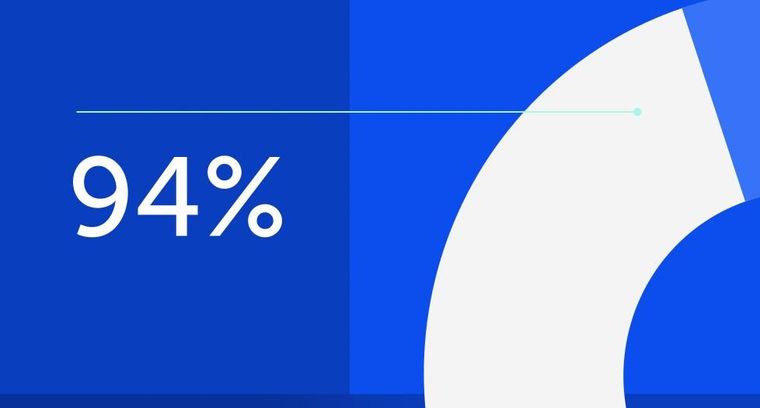
94% of researchers rate our articles as excellent or good
Learn more about the work of our research integrity team to safeguard the quality of each article we publish.
Find out more
ORIGINAL RESEARCH article
Front. Oncol., 20 July 2023
Sec. Thoracic Oncology
Volume 13 - 2023 | https://doi.org/10.3389/fonc.2023.1224455
This article is part of the Research TopicNovel Biomarkers for Potential Clinical Applications in Lung CancerView all 41 articles
Background: Preoperative prediction models for histologic subtype and grade of stage IA lung adenocarcinoma (LUAD) according to the update of the WHO Classification of Tumors of the Lung in 2021 and the 2020 new grade system are yet to be explored. We aim to develop the noninvasive pathology and grade evaluation approach for patients with stage IA LUAD via CT-based radiomics approach and evaluate their performance in clinical practice.
Methods: Chest CT scans were retrospectively collected from patients who were diagnosed with stage IA LUAD and underwent complete resection at two hospitals. A deep learning segmentation algorithm was first applied to assist lesion delineation. Expansion strategies such as bounding-box annotations were further applied. Radiomics features were then extracted and selected followed by radiomics modeling based on four classic machine learning algorithms for histologic subtype classification and grade stratification. The area under the receiver operating characteristic curve (AUC) was used to evaluate model performance.
Results: The study included 294 and 145 patients with stage IA LUAD from two hospitals for radiomics analysis, respectively. For classification of four histological subtypes, multilayer perceptron (MLP) algorithm presented no annotation strategy preference and achieved the average AUC of 0.855, 0.922, and 0.720 on internal, independent, and external test sets with 1-pixel expansion annotation. Bounding-box annotation strategy also enabled MLP an acceptable and stable accuracy among test sets. Meanwhile, logistic regression was selected for grade stratification and achieved the average AUC of 0.928, 0.837, and 0.748 on internal, independent, and external test sets with optimal annotation strategies.
Conclusions: DL-enhanced radiomics models had great potential to predict the fine histological subtypes and grades of early-stage LUADs based on CT images, which might serve as a promising noninvasive approach for the diagnosis and management of early LUADs.
Lung cancer remained the leading cause of cancer death worldwide with annually 2.1 million new lung cancer cases and 1.8 million deaths (1). Unfortunately, approximately 70% of these patients are diagnosed with locally advanced stages and metastatic disease, which results in low survival rates (2). Thus, early detection and treatment of lung cancer are essential to reduce mortality. With the widespread development of low-dose chest CT screening programs, the detection of ground-glass nodules (GGNs) is rapidly increasing. Early-stage lung adenocarcinomas (LUADs) often manifest as pure ground-glass nodules (GGNs) and part-solid nodules (PSNs), and the prognosis is significantly related to pathological subtypes of LUADs (3, 4). Sublobar resection (including wedge resection and segmentectomy) could be considered for some stage I non-small cell lung cancer (NSCLC) patients with pre-invasive adenocarcinoma (adenocarcinoma in situ, AIS), minimally invasive adenocarcinoma (MIA), or lepidic predominant adenocarcinoma, owing to its favorable prognosis (5). However, some subtypes (solid, micropapillary, and complex glandular) of LUADs often have a poor prognosis (6), indicating the necessity of lobectomy for these patients. Therefore, the accurate pre-judgment of pathological subtypes and gradings would benefit the selection of surgery type, prognosis, and personalized postoperative follow-up of stage I LUADs.
Currently, many radiomics models have been developed to classify main histologic subtypes of lung cancer, such as the differentiation of non-small cell lung cancer (NSCLC) and small cell lung cancers (SCLC) (7), the classification of lung adenocarcinomas (ADC) and squamous cell carcinomas (SCC) (8), the differentiation of ADC, SCC, and SCLC (9). Of note, studies on LUADs also focused on the histologic subtype classification, and most studies simplified the problem by dividing LUADs into a 2-category classification (IAC; non-IAC) according to their invasiveness (10). In addition to the invasiveness, subtypes indicative of poor prognoses, such as the invasive mucinous adenocarcinoma (IMA), are still rarely included in classification studies, especially for stage IA LUADs. Additionally, although some reports studied the identification of high-grade LUADs via radiomics, the systematic stratification of IAC grades according to the 2020 new grade system from the International Association for the Study of Lung Cancer (IASLC) Pathology Committee (6) was yet to be explored.
In this study, we focused on patients with stage IA LUADs and aimed to develop two consecutive radiomics models for their non-invasive histologic subtype classification and grade stratification. Of note, Deep learning (DL)-based pre-annotation strategy and expansion annotation strategies were utilized to study the influence of ROIs delineation on the performance of radiomics. In combination with multiple machine learning algorithms, stable radiomics models were selected based on their performance on internal, independent, and external testing sets and further underwent subgroup analysis, validating their potential in supporting the clinical decisions in the era of precise and personal medicine.
