub-Saharan Africa: how does it relate to breast cancer in African-American women? Cancer: Interdiscip Int J Am Cancer Soc (2005) 103:1540–50. doi: 10.1002/cncr.20978
14. Rosner B, Colditz GA, Willett WC. Reproductive risk factors in a prospective study of breast cancer: the Nurses’ Health Study. Am J Epidemiol (1994) 139:819–35. doi: 10.1093/oxfordjournals.aje.a117079
15. Male DA, Fergus KD, Cullen K. Sexual identity after breast cancer: sexuality, body image, and relationship repercussions. Curr Opin Support Palliat Care (2016) 10:66–74. doi: 10.1097/SPC.0000000000000184
16. Avis NE, Crawford S, Manuel J. Psychosocial problems among younger women with breast cancer. Psycho-Oncol: J Psychological Soc Behav Dimensions Cancer (2004) 13:295–308. doi: 10.1002/pon.744
17. Guo Q, Liu L, Chen Z, Fan Y, Zhou Y, Yuan Z, et al. The current treatments for non-small cell lung cancer. Front Oncol (2022), 3862. doi: 10.3389/fonc.2022.945102
18. Li BT, Smit EF, Goto Y, Nakagawa K, Udagawa H, Mazières J, et al. Trastuzumab deruxtecan in HER2-mutant non–small-cell lung cancer. N Engl J Med (2022) 386:241–51. doi: 10.1056/NEJMoa2112431
19. Carrillo-Perez F, Morales JC, Castillo-Secilla D, Molina-Castro Y, Guillén A, Rojas I, et al. Non-small-cell lung cancer classification. via RNA-Seq Histol Imaging probability fusion. BMC Bioinf (2021) 22:1–19. doi: 10.1186/s12859-021-04376-1
20. Fernández LP, Merino M, Colmenarejo G, Moreno-Rubio J, Sánchez-Martínez R, Quijada-Freire A, et al. Metabolic enzyme ACSL3 is a prognostic biomarker and correlates with anticancer effectiveness of statins in non-small cell lung cancer. Mol Oncol (2020) 14:3135–52. doi: 10.1002/1878-0261.12816
21. Basak D, Arrighi S, Darwiche Y, Deb S. Comparison of anticancer drug toxicities: paradigm shift in adverse effect profile. Life (2021) 12:48. doi: 10.3390/life12010048
22. Mamedov NA, Egamberdieva D. Phytochemical constituents and pharmacological effects of licorice: a review. In: Plant and human health, Volume 3: Pharmacology and therapeutic uses (2019). p. 1–21.
23. Spagnuolo C, Russo GL, Orhan IE, Habtemariam S, Daglia M, Sureda A, et al. Genistein and cancer: current status, challenges, and future directions. Adv Nutr (2015) 6:408–19. doi: 10.3945/an.114.008052
24. Rasheed S, Rehman K, Shahid M, Suhail S, Akash MSH. Therapeutic potentials of genistein: New insights and perspectives. J Food Biochem (2022) 46:e14228. doi: 10.1111/jfbc.14228
25. Kumar A, Malik JK, Arya K. In-silico analysis to access the antibacterial effect of genistein: molecular docking approach. EAS J Pharm Pharmacol (2019) 1:125–9. doi: 10.36349/easjpp.2019.v01i05.004
26. Banerjee S, Li Y, Wang Z, Sarkar FH. Multi-targeted therapy of cancer by genistein. Cancer Lett (2008) 269:226–42. doi: 10.1016/j.canlet.2008.03.052
27. Goh YX, Jalil J, Lam KW, Husain K, Premakumar CM. Genistein: a review on its anti-inflammatory properties. Front Pharmacol (2022) 13:820969. doi: 10.3389/fphar.2022.820969
