- 1Department of Graduate, Shandong First Medical University and Shandong Academy of Medical Sciences, Jinan, Shandong, China
- 2Department of Nuclear Medicine, Shandong Cancer Hospital and Institute, Shandong First Medical University and Shandong Academy of Medical Sciences, Jinan, Shandong, China
- 3Department of Radiation Oncology, Shandong Cancer Hospital and Institute, Shandong First Medical University and Shandong Academy of Medical Sciences, Jinan, Shandong, China
Objective: To explore a prediction model for lymphovascular invasion (LVI) on cT1–2N0M0 radiologic solid non-small cell lung cancer (NSCLC) based on a 2-deoxy-2[18F]fluoro-D-glucose ([18F]F-FDG) positron emission tomography-computed tomography (PET-CT) radiomics analysis.
Methods: The present work retrospectively included 148 patients receiving surgical resection and verified pathologically with cT1–2N0M0 radiologic solid NSCLC. The cases were randomized into training or validation sets in the ratio of 7:3. PET and CT images were used to select optimal radiomics features. Three radiomics predictive models incorporating CT, PET, as well as PET/CT images radiomics features (CT-RS, PET-RS, PET/CT-RS) were developed using logistic analyses. Furthermore, model performance was evaluated by ROC analysis for predicting LVI status. Model performance was evaluated in terms of discrimination, calibration along with clinical utility. Kaplan-Meier curves were employed to analyze the outcome of LVI.
Results: The ROC analysis demonstrated that PET/CT-RS (AUCs were 0.773 and 0.774 for training and validation sets) outperformed both CT-RS(AUCs, 0.727 and 0.752) and PET-RS(AUCs, 0.715 and 0.733). A PET/CT radiology nomogram (PET/CT-model) was developed to estimate LVI; the model demonstrated conspicuous prediction performance for training (C-index, 0.766; 95%CI, 0.728–0.805) and validation sets (C-index, 0.774; 95%CI, 0.702–0.846). Besides, decision curve analysis and calibration curve showed that PET/CT-model provided clinically beneficial effects. Disease-free survival and overall survival varied significantly between LVI and non-LVI cases (P<0.001).
Conclusions: The PET/CT radiomics models could effectively predict LVI on early stage radiologic solid lung cancer and provide support for clinical treatment decisions.
Introduction
Lung cancer contributes significantly to cancer-associated fatalities worldwide, which caused 18% of total cancer-associated death cases in 2020 (1). Worldwide, non-small cell lung cancer (NSCLC) accounts for 85% of lung cancers (2). As medical treatment develops and the awareness about early diagnosis and treatment of lung disease increases, more cases are detected at an early stage. In patients with early-stage NSCLC, radical surgery and stereotactic ablative body radiotherapy (SABR) remains the key treatment approaches (3–5). Despite providing radical surgical treatment, the relapse rate of early NSCLC is approximately 30–40% (6), with distant metastasis (DM) and regional nodal relapse being the major factors behind treatment failure(65% and 20%, respectively) (7, 8).
Lymphovascular invasion (LVI) refers to cancer cell occurrence in the endothelium-lined lumen or cancer cells-mediated lymphovascular wall destruction. LVI has been reported to occur only in partially solid nodes with a predominantly solid imaging presentation and solid nodes with a solid component of >10 mm (9). In an early-stage NSCLC with a solid imaging presentation, the 5-year recurrence rate increases among cancer cases showing pathological LVI (21.7%) compared with those with no pathological LVI (7.4%), considered a high-risk pathologic feature remarkably increasing relapse and lymph node metastasis incidence (10–13). Patients at high risk for LVI in early-stage NSCLC may benefit from more advanced therapeutic approaches, patients undergoing surgery will require lymph node dissection, which will influence the choice of surgical procedure, while non-surgical patients treated with SABR may require more aggressive adjuvant therapy. Nonetheless, pathology after surgery remains the only tool to confirm LVI, and there is no other validated noninvasive method to predict LVI status preoperatively.
2-deoxy-2[18F]fluoro-D-glucose ([18F]F-FDG) positron emission tomography-computed tomography (PET-CT) has an important effect on lung cancer stage classification before surgery, relapse, and the assessment of treatment response. Several studies have documented that the fludeoxyglucose (FDG) uptake rate of primary NSCLC lesions correlates with tumor aggressiveness and that some metabolic parameters such as metabolic tumor volume (MTV) and maximum standardized uptake values (SUVmax) are important prognostic factors for lung cancer cases receiving surgical resection. Li C et al. (14) reported that MTV before surgery independently predicted LVI within NSCLC. Noda Y et al. (15)demonstrated that SUVmax of lung cancer could be employed for the identification of LVI. Satoshi et al. (16) reported that LVI and SUVmax were significantly associated with the recurrence of lung cancer. However, further research is warranted to evaluate whether 18F-FDG PET/CT could be used to predict LVI before surgery.
Radiomics, a method for high-throughput extraction of features based on medical images, is a useful predictive marker for identifying tumor heterogeneity and other features of the microenvironment invisible to the naked eye (17, 18). Radiomics has proven successful in tumor detection (19), histological and mutational precession (20), prognosis prediction (21), and treatment outcome assessment in lung cancer (22). Nie P et al. (23) constructed a PET/CT-based radiomics nomogram in predicting LVI among 272 lung adenocarcinoma (LAC) cases of stages I-IV, and the results demonstrated the favorable predictive efficacy, however, the group of patients with early-stage solid NSCLC in this study was relatively small, and there is no predictive model for LVI in the solid NSCLC patient population.
Therefore, this study focused on investigating whether PET/CT radiomics nomogram could be applied to predict LVI and its association with patterns of recurrence in cT1–2N0M0 radiologic solid NSCLC patients.
Materials and methods
Patients
The Institutional Review Board of our institution approved this retrospective study, which waived the requirement for signed informed consent forms. We retrospectively collected 272 lung cancer cases who underwent PET/CT examination at the PET/CT unit in the Affiliated Cancer Hospital of Shandong First Medical University, China, between April 2014 and September 2021; of all the screened cases, 148 fulfilled the criteria. The inclusion criteria were: 1. patients with pathologically confirmed cT1–2N0M0 lung cancer; 2. patients who underwent PET/CT scan before surgery; 3. patients in whom PET/CT showed positive FDG uptake at the primary tumor site. Exclusion criteria were: 1. CT in patients demonstrated lesions showing ground glass components; 2. patients had received preoperative antitumor therapy; 3. Patients did not undergo surgery at our institution earlier;4. patients with other malignancies; 5. patients with other lung diseases that could affect image analysis. Figure 1 illustrates the screening process of patients who were stratified into training and validation groups (7:3 ratio). Training set and validation set samples were applied in predicting the training model and in evaluating model performance, respectively.
All cases were retrieved to collect participants’ baseline clinical information, including their age, gender, smoking history, clinical T-stage, AJCC cancer staging system-based TNM stage, primary foci SUVmax, SUVmean, MTV, total lesion glycolysis (TLG), and tumor location by deriving PET/CT image information. An expert pathologist with ten years of clinical experience interpreted the pathology, including pathological type, pleural invasion, and LVI statuses of the patients.
PET/CT image acquisition
All patients underwent 6-h fasting before obtaining 18F-FDG PET/CT images. Blood glucose (BG) contents of the patients were <11.0 mmol/L measured prior to the scan. All patients underwent scanning using Gemini TF Big Bore PET/CT system (Philips Medical Systems); the PET tracer was produced using the MINI Trace (GE Tracer Lab, USA), which produces its own labeled nuclide 18F-FDG was automatically synthesized with a purity of ≥5%. FDG (3.7–5.5 MBq/kg) was injected intravenously in the patients who rested calmly for 60 min; the bladder was emptied before image acquisition, and the spiral CT scan was provided 120 kV, 50 mA tube current, as well as 4 mm layer thickness, and then PET scan with 4 mm layer thickness, corrected for CT attenuation and ordered subsets. Through adopting ordered subsets maximization and CT attenuation correction, patient reconstruction was implemented. Besides, all patients underwent end-inspiratory breath-hold spiral chest CT scans (layer thickness, 5 mm).
Image analysis
Two Nuclear Medicine physicians who were blind to pathological or clinical data analyzed the PET/CT images and consistently analyzed the following features: “According to the thin-layer CT presentation, the solid component is the part of the nodule without identifiable vascular and bronchial structures.” The current study deemed a “solid” tumor to be the maximal solid tumor diameter to maximal tumor diameter ratio (called consolidation-to-tumor ratio, CTR >0.5 (24, 25). SUVmax to be the maximum value on the highest counted pixel within one region of interest (ROI) containing the whole tumor by plotting it onto an axial PET image. MTV was extracted by the target area outlining software 3D-Slicer through an iterative adaptive algorithm to compute the threshold value for outlining the tumor edge. TLG = SUVmean*MTV (cm3).
Tumor segmentation and radiomics feature extraction
Figure 2 shows the radiomics collection flowchart. Digital Imaging and Communications (DICOM) standard format images of the patient’s 18F-FDG PET/CT were imported into the 3D-Slicer software tool (version 4.13.0, 1.0, www.slicer.org) to segment the tumor. Thereafter, the tumor lesions were outlined on the axial PET as well as CT images, and the CT images were manually outlined layer-wise by the radiologist. Initially, the PET images were automatically outlined by a fixed threshold of SUVmax>2.5 and subsequently manually corrected by the radiologist. In total, 1702 radiomics features (851 for CT and 851 for PET) were obtained from the volume-of-interest (VOI), including 162 first-order features, 14 shape features, 264 gray-level co-occurrence matrix (GLCM) features, 144 gray-level size region matrix (GLSZM) features, 144 gray-level run length matrix (GLRLM) features, 45 neighborhood gray level difference matrix (NGTDM) features, and 126 gray level dependence matrix (GLDM) features.
The intra- and inter-class correlation coefficients (ICCs) were used to evaluate feature collection reproducibility in and between the two readers. Readers 1 and 2 randomly selected 20 (10 with LVI, 10 without LVI) PET and CT images in the entire set. Reader 1 repeated this segmentation process after two weeks. The ICC >0.80 indicates high consistency in feature collection. Reader 1 was responsible for segmenting the ROI of all the remaining patients.
Feature selection and radiomics signature establishment
To avoid overfitting, the features were downscaled according to the following three steps before feature construction. First, radiomics features whose intra- and inter-reader ICCs >0.80 were retained to avoid any subjective heterogeneity of ROI segmentation. Second, a one-way analysis of variance (ANOVA) was performed to select features of p<0.05 between the patients with LVI and without LVI. Finally, optimal radiomics features were selected using the least absolute shrinkage and selection operator algorithm (LASSO) to construct a radiomics signature (RS). Thereafter, patients’ Rad-scores were determined.
Predictive model development and validation
The current study developed the CT-RS, PET-RS, and PET/CT-RS machine learning models to predict LVI in cT1–2N0M0 lung cancer. Values of sensitivity, specificity, and area under the ROC curve (AUC) were determined to assess their diagnostic accuracy. Then, an optimal model for generating a radiomics nomogram was selected. Model clinical utility was studied using decision curve analysis (DCA) as well as calibration curve analysis.
Follow-up and survival
CT examination was conducted during the follow-up period at 6–12-month intervals within the initial 2 years after surgery but at 1-year intervals within 5 years. Our study endpoints included overall survival (OS) and Disease-free survival (DFS); OS was deemed as the duration between surgery and death, and DFS as the duration between relapse and disease progression. Kaplan-Meier approach was used for plotting survival curves.
Statistical analyses
IBM SPSS version 26.0 (https://www.ibm.com) was employed to select key statistical variables through univariate (Mann-Whitney U test and chi-square test) as well as multivariate regression. R software (version 4.1.3, https://www.r-project.org) was adopted for ICC, DCA, calibration plots, and survival analysis. The MedCalc statistical software (version 19.0.7, https://www.medcalc.org) was used to analyze the ROC curve. A p-value of <0.05 (two-sided) indicated statistical significance.
Results
Patient characteristics
In this study, a total of 148 patients (94 M and 54 F, aged 31–85 (median, 64) years) were recruited, including 69(47%) patients with pathological LVI and 79 (53%) patients without pathological LVI. Table 1 shows clinical factors, pathological information, and PET metabolic factors. Patients with LVI had higher values of SUVmax, SUVmean, MTV, and TLG than those without LVI. Gender and tumor location were found statistically significant (P<0.05). The distribution of age, smoking history, type of pathology, pleural invasion, and AJCC stage showed similarity in both groups (P>0.05), but there was no statistically significant difference (P>0.05) upon multivariate regression (Table 2).
Construction and validation of radiomics model
