- 1Victor Phillip Dahdaleh Institute of Genomic Medicine and Department of Human Genetics, McGill University, Montreal, QC, Canada
- 2Alberta Children’s Hospital Research Institute, Arnie Charbonneau Cancer Institute, and Department of Biochemistry and Molecular Biology, Cumming School of Medicine, University of Calgary, Calgary, AB, Canada
- 3Department of Pathology and Center for Cancer Research, Massachusetts General Hospital and Harvard Medical School, Boston, MA, United States
- 4Broad Institute of Harvard and Massachusetts Institute of Technology (MIT), Cambridge, MA, United States
- 5BioBox Analytics Inc., Toronto, ON, Canada
- 6Department of Human Genetics, McGill University, Montreal, QC, Canada
- 7The Research Institute of the McGill University Health Centre, Montreal, QC, Canada
- 8Department of Pediatrics, McGill University, Montreal, QC, Canada
- 9Division of Neurosurgery, The Arthur and Sonia Labatt Brain Tumour Research Centre and the Developmental and Stem Cell Biology Program, The Hospital for Sick Children, Toronto, ON, Canada
- 10Texas Children’s Cancer Center , Hematology-Oncology Section and Department of Pediatrics – Hematology/Oncology and Neurosurgery, Baylor College of Medicine, Houston, TX, United States
Introduction: Medulloblastoma is the most common type of malignant pediatric brain tumor with group 4 medulloblastomas (G4 MBs) accounting for 40% of cases. However, the molecular mechanisms that underlie this subgroup are still poorly understood. Point mutations are detected in a large number of genes at low incidence per gene while the detection of complex structural variants in recurrently affected genes typically requires the application of long-read technologies.
Methods: Here, we applied linked-read sequencing, which combines the long-range genome information of long-read sequencing with the high base pair accuracy of short read sequencing and very low sample input requirements.
Results: We demonstrate the detection of complex structural variants and point mutations in these tumors, and, for the first time, the detection of extrachromosomal DNA (ecDNA) with linked-reads. We provide further evidence for the high heterogeneity of somatic mutations in G4 MBs and add new complex events associated with it.
Discussion: We detected several enhancer-hijacking events, an ecDNA containing the MYCN gene, and rare structural rearrangements, such a chromothripsis in a G4 medulloblastoma, chromoplexy involving 8 different chromosomes, a TERT gene rearrangement, and a PRDM6 duplication.
1 Introduction
Medulloblastoma (MB) is the most common malignant pediatric brain tumor with an incidence of 0.16-0.53 per 100,000 population, with children 0-9 years having the highest incidence (1). MBs are split into four molecularly distinct subgroups each with their own prognosis, expression, epigenetic, and mutational profiles (2). The groups are wingless medulloblastomas (WNT-MB), sonic-hedgehog medulloblastomas (SHH-MB), Group 3 medulloblastomas (G3-MB), and Group 4 medulloblastomas (G4-MB). In children, WNT-MB have the best prognosis of any MB subtype (3). They are characterized by activation of the WNT pathway mainly by means of mutations in CTNNB1 and a recurrent complete or partial monosomy of chromosome 6 (4, 5; 3). A subset of WNT-MBs are caused by germline APC mutations which causes a predisposition to MB (3). SHH-MBs are characterized by the activation of the SHH pathway with the most commonly affected genes being PTCH1, SUFU, SMO, GLI1, GLI2 and MYCN (6) as well as mutations in the TERT promoter (7), TP53 and PTEN (8). Recently, a non-coding mutation in the U1 spliceosomal small nuclear RNAs (snRNAs) which was found to occur in 50% of SHH-MBs and leads to the inactivation of PTCH1 and activation of GLI1 and GLI2 (9).
Until recently, the molecular mechanisms that differentiated group 3 and group 4 medulloblastomas were poorly understood since many genes were mutated in both subtypes (2, 3). In G4-MBs in particular, recurrent mutations were detected in a plethora of different driver genes but only in a small subset of tumors (2, 3). However, recent work by Hendrikse et al. has shown that most of the genes mutated in G4-MBs are either part of or interact with the core binding factor alpha (CBFA) complex which they suggest is required for the normal development of the rhombic lip (RL) into the ventricular zone (VZ) and sub-ventricular zone (SVZ) (10). These genes include CBFA2T2, CBFA2T3, RUNX1T1, KDM6A, and KDM2B, which are typically mutated or deleted, and GFI1, GFI1B, PRDM6, and OTX2, which are recurrently overexpressed. Three of the upregulated genes are affected by structural variants (such as deletions, duplications and inversions) that lead to the enhancer hijacking and overexpression of GFI1, GFI1B and PRDM6 (via SNCAIP amplification) (11, 12).
Gain of the 17q and loss of 17p (termed isochromosome 17q) is also recurrently found in both G3-MBs and G4-MBs (13) as well as loss of chromosomes 8, 11p and X, and gain of chromosomes 7 and 18q in G4s (14). Additionally, MYCN is found amplified in 5-6% of G3 and G4 tumours while MYC amplification are found exclusively in G3 tumors (about 17% of cases) (12). TERT mutations are also found in all MB subtypes with the exception of WNT-MB although they occur at the highest rate in SHH-MBs (12). Oncogene amplification occurs in all MBs (except WNT-MB) by means of extrachromosomal DNA (ecDNA) with MYCN and MYC being the genes most commonly involved across all subtypes (15).
Structural variants (SVs) and their breakpoints can be difficult to detect using short-read Illumina sequencing since the read length is much smaller than the variants of interest. Long-read sequencing with Oxford Nanopore Technology (ONT) or PacBio (PB) is proving itself as an effective tool to identify structural variants in both normal and cancer genomes (16–20), however, long-read technologies are still costly and require much more high molecular weight (HMW) DNA input (at least 1.5µg). Linked-read sequencing has also been shown to be effective in identifying complex structural rearrangements, including complex events such as chromothripsis (21–24) as well as point mutations (25). It combines long-range genome information with the accuracy of short-read Illumina sequencing while requiring only low DNA input amounts (1-10ng). This low input requirement allows the method to be applied in samples where DNA quantity is limited and costs are comparable to standard WGS with Illumina. Although 10x Genomics has discontinued their linked-read technology (10X-LR), alternatives have been developed by Illumina (Complete Long Read sequencing), MGI (stLFR) (26), Universal Sequencing Technology (TELL-Seq) (27) and others (28).
This paper aims to perform a comprehensive analysis of medulloblastoma genomes by characterizing single nucleotide variants (SNVs) as well as rare driver events caused by large SVs using 10x Genomics linked reads. Additional validation and integration was done using short-read WGS, RNA-Seq and long-read Nanopore and PacBio sequencing. We also show for the first time that 10X-LR can be used for the detection of ecDNAs as validated by Hi-C. In order to explore the application of alternative linked-read technologies, we generated TELL-Seq (27) libraries for 4 of the tumor samples and validated the somatic SVs detected by 10X-LR. Using these datasets, we aim to expand the understanding of medulloblastoma biology by identifying previously uncharacterized structural variation. Identification of SVs can be used to guide diagnosis, personalize the selection of chemotherapies and monitor patient response to treatment (12) highlighting the importance of developing highly sensitive, low-cost genomic assays which could eventually be used in routine clinical practice.
2 Results
We generated 10X-LR tumor and normal data for 25 patients (21 G4-MB, 2 G3-MBs and 2 SHH MBs) in order to detect complex structural events driving tumorigenesis (Table 1; Supplemental Table 1; Additional Table 1). Of these 25 samples, 13 were previously characterized by WGS (12) which we reanalyzed using our high-sensitivity pipeline. RNA-Seq data was also produced for 13 samples and used for validation of enhancer hijacking events and expression of somatic SNVs.
2.1 Structural variants and point mutations in G4 medulloblastomas
In first instance, we generated tumor-normal 10X-LR datasets for the 13 samples that were part of the Northcott et al. study, and analyzed them using our in-house 10X-LR pipeline (see Methods) to identify previously undiscovered structural variants and provide a comprehensive list of somatic structural rearrangements. In addition, we re-analyzed the existing WGS data using an enhanced SV detection pipeline which uses 6 different SV callers in order to improve sensitivity (see Methods) (29). Combining the results from our WGS and 10X linked-read pipelines, we detected 265 somatic SV (which were manually confirmed using Loupe) in the 13 samples previously characterized by WGS (Additional Table 2, contains breakpoint coordinates for each technology and caller). Of these, 74 were detected by both 10X-LR and WGS, 173 were found by 10X only, and 18 were called by WGS only but visually confirmed in the 10X data. Our findings include mutations in recurrently mutated genes such as a SNCAIP duplication (Figure 1A), an inversion and an amplification in GFI1B (Figures 1B, C), an intrachromosomal rearrangement in GFI1 (Figure 1D), and 2 large amplifications which include the CDK6 locus (MDT-AP-2878, chr7: 86,891,518- 95,624,126, Figure 1E, MDT-AP-1209, chr7:90,074,594-93,426,132, Figure 1F) all of which were previously detected by Northcott et al. and validated by 10X-LR (12). We also detected a novel complex rearrangement on chromosome 8 in MDT-AP-2130 (validated by WGS, Figure 2A). Additionally, we detected a complex event in MDT-AP-2878 involving chromosomes 2 and 16 with a breakpoint downstream of IDH1 that had not been previously characterized but was validated in the re-analyzed WGS data (Supplemental Figures 1A, B).