28. Russo M, Russo GL, Daglia M, Kasi PD, Ravi S, Nabavi SF, et al. Understanding genistein in cancer: The “good” and the “bad” effects: A review. Food Chem (2016) 196:589–600. doi: 10.1016/j.foodchem.2015.09.085
29. Bhat SS, Prasad SK, Shivamallu C, Prasad KS, Syed A, Reddy P, et al. Genistein: a potent anti-breast cancer agent. Curr Issues Mol Biol (2021) 43:1502–17. doi: 10.3390/cimb43030106
30. Liu R, Yu X, Chen X, Zhong H, Liang C, Xu X, et al. Individual factors define the overall effects of dietary genistein exposure on breast cancer patients. Nutr Res (2019) 67:1–16. doi: 10.1016/j.nutres.2019.03.015
31. Lamartiniere CA, Zhang J-X, Cotroneo MS. Genistein studies in rats: potential for breast cancer prevention and reproductive and developmental toxicity. Am J Clin Nutr (1998) 68:1400S–5S. doi: 10.1093/ajcn/68.6.1400S
32. Abdullahi M, Adeniji SE. In-silico molecular docking and ADME/pharmacokinetic prediction studies of some novel carboxamide derivatives as anti-tubercular agents. Chem Afr (2020) 3:989–1000. doi: 10.1007/s42250-020-00162-3
33. Amin MR, Yasmin F, Dey S, Mahmud S, Saleh MA, Emran TB, et al. Methyl β-D-galactopyranoside esters as potential inhibitors for SARS-CoV-2 protease enzyme: synthesis, antimicrobial, PASS, molecular docking, molecular dynamics simulations and quantum computations. Glycoconjugate J (2021), 1–30. doi: 10.1007/s10719-021-10039-3
34. Poroikov VV, Filimonov DA, Ihlenfeldt W-D, Gloriozova TA, Lagunin AA, Borodina YV, et al. PASS biological activity spectrum predictions in the enhanced open NCI database browser. J Chem Inf Comput Sci (2003) 43:228–36. doi: 10.1021/ci020048r
35. Yasmin F, Amin MR, Hosen MA, Bulbul MZ, Dey S, Kawsar SM. Monosaccharide derivatives: synthesis, antimicrobial, pass, antiviral and molecular docking studies against SARS-COV-2 Mpro inhibitors. J Cellulose Chem Technol (2021) 55:477–99. doi: 10.35812/CelluloseChemTechnol.2021.55.44
36. Shankar U, Gogoi R, Sethi SK, Verma A. Introduction to Materials Studio Software for the Atomistic-Scale Simulations. In: Forcefields for Atomistic-Scale Simulations: Materials and Applications. Springer (2022). p. 299–313.
37. Hsiao Y, Su B-H, Tseng YJ. Current development of integrated web servers for preclinical safety and pharmacokinetics assessments in drug development. Briefings Bioinf (2021) 22:bbaa160. doi: 10.1093/bib/bbaa160
38. Pachiappan S, Arul Balasubramanian MG, RaMalingam K. Pharmacoinformatics based in silico Molecular Dynamics Simulation for Screening Phytochemicals as AMPK and INSR Modulators for Polycystic Ovarian Syndrome from Medicinal Plants.
39. Azzam KA. SwissADME and pkCSM webservers predictors: An integrated online platform for accurate and comprehensive predictions for in silico ADME/T properties of artemisinin and its derivatives. Kompleksnoe Ispolzovanie Mineralnogo Syra= Complex Use mineral Resour (2023) 325:14–21. doi: 10.31643/2023/6445.13
40. Mazumdar M, Fournier D, Zhu D-W, Cadot C, Poirier D, Lin S-X. Binary and ternary crystal structure analyses of a novel inhibitor with 17β-HSD type 1: a lead compound for breast cancer therapy. J.B.J (2009) 424:357–66.
