- 1Department of Neurosurgery, Jiangmen Central Hospital, Jiangmen, China
- 2Department of Neurology, The First Hospital of Jilin University, Changchun, China
- 3Department of Neurosurgery, Cancer Hospital of Sun Yat sen University, Guangzhou, China
- 4Department of Neurosurgery, State Key Laboratory of Oncology in South China, Collaborative Innovation Center for Cancer Medicine, Sun Yat-sen University Cancer Center, Guangzhou, China
- 5Department of Hepatology, The First Hospital of Jilin University, Changchun, China
- 6Clinical College, Jilin University, Changchun, China
- 7Department of Bioengineering, University of Texas at Arlington, Arlington, TX, United States
Background: As a result of the COVID-19 pandemic, patients with glioblastoma (GBM) are considered a highly vulnerable population. Despite this, the extent of the causative relationship between GBM and COVID-19 infection is uncertain.
Methods: Genetic instruments for SARS-CoV-2 infection (38,984 cases and 1,644,784 control individuals), COVID-19 hospitalization (8,316 cases and 1,549,095 control individuals), and COVID-19 severity (4,792 cases and 1,054,664 control individuals) were obtained from a genome-wide association study (GWAS) from European populations. A total of 6,183 GBM cases and 18,169 controls from GWAS were enrolled in our study. Their associations were evaluated by applying Mendelian randomization (MR) including IVW meta-analysis, MR-Egger regression, and weighted-median analysis. To make the conclusions more robust and reliable, sensitivity analyses were performed.
Results: Our results showed that genetically predicted COVID−19 hospitalization increases the risk of GBM (OR = 1.202, 95% CI = 1.035–1.395, p = 0.016). In addition, no increased risk of SARS-CoV-2 infection, COVID-19 hospitalization and severity were observed in patients with any type of genetically predicted GBM.
Conclusion: Our MR study indicated for the first time that genetically predicted COVID−19 hospitalization was demonstrated as a risk factor for the development of GBM.
1 Introduction
In Coronavirus disease 2019 (COVID-19), the severe acute respiratory syndrome Coronavirus 2 (SARS-CoV-2) is responsible for the illness (1). Since January 1, 2023, more than 661 million people have died from COVID-19, which has caused the tragic loss of over 6 million lives. Coronavirus infections may cause acute cardiac or kidney injury, acute respiratory distress syndrome, shock, secondary infection, cancer, and high mortality risk (2). According to epidemiological studies, cancer is an independent adverse prognostic factor for COVID-19 outcomes, including admission to the intensive care unit and invasive ventilation (3–5). It is especially true for patients suffering from glioblastoma (GBM), which is one of the most aggressive and common types of primary brain tumor. Several factors make GBM patients one of the most fragile and vulnerable cancer populations. Firstly, GBM patients tend to be old age and have multiple age-related comorbidities. Additionally, their large use of steroid medications further increases immunosuppression. Furthermore, there is an increased risk of tumor and/or chemotherapy-related thromboembolic events due to the patient’s loss of autonomy. These result in a greater susceptibility to infection (6).
Besides, the role of COVID-19 in GBM was also a topic of interest. Angiotensin-converting enzyme 2 (ACE2) receptor molecules on the cell membrane interact with the viral spike (S) glycoprotein to allow viral entry (7). According to several studies, the viral S protein binds to the VEGFR (Vascular Endothelial Growth Factor Receptor) and the EGFR (Epidermal Growth Factor Receptor) more frequently in GBM cells than in other types of cancer cells, contributing to their development (8). It has been suggested that COVID-19 infections are associated with a unique brain predisposition to thrombosis caused by cytokine storms (9), which is correlated with faster GBM development. Poor prognosis is associated with tumor thrombus in GBM (10).
Several studies have shown a close relationship between GBM and COVID-19 susceptibility and severity, and traditional observational studies are biased by unmeasured confounding factors, making it difficult to speculate on their causal relationship (6, 11–13). However, observational studies are susceptible to unmeasured confounding or reverse causality. Single-nucleotide polymorphisms (SNPs) are used as instrumental variables (IVs) in Mendelian randomization (MR) studies to examine causal relationships between risk factors and outcomes (14, 15). We used the MR method to evaluate the causal associations between GBM and COVID-19 outcomes, given the limitations of the current research.
2 Methods
2.1 Study design
The overall design of our MR study exploring the causal relationships between GBM and COVID-19 outcomes can be seen in Figure 1. The study was conducted on a bidirectional two-sample univariable design. To estimate the causal effects of GBM on COVID-19, a genetically predicted GBM risