- 1Department of Gynecology and Obstetrics, The Second Affiliated Hospital of Zhengzhou University, Zhengzhou, China
- 2Henan Gynecological Diseases (Gynecology Oncology) Clinical Research Center, Zhengzhou, China
- 3Department of Urology, Henan Provincial Hospital of Traditional Chinese Medicine, Zhengzhou, China
Aims: This study aimed to construct a lymph node metastasis-related gene signature to predict prognosis and immune infiltration in patients with cervical cancer.
Methods: Clinical and RNA sequencing data of 193 patients with cervical cancer, which were divided into lymph node metastasis (N1) and non-lymph node metastasis (N0) groups, were acquired from TCGA. Differentially expressed genes (DEGs) between the N1 and N0 groups were detected, and protein-protein interaction combined with LASSO analysis was conducted to further screen lymph node metastasis-related genes. Univariate and multivariate Cox regression analyses were performed to establish a predictive signature. The genetic features, potential biological behavior, and immune infiltration characteristics of the predictive signature were explored. Furthermore, the sensitivity of patients to chemotherapy drugs was estimated based on the predictive signature and the expression of TEKT2 and RPGR was investigated in the cervical cancer tissue samples.
Results: A total of 271 lymph node metastasis-related DEGs, including 100 upregulated and 171 downregulated genes, were identified. Two genes, TEKT2 and RPGR, were associated with lymph node metastasis and prognosis in cervical cancer, and were used to construct a lymph node metastasis-related predictive signature. Based on the predictive signature, patients with cervical cancer were divided into high- and low-risk groups. The high-risk group, characterized by a higher tumor mutation burden and somatic mutation rate, indicated a poor overall survival. The activation of immune infiltration and increased expression of checkpoint genes were observed in the high-risk group, indicating that they might benefit from immunotherapy. Cytarabine, FH535, and procaspase-activating compound-1 were estimated as reasonable chemotherapy options for patients in the high-risk group, whereas two taxanes and five tyrosine kinase inhibitors, including etoposide and vinorelbine, had therapeutic significance for patients in the low-risk group. The expression of TEKT2 and RPGR was significantly downregulated in cervical cancer tissues, especially in metastatic lymph node tissues.
Discussion: The lymph node metastasis-related predictive signature based on TEKT2 and RPGR showed good performance in predicting the survival outcomes of patients with cervical cancer. The risk score of the predictive signature was related to genetic variation and immune infiltration, which could guide immunotherapy and chemotherapy strategies.
1 Introduction
Cervical cancer is the fourth most common cancer in women globally, with 341,831 deaths and 604,127 new cases recorded in 2020 (1). Radical hysterectomy and chemoradiotherapy are performed at different stages of cervical cancer (2). However, metastasis and recurrence are the main causes of cancer-related mortality (3). Therefore, it is necessary to identify the key factors that influence the development and metastasis of cervical cancer.
Lymph node metastasis, a multistep process involving complex biological mechanisms, is one of the most crucial prognostic indicators in cervical cancer (4). According to the recently revised 2018 International Federation of Gynecology and Obstetrics staging system for cervical cancer, lymph node status affects the staging of patients with cervical cancer (5). In addition, a study revealed that metastatic lymph nodes exhibited an early stage of immune response, characterized by the infiltration of cytotoxic CD8+ T cells, central memory CD4+ T cells, and effector memory CD8+ T cells, suggesting a correlation between lymph node metastasis and immune infiltration (6). However, the mechanisms underlying lymph node metastasis in cervical cancer remain unclear.
Large-scale genome sequencing studies have identified the molecular signatures of cervical cancer (7). Herein, a comprehensive study of lymph node metastasis in cervical cancer was conducted. A lymph node metastasis-related gene signature was constructed to predict cervical cancer prognosis. We aimed to identify the molecular signature, immune infiltration, and drug sensitivity based on lymph node metastasis to guide the selection of more effective therapeutic strategies for cervical cancer.
2 Materials and methods
2.1 Data acquisition
The expression profiles and clinical data of 307 cervical squamous cell carcinoma and endocervical adenocarcinoma (CESC) tissue samples were obtained from the Cancer Genome Atlas Genomic Data Commons (TCGA-GDC) Data Portal (https://portal.gdc.cancer.gov/, accessed on November 29, 2022). Perl scripts were used to generate the mRNA, long non-coding RNA (lncRNA) and micro RNA (miRNA). symbol matrix. The Masked Somatic Mutation data were processed through VarScan software and analyzed using “maftools” package in R (version 4.1.2).
2.2 Differential analysis of gene expression
Excluding data without lymph node metastases (Nx), patients with CESC (n = 193) were divided into non-lymph node metastasis (N0) and lymph node metastasis (N1) groups. The Kaplan–Meier survival curve was used to compare the differences in overall survival (OS) between the N0 and N1 groups. Differentially expressed mRNA, lncRNA, and miRNA were analyzed using the “limma” package with the criteria of an absolute log2-fold-change (FC) > 0.5 and P value <0.05. Heatmaps and volcano plots were constructed using the “ggplot2” and “pheatmap” packages, respectively, to display the expression of differentially expressed genes (DEGs), which were defined as lymph node metastasis-related genes.
2.3 Construction of a ceRNA regulatory network
To investigate the potential existence of DEGs involved in a competing endogenous RNA (ceRNA) network mediated by differentially expressed lncRNA and miRNA, we conducted miRanda (http://www.microrna.org/), miRWalk (http://129.206.7.150/), and Targetscan (http://www.targetscan.org/) analyses to predict miRNA-mRNA interactions, while StarBase (https://starbase.sysu.edu.cn/) was used to predict lncRNA-miRNA interactions. The resulting interactions were then visualized using Cytoscape software (version 3.9.1).
2.4 Functional enrichment analysis
To investigate the different biological processes of lymph node metastasis-related DEGs, Gene Ontology (GO) and Kyoto Encyclopedia of Genes and Genomes (KEGG) enrichment analyses were performed using the “org.Hs.eg.db,” “clusterProfiler,” “enrichplot,” and “ggplot2” packages, with the P value threshold set at <0.05. Gene set variation analysis (GSVA) was performed using the “GSVA” package in R to investigate the common activated/suppressed biological process. The gene sets of the “c2.cp.kegg.v7.4. symbols.gmt,” and “c5.go.v7.4. symbols.gmt” were downloaded from the Molecular Signatures Database (8). Statistical significance was set at P <0.05.
2.5 Protein-protein interaction network construction
To analyze the protein interactions among lymph node metastasis-related DEGs, the Search Tool for the Retrieval of Interacting Genes/Proteins Database (http://string-db.org) was used to construct a PPI network with a reliable filtering condition (score >0.3). The node gene score was determined using the “cytoHubba” plugin of the Cytoscape software (version 3.9.1), and the top 30 node genes scored by the degree algorithm were defined as hub genes.
2.6 Predictive signature construction
The least absolute shrinkage and selection operator (LASSO) binomial logistic regression analysis was used to regress the high-dimensional prediction factors, as previously reported (9). Univariate and multivariate Cox regression analyses were conducted to further screen for lymph node metastasis-related genes and establish a predictive signature. The risk score of the predictive signature was calculated as follows: Risk score = (exp-Gene1*coef-Gene1) + (exp-Gene2*coef-Gene2) +……+ (exp-Genei*coef-Genei). The patients were divided into high- and low-risk groups based on their median risk scores. The Kaplan–Meier survival curve was used to evaluate survival differences between the two groups. Time-dependent receiver operating characteristic (ROC) curves were used to evaluate survival predictions, and the “timeROC” package was used to calculate the area under the ROC curve (AUC) to assess the accuracy of the prognostic predictive signature. Univariate and multivariate Cox regression analyses were conducted to compare the hazard ratio of the predictive signature with characteristic clinical features of cervical cancer.
2.7 Nomogram construction and validation
To determine the effective clinical application of the predictive signature, a nomogram for predicting the 1-, 3- and 5-year survival rate of patients with CESC was constructed by combining the results of the risk score through the “rms” package in R. The predictive accuracy and discrimination ability of the nomogram were assessed using a calibration plot and the c-index. The sensitivity and specificity of the nomogram were evaluated using the AUC.
2.8 Copy number variation and somatic alteration data analysis
Compliant datasets were subjected to CNV analysis. CNVs of the genes screened through LASSO binomial logistic regression analysis in patients with CESC were analyzed. The somatic mutation data of CESC between the high- and low-risk groups, which were classified using the predictive signature, were obtained using the “maftools” package. The tumor mutation burden (TMB), which has been proposed as an immunotherapy efficacy predictor, of each patient with CESC was calculated using the “tmb ()” function of the “maftools” package in R. The correlation between the TMB and risk score was analyzed using the Wilcoxon rank-sum test.
2.9 Immune cell infiltration and immunotherapy response analyses
The CIBERSORT deconvolution algorithm, which is based on the principles of linear support vector regression, was used to obtain 22 immune cell infiltrations in CESC samples according to the known reference set LM22 with P values <0.05 (10). The stromal content (StromalScore), immune infiltration (ImmuneScore), and combined score (ESTIMATEScore) of each CESC sample were calculated by applying the ESTIMATE algorithm. The single-sample gene set enrichment analysis (ssGSEA) algorithm was used in the “GSVA” package to evaluate the relative abundance of infiltrated immune cells. Immune cell type-related marker genes were obtained from Bindea et al (11). The association between the risk score of the predictive signature and the expression of immune checkpoint genes, such as programmed cell death protein ligand 1 (PD-L1), cytotoxic T-lymphocyte-associated protein 4 (CTLA-4), and human leukocyte antigen (HLA), was assessed.
2.10 Drug sensitivity analysis
The Genomics of Drug Sensitivity in Cancer (GDSC) (https://www.cancerrxgene.org/ ) database was used to obtain tumor drug response data and genomic drug sensitivity markers (12). The half-maximal inhibitory concentration (IC50) values of common drugs between the high-risk and low-risk groups of patients with CESC, which were grouped using the predictive signature, were estimated using the pRRophetic algorithm in R.
2.11 Human tissue samples and cell line
Seven cervical cancer (three cases with lymph node metastasis and four cases without lymph node metastasis) and three normal cervical tissue samples were collected from patients with cervical cancer who underwent hysterectomy before chemotherapy and radiotherapy. The median age of the seven cases was 48 years (range, 32–58). Six cases were HPV positive, and one case was HPV negative. Five cases were squamous cell carcinoma, and two cases was adenocarcinoma. All tissues were stored at −80°C for RNA extraction. The studies involving human participants was approved by the Ethics Committee of the Second Affiliated Hospital of Zhengzhou University (2021040).