41. Smith BD, Sanders JL, Porubsky PR, Lushington GH, Stout CD, Scott EE. Structure of the human lung cytochrome P450 2A13. J Biol Chem (2007) 282:17306–13. doi: 10.1074/jbc.M702361200
42. Kumer A, Khan MW. The effect of alkyl chain and electronegative atoms in anion on biological activity of anilinium carboxylate bioactive ionic liquids and computational approaches by DFT functional and molecular docking. Heliyon (2021), 7. doi: 10.1016/j.heliyon.2021.e07509
43. Herowati R, Widodo GP. Molecular Docking studies of chemical constituents of Tinospora cordifolia on glycogen phosphorylase. Proc Chem (2014) 13:63–8. doi: 10.1016/j.proche.2014.12.007
44. Pavan M, Menin S, Bassani D, Sturlese M, Moro S. Qualitative estimation of protein–ligand complex stability through thermal titration molecular dynamics simulations. J Chem Inf Model (2022) 62:5715–28. doi: 10.1021/acs.jcim.2c00995
45. Bradley RP. Molecular simulations of protein-induced membrane remodeling. University of Pennsylvania (2016).
46. Bowers KJ, Chow E, Xu H, Dror RO, Eastwood MP, Gregersen BA, et al. (2006). Scalable algorithms for molecular dynamics simulations on commodity clusters, in: Proceedings of the Proceedings of the 2006 ACM/IEEE Conference on Supercomputing, . pp. 84–es.
47. Saini G, Dalal V, Savita BK, Sharma N, Kumar P, Sharma AK. Molecular docking and dynamic approach to virtual screen inhibitors against Esbp of Candidatus Liberibacter asiaticus. J Mol Graphics Model (2019) 92:329–40. doi: 10.1016/j.jmgm.2019.08.012
48. Kwofie SK, Broni E, Teye J, Quansah E, Issah I, Wilson MD, et al. Pharmacoinformatics-based identification of potential bioactive compounds against Ebola virus protein VP24. Comput Biol Med (2019) 113:103414. doi: 10.1016/j.compbiomed.2019.103414
49. Islam S, Hosen MA, Ahmad S, ul Qamar MT, Dey S, Hasan I, et al. Synthesis, antimicrobial, anticancer activities, PASS prediction, molecular docking, molecular dynamics and pharmacokinetic studies of designed methyl α-D-glucopyranoside esters. J Mol Struct (2022) 1260:132761. doi: 10.1016/j.molstruc.2022.132761
50. Walters WP. Going further than Lipinski’s rule in drug design. Expert Opin Drug Discov (2012) 7:99–107. doi: 10.1517/17460441.2012.648612
51. Morris GM, Lim-Wilby M. Molecular docking. Mol Model Proteins (2008), 365–82. doi: 10.1007/978-1-59745-177-2_19
52. Latha MS, Saddala MS. Molecular docking based screening of a simulated HIF-1 protein model for potential inhibitors. Bioinformation (2017) 13:388. doi: 10.6026/97320630013388
53. Rahman MA, Matin MM, Kumer A, Chakma U, Rahman MR. Modified D-glucofuranoses as new black fungus protease inhibitors: Computational screening, docking, dynamics, and QSAR study. Phys Chem Res (2022) 10:195–209. doi: 10.22036/pcr.2021.294078.1934
54. Nath A, Kumer A, Zaben F, Khan M. Investigating the binding affinity, molecular dynamics, and ADMET properties of 2, 3-dihydrobenzofuran derivatives as an inhibitor of fungi, bacteria, and virus protein. Beni-Suef Univ J Basic Appl Sci (2021) 10:1–13. doi: 10.1186/s43088-021-00117-8
55. Benet LZ, Kroetz D, Sheiner L, Hardman J, Limbird L. Pharmacokinetics: the dynamics of drug absorption, distribution, metabolism, and elimination. Goodman Gilman’s Pharmacol basis Ther (1996) 3:e27. Available at: https://accessmedicine.mhmedical.com/content.aspx?bookid=2189§ionid=166182905.