From the 1702 radiomics features collected in 3D ROI on PET and CT images, those with ICC value <0.80 (numbering 457) were eliminated, and 551 PET and 694 CT features were retained. Overall, 40 PET and 238 CT features were found to be insignificant in LVI compared with non-LVI patients (P = 0.00–0.05) and were incorporated in LASSO analysis. Finally, we chose 5 CT and 3 PET features to construct CT-RS, PET-RS, and PET/CT-RS, respectively, for predicting LVI status within cT1–2N0M0 lung cancer. The Supplementary Table demonstrates radiomics features as well as the associated Rad-score formulas and coefficients.
Differences in PET/CT-RS features between the LVI and non-LVI patients were found statistically significant (P<0.05) for both sets. The maximum AUC value was attained by PET/CT-RS (AUC values for training and validation, 0.773, 0.774, respectively), and both datasets achieved remarkable sensitivity and specificity (sensitivity = 0.774, 0.538; specificity = 0.667, 0.944, training and validation, respectively). Figure 3 depicts the ROC curves. CT-RS and PET-RS had AUC values of 0.727 and 0.715, respectively, for the training set, whereas values of 0.752 and 0.733, respectively, for the validation set. Sensitivities and specificities achieved by the CT-RS model were 0.774 and 0.608, respectively, while those by the PET-RS model were 0.642 and 0.652, respectively (Table 3).
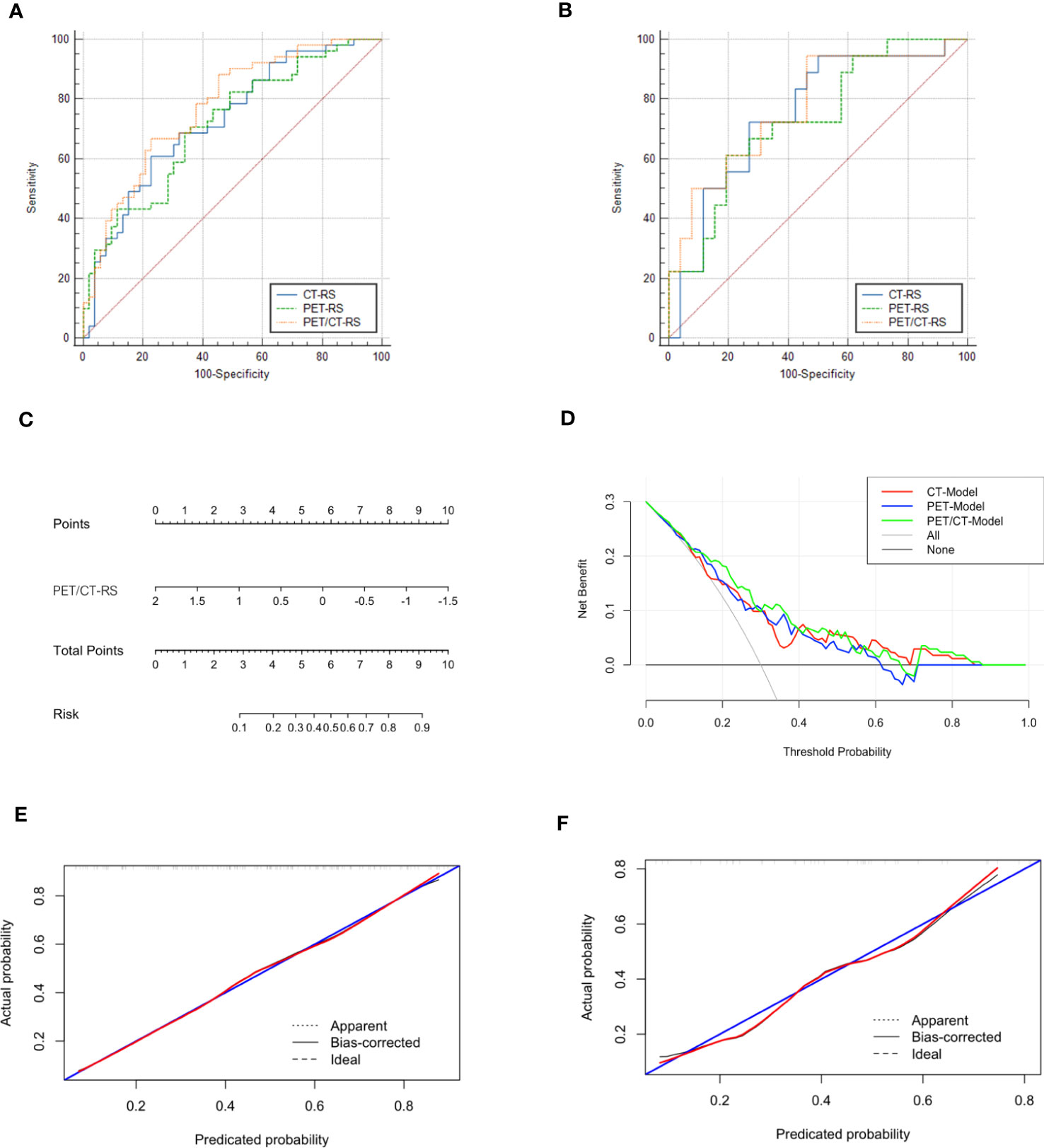
Figure 3 (A) ROCs of different radiomics models in the training set, The three colors represent the three models, blue: CT-RS model, green: PET-RS model, yellow: PET/CT-RS model; (B) ROCs of different radiomics models in the validation set; (C) The radiomics nomogram: PET/CT RadScore-Model (PET/CT-Model); (D) Decision curve analysis for CT-Model, PET-Model, and PET/CT-Model; (E) Calibration curve of the PET/CT Model in the training set; (F) Calibration curve of the PET/CT Model in the validation set.
PET/CT-RS showed the best efficacy in predicting LVI, and the C-index of radiomics nomogram (PET/CT-Model) was developed based on PET/CT-RS with 0.766 (95% CI: 0.728–0.805, training set) and 0.774 (95% CI: 0.702–0.846, validation set). Figure 3 depicts the diagnostic performance, calibration curves, and decision curves of the model. The calibration curve revealed that the estimated risks conformed to the observed LVI outcomes. Besides, the diagonal curved line approximated the ideal straight line, suggesting the accuracy of our PET/CT-Model-based nomogram in prognosis prediction. Furthermore, DCA revealed the benefits of the PET/CT model in predicting LVI.
Survival outcomes
By April 10, 2022, we had successfully followed up on 148 patients (median, 34.33 months; 95% CI, 27.88–40.79). The overall recurrence rate was 22% (33/148, including 41% (28/69) of patients with LVI and 6% (5/79) of patients without LVI), and DM occurred in 16% of the patients (24/148, including 28% (19/69) of patients with LVI and 6% (5/79) without LVI); median DFS for overall patients was 73.67 (range 1–85) and 44.13 (95% CI, 37.57–77.60) months for patients with LVI. The overall mortality rate was 7% (10/148), and all patients had LVI status. Figure 4 shows the relapse rate, mortality, and survival curves.