The human cervical cancer cell line, SiHa, was obtained from Procell Life Science & Technology Co., Ltd. (Wuhan, China) and cultured in Dulbecco’s modified Eagle’s medium (DMEM) supplemented with 10% fetal bovine serum (Gibco, Grand Island, USA). SiHa cells were maintained in a 5% CO2 and 95% air incubator.
2.12 RNA extraction and quantitative real-time PCR
Total RNA was extracted from tissues and cells using TRIzol reagent (Invitrogen, Carlsbad, CA, USA). The purity and quantity of RNA was determined by measuring the absorbance at 260/280 nm using a SmartSpec Plus Spectrophotometer (Bio-Rad Laboratories, Inc., Hercules, CA, USA). Reverse transcription was carried out using the ReverTra Ace qPCR RT Kit (TOYOBO Life Science, Shanghai, China), according to the manufacturer’s instructions. qRT-PCR was performed using the Bestar SYBR Green qPCR Master Mix (TOYOBO) on a Bio-Rad S1000 system. For quantitative RT-PCR, GAPDH was used as an endogenous control, using the 2−ΔΔCT method. All primer sequences are listed in Supplementary Table 1.
2.13 Cellular transfection
Small interfering RNAs (siRNA) directed against TEKT2 (si-TEKT2) and negative control RNAs (si-NC) were synthesized by GeneCreate Bioengineering Co., Ltd. (Wuhan, P.R. China). Transfections were performed using Lipofectamine 2000 (Invitrogen). The siRNA sequences are listed in Supplementary Table 2.
2.14 Colony formation assay
For the colony formation assay, 1.5 × 103 SiHa cells were maintained in 6-well culture plates. After 14 d of incubation, the colonies were fixed with methanol and stained with a crystal violet solution. Colonies containing more than 50 cells were counted.
2.15 Wound healing assay
Approximately 5 × 105 SiHa cells per well were seeded in 6-well culture plates. After 24 h of incubation, the center of each well was scraped with a 200 μl sterile pipette tip. The cells were washed three times with phosphate-buffered saline, and fresh DMEM was added. The wound was imaged at three time points after 0, 24, and 48 h.
2.16 Apoptosis analysis
Cell apoptosis was analyzed using an Annexin V-FITC Apoptosis Detection Kit (Solarbio, Beijing, China), according to the manufacturer’s recommendations, using a FACSCalibur flow cytometer (BD Biosciences, USA).
2.17 Statistical analysis
All statistical analyses and illustrations were performed using R software (version 4.1.2, https://www.r-project.org/). All P values were two-sided, with P <0.05 defined as statistically significant. Wilcoxon’s test was used to compare differences between two groups, and one-way analysis of variance and Kruskal–Wallis tests were used as parametric and non-parametric methods, respectively, for comparisons among three or more groups.
3 Results
3.1 Identification of DEGs associated with lymph node metastasis
We downloaded the expression profiles and clinical data of 307 patients with CESC from the TCGA-CESC database. Excluding data without lymph node metastases (Nx), 193 CESC patients were enrolled for subsequent analysis. The baseline data, including clinical and pathological characteristics, are presented in Table 1. The median patient age was 46 years (range, 29–72 years). Kaplan–Meier survival curves showed that the OS rate of patients with CESC in the lymph node metastasis (N1) group was significantly lower than that in the non-lymph node metastasis (N0) group (P = 0.0029, Figure 1A), indicating that lymph node metastasis was associated with survival of patients with CESC and lymph node metastasis-related genes might serve as prognostic biomarkers.
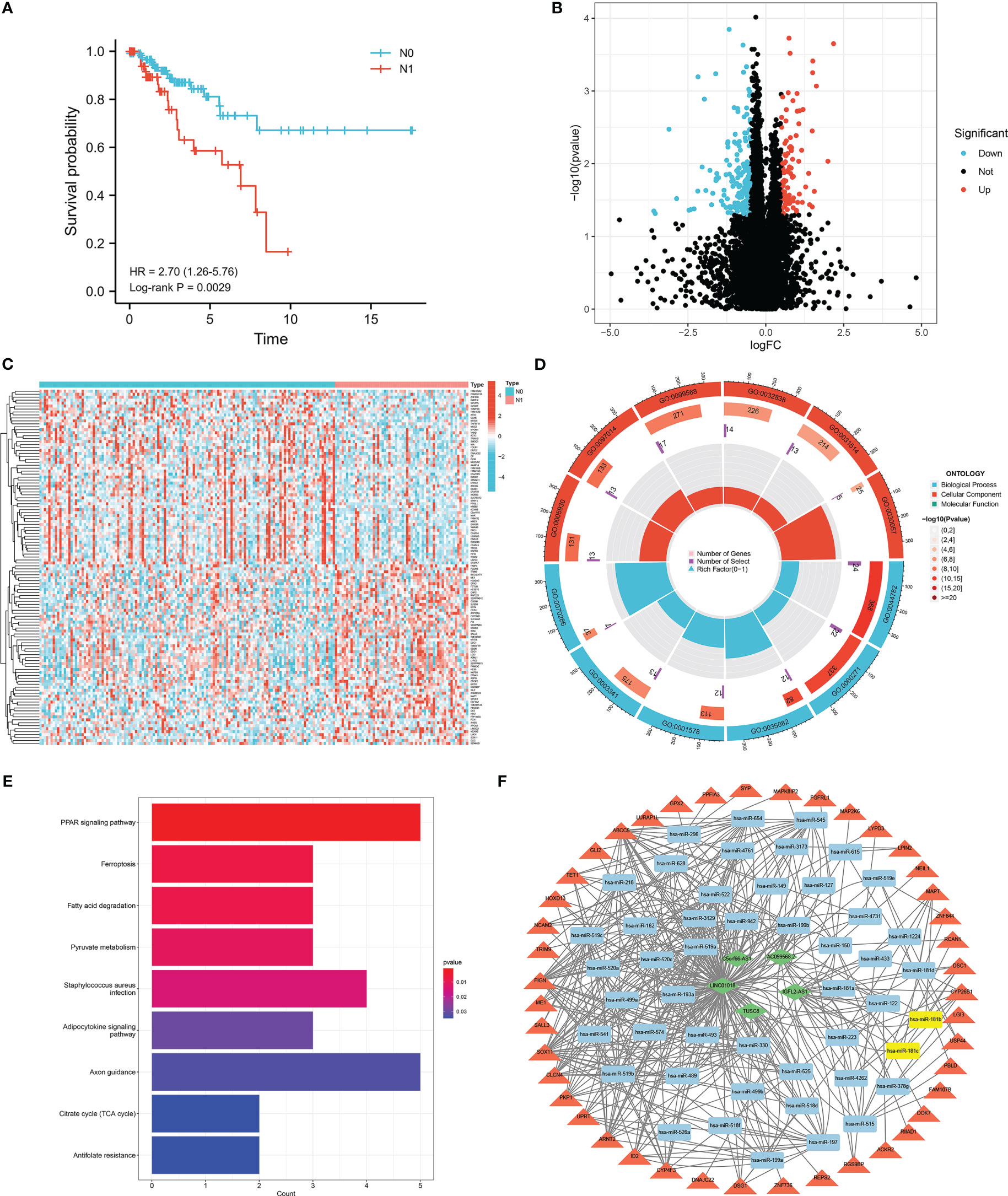
Figure 1 Identification of DEGs related to lymph node metastasis. (A) Survival analyses of lymph node metastasis and non-lymph node metastasis in TCGA-CESC database. (B) Volcano plot of DEGs related to lymph node metastasis. (C) The heatmap of 60 up-regulated and 60 down-regulated DEGs between cervical cancer patients with and without lymph node metastasis. (D) Gene Ontology (GO) functional analysis of 271 DEGs, including biological process (BP), and cellular component (CC). (E) Kyoto Encyclopedia of Genes and Genomes (KEGG) pathways of 271 DEGs related to lymph node metastasis. (F) lncRNA-miRNA-mRNA ceRNA network in cervical cancer with lymph node metastasis. Diamond, round rectangle and triangle represent differentially expressed lncRNA, miRNA, and DEGs, respectively. Yellow nodes indicate differentially expressed miRNA.
A total of 271 lymph node metastasis-related DEGs, including 100 upregulated and 171 downregulated genes, were obtained (Figure 1B). The heatmap showed 60 upregulated and 60 downregulated genes between the N0 and N1 groups of patients with CESC (Figure 1C). To investigate the different biological processes of the DEGs, GO and KEGG pathway enrichment analyses were performed. The main biological functions were the axoneme, cytoplasmic region, cilium organization, and cilium assembly (Figure 1D). DEGs were mainly enriched in the PPAR signaling pathway, ferroptosis, fatty acid degradation, and pyruvate metabolism (Figure 1E).
To find out whether these DEGs existing competing endogenous regulating network, a lncRNA-miRNA-mRNA ceRNA network of cervical cancer with lymph node metastasis was constructed. A total of 5 differentially expressed lncRNAs and 48 miRNAs were paired into 228 lncRNA-miRNA interactions, whereas 48 miRNAs and 43 DGEs were matched to form 228 pairs of miRNA-mRNA interactions (Figure 1F).
3.2 PPI network construction and lymph node metastasis-related hub gene screening
We conducted further interactive network analysis of the lymph node metastasis-related DEGs and found that there were 30 hub genes scored by the degree algorithm (Figures 2A, C). The expression of the 30 hub genes between the N0 and N1 groups is shown in Figure 2B. LASSO binomial logistic regression analysis was performed to further screen hub genes related to lymph node metastasis. The analysis results revealed 16 hub genes (Figure 2D, E). The expression of FAM163B, TEKT2, EFHC1, ENKUR, PPARGC1A, EFHC2, and RPGR was lower in the N1 group than in the N0 group (Figure 2F). In contrast, EEF1A2, MAPT, CALML5, SERPINB13, CALB1, KRT4, HCN2, SYP, and AHSG expression was upregulated in the N1 group (Figure 2F). Additionally, a correlation was found among the 16 hub genes (Figure 2G).