The retrospective study was approved by the Institutional Reviewing Board (IRB) of Beijing Haidian Hospital and Peking University People’s Hospital and the informed consent was waived by IRBs since patient information was anonymized to ensure privacy.
Patients who underwent chest surgery and were diagnosed with stage IA LUAD were enrolled from two medical centers for radiomics model development and external validation according to the following including and exclusion criteria. Three cohorts were eventually included from two hospitals and constitute three datasets, including development set, independent test set, and external test set.
The first cohort, comprising 236 patients treated at our institution between February 27, 2017, and May 7, 2021, included 180 primary lung cancer (PLC) patients with a single lesion and 56 multiple primary lung cancer (MPLC) patients. This dataset was used for radiomics development and was divided into training, validation, and internal testing subsets at a ratio of 16: 4: 5. The second cohort included 58 eligible patients treated between May 10, 2021, and Nov 3, 2021, and was used as an independent test set. Of note, to further evaluate the robustness and generalization of proposed radiomics models, 145 eligible patients who underwent treatment at the other hospital between Sep 15, 2016, and Nov 1, 2021, were enrolled in cohort 3 and served as the external test set. Diagrams of patient enrollment and data partition details can be found in Figure 1.
Figure 1 Diagram of patients enrollment and data partition. PLC = primary lung cancer, MPLC = multiple primary lung cancer, DICOM: Digital Imaging and Communications in Medicine.
The inclusion criteria were as follows: a) patients with stage IA lung adenocarcinoma; b) those who underwent complete surgical excision; c) those with preoperative thin-sliced chest CT images. Patients were excluded if a) histological subtype or clinical information was missing; b) their CT images were not in compliance with the Digital Imaging and Communications in Medicine (DICOM) standards; c) CT images were discontinuous, missing, or damaged; d) annotating radiologists could not confidently annotate images.
All the enrolled patients underwent chest CT examinations before surgical excision. Particularly, multi-slice spiral CT low-dose scans were performed using instruments from GE Healthcare (Chicago, Ill, USA), Philips Healthcare (Amsterdam, Netherlands), and United Imaging (Shanghai, China). The key scanning parameters were as follows: tube voltage of 120KV; reconstruction slice thickness from 0.625 to 2mm. All CT scans were saved in the picture archiving and communication system.
Given that deep learning (DL)-based auxiliary diagnosis systems for pulmonary nodules have been well developed and launched in clinical settings (11, 12), a modified Faster R-CNN model trained on more than 11,000 chest CT scans to detect different types of pulmonary nodules was utilized to aid the annotation of targeted nodules (12). Briefly, the employed modified Faster R-CNN first detected the targeted nodules and a U-Net segmentation algorithm output the contour. Then, senior radiologists further corrected the delineation of interested pulmonary nodules and deleted untargeted nodule lesions. In such a way, consumption of the medical labor force was significantly reduced, and the annotation efficiency was greatly improved. The credibility of the DL-based segmentation algorithm in annotating pulmonary nodules was examined by comparing it with manual-corrected lesion contours.
Previous studies revealed that peritumoral information could improve the model performance on invasiveness prediction of ADC (13) and histological subtype stratification in patients with NSCLC (14). Another previous radiomics studies reported that bounding-box delineation of ROI could achieve equivalent performance to precisely annotated ones (15). Considering the potential advantages of peritumoral areas in histologic classification tasks, in addition to the DL-aided manual-correction annotation strategy, we further explored the pixel-expansion annotation strategy for radiomics modeling by expanding lesion contours based on manual corrected ones. Particularly, we performed 1-pixel, 3-pixel, 5-pixel, and bounding-box expansions after the manual correction was completed. The representation of annotated lesions was presented in the Supplementary Figure 1. Summarily, we selected different ROIs in this study, encompassing the precise lesion ROI, the expanded ROI, and the bounding-box ROI of the designated lesions. To ensure the accurate localization of the targeted lesion on CT images, a multidisciplinary team consisting of radiologists, thoracic surgeons, and pathologists collaborated in defining the targeted lesions. The impact of different annotation strategies on stage IA LUAD histologic subtype classification and invasive non-mucinous adenocarcinoma (IAC) grade stratification was analyzed in this study by comparing the performance of radiomics models.
The PyRadiomics package (version 2.2.0) was called using Python (version 3.8.1) when performing radiomics feature extraction. Summarily, a total of 1454 features were extracted from the annotated ROIs, which belonged to 7 classes, including first-order (FOS), shape, Gray Level Co-occurrence Matrix (GLCM), Gray Level Run Length Matrix (GLRLM), Gray Level Size Zone Matrix (GLSZM), Neighbouring Gray Tone Difference Matrix (NGTDM), and Gray Level Dependence Matrix (GLDM) features. Detailed information on extracted features was summarized in supplementary table 1.
Pearson correlation coefficient (PCC) was first calculated and used to reduce the redundancy of the primary feature set, followed by the principal component analysis (PCA) approach which converted potentially correlated features into principal components that are linearly uncorrelated via orthogonal transformation (16). Features with a PCC <0.8 were retained after the first-round examination of feature redundancy. Subsequently, uncorrelated principal feature components were further obtained via PCA and utilized to develop radiomics models for histologic subtype classification and IAC grade stratification. Feature selection was accomplished by calling the scikit-learn (version 0.20.2) package.
Chest CT scans, pathological information, and clinical information was retrospectively collected from all included eligible patients and used to generate gold standard labels. Given the update of the WHO Classification of Tumors of the Lung in 2021 and IASLC grading system of IAC in 2020, histologic subtypes and IAC gradings of enrolled patients were all re-evaluated by an experienced pathologist before being utilized as the gold standard label in model development. In particular, histologic subtyping and grading were performed using the largest tumor sections in all cases, and the percentage of each histologic component was recorded in 5% increments according to the proposed IASLC grading system as follows: Grade 1, lepidic predominant tumors with no or less than 20% high-grade patterns (solid, micropapillary, and/or complex glandular patterns); Grade 2, acinar or papillary predominant tumors with no or less than 20% high-grade patterns; and Grade 3, any tumor with 20% or more of high-grade patterns.