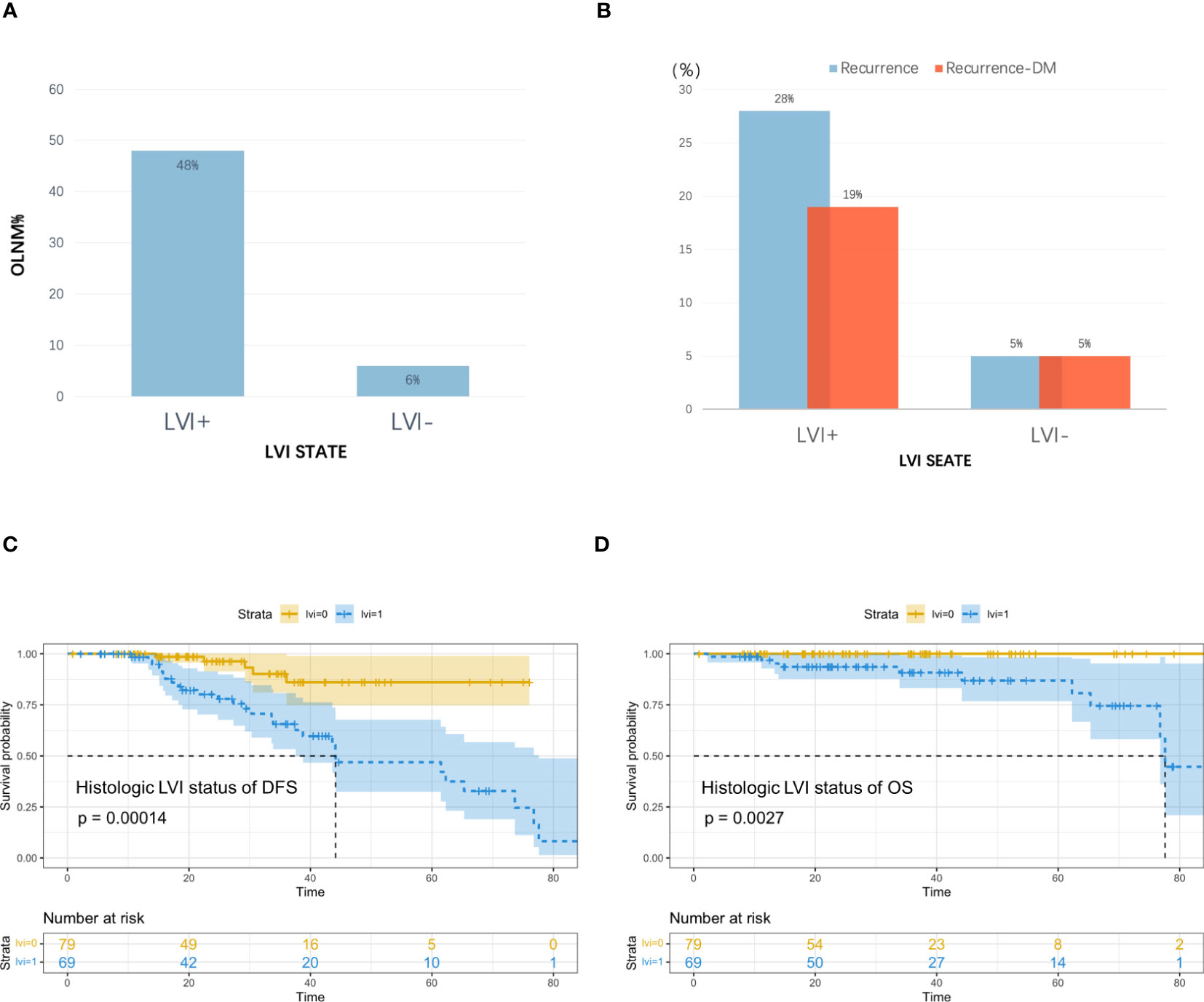
Figure 4 (A) Incidence of occult lymph node metastasis (OLNM), (B) Recurrence rate and distant metastasis rate in patients with and without pathological LVI; (C) Disease Free Survival (DFS) curves according to pathologic LVI status, (D) Overall Survival (OS) curves according to pathologic LVI status.
Analyses of the recurrence pattern of 148 cT1–2N0M0 lung cancer patients treated with radical surgery showed that 26% of cases had occult lymph node metastases (OLNM), including 6% (5/79) of cases without LVI and 48% (33/69) with LVI, and the incidence of OLNM among LVI cases showed marked increase and they were at greater risk of lymph node metastases (LNM). The overall cohort had a recurrence rate of 22% after radical surgery, with DM being the most common cancer type. The recurrence rate increased significantly among patients with LVI compared with those without LVI; therefore, because of the worse prognosis for survival, more aggressive adjuvant therapy may be needed for this group of high-risk patients with LVI to ensure radical treatment, improve tumor control rates, and improve patient recurrence-free survival and overall survival.
Discussion
The current study focused on investigating the significance of PET/CT radiomics in predicting LVI status and prognosis before surgery in cases developing early radiologic solid lung cancer. It was found that PET/CT radiomics nomogram achieved good LVI predictive ability among cases developing cT1–2N0M0 radiologic solid lung cancer. Besides, the incidence of OLNM and postoperative recurrence rates were found to be remarkably higher in patients with LVI, suggesting a greater risk of LNM as well as a worse prognosis. OS and DFS differed significantly between cases with LVI and without LVI, highlighting the importance of LVI as an aid to clinical treatment decisions in patients with radiologic solid lung cancer at an early stage.
18F-FDG PET/CT plays an important role in evaluating the clinical staging as well as the prognosis of lung cancer. In our study, we observed that SUVmax and SUVmean, MTV, and TLG were statistically different in patients with LVI and without LVI (P<0.05). Partially corroborating the earlier findings by C Li et al., C Li et al. (14) examined PET/CT image parameters along with clinical features in 161 cases with NSCLC and documented that tumor MTV independently predicted LVI (p<0.05). In addition, we found that no variable was considered to be an independent risk factor for LVI, probably because the training set had fewer samples. It indicates that conventional features collected on traditional images contributed little to LVI. Therefore, it is suggested to develop further credible and objective biomarkers for identifying LVI status among lung cancer cases.