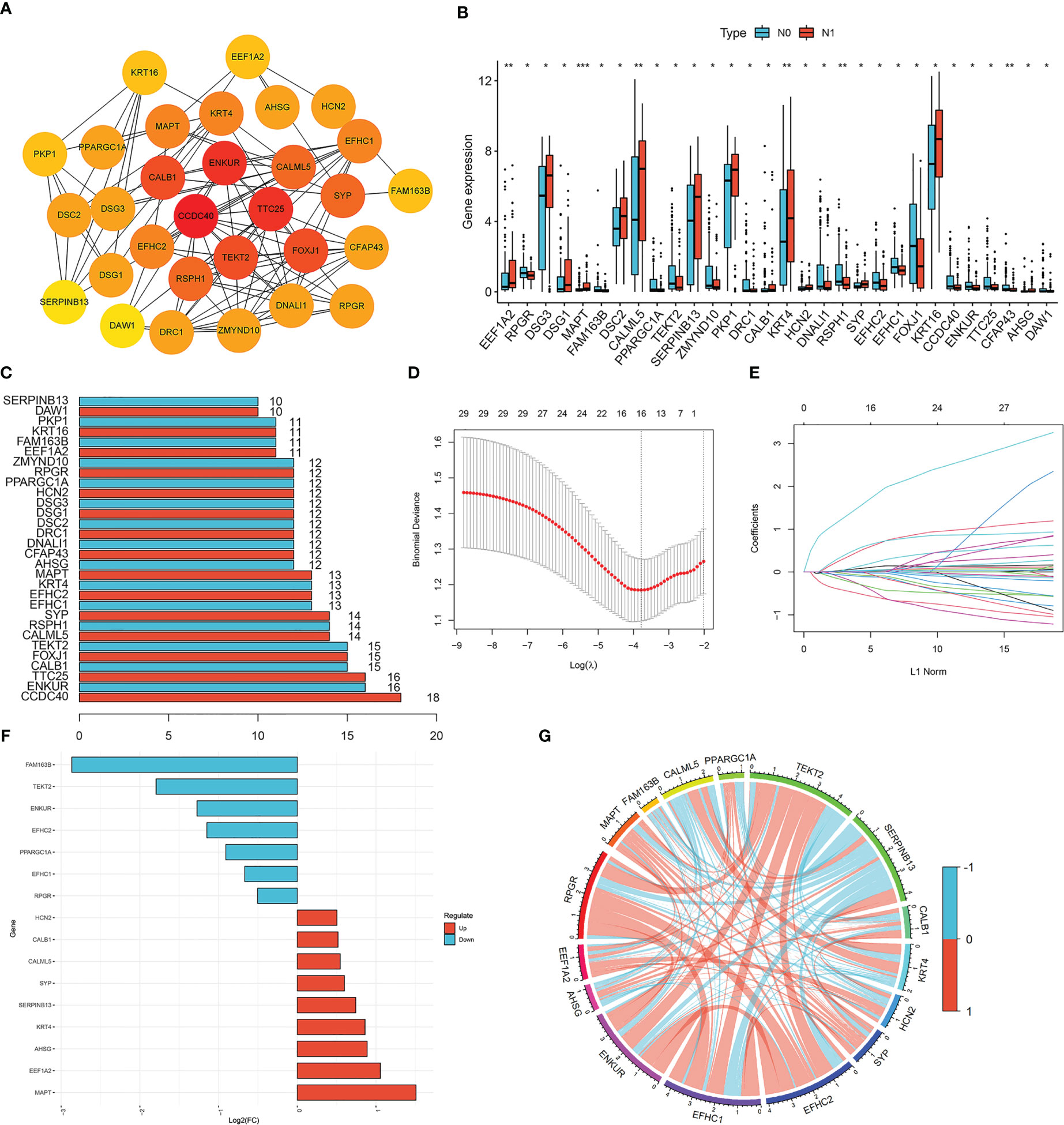
Figure 2 Screening of lymph node metastasis-related hub genes. (A) Protein-protein interaction (PPI) network of DEGs related to lymph node metastasis. (B) The expression of 30 hub genes between cervical cancer patients with and without lymph node metastasis. Red represents lymph node metastasis, and blue represents non-lymph node metastasis. The asterisks represented the statistical p value (*P < 0.05; **P < 0.01; ***P < 0.001). (C) The number of genes associated with other hub genes. (D, E) LASSO binomial logistic regression analysis for further screening of hub genes. (F) The expression of 16 hub genes between cervical cancer patients with and without lymph node metastasis. (G) Correlation analysis of 16 hub genes in cervical cancer. Blue represents negative correlation, and red represents positive correlation.
3.3 Establishment of the prediction signature and nomogram
To investigate the prognostic value of the 16 lymph node metastasis-related hub genes, univariate and multivariate Cox regression analyses were performed to calculate the hazard ratio and establish a lymph node metastasis-related predictive signature. Risk score = (−0.7983 × expression level of RPGR) + (−0.6974 × expression level of TEKT2). A total of 193 patients with CESC were divided into high- and low-risk groups according to the median risk score, and the risk score curve was plotted (Figure 3B). Survival curves demonstrated that the survival rate in the high-risk group was notably lower than that in the low-risk group (P=0.003; Figures 3A, C). The expression heatmap showed that RPGR and TEKT2 was downregulated in the high-risk group (Figure 3D). The ROC curve was used to predict prognosis at 1, 3, and 5 years, and the prediction efficiency was satisfactory (1-year AUC = 0.765; 3-year AUC = 0.713; 5-year AUC = 0.667; Figure 3E). Univariate and multivariate Cox analyses showed that the predictive signature was an independent prognostic factor in patients with CESC (P<0.001; Figures 3F, G). Furthermore, a nomogram was constructed to predict the 1-, 3-, and 5-year survival rates of patients with CESC (Supplementary Figure 1A), and the calibration plot confirmed its effective predictive performance (Supplementary Figure 1B–D). The c-index was 0.705, and the AUCs for the 1-, 3-, and 5-year survival curves of patients with CESC were 0.760, 0.718, and 0.687, respectively (Supplementary Figure 1E).
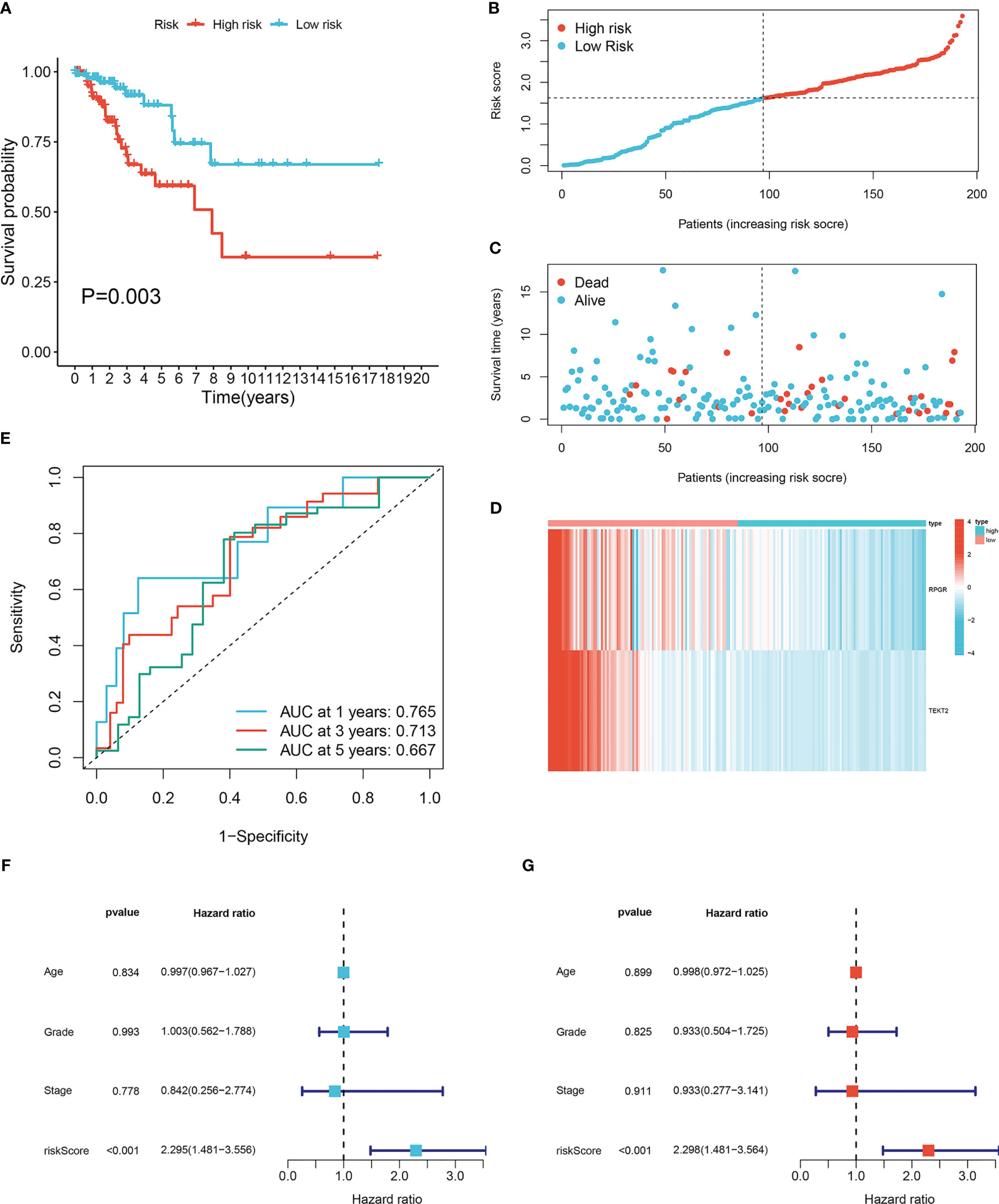
Figure 3 Construction lymph node metastasis-related predictive signature. (A) Kaplan-Meier survival curves of high- and low-risk groups in cervical cancer. (B) Cervical cancer patients were divided into high- and low-risk groups according to the median of the risk score. (C) Risk score and survival status for each cervical cancer patient. (D) The heatmap of the expression levels of RPGR and TEKT2 between high- and low-risk groups. (E) ROC curve for 1-, 3-, and 5-years survival of the predictive signature. (F) Univariate Cox analysis of the clinicopathological features and risk predictive signature. (G) Multivariate Cox analysis of the clinicopathological features and risk predictive signature.