Based on the five ROI annotation strategies mentioned above, four classic machine learning (ML) algorithms were utilized to develop radiomics models, including support vector machine (SVM), logistic regression (LR), and multi-layer perceptron (MLP), and eXtreme Gradient Boosting (XGBoost). The optimal hyper-parameters of ML algorithms were determined by the model performance on the validation set. The stable ML algorithm and potential practical annotation strategy were explored according to the model performance on the test datasets.
Radiomics models’ performance was evaluated by classification sensitivity, specificity, precision, accuracy, F1 score, G-Mean, and area under the ROC curve (AUC). According to the study design, the first batch radiomics models focused on the classification of stage IA LUAD histological subtype classification, including precursor glandular lesions (PGL), MIA, IAC, and IMA. The second batch radiomics models were responsible for the stratification of IAC grade (6), which ranged from grade 1 to grade 3 (Figure 2).
Figure 2 Illustration of the radiomics models for histologic subtype classification and IAC grading (1). Pre-operative chest CT scans were collected from enrolled patients for model development (2). Deep learning (DL)-based pulmonary nodule segmentation algorithm was utilized to pre-segment the target nodular lesions, followed by manual correction. Based on the manually edited region of interest (ROI), expansion strategies were applied to generate 1-pixel, 3-pixel, 5-pixel, and bounding-box masks of targeted lesions (3). PyRadiomics was utilized to extract radiomics features of different categories, including shape, intensity, wavelet, and texture features (4). Pearson correlation coefficient (PCC) and principal component analysis (PCA) were employed to reduce the dimensionality of extracted features (5). Classic machine learning (ML) algorithms were then used to develop radiomics models for classifying histologic subtypes of stage IA LUADs (6). Furthermore, ML algorithms were used to develop radiomics models for stratifying grades of invasive non-mucinous adenocarcinoma (IAC).
Continuous variables were represented by the means ± SD while the categorical variables were expressed in terms of frequency and statistically analyzed by the Chi-square test. P <0.05 was considered statistically significant. A two-sided 95% confidence interval for AUC was constructed following the approach of Hanley and McNeil (1982) (17). Cohen’s Kappa coefficient was calculated in a confusion matrix to measure the agreement between pathological gold-standard and model predictions. All statistical analyses were performed with the R statistical package (The R Foundation for Statistical Computing, Vienna, Austria).
From the two institutions, 256, 63, and 173 patients were initially eligible for the development set, independent test set, and external test set, respectively. However, due to missing histological subtype or clinical information, 20 (7.8%) and 3 (4.7%) patients were excluded. Additionally, 2 (3.2%) patients with motion-artifact induced poor quality CT scans and 28 (16.2%) patients with damaged CT scans were omitted. Thus, the final sample comprised 236, 58, and 145 patients in the development set, independent test set, and external test set (Figure 1).
In general, most of the included patients (79.04%, n=347) were non-smokers. Current (12.76%, n=56) and former smokers (8.20%, n=36) just count for a small portion of the studied population. Of note, 23.01% (n=101) of the population had a family history of cancer while 14.12% (n=62) of them had an alcohol intake history. The most frequent surgical procedure was lobectomy (38.95%, n=171), followed by segmentectomy (26.65%, n=117) and wedge resection (21.41%, n=94); the rest of included patients (12.98%, n=57) received hybrid surgical procedures due to the presence of multiple primary lung cancer lesions. At adenocarcinoma lesion level, most of them presented as PSNs (53.22%, n=322), followed by GGN (30.58%, n=185), solid nodule (12.07%, n=73), and mass (4.13%, n=25). With respect to histologic subtypes, IAC (57.85%, n=350), MIA (30.91%, n=187), PGL (7.44%, n=45), and IMA (3.80%, n=23) were included. Additionally, most IAC lesions (84.57%, n=296) were categorized as Grade 2 according to the latest released grading system by the IASLC Pathology Committee.
Detailed characteristics of the included population in different datasets was summarized in Table 1. Notably, patients in the external test set were significantly older than those in the development set. Furthermore, family history of cancer was significantly less common among patients in external test set. It is also worth noting that the distribution of nodule types by density, histologic subtypes, and IAC gradings significantly varied across datasets due to different data collection timeframes. Notably, the independent test set lacked PGL and IMA lesions.
A total of 1454 features were extracted from the annotated ROIs. A sum of 303 features with a Pearson correlation coefficient <0.8 was obtained after the first-round reduction of feature dimensionality. The correlation heatmap of selected features was presented in Supplementary Figure 2A. Subsequently, 40 principal feature components were preserved via PCA for the development of radiomics models. Principal component contribution rate was displayed in Supplementary Figure 2B. Details information about the extracted and selected features can be found in Supplementary Table 1.
Since PCA analysis selected feature components rather than certain features, we analyzed the significantly distinguished features (SDF) between each subtype based on PCC selected features in advance before developing the four-class histologic subtypes classification model and obtained 6 pairwise comparisons (PCs). Of the first-round selected 303 features, SDFs between each subtype were identified and grouped according to their identifying frequencies. Features were eventually divided into 7 groups, including SDFs in all PCs (n=46), 5PCs (n=19), 4PCs (n=17), 3PCs (n=17), 2PCs (n=16), 1PC (n=19), and none of the 6 PCs (n=169). These divided feature groups and their corresponding categories were displayed in the feature heatmap (Figure 3), and the details of features in each group were listed in Supplementary Table 2.
