- 1Biotechnology Research Unit, ‘A. Sforza’ Foundation, Cosenza, Italy
- 2Department of Mathematics and Computer Science, University of Calabria, Cosenza, Italy
- 3Mutagenesis and Cancer Prevention Unit, Istituto di Ricovero e Cura a Carattere Scientifico (IRCCS) Ospedale Policlinico San Martino, Genoa, Italy
- 4Tumor Epigenetics Unit, Istituto di Ricovero e Cura a Carattere Scientifico (IRCCS) Ospedale Policlinico San Martino, Genoa, Italy
- 5Molecular Pathology Unit, Istituto di Ricovero e Cura a Carattere Scientifico (IRCCS) Ospedale Policlinico San Martino, Genoa, Italy
- 6Consiglio Nazionale delle Ricerche, Istituto di Fisiologia Clinica del Consiglio Nazionale delle Ricerche (CNR), Reggio Calabria, Italy
- 7Department of Oncology and Hemato-Oncology, University of Milan, Milan, Italy
- 8Laboratory of Translational Research, Azienda Unità Sanitaria Locale - Istituto di Ricovero e Cura a Crabtree Scientifico (USL-IRCCS) of Reggio Emilia, Reggio Emilia, Italy
- 9Scientific Directorate, Azienda Unità Sanitaria Locale - Istituto di Ricovero e Cura a Carattere Scientifico (USL-IRCCS) of Reggio Emilia, Reggio Emilia, Italy
- 10Unità Operariva (UO) Molecular Pathology, Ospedale Policlinico San Martino Istituto di Ricovero e Cura a Carattere Scientifico (IRCCS), Genoa, Italy
- 11Hematology Unit, Department of Onco-Hematology, Azienda Ospedaliera (A.O.) of Cosenza, Cosenza, Italy
- 12Department of Pharmacy and Health and Nutritional Sciences, University of Calabria, Cosenza, Italy
Analyzing gene expression profiles (GEP) through artificial intelligence provides meaningful insight into cancer disease. This study introduces DeepSHAP Autoencoder Filter for Genes Selection (DSAF-GS), a novel deep learning and explainable artificial intelligence-based approach for feature selection in genomics-scale data. DSAF-GS exploits the autoencoder’s reconstruction capabilities without changing the original feature space, enhancing the interpretation of the results. Explainable artificial intelligence is then used to select the informative genes for chronic lymphocytic leukemia prognosis of 217 cases from a GEP database comprising roughly 20,000 genes. The model for prognosis prediction achieved an accuracy of 86.4%, a sensitivity of 85.0%, and a specificity of 87.5%. According to the proposed approach, predictions were strongly influenced by CEACAM19 and PIGP, moderately influenced by MKL1 and GNE, and poorly influenced by other genes. The 10 most influential genes were selected for further analysis. Among them, FADD, FIBP, FIBP, GNE, IGF1R, MKL1, PIGP, and SLC39A6 were identified in the Reactome pathway database as involved in signal transduction, transcription, protein metabolism, immune system, cell cycle, and apoptosis. Moreover, according to the network model of the 3D protein-protein interaction (PPI) explored using the NetworkAnalyst tool, FADD, FIBP, IGF1R, QTRT1, GNE, SLC39A6, and MKL1 appear coupled into a complex network. Finally, all 10 selected genes showed a predictive power on time to first treatment (TTFT) in univariate analyses on a basic prognostic model including IGHV mutational status, del(11q) and del(17p), NOTCH1 mutations, β2-microglobulin, Rai stage, and B-lymphocytosis known to predict TTFT in CLL. However, only IGF1R [hazard ratio (HR) 1.41, 95% CI 1.08-1.84, P=0.013), COL28A1 (HR 0.32, 95% CI 0.10-0.97, P=0.045), and QTRT1 (HR 7.73, 95% CI 2.48-24.04, P<0.001) genes were significantly associated with TTFT in multivariable analyses when combined with the prognostic factors of the basic model, ultimately increasing the Harrell’s c-index and the explained variation to 78.6% (versus 76.5% of the basic prognostic model) and 52.6% (versus 42.2% of the basic prognostic model), respectively. Also, the goodness of model fit was enhanced (χ2 = 20.1, P=0.002), indicating its improved performance above the basic prognostic model. In conclusion, DSAF-GS identified a group of significant genes for CLL prognosis, suggesting future directions for bio-molecular research.
1 Introduction
A precise prognostic methodology in chronic lymphocytic leukemia (CLL) patients is critical from the clinical standpoint since progression to a more advanced disease stage requires therapy and often implies an adverse prognosis. At first presentation/diagnosis, over three-quarters of CLL patients are classified as early/asymptomatic disease phase and not requiring immediate therapy (1). Although most patients have a low-risk profile as indicated by the high frequency of the immunoglobulin heavy chain variable (IGHV) gene mutated (IGHVmut) status (2) and the low del(17p) occurrence involving the TP53 locus (3), the time to first treatment (TTFT) is rather heterogeneous, and it can be partially predicted using combinations of risk-associated markers, which include staging systems and β2-microglobulin (β2-M) (2, 4–8).
Despite the proven prognostic power of this approach, the clinical course of a number of patients does not follow the pattern predicted, possibly indicating the requirement for additional prognosticators. In this respect, gene expression profiles (GEP), that is, the measurement of the activity (the expression) of all genes of interest to depict a synthetic picture of cellular function, is exploited to increase the ability to predict the prognosis of CLL patients (9–11).
Although GEP datasets represent a valuable source of information in healthcare, being currently used for diagnosis, prognosis, and precision medicine of hematological malignancies (12), their analysis results are challenging for three main reasons. The first one is the course of dimensionality: genomic-scale datasets typically consist of a very large number of features (genes) and a relatively small number of samples (patients). The second problem concerns imbalanced classes: genomics data are often collected from multiple sources and stratified based on pathologies. In most cases, there is a significant difference between the number of instances in each class. Finally, sequencing data are typically collected from multiple sources, different laboratories, and sequencing tools. This results in noisy datasets which are difficult to analyze (13).
A bioinformatic analysis is necessary to fully realize the potential of these large-scale sequencing data for prognosis in hematological malignancies (14–16) and solid tumors (17). Machine learning (ML) approaches have been widely used to enhance the performance of diagnostic and predictive models for different diseases and CLL as well (14–20). Resources and guidelines for using ML in CLL have been made available (21, 22).
However, most ML prognostic models for CLL fail to consider numerous variables and do not account for non-linear interactions between them (22). This limits the accuracy of the models and the ability to make informed predictions about the disease progression. Therefore, promising tools such as deep learning (DL) methods, a subset of ML methods based on artificial neural networks (NNs), may be used to overcome the aforementioned ML limitations. DL approaches recognize hidden patterns in large-scale datasets that are typically difficult to detect with traditional statistical and ML models. Recent studies propose and evaluate new feature selection (FS) approaches on genomic-scale datasets for cancer diagnosis and prognosis (23, 24). Such FS methodologies mainly aim at selecting the most informative genes, which can characterize classes and identify groups of patients.
Although very powerful, DL models are in general not immediately interpretable, meaning that it is difficult to understand the causal relationship between the inputs and their outcomes. This is an even more severe problem in the bioinformatics domain, where it is crucial to understand, for example, in the case of genomics, how the expression of a gene can affect the progression of oncological patients. In this context, the adoption of explainable artificial intelligence (XAI) methods has started to gain momentum for interpretability purposes as well as to enhance FS (25–27).
On the other hand, a widely used approach to overcome the course of dimensionality problem is to perform dimensionality reduction using autoencoders (AE) (24). While this has been proven effective, the encoding is typically a non-linear projection of the variables into a lower-dimensional space, making it difficult to provide the interpretations of the proper results.
This study introduces DeepSHAP Autoencoder Filter for Genes Selection (DSAF-GS), a novel DL and XAI-based FS method for genomics-scale data analysis. Such a method uses AEs for selecting the most informative genes without any change into the original features space, hence enhancing the explainability of the results and still exploiting the representation abilities of AEs. Such selection of genes is used to design and train a prediction model for diagnosis or prognosis. Eventually, the Shapely Additive ex-Planation (SHAP) (28) XAI method is applied to interpret the model results and select the most meaningful genes for the disease.
In the present paper, the proposed XAI method has been used to identify those genes whose expression levels are relevant for predicting the need of therapy in CLL patients from a prospective cohort of newly diagnosed Binet stage A CLL (O-CLL protocol) (29, 30) who are being monitored under a watch-and-wait strategy. This innovative approach enabled meaningful insights into CLL prognosis from genomic data by locating a group of significant genes to boost the prognostic power of a basic prognostic model. We point out that while our contribution is fully positioned within the research in oncology, our XAI method has broader applicability; in fact, from the bioinformatics and computational genomics point of view (18–20), an interesting avenue of further research is to assess its efficacy as a general feature-selection method, for instance, by considering datasets for which we already have some a priori semantic information on the most relevant features and by using classical comparison metrics for predictive models.
2 Materials and methods
2.1 Patients
A total of 224 of 523 newly diagnosed Binet A CLL cases belonging to the observational O-CLL1 study (clinicaltrials.gov identifier NCT00917540) were prospectively enrolled from 40 Italian institutions (29, 30) and studied for GEP. All participants provided written informed consent, and the relevant institutional review boards approved the study. The inclusion and exclusion criteria have been previously detailed (29). In particular, cases could be recruited only within 12 months of diagnosis and if they were aged <70 years and were Binet stage A. The biologic review committee confirmed the diagnosis using flow cytometry analysis and GEP analyses were centralized at Prof Ferrarini’s (Istituto Studio Tumori, Genoa, Italy) and Prof Neri’s (Fondazione Ca’ Granda, IRCCS, Ospedale Maggiore, Policlinico, Milan) labs, respectively. Recruitment began in January 2007. According to the guidelines, treatment was decided uniformly for all participating centers based on documented progressive and symptomatic disease.
2.2 Assessment of biological markers
Cytogenetic abnormalities involving deletions at chromosomes 11q23 and 17p13 were evaluated by FISH in a purified CD19+ population as previously described (31). IGHV gene mutational status was assessed on cDNA specimens (32). Sequences were aligned to the IMGT directory and analyzed using IMGT/VQUEST software. NOTCH1 mutation hotspot was set by next-generation deep sequencing as previously described (29).
2.3 GEP analysis
GEP experiments were performed as previously described (29, 30). Briefly, total RNA fraction was obtained from CD19+-enriched B-cell samples (EasySep-Human B cell enrichment kit without CD43 depletion, Stem Cell Technologies, Voden Medical Instruments S.p.A, Milan, Italy) using the fully automated protocol of immunomagnetic cell separation with RoboSepTM (Stem Cell Technologies). Purified B-cells (CD19+) exceeded 95% were employed as total RNA sources for GEP analysis.
Preparation of DNA single-stranded sense target, hybridization to GeneChip® Gene 1.0 ST Array (Affymetrix, Santa Clara, CA), and scanning of the chips (7G Scanner, Affymetrix) were carried out according to manufacturer’s protocols. RNA fraction was obtained from samples using Trizol reagent (Life Technologies, Monza, Italy). RNA quality was assessed using the Agilent 2100 Bioanalyzer (Agilent Technologies). The raw intensity expression values were processed by robust multi-array average (RMA) procedure 19 with the reannotated Chip definition files (CDF) from BrainArray libraries version 15.0.0 20 available at http://brainarray.mbni.med.umich.edu, as previously described (22). The gene and miRNA expression data have been deposited at the National Center for Biotechnology Information (NCBI) Gene Expression Omnibus repository (http://www.ncbi.nlm.nih.gov/geo/) and are accessible through GEO Series accession number GSE40570.
2.4 O-CLL dataset
For each patient, 19,367 genes profiles were provided. Patients are labeled according to the occurrence of an event (or not). The considered outcome was the need for therapy starting or death [dichotomous, not (event=0) vs yes (event=1)]. From the 217 patients in the final dataset, 120 were labeled as event=0 and 97 as event=1.
2.5 Feature selection
GEP studies generate large, high-dimensional, and unbalanced datasets, where each sample can have up to thousands of variables. This results in high computational costs and the possibility of overfitting. Such overfitting may mistake small changes in the data as significant differences, leading to misclassification errors. This study addresses these risks by applying FS techniques to reduce the dimensionality problem by selecting the most relevant features and removing noise and redundancy. FS techniques can be filter-based, wrapper, or embedded (33–35). The integration of multiple FS techniques is denoted as hybrid FS.
The proposed DSAF-GS approach is a hybrid FS method that combines filter-based and wrapper techniques to achieve a representative and meaningful subset of genes. DSAF-GS uses autoencoders (AE) as wrappers along with statistical filters to remove redundant genes. An NN is trained on the remaining genes as an event predictor. Finally, the SHAP XAI method is used to evaluate the contribution of genes to NN decisions. The genes with the strongest contribution are selected.