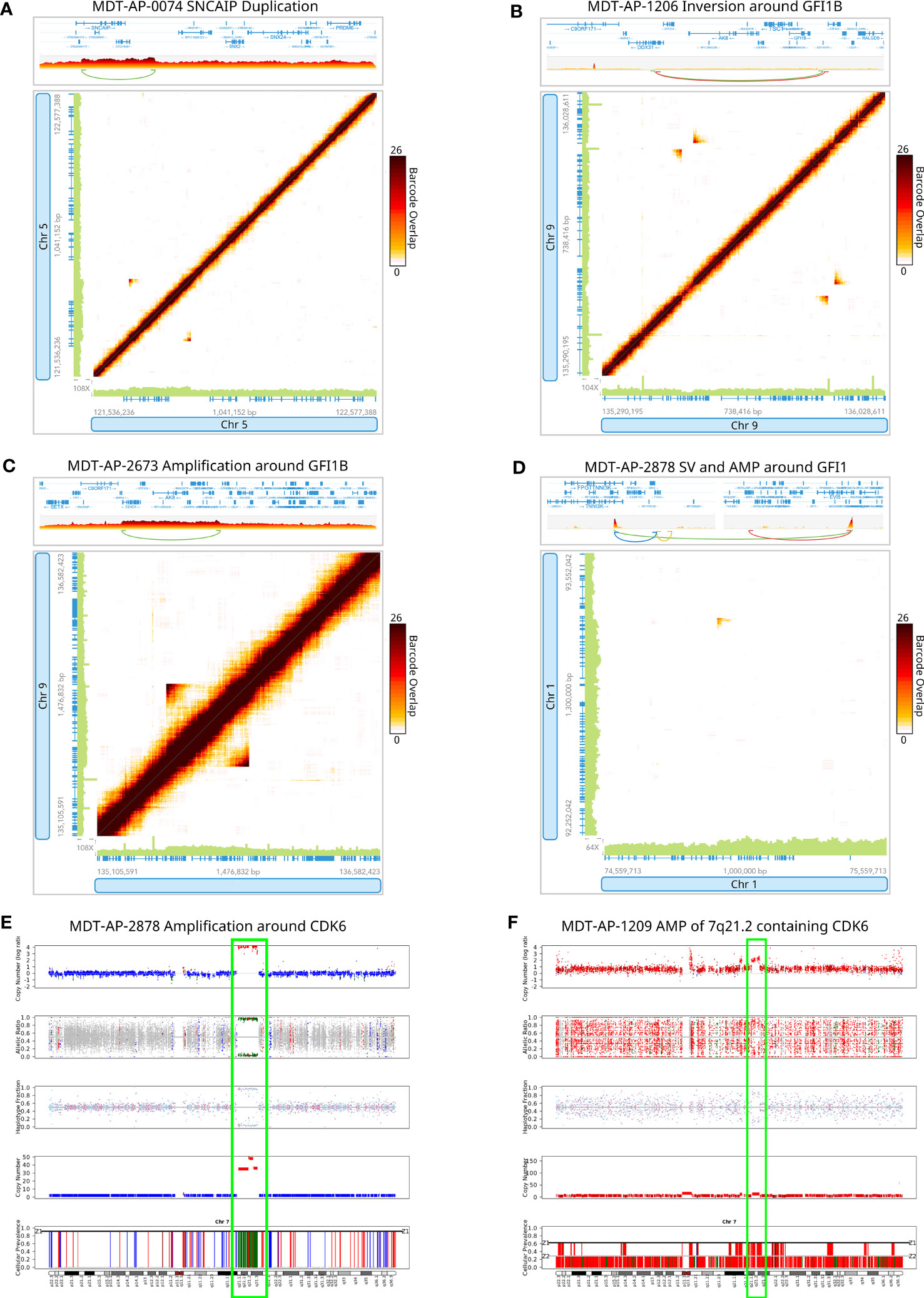
Figure 1 Detection of structural variants around recurrently mutated genes. 10X-LR data supporting (A) a SNCAIP duplication in MDT-AP-0074, (B) an inversion around GFI1B in MDT-AP-1206, (C) an amplification around GFI1B in MDT-AP-2673, (D) an structural variant and amplification around GFI1 in MDT-AP-2878, and (E) an amplification around CDK6 in MDT-AP-2878, visualization of the barcode overlap shown as heat maps in Loupe. Axes represent genomic regions and the colour of the points represents the number of barcodes that map to both of these regions. (F) Copy number profile of chromosome 7 showing the amplification of 7q21.1, which contains CDK6, in MDT-AP-1209, calculated and plotted with TitanCNA.
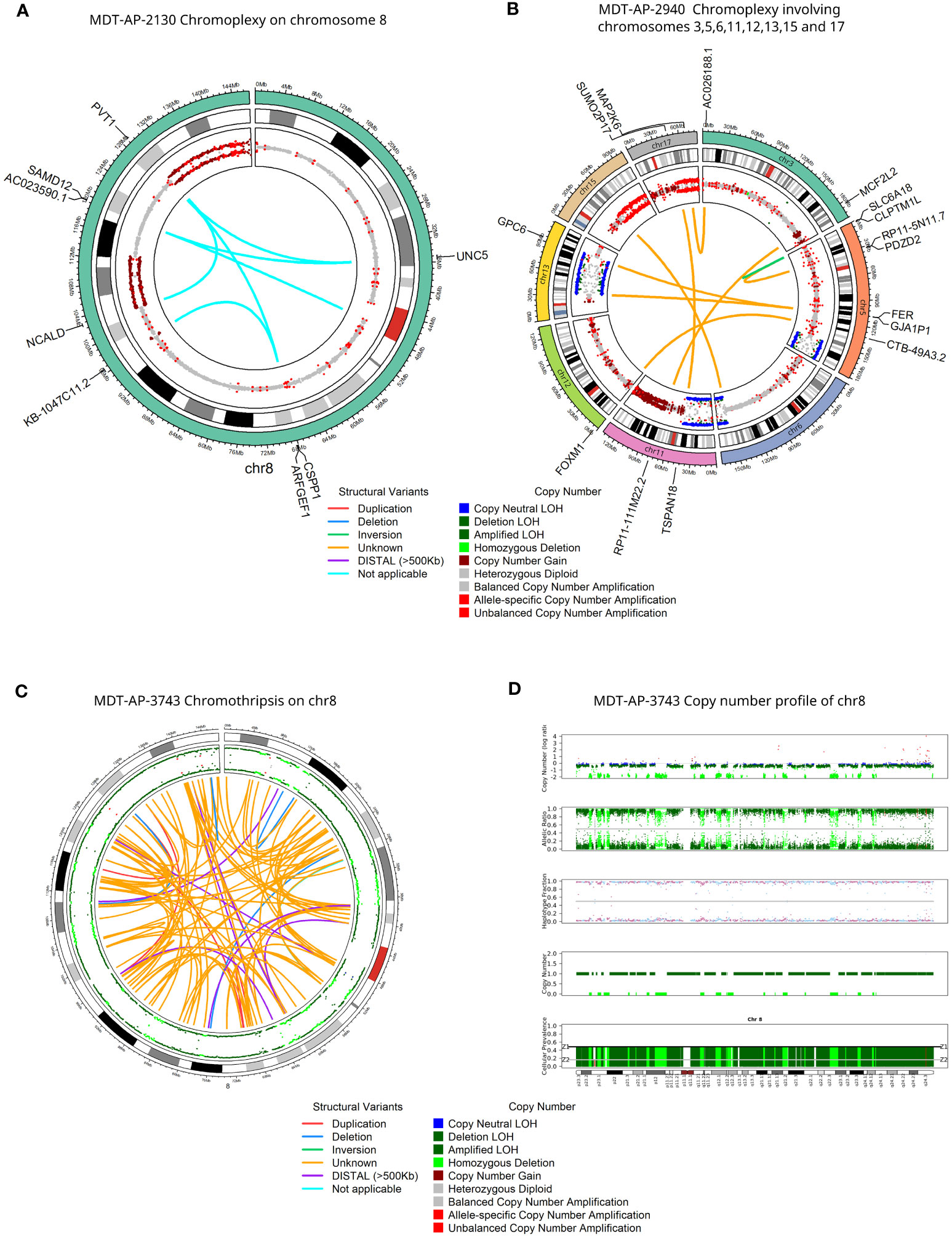
Figure 2 Detection of rare complex structural variants in G4 medulloblastomas. Circos plots for 10X-LR datasets showing (A) chromoplexy on chromosome 8 in MDT-AP-2130, (B) chromoplexy involving chromosomes 3, 5, 6, 11, 12, 13, 15 and 17 in MDT-AP-2940, and (C) chromothripsis on chromosome 8 in MDT-AP-3743. Outer circle shows allele frequency, as calculated by TitanCNA, were colour indicates the type of copy number change relative to the normal sample. Inner circle shows manually confirmed somatic SVs detected by 10X-LR and/or WGS and/or ONT and/or PacBio, colour indicates the type of SV (D) Copy number profile of chromosome 8 showing chromothripsis in MDT-AP-3743, calculated and plotted with TitanCNA.