Figure 3 The most discriminative features for each histologic subtype. Based on PCC dimensionality reduction, distinguished features in a pair-wise comparison were analyzed to explain the potential key factors that distinguish them from each other. The detailed composition of each pair-wise comparison in each row is indicated in the right panel. Features were color-coded according to their category and listed from left to right based on their frequencies in pair-wise comparisons.
DL-based nodule segmentation algorithms have enhanced the practicality of radiomics models. In the current study, we further employed five annotation strategies and four ML algorithms to develop two batches of models for LUAD diagnosis, including histologic subtype classification and IAC grade stratification. We first selected the optimal ML algorithms for both tasks by comparing the models’ performance under different annotation strategies on three test sets. As depicted in Figures 4A–C, MLP with 1-pixel annotation exhibited optimal performance on histologic subtype classification on the internal test set, and maintained consistent and excellent performance on independent and external test sets, regardless of annotation strategies. Notably, the bounding-box annotation strategy yielded comparable results for histologic subtype classification on the independent and external sets. Concurrently, LR displayed an overall superior performance on IAC grade stratification in terms of accuracy (Figures 4D–F). However, the performance of LR varied with different annotation strategies for IAC grade stratification.
Figure 4 Impact of different annotation strategies on radiomics model performance. The performance of radiomics models developed on features from different annotation strategies were evaluated and compared in terms of accuracy. (A-C) displayed the accuracy of radiomics models for histologic subtype classification on the internal, independent, and external testing sets, respectively. (D–F) demonstrated the accuracy of radiomics models for IAC grade stratification on the internal, independent, and external testing sets.
Subsequently, impacts of annotations on selected ML algorithms were further evaluated on three test sets in terms of AUC, sensitivity, specificity, precision, F1-score, and G-Mean (Supplementary Figure 3). It was observed that MLP for histologic subtype classification had no preference for a specific annotation strategy, while LR for IAC grade stratification showed a preference for certain data labeling strategies. Regarding the performance of the radiomics models on each class, we noted inferior results for those classes with insufficient sample sizes.
We first evaluated the performance of the radiomics model on histologic subtype classification. The MLP with 1-pixel expansion was selected as the representative model. This model achieved an AUC of 0.903, 0.905, 0.951, and 0.661 for PGL, MIA, IAC, and IMA lesions, respectively, on the internal test set. On the external test set, it achieved an AUC of 0.929 and 0.914 for MIA and IAC lesions. On the external test set, it achieved an AUC of 0.691, 0.841,0.747, and 0.600 for PGL, MIA, IAC, and IMA lesions, respectively (Figures 5A–C). Notably, the performance of MLP was compromised on the external test set. Meanwhile, the kappa coefficient of MLP reached 0.696, 0.534, and 0.473, which presented a substantial and moderate agreement between model-predicted histologic subtypes and ground truth (Figures 5D–F). A decrease in the accuracy of MLP was also observed among the internal, independent, and external test sets (Table 2). This discrepancy could potentially be attributed to the prevalence of challenging GGN lesions in the independent set and MPLC lesions in the external set. Of note, the accuracy of MLP remained stable on the external test sets (0.714 vs. 0.763 vs. 0.756) when the bounding-box annotation strategy was applied. The detailed performance metrics were summarized in Table 2.
Figure 5 Performance of radiomics models on histologic subtype classification and IAC grading stratification. For histologic subtype classification, ROC curves were plotted to evaluate the performance of the Radiomic model in discriminating PGL, MIA, IAC, and IMA from the other three categories on internal (A), independent (B), and external (C) testing sets, respectively. Confusion matrices for four-category classification of PGL, MIA, IAC, and IM on internal (D), independent (E), and external (F) testing sets, respectively. For IAC grading stratification, ROC curves were plotted to evaluate the performance of the Radiomic model on internal (G), independent (H), and external (I) testing sets, respectively. Confusion matrices for the stratification of IAC grades (grade 1 to 3) on internal (J), independent (K), and external (L) testing sets, respectively. The exact number of true positives, false positives, true negatives and false negatives were listed. Kappa coefficients were calculated.
Table 2 Detailed diagnostic metrics of radiomics models on internal, independent, and external test datasets.
We next evaluated the performance of the selected LR with optimal annotation strategies for IAC grade stratification. The LR model achieved an AUC of 0.911, 0.873, and 1.000 for grade 1, grade 2, and grade 3, respectively, on the internal testing set (Figure 5G), with a corresponding kappa coefficient of 0.547 (Figure 5J). However, on the independent test set, the LR model yielded a lower AUC of 0.771, 0.740, and 1.000 for grade 1, grade 2, and grade 3 respectively, and on the external test set, an AUC of 0.772, 0.644, and 0.878 for grade 1, grade 2, and grade 3, respectively. This suboptimal performance could be attributed to the imbalanced in sample size across the different grades (Figures 5H, I). The kappa coefficients of the LR model on the independent and external sets were 0.562 and 0.169, respectively (Figures 5K, L). Detailed performance metrics were summarized in Table 2.
Notably, subgroup analyses of lesion numbers (PLC Vs MPLC), sex, nodule types by density (GGN vs PSNs vs solid), and age range were further performed (Figure 6). For histologic subtype classification, lower accuracy of MLP were observed on MPLC patients, significantly lower level was found on external test sets. Besides, significantly lower accuracy of MLP was also seen in GGN lesions on independent and external test sets. For IAC grade stratification, LR displayed significantly lower accuracy on male patients and solid nodules on the external test set. No significant difference of both two models was observed among other subgroups.
Figure 6 Subgroup analysis of selected representative ML model performance on test sets. Subgroup analyses were performed on histologic subtype classification and IAC grade stratification on internal, independent, and external testing sets, including target lesion numbers per patient, sex, nodule types, and age periods.