2.6 Neural networks
NNs are ML computational models inspired by the structure and function of the human brain. They consist of consecutive layers of interconnected artificial neurons, which process and transmit information through weighted connections. Training an NN amounts to providing a dataset of input-output pairs and identifying, via proper optimization methods, the NN parameters that minimize some given loss function, usually meant to measure the distance between the output at hand and the result of the NN computation on the given input. In a research context, NNs can be trained on large datasets of genetic data to identify patterns and predict the effects of genetic mutations on traits of interest (36–38). These predictions can then be used to understand further the genetic basis of diseases (38) and other phenotypic traits (39, 40) and inform the development of personalized medical treatments (37, 41). In addition, by using NNs and other computational and experimental methods (e.g. clustering and statistical analysis, such as F-test or XAI) researchers can gain deeper insights into the complex interactions between genetics and biology.
Autoencoders (AE) are particular architectures of NNs that uncover the underlying structure of the data and generate a latent code for further analysis (42). An AE maps an input to a lower dimensional representation (latent code). Such code is expected to have uncorrelated features, being able to reconstruct the original input data. Therefore, AEs can be used for dimensionality reduction, denoising, and data generation.
2.7 SHapley additive exPlanation
The black-box nature of NNs often limits the interpretability of their results. Advances in XAI provide various methods for interpreting black-box models, offering a clearer understanding of their predictions. For example, Shapely Additive ex-Planation (SHAP) is a game theory-based approach for interpreting black-box models. SHAP determines the importance of a feature by observing the variations in predictions when the feature is included or excluded from the model. It assigns an importance value, called a SHAP value, to each feature, based on its contribution to the predictions (28). SHAP values are quantitative estimates, indicating ‘how’ and ‘how much’ every single gene contributes to the model decisions, providing insight into the gene’s role in the event prediction. The SHAP method provides a way to understand the underlying workings of NNs predictions, leading to improved insights and better decision-making.
An alternative XAI approach, named LIME (Local Interpretable Model-Agnostic Explanations) (43), approximates the behavior of complex models via local interpretable explanations. Such explanations are obtained by fitting simpler and interpretable surrogate models with perturbed input data and observing the resulting changes in the model’s predictions.
While LIME approximates the behavior of complex models with simpler ones, SHAP provides a more direct and explicit connection between feature importance and predictions. This transparency, along with a solid theoretical foundation rooted in game theory, enhances a deeper understanding of the underlying mechanisms driving the model’s decisions.
2.8 Proposed algorithm
DSAF-GS consists of the following steps (Figure 1):
1. The pairwise correlation (Pearson) was computed over the whole set of genes.
2. The resulting correlation matrix was clustered using hierarchical clustering such that similarly correlated genes belong to the same cluster.
3. For each cluster, all patient data were retrieved from the original dataset. An AE is then trained for each cluster using patients as features and genes as samples. The AEs selected the most representative gene of the respective cluster. The most representative gene was the one associated with the lowest reconstruction error. This step eliminates redundant genes hence reducing the dimensionality, still working at the level of the original feature.
4. Genes selected in the previous step were then ranked with the F-test. The F-value was computed by considering, for each gene, the ratio of the variance between and within the groups (event=0 and event=1). A subset of genes with the highest F-value was then selected. The final subset size was empirically selected by considering different subset sizes.
5. A NN was trained to perform binary classification on the event class, using the previously selected set of genes as input variables and by considering the standard binary cross-entropy loss function. A model selection phase identified the most appropriate architecture of the NN. A grid-search approach is applied over a hyperparameters space defined by the number of layers and neurons per layer. In particular, we considered 2, 3, and 4 layers and 8, 16, 32, 64, 128, and 256 neurons for the first layer. In every successive layer, the number of neurons was determined as half of the number of neurons in the preceding layer. For each given configuration (layers/neurons), we built the corresponding NN whose performances were evaluated using a cross-validation (cv) algorithm, which assesses the model’s ability to generalize by repeatedly training and testing on different subsets of the data for multiple iterations. The hyperparameters configuration is chosen as the one with the highest average performances (according to the average binary accuracy) over the cv iterations; the best model is finally selected as the one with the best performances among the cv iterations of the chosen configuration.
6. SHAP XAI method was used to explain the chosen NN classifier for the CLL event. SHAP evaluates the importance of each gene on the predictions by providing information on how such genes affect the prognosis.
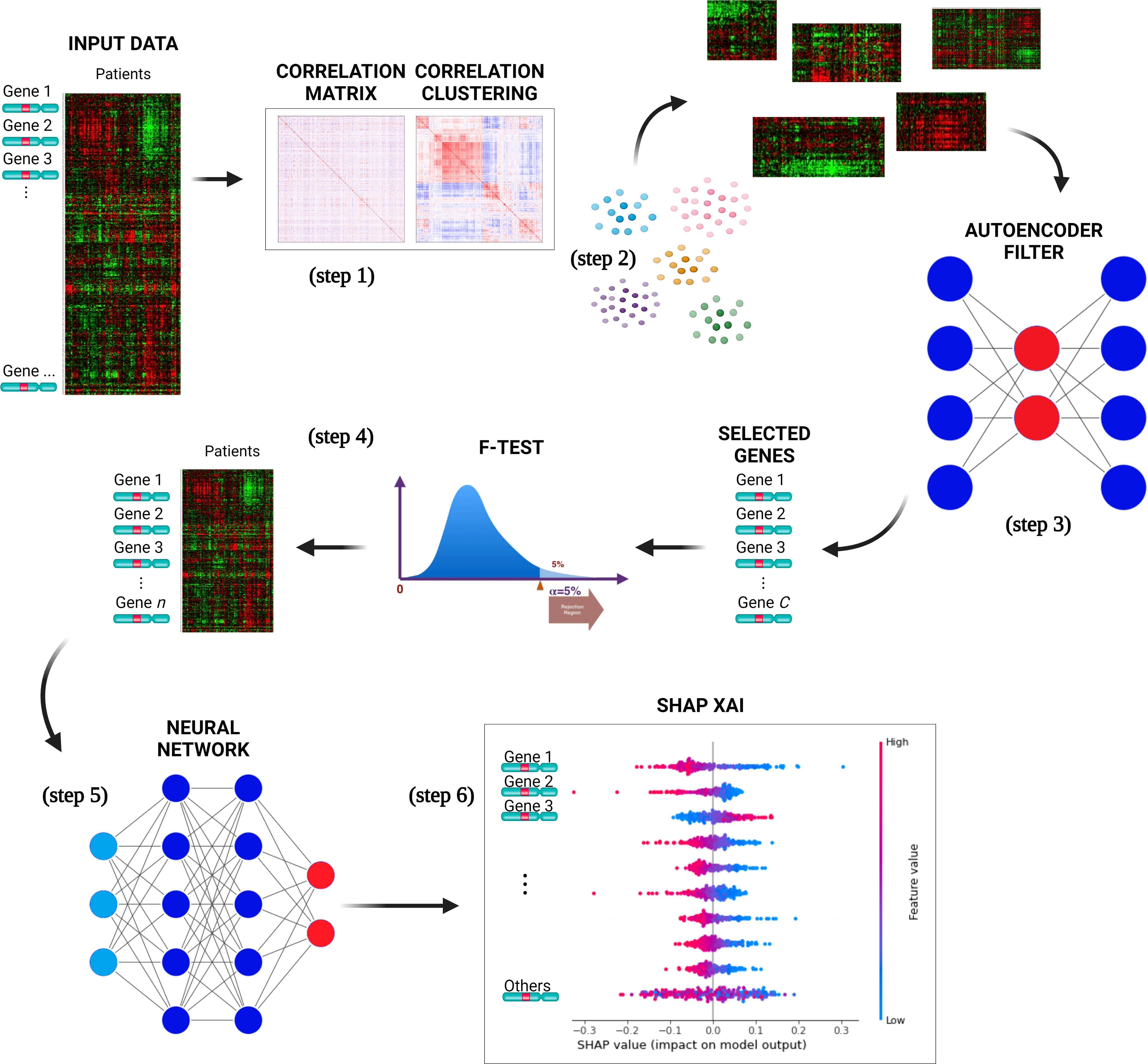
Figure 1 The pipeline proposed for selecting a subset of genes relevant to predict CLL events. The input data is used to compute the genes pairwise correlation matrix (step 1), and the correlation matrix is clustered (step 2) to group similarly correlated genes. The clusters are then mapped to the original input data and transposed. AEs are trained for each cluster to select the most representative gene, reducing dimensionality (step 3). The genes are ranked with F-test, selecting a subset with the highest F-value (step 4). A neural network is trained with a selected set of genes to perform binary classification of the CLL patients (event=0 and event=1) (step 5). The best NNs architecture is determined through model selection, and the SHAP XAI method explains each gene’s importance in the predictions (step 6).
2.9 Implementation
The DSAF-GS algorithm has been implemented using the Python (v3.8.11) programming language. NNs have been implemented using the Tensorflow (v2.6.0) framework and the Keras library. XAI analysis was performed using the SHAP (28) library.
For GEP analysis, 500 clusters were identified (step 2). Each AE architecture (step 3) consisted of five layers with the following number of neurons: 217, 43, 21, 43, and 217, respectively; relu was used as activation function and Adam as optimizer with a learning rate of 0.01; Mean Absolute Error was used as reconstruction loss, and each AE was trained for 1000 epochs. Out of the 500 genes selected by the AEs (one for each cluster), different subset sizes were used to train the NN event predictor. The F-test (step 4) was used to select a subset of genes of size 5, 10, 50, 100, and 300 (Table 1). Different NNs were trained (step 5) for each gene subset. The best model for GEP takes in 50 genes as input and consists of 4 layers of 46, 22, 12, and 1 neuron, respectively.
During grid-search (step 5), Adam was used as an optimizer with a learning rate of 0.001, and relu was used as an activation function. A total of 10 cv iterations have been performed for each configuration by randomly splitting the data into a training set and test set (90% and 10% of the whole dataset, respectively). While the training set has been enriched with synthetic samples (by using SMOTE) (44) to guarantee a balanced training of the NN, the test set only comprised real data samples. While the computation of SHAP values was found to be inefficient for NN models, the authors (36) demonstrated that Shapley values could be calculated through a weighted linear least square regression with a shapely kernel. Such a method was adopted for computing SHAP values (step 6) using a subsample of 100 patients. Note training and test sets are different parts of the same O-CLL dataset. Indeed, we are not aware of any further public dataset having the clinical and genomic information required by our method and that can be used as a validation set, by fitting our needs and the prospective nature of our study.
2.10 Statistical analysis
TTFT was calculated during the watch and wait period from the date of the diagnosis to the date of therapy start or last follow-up. The prognostic impact of predictors was investigated by univariable and multiple Cox regression analysis. Data were expressed as hazard ratio (HR) and 95% confidence intervals (CIs). The predictive accuracy of the prognostic models was quantified by calculating Harrell’s c-index (HC-index), ranging from 0.5 to 1.0, and the explained variation on the outcome (i.e., an index combining calibration and discrimination) (45). The improvement of model fitting due to the inclusion of specific genes was assessed by the log-likelihood ratio statistics. The receiver operator curves (ROC) Figure 2B illustrate the model performance by plotting the actual positive rate (sensitivity) versus the false positive rate (1 - specificity). A value of P <.05 was considered significant for all statistical calculations. Data analysis was performed by SPSS for Windows v.21, IBM, Chicago, Illinois, USA, and by Stata 16, StataCorp, Texas, USA.
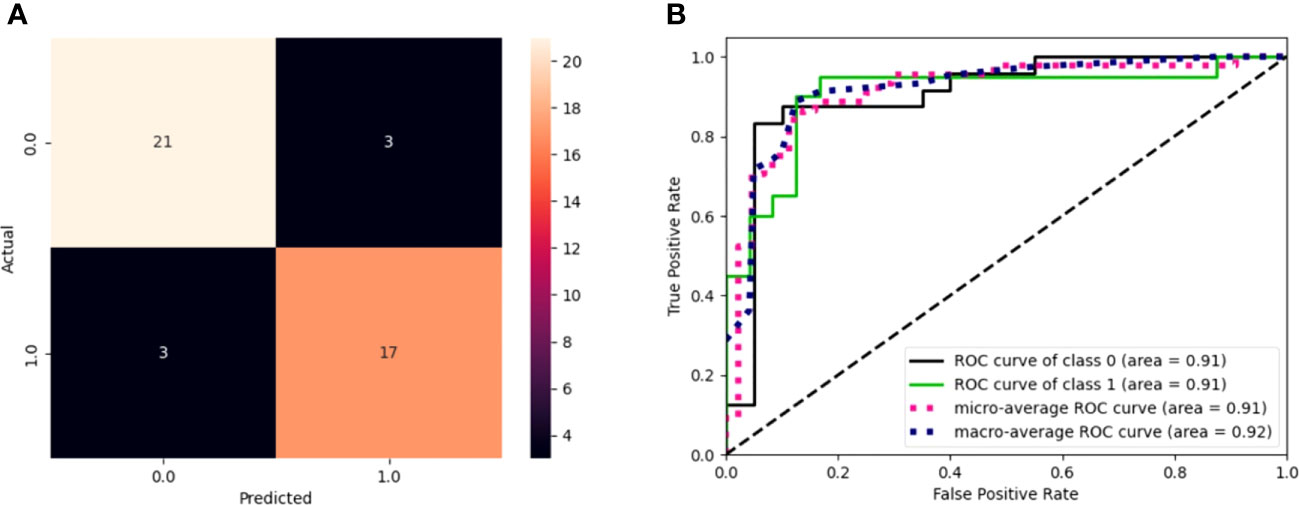
Figure 2 (A) Confusion matrix of model performance on the test set in predicting the event or non-event of new patients. Black squares refer to wrongly classified patients (false positives and false negatives), while colored squares refer to well-classified patients (true positives and true negatives). (B) ROC curves for the model. The graph plot sensitivity against specificity at various threshold settings. The classifier performs better as the curve approaches the upper left corner. An AUC value of 0.91 for GEP predictions indicates the solid overall performance of the model.