3.4 Correlations between prediction signature and clinicopathological characteristics
We investigated the correlation between the lymph node metastasis-related prediction signature and clinicopathological characteristics of cervical cancer. Stratified analysis indicated significant differences in the risk score of the prediction signature among groups divided by age and stage and pathological type, but not by grade (Figures 4A, C, E, G). The proportion of patients with CESC in the high-risk group with clinicopathological characteristics including age ≤35 years, age >65 years, stage IIB-IVB, and stage G2 and pathological type squamous cell carcinoma (SCC) was higher than those in the low-risk group (Figures 4B, D, F, H). Kaplan–Meier survival curves showed that the patients with CESC in the low-risk group with clinicopathological characteristics including age >35 years, age ≤65 years, and stage IA-IIA, G1, and G3, and pathological type SCC had better prognosis than those in the high-risk group (P < 0.05; Figure 4I–L). Conversely, there was no significant difference in prognosis between the high- and low-risk groups of patients with CESC characterized by age ≤35 years, age >65 years, and stage IIB-IVB and G2 and pathological type adenocarcinoma (AC), adenosquamous carcinoma (ACS).
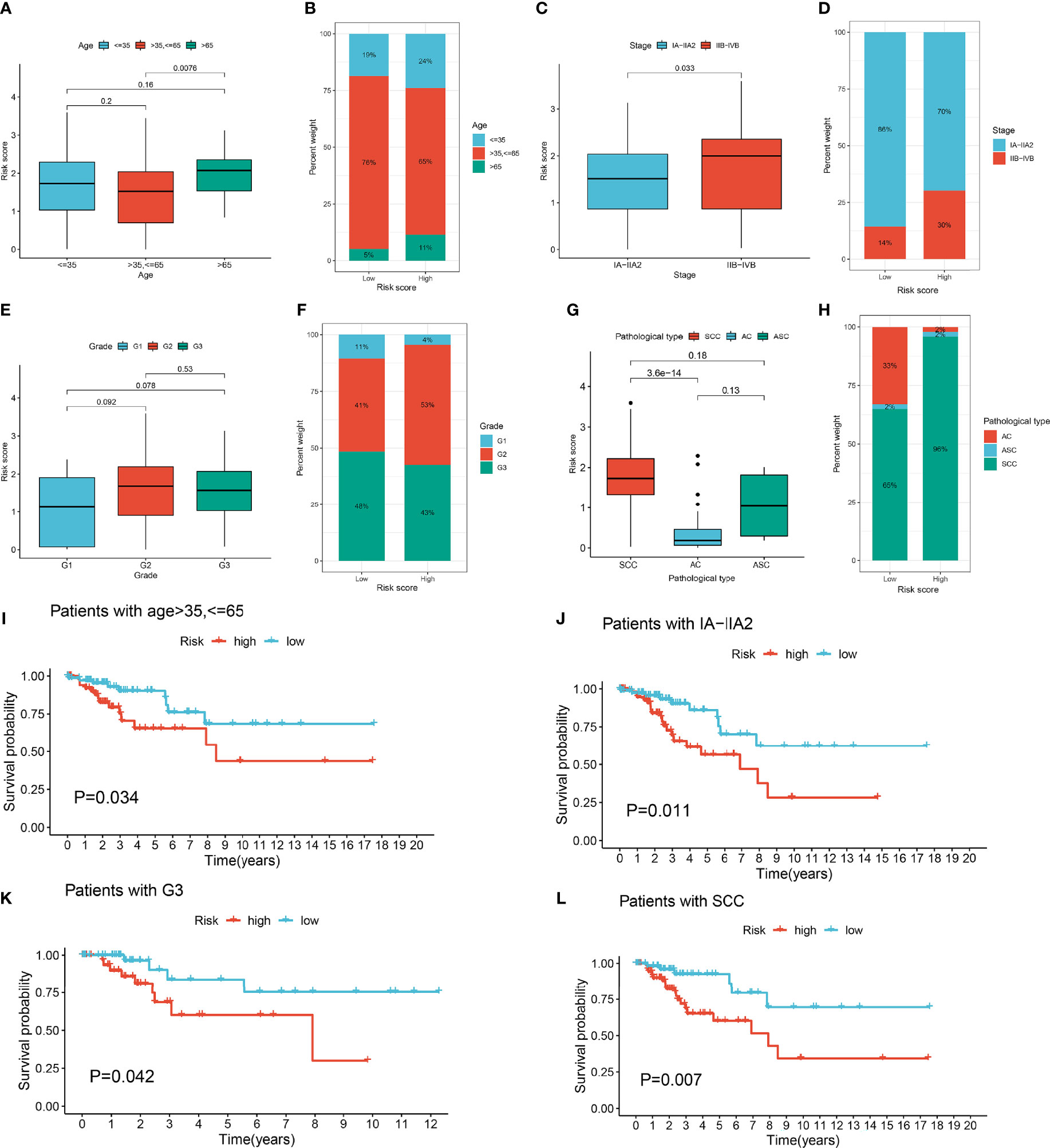
Figure 4 Clinical correlation analysis of lymph node metastasis-related prediction signature in cervical cancer. (A) Boxplots of risk score in different age groups. (B) The proportion of different age groups between high- and low-risk groups. (C) Boxplots of risk score in different stage groups. (D) The proportion of different stage groups between high- and low-risk groups. (E) Boxplots of risk score in different grade groups. (F) The proportion of different grade groups between high- and low-risk groups. (G) Boxplots of risk score in different pathological type groups. (H) The proportion of different pathological type groups between high- and low-risk groups. (I) Kaplan-Meier survival curves of age >35, <=65 in high- and low-risk groups. (J) Kaplan-Meier survival curves of IA-IIA2 in high- and low-risk groups. (K) Kaplan-Meier survival curves of G3 in high- and low-risk groups. (L) Kaplan-Meier survival curves of pathological type squamous cell carcinoma (SCC) in high- and low-risk groups.
3.5 Influence of prediction signature on genomic changes and biological function
To detect the genetic features and potential biological behavior of the lymph node metastasis-related prediction signature, we first analyzed the CNVs of the 16 lymph node metastasis-related hub genes. Analysis revealed that CNV alterations in the 16 hub genes were prevalent, with higher probabilities of CNV deletions in HCN2, TEKT2, and PPARGC1A and CNV amplifications in AHSG, FAM163B, EFHC1, and RPGR (Figure 5A).
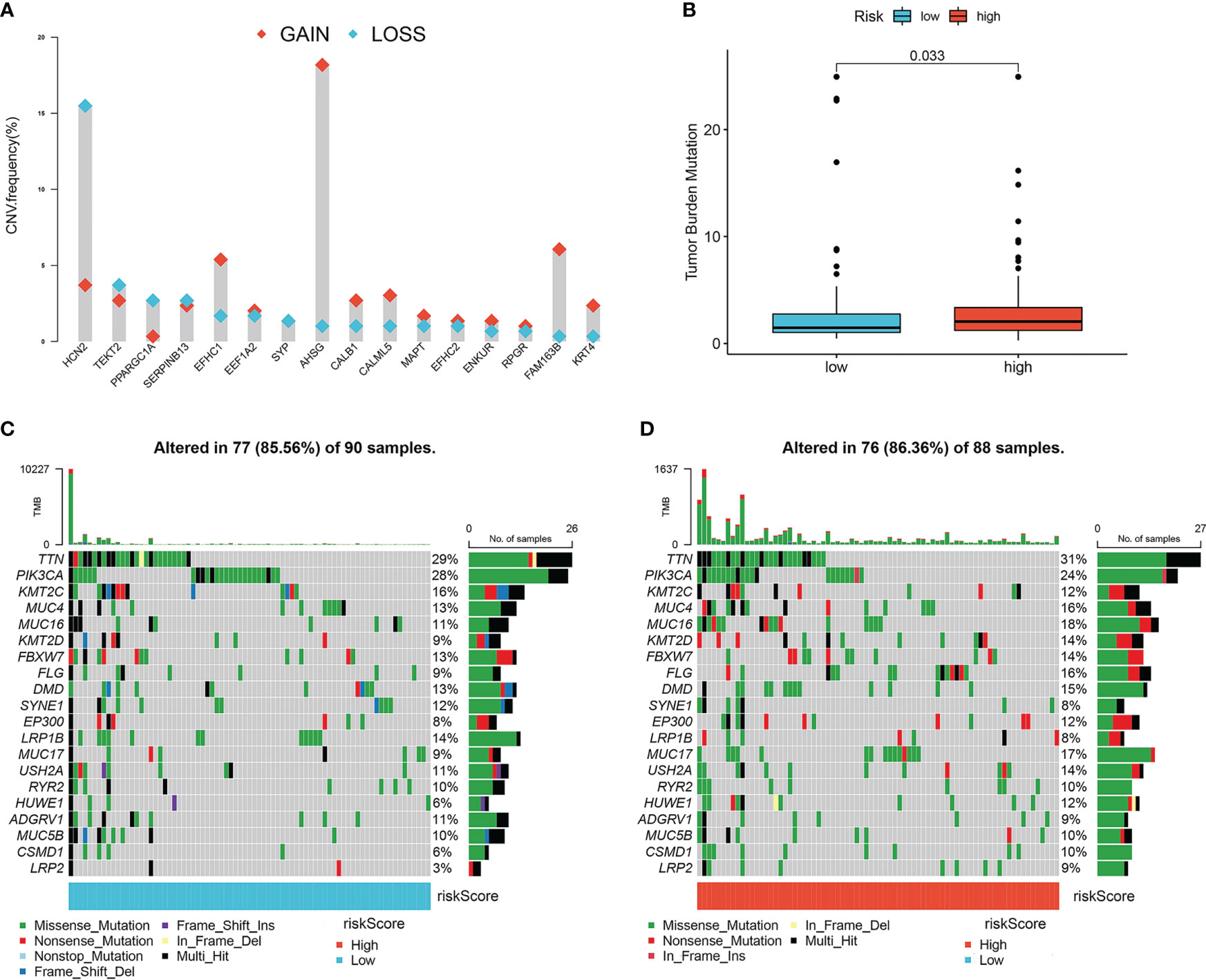
Figure 5 Characteristics of tumor somatic mutations in lymph node metastasis-related predictive signature. (A) The CNV variation frequency of 16 hub genes. Red represents an increase in copy number, and blue represents loss of copy number. (B) Boxplots of tumor mutation burden between high- and low-risk groups. (C) The mutation frequency of low-risk groups of cervical cancer patients. (D) The mutation frequency of high-risk groups of cervical cancer patients.
We then compared the TMB and somatic variations between the high- and low-risk groups. The TMB score in the high-risk group was higher than that in the low-risk group (P=0.033; Figure 5B), which indicated that the high-risk group was more likely to benefit from PD-1 inhibitors. Missense mutations accounted for most mutations, and TTN was the most common mutated gene (Figures 5C, D). The high-risk group had a higher somatic mutation rate than the low-risk group (86.63% vs. 85.56%).