Non-invasive preoperative prediction of pathological subtype and grade would greatly benefit the patients with stage IA LUADs in terms of the selection of surgery type, prognosis, and personalized postoperative follow-up. In this current study, we proposed two consecutive radiomics models for the diagnosis of patients with LUADs, including histologic subtype classification (PGL, MIA, IAC, and IMA) and IAC grade stratification (grade 1-3). Five annotation strategies and four ML algorithms were utilized for modeling. MLP and LR were selected as the optimal algorithms for histologic subtype classification and IAC grading stratification tasks, respectively, as supported by the overall better performance on different annotations on internal, independent, and external test sets. For histologic subtype classification, bounding-box annotation enabled an equivalent performance of MLP. Besides, distinguishing features between each pairwise comparison were revealed. Additionally, subgroup analyses validated the applicability of the radiomics models across cohorts with different sex, ages, and number of lesions.
Radiomics has been used since 2014 to solve clinical problems (18), and as its applications expand, efforts to streamline the process for clinical implementation are ongoing. Lesion annotation is often time-consuming and labor-intensive, limiting the clinical deployment of radiomics tools. Previous studies (19, 20) reported that semiautomatic lesion segmentation exhibited high agreement with manual delineations and could provide a significant reduction in interobserver variability. Some other studies utilized certain whole CT images (21), certain annotated slides (22), or bounding-box annotation (15) to develop models which could also avoid heavy annotation workload but might result in insufficient features. Given that DL segmentation algorithms for pulmonary nodules were well trained (11, 12), we then employed one to pre-segment the targeted lesions followed by a manual edition. The employed DL algorithm achieved an averaged Dice index of 0.94 (compared with manually edited contours), indicating the potential of the end-to-end or enhanced radiomics models by integrating DL segmentation algorithms into the classic radiomics modeling pathway. However, unlike the DL-enable end-to-end radiomics model in differentiating COVID-19 (22), we enrolled MPLC patients with other untargeted nodules that needed to be manually excluded before developing radiomics models. After all, as previously reported (23, 24), our hybrid approach avoided intensive labor force for lesion annotation.
Since the easy-to-use bounding box annotation strategy was proved to be efficient in developing radiomics models for the diagnoses of gastric cancer and breast lesions (15, 25), we also examined the efficiency of an expansion strategy for the LUADs related tasks in our study by generating 1, 3, 5-pixel expanded and bounding-box (based on 5-pixel expansion) annotations. Notably, the 1-pixel expansion strategy, to some extent, enabled an overall stable performance of selected ML algorithms. An expansion strategy on cancerous lesions seemed to be a good option to enhance the model performance possibly by including more peritumoral features. Of course, the degree of expansion will need to be determined according to the situation. For histologic subtype classification, although the 1-pixel expansion strategy enabled an overall better performance, we also noticed the accuracy decline of MLP algorithm from internal to external test sets. Of note, accuracy of MLP remained acceptable and stable among test sets when applying the bounding-box strategy, indicating the practicality of the bounding-box strategy in this histologic subtype classification task. In contrast, the bounding-box strategy didn’t perform well on the three-grade classification tasks in this study, indicating its applicability is algorithm- and context-dependent.
Another essential procedure for radiomics is dimensionality reduction which plays a key role in alleviating ML artifacts in the scenario of unbalanced datasets with small sample sizes (26). We utilized two classic approaches, PCC and PCA, to perform the dimensionality reduction in this study (27, 28). As an unsupervised method, PCA projected features into a dimensionally reduced set of uncorrelated variables called principal components via the linear orthogonal transformation, and outperformed the supervised technique in terms of generalizability capability (26). However, to solve the main problem of the variable’s interpretation loss, we analyzed the distinguished features in a pair-wise comparison after PCC-based dimensionality reduction. The significant discriminating features between pair-wise comparisons may explain, to some extent, the key factors that distinguish them from each other.
Most previous related radiomics studies focused on binary classification in distinguishing NSCLC from SCLC, ADC from SCC, and IAC from other less invasive LUADs (7, 8). Given the update of the WHO Classification of Tumors of the Lung in 2021 and IASLC grading system of IAC in 2020 and the unique manifestations of IMA, we developed the first radiomics models for identifying four-category subtypes (PGL, IMA, IAC, and IMA) and three-category grades (grade1 to 3). We employed 4 classic ML algorithms and found that MLP and LR displayed an overall stable performance for four-category subtypes and three-category grades tasks, respectively. With the respect to identifying multi-class histologic subtypes, the selected representative MLP model in the current study achieved an average AUC of 0.855 and 0.922 on internal and independent testing sets, outperforming other models with an average AUC of 0.747 (4-category of NSCLC) (29), 0.833 (3-category subtypes of central lung cancer) (9), and 0.896 (4-category subtype of AAH, AIS, MIA, and IA) (30) in previous studies. Notably, the multiclass histological subtype classification model was not externally tested in previous studies, whereas the MLP achieved a mean AUC of 0.720 on external test set in this study. Meanwhile, few studies have reported the radiomics approach to stratify IAC grades according to the newly updated grading system. Instead, the radiomics approach was used to predict the micropapillary pattern that was reported to have a poor prognosis in a previous study (31). In comparison to multiparametric MRI-based radiomics approach for NSCLC grading (AUC 0.767) and contrast-enhanced CT-based radiomics signature for prediction of tumor differentiation degree (low and high degree, AUC 0.782) (32, 33), the selected representative LR algorithm for IAC grade stratification in this study achieved better performance on both internal and independent testing sets (averaged AUC 0.928 and 0.837) and equivalent performance on external test set (averaged AUC 0.748), indicating the potential of CT-based radiomics approach in predicting histologic grades of IAC. Meanwhile, we noticed a dramatically decreased Kappa coefficient of LR algorithm on external test set, which caused by the miss classifications of grade1 and 3 into grade 2, suggesting the need of further improvement for IAC grading stratification algorithms by including more balanced data.