2.11 Pathway and gene network analysis
Pathway analysis was performed using the Reactome Pathway Analysis tool (reactome.org) to group genes into specific pathways. Reactome analysis with statistical hypergeometric distribution test determines whether certain pathways are over-represented in the submitted data. This test produces a probability score, which is corrected for false discovery rate using the Benjamani-Hochberg method (46, 47).
Gene network was constructed with the free NetworkAnalyst tool (48) using IMEx Interactome [Literature-curated comprehensive data from InnateDB (49)].
3 Results
3.1 Gene selection by explainable artificial intelligence
Of the 224 enrolled patients, 217 were used for the analysis. The remaining 7 were removed for defective gene profiles. The performance of the best model on the test set is reported in Table 1. The results are shown according to the number of genes used as independent variables (first column). The best model accuracy is 86.36%, achieved using 50 genes as predictors, with a sensitivity and specificity of 85% and 87.5%, respectively. The second column reports the 95% CIs computed using the model accuracy’s mean and standard deviation over the cv iterations.
The model sensitivity and specificity are reported in Figures 2A, B, in terms of a confusion matrix and ROC curve, respectively. Despite the three false positives and three false negatives, the model is capable of detecting the underlying patterns in the data, as shown by the overall performance. Figure 2B shows that the models have an area under the curve (AUC) of 0.91.
Figure 3A shows a waterfall plot of absolute mean SHAP values, reporting the average importance of each gene in the model, evaluated using SHAP. Genes are reported in order of importance. For example, the model was strongly influenced by CEACAM19 and PIGP (+0.09 and +0.08), moderately influenced by MKL1 (alias of MRTFA gene) and GNE (+0.04), and poorly influenced by others (<0.03).
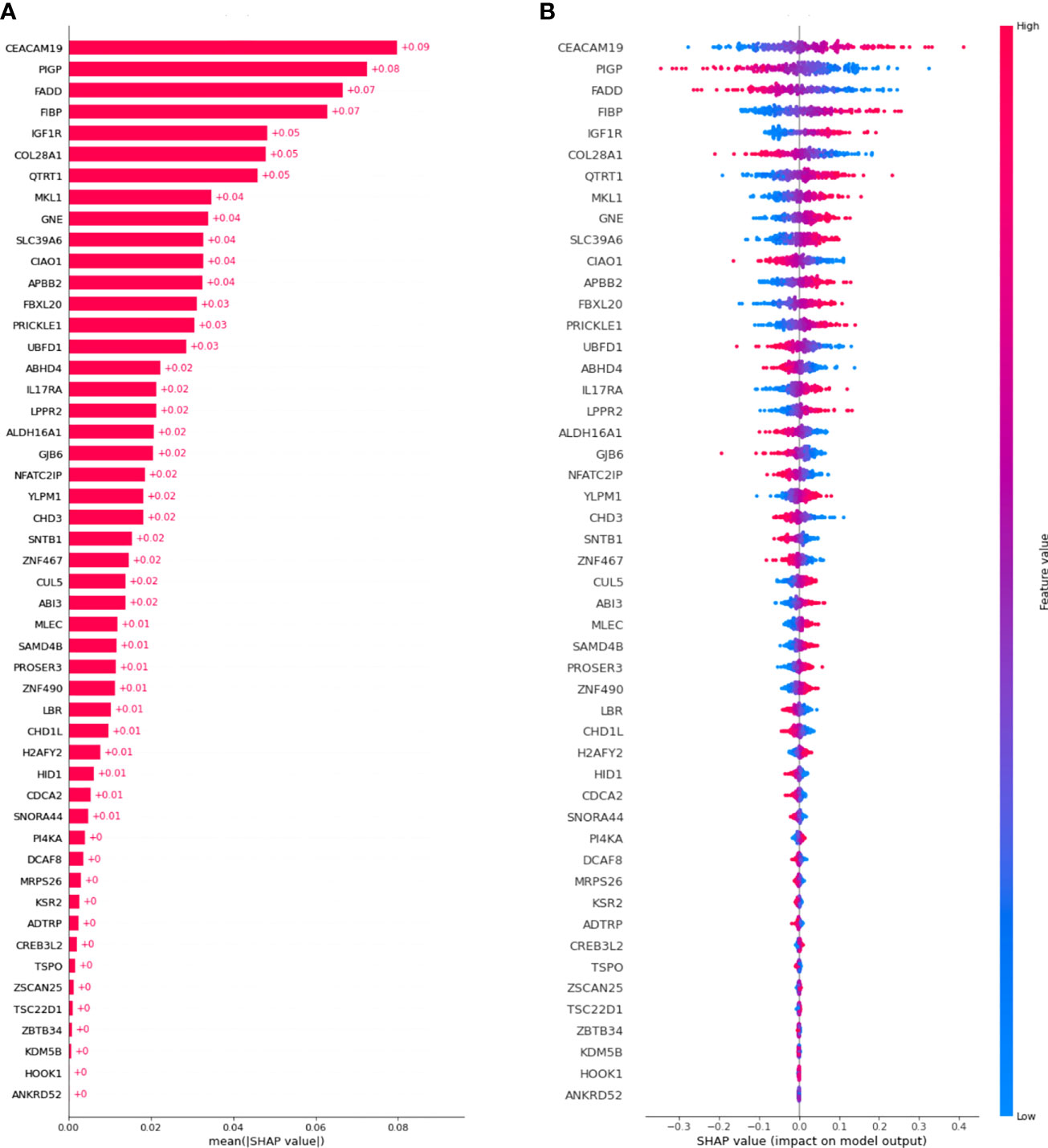
Figure 3 SHAP values were computed for the best model. (A) Waterfall plot of absolute mean SHAP values (average absolute importance of each gene in the model), (B) Beeswarm plot of SHAP values (shows how and how much each gene influences the predictions).
Moreover, as shown in Figure 3B, higher values of the gene CEACAM19 are associated with positive SHAP values, meaning that they will increase the prediction towards the occurrence of the event. Moreover, lower values of the variable are associated with negative SHAP values, meaning that they will decrease the prediction towards the absence of an event. Conversely, for the PIGP gene lower values are associated with positive SHAP values, increasing the prediction towards the event’s occurrence. PIGP higher values are associated with negative SHAP values, meaning they will decrease the forecast towards no event occurrence.
3.2 Pathways and networks overview based on Reactome database of the top 10 genes
The description and chromosome localization of the top ten genes are described in Table 2. Eight of the top ten genes (i.e., FADD, FIBP, FIBP, GNE, IGF1R, MKL1, PIGP, SLC39A6) selected by the NN algorithm were found in the Reactome pathway database, showing involvement in various pathways such as signal transduction, gene expression (transcription), protein metabolism, immune system, cell cycle and apoptosis (Figure 4).
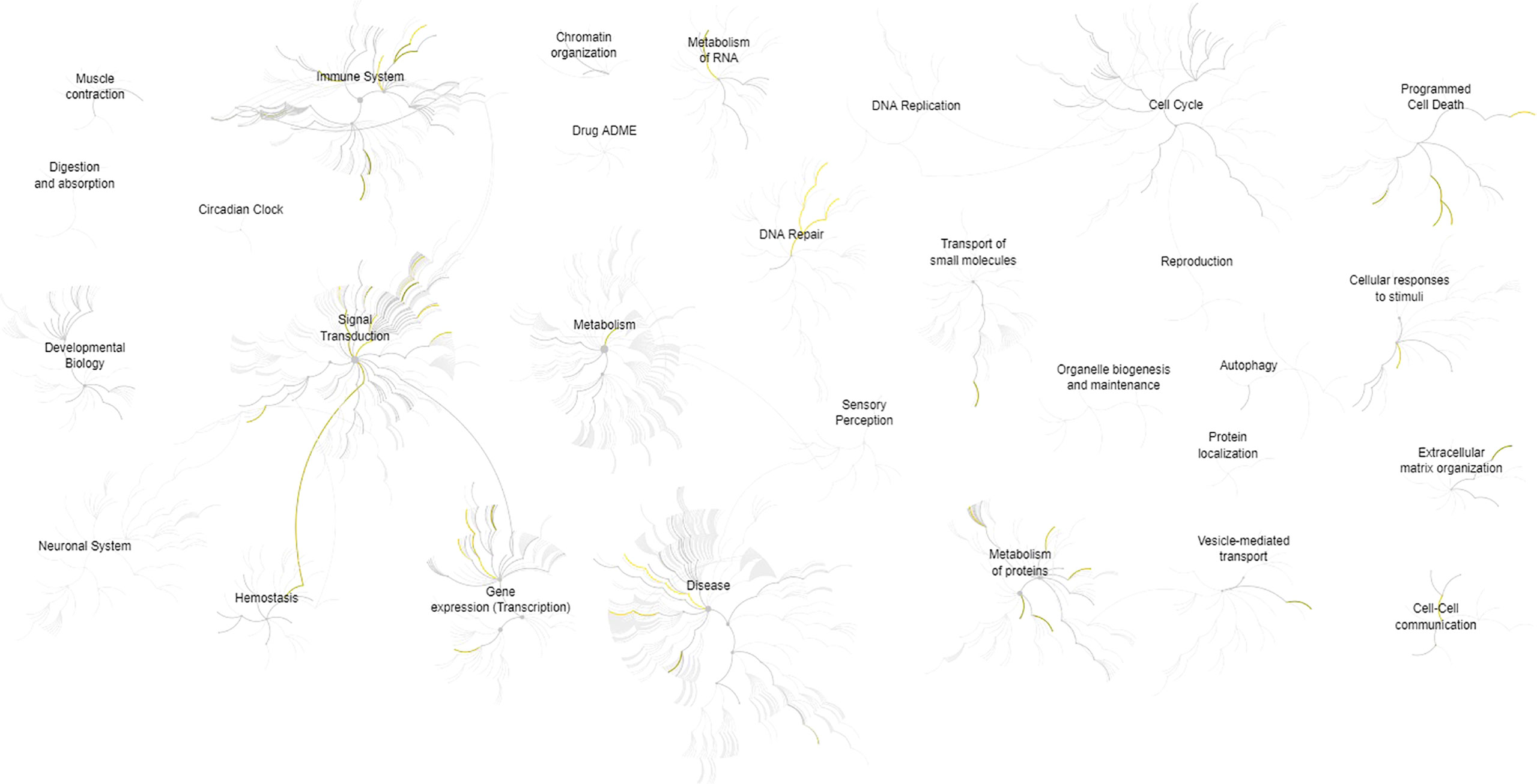
Figure 4 Pathways overview based on the Reactome database of the top 10 genes identified by the (SHAP) XAI method. A genome-wide overview of the results of pathway analysis is shown. Reactome pathways are arranged in a hierarchy. The center of each of the circular “bursts” is the root of one top-level pathway, for example, Cell Cycle. Each step away from the center represents the next lower level in the pathway hierarchy. The color code denotes the over-representation of that pathway in the input dataset. The closer the color is to yellow, the more significant the over-represented pathway is; light grey indicates pathways that are not significantly over-represented.
Interestingly, seven (i.e., FADD, FIBP, IGF1R, QTRT1, GNE, SLC39A6, MKL1) of the top 10 genes appear to be connected into a complex network as shown in Figure 5 by the network model of the 3D protein-protein interaction (PPI) explored using the NetworkAnalyst tool (48).