High-throughput collection for high-level quantitative features can be employed in radiomics to characterize tumor phenotypes objectively and quantitatively; they can be collected in medical studies by adopting advanced mathematical algorithms for imaging data identifying tumor biological and histological features, besides visually assessing CT, MRI, and PET/CT images. The diagnostic efficacy of radiomics has previously been demonstrated in preoperatively predicting LVI in different cancers. Zhang Y et al. (26) constructed the multimodal radiomics model (MR/CT) to predict LVI in 94 rectal cancer cases; this model was validated based on the training set (AUC, 0.884; 95% CI 0.803- 0.964) as well as the validation set (AUC, 0.876; 95% CI 0.721–1.000) and demonstrated remarkable predictive power. In a radiomics study on predicting LVI status before surgery in gastric cancer patients, Chen et al. (27) proposed that a combined model based on radiomics features of enhanced CT combined with clinical factors could effectively predict LVI status before surgery in gastric cancer patients (AUC, 0.856) of training cohort; Yang et al. (28) stated that AUCs of PET/CT-based radiomics models reached 0.881 and 0.854 (training and validation groups). In constructing a radiomics model for 272 patients with LAD, Nie P et al. (23) documented that AUC was not significantly different in CT compared with PET radiomics models, and the PET/CT-based radiomics model achieved an increased net benefit compared with PET and CT models. In partial agreement with the earlier findings of Nie P et al., we observed that the AUC of PET/CT-RS was better than that of both CT-RS and PET-RS. Our constructed PET/CT model proved feasible in predicting LVI. However, at the same time, our results found that the sensitivity of PET/CT models in the validation group was low, which was related to the significant improvement of model specificity in the validation group, and the improvement of model specificity was accompanied by the reduction of sensitivity. Further improvement of model performance may reduce the gap between the two. The reason for this result may due to the relatively small amount of data in the validation set and the bias of some missing values when performing model validation verification, but the overall performance of the PET/CT model is still optimal. In the follow-up study, we will try to increase the sample size as much as possible.
Currently, LVI is not included in the AJCC guidelines to independently predict prognosis in the TNM classification system for lung cancer. However, LVI has been identified as a factor predicting the prognosis of surgically treated patients with lung cancer, which is associated with tumor recurrence (16). In the present study, the OLNM rate significantly increased among LVI cases, the DFS and OS of patients with LVI were significantly worse than that of patients without LVI. A potential explanation for the results may be as follows: patients with LVI showed more aggressive disease than those without LVI. Presently, for patients with early-stage lung cancer without obvious contraindications to surgery, surgery is clinically recommended as the preferred treatment intervention. For patients who are older, have poor lung function and cardiac insufficiency, and cannot tolerate surgical operations, other treatment modalities, such as radical radiotherapy, can be employed. Previous studies have shown that suitable radical radiotherapy can achieve results similar to those in surgery (29). In patients with early lung cancer who cannot tolerate surgery, precise pathological lymph node staging is not available, which may underestimate the risk of recurrence and delay treatment of the disease. PET/CT radiomics may offer good predictive value for LVI in patients with early-stage inoperable radiologic solid lung cancer.
However, this study has a few limitations. First, the included cases were limited in number, more samples are needed to further validate our results and assumptions and analyze model stability. Second, Multi-center research perhaps provide more data to build more representative models, so the inclusion of external validation would have been better. Given that radiomics involves extracting information from images when conducting multi-center research, we need to review the machine parameters and operating procedures of the collected images to exclude errors caused by human or machine differences. Finally, because CT tumor segmentation is done manually, the development of a more efficient tumor segmentation method remains a key consideration.
Conclusions
In the current work, radiomics features extracted from PET/CT imaging can be adopted for efficiently predicting LVI status on cT1–2N0M0 radiologic solid lung cancer, which can improve patient diagnosis before surgery and make personalized treatment planning.
Data availability statement
The raw data supporting the conclusions of this article will be made available by the authors, without undue reservation.
Ethics statement
This retrospective study was approved by the Institutional Review Board (IRB) of Shandong Cancer Hospital, which waived the requirement for signed informed consent. Written informed consent for participation was not required for this study in accordance with the national legislation and the institutional requirements.
Author contributions
Among the authors in the list, JW and XS has done a lot of work in research design, data collection, paper writing modification, and paper finalization. JW and JL participated in the data collection. ZZ made some contributions to the data analysis and writing of the article. XS and LX has done a lot of work in research design and paper finalization. The first draft of the manuscript was written by JW and XS. All authors contributed to the article and approved the submitted version.
Funding
This work was supported by grants from the National Natural Science Foundation of China (Grant NO. 82172866), the Shandong Provincial Natural Science Foundation (Grant NO. ZR2021LZL005), the Shandong Provincial Natural Science Foundation (Grant NO. ZR2019LZL019), the Department of Science & Technology of Shandong Province (Grant NO. 2021CXGC011102), and the Start-up fund of Shandong Cancer Hospital (2020PYA04).
Conflict of interest
The authors declare that the research was conducted in the absence of any commercial or financial relationships that could be construed as a potential conflict of interest.
Publisher’s note
All claims expressed in this article are solely those of the authors and do not necessarily represent those of their affiliated organizations, or those of the publisher, the editors and the reviewers. Any product that may be evaluated in this article, or claim that may be made by its manufacturer, is not guaranteed or endorsed by the publisher.