Finally, we conducted GSVA analysis between the high- and low-risk groups. The main biological roles were wide pore channel activity, desmosome organization, and regulation of epidermis development in the high-risk group and extracellular transport and protein transport along microtubules in the low-risk group (Supplementary Figure 2A). Toll-like receptor, VEGF, P53, T cell receptor, B cell receptor, JAK STAT, TGF-β, and natural killer cell-mediated cytotoxicity signaling pathways were positively correlated with the risk score of the prediction signature (Supplementary Figures 2B, C).
3.6 Influence of prediction signature on immune characteristics
To investigate the relationship between the lymph node metastasis-related prediction signature and immune cell infiltration in the CESC microenvironment, we calculated the proportions of 22 immune cell types in 193 samples using the CIBERSORT algorithm. The panorama of immune cell infiltration in the CESC microenvironment is shown in Figure 6A, and the correlations between the 22 immune cell types is shown in Figure 6B. Resting memory CD4 T cells showed the highest negative correlation with CD8 T cells (cor =−0.59), followed by activated memory CD4 T cells (cor = −0.46). Activated memory CD4 T cells displayed the highest positive correlation with CD8 T cells (cor = 0.49). We then compared the infiltration of immune cell types between the high- and low-risk groups using ssGSEA (Figure 6C). Immune infiltration of activated CD8 T cells, activated CD4 T cells, activated dendritic cells, monocytes, regulatory T cells, and type 1 T helper cells was higher in the high-risk group than in the low-risk group (P <0.05). Using ssGSEA, we also compared the relationship between TEKT2 and RPGR and differentially distributed immune cells (Supplementary Figure 3A). TEKT2 and RPGR expression levels were negatively correlated with almost all immune cells, including gamma delta T cells, macrophages, natural killer T cells, regulatory T cells, T follicular helper cells, and type 1 T helper cells (P <0.05). Furthermore, the ESTIMATE algorithm was applied to determine the differences in the stromal content, immune infiltration, and combined score between the high- and low-risk groups (Figure 6D). The results showed that the ImmuneScore and ESTIMATEScore in the high-risk group were higher than those in the low-risk group (P <0.05).
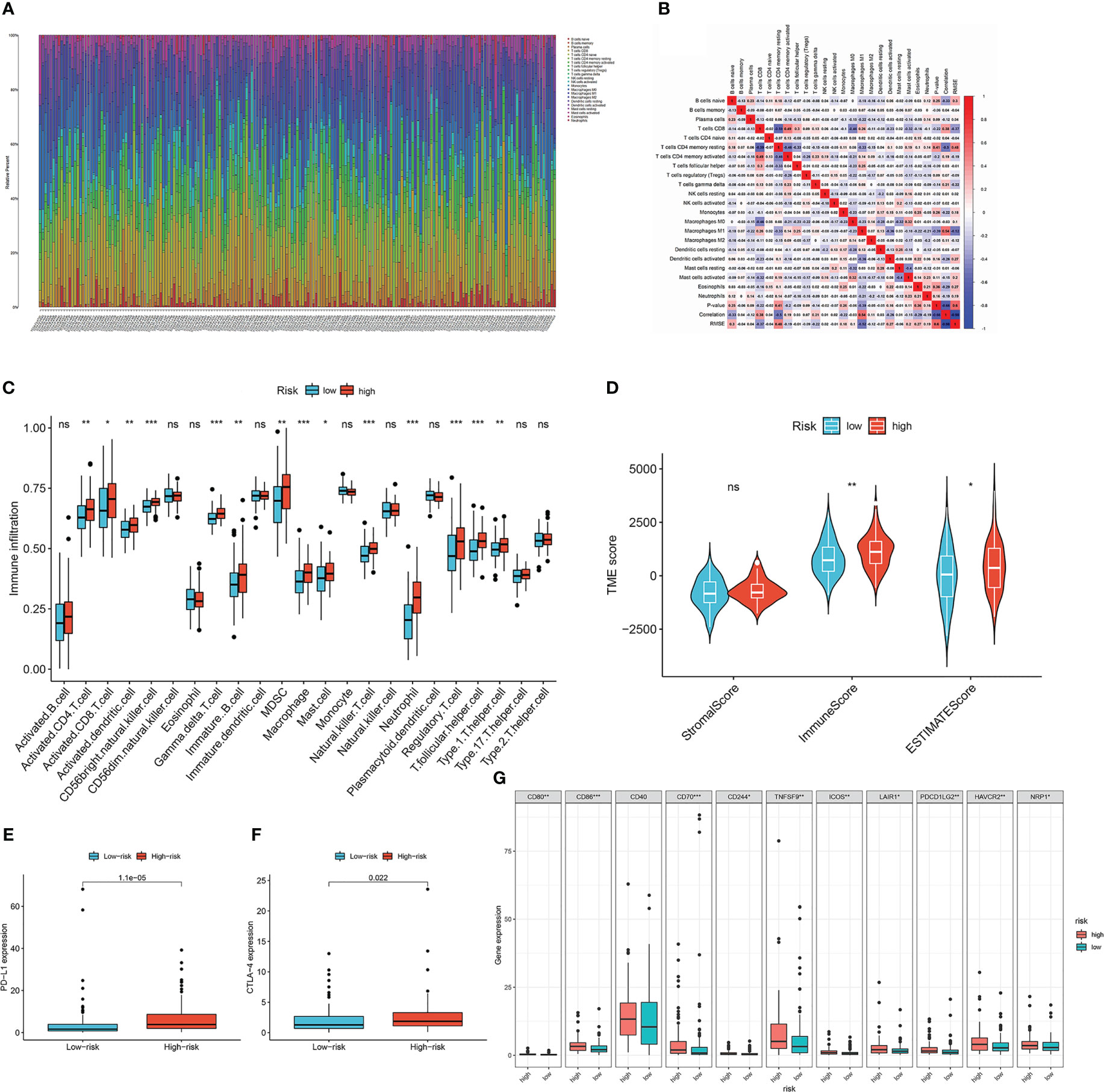
Figure 6 Characteristics of tumor microenvironment in lymph node metastasis-related predictive signature. (A) The panorama of immune cell infiltration in TME of cervical cancer. (B) Correlation analysis of 22 types of immune cells in cervical cancer. Blue represents negative correlation, and red represents positive correlation. (C) The infiltration of immune cell types between the high- and low-risk groups by ssGSEA analysis. The asterisks represented the statistical p value (*P < 0.05; **P < 0.01; ***P < 0.001). (D) Immunological score versus stromal score in high- and low-risk groups by ESTIMATE analysis. The asterisks represented the statistical p value (*P < 0.05; **P < 0.01). (E) Boxplots of the expression of PD-L1 in high- and low-risk groups. (F) Boxplots of the expression of CTLA-4 in high- and low-risk groups. (G) Differential expression analysis of other checkpoint genes in high- and low-risk groups. The asterisks represented the statistical p value (*P < 0.05; **P < 0.01; ***P < 0.001).
To predict the immunotherapy response of patients with CESC, we detected the differential expression of checkpoint genes in the low- and high-risk groups (Figures 6E–G). Patients in the high-risk group showed high expression of PD-L1, CTLA-4, CD80, CD86, CD70, CD244, TNFSF9, ICOS, LAIR1, PDCD1LG2, HAVCR2, and NRP1 (P <0.05). TEKT2, RPGR, and the risk score of the lymph node metastasis-related prediction signature were significantly correlated with checkpoint genes (Supplementary Figure 3B). The expression of TEKT2 and RPGR was negatively correlated with that of PD-L1 and CTLA-4, whereas the risk score was positively correlated with the expression of PD-L1 and CTLA-4, which was consistent with the results shown in Figures 6E–G. Moreover, we analyzed the differential expression of human leukocyte antigen (HLA) family genes in the high- and low-risk groups (Supplementary Figure 3C). Excluding HLA-E and HLA-DQA2, there were no statistically significant differences between the two groups (P >0.05).
3.7 Prediction signature sensitivity-based analysis of chemotherapy drugs
Considering that chemotherapy is an adjuvant treatment for CESC, we accessed the GDSC database to evaluate susceptibility to common antineoplastic agents in the high- and low-risk groups. We calculated the IC50 values of 138 chemotherapy drugs and analyzed the relationship between the risk groups and IC50 values. The IC50 estimates of docetaxel, paclitaxel, etoposide, erlotinib, lapatinib, sunitinib, gefitinib, and vinorelbine in the low-risk group were significantly higher than those in the high-risk group, whereas the IC50 estimates of cytarabine, FH535, and procaspase-activating compound-1 (PAC-1) in high-risk group were significantly higher than those in the low-risk group (Figure 7). Collectively, these results proved that the risk score of the lymph node metastasis-related prediction signature had good reliability.