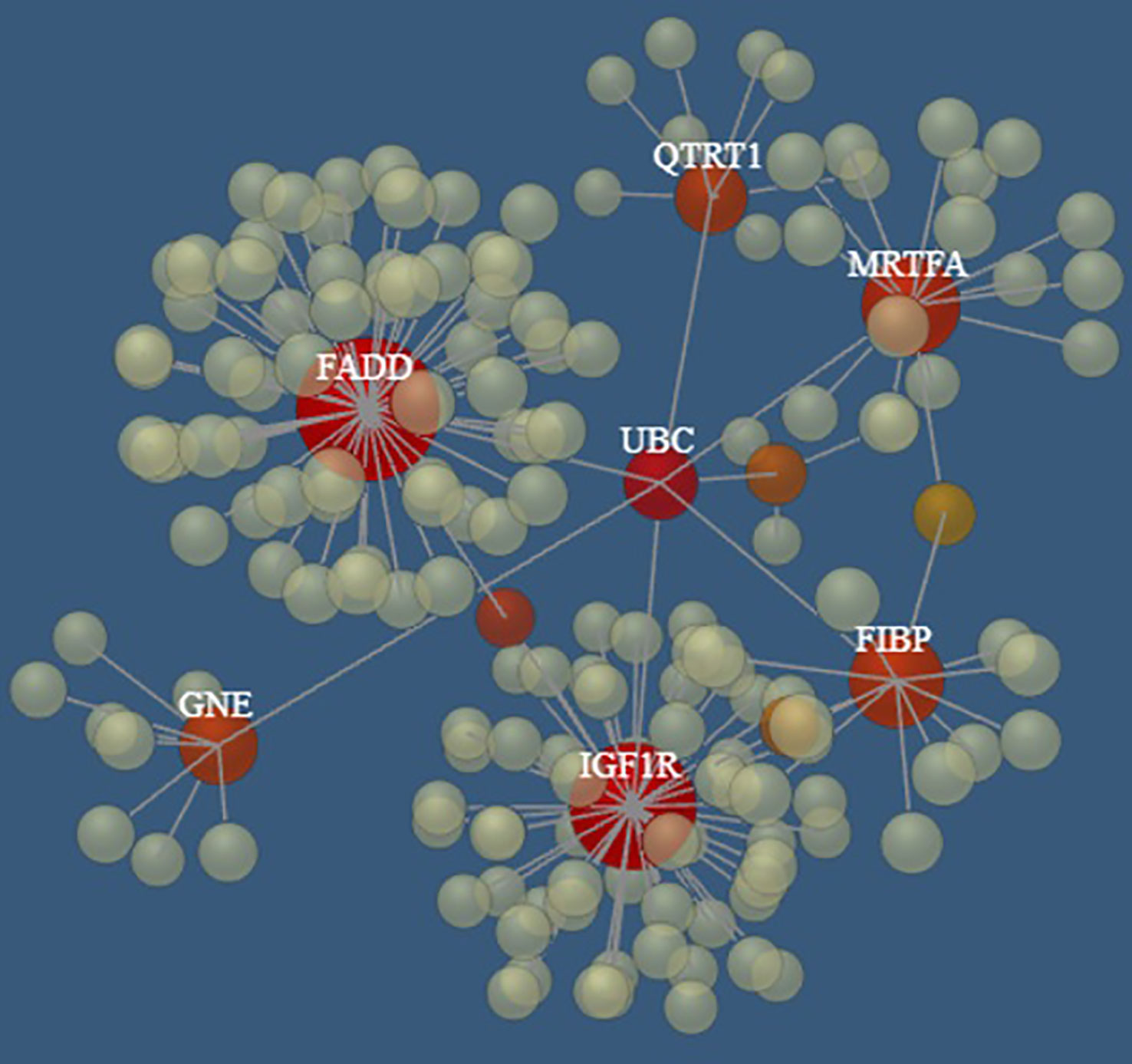
Figure 5 The PPI networks created by FADD, FIBP, IGF1R, QTRT1, GNE, SLC39A6, and MRTFA genes. Node size and color correspond to the number of connected edges; gene name is displayed only for nodes with ≥ 4 edges, and the closer the color is to red, the bigger the node size is.
3.3 Multivariate analysis of the top 10 genes
The top 10 genes selected by the NN models were chosen to estimate their prognostic influence on noticeable clinical and biomolecular variables (named basic prognostic model) consisting of IGHV mutational status, del(11q) and del(17p), NOTCH1 mutation, β2-M, Rai stage, and B-lymphocytosis. Table 3 shows their prediction power on TTFT in univariable analysis.
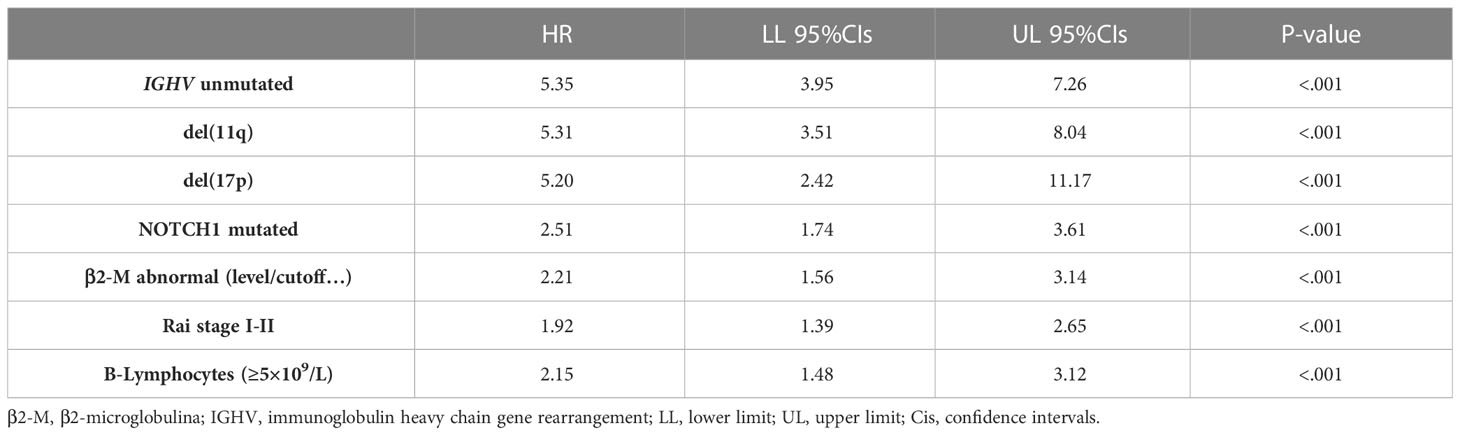
Table 3 Univariable Cox analyses for time to first treatment of several well-known clinical and biomolecular variables belonging to the basic prognostic model.
As expected, all ten top genes showed a predictive power on TTFT in univariable analyses (Figure 6). Specifically, for COL28A1(HR 0.32, 95% CI 0.12-0.82, P=0.018), FADD (HR 0.21, 95% CI 0.07-0.62, P=0.005), and PIGP (HR 0.39, 95% CI 0.15-0.98, P=0.047) high expression was associated with a reduced risk to be treated, while the remaining genes showed an inverse prognostic association with therapy need. However, CEACAM19 (HR 2.44, 95% CI 0.84-7.14, P=0.10), PIGP (HR 0.57, 95% CI 0.19-1.72, P=0.32), FADD (HR 0.45, 95% CI 0.12-1.64, P=0.22), FIBP (HR 1.95, 95% CI 0.96-3.96, P=0.06), MKL1 (HR 2.31, 95% CI 0.73-7.34, P=0.15), GNE (HR 1.86, 95% CI 0.82-4.26, P=0.14) and SLC39A6 (HR 1.44, 95% CI 0.64-3.22, P=0.37) lost their independent predictive power when analyzed with variables belonging to the basic prognostic model. Conversely, IGF1R (HR 1.41, 95% CI 1.08-1.84, P=0.013), COL28A1 (HR 0.32, 95% CI 0.10-0.97, P=0.045), and QTRT1 (HR 7.73, 95% CI 2.48-24.04, P<0.001) genes were significantly associated with TTFT in multivariable analyses (Table 4). When these three significant genes were evaluated in a final multivariable model, including the basic prognostic variables, COL28A1, along with B-lymphocytosis, lost its significance, while IGF1R and QTRT1 maintained their prognostic independence (Table 4).
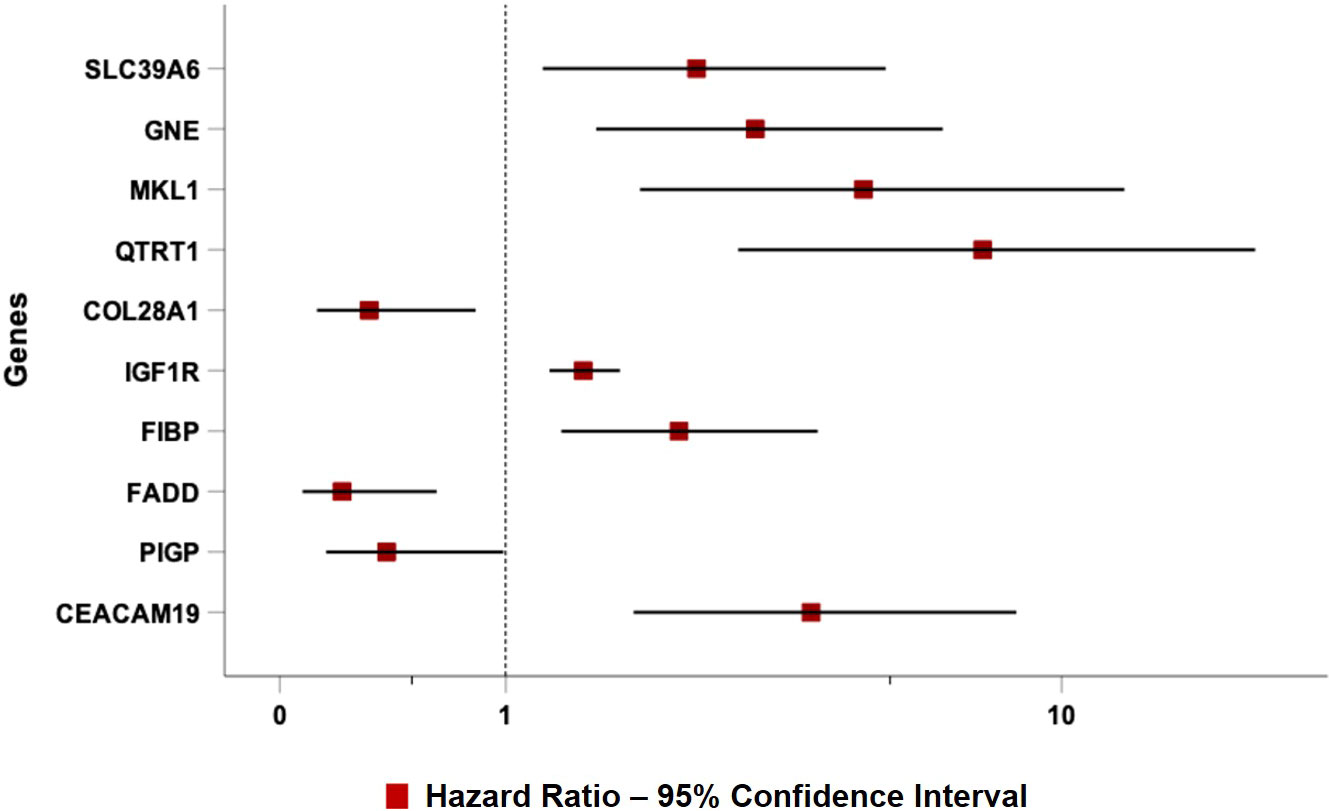
Figure 6 Forest plot of Cox univariable analysis for time to TTFT according to the top 10 genes selected by the NN algorithm.
The basic prognostic model provided an HC-index of 76.5% and an explained variation to predict the TTFT of 42.2%. When the three significant genes (i.e., IGF1R, COL28A1, and QTRT1) were jointly considered in the final multivariable model, the HC-index and the explained variation significantly increased to 78.6% and 52.6%, respectively, along with an improvement of the goodness of model fit (χ2 = 20.1, P=0.002). In a more parsimonious model, only including IGF1R and QTRT1 (i.e., the two genes that remained significantly associated with the TTFT in the final model) and excluding CLO28A1, the HC-index (78.2%) and the explained variation (52.4%) retained a better performance as compared with the basic prognostic model, with a concomitant rise in the goodness of the model fit (χ2 = 18.8, P=0.001).
4 Discussion
The considerable innovations in genomics engendering a vast and miscellaneous bulk of information from sizable cohorts of patients and the concurrent computer science knowledge improvements have guided the growing use of AI and, more specifically, of ML approaches that acquire knowledge from available data, devising variable selections without pre-setting programming (50). Well-defined examples of the ML approach in the analysis of hematological malignancies are the association of BCL6 and PDL1/2 rearrangements in primary testicular diffuse large B-cell lymphoma (DLBCL) with central nervous system relapse (51); the involvement of six prognosis-related long non-coding genes in acute myeloid leukemia (AML) patients (52); or the relevance of tumor mutation burden for the DLBCL overall survival prognostication (53) are. In CLL, the ML algorithm identified six hub genes as possible biomarkers to improve the diagnosis (14). Moreover, baseline clinical data added to the international prognostic index for CLL (CLL-IPI) variables demonstrated improved predictive performance over CLL-IPI, using a range of ML boosting algorithms to identify the individual risk of death, treatment, infection, and a combination of them (16). In contrast, no additional improvement was observed when comprising recurrent genetic mutation information (16). Moreover, an ML algorithm called CLL Treatment Infection Model (CLL-TIM) was applied to recognize patients at high risk of infection and/or treatment based on CLL-IPI variables and routine clinical data (17).
However, differently from our prospective study, the CLL-IPI score system only included 32% of Binet stage A patients and, more importantly, 4% of IGHV mutated cases, thereby rendering it less representative of the real-world setting and may lower the TTFT’s predictive efficacy. In contrast, the Brno-Barcelona cohort (54) had a significantly higher proportion of early-stage/low-risk Binet A (83%) and IGHV mutated cases (43%), as did the German CLL study group which also developed a predictive model for newly diagnosed Binet stage A patients (55), with roughly 71% of the population having an IGHV mutation status.