Next, we applied 10x Genomics linked-read sequencing in 12 uncharacterized samples: 8 new G4 medulloblastomas, two G3 and two SHH medulloblastomas (see Findings in non-G4 medulloblastomas below). In the 8 G4 medulloblastomas that had not been characterized previously, we detected 147 somatic SVs that were manually confirmed using Loupe (Additional Table 2). MDT-AP-2940 was found to have chromoplexy involving chromosomes 3, 5, 6, 11, 12, 13, 15 and 17 (Figure 2B) as well as a complex event on chr5 leading to the amplification of TERT (Supplemental Figure 1C). Additionally, we detected chromothripsis in one sample involving chromosome 8 co-occurring with loss of 17p which contains TP53 (MDT-AP-3743, Figures 2C, D). Loss of TP53 is thought to be required for chromothripsis and although 17p loss in common in G4s, chromothripsis is rare (30).
MYCN was found amplified in MDT-AP-3670 and further analysis showed that this was part of a much larger complex SV and amplification with a breakpoint connecting it to a region 27.4Mb downstream on chromosome 2 (Figures 3A–D). Interestingly, both of these events were shown to share barcodes across the genome which suggests that there are many copies of these regions within the nucleus that are being caught within the emulsion created by the 10X-LR protocol (Figures 3C, D). We hypothesized that this patterning indicated ecDNA which we then validated using Hi-C data from the same sample (Supplementary Figure 2). Hi-C has previously been shown to be able to detect ecDNAs in a wide-range of tumors and cell lines (31–34). Additionally, copy number calls generated from 10X-LR using TitanCNA indicated approximately 75 copies of chromosome 2 from 14.6-16.3Mb and 41.7-41.9Mb as well as even higher amplification (~125 copies) of chromosome 2 from 15.5-15.74Mb and 15.75-15.96Mb which contains additional rearrangements (Figure 3E). As far as we are aware, this represent the first time ecDNA has been identified using 10X linked-reads.
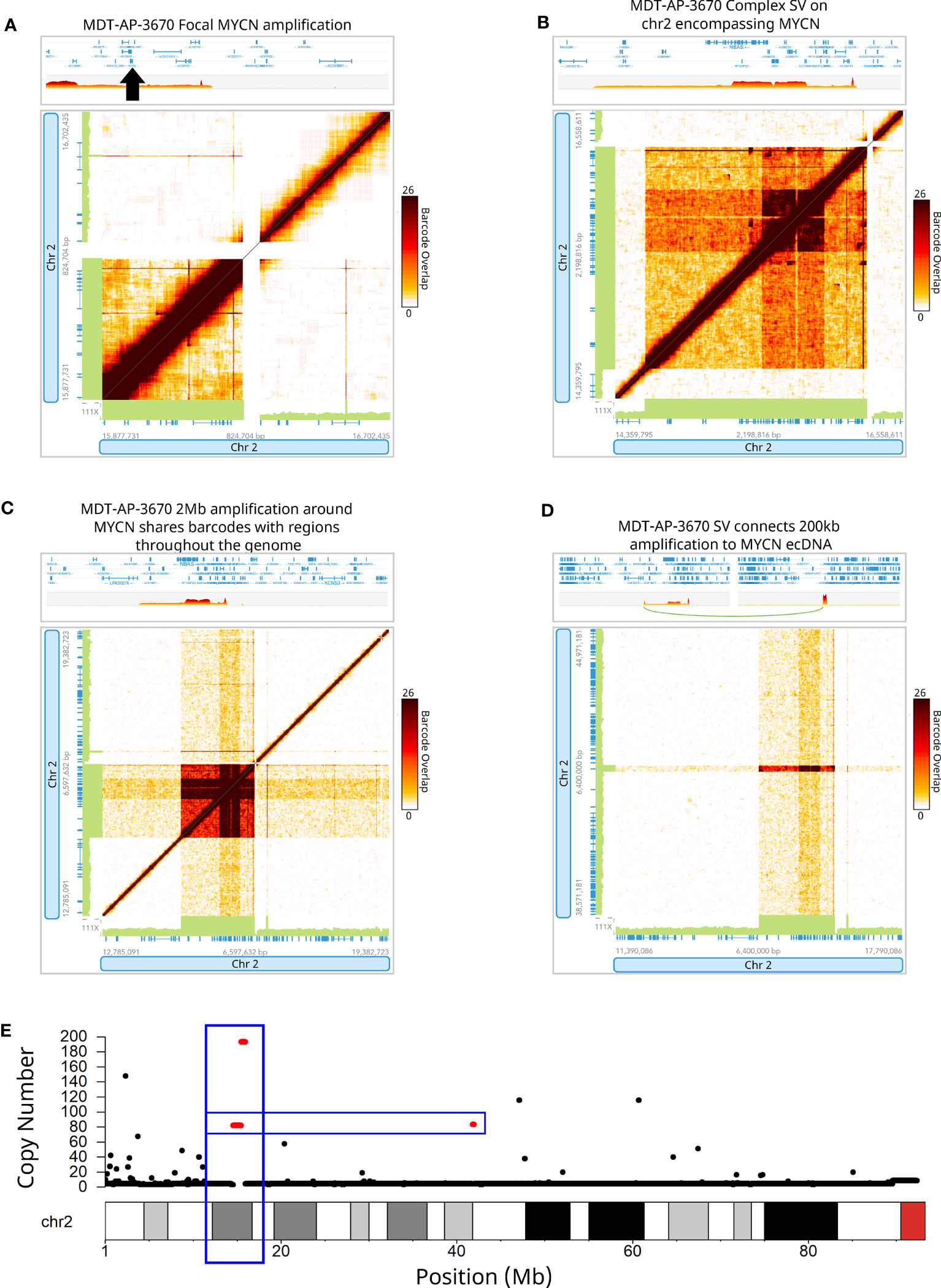
Figure 3 Detection of extrachromosomal DNA using linked-reads. 10X-LR data supporting (A) a duplication of MYCN, (B) a complex SV on chromosome 2 encompassing MYCN, (C) 2Mb amplification around MYCN shares barcodes with regions throughout the genome indicating ecDNA, (D) an SV which connects a 200kb amplification at 42Mb to the MYCN ecDNA, visualization of the barcode overlap shown as heat maps in Loupe. Axes represent genomic regions and the colour of the points represents the number of barcodes that map to both of these regions. (E) Copy number profile of chromosome 2 showing the amplification of the MYCN region (chr2:15Mb) and upstream region (chr2:42Mb), calculated and plotted with TitanCNA.
In terms of point mutations, SNVs described as functional SNVs and indels by Northcott et al. were manually validated in the linked-read data and as well as in the RNA-Seq where applicable (Additional Table 3) (12). These include a TERT promotor mutation in MDT-AP-2130 (C228T, Supplemental Figure 1D) as well as germline mutations in BRCA2 (p.Tyr3225IlefsTer30, Pathogenic/Likely pathogenic in ClinVar), RAD51D (p.Asp98ValfsTer25, likely pathogenic in ClinVar) and ATM (p.Arg2136Ter, Pathogenic/Likely pathogenic in ClinVar), all of which are associated with the double-stranded break repair pathway and cancer predisposition syndromes (35). Additionally, analysis of the linked-red data allowed us to detect two mutations in KDM6A, a frameshift variants in KMT2D [known to be recurrently mutated in G4-MB (10)], a mutation in CREBBP annotated as likely pathogenic in ClinVar, and a second TERT promoter mutation (C228T).KDM6A is a lysine demethylase recurrently mutated in both G3 and G4 medulloblastomas (36). The mutations were a missense mutation (p.R1255W, MDT-AP-2151, validated in WGS) previously detected in carcinomas of the pancreas, endometrium, prostate, breast, and skin as well as a truncating mutation annotated as likely pathogenic in ClinVar (R1331fs, MDT-AP-2857) found in the germline of three patients with Kabuki syndrome 2 (https://www.ncbi.nlm.nih.gov/clinvar/variation/216950/).
2.2 Enhancer hijacking in G4 medulloblastomas
In the G4 medulloblastomas, SVs affecting GFI1 and GFI1B and the recurrent tandem duplication of SNCAIP are known to cause overexpression of GFI1, GFI1B and PRDM6, respectively, by putting them under the control of super-enhancer regions (termed enhancer hijacking, EH). We used the RNA-Seq data, which was available for 13 samples, to validate these EH events. Expression levels supported enhancer hijacking of GFI1B in MDT-AP-1206 and MDT-AP-2673 as well as PRDM6 in MDT-AP-0074 (Figures 4A, B). Interestingly, MDT-AP-2151 was also shown to have overexpression of PRDM6 despite no evidence of a tandem duplication of SNCAIP by either Northcott et al. or us (12) (Figure 4C). However, copy number data from TitanCNA suggests a small duplication over PRDM6 which explains the increased expression and suggests that tandem duplication of SNCAIP may not be the only mechanism leading to overexpression of PRDM6 (Figure 4D).