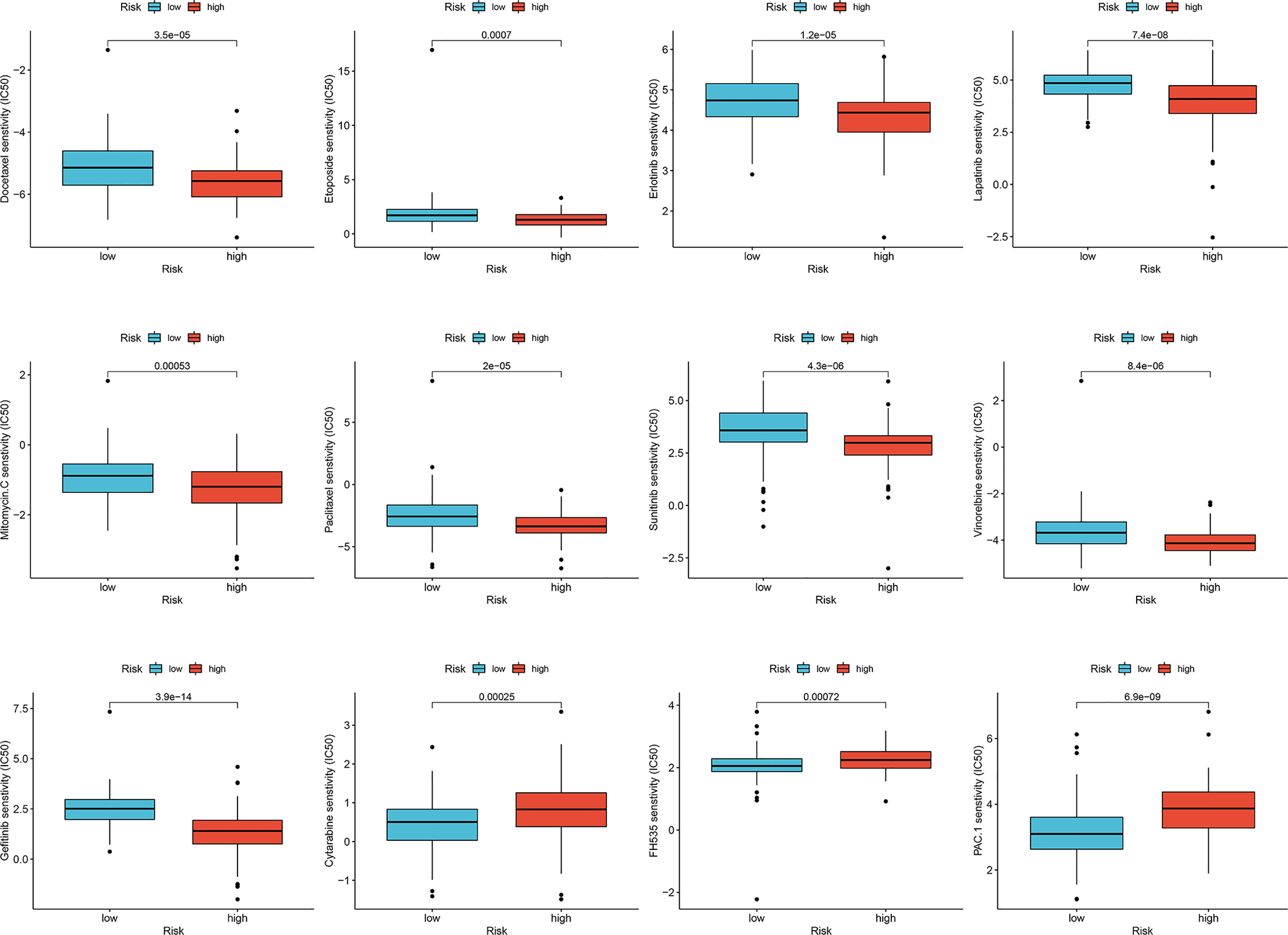
Figure 7 Sensitivity of drugs in lymph node metastasis-related predictive signature. Differences in IC50 values for high- and low-risk groups in cervical cancer.
3.8 Expression and function of TEKT2 and RPGR in cervical cancer
To clarify the role of TEKT2 and RPGR in cervical cancer, we investigated TEKT2 and RPGR expression in seven cervical cancer tissues and three normal cervical tissues. RT-qPCR results revealed that the mRNA expression of TEKT2 and RPGR was significantly downregulated in tumor samples, especially in metastatic lymph node samples (Figure 8A). Analysis of the Human Protein Atlas data revealed that TEKT2 and RPGR protein levels were also downregulated in cervical cancer samples (Figure 8C).
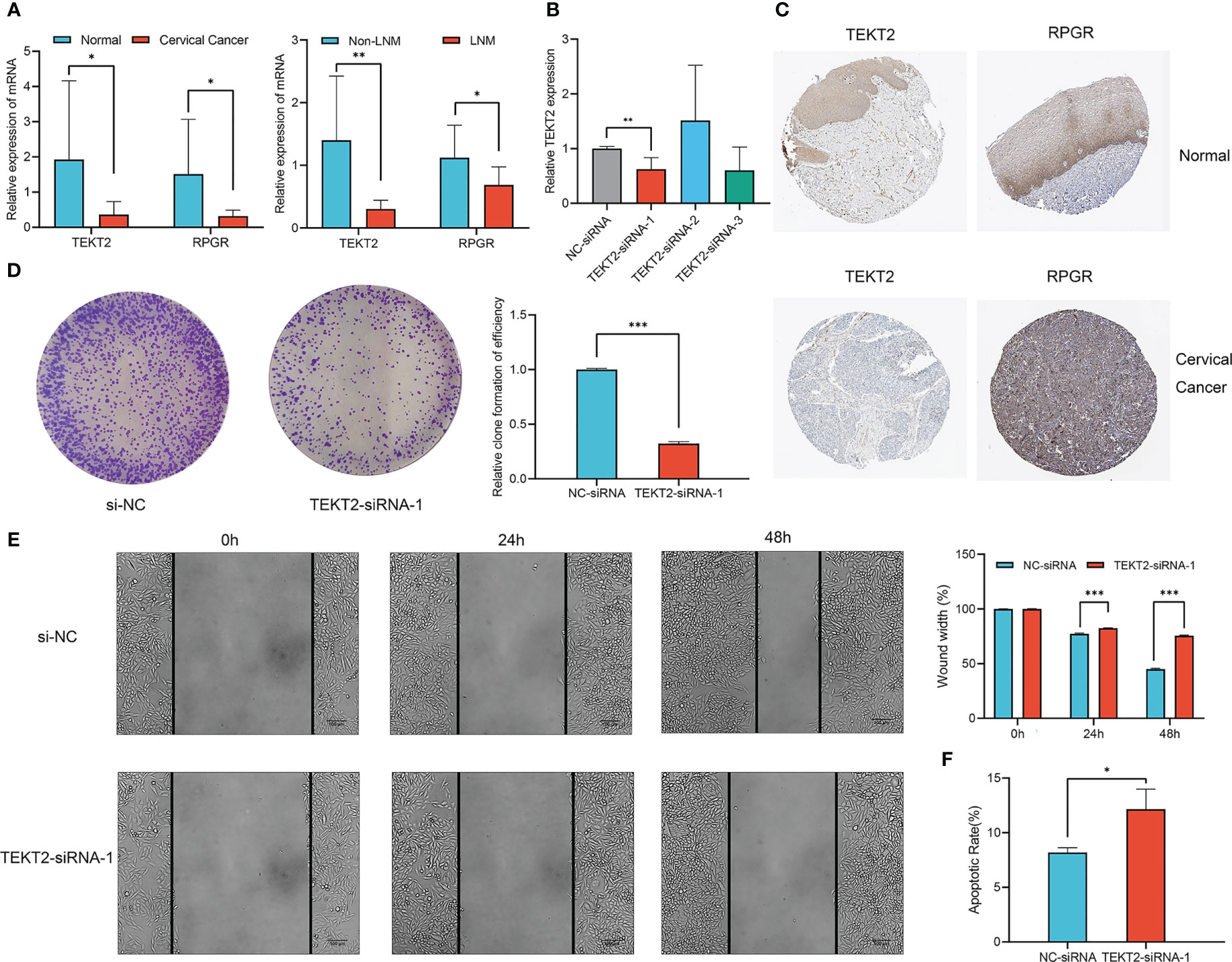
Figure 8 Expression and function of TEKT2 and RPGR in Cervical Cancer. (A) The mRNA expression of TEKT2 and RPGR in cervical cancer tissues versus normal cervical tissues, and cervical cancer tissues with lymph node metastatic versus cervical cancer tissues without lymph node metastatic. The asterisks represented the statistical p value (*P < 0.05; **P < 0.01). (B) The transfection knockdown efficiency of TEKT2 in the SiHa cell line using siRNA. The asterisks represented the statistical p value (**P < 0.01). (C) The protein expression of TEKT2 and RPGR in cervical cancer tissues and normal cervical tissues in Human Protein Atlas data. (D) Proliferation of SiHa cells was detected by colony formation after TEKT2 knockdown. The asterisks represented the statistical p value (***P < 0.001). (E) Migration of SiHa cells was detected by wound healing assay after TEKT2 knockdown. The asterisks represented the statistical p value (***P < 0.001). (F) Apoptosis of SiHa cells after TEKT2 knockdown. The asterisks represented the statistical p value (*P < 0.05).
To explore the function of TEKT2 in cervical cancer, TEKT2 was efficiently knocked down in the SiHa cell line using siRNA. RT-qPCR validated the transfection knockdown efficiency (Figure 8B). The colony formation assay revealed that the proliferation of SiHa cells decreased after TEKT2 knockdown (Figure 8D). Moreover, TEKT2 knockdown remarkably reduced the migration and increased the apoptosis of SiHa cells (Figure 8E, F).
4 Discussion
Cervical cancer is a common malignancy that affects women’s health. In developing countries, especially India and China, cervical cancer has higher incidence and mortality rates (13). Human papillomavirus (HPV) is a critical inducing agent in the development of cervical cancer and is detected in more than 90% of cases (14, 15). Early stage cervical cancer is successfully managed with radical hysterectomy with pelvic lymphadenectomy, and more advanced-stage tumors are treated with chemoradiotherapy (16). However, few effective treatment options exist for recurrent or metastatic cervical cancer (17). Recently, sequencing analysis of a large number of clinical tissues has improved our understanding of the molecular signatures of cervical cancer (18). Thus, exploring new therapeutic targets and biomarkers using bioinformatic analyses has enabled precise treatment options, which could significantly improve patient outcomes.
Lymph node metastasis is common in cervical cancer and has prognostic implications for patients (19). Recent studies suggest that lymphatic vessels do not merely provide channels for tumor spread, but also promote tumor cell recruitment to lymph nodes, immune modulation, and cancer stem cell survival (20). In addition, epithelial-mesenchymal transition (EMT) is considered a biomarker of tumor cell metastasis and is closely related to lymph node metastasis (21). However, a detailed understanding of lymph node metastasis in cervical cancer is lacking.
Here, we downloaded the expression profiles and clinical data of 193 patients with CESC from the TCGA-CESC database. A total of 271 lymph node metastasis-related DEGs were identified, including 100 upregulated and 171 downregulated genes. Through interactive network and LASSO binomial logistic regression analyses, we identified 16 hub genes. Two genes, TEKT2 and RPGR, were screened from these 16 hub genes using univariate and multivariate Cox regression analyses to construct the lymph node metastasis-related prognostic gene signature. By calculating the risk score for each patient, we divided them into high- and low-risk groups. The signature demonstrated good performance in predicting the survival outcomes of patients with cervical cancer.