Herein, we selected the top 10 genes (CEACAM19, PIGP, FADD, FIBP, FIBP, GNE, IGF1R, MKL1, PIGP, and SLC39A6) from a GEP dataset of 217 CLL cases comprising roughly 20,000 genes using a novel deep ML-based approach to estimate how much every single gene had a role in predicting the therapy need occurrence. The GEP model was strongly influenced by CEACAM19 and PIGP (SHAP value +0.09 and +0.08) in making decisions, moderately influenced by MKL1 and GNE (SHAP value +0.04), and poorly influenced by the others (SHAP value <0.03). IGF1R, COL28A1, and QTRT1 moderately influenced quite the GEP model (SHAP value +0.05).
Some variables, namely Rai stage, IGHV mutational status, β2-M, and 17(p) and 11(q) deletions previously validated in the CLL-IPI score system (5, 56) and by our group (57, 58), were used as a basic risk model in predicting TTFT. We found that IGF1R, COL28A1, and QTRT1 genes maintained their own independent prognostic value in predicting the time-to-event when tested in a multivariable model, including the variables of the basic prognostic model. However, in a final multivariable model, in which the three genes (IGF1R, COL28A1, and QTRT1) were tested all together with the prognostic variables of the basic model IGF1R and QTRT1, but not COL28A, maintained their predictive independence on TTFT. Notably, the presence of these genes in the model significantly increased the prognostic accuracy of a basic risk model. In this regard, the HC-index and the explained variation significantly increased from 76.5% in the basic model to more than 78% and from 42.2% to roughly 52% in the IGF1R/QTRT1-gene model, respectively. These data indicate that the IGF1R/QTRT1-gene model retained a better performance than the basic prognostic model.
IGF1R encoding the insulin-like growth factor 1 receptor (IGFR1) is not only implicated in numerous cellular bio-functional processes, i.e., growth, proliferation, differentiation, and apoptosis (59), but also it plays a critical role in cancer development, progression, and metastasis (60). Moreover, IGF1R is involved in CLL (61–63) and overexpressed in various CLL cell subsets. Its inhibition induced apoptosis and efficiently reduced CLL growth in an Eμ-TCL1 transgenic murine model (62). Moreover, IGF1R seems to be a direct target of sorafenib since the latter decreased its expression and phosphorylation by offsetting the insulin-like growth factor-1 binding to CLL cells and ultimately dropping the in vitro IGF1R kinase activity (62). Finally, we previously demonstrated the IGF1R gene expression as an independent prognostic factor related to TTFT in our O-CLL prospective cohort after a shorter follow-up (63).
Unlike the IGF1R gene, QTRT1 encoding the queuine tRNA-ribosyltransferase 1, a key enzyme involved in the post-transcriptional modification of tRNAs (64), has never been implicated in the pathogenesis or prognosis of CLL. Conversely, a significant increase in QTRT1 expression and a striking down-regulation in its methylation was also found in lung cancer (65). Furthermore, it was discovered to be a risk factor for the disease onset and progression and adversely associated with survival outcomes (65).
Among various CLL prognostic models involving genes (66–69), Herold et al. (11) provided evidence of the association between overall survival and TTFT and the expression of 8 genes in CLL cells (PS.8 score). For TTFT, PS.8 showed an improved prognostic effect than the single parameters and even to a combined FISH and IGVH status model, which, in turn, failed to increase the performance of the PS.8 score in a multivariable analysis.
Huang et coll (70). showed that high NRIP1, BCL11B, and SIRT1 expressions were associated with more prolonged survival, while high expression of CDKN2A and SREBF2 with a poor prognosis (70). However, a substantial fraction of patients in the dataset chosen by the authors was not analyzed at the diagnosis/first presentation but at the time of progressive disease or relapse (70). Conversely, patients of our O-CLL cohort were prospectively followed-up, and all the biomolecular analyses were performed at the disease onset. Moreover, both Herold’s (11) and Huang’s (70) studies did not consider, unlike our study, unavailable risk factors included in the CLL-IPI score, somewhat misinterpreting the final results.
Two recently published articles represent interesting innovations in CLL’s gene-oriented prognosis (71, 72). Liang X et al., following the super-enhancer (SE) new hypothesis, generated a prognostic score to predict the time-to-therapy-need in CLL by the expression levels of nine SE-associated genes (71). Yet, since several data suggest the high dependency of CLL cells on microenvironment support, Abrisqueta and coll (72). described the prediction power of a signature for predicting progression based on the analysis of two hundred genes linked to microenvironment signaling by the NanoString approach. This novel approach established a 15 genes-based signature that predicted disease outcome independently of the IGHV mutational status, the CLL-IPI, and the International Prognostic Score for Early-stage (IPS-E) CLL score (72). Notably, the nanoString platform, overcoming GEP methodological drawbacks and reproducibility, could represent the future, facilitating its use in clinical settings.
Notably, several pathways involved in cancer and hematopoietic malignancies development were identified by Reactome analysis of the top ten genes analyzed in this study, including Interferon alpha/beta signaling (73–75), caspases and Rho GTPase activity (76), GHR signaling pathway (77–79), Integrin signaling (80), non-receptor Tyrosine Kinases activity (81), and FGF/FGFR pathways (82). Moreover, among the top ten genes, FIBP was found to be overexpressed in a specific group of CLL patients affected by a large loss at the 13q14 locus (83); as previously noted also, IGF1R was identified as overexpressed in various CLL subsets, suggesting a contribution to CLL pathology (63, 81, 84, 85). Finally, seven of the top 10 genes (which appeared to be connected in a complex PPI network) are, in turn, interconnected through the UBC gene, encoding for Polyubiquitin C, which represents one of the sources of ubiquitin in human cells. Polyubiquitin C plays a crucial role in maintaining cellular ubiquitin levels, especially during the stress response. The process of ubiquitination has been associated with protein degradation, DNA repair, cell cycle regulation, kinase modification, endocytosis, and regulation of other cell signaling pathways (86). Interestingly, Zhang et al. (87), through bioinformatic analysis of gene expression profiles in CLL cells, identified the UBC gene as the key node in the PPI network of genes up-regulated in B cells co-stimulated with immobilized anti-IgM with respect to untreated cells, revealing the proteasome pathway as the most significant in this network.
Finally, it should be emphasized that this preliminary study lacks a validation cohort. To the best of our knowledge, we are not aware of any further public dataset fitting the prospective nature of our study as well as the clinical and genomic information required to answer our aims. Specifically, the GEO dataset (GSE39671) (88) does not have the characteristics required to run the method presented in this article but the characteristics are instead collected in the ICGC CLL dataset (89, 90). However, in the latter, sampling was completed within a year, as in our study, in approximately 26% of Binet A untreated CLL cases. In contrast, the median sampling time for the remaining cases of the ICGC CLL cohort was approximately 5 years (IQR 2.6-9.1), a bias that might invalidate the analysis’ conclusions. Moreover, information on the Rai stage system is lacking and the Binet stage information at sampling is not available.
5 Conclusions
A novel deep ML-based approach was proposed in the current analysis, exploiting the reconstruction capabilities of AEs and XAI to select the most informative genes for predicting the therapy need event. This study’s strengths lie in the use of an original ML method and the prospective nature of our study. The results, although preliminary, evidenced the effectiveness of this approach in identifying genes with independent predictive power, suggesting a set of meaningful genes for further investigation. Finally, it should be emphasized that this pilot study requires external validation using a different prospective cohort of patients with similar characteristics. Finally, it should be emphasized that this pilot study requires external validation using a different prospective cohort of patients with similar characteristics.
Data availability statement
The original contributions presented in the study are included in the article/supplementary material. Further inquiries can be directed to the corresponding authors.
Ethics statement
The studies involving humans were approved by Unità di Ematologia e Trapianto di Cellule Staminali, Istituto di Oncologia “Giovanni Paolo II”, Bari comitato etico Istituto di Oncologia “Giovanni Paolo II”, di Bari, Italy; Dipartimento di Ematologia, Ospedale di Venere, Bari comitato etico Ospedale di Venere, Bari, Italy; Unità Operativa Complessa Ematologia e Trapianto, Ospedale “Mons. R. Dimiccoli” - Barletta comitato etico Ospedale “Mons. R. Dimiccoli” - Barletta, Italy Divisione di Ematologia, Presidio Ospedaliero “A. Perrino”, Brindisi comitato etico Presidio Ospedaliero “A. Perrino”, Brindisi, Italy; Unità Operativa Complessa di Oncoematologia Ospedale “S. Anna e S. Sebastiano”, Caserta comitato etico Ospedale “S. Anna e S. Sebastiano”, Caserta, Italy; Divisione di Ematologia, Università di Catania Ospedale Ferrarotto, Catania comitato etico Ospedale Ferrarotto, Catania, Italy; Unità Operativa Complessa di Emato-Oncologia, Ospedale Garibaldi-Nesima, Catania comitato etico Ospedale Garibaldi - Nesima, Catania, Italy; Dipartimento di Oncologia ed Ematologia, Pugliese-Ciaccio Hospital, Catanzaro comitato etico Pugliese-Ciaccio Hospital, Catanzaro, Italy; Unità Operativa Complessa di Ematologia, Azienda Ospedaliera Cosenza comitato etico Azienda Ospedaliera Cosenza, Italy; Unità Operativa Complessa di Oncologia, Ospedale Giannettasio, Rossano Calabro, Cosenza comitato etico Ospedale Giannettasio, Rossano Calabro, Italy; Divisione di Ematologia, Ospedale Policlinico, Palermo, comitato etico Ospedale Policlinico, Palermo, Italy; Ematologia ospedale Goretti, Latina comitato etico ospedale Goretti, Latina, Italy, Clinica Ematologica, DIMI, Genova, comitato etico IRCCS Ospedale Policlinico San Martino, Genova, Italy; Oncologia medica C IRCCS Ospedale Policlinico San Martino, Genoa, Italy comitato etico IRCCS Ospedale Policlinico San Martino, Genova, Italy; Ospedale Villa Scassi Sampierdarena, Genova comitato etico Ospedale Villa Scassi, Genova, Italy; Ematologia, Azienda Ospedaliera San Martino, Genova comitato etico IRCCS Policlinico San Martino, Genova, Italy; Unità di Ematologia, Ospedale Vito Fazzi, Lecce comitato etico Ospedale Vito Fazzi, Lecce, Italy; Unità Operativa Complessa di Ematologia Ospedale di Matera comitato etico Ospedale di Matera, Italy; Divisione di Ematologia, Ospedale Papardo, Messina, Italy comitato etico Ospedale Papardo, Messina, Italy; Divisione di Ematologia, Università di Messina comitato etico Ospedale di Messina, Italy; Ematologia and Centro Trapianti Midollo Osseo, Foundation IRCCS Ca’ Granda Ospedale Maggiore Policlinico, Milano comitato etico Ospedale Maggiore Policlinico di Milano, Italy; Dipartimento di Oncologia, Ospedale Civile, Noale, Venezia comitato etico Ospedale Civile, Noale, Italy; Oncoematologia Policlinico di Modena comitato etico provinciale di Modena Italy; Divisione di Ematologia, Ospedale Cardarelli, Napoli comitato etico Ospedale Cardarelli, Napoli, Italy; Ematologia, Centro Trapianti Midollo Osseo, Azienda Ospedaliera Universitaria di Parma comitato etico Azienda Ospedaliera Universitaria di Parma, Italy; Dipartimento di Ematologia, Ospedale Santo Spirito, Pescara comitato etico Ospedale Santo Spirito, Pescara, Italy; Unità di Ematologia, Dipartimento di Onco-Ematologia, Guglielmo da Saliceto Hospital, Piacenza comitato etico Guglielmo da Saliceto Hospital, Piacenza, Italy; Unità di Ematologia, Azienda Ospedaliera of Reggio Calabria comitato etico Azienda Ospedaliera of Reggio Calabria, Italy; Unità Operativa di Ematologia, Azienda Ospedaliera Maria Nuova, Reggio Emilia comitato etico Azienda Ospedaliera Maria Nuova, Reggio Emilia, Italy; Unità Operativa Complessa di Ematologia e Trapianto di Cellule Staminali IRCCS-CROB di Rionero in Vulture, Potenza comitato etico IRCCS-CROB di Rionero in Vulture, Italy; Dipartimento di Ematologia, Ospedale Nuovo Regina Margherita, Roma comitato etico Ospedale Nuovo Regina Margherita, Roma, Italy; Ematologia, Azienda Ospedaliera Sant’Andrea, Università La Sapienza, Roma comitato etico Azienda Ospedaliera Sant’Andrea, Roma, Italy; Divisione di Ematologia, Università La Sapienza, Roma comitato etico Università La Sapienza, Roma, Italy; Unità di Ematologia e Trapianto di Cellule Staminali, IRCCS Ospedale Casa Sollievo della Sofferenza, San Giovanni Rotondo comitato etico IRCCS Ospedale Casa Sollievo della Sofferenza, San Giovanni Rotondo, Italy; Unità di Ematologia, Ospedale San Vincenzo, Taormina, comitato etico Ospedale San Vincenzo, Taormina, Italy; Unità di Ematologia, Ospedale San Nicola Pellegrino, Trani comitato etico Ospedale San Nicola Pellegrino, Trani, Italy; Centro di Riferimento Ematologico-Seconda Medicina, Azienda Ospedaliero-Universitaria, Ospedali Riuniti, Trieste comitato etico Ospedali Riuniti, Trieste, Italy; Unità Operativa Oncologia Medica, Ospedale di Circolo Fondazione Macchi, Varese comitato etico Ospedale di Circolo Fondazione Macchi, Varese, Italy; Unità Operativa di Ematologia, Ospedale dell’Angelo, Venezia-Mestre comitato etico Ospedale dell’Angelo, Venezia-Mestre, Italy; IRCCS Cà Granda-Maggiore Policlinico, Milano comitato etico Policlinico, Milano, Italy. The studies were conducted in accordance with the local legislation and institutional requirements. Written informed consent for participation was not required from the participants or the participants’ legal guardians/next of kin in accordance with the national legislation and institutional requirements.