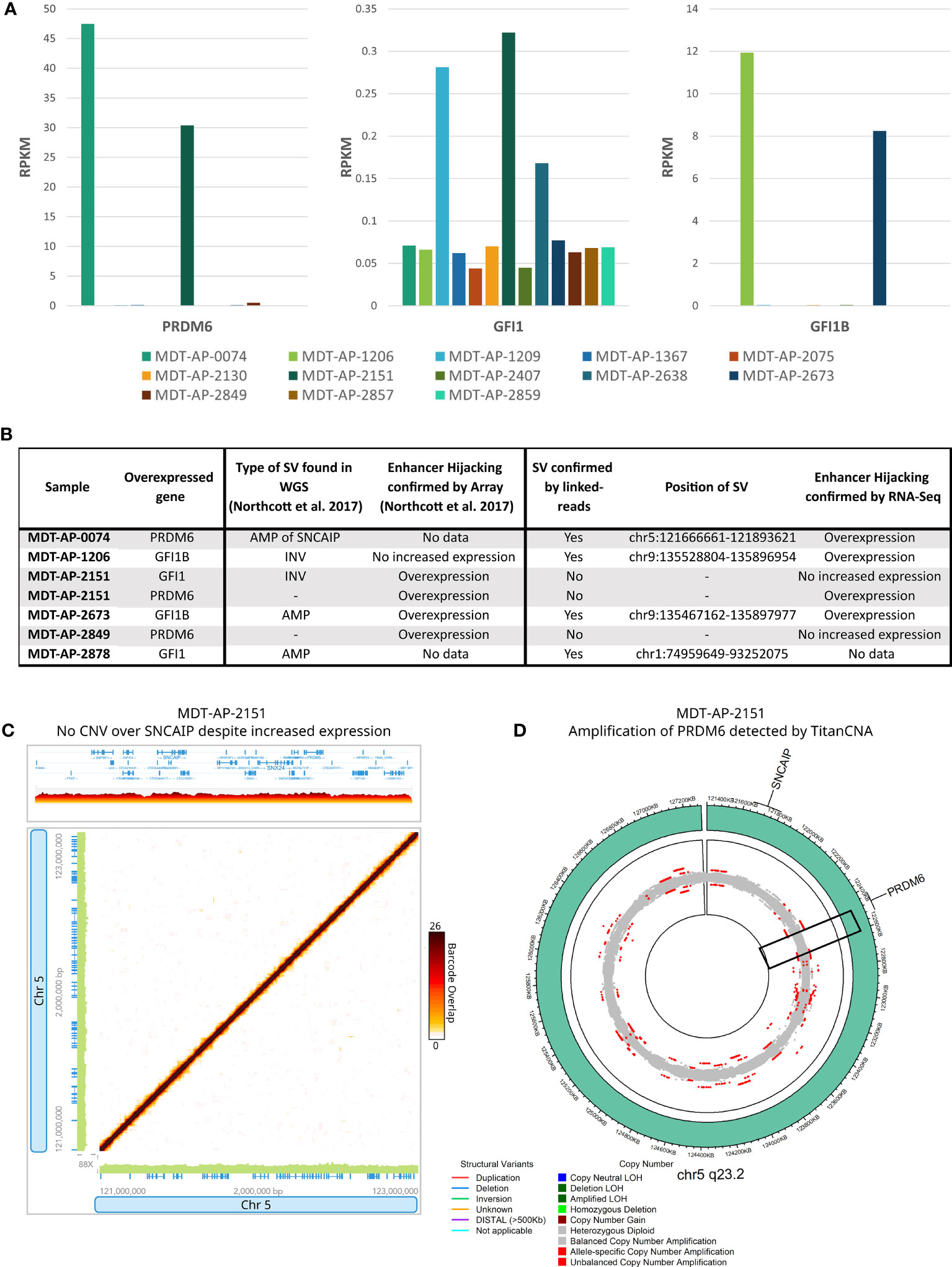
Figure 4 Enhancer hijacking in G4 medulloblastomas. (A) Bar graphs showing expression of PRDM6, GFI1 and GFI1B in all samples with RNA-Seq data available. (B) Table showing cases of enhancer hijacking in terms of SV calls and expression as described in Northcott et al. and this paper. (C) 10X-LR data showing no CNV over SNCAIP or PRDM6 in MDT-AP-2151 despite increased RNA-Seq expression, visualization of the barcode overlap shown as heat maps in Loupe. Axes represent genomic regions and the colour of the points represents the number of barcodes that map to both of these regions. (D) Circos plots for 5q23.3 showing a duplication of PRDM6 in MDT-AP-2151 as allele frequency, as calculated by TitanCNA, were colour indicates the type of copy number change relative to the normal sample.
2.3 Copy-number variants in G4 medulloblastomas
Group 4 medulloblastomas are also known to be tetraploid, with 11/21 samples in this study having a ploidy of 4 (52%, Supplemental Table 1). Group 4 MBs are also characterized by extensive copy number variants, two of the most characteristic being gain of chromosome 17q (14/21, 66%) with or without loss of chromosome 17p (13/21, 62%, contains TP53), as well as a gain of chromosome 7 (11/21, 52%) and loss of chromosome 8 (10/21, 47%) (14) (Supplemental Figure 3).
2.4 Findings in non-G4 medulloblastomas
We detected 11 manually confirmed somatic variants in the previously uncharacterized non-G4 medulloblastomas (2 in 2 SHH-MBs and 9 in 2 G3-MBs, Additional Table 2). One SHH tumor was found to have a TERT promoter mutation (C228T, MDT-AP-3724) as well as a previously described CREBBP mutation (p.R1446L c.4337G>T), a single-base deletion in exon 34 of lysine-specific methyltransferase 2D (KMT2D), an interchromosomal translocation between chromosomes 3 and 14 (Supplemental Figure 4A), 4 copies of 3q and loss of 14q24.1-q32.33 (Supplemental Figure 4B). The other was characterized by an interchromosomal translocation between chromosomes 7 and 18 (MDT-AP-3862, Supplemental Figure 4C), a gain of 7q31.2-36.3, loss of 20 and loss of homozygosity on 10q which contains SUFU (Supplemental Figure 4D).
The group 3 medulloblastomas were mainly characterized by copy number changes and structural variants although none were recurrent between the two samples (Additional Table 2). Of note, one G3-MB was found to have a germline interchromosomal translocation between chromosomes 2 and 5 occurring near 2 protocadherin genes and a protocadherin gene cluster (MDT-AP-4037, Supplemental Figures 4E, F). Previous work suggests that protocadherins may play a role in tumorigenesis in medulloblastomas (37, 38).
2.5 Cross-validation using long-read PacBio and Oxford Nanopore data
We generated PacBio data from 5 G4 MB tumor-normal pairs where additional DNA material was available (7-19X coverage, Supplemental Table 1). In addition, we were able to generate paired tumor-normal Nanopore data from two of these G4 tumor samples at 15-30X coverage plus deep sequencing data from the tumor of MDT-AP-2673 (53x coverage). All samples with long-read data also had WGS and RNA-Seq data available (Supplemental Table 1). Analysis of the long-read data allowed the detection of 16 somatic SVs that had been confirmed as somatic and included the focal events around GFI1B and SNCAIP leading to enhancer hijacking (Additional Table 2). Additionally, we detected 4 SVs found uniquely by long-reads which we validated as somatic (Additional Table 2).
2.6 Comparison of linked-read technologies
Since 10x Genomics has discontinued their linked-read kit, we decided to test the TELL-Seq library kit by generating data for 4 tumor samples in order to compare the SV calls. We chose samples which had somatic SVs in GFI1B (MDT-AP-1206 and MDT-AP-2673), in TERT (MDT-AP-2940) and GFI1 (MDT-AP-2878) that had previously been detected by 10X-LR.QC metrics for both technologies were generated using the LongRanger pipeline. On average, the 10X-LR samples had longer mean molecules lengths compared to TELL-Seq although this is likely due to sample degradation over time since the same HMW DNA extractions we used to generate both tumor linked-read datasets about 2 years apart (Supplemental Table 2). As a result, the 10X-LR data out-performed TELL-Seq in terms of the number of phased SNPs and longest phase block. Both technologies had similar numbers of large SV and short deletions calls made by LongRanger (Supplemental Table 2 and Additional Table 1); however, the TELL-Seq data had much more even coverage compared to the 10X-LR data (Supplemental Figure 5).