Of note, a previous study performed radiogenomic analyses of patients with stage I LUAD by an unsupervised consensus clustering approach to better classify patients with different prognoses, complementing the TNM system (34). In consistent, we developed supervised radiomic models on the patients with stage IA LUAD (not including IB) to enable the accurate differentiation of patients with poor prognosis at early stages according to histologic subtypes. To address the heterogeneity of LUAD, we further included the histologic type of IMA in the proposed model. IMA has different characteristics than non-mucinous adenocarcinoma in terms of histology, radiological and clinical features. Although IMA can show a lepidic growth pattern, invasive patterns are always present. Several studies have shown that IMA has a poor prognosis than non-mucinous adenocarcinoma (35–37). Additionally, IMA is commonly detected in the advanced stage and cannot be surgically treated. Therefore, our proposed radiomics models, to some extent, aided the accurate pre-judgment of patients’ prognoses. Furthermore, they validated the revealed associations between CT-based radiomic features and known prognostic histologic factors, genomic drivers, and patient outcomes in the solid-type subgroup. In our subgroup analysis, the accuracy for differentiating histologic subtypes between GGN and PSNs lesions on both independent and external test sets were found to be significantly different.
There are some limitations to our study. The imbalance in histologic subtypes in the dataset compromised the performance of our proposed classification models, especially for PGL and IMA subtypes, and grade 3 lesions, which were less common in patients with operable clinical stage IA lung adenocarcinoma in clinical practice. The short follow-up of enrolled patients limited our ability to investigate the associations between radiomics and clinical features and the prognosis of patients with clinical stage IA LUAD. Although it is difficult for doctors to precisely classify those subtypes and grades, future work is also necessary to reveal the auxiliary effect of both models in promoting the diagnostic capabilities of these histologic subtypes, especially the identification of IMA, and IAC grades.
Despite these limitations, our results suggest that radiomics model, represented by MLP and LR, have great potential to predict the fine histological subtypes and grades of early-stage LUADs based on CT images, potentially providing a promising noninvasive approach for the diagnosis and management of early-stage LUADs.
The raw data supporting the conclusions of this article will be made available by the authors, without undue reservation.
The studies involving human participants were reviewed and approved by The Institutional Reviewing Board of Beijing Haidian Hospital and Peking University People’s Hospital. Written informed consent for participation was not required for this study in accordance with the national legislation and the institutional requirements.
YH designed the study and controlled the data used in this study. GP, KS, YY, QL, and SW participated in the collection of patients’ data and manual correction of ROIs and provided clinical expertise. DW, WT, YS, and SY were responsible for modeling and testing. KS was responsible for quality control of the pathological samples. GP and DW prepared the main manuscript text. YH further polished the manuscript. All authors reviewed the manuscript. All authors contributed to the article and approved the submitted version.
This work was supported by Youth Foundation of Beijing Haidian Hospital (Grant No. KYQ2021002).
The abstract (Abstract Title: Classification of Stage IA Lung Adenocarcinoma into Histologic Subtypes on Computed Tomography Images Using Radiomics and Abstract Title: Grade stratification of stage IA invasive pulmonary adenocarcinoma on computed tomography images using radiomics) related to the content of this manuscript was presented as an e-poster at the Society of Thoracic Surgeons (STS) 2022 and presented as an EPOS Radiologist (educational) at the European Congress of Radiology (ECR) 2023 Annual Meeting, respectively.
Authors DW, WT, YS, and SY are employees of the company Infervision Medical Technology Co. Ltd.
The remaining authors declare that the research was conducted in the absence of any commercial or financial relationships that could be construed as a potential conflict of interest.
All claims expressed in this article are solely those of the authors and do not necessarily represent those of their affiliated organizations, or those of the publisher, the editors and the reviewers. Any product that may be evaluated in this article, or claim that may be made by its manufacturer, is not guaranteed or endorsed by the publisher.
The Supplementary Material for this article can be found online at: https://www.frontiersin.org/articles/10.3389/fonc.2023.1224455/full#supplementary-material
NSCLC, non-small cell lung cancer; GGNs, ground-glass nodules; LUAD, lung adenocarcinoma; IAC, invasive non-mucinous adenocarcinoma; AUC, area under the curve; DL, deep learning; ML, machine learning; LR, logistic regression; PCC, Pearson correlation coefficient; PCA, principal component analysis.