TEKT2 and other members of the tektin protein family are critical for cytoskeleton formation (22). Several studies have revealed that TEKT2 is associated with spermatogenesis and sperm flagellar movement (23). The expression of TEKT2 was downregulated in the lymph node metastasis group, suggesting that it is a protective factor in cervical cancer, which is consistent with the results of Yang et al. (24). RPGR encodes the GTPase regulator in retinitis pigmentosa, and its mutation is linked to severe multisystem diseases with strong retinal involvement of photoreceptor neurons (25). In addition, RPGR is one of the top driver oncogenes in breast cancer (26). Lin et al. (27) identified the regulatory competing endogenous RNAs of RPGR in nasopharyngeal carcinoma. However, the role of RPGR in cervical cancer remains unclear and requires further investigation. The expression of TEKT2 and RPGR was significantly downregulated in cervical cancer tissues, especially in lymph node metastatic tissues. TEKT2 knockdown inhibited cervical cancer cell proliferation and migration but promoted apoptosis. However, the biological function of TEKT2 in SiHa cells was inconsistent with the tissue expression analysis results, and thus requires further study.
TEKT2 and RPGR have been found to be associated with lymph node metastasis in cervical cancer. Interestingly, several studies have constructed different models for lung adenocarcinoma (28), bladder cancer (29), and thyroid cancer (30) to predict lymph node metastasis or prognosis. To better understand the genetic features and potential biological behavior of the lymph node metastasis-related predictive signature, we compared the TMB between high- and low-risk groups and performed GSVA analysis. The TMB score in the high-risk group was higher than that in the low-risk group. Higher TMB results in more neoantigens, increasing the chances of T cell recognition, and clinically correlates with better immune checkpoint inhibitor (ICI) efficacy (31). GSVA analysis revealed that T and B cell receptors were positively correlated with the risk score, which indicated that the prediction signature had a strong relationship with immunity.
Cervical cancer is significantly associated with persistent HPV infection. Lymph nodes are common sites of cervical cancer metastasis, and tumor cells can interact with the host immune system by controlling the infiltration and reactivity of immune cells (32). This indicates that lymph node metastasis may be closely associated with immune cell infiltration. Therefore, we compared the infiltration of immune cells between the high- and low-risk groups and found that the abundance of CD8 + T cells, activated CD4 + T cells, and activated dendritic cells was significantly higher in the high-risk group than in the low-risk group. Similarly, our findings illustrated that the ESTIMATEScore, which is defined as the percentage of tumor cells in the tumor microenvironment (TME), was also higher in the high-risk group than in the low-risk group.
Our results showed that the high-risk group was characterized by immune activation and immune cell infiltration, classified as an immune-inflamed phenotype, and the low-risk group was characterized by the suppression of immunity, classified as an immune-desert phenotype. The immune-inflamed phenotype, known as hot tumor, is sensitive to immunotherapy, while the immune-desert phenotype, known as cold tumor, is insensitive to immunotherapy, revealing that the abundance of immune cell infiltration in the TME is closely linked to immunotherapy efficacy (33). To date, cervical cancer immunotherapy has mainly included ICIs, HPV-related vaccines, dendritic cell-based immunotherapy, and adoptive T cell immunotherapy (34). ICIs have demonstrated marked clinical effects worldwide, and the FDA has approved pembrolizumab for PD-L1 positive metastatic or recurrent cervical cancer (35). To predict the immunotherapy response, we detected the differential expression of checkpoint genes in the low- and high-risk groups. The high-risk group showed a notably high expression of checkpoint genes and was likely to respond well to ICIs. Thus, the lymph node metastasis-related predictive signature could be a reliable tool to evaluate immune cell infiltration in the TME and predict the clinical response to ICI treatment.
Although chemotherapy is a multimodal approach for the treatment of cervical cancer, responses to chemotherapy are limited (36). To identify the most effective antineoplastic agents in the high- and low-risk groups of patients with cervical cancer, we analyzed the IC50 values of 138 chemotherapeutic drugs using the GDSC database. Drug sensitivity analysis showed that docetaxel, paclitaxel, etoposide, erlotinib, lapatinib, sunitinib, gefitinib, and vinorelbine had stronger effects in the low-risk group, whereas cytarabine, PAC-1, and FH535 had stronger effects in the high-risk group. Docetaxel and paclitaxel are commonly used to treat cervical cancer. Both could promote tubulin assembly in microtubules, stabilize microtubules, and inhibit depolymerization to free tubulin, thus blocking cells in the M phase of the cell cycle, representing a class of antineoplastic agents (37). Erlotinib, lapatinib, sunitinib, and gefitinib are tyrosine kinase inhibitors, each of which inhibits cell proliferation and angiogenesis, and their therapeutic effects in advanced and recurrent cervical cancer are still in phase II/III clinical trials (38). Cytarabine is a nucleoside analog that affects cell division in the S phase and acts primarily through inhibition of DNA polymerase; it is mainly used to treat acute myeloid leukemia (39). PAC-1 is the first FDA-approved orphan drug, and its synthetic derivative WF-208 showed fascinating caspase-3-mediated anticancer activity (40). FH535 is a small molecule and dual inhibitor of β-catenin/TCF and PPARs, which has been demonstrated to selectively inhibit the proliferation of pancreatic, hepatocellular, breast, and colorectal carcinoma cells (41). According to current research, cytarabine, PAC-1, and FH535 are less commonly used for the treatment of cervical cancer. However, according to our results, they could also be effective in high-risk lymph node metastasis-related predictive signatures in cervical cancer.
Our study has some limitations. There is still a lack of large-scale clinical trials on TEKT2 and RPGR in cervical cancer with lymph node metastasis, which will be demonstrated in future studies. In addition, we only focused on mRNAs in this study. Several studies have found that non-coding RNAs, such as miRNAs, lncRNAs, and circRNAs, also have significant correlations with lymph node metastasis, which should be considered in further studies.
In summary, we constructed a lymph node metastasis-related predictive signature based on TEKT2 and RPGR expression to predict the prognosis, immune infiltration, and efficiency of individualized therapeutic agents. Our findings not only enrich our understanding of immunotherapy and chemotherapy response in patients with cervical cancer but also provide a new strategy for combining risk stratification with precision treatment of cervical cancer, which might provide novel insights for clinicians.
Data availability statement
The original contributions presented in the study are included in the article/Supplementary Material. Further inquiries can be directed to the corresponding authors.
Ethics statement
The studies involving human participants were reviewed and approved by the Ethics Committee of the Second Affiliated Hospital of Zhengzhou University (2021040). The patients/participants provided their written informed consent to participate in this study.
Author contributions
YG, LW, ZX and HZ designed the research. YG, LW, ML, XX and YB performed data collection and analysis. RL, WW, ML and ZX wrote the manuscript draft. YG, ML, XX, RL and HZ prepared the manuscript. All authors contributed to the article and approved the submitted version.
Funding
This research was supported by Henan Province Key Research and Development and Promotion (Science and Technology) Project (222102310651), Henan Province Medical Science and Technology Research Program Joint Construction Project (LHGJ20220476).
Acknowledgments
We thank all the researchers involved in the consolidation and submission of the data from the TCGA and GEO database, which may provide convenience and possibility of tumors studies in a large cohort.
Conflict of interest
The authors declare that the research was conducted in the absence of any commercial or financial relationships that could be construed as a potential conflict of interest.
The reviewer HL declared a shared parent affiliation with the authors to the handling editor at the time of the review.
Publisher’s note
All claims expressed in this article are solely those of the authors and do not necessarily represent those of their affiliated organizations, or those of the publisher, the editors and the reviewers. Any product that may be evaluated in this article, or claim that may be made by its manufacturer, is not guaranteed or endorsed by the publisher.
Supplementary material
The Supplementary Material for this article can be found online at: https://www.frontiersin.org/articles/10.3389/fonc.2023.1190251/full#supplementary-material
Supplementary Figure 1 | Establishment of nomogram for survival prediction. (A) Nomogram of risk score in lymph node metastasis-related predictive signature. (B) Calibration curve for 1-year survival of the nomogram. (C) Calibration curve for 3-years survival of the nomogram. (D) Calibration curve for 5-years survival of the nomogram. (E) ROC curve for 1-, 3-, and 5-years survival of the nomogram.
Supplementary Figure 2 | Functional enrichment analysis in lymph node metastasis-related predictive signature. (A) GO functional analysis between high- and low-risk groups in cervical cancer. (B) KEGG functional analysis between high- and low-risk groups in cervical cancer. (C) Correlation analysis between KEGG pathways and the expression of risk score, TEKT2 and RPGR. Blue represents negative correlation, and red represents positive correlation.
Supplementary Figure 3 | Assessment of immune microenvironment in lymph node metastasis-related predictive signature. (A) Correlation analysis between immune cells and the expression of TEKT2 and RPGR. Blue represents negative correlation, and red represents positive correlation. (B) Correlation analysis between checkpoint genes and the expression of TEKT2 and RPGR. Blue represents negative correlation, and red represents positive correlation. (C) Differential expression analysis of HLA family genes in high- and low-risk groups. The asterisks represented the statistical p value (*P < 0.05; **P < 0.01).
References
1. Sung H, Ferlay J, Siegel RL, Laversanne M, Soerjomataram I, Jemal A, et al. Global cancer statistics 2020: GLOBOCAN estimates of incidence and mortality worldwide for 36 cancers in 185 countries. CA Cancer J Clin (2021) 71(3):209–49. doi: 10.3322/caac.21660
2. Pecorino B, D'Agate MG, Scibilia G, Scollo P, Giannini A, Di Donna MC, et al. Evaluation of surgical outcomes of abdominal radical hysterectomy and total laparoscopic radical hysterectomy for cervical cancer: a retrospective analysis of data collected before the LACC trial. Int J Environ Res Public Health (2022) 19(20):13176. doi: 10.3390/ijerph192013176
3. Adiga D, Eswaran S, Pandey D, Sharan K, Kabekkodu SP. Molecular landscape of recurrent cervical cancer. Crit Rev Oncol Hematol (2021) 157:103178. doi: 10.1016/j.critrevonc.2020.103178
4. Delgado G, Bundy BN, Fowler WC Jr, Stehman FB, Sevin B, Creasman WT, et al. A prospective surgical pathological study of stage I squamous carcinoma of the cervix: a gynecologic oncology group study. Gynecol Oncol (1989) 35(3):314–20. doi: 10.1016/0090-8258(89)90070-x
5. Bhatla N, Berek JS, Cuello Fredes M, Denny LA, Grenman S, Karunaratne K, et al. Revised FIGO staging for carcinoma of the cervix uteri. Int J Gynaecol Obstet (2019) 145(1):129–35. doi: 10.1002/ijgo.12749
6. Li C, Hua K. Dissecting the single-cell transcriptome network of immune environment underlying cervical premalignant lesion, cervical cancer and metastatic lymph nodes. Front Immunol (2022) 13:897366. doi: 10.3389/fimmu.2022.897366
7. Cancer Genome Atlas Research Network. Integrated genomic and molecular characterization of cervical cancer. Nature (2017) 543(7645):378–84. doi: 10.1038/nature21386
8. Liberzon A, Birger C, Thorvaldsdottir H, Ghandi M, Mesirov JP, Tamayo P. The molecular signatures database (MSigDB) hallmark gene set collection. Cell Syst (2015) 1(6):417–25. doi: 10.1016/j.cels.2015.12.004
9. Ikeda N, Nakajima Y, Tokuhara T, Hattori N, Sho M, Kanehiro H, et al. Clinical significance of aminopeptidase N/CD13 expression in human pancreatic carcinoma. Clin Cancer Res (2003) 9(4):1503–8.