Nearly all high-quality somatic calls (detect by at least 2 callers and >10kb) made in the 10X-LR data were validated by TELL-Seq. 84-125 calls were made by both technologies, 21-44 calls were made by 10X only, and 3-6 were made by TELL-Seq only (Figure 5A and Additional Table 4). 63 somatic calls were made across the 4 samples of which 49 were called by at least 2 callers in the 10X-LR dataset (the rest where either detected by WGS or a single caller in the 10X-LR datasets). Of these 49 calls, 32 were detected in both the 10X-LR and TELL-Seq datasets from the same patient (Figure 5B). Of the 22 somatic SVs which occurred in a gene of interest or are part of a complex genomic event such as chromoplexy, 14 were detected in both the 10X-LR and TELL-Seq datasets (Additional Table 4) and included both enhancer hijacking events in GFI1B (Figures 5C, D) and the amplification around TERT (Figure 5E). Eight somatic SVs were only detected in the 10X-LR, however, manual inspection of the TELL-Seq data in Loupe allowed us to confirm visually the presence of the SVs not called by TELL-Seq including the SV affecting GFI1 in MDT-AP-2878 (Figure 5F).
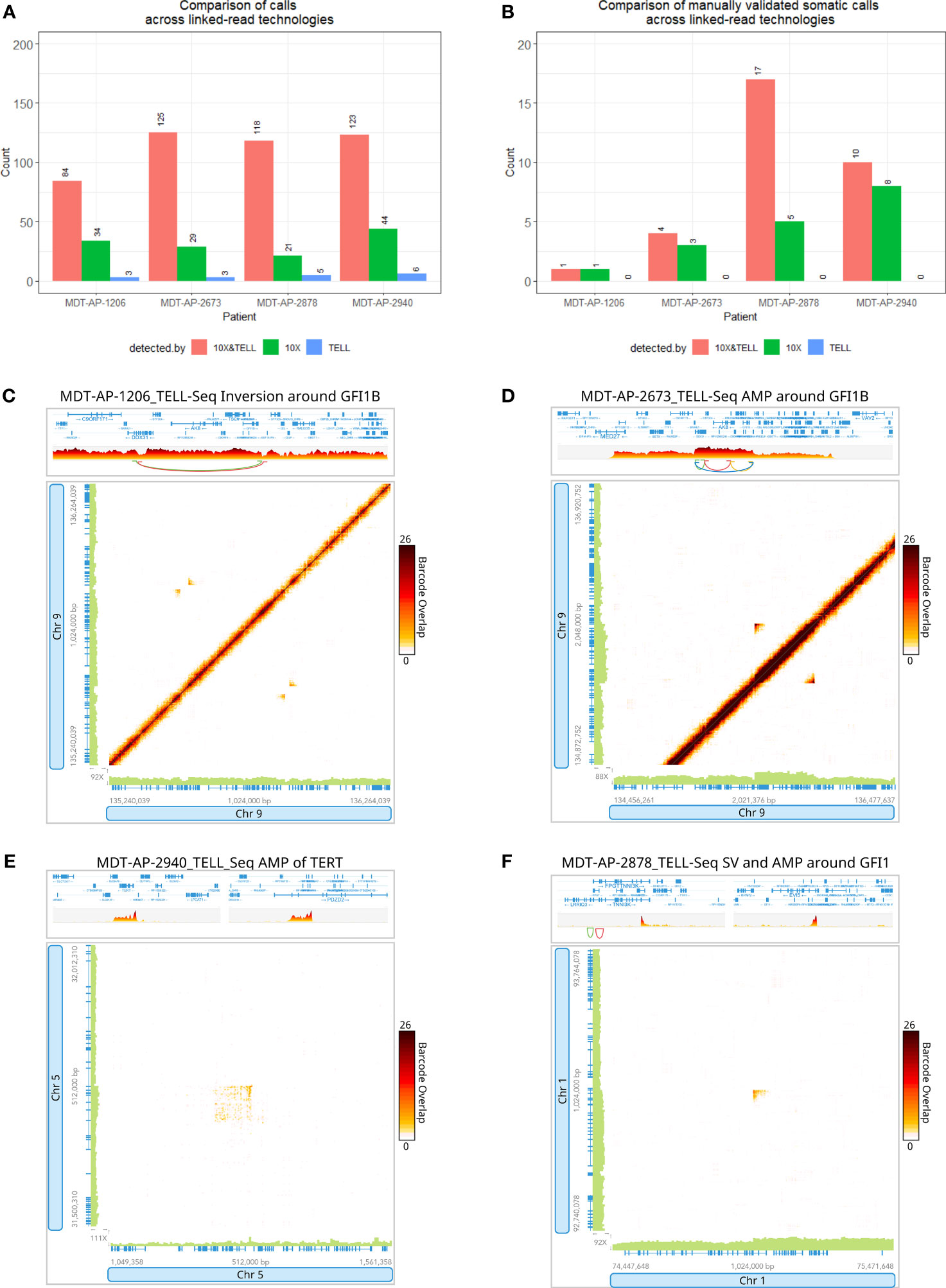
Figure 5 Detection of variants with 10x Genomics and Universal Sequencing Technologies’ linked-read protocols. Bar graphs comparing (A) the number of SV calls and (B) the number of manually validated SV calls detected by both 10X-LR and TELL-Seq, 10X-LR only and TELL-Seq only. TELL-Seq data supporting (C) an inversion around GFI1B in MDT-AP-1206, (D) an amplification around GFI1B in MDT-AP-2673 and, (E) a structural variant and amplification around GFI1 in MDT-AP-2878, and (F) the amplification of TERT in MDT-AP-2940, visualization of the barcode overlap shown as heat maps in Loupe after conversion of TELL-Seq data to LongRanger format. Axes represent genomic regions and the colour of the points represents the number of barcodes that map to both of these regions.
3 Discussion
In this paper, we applied 10x Genomics linked-read data to 25 medulloblastomas in order to identify additional rearrangements in 13 samples previously characterized by WGS and establish cross validation of findings. Using our custom 10X-LR analysis pipeline, we were able to detect 96 SVs not previously described in these samples, of which 86 could be validated when using our high-sensitivity WGS pipeline. Additionally, we characterized 12 new samples in which we detected 158 manually confirmed somatic SV including a TERT promoter mutation and complex SV involving the TERT gene, chromoplexy involving 8 chromosomes, chromothripsis involving chromosome 8, ecDNA amplification of MYCN and a germline interchromosomal SV occurring near a medulloblastoma candidate gene family. A summary of all variants of interest identified in our datasets can be found in Table 2 and all SV calls that were manually validated as somatic across all technologies and callers can be found in Additional Table 2.
Using linked-reads, we identified both rare and novel mutational events in G4 medulloblastomas. These mutations include chromothripsis in a G4-MB which is rare despite the high frequency of loss of TP53 (via loss of 17p) which is believed to be a requirement for chromothripsis (30). We identified two point mutations in KDM6A, which had not previously been identified in medulloblastomas, and validated germline mutations in BRCA2, RAD51D and ATM, which are all involved in DNA repair of double-stranded breaks as well as hereditary cancer syndromes (35). Medulloblastomas have long since been associated with germline mutations in APC, PTCH1, SUFU and TP53 (39) and more recently in BRCA2 and PALB2 (40). To date, germline RAD51D mutations have only been associated with increased risk of ovarian cancer and ATM germline mutations have primarily been shown to increased risk of breast cancer as well as case familial cases of ovarian, prostate, and pancreatic cancers. However, both RAD51D and ATM are involved in the homologous repair pathway that also includes medulloblastoma susceptibility genes TP53, BRCA2, and PALB2 (40). Additionally, we detected a novel germline interchromosomal variant in a G3 medulloblastoma. Interestingly, the breakpoint for this translocation on chromosome 5 falls 120kb way from protocadherin 12 (PCDH12), 200kb away from protocadherin 1 (PCDH1), and 500kb away from the protocadherin gamma (PCDHG) gene cluster. While none of these genes have been specifically investigated, mutations in other protocadherins, PCDH9 (38) and PCDH10 (37) have been identified as potential drivers in medulloblastoma. Despite the identification of rare variants and new SVs in recurrently affected genes, no novel recurrently mutated genes could be identified which is unsurprising considering the modest size of our dataset.
Lastly, we showed for the first time that ecDNA can be identified using linked-reads alone. Due to the high number of copies circulating within the nucleus, ecDNAs are randomly captured within the emulsions created by the 10x Genomics linked-read protocol. This makes the amplified region appear to be found at low levels throughout the genome and generates a similar pattern to Hi-C data were the ecDNA is shown to interact with the entirety of the genome (31–34).
In this paper, we show that linked-reads provide detailed characterization of many types of variants including SNPs, SVs, CNVs, chromothripsis and ecDNAs while also providing phasing and breakpoint information. The minimal input required by linked-read technologies makes them an appealing option for clinical diagnosis particularly when tumors are small or occur in regions which are surgically inaccessible but can still be biopsied. Limitations to linked-read technologies include evenness of coverage and difficulty with repetitive regions. The 10x Genomics protocol uses a polymerase with stand displacement to generate barcoded amplicons during the isothermal incubation step, however this leads to uneven coverage compared to standard PCR-free WGS although this seems to be less of an issue with the TELL-Seq protocol (Supplemental Figure 5). Additionally, since linked-reads are a short-read based technology, repetitive regions larger than the length of a read are still difficult to align with precision. Long-reads are more likely to span the entirety of a low complexity region, making alignment less difficult. Other alternatives to both linked-reads and long-reads include Illumina’s new Complete Long Read (CLR) protocol which land-marks long DNA fragments before tagmenting them and sequencing them with their existing chemistry. The land-marking allows long DNA fragments to be fully reconstructed computationally as opposed to linked-read technologies where barcoded reads represent a sampling of a HMW DNA molecule.