1. Sung H, Ferlay J, Siegel RL, Laversanne M, Soerjomataram I, Jemal A, et al. Global cancer statistics 2020: GLOBOCAN estimates of incidence and mortality worldwide for 36 cancers in 185 countries. CA Cancer J Clin (2021) 71(3):209–49. doi: 10.3322/caac.21660
2. Arriagada R, Dunant A, Pignon JP, Bergman B, Chabowski M, Grunenwald D, et al. Long-term results of the international adjuvant lung cancer trial evaluating adjuvant cisplatin-based chemotherapy in resected lung cancer. J Clin Oncol (2010) 28(1):35–42. doi: 10.1200/JCO.2009.23.2272
3. Wang Y, Zheng D, Luo J, Zhang J, Pompili C, Ujiie H, et al. Risk stratification model for patients with stage I invasive lung adenocarcinoma based on clinical and pathological predictors. Transl Lung Cancer Res (2021) 10(5):2205–17. doi: 10.21037/tlcr-21-393
4. Yotsukura M, Asamura H, Motoi N, Kashima J, Yoshida Y, Nakagawa K, et al. Long-term prognosis of patients with resected adenocarcinoma In situ and minimally invasive adenocarcinoma of the lung. J Thorac Oncol (2021) 16(8):1312–20. doi: 10.1016/j.jtho.2021.04.007
5. Ito H, Suzuki K, Mizutani T, Aokage K, Wakabayashi M, Fukuda H, et al. Long-term survival outcome after lobectomy in patients with clinical T1 N0 lung cancer. J Thorac Cardiovasc Surg (2020) 11:S0022-5223(20)30054-4. doi: 10.1016/j.jtcvs.2019.12.072
6. Moreira AL, Ocampo PSS, Xia Y, Zhong H, Russell PA, Minami Y, et al. A grading system for invasive pulmonary adenocarcinoma: a proposal from the international association for the study of lung cancer pathology committee. J Thorac Oncol (2020) 15(10):1599–610. doi: 10.1016/j.jtho.2020.06.001
7. E L, Lu L, Li L, Yang H, Schwartz LH, Zhao B. Radiomics for classification of lung cancer histological subtypes based on nonenhanced computed tomography. Acad Radiol (2019) 26(9):1245–52. doi: 10.1016/j.acra.2018.10.013
8. Han Y, Ma Y, Wu Z, Zhang F, Zheng D, Liu X, et al. Histologic subtype classification of non-small cell lung cancer using PET/CT images. Eur J Nucl Med Mol Imaging (2021) 48(2):350–60. doi: 10.1007/s00259-020-04771-5
9. Li H, Gao L, Ma H, Arefan D, He J, Wang J, et al. Radiomics-based features for prediction of histological subtypes in central lung cancer. Front Oncol (2021) 11:658887. doi: 10.3389/fonc.2021.658887
10. Shi L, Shi W, Peng X, Zhan Y, Zhou L, Wang Y, et al. Development and validation a nomogram incorporating CT radiomics signatures and radiological features for differentiating invasive adenocarcinoma from adenocarcinoma In situ and minimally invasive adenocarcinoma presenting as ground-glass nodules measuring 5-10mm in diameter. Front Oncol (2021) 11:618677. doi: 10.3389/fonc.2021.618677
11. Wang Y, Yan F, Lu X, Zheng G, Zhang X, Wang C, et al. IILS: intelligent imaging layout system for automatic imaging report standardization and intra-interdisciplinary clinical workflow optimization. EBioMedicine (2019) 44:162–81. doi: 10.1016/j.ebiom.2019.05.040
12. Liu K, Li Q, Ma J, Zhou Z, Sun M, Deng Y, et al. Evaluating a fully automated pulmonary nodule detection approach and its impact on radiologist performance. Radiol Artif Intell (2019) 1(3):e180084. doi: 10.1148/ryai.2019180084
13. Wang X, Chen K, Wang W, Li Q, Liu K, Li Q, et al. Can peritumoral regions increase the efficiency of machine-learning prediction of pathological invasiveness in lung adenocarcinoma manifesting as ground-glass nodules? J Thorac Dis (2021) 13(3):1327–37. doi: 10.21037/jtd-20-2981
14. Tang X, Huang H, Du P, Wang L, Yin H, Xu X. Intratumoral and peritumoral CT-based radiomics strategy reveals distinct subtypes of non-small-cell lung cancer. J Cancer Res Clin Oncol (2022) 148(9):2247–60. doi: 10.1007/s00432-022-04015-z
15. Liu D, Zhang W, Hu F, Wang L, Yin H, Xu X. A bounding box-based radiomics model for detecting occult peritoneal metastasis in advanced gastric cancer: a multicenter study. Front Oncol (2021) 11:777760. doi: 10.3389/fonc.2021.777760
16. Zhao Z, Xiao D, Nie C, Zhang H, Jiang X, Jecha AR, et al. Development of a nomogram based on preoperative bi-parametric MRI and blood indices for the differentiation between cystic-solid pituitary adenoma and craniopharyngioma. Front Oncol (2021) 11:709321. doi: 10.3389/fonc.2021.709321
17. Hanley JA, McNeil BJ. The meaning and use of the area under a receiver operating characteristic (ROC) curve. Radiology (1982) 143(1):29–36. doi: 10.1148/radiology.143.1.7063747
18. Aerts HJ, Velazquez ER, Leijenaar RT, Parmar C, Grossmann P, Carvalho S, et al. Decoding tumour phenotype by noninvasive imaging using a quantitative radiomics approach. Nat Commun (2014) 5:4006. doi: 10.1038/ncomms5006
19. Heye T, Merkle EM, Reiner CS, Davenport MS, Horvath JJ, Feuerlein S, et al. Reproducibility of dynamic contrast-enhanced MR imaging. part II. comparison of intra- and interobserver variability with manual region of interest placement versus semiautomatic lesion segmentation and histogram analysis. Radiology (2013) 266(3):812–21.