10. Newman AM, Liu CL, Green MR, Gentles AJ, Feng W, Xu Y, et al. Robust enumeration of cell subsets from tissue expression profiles. Nat Methods (2015) 12(5):453–7. doi: 10.1038/nmeth.3337
11. Bindea G, Mlecnik B, Tosolini M, Kirilovsky A, Waldner M, Obenauf AC, et al. Spatiotemporal dynamics of intratumoral immune cells reveal the immune landscape in human cancer. Immunity (2013) 39(4):782–95. doi: 10.1016/j.immuni.2013.10.003
12. Yang W, Soares J, Greninger P, Edelman EJ, Lightfoot H, Forbes S, et al. Genomics of drug sensitivity in cancer (GDSC): a resource for therapeutic biomarker discovery in cancer cells. Nucleic Acids Res (2013) 41(Database issue):D955–61. doi: 10.1093/nar/gks1111
13. LaVigne AW, Triedman SA, Randall TC, Trimble EL, Viswanathan AN. Cervical cancer in low and middle income countries: addressing barriers to radiotherapy delivery. Gynecol Oncol Rep (2017) 22:16–20. doi: 10.1016/j.gore.2017.08.004
14. Walboomers JM, Jacobs MV, Manos MM, Bosch FX, Kummer JA, Shah KV, et al. Human papillomavirus is a necessary cause of invasive cervical cancer worldwide. J Pathol (1999) 189(1):12–9. doi: 10.1002/(SICI)1096-9896(199909)189:1<12::AID-PATH431>3.0.CO;2-F
15. Burd EM. Human papillomavirus and cervical cancer. Clin Microbiol Rev (2003) 16(1):1–17. doi: 10.1128/CMR.16.1.1-17.2003
16. Cohen PA, Jhingran A, Oaknin A, Denny L. Cervical cancer. Lancet (2019) 393(10167):169–82. doi: 10.1016/S0140-6736(18)32470-X
17. Gennigens C, Jerusalem G, Lapaille L, De Cuypere M, Streel S, Kridelka F, et al. Recurrent or primary metastatic cervical cancer: current and future treatments. ESMO Open (2022) 7(5):100579. doi: 10.1016/j.esmoop.2022.100579
18. Ojesina AI, Lichtenstein L, Freeman SS, Pedamallu CS, Imaz-Rosshandler I, Pugh TJ, et al. Landscape of genomic alterations in cervical carcinomas. Nature (2014) 506(7488):371–5. doi: 10.1038/nature12881
19. Wright JD, Matsuo K, Huang Y, Tergas AI, Hou JY, Khoury-Collado F, et al. Prognostic performance of the 2018 international federation of gynecology and obstetrics cervical cancer staging guidelines. Obstet Gynecol (2019) 134(1):49–57. doi: 10.1097/AOG.0000000000003311
20. Karaman S, Detmar M. Mechanisms of lymphatic metastasis. J Clin Invest (2014) 124(3):922–8. doi: 10.1172/JCI71606
21. Karlsson MC, Gonzalez SF, Welin J, Fuxe J. Epithelial-mesenchymal transition in cancer metastasis through the lymphatic system. Mol Oncol (2017) 11(7):781–91. doi: 10.1002/1878-0261.12092
22. Setter PW, Malvey-Dorn E, Steffen W, Stephens RE, Linck RW. Tektin interactions and a model for molecular functions. Exp Cell Res (2006) 312(15):2880–96. doi: 10.1016/j.yexcr.2006.05.014
23. Cao H, Wan Z, Wang F, Liu Z, Li X, Hou J. Downregulation of KIF2C and TEKT2 is associated with male infertility and testicular carcinoma. Aging (Albany NY) (2021) 13(19):22898–911. doi: 10.18632/aging.203583
24. Yang P, Ruan Y, Yan Z, Gao Y, Yang H, Wang S. Comprehensive analysis of lymph nodes metastasis associated genes in cervical cancer and its significance in treatment and prognosis. BMC Cancer (2021) 21(1):1230. doi: 10.1186/s12885-021-08945-8
25. Patil H, Tserentsoodol N, Saha A, Hao Y, Webb M, Ferreira PA. Selective loss of RPGRIP1-dependent ciliary targeting of NPHP4, RPGR and SDCCAG8 underlies the degeneration of photoreceptor neurons. Cell Death Dis (2012) 3:e355. doi: 10.1038/cddis.2012.96
26. Pongor L, Kormos M, Hatzis C, Pusztai L, Szabó A, Győrffy B. A genome-wide approach to link genotype to clinical outcome by utilizing next generation sequencing and gene chip data of 6,697 breast cancer patients. Genome Med (2015) 7:104. doi: 10.1186/s13073-015-0228-1
27. Lin X, Wang S, Lin K, Zong J, Zheng Q, Su Y, et al. Competitive endogenous RNA landscape in Epstein-Barr virus associated nasopharyngeal carcinoma. Front Cell Dev Biol (2021) 9:782473. doi: 10.3389/fcell.2021.782473
28. Jia R, Sui Z, Zhang H, Yu Z. Identification and validation of immune-related gene signature for predicting lymph node metastasis and prognosis in lung adenocarcinoma. Front Mol Biosci (2021) 8:679031. doi: 10.3389/fmolb.2021.679031
29. Luo C, Huang B, Wu Y, Xu Y, Ou W, Chen J, et al. Identification of lymph node metastasis-related key genes and prognostic risk model in bladder cancer by Co-expression analysis. Front Mol Biosci (2021) 8:633299. doi: 10.3389/fmolb.2021.633299
30. Wu L, Zhou Y, Guan Y, Xiao R, Cai J, Chen W, et al. Seven genes associated with lymphatic metastasis in thyroid cancer that is linked to tumor immune cell infiltration. Front Oncol (2021) 11:756246. doi: 10.3389/fonc.2021.756246
31. Jardim DL, Goodman A, de Melo Gagliato D, Kurzrock R. The challenges of tumor mutational burden as an immunotherapy biomarker. Cancer Cell (2021) 39(2):154–73. doi: 10.1016/j.ccell.2020.10.001
32. Garnier L, Gkountidi AO, Hugues S. Tumor-associated lymphatic vessel features and immunomodulatory functions. Front Immunol (2019) 10:720. doi: 10.3389/fimmu.2019.00720
33. Chen DS, Mellman I. Elements of cancer immunity and the cancer-immune set point. Nature (2017) 541(7637):321–30. doi: 10.1038/nature21349
34. Wendel Naumann R, Leath CA 3rd. Advances in immunotherapy for cervical cancer. Curr Opin Oncol (2020) 32(5):481–7. doi: 10.1097/CCO.0000000000000663
35. Chung HC, Ros W, Delord JP, Perets R, Italiano A, Shapira-Frommer R, et al. Efficacy and safety of pembrolizumab in previously treated advanced cervical cancer: results from the phase II KEYNOTE-158 study. J Clin Oncol (2019) 37(17):1470–8. doi: 10.1200/JCO.18.01265
36. Marquina G, Manzano A, Casado A. Targeted agents in cervical cancer: beyond bevacizumab. Curr Oncol Rep (2018) 20(5):40. doi: 10.1007/s11912-018-0680-3
37. Vallejo CT, Machiavelli MR, Perez JE, Romero AO, Bologna F, Vicente H, et al. Docetaxel as neoadjuvant chemotherapy in patients with advanced cervical carcinoma [J]. Am J Clin Oncol (2003) 26(5):477–82. doi: 10.1097/01.coc.0000037109.12434.52
38. Seol HJ, Ulak R, Ki KD, Lee JM. Cytotoxic and targeted systemic therapy in advanced and recurrent cervical cancer: experience from clinical trials. Tohoku J Exp Med (2014) 232(4):269–76. doi: 10.1620/tjem.232.269
39. Blair HA. Daunorubicin/Cytarabine liposome: a review in acute myeloid leukaemia. Drugs (2018) 78(18):1903–10. doi: 10.1007/s40265-018-1022-3
40. Yadav P, Yadav R, Jain S, Vaidya A. Caspase-3: a primary target for natural and synthetic compounds for cancer therapy. Chem Biol Drug Des (2021) 98(1):144–65. doi: 10.1111/cbdd.13860
Keywords: cervical cancer, lymph node metastasis, prognosis, immune infiltration, immunotherapy
Citation: Guo Y, Wang L, Xu Z, Li M, Wang W, Bai Y, Xu X, Li R and Zhao H (2023) Lymph node metastasis-related gene signature shows good performance in predicting prognosis and immune infiltration in cervical cancer. Front. Oncol. 13:1190251. doi: 10.3389/fonc.2023.1190251
Received: 20 March 2023; Accepted: 06 June 2023;
Published: 22 June 2023.
Edited by:
Paolo Scollo, Kore University of Enna, ItalyReviewed by:
Huaimin Liu, Department of Integrated Traditional Chinese and Western Medicine, Henan Cancer Hospital (Affiliated Cancer Hospital of Zhengzhou University), ChinaBasilio Pecorino, Cannizzaro Hospital, Italy
Giuseppe Scibilia, “Giovanni Paolo II” Hospital, Italy
Copyright © 2023 Guo, Wang, Xu, Li, Wang, Bai, Xu, Li and Zhao. This is an open-access article distributed under the terms of the Creative Commons Attribution License (CC BY). The use, distribution or reproduction in other forums is permitted, provided the original author(s) and the copyright owner(s) are credited and that the original publication in this journal is cited, in accordance with accepted academic practice. No use, distribution or reproduction is permitted which does not comply with these terms.
*Correspondence: Zhen Xu, eHV6aGVuNDEzQDEyNi5jb20=; Hu Zhao, enhqMTIwNEAxNjMuY29t