In conclusion, our work provides further evidence for the high heterogeneity of variants seen across G4 medulloblastoma and adds new complex events including a new mechanism of PRDM6 overexpression via gene duplication. G3 and G4 medulloblastomas have been shown to be driven by SVs across many different genes (39). Our group and others have shown that technologies that provide long-range information are required to characterize the full spectrum of SVs in medulloblastomas (41).
4 Methods
4.1 10x Genomics linked-reads
10x Genomics linked-read libraries were generated for 25 tumors and corresponding normal samples. HMW DNA was extracted from tumors using phenol chloroform extractions or the Nanobind tissue kit (PacBio, Menlo Park, California, United States, cat# SKU 102-302-100) while matching blood samples were extracted using the QiaAmpBlood Kit (Qiagen, Hilden, Germany) or the Nanobind tissue kit (PacBio, Menlo Park, California, United States, cat# SKU 102-302-100) (Additional Table 1). Size selection was done with the SRE and SRE XS kits (PacBio, Menlo Park, California, United States, cat# SKU 102-208-200 and SKU 102-208-300) as needed and dependent on the availability of DNA (Additional Table 1). Concentration was assessed by Qubit™ dsDNA BR Assay Kit (ThermoFisher Scientific, cat# Q32853) and size distribution was profiled using the Femto Pulse (Genomic DNA 165 kb Kit, 3 hours run, Agilent Technologies, Inc., Santa Clara, California, United States, cat# FP-1002-0275). Samples and library preparation were done following the Chromium™ Genome Reagent Kits v2 User Guide (10x Genomics, Pleasanton, California, United States). Libraries concentration was assessed by qPCR (Roche, Basel, Switzerland, KAPA Library Quantification Kits - Complete kit (Universal), cat# 07960140001) and the size distribution of the libraries was evaluated using the Caliper LabChip (DNA High Sensitivity assay, PerkinElmer, Inc., Waltham, Massachusetts, United States). Libraries were sequenced using 150PE Illumina reads, either on a single lane of HiSeqX or pooled on a NovaSeq S4 flowcell. Average molecule length, calculated by LongRanger, ranged from 19kb-85kb for tumor samples and 42kb-104kb for normal samples (Additional Table 1).
Data was analyzed as detailed in Zwaig et al. (42). In brief, alignment and variant calling was done using 10x Genomics’ LongRanger pipeline followed by additional SV calling with GROC-SV (43), NAIBR (44) and LinkedSV (45) and SvABA (46) and copy number calling with TitanCNA 10x workflow (23). A custom R script was used to find calls made by multiple callers and we manually confirmed all SV calls detected by at least 2 callers and over 10kb in Loupe which are listed with breakpoint information and gene annotation in Additional Table 2. SVs labeled as “variants of interest” in Additional Table 2 include all SVs occurring in a genes known to be recurrently mutated in G4 medulloblastomas (CDK6, GFI1, GFI1B, MYCN, SNCAIP/PRDM6), those occurring in or near genes known to be recurrently mutated in other cancers types (IDH1 and TERT), those near suspected medulloblastoma genes (procadherin genes), and complex somatic variants such as chromoplexy and chromothripsis). These variants of interest are discussed in more detail within the results section. Only 3 other genes were mutated in more than one patient; these include ARFGEF1 and KB-1047C11.2 which contain breakpoints associated with chromoplexy and chromothripsis on chromosome 8 in MDT-AP-2130 and MDT-AP-3743, respectively, and STEAP2-AS1 which is found near CDK6 and contains breakpoints in both samples with CDK6 amplifications.
4.2 Whole-genome sequencing
WGS data was available through Northcott et al. (12) and processed using the GenPipes Tumor-Pair pipeline for SV calling (-t sv) and SNP calling (-t ensemble) (29). We also ran SvABA on the WGS data (46).
4.3 Nanopore sequencing
Two tumors and their matching normal samples (MDT‐AP‐1367 and MDT‐AP‐1405) were sequenced on the MinIon (Oxford Nanopore Technologies Limited, Oxford, United Kingdom). MDT-AP-2673 was sequencing on 2 PromethION flowcells (Oxford Nanopore Technologies Limited, Oxford, United Kingdom). Both the MinIon and PromethIon libraries used 2µg of HMW DNA as input. Nanopore data was aligned to genome build b37 with minimap2 (version 2.17) using parameter -ax map-ont (47). Structural variants were called SVIM (48) (parameters –min_mapq 7 –min_sv_size 50 –max_sv_size 100000), NanoVar (49) (version 1.3.9, parameters –data_type ont –mincov 2 –minlen 50), CuteSV (50) (version 1.0.11, parameters –min_size 50 –max_size 100000 –min_support 2 –min_mapq 7 –max_cluster_bias_INS 100 –diff_ratio_merging_INS 0.3 –max_cluster_bias_DEL 100 –diff_ratio_merging_DEL 0.3), and Sniffles2 (51) (version 2.0.6, parameters –minsupport 2 –mapq 7 –minsvlen 50 –non-germline).
4.4 PacBio sequencing
PacBio data was available for 5 tumors and their matching normal samples. Samples were normalized to a concentration of 125pM and sequenced with 4-hour movies. Data was aligned to genome build b37 using NGMLR (51) (version 0.2.7) and SVs were called using Sniffles (51) (version 1.0.10, parameters –min_support 2 –min_length 30).
4.5 TELL-Seq
TELL-Seq libraries were generated using the same HMW DNA aliquots as the 10X-LR libraries. 5ng of HMW DNA was used per library (as recommended by the UST TELL-Seq™ WGS Library Prep User Guide V8) and quantified by Qubit™ dsDNA HS Assay Kit (ThermoFisher Scientific, cat# Q32854). Final libraries were assessed by qPCR (KAPA Library Quantification Kits) and Caliper LabChip. Libraries were sequenced using 150PE Illumina reads on 1 lane of NovaSeq SP. QC and barcodes correction was done using the TELL-Read pipeline, and SNP calling was done using the TELL-Sort pipeline. We used the ust10x tools to convert the TELL-Seq data to 10X-LR format and ran our in-house pipeline detailed above with the exception of GROC-SV which did not run to completion on the TELL-Seq data.
4.6 RNA sequencing
Bulk RNA-Seq data was generated for 13 samples and analyzed using the GenPipes RNA-Seq pipeline (29). Overexpression of genes affected by enhancer hijacking was measured using the reads per kilobase of transcript, per million mapped reads (RPKM) calculated by the pipeline.
4.7 Hi-C
Hi-C data was available for MDT-AP-3670 (unpublished work). Detailed description of the library preparation protocol and analysis workflow can be found in (42).
4.8 Comparison of SV calls across genomic technologies (10X-LR, WGS, ONT, PacBio)
A custom R script was used to find SVs detected by multiple technologies (i.e. were both the start and end breakpoints fell within ±1000bp of each other) and we manually assessed all SV calls made by 2 or more callers and larger than 10kb in size using Loupe. All manually confirmed somatic structural variant calls can be found in Additional Table 2.
4.9 Comparison between 10X-LR and TELL-Seq
Evenness of coverage was compared using BVAtools depthofcoverage (parameters, –gc–minMappingQuality 15 –minBaseQuality 15 –ommitN –maxDepth 1000 –binsize 50000–summaryCoverageThresholds 0,1,2,3,4,5,6,7,8,9,10,11,12,13,14,15,16,17,18,19,20,21,22,23,24,25,26,27,28,29,30,31,32,33,34,35,36,37,38,39,40) and plotted using the karyoploteR package in R. A custom R script was used to compare SVs made by both 10X-LR and TELL-Seq (i.e. were both the start and end breakpoints fell within ±1000bp of each other). All SV calls made by 2 or more callers and large than 10kb in size were manually validated using Loupe. All structural variant calls across both linked-read technologies and all callers can be found in Additional Table 4.
Data availability statement
The datasets presented in this study can be found in online repositories. The names of the repository/repositories and accession number(s) can be found below: https://ega-archive.org, EGAS00001007064, https://ega-archive.org, EGAS00001001953.
Ethics statement
Protocols for this study were approved by the Research Ethicsand Review Board (REB) at the McGill University Health Centre(Project number: 2018-4511) and affiliated hospital ResearchInstitutes. Patient samples were collected with informed consentfrom all research participants or their delegates.
Author contributions
MZ and JR contributed to the study conception and design. Generation and analysis of linked-read data, analysis of Nanopore, PacBio, WGS and RNA-Seq data and first draft of the manuscript was done by MZ. Hi-C data was generated by JL and analysis was done by MJ. PacBio and MinION data was generated by HF. All authors read and approved the final manuscript.