Supplementary material
The Supplementary Material for this article can be found online at: https://www.frontiersin.org/articles/10.3389/fonc.2023.1185808/full#supplementary-material
Abbreviations
NSCLC, non-small cell lung cancer; LVI, lymphovascular invasion; OS, overall survival; DFS, disease-free survival; CT, computed tomography; PET, positron emission tomography; PET-CT, positron emission tomography-computed tomography; SUVmax, maximum standardized uptake value; SUVmean, mean standardized uptake value; TLG, total lesion glycolysis; MTV, metabolic tumor volume; ICC, intraclass correlation coefficient; Rad-score, Radiomics Score; ROI, region of Interest; C-index, Harrell concordance index; DCA curve, decision curve analysis curve; LASSO, least absolute shrinkage and selection operator; ROC, receiver operator characteristic curve; AUC, area under receiver-operating characteristic curve.
References
1. Sung H, Ferlay J, Siegel RL, Laversanne M, Soerjomataram I, Jemal A, et al. Global cancer statistics 2020: GLOBOCAN estimates of incidence and mortality worldwide for 36 cancers in 185 countries. CA Cancer J Clin (2021) 71(3):209–49. doi: 10.3322/caac.21660
2. Bray F, Ferlay J, Soerjomataram I, Siegel RL, Torre LA, Jemal A. Global cancer statistics 2018: GLOBOCAN estimates of incidence and mortality worldwide for 36 cancers in 185 countries. CA Cancer J Clin (2018) 68(6):394–424. doi: 10.3322/caac.21492
3. Hoy H, Lynch T, Beck M. Surgical treatment of lung cancer. Crit Care Nurs Clin North Am (2019) 31(3):303–13. doi: 10.1016/j.cnc.2019.05.002
4. Nasim F, Sabath BF, Eapen GA. Lung cancer. Med Clin North Am (2019) 103(3):463–73. doi: 10.1016/j.mcna.2018.12.006
5. Deng HY, Peng L. Surgery or stereotactic body radiotherapy for early-stage lung cancer: what is the current evidence? Clin Lung Cancer (2020) 21(1):e33–e4. doi: 10.1016/j.cllc.2019.10.011
6. Slim A, Kamoun H, Hadidene Y, Smadhi H, Meddeb A, Megdiche ML. Postoperative recurrence of primary lung cancer: anatomo-clinical and therapeutic study. Tunis Med (2021) 99(5):560–8.
7. Ekeke CN, Mitchell C, Schuchert M, Dhupar R, Luketich JD, Okusanya OT. Early distant recurrence in patients with resected stage I lung cancer: a case series of “Blast metastasis”. Clin Lung Cancer (2021) 22(1):e132–e5. doi: 10.1016/j.cllc.2020.09.002
8. Gwóźdź P, Pasieka-Lis M, Kołodziej K, Pankowski J, Banaś R, Wiłkojć M, et al. Prognosis of patients with stages I and II non-small cell lung cancer with nodal micrometastases. Ann Thorac Surg (2018) 105(5):1551–7. doi: 10.1016/j.athoracsur.2017.12.006
9. Choe J, Kim MY, Yun JK, Lee GD, Kim YH, Choi S, et al. Sublobar resection in stage IA non-small cell lung cancer: role of preoperative CT features in predicting pathologic lymphovascular invasion and postoperative recurrence. AJR Am J Roentgenol (2021) 217(4):871–81. doi: 10.2214/AJR.21.25618
10. Wang S, Zhang B, Qian J, Qiao R, Xu J, Zhang L, et al. Proposal on incorporating lymphovascular invasion as a T-descriptor for stage I lung cancer. Lung Cancer (2018) 125:245–52. doi: 10.1016/j.lungcan.2018.09.024
11. Sung SY, Kwak YK, Lee SW, Jo IY, Park JK, Kim KS, et al. Lymphovascular invasion increases the risk of nodal and distant recurrence in node-negative stage I-IIA non-Small-Cell lung cancer. Oncology (2018) 95(3):156–62. doi: 10.1159/000488859
12. Ramnefjell M, Aamelfot C, Aziz S, Helgeland L, Akslen LA. Microvascular proliferation is associated with aggressive tumour features and reduced survival in lung adenocarcinoma. J Pathol Clin Res (2017) 3(4):249–57. doi: 10.1002/cjp2.78
13. Mollberg NM, Bennette C, Howell E, Backhus L, Devine B, Ferguson MK. Lymphovascular invasion as a prognostic indicator in stage I non-small cell lung cancer: a systematic review and meta-analysis. Ann Thorac Surg (2014) 97(3):965–71. doi: 10.1016/j.athoracsur.2013.11.002
14. Li C, Tian Y, Shen Y, Wen B, He Y. Utility of volumetric metabolic parameters on preoperative FDG PET/CT for predicting tumor lymphovascular invasion in non-small cell lung cancer. AJR Am J Roentgenol (2021) 217(6):1433–43. doi: 10.2214/AJR.21.25814
15. Noda Y, Goshima S, Kanematsu M, Watanabe H, Kawada H, Kawai N, et al. F-18 FDG uptake on positron emission tomography as a predictor for lymphovascular invasion in patients with lung adenocarcinoma. Ann Nucl Med (2016) 30(1):11–7. doi: 10.1007/s12149-015-1023-1
16. Shiono S, Abiko M, Sato T. Positron emission tomography/computed tomography and lymphovascular invasion predict recurrence in stage I lung cancers. J Thorac Oncol (2011) 6(1):43–7. doi: 10.1097/JTO.0b013e3181f9abca