56. Yadav R, Imran M, Dhamija P, Chaurasia DK, Handu S. Virtual screening, ADMET prediction and dynamics simulation of potential compounds targeting the main protease of SARS-CoV-2. J Biomol Struct Dyn (2021) 39:6617–32. doi: 10.1080/07391102.2020.1796812
57. Arefin A, Ema TI, Islam T, Hossen MS, Islam T, Al Azad S, et al. Target specificity of selective bioactive compounds in blocking α-dystroglycan receptor to suppress Lassa virus infection: an in silico approach. J Biomed Res (2021) 35:459. doi: 10.7555/JBR.35.20210111
58. Podder A, Pandit M, Narayanan L. Drug target prioritization for Alzheimer’s disease using protein interaction network analysis. OMICS: A J Integr Biol (2018) 22:665–77. doi: 10.1089/omi.2018.0131
59. Clark DE. What has polar surface area ever done for drug discovery? Future Med Chem (2011) 3:469–84. doi: 10.4155/fmc.11.1
60. Vincenzi M, Mercurio FA, Leone M. Looking for SARS-coV-2 therapeutics through computational approaches. Curr Med Chem (2023) 30:3158–214. doi: 10.2174/0929867329666221004104430
61. Lakhera S, Devlal K, Ghosh A, Chowdhury P, Rana M. Modelling the DFT structural and reactivity study of feverfew and evaluation of its potential antiviral activity against COVID-19 using molecular docking and MD simulations. Chem Pap (2022) 76:2759–76. doi: 10.1007/s11696-022-02067-6
62. Geballe MT. Part I: Structure and function in the NMDA ligand binding domain. Part II: Comparison of paclitaxel analogs through molecular dynamics simulation; solution conformations of cyclic peptides. Emory University (2009).
Keywords: drug design, genistein, breast cancer, lung cancer, Glycyrrhiza glabra, molecular docking, molecular dynamics simulation
Citation: Akash S, Bibi S, Biswas P, Mukerjee N, Khan DA, Hasan MN, Sultana NA, Hosen ME, Jardan YAB, Nafidi H-A and Bourhia M (2023) Revolutionizing anti-cancer drug discovery against breast cancer and lung cancer by modification of natural genistein: an advanced computational and drug design approach. Front. Oncol. 13:1228865. doi: 10.3389/fonc.2023.1228865
Received: 25 May 2023; Accepted: 15 August 2023;
Published: 25 September 2023.
Edited by:
Georgios D Lianos, University Hospital of Ioannina, GreeceReviewed by:
Nosheen Masood, Fatima Jinnah Women University, PakistanCopyright © 2023 Akash, Bibi, Biswas, Mukerjee, Khan, Hasan, Sultana, Hosen, Jardan, Nafidi and Bourhia. This is an open-access article distributed under the terms of the Creative Commons Attribution License (CC BY). The use, distribution or reproduction in other forums is permitted, provided the original author(s) and the copyright owner(s) are credited and that the original publication in this journal is cited, in accordance with accepted academic practice. No use, distribution or reproduction is permitted which does not comply with these terms.
*Correspondence: Shopnil Akash, c2hvcG5pbC5waEBnbWFpbC5jb20=; Shabana Bibi, c2hhYmFuYS5iaWJpLnN0bXVAZ21haWwuY29t; Mohammed Bourhia, Ym91cmhpYS5tb2hhbWVkQGdtYWlsLmNvbQ==
†These authors have contributed equally to this work
Disclaimer: All claims expressed in this article are solely those of the authors and do not necessarily represent those of their affiliated organizations, or those of the publisher, the editors and the reviewers. Any product that may be evaluated in this article or claim that may be made by its manufacturer is not guaranteed or endorsed by the publisher.
Research integrity at Frontiers
Learn more about the work of our research integrity team to safeguard the quality of each article we publish.