20. Rios Velazquez E, Aerts HJ, Gu Y, Goldgof DB, De Ruysscher D, Dekker A. A semiautomatic CT-based ensemble segmentation of lung tumors: comparison with oncologists' delineations and with the surgical specimen. Radiother Oncol (2012) 105(2):167–73. doi: 10.1016/j.radonc.2012.09.023
21. Wu X, Hui H, Niu M, Li L, Wang L, He B, et al. Deep learning-based multi-view fusion model for screening 2019 novel coronavirus pneumonia: a multicentre study. Eur J Radiol (2020) 128:109041. doi: 10.1016/j.ejrad.2020.109041
22. Zhang X, Wang D, Shao J, Tian S, Tan W, Ma Y, et al. A deep learning integrated radiomics model for identification of coronavirus disease 2019 using computed tomography. Sci Rep (2021) 11(1):3938. doi: 10.1038/s41598-021-83237-6
23. Zhang B, Ni-Jia-Ti MY, Yan R, An N, Chen L, Liu S, et al. CT-based radiomics for predicting the rapid progression of coronavirus disease 2019 (COVID-19) pneumonia lesions. Br J Radiol (2021) 94(1122):20201007. doi: 10.1259/bjr.20201007
24. Zhang M, Zeng X, Huang C, Liu J, Liu X, Xie X, et al. An AI-based radiomics nomogram for disease prognosis in patients with COVID-19 pneumonia using initial CT images and clinical indicators. Int J Med Inform (2021) 154:104545. doi: 10.1016/j.ijmedinf.2021.104545
25. Zhou J, Zhang Y, Chang KT, Lee KE, Wang O, Li J, et al. Diagnosis of benign and malignant breast lesions on DCE-MRI by using radiomics and deep learning with consideration of peritumor tissue. J Magn Reson Imaging (2020) 51(3):798–809. doi: 10.1002/jmri.26981
26. Ligero M, Torres G, Sanchez C, Diaz-Chito K, Perez R, Gil D. Selection of radiomics features based on their reproducibility. Annu Int Conf IEEE Eng Med Biol Soc (2019) 2019:403–8. doi: 10.1109/EMBC.2019.8857879
27. Chen C, Qin Y, Cheng J, Gao F, Zhou X. Texture analysis of fat-suppressed T2-weighted magnetic resonance imaging and use of machine learning to discriminate nasal and paranasal sinus small round malignant cell tumors. Front Oncol (2021) 11:701289. doi: 10.3389/fonc.2021.701289
28. Li Z, Zhang J, Song Y, Yin X, Chen A, Tang N, et al. Utilization of radiomics to predict long-term outcome of magnetic resonance-guided focused ultrasound ablation therapy in adenomyosis. Eur Radiol (2021) 31(1):392–402. doi: 10.1007/s00330-020-07076-1
29. Khodabakhshi Z, Mostafaei S, Arabi H, Oveisi M, Shiri I, Zaidi H. Non-small cell lung carcinoma histopathological subtype phenotyping using high-dimensional multinomial multiclass CT radiomics signature. Comput Biol Med (2021) 136:104752. doi: 10.1016/j.compbiomed.2021.104752
30. Deng J, Zhao M, Li Q, Zhang Y, Ma M, Li C, et al. Implementation of artificial intelligence in the histological assessment of pulmonary subsolid nodules. Transl Lung Cancer Res (2021) 10(12):4574–86. doi: 10.21037/tlcr-21-971
31. Song SH, Park H, Lee G, Lee HY, Sohn I, Kim HS, et al. Imaging phenotyping using radiomics to predict micropapillary pattern within lung adenocarcinoma. J Thorac Oncol (2017) 12(4):624–32. doi: 10.1016/j.jtho.2016.11.2230
32. Tang X, Bai G, Wang H, Guo F, Yin H. Elaboration of multiparametric MRI-based radiomics signature for the preoperative quantitative identification of the histological grade in patients with non-Small-Cell lung cancer. J Magn Reson Imaging (2022) 56(2):579–89. doi: 10.1002/jmri.28051
33. Chen X, Fang M, Dong D, Wei X, Liu L, Xu X, et al. A radiomics signature in preoperative predicting degree of tumor differentiation in patients with non-small cell lung cancer. Acad Radiol (2018) 25(12):1548–55. doi: 10.1016/j.acra.2018.02.019
34. Perez-Johnston R, Araujo-Filho JA, Connolly JG, Caso R, Whiting K, Tan KS, et al. CT-based radiogenomic analysis of clinical stage I lung adenocarcinoma with histopathologic features and oncologic outcomes. Radiology (2022) 303(3):664–72. doi: 10.1148/radiol.211582
35. Lee HY, Cha MJ, Lee KS, Lee HY, Kwon OJ, Choi JY, et al. Prognosis in resected invasive mucinous adenocarcinomas of the lung: related factors and comparison with resected nonmucinous adenocarcinomas. J Thorac Oncol (2016) 11:1064–73. doi: 10.1016/j.jtho.2016.03.011
36. Motono N, Matsui T, Machida Y, Usuda K, Uramoto H. Prognostic significance of histologic subtype in pStage I lung adenocarcinoma. Med Oncol (2017) 34:100. doi: 10.1007/s12032-017-0962-x
37. Kadota K, Nitadori J-I, Sima CS, Ujiie H, Rizk NP, Jones DR, et al. Tumor spread through air spaces is an important pattern of invasion and impacts the frequency and location of recurrences after limited resection for small stage I lung adenocarcinomas. J Thorac Oncol (2015) 10:806–14. doi: 10.1097/JTO.0000000000000486
Keywords: deep learning, artificial intelligence, radiomics, model, lung adenocarcinoma
Citation: Pei G, Wang D, Sun K, Yang Y, Tang W, Sun Y, Yin S, Liu Q, Wang S and Huang Y (2023) Deep learning-enhanced radiomics for histologic classification and grade stratification of stage IA lung adenocarcinoma: a multicenter study. Front. Oncol. 13:1224455. doi: 10.3389/fonc.2023.1224455
Received: 17 May 2023; Accepted: 03 July 2023;
Published: 20 July 2023.
Edited by:
Hongda Liu, Nanjing Medical University, ChinaReviewed by:
Mang Yu, Stanford University, United StatesCopyright © 2023 Pei, Wang, Sun, Yang, Tang, Sun, Yin, Liu, Wang and Huang. This is an open-access article distributed under the terms of the Creative Commons Attribution License (CC BY). The use, distribution or reproduction in other forums is permitted, provided the original author(s) and the copyright owner(s) are credited and that the original publication in this journal is cited, in accordance with accepted academic practice. No use, distribution or reproduction is permitted which does not comply with these terms.
*Correspondence: Yuqing Huang, aHVhbmd5dXFpbmc1NTVAZ21haWwuY29t
†These authors have contributed equally to this work and share first authorship
Disclaimer: All claims expressed in this article are solely those of the authors and do not necessarily represent those of their affiliated organizations, or those of the publisher, the editors and the reviewers. Any product that may be evaluated in this article or claim that may be made by its manufacturer is not guaranteed or endorsed by the publisher.
Research integrity at Frontiers
Learn more about the work of our research integrity team to safeguard the quality of each article we publish.