Author contributions
The authors confirm contributions to the paper as follows: study conception and design: FM, AN, CA, GG; data collection: CG and MG; Artificial Intelligence analysis and interpretation of results: CA, YR-A, GG; Pathways and network analysis and interpretation of results: PM, AA, FR, MC, FT, TR; Multivariate statistical analysis and interpretation of results: FM, GD’A, GT; draft manuscript preparation: CA and YR-A, and FM; results discussion and contribution to the final manuscript: AN, FM, CA, MG, CV, MF. All authors reviewed and approved the final version of the manuscript.
Funding
This work was partially founded by Italian Ministry of Health (Ricerca Corrente 2024) and supported by Associazione Italiana Ricerca sul Cancro (AIRC) Grant 5 × mille ID.9980, (FM, MF, and AN); AIRC and Fondazione CaRiCal co-financed Multi-Unit Regional Grant 2014 n.16695 (to FM); Italian Ministry of Health 5 × 1000 funds 2014 (to CG), 2016 (CG), 2018 (to AA) and 2020 (to CG and PM); Associazione Italiana contro le Leucemie-Linfomi e Mieloma (AIL, Cosenza, to FF); and Gilead fellowship program 2016 (MC) and 2017 (CG). The research reported in the paper was partially supported by the PNRR projects “FAIR (PE00000013) – Spoke 9” and “Tech4You (ECS00000009) – Spoke 6”, under the NRRP MUR program funded by the NextGenerationEU – The National Plan for NRRP Complementary Investments (PNC, established with the decree-law 6 May 2021, n. 59, converted by law n. 101 of 2021) in the call for the funding of research initiatives for technologies and innovative trajectories in the health and care sectors (Directorial Decree n. 931 of 06-06-2022) – project n. PNC0000003 – AdvaNced Technologies for Human-centrEd Medicine (project acronym: ANTHEM). This work reflects only the authors’ views and opinions, neither the Ministry for University and Research nor the European Commission can be considered responsible for them.
Acknowledgments
In addition to the listed authors, the following investigators participated in this study as part of the Gruppo Italiano Studio Linfomi (GISL): Gianni Quintana, Divisione di Ematologia, Presidio Ospedaliero “A.Perrino”, Brindisi; Giovanni Bertoldero, Dipartimento di Oncologia, Ospedale Civile, Noale, Venezia; Paolo Di Tonno, Dipartimento di Ematologia, Ospedale di Venere, Bari; Robin Foà and Francesca R Mauro, Divisione di Ematologia, Università La Sapienza, Roma; Nicola Di Renzo, Unità di Ematologia, Ospedale Vito Fazzi, Lecce; Maria Cristina Cox, Ematologia, Azienda Ospedaliera Sant’Andrea, Università La Sapienza, Roma; Stefano Molica, Dipartimento di Oncologia ed Ematologia, Pugliese-Ciaccio Hospital, Catanzaro; Attilio Guarini, Unità di Ematologia e Trapianto di Cellule Staminali, Istituto di Oncologia “Giovanni Paolo II”, Bari; Antonio Abbadessa, Unità Operativa Complessa di Oncoematologia Ospedale “S. Anna e S. Sebastiano”, Caserta; Francesco Iuliano, Unità Operativa Complessa di Oncologia, Ospedale Giannettasio, Rossano Calabro, Cosenza; Omar Racchi, Ospedale Villa Scassi Sampierdarena, Genova; Mauro Spriano, Ematologia, IRCCS Policlinico San Martino, Genova; Felicetto Ferrara, Divisione di Ematologia, Ospedale Cardarelli, Napoli; Monica Crugnola, Ematologia, CTMO, Azienda Ospedaliera Universitaria di Parma; Alessandro Andriani, Dipartimento di Ematologia, Ospedale Nuovo Regina Margherita, Roma; Nicola Cascavilla, Unità di Ematologia e Trapianto di Cellule Staminali, IRCCS Ospedale Casa Sollievo della Sofferenza, San Giovanni Rotondo; Lucia Ciuffreda, Unità di Ematologia, Ospedale San Nicola Pellegrino, Trani; Graziella Pinotti, Unità Operativa Oncologia Medica, Ospedale di Circolo Fondazione Macchi, Varese; Anna Pascarella, Unità Operativa di Ematologia, Ospedale dell’Angelo; Venezia-Mestre, Maria Grazia Lipari, Divisione di Ematologia, Ospedale Policlinico, Palermo; Francesco Merli, Unità Operativa di Ematologia, Azienda Ospedaliera Maria Nuova; Reggio Emilia, Luca Baldini Istituto di Ricovero e Cura a Carattere Scientifico Cà Granda-Maggiore Policlinico, Milano; Caterina Musolino, Divisione di Ematologia, Università di Messina; Agostino Cortelezzi, Ematologia and Centro Trapianti Midollo Osseo, Foundation IRCCS Ca’ Granda Ospedale Maggiore Policlinico, Milano; Francesco Angrilli, Dipartimento di Ematologia, Ospedale Santo Spirito, Pescara; Ugo Consoli, Unità Operativa Semplice di Emato-Oncologia, Ospedale Garibaldi-Nesima, Catania; Gianluca Festini, Centro di Riferimento Ematologico-Seconda Medicina, Azienda Ospedaliero-Universitaria; Ospedali Riuniti, Trieste, Giuseppe Longo, Unità di Ematologia, Ospedale San Vincenzo, Taormina; Daniele Vallisa and Annalisa Arcari, Unità di Ematologia, Dipartimento di Onco-Ematologia, Guglielmo da Saliceto Hospital, Piacenza; Francesco Di Raimondo and Annalisa Chiarenza, Divisione di Ematologia, Università di Catania Ospedale Ferrarotto, Catania; Iolanda Vincelli, Unità di Ematologia, Azienda Ospedaliera of Reggio Calabria; Donato Mannina, Divisione di Ematologia, Ospedale Papardo, Messina, Italy. For Each Institution, the Ethics Review Committee that approved the protocol is listed below. Unità di Ematologia e Trapianto di Cellule Staminali, Istituto di Oncologia “Giovanni Paolo II”, Bari comitato etico Istituto di Oncologia “Giovanni Paolo II”, di Bari, Italy; Dipartimento di Ematologia, Ospedale di Venere, Bari comitato etico Ospedale di Venere, Bari, Italy; Unità Operativa Complessa Ematologia e Trapianto, Ospedale “Mons. R. Dimiccoli” - Barletta comitato etico Ospedale “Mons. R. Dimiccoli” - Barletta, Italy Divisione di Ematologia, Presidio Ospedaliero “A. Perrino”, Brindisi comitato etico Presidio Ospedaliero “A. Perrino”, Brindisi, Italy; Unità Operativa Complessa di Oncoematologia Ospedale “S. Anna e S. Sebastiano”, Caserta comitato etico Ospedale “S. Anna e S. Sebastiano”, Caserta, Italy; Divisione di Ematologia, Università di Catania Ospedale Ferrarotto, Catania comitato etico Ospedale Ferrarotto, Catania, Italy; Unità Operativa Complessa di Emato-Oncologia, Ospedale Garibaldi-Nesima, Catania comitato etico Ospedale Garibaldi - Nesima, Catania, Italy; Dipartimento di Oncologia ed Ematologia, Pugliese-Ciaccio Hospital, Catanzaro comitato etico Pugliese-Ciaccio Hospital, Catanzaro, Italy; Unità Operativa Complessa di Ematologia, Azienda Ospedaliera Cosenza comitato etico Azienda Ospedaliera Cosenza, Italy; Unità Operativa Complessa di Oncologia, Ospedale Giannettasio, Rossano Calabro, Cosenza comitato etico Ospedale Giannettasio, Rossano Calabro, Italy; Divisione di Ematologia, Ospedale Policlinico, Palermo, comitato etico Ospedale Policlinico, Palermo, Italy; Ematologia ospedale Goretti, Latina comitato etico ospedale Goretti, Latina, Italy, Clinica Ematologica, DIMI, Genova, comitato etico IRCCS Ospedale Policlinico San Martino, Genova, Italy; Oncologia medica C IRCCS Ospedale Policlinico San Martino, Genoa, Italy comitato etico IRCCS Ospedale Policlinico San Martino, Genova, Italy; Ospedale Villa Scassi Sampierdarena, Genova comitato etico Ospedale Villa Scassi, Genova, Italy; Ematologia, Azienda Ospedaliera San Martino, Genova comitato etico IRCCS Policlinico San Martino, Genova, Italy; Unità di Ematologia, Ospedale Vito Fazzi, Lecce comitato etico Ospedale Vito Fazzi, Lecce, Italy; Unità Operativa Complessa di Ematologia Ospedale di Matera comitato etico Ospedale di Matera, Italy; Divisione di Ematologia, Ospedale Papardo, Messina, Italy comitato etico Ospedale Papardo, Messina, Italy; Divisione di Ematologia, Università di Messina comitato etico Ospedale di Messina, Italy; Ematologia and Centro Trapianti Midollo Osseo, Foundation IRCCS Ca’ Granda Ospedale Maggiore Policlinico, Milano comitato etico Ospedale Maggiore Policlinico di Milano, Italy; Dipartimento di Oncologia, Ospedale Civile, Noale, Venezia comitato etico Ospedale Civile, Noale, Italy; Oncoematologia Policlinico di Modena comitato etico provinciale di Modena Italy; Divisione di Ematologia, Ospedale Cardarelli, Napoli comitato etico Ospedale Cardarelli, Napoli, Italy; Ematologia, Centro Trapianti Midollo Osseo, Azienda Ospedaliera Universitaria di Parma comitato etico Azienda Ospedaliera Universitaria di Parma, Italy; Dipartimento di Ematologia, Ospedale Santo Spirito, Pescara comitato etico Ospedale Santo Spirito, Pescara, Italy; Unità di Ematologia, Dipartimento di Onco-Ematologia, Guglielmo da Saliceto Hospital, Piacenza comitato etico Guglielmo da Saliceto Hospital, Piacenza, Italy; Unità di Ematologia, Azienda Ospedaliera of Reggio Calabria comitato etico Azienda Ospedaliera of Reggio Calabria, Italy; Unità Operativa di Ematologia, Azienda Ospedaliera Maria Nuova, Reggio Emilia comitato etico Azienda Ospedaliera Maria Nuova, Reggio Emilia, Italy; Unità Operativa Complessa di Ematologia e Trapianto di Cellule Staminali IRCCS-CROB di Rionero in Vulture, Potenza comitato etico IRCCS-CROB di Rionero in Vulture, Italy; Dipartimento di Ematologia, Ospedale Nuovo Regina Margherita, Roma comitato etico Ospedale Nuovo Regina Margherita, Roma, Italy; Ematologia, Azienda Ospedaliera Sant’Andrea, Università La Sapienza, Roma comitato etico Azienda Ospedaliera Sant’Andrea, Roma, Italy; Divisione di Ematologia, Università La Sapienza, Roma comitato etico Università La Sapienza, Roma, Italy; Unità di Ematologia e Trapianto di Cellule Staminali, IRCCS Ospedale Casa Sollievo della Sofferenza, San Giovanni Rotondo comitato etico IRCCS Ospedale Casa Sollievo della Sofferenza, San Giovanni Rotondo, Italy; Unità di Ematologia, Ospedale San Vincenzo, Taormina, comitato etico Ospedale San Vincenzo, Taormina, Italy; Unità di Ematologia, Ospedale San Nicola Pellegrino, Trani comitato etico Ospedale San Nicola Pellegrino, Trani, Italy; Centro di Riferimento Ematologico-Seconda Medicina, Azienda Ospedaliero-Universitaria, Ospedali Riuniti, Trieste comitato etico Ospedali Riuniti, Trieste, Italy; Unità Operativa Oncologia Medica, Ospedale di Circolo Fondazione Macchi, Varese comitato etico Ospedale di Circolo Fondazione Macchi, Varese, Italy; Unità Operativa di Ematologia, Ospedale dell’Angelo, Venezia-Mestre comitato etico Ospedale dell’Angelo, Venezia-Mestre, Italy; IRCCS Cà Granda-Maggiore Policlinico, Milano comitato etico Policlinico, Milano, Italy.
Conflict of interest
The authors declare that the research was conducted in the absence of any commercial or financial relationships that could be construed as a potential conflict of interest.
Publisher’s note
All claims expressed in this article are solely those of the authors and do not necessarily represent those of their affiliated organizations, or those of the publisher, the editors and the reviewers. Any product that may be evaluated in this article, or claim that may be made by its manufacturer, is not guaranteed or endorsed by the publisher.