17. Miao H, Shaolei L, Nan L, Yumei L, Shanyuan Z, Fangliang L, et al. Occult mediastinal lymph node metastasis in FDG-PET/CT node-negative lung adenocarcinoma patients: risk factors and histopathological study. Thorac Cancer (2019) 10(6):1453–60. doi: 10.1111/1759-7714.13093
18. Gillies RJ, Kinahan PE, Hricak H. Radiomics: images are more than pictures, they are data. Radiology (2016) 278(2):563–77. doi: 10.1148/radiol.2015151169
19. Kirienko M, Cozzi L, Rossi A, Voulaz E, Antunovic L, Fogliata A, et al. Ability of FDG PET and CT radiomics features to differentiate between primary and metastatic lung lesions. Eur J Nucl Med Mol Imag (2018) 45(10):1649–60. doi: 10.1007/s00259-018-3987-2
20. Tu W, Sun G, Fan L, Wang Y, Xia Y, Guan Y, et al. Radiomics signature: a potential and incremental predictor for EGFR mutation status in NSCLC patients, comparison with CT morphology. Lung Cancer (2019) 132:28–35. doi: 10.1016/j.lungcan.2019.03.025
21. Huang Y, Liu Z, He L, Chen X, Pan D, Ma Z, et al. Radiomics signature: a potential biomarker for the prediction of disease-free survival in early-stage (I or II) non-small cell lung cancer. Radiology (2016) 281(3):947–57. doi: 10.1148/radiol.2016152234
22. Xu Y, Hosny A, Zeleznik R, Parmar C, Coroller T, Franco I, et al. Deep learning predicts lung cancer treatment response from serial medical imaging. Clin Cancer Res (2019) 25(11):3266–75. doi: 10.1158/1078-0432.CCR-18-2495
23. Nie P, Yang G, Wang N, Yan L, Miao W, Duan Y, et al. Additional value of metabolic parameters to PET/CT-based radiomics nomogram in predicting lymphovascular invasion and outcome in lung adenocarcinoma. Eur J Nucl Med Mol Imag (2021) 48(1):217–30. doi: 10.1007/s00259-020-04747-5
24. Su H, Dai C, Xie H, Ren Y, She Y, Kadeer X, et al. Risk factors of recurrence in patients with clinical stage IA adenocarcinoma presented as ground-glass nodule. Clin Lung Cancer (2018) 19(5):e609–e17. doi: 10.1016/j.cllc.2018.04.020
25. Du Q, Peng J, Wang X, Ji M, Liao Y, Tang B. Dynamic observation of lung nodules on chest CT before diagnosis of early lung cancer. Front Oncol (2022) 12:713881. doi: 10.3389/fonc.2022.713881
26. Zhang Y, He K, Guo Y, Liu X, Yang Q, Zhang C, et al. A novel multimodal radiomics model for preoperative prediction of lymphovascular invasion in rectal cancer. Front Oncol (2020) 10:457. doi: 10.3389/fonc.2020.00457
27. Chen X, Yang Z, Yang J, Liao Y, Pang P, Fan W, et al. Radiomics analysis of contrast-enhanced CT predicts lymphovascular invasion and disease outcome in gastric cancer: a preliminary study. Cancer Imag (2020) 20(1):24. doi: 10.1186/s40644-020-00302-5
28. Yang L, Chu W, Li M, Xu P, Wang M, Peng M, et al. Radiomics in gastric cancer: first clinical investigation to predict lymph vascular invasion and survival outcome using (18)F-FDG PET/CT images. Front Oncol (2022) 12:836098. doi: 10.3389/fonc.2022.836098
29. Tomita N, Okuda K, Osaga S, Miyakawa A, Nakanishi R, Shibamoto Y. Surgery versus stereotactic body radiotherapy for clinical stage I non-small-cell lung cancer: propensity score-matching analysis including the ratio of ground glass nodules. Clin Transl Oncol (2021) 23(3):638–47. doi: 10.1007/s12094-020-02459-8
Keywords: lymphovascular invasion, lung cancer, positron emission tomography computed tomography, radiomics, nomogram
Citation: Wang J, Zheng Z, Zhang Y, Tan W, Li J, Xing L and Sun X (2023) 18F-FDG PET/CT radiomics for prediction of lymphovascular invasion in patients with early stage non-small cell lung cancer. Front. Oncol. 13:1185808. doi: 10.3389/fonc.2023.1185808
Received: 10 May 2023; Accepted: 03 July 2023;
Published: 21 July 2023.
Edited by:
Xin Tang, Hangzhou Wuyunshan Hospital, ChinaReviewed by:
Shuheng Bai, The First Affiliated Hospital of Xi’an Jiaotong University, ChinaZhongxiang Ding, Zhejiang University, China
Copyright © 2023 Wang, Zheng, Zhang, Tan, Li, Xing and Sun. This is an open-access article distributed under the terms of the Creative Commons Attribution License (CC BY). The use, distribution or reproduction in other forums is permitted, provided the original author(s) and the copyright owner(s) are credited and that the original publication in this journal is cited, in accordance with accepted academic practice. No use, distribution or reproduction is permitted which does not comply with these terms.
*Correspondence: Xiaorong Sun, eHJzdW5Ac2RmbXUuZWR1LmNu
†ORCID: Jie Wang, orcid.org/0000-0001-7716-1302
Xiaorong Sun, orcid.org/0000-0001-8520-9556