Funding
This work was supported by funding from a large-scale applied research project grant from Genome Quebec, Genome Canada, the Government of Canada, and the Ministère de l’Économie, de la Science et de l’Innovation du Québec with support from the Ontario Institute for Cancer Research through funding provided by the Government of Ontario (to NJ, MT, and JR), the CFI project Canada’s Genome Enterprise (CGEn) 35444, 33408 and 40104, (NJ, JR), the Genome Canada Platform grant: McGill Applied Genomics Innovation Core (MAGIC) (JR), as well as funding from the Fondation Charles-Bruneau to NJ. MT is a CPRIT Scholar in Cancer Research. MT is supported by the NIH (R01NS106155, R01CA159859 and R01CA255369), The Pediatric Brain Tumour Foundation, The Terry Fox Research Institute, The Canadian Institutes of Health Research, The Cure Search Foundation, Matthew Larson Foundation (IronMatt), b.r.a.i.n.child, Meagan’s Walk, SWIFTY Foundation, The Brain Tumour Charity, Genome Canada, Genome BC, Genome Quebec, the Ontario Research Fund, Worldwide Cancer Research, V-Foundation for Cancer Research, and the Ontario Institute for Cancer Research through funding provided by the Government of Ontario. MT is also supported by a Canadian Cancer Society Research Institute Impact grant, a Cancer Research UK Brain Tumour Award, and by a Stand Up To Cancer (SU2C) St. Baldrick’s Pediatric Dream Team Translational Research Grant (SU2C-AACR-DT1113) and SU2C Canada Cancer Stem Cell Dream Team Research Funding (SU2C-AACR-DT-19-15) provided by the Government of Canada through Genome Canada and the Canadian Institutes of Health Research, with supplementary support from the Ontario Institute for Cancer Research through funding provided by the Government of Ontario. Stand Up to Cancer is a program of the Entertainment Industry Foundation administered by the American Association for Cancer Research. MT is also supported by the Garron Family Chair in Childhood Cancer Research at the Hospital for Sick Children and the University of Toronto.
Acknowledgments
The authors would like to thank Jim Loukides (Manager, Brain Tumour Biobank at SickKids) and recognize the Labatt Brain Tumor Research Centre and The Michael and Amira Dan Brain Tumour Bank Network.
Conflict of interest
Author HF was employed by the company BioBox Analytics Inc.
The remaining authors declare that the research was conducted in the absence of any commercial or financial relationships that could be construed as a potential conflict of interest.
Publisher’s note
All claims expressed in this article are solely those of the authors and do not necessarily represent those of their affiliated organizations, or those of the publisher, the editors and the reviewers. Any product that may be evaluated in this article, or claim that may be made by its manufacturer, is not guaranteed or endorsed by the publisher.
Supplementary material
The Supplementary Material for this article can be found online at: https://www.frontiersin.org/articles/10.3389/fonc.2023.1221611/full#supplementary-material
References
1. Ostrom QT, Gittleman H, Truitt G, Boscia A, Kruchko C, Barnholtz-Sloan JS. CBTRUS statistical report: primary brain and other central nervous system tumors diagnosed in the United States in 2011-2015. Neuro Oncol (2018) 20:iv1–iv86. doi: 10.1093/neuonc/noy131
2. Menyhárt O, Giangaspero F, Győrffy B. Molecular markers and potential therapeutic targets in non-WNT/non-SHH (group 3 and group 4) medulloblastomas. J Hematol Oncol (2019) 12:29. doi: 10.1186/s13045-019-0712-y
3. Kumar R, Liu APY, Northcott PA. Medulloblastoma genomics in the modern molecular era. Brain Pathol (2020) 30:679–90. doi: 10.1111/bpa.12804
4. Kool M, Korshunov A, Remke M, Jones DTW, Schlanstein M, Northcott PA, et al. Molecular subgroups of medulloblastoma: an international meta-analysis of transcriptome, genetic aberrations, and clinical data of WNT, SHH, Group 3, and Group 4 medulloblastomas. Acta neuropathol (2012) 123:473–84. doi: 10.1007/s00401-012-0958-8
5. Northcott PA, Shih DJH, Peacock J, Garzia L, Morrissy AS, Zichner T, et al. Subgroup-specific structural variation across 1,000 medulloblastoma genomes. Nature (2012) 488:49–56. doi: 10.1038/nature11327
6. Skowron P, Farooq H, Cavalli FMG, Morrissy AS, Ly M, Hendrikse LD, et al. The transcriptional landscape of Shh medulloblastoma. Nat Commun (2021) 12:1749. doi: 10.1038/s41467-021-21883-0
7. Remke M, Ramaswamy V, Peacock J, Shih DJ, Koelsche C, Northcott PA, et al. TERT promoter mutations are highly recurrent in SHH subgroup medulloblastoma. Acta Neuropathol (2013) 126:917–29. doi: 10.1007/s00401-013-1198-2
8. Cavalli FMG, Remke M, Rampasek L, Peacock J, Shih DJH, Luu B, et al. Intertumoral heterogeneity within medulloblastoma subgroups. Cancer Cell (2017) 31:737–54.e6. doi: 10.1016/j.ccell.2017.05.005
9. Suzuki H, Kumar SA, Shuai S, Diaz-Navarro A, Gutierrez-Fernandez A, De Antonellis P, et al. Recurrent noncoding U1 snRNA mutations drive cryptic splicing in SHH medulloblastoma. Nature (2019) 574:707–11. doi: 10.1038/s41586-019-1650-0
10. Hendrikse LD, Haldipur P, Saulnier O, Millman J, Sjoboen AH, Erickson AW, et al. Failure of human rhombic lip differentiation underlies medulloblastoma formation. Nature (2022) 609:1021–28. doi: 10.1038/s41586-022-05215-w
11. Northcott PA, Lee C, Zichner T, Stütz AM, Erkek S, Kawauchi D, et al. Enhancer hijacking activates GFI1 family oncogenes in medulloblastoma. Nature (2014) 511:428–34. doi: 10.1038/nature13379
12. Northcott PA, Buchhalter I, Morrissy AS, Hovestadt V, Weischenfeldt J, Ehrenberger T, et al. The whole-genome landscape of medulloblastoma subtypes. Nature (2017) 547:311–17. doi: 10.1038/nature22973
13. Taylor MD, Northcott PA, Korshunov A, Remke M, Cho YJ, Clifford SC, et al. Molecular subgroups of medulloblastoma: the current consensus. Acta Neuropathol (2012) 123:465–72. doi: 10.1007/s00401-011-0922-z
14. Juraschka K, Taylor MD. Medulloblastoma in the age of molecular subgroups: a review. J Neurosurgery: Pediatr PED (2019) 24:353–63. doi: 10.3171/2019.5.PEDS18381
15. Chapman OS, Luebeck J, Wani S, Tiwari A, Pagadala M, Wang S, et al. The landscape of extrachromosomal circular DNA in medulloblastoma. bioRxiv (2021). doi: 10.1101/2021.10.18.464907
16. Chaisson MJP, Sanders AD, Zhao X, Malhotra A, Porubsky D, Rausch T, et al. Multi-platform discovery of haplotype-resolved structural variation in human genomes. Nat Commun (2019) 10:1784. doi: 10.1038/s41467-018-08148-z
17. Daiger SP, Sullivan LS, Bowne SJ, Cadena ED, Koboldt D, Bujakowska KM, et al. Detection of large structural variants causing inherited retinal diseases. Adv Exp Med Biol (2019) 1185:197–202. doi: 10.1007/978-3-030-27378-1_32
18. Aganezov S, Goodwin S, Sherman RM, Sedlazeck FJ, Arun G, Bhatia S, et al. Comprehensive analysis of structural variants in breast cancer genomes using single-molecule sequencing. Genome Res (2020) 30(9):1258–73. doi: 10.1101/gr.260497.119
19. Knapp KM, Sullivan R, Murray J, Gimenez G, Arn P, D’Souza P, et al. Linked-read genome sequencing identifies biallelic pathogenic variants in DONSON as a novel cause of Meier-Gorlin syndrome. J Med Genet (2020) 57:195–202. doi: 10.1136/jmedgenet-2019-106396
20. Valle-Inclan JE, Stangl C, de Jong AC, van Dessel LF, van Roosmalen MJ, Helmijr JCA, et al. Optimizing Nanopore sequencing-based detection of structural variants enables individualized circulating tumor DNA-based disease monitoring in cancer patients. Genome Med (2021) 13:86. doi: 10.1186/s13073-021-00899-7
21. Greer SU, Nadauld LD, Lau BT, Chen J, Wood-Bouwens C, Ford JM, et al. Linked read sequencing resolves complex genomic rearrangements in gastric cancer metastases. Genome Med (2017) 9:57. doi: 10.1186/s13073-017-0447-8