References
1. Hallek M. Chronic lymphocytic leukemia: 2020 update on diagnosis, risk stratification and treatment. Am J Hematol (2019) 94(11):1266–87. doi: 10.1002/ajh.25595
2. Baliakas P, Mattsson M, Stamatopoulos K, Rosenquist R. Prognostic indices in chronic lymphocytic leukaemia: where do we stand how do we proceed? J Intern Med (2016) 279(4):347–57. doi: 10.1111/joim.12455
3. Döhner H, Stilgenbauer S, Benner A, Leupolt E, Kröber A, Bullinger L, et al. Genomic aberrations and survival in chronic lymphocytic leukemia. New Engl J Med (2000) 343(26):1910–6. doi: 10.1056/NEJM200012283432602
4. Kreuzberger N, Damen JAAG, Trivella M, Estcourt LJ, Aldin A, Umlauff L, et al. Prognostic models for newly-diagnosed chronic lymphocytic leukaemia in adults: a systematic review and meta-analysis. Cochrane Database Systematic Rev (2020) 7(CD012022):1–233. doi: 10.1002/14651858.CD012022.pub2
5. International CLL-IPI Working Group. An international prognostic index for patients with chronic lymphocytic leukaemia (CLL-IPI): a meta-analysis of individual patient data. Lancet Oncol (2016) 17(6):779–90. doi: 10.1016/S1470-2045(16)30029-8
6. Condoluci A, di Bergamo L, Langerbeins P, Hoechstetter MA, Herling CD, De Paoli L, et al. International prognostic score for asymptomatic early-stage chronic lymphocytic leukemia. Blood (2020) 135(21):1859–69. doi: 10.1182/blood.2019003453
7. Morabito F, Tripepi G, Vigna E, Bossio S, D’Arrigo G, Martino EA, et al. Validation of the Alternative International Prognostic Score-E (AIPS-E): Analysis of Binet stage A chronic lymphocytic leukemia patients enrolled into the O-CLL1-GISL protocol. Eur J Haematol (2021) 106(6):831–5. doi: 10.1111/ejh.13614
8. Gentile M, Shanafelt TD, Rossi D, Laurenti L, Mauro FR, Molica S, et al. Validation of the CLL-IPI and comparison with the MDACC prognostic index in newly diagnosed patients. Blood J Am Soc Hematol (2016) 128(16):2093–5. doi: 10.1182/blood-2016-07-728261
9. Rodriguez A, Villuendas R, Yanez L, Gomez ME, Diaz R, Pollan M, et al. Molecular heterogeneity in chronic lymphocytic leukemia is dependent on BCR signaling: clinical correlation. Leukemia (2007) 21(9):1984–91. doi: 10.1038/sj.leu.2404831
10. Calin GA, Ferracin M, Cimmino A, di Leva G, Shimizu M, Wojcik SE, et al. A MicroRNA signature associated with prognosis and progression in chronic lymphocytic leukemia. New Engl J Med (2005) 353(17):1793–801. doi: 10.1056/NEJMoa050995
11. Herold T, Jurinovic V, Metzeler KH, Boulesteix AL, Bergmann M, Seiler T, et al. An eight-gene expression signature for the prediction of survival and time to treatment in chronic lymphocytic leukemia. Leukemia (2011) 25(10):1639–45. doi: 10.1038/leu.2011.125
12. Taylor J, Xiao W, Abdel-Wahab O. Diagnosis and classification of hematologic Malignancies on the basis of genetics. Blood J Am Soc Hematology. (2017) 130(4):410–23. doi: 10.1182/blood-2017-02-734541
13. Koumakis L. Deep learning models in genomics; are we there yet? Comput Struct Biotechnol J (2020) 18:1466–73. doi: 10.1016/j.csbj.2020.06.017
14. Zhu Y, Gan X, Qin R, Lin Z. Identification of six diagnostic biomarkers for chronic lymphocytic leukemia based on machine learning algorithms. J Oncol (2022) 2022(3652107):1–19. doi: 10.1155/2022/3652107
15. Chen P, El Hussein S, Xing F, Aminu M, KannapIran A, Hazle JD, et al. Chronic lymphocytic leukemia progression diagnosis with intrinsic cellular patterns via unsupervised clustering. Cancers (Basel) (2022) 14(10):2398. doi: 10.3390/cancers14102398
16. Parviz M, Brieghel C, Agius R, Niemann CU. Prediction of clinical outcome in CLL based on recurrent gene mutations, CLL-IPI variables, and (para) clinical data. Blood Adv (2022) 6(12):3716–28. doi: 10.1182/bloodadvances.2021006351
17. Agius R, Brieghel C, Andersen MA, Pearson AT, Ledergerber B, Cozzi-Lepri A, et al. Machine learning can identify newly diagnosed patients with CLL at high risk of infection. Nat Commun (2020) 11(1):363–80. doi: 10.1038/s41467-019-14225-8
18. Xie R, Wen J, Quitadamo A, Cheng J, Shi X. A deep auto-encoder model for gene expression prediction. BMC Genomics (2017) 18:39–49. doi: 10.1186/s12864-017-4226-0
19. Chen H, Zhang Y, Gutman I. A kernel-based clustering method for gene selection with gene expression data. J BioMed Inform (2016) 62:12–20. doi: 10.1016/j.jbi.2016.05.007
20. Das P, Roychowdhury A, Das S, Roychoudhury S, Tripathy S. sigFeature: novel significant feature selection method for classification of gene expression data using support vector machine and t statistic. Front Genet (2020) 11:247. doi: 10.3389/fgene.2020.00247
21. Salah HT, Muhsen IN, Salama ME, Owaidah T, Hashmi SK. Machine learning applications in the diagnosis of leukemia: Current trends and future directions. Int J Lab Hematol (2019) 41(6):717–25. doi: 10.1111/ijlh.13089
22. Agius R, Parviz M, Niemann CU. Artificial intelligence models in chronic lymphocytic leukemia–recommendations toward state-of-the-art. Leuk Lymphoma. (2022) 63(2):265–78. doi: 10.1080/10428194.2021.1973672
23. Alhenawi E, Al-Sayyed R, Hudaib A, Mirjalili S. Feature selection methods on gene expression microarray data for cancer classification: A systematic review. Comput Biol Med (2022) 140:105051. doi: 10.1016/j.compbiomed.2021.105051
24. Danaee P, Ghaeini R, Hendrix DA. A deep learning approach for cancer detection and relevant gene identification. In: Pacific symposium biocomputing (2017) 2017:219–29. doi: 10.1142/9789813207813_0022
25. Graham G, Csicsery N, Stasiowski E, Thouvenin G, Mather WH, Ferry M, et al. Genome-scale transcriptional dynamics and environmental biosensing. Proc Natl Acad Sci (2020) 117(6):3301–6. doi: 10.1073/pnas.1913003117
26. Meena J, Hasija Y. Application of explainable artificial intelligence in the identification of Squamous Cell Carcinoma biomarkers. Comput Biol Med (2022) 146:105505. doi: 10.1016/j.compbiomed.2022.105505
27. Karim MR, Cochez M, Beyan O, Decker S, Lange C. OncoNetExplainer: explainable predictions of cancer types based on gene expression data, In: 2019 IEEE 19th International conference on bioinformatics and bioengineering (BIBE). Athens, Greece (2019). pp. 415–22. doi: 10.1109/BIBE.2019.00081
28. Lundberg SM, Lee SI. A unified approach to interpreting model predictions. Adv Neural Inf Process Syst (2017) 30:1–10.
29. Morabito F, Mosca L, Cutrona G, Agnelli L, Tuana G, Ferracin M, et al. Clinical monoclonal B lymphocytosis versus Rai 0 chronic lymphocytic leukemia: a comparison of cellular, cytogenetic, molecular, and clinical features. Clin Cancer Res (2013) 19(21):5890–900. doi: 10.1158/1078-0432.CCR-13-0622
30. Negrini M, Cutrona G, Bassi C, Fabris S, Zagatti B, Colombo M, et al. microRNAome expression in chronic lymphocytic leukemia: comparison with normal B-cell subsets and correlations with prognostic and clinical parametersmicroRNA expression in CLL. Clin Cancer Res (2014) 20(15):4141–53. doi: 10.1158/1078-0432.CCR-13-2497
31. Fabris S, Mosca L, Todoerti K, Cutrona G, Lionetti M, Intini D, et al. Molecular and transcriptional characterization of 17p loss in B-cell chronic lymphocytic leukemia. Genes Chromosomes Cancer (2008) 47(9):781–93. doi: 10.1002/gcc.20579
32. Fais F, Ghiotto F, Hashimoto S, Sellars B, Valetto A, Allen SL, et al. Chronic lymphocytic leukemia B cells express restricted sets of mutated and unmutated antigen receptors. J Clin Invest. (1998) 102(8):1515–25. doi: 10.1172/JCI3009
33. Jović A, Brkić K, Bogunović N. A review of feature selection methods with applications. (2015). pp. 1200–5. doi: 10.1109/MIPRO.2015.7160458
34. Solorio-Fernández S, Carrasco-Ochoa JA, Martíinez-Trinidad JF. A review of unsupervised feature selection methods. Artif Intell Rev (2020) 53(2):907–48. doi: 10.1007/s10462-019-09682-y
35. Khaire UM, Dhanalakshmi R. Stability of feature selection algorithm: A review. J King Saud University-Computer Inf Sci (2022) 34(4):1060–73. doi: 10.1016/j.jksuci.2019.06.012
36. Daoud M, Mayo M. A survey of neural network-based cancer prediction models from microarray data. Artif Intell Med (2019) 97:204–14. doi: 10.1016/j.artmed.2019.01.006
37. Lopez-Garcia G, Jerez JM, Franco L, Veredas FJ. Transfer learning with convolutional neural networks for cancer survival prediction using gene-expression data. PloS One (2020) 15(3):e0230536. doi: 10.1371/journal.pone.0230536
38. Flagel L, Brandvain Y, Schrider DR. The unreasonable effectiveness of convolutional neural networks in population genetic inference. Mol Biol Evol (2019) 36(2):220–38. doi: 10.1093/molbev/msy224
39. Ma W, Qiu Z, Song J, Li J, Cheng Q, Zhai J, et al. A deep convolutional neural network approach for predicting phenotypes from genotypes. Planta (2018) 248:1307–18. doi: 10.1007/s00425-018-2976-9
40. Luo P, Ding Y, Lei X, Wu FX. deepDriver: predicting cancer driver genes based on somatic mutations using deep convolutional neural networks. Front Genet (2019) 10:13. doi: 10.3389/fgene.2019.00013
41. Katzman JL, Shaham U, Cloninger A, Bates J, Jiang T, Kluger Y. DeepSurv: personalized treatment recommender system using a Cox proportional hazards deep neural network. BMC Med Res Methodol (2018) 18(1):1–12. doi: 10.1186/s12874-018-0482-1
42. Bank D, Koenigstein N, Giryes R. Autoencoders. arXiv (2020) arXiv:2003.05991v2):1–22. doi: 10.48550/arXiv.2003.05991
43. Ribeiro MT, Singh S, Guestrin C. “Why should i trust you?” Explaining the predictions of any classifier. In: Proceedings of the 22nd ACM SIGKDD International conference on knowledge discovery and data mining (KDD '16). New York, NY, USA: Association for Computing Machinery (2016). pp. 1135–44. doi: 10.1145/2939672.2939778
44. Chawla N v, Bowyer KW, Hall LO, Kegelmeyer WP. SMOTE: synthetic minority over-sampling technique. J Artif Intell Res (2002) 16:321–57. doi: 10.1613/jair.953
45. Tripepi G, Heinze G, Jager KJ, Stel VS, Dekker FW, Zoccali C. Risk prediction models. Nephrol Dialysis Transplantation. (2013) 28(8):1975–80. doi: 10.1093/ndt/gft095
46. Fabregat A, Sidiropoulos K, Viteri G, Forner O, Marin-Garcia P, Arnau V, et al. Reactome pathway analysis: a high-performance in-memory approach. BMC Bioinf (2017) 18(1):1–9. doi: 10.1186/s12859-017-1559-2
47. Jassal B, Matthews L, Viteri G, Gong C, Lorente P, Fabregat A, et al. The reactome pathway knowledgebase. Nucleic Acids Res (2020) 48(D1):D498–503. doi: 10.1093/nar/gkz1031
48. Zhou G, Soufan O, Ewald J, Hancock REW, Basu N, Xia J. NetworkAnalyst 3.0: a visual analytics platform for comprehensive gene expression profiling and meta-analysis. Nucleic Acids Res (2019) 47(W1):W234–41. doi: 10.1093/nar/gkz240
49. Breuer K, Foroushani AK, Laird MR, Chen C, Sribnaia A, Lo R, et al. InnateDB: systems biology of innate immunity and beyond—recent updates and continuing curation. Nucleic Acids Res (2013) 41(D1):D1228–33. doi: 10.1093/nar/gks1147
50. Li Y, Wu FX, Ngom A. A review on machine learning principles for multi-view biological data integration. Brief Bioinform (2018) 19(2):325–40. doi: 10.1093/bib/bbw113
51. Twa DDW, Lee DG, Tan KL, Slack GW, Ben-Neriah S, Villa D, et al. Genomic predictors of central nervous system relapse in primary testicular diffuse large B-cell lymphoma. Blood (2021) 137(9):1256–9. doi: 10.1182/blood.2020006338
52. Chen CT, Wang PP, Mo WJ, Zhang YP, Zhou W, Deng TF, et al. Expression profile analysis of prognostic long non-coding RNA in adult acute myeloid leukemia by weighted gene co-expression network analysis (WGCNA). J Cancer (2019) 10(19):4707. doi: 10.7150/jca.31234
53. Chen C, Liu S, Jiang X, Huang L, Chen F, Wei X, et al. Tumor mutation burden estimated by a 69-gene-panel is associated with overall survival in patients with diffuse large B-cell lymphoma. Exp Hematol Oncol (2021) 10:1–11. doi: 10.1186/s40164-021-00215-4
54. Delgado J, Doubek M, Baumann T, Kotaskova J, Molica S, Mozas P, et al. Chronic lymphocytic leukemia: a prognostic model comprising only two biomarkers (IGHV mutational status and FISH cytogenetics) separates patients with different outcome and simplifies the CLL-IPI. Am J Hematol (2017) 92(4):375–80. doi: 10.1002/ajh.24660
55. Hoechstetter MA, Busch R, Eichhorst B, Bühler A, Winkler D, Bahlo J, et al. Prognostic model for newly diagnosed CLL patients in Binet stage A: results of the multicenter, prospective CLL1 trial of the German CLL study group. Leukemia (2020) 34(4):1038–51. doi: 10.1038/s41375-020-0727-y
56. Gentile M, Shanafelt TD, Mauro FR, Laurenti L, Rossi D, Molica S, et al. Comparison between the CLL-IPI and the Barcelona-Brno prognostic model: analysis of 1299 newly diagnosed cases. Am J Hematol (2018) 93(2):E35–7. doi: 10.1002/ajh.24960
57. Monti P, Lionetti M, De Luca G, Menichini P, Recchia AG, Matis S, et al. Time to first treatment and P53 dysfunction in chronic lymphocytic leukaemia: Results of the O-CLL1 study in early stage patients. Sci Rep (2020) 10(1):1–13. doi: 10.1038/s41598-020-75364-3
58. Morabito F, Tripepi G, Moia R, Recchia AG, Boggione P, Mauro FR, et al. Lymphocyte doubling time as a key prognostic factor to predict time to first treatment in early-stage chronic lymphocytic leukemia. Front Oncol (2021) 11:684621. doi: 10.3389/fonc.2021.684621
59. Werner H. For debate: the pathophysiological significance of IGF-I receptor overexpression: new insights. Pediatr Endocrinol Rev (2009) 7(1):2–5.