22. Nattestad M, Goodwin S, Ng K, Baslan T, Sedlazeck FJ, Rescheneder P, et al. Complex rearrangements and oncogene amplifications revealed by long-read DNA and RNA sequencing of a breast cancer cell line. Genome Res (2018) 28:1126–35. doi: 10.1101/gr.231100.117
23. Viswanathan SR, Ha G, Hoff AM, Wala JA, Carrot-Zhang J, Whelan CW, et al. Structural alterations driving castration-resistant prostate cancer revealed by linked-read genome sequencing. Cell (2018) 174:433–47.e19. doi: 10.1016/j.cell.2018.05.036
24. Zhou B, Ho SS, Greer SU, Zhu X, Bell JM, Arthur JG, et al. Comprehensive, integrated, and phased whole-genome analysis of the primary ENCODE cell line K562. Genome Res (2019) 29(3):472–84. doi: 10.1101/gr.234948.118
25. Tarabichi M, Demeulemeester J, Verfaillie A, Flanagan AM, Loo PV, Konopka T. A pan-cancer landscape of somatic mutations in non-unique regions of the human genome. Nat Biotechnol (2021) 39:1589–96. doi: 10.1038/s41587-021-00971-y
26. Wang O, Chin R, Cheng X, Wu MKY, Mao Q, Tang J, et al. Efficient and unique cobarcoding of second-generation sequencing reads from long DNA molecules enabling cost-effective and accurate sequencing, haplotyping, and de novo assembly. Genome Res (2019) 29:798–808. doi: 10.1101/gr.245126.118
27. Chen Z, Pham L, Wu TC, Mo G, Xia Y, Chang PL, et al. Ultralow-input single-tube linked-read library method enables short-read second-generation sequencing systems to routinely generate highly accurate and economical long-range sequencing information. Genome Res (2020) 30:898–909. doi: 10.1101/gr.260380.119
28. Redin D, Frick T, Aghelpasand H, Käller M, Borgström E, Olsen R-A, et al. High throughput barcoding method for genome-scale phasing. Sci Rep (2019) 9:18116. doi: 10.1038/s41598-019-54446-x
29. Bourgey M, Dali R, Eveleigh R, Chen KC, Letourneau L, Fillon J, et al. GenPipes: an open-source framework for distributed and scalable genomic analyses. GigaScience (2019) 8. doi: 10.1093/gigascience/giz037
30. Rausch T, Jones DTW, Zapatka M, Stütz AM, Zichner T, Weischenfeldt J, et al. Genome sequencing of pediatric medulloblastoma links catastrophic DNA rearrangements with TP53 mutations. Cell (2012) 148:59–71. doi: 10.1016/j.cell.2011.12.013
31. Harewood L, Kishore K, Eldridge MD, Wingett S, Pearson D, Schoenfelder S, et al. Hi-C as a tool for precise detection and characterisation of chromosomal rearrangements and copy number variation in human tumours. Genome Biol (2017) 18:125–25. doi: 10.1186/s13059-017-1253-8
32. Helmsauer K, Valieva ME, Ali S, González RC, Schöpflin R, Röefzaad C, et al. Enhancer hijacking determines extrachromosomal circular MYCN amplicon architecture in neuroblastoma. Nat Commun (2020) 11:5823. doi: 10.1038/s41467-020-19452-y
33. Shoshani O, Brunner SF, Yaeger R, Ly P, Nechemia-Arbely Y, Kim DH, et al. Chromothripsis drives the evolution of gene amplification in cancer. Nature (2021) 591:137–41. doi: 10.1038/s41586-020-03064-z
34. Zhu Y, Gujar AD, Wong C-H, Tjong H, Ngan CY, Gong L, et al. Oncogenic extrachromosomal DNA functions as mobile enhancers to globally amplify chromosomal transcription. Cancer Cell (2021) 39:694–707.e7. doi: 10.1016/j.ccell.2021.03.006
35. Piombino C, Cortesi L, Lambertini M, Punie K, Grandi G, Toss A. Secondary prevention in hereditary breast and/or ovarian cancer syndromes other than BRCA. J Oncol (2020) 2020:6384190. doi: 10.1155/2020/6384190
36. Roussel MF, Stripay JL. Epigenetic drivers in pediatric medulloblastoma. Cerebellum (London England) (2018) 17:28–36. doi: 10.1007/s12311-017-0899-9
37. Bertrand KC, Mack SC, Northcott PA, Garzia L, Dubuc A, Pfister SM, et al. PCDH10 is a candidate tumour suppressor gene in medulloblastoma. Child’s Nervous System (2011) 27:1243–49. doi: 10.1007/s00381-011-1486-x
38. Robbins CJ, Bou-Dargham MJ, Sanchez K, Rosen MC, Sang QA. Decoding somatic driver gene mutations and affected signaling pathways in human medulloblastoma subgroups. J Cancer (2018) 9:4596–610. doi: 10.7150/jca.27993
39. Northcott PA, Jones DTW, Kool M, Robinson GW, Gilbertson RJ, Cho Y-J, et al. Medulloblastomics: the end of the beginning. Nat Rev Cancer (2012) 12:818–34. doi: 10.1038/nrc3410
40. Waszak SM, Northcott PA, Buchhalter I, Robinson GW, Sutter C, Groebner S, et al. Spectrum and prevalence of genetic predisposition in medulloblastoma: a retrospective genetic study and prospective validation in a clinical trial cohort. Lancet Oncol (2018) 19:785–98. doi: 10.1016/S1470-2045(18)30242-0
41. Rausch T, Snajder R, Leger A, Simovic M, Giurgiu M, Villacorta L, et al. Long-read sequencing of diagnosis and post-therapy medulloblastoma reveals complex rearrangement patterns and epigenetic signatures. Cell Genomics (2023) 3(4). doi: 10.1101/2022.02.20.480758
42. Zwaig M, Baguette A, Hu B, Johnston M, Lakkis H, Nakada EM, et al. Detection and genomic analysis of BRAF fusions in Juvenile Pilocytic Astrocytoma through the combination and integration of multi-omic data. BMC Cancer (2022) 22:1297. doi: 10.1186/s12885-022-10359-z
43. Spies N, Weng Z, Bishara A, McDaniel J, Catoe D, Zook JM, et al. Genome-wide reconstruction of complex structural variants using read clouds. Nat Methods (2017) 14:915–20. doi: 10.1038/nmeth.4366
44. Elyanow R, Wu H-T, Raphael BJ. Identifying structural variants using linked-read sequencing data. Bioinf (Oxford England) (2017) 34:353–60. doi: 10.1101/190454
45. Fang L, Kao C, Gonzalez MV, Mafra FA, Silva RPd, Li M, et al. LinkedSV for detection of mosaic structural variants from linked-read exome and genome sequencing data. Nat Commun (2019) 10:5585. doi: 10.1038/s41467-019-13397-7
46. Wala JA, Bandopadhayay P, Greenwald NF, O’Rourke R, Sharpe T, Stewart C, et al. SvABA: genome-wide detection of structural variants and indels by local assembly. Genome Res (2018) 28:581–91. doi: 10.1101/gr.221028.117
47. Li H. Minimap2: pairwise alignment for nucleotide sequences. Bioinformatics (2018) 34:3094–100. doi: 10.1093/bioinformatics/bty191
48. Heller D, Vingron M. SVIM: structural variant identification using mapped long reads. Bioinformatics (2019) 35(17):2907–15. doi: 10.1093/bioinformatics/btz041
49. Tham CY, Tirado-Magallanes R, Goh Y, Fullwood MJ, Koh BTH, Wang W, et al. NanoVar: accurate characterization of patients’ genomic structural variants using low-depth nanopore sequencing. Genome Biol (2020) 21:56. doi: 10.1186/s13059-020-01968-7
50. Jiang T, Liu Y, Jiang Y, Li J, Gao Y, Cui Z, et al. Long-read-based human genomic structural variation detection with cuteSV. Genome Biol (2020) 21:189. doi: 10.1186/s13059-020-02107-y
Keywords: medulloblastoma, linked-reads, enhancer hijacking, extrachromosomal DNA, whole-genome sequencing, RNA sequencing
Citation: Zwaig M, Johnston MJ, Lee JJY, Farooq H, Gallo M, Jabado N, Taylor MD and Ragoussis J (2023) Linked-read based analysis of the medulloblastoma genome. Front. Oncol. 13:1221611. doi: 10.3389/fonc.2023.1221611
Received: 12 May 2023; Accepted: 06 July 2023;
Published: 28 July 2023.
Edited by:
Rengyun Liu, The First Affiliated Hospital of Sun Yat-sen University, ChinaReviewed by:
Katherine E. Miller, Nationwide Children’s Hospital, United StatesAndrea Degasperi, University of Cambridge, United Kingdom
Copyright © 2023 Zwaig, Johnston, Lee, Farooq, Gallo, Jabado, Taylor and Ragoussis. This is an open-access article distributed under the terms of the Creative Commons Attribution License (CC BY). The use, distribution or reproduction in other forums is permitted, provided the original author(s) and the copyright owner(s) are credited and that the original publication in this journal is cited, in accordance with accepted academic practice. No use, distribution or reproduction is permitted which does not comply with these terms.
*Correspondence: Jiannis Ragoussis, aW9hbm5pcy5yYWdvdXNzaXNAbWNnaWxsLmNh; Michael D. Taylor, bWR0LmNuc0BnbWFpbC5jb20=