60. Pollak MN, Schernhammer ES, Hankinson SE. Insulin-like growth factors and neoplasia. Nat Rev Cancer. (2004) 4(7):505–18. doi: 10.1038/nrc1387
61. Schillaci R, Galeano A, Becu-Villalobos D, Spinelli O, Sapia S, Bezares RF. Autocrine/paracrine involvement of insulin-like growth factor-I and its receptor in chronic lymphocytic leukaemia. Br J Haematol (2005) 130(1):58–66. doi: 10.1111/j.1365-2141.2005.05579.x
62. Yaktapour N, Übelhart R, Schüler J, Aumann K, Dierks C, Burger M, et al. Insulin-like growth factor-1 receptor (IGF1R) as a novel target in chronic lymphocytic leukemia. Blood J Am Soc Hematology. (2013) 122(9):1621–33. doi: 10.1182/blood-2013-02-484386
63. Maura F, Mosca L, Fabris S, Cutrona G, Matis S, Lionetti M, et al. Insulin growth factor 1 receptor expression is associated with NOTCH1 mutation, trisomy 12 and aggressive clinical course in chronic lymphocytic leukaemia. PloS One (2015) 10(3):e0118801. doi: 10.1371/journal.pone.0118801
64. Liu F, Clark W, Luo G, Wang X, Fu Y, Wei J, et al. ALKBH1-mediated tRNA demethylation regulates translation. Cell (2016) 167(3):816–28. doi: 10.1016/j.cell.2016.09.038
65. Ma Q, He J. Enhanced expression of queuine tRNA-ribosyltransferase 1 (QTRT1) predicts poor prognosis in lung adenocarcinoma. Ann Transl Med (2020) 8(24):1658. doi: 10.21037/atm-20-7424
66. Mosquera Orgueira A, Antelo Rodriguez B, Diaz Arias JÁ, Diaz Varela N, Bello López JL. A three-gene expression signature identifies a cluster of patients with short survival in chronic lymphocytic leukemia. J Oncol (2019) 2019(9453539):1–4. doi: 10.1155/2019/9453539
67. Gong H, Li H, Yang Q, Zhang G, Liu H, Ma Z, et al. A ferroptosis molecular subtype-related signature for predicting prognosis and response to chemotherapy in patients with chronic lymphocytic leukemia. BioMed Res Int (2022) 2022(5646275):1–24. doi: 10.1155/2022/5646275
68. Improgo MR, Tesar B, Klitgaard JL, Magori-Cohen R, Yu L, Kasar S, et al. MYD 88 L265P mutations identify a prognostic gene expression signature and a pathway for targeted inhibition in CLL. Br J Haematol (2019) 184(6):925–36. doi: 10.1111/bjh.15714
69. Sevov M, Rosenquist R, Mansouri L. RNA-based markers as prognostic factors in chronic lymphocytic leukemia. Expert Rev Hematol (2012) 5(1):69–79. doi: 10.1586/ehm.11.80
70. Huang H y, Wang Y, Herold T, Gale RP, Wang J z, Li L, et al. A survival prediction model and nomogram based on immune-related gene expression in chronic lymphocytic leukemia cells. Front Med (Lausanne) (2022) 9:1026812. doi: 10.3389/fmed.2022.1026812
71. Liang X, Meng Y, Li C, Liu L, Wang Y, Pu L, et al. Super-Enhancer–Associated nine-gene prognostic score model for prediction of survival in chronic lymphocytic leukemia patients. Front Genet (2022) 13:1001364. doi: 10.3389/fgene.2022.1001364
72. Abrisqueta P, Medina D, Villacampa G, Lu J, Alcoceba M, Carabia J, et al. A gene expression assay based on chronic lymphocytic leukemia activation in the microenvironment to predict progression. Blood Adv (2022) 6(21):5763–73. doi: 10.1182/bloodadvances.2022007508
73. Bauvois B, Pramil E, Jondreville L, Quiney C, Nguyen-Khac F, Susin SA. Activation of interferon signaling in chronic lymphocytic leukemia cells contributes to apoptosis resistance via a JAK-Src/STAT3/mcl-1 signaling pathway. Biomedicines (2021) 9(2):188. doi: 10.3390/biomedicines9020188
74. Bauvois B, Durant L, Laboureau J, Barthelemy E, Rouillard D, Boulla G, et al. Upregulation of CD38 gene expression in leukemic B cells by interferon types I and II. J Interferon Cytokine Res (1999) 19(9):1059–66. doi: 10.1089/107999099313299
75. Fluckiger AC, Rossi JF, Bussel A, Bryon P, Banchereau J, DeFrance T. Responsiveness of chronic lymphocytic leukemia B cells activated via surface Igs or CD40 to B-cell tropic factors. Blood (1992) 80(12):3173–81. doi: 10.1182/blood.V80.12.3173.3173
76. Infante E, Ridley AJ. Roles of Rho GTPases in leucocyte and leukaemia cell transendothelial migration. Philos Trans R Soc B: Biol Sci (2013) 368(1629):20130013. doi: 10.1098/rstb.2013.0013
77. Abu El-Makarem MA, Kamel MF, Mohamed AA, Ali HA, Mohamed MR, Mohamed AEDM, et al. Down-regulation of hepatic expression of GHR/STAT5/IGF-1 signaling pathway fosters development and aggressiveness of HCV-related hepatocellular carcinoma: Crosstalk with Snail-1 and type 2 transforming growth factor-beta receptor. PloS One (2022) 17(11):e0277266. doi: 10.1371/journal.pone.0277266
78. Jiang F, Chen X, Shen Y, Shen X. Identification and validation of an m6A modification of JAK-STAT signaling pathway–related prognostic prediction model in gastric cancer. Front Genet (2022) 13:891744. doi: 10.3389/fgene.2022.891744
79. Yan HZ, Wang HF, Yin Y, Zou J, Xiao F, Yi LN, et al. GHR is involved in gastric cell growth and apoptosis via PI3K/AKT signalling. J Cell Mol Med (2021) 25(5):2450–8. doi: 10.1111/jcmm.16160
80. Polcik L, Dannewitz Prosseda S, Pozzo F, Zucchetto A, Gattei V, Hartmann TN. Integrin signaling shaping BTK-inhibitor resistance. Cells (2022) 11(14):2235. doi: 10.3390/cells11142235
81. Siveen KS, Prabhu KS, Achkar IW, Kuttikrishnan S, Shyam S, Khan AQ, et al. Role of non receptor tyrosine kinases in hematological Malignances and its targeting by natural products. Mol Cancer (2018) 17(1):1–21. doi: 10.1186/s12943-018-0788-y
82. Sinha S, Boysen J, Nelson M, Warner SL, Bearss D, Kay NE, et al. Axl activates fibroblast growth factor receptor pathway to potentiate survival signals in B-cell chronic lymphocytic leukemia cells. Leukemia (2016) 30(6):1431–6. doi: 10.1038/leu.2015.323
83. Mosca L, Fabris S, Lionetti M, Todoerti K, Agnelli L, Morabito F, et al. Integrative genomics analyses reveal molecularly distinct subgroups of B-cell chronic lymphocytic leukemia patients with 13q14 deletionIntegrative genomics analysis of 13q14-deleted CLL. Clin Cancer Res (2010) 16(23):5641–53. doi: 10.1158/1078-0432.CCR-10-0151
84. Kosalai ST, Morsy MHA, Papakonstantinou N, Mansouri L, Stavroyianni N, Kanduri C, et al. EZH2 upregulates the PI3K/AKT pathway through IGF1R and MYC in clinically aggressive chronic lymphocytic leukaemia. Epigenetics (2019) 14(11):1125–40. doi: 10.1080/15592294.2019.1633867
85. Scheffold A, Jebaraj BMC, Tausch E, Bloehdorn J, Ghia P, Yahiaoui A, et al. IGF1R as druggable target mediating PI3K-δ inhibitor resistance in a murine model of chronic lymphocytic leukemia. Blood J Am Soc Hematol (2019) 134(6):534–47. doi: 10.1182/blood.2018881029
86. Kliza K, Husnjak K. Resolving the complexity of ubiquitin networks. Front Mol Biosci (2020) 7:21. doi: 10.3389/fmolb.2020.00021
87. Zhang L, Liang A, Zhang W, Wang H, Wang C. Bioinformatics analysis of gene expression profiles in chronic lymphocytic leukemia. Int J Clin Exp Pathol (2016) 9(9):9126–37.
88. Chuang HY, Rassenti L, Salcedo M, Licon K, Kohlmann A, Haferlach T, et al. Subnetwork-based analysis of chronic lymphocytic leukemia identifies pathways that associate with disease progression. Blood J Am Soc Hematol (2012) 120(13):2639–49. doi: 10.1182/blood-2012-03-416461
89. Puente XS, Beà S, Valdés-Mas R, Villamor N, Gutiérrez-Abril J, Martín-Subero JI, et al. Non-coding recurrent mutations in chronic lymphocytic leukaemia. Nature (2015) 526(7574):519–24. doi: 10.1038/nature14666
Keywords: chronic lymphocytic leukemia, gene expression profile, deep learning, explainable artificial intelligence, feature selection
Citation: Morabito F, Adornetto C, Monti P, Amaro A, Reggiani F, Colombo M, Rodriguez-Aldana Y, Tripepi G, D’Arrigo G, Vener C, Torricelli F, Rossi T, Neri A, Ferrarini M, Cutrona G, Gentile M and Greco G (2023) Genes selection using deep learning and explainable artificial intelligence for chronic lymphocytic leukemia predicting the need and time to therapy. Front. Oncol. 13:1198992. doi: 10.3389/fonc.2023.1198992
Received: 02 April 2023; Accepted: 31 July 2023;
Published: 31 August 2023.
Edited by:
Giuseppe Alberto Palumbo, University of Catania, ItalyReviewed by:
Marta Sonia González Pérez, University Clinical Hospital of Santiago, SpainSara Galimberti, University of Pisa, Italy
Copyright © 2023 Morabito, Adornetto, Monti, Amaro, Reggiani, Colombo, Rodriguez-Aldana, Tripepi, D’Arrigo, Vener, Torricelli, Rossi, Neri, Ferrarini, Cutrona, Gentile and Greco. This is an open-access article distributed under the terms of the Creative Commons Attribution License (CC BY). The use, distribution or reproduction in other forums is permitted, provided the original author(s) and the copyright owner(s) are credited and that the original publication in this journal is cited, in accordance with accepted academic practice. No use, distribution or reproduction is permitted which does not comply with these terms.
*Correspondence: Antonino Neri, YW50b25pbm8ubmVyaUBhdXNsLnJlLml0; Carlo Adornetto, Y2FybG8uYWRvcm5ldHRvQHVuaWNhbC5pdA==
†These authors share first authorship
‡These authors share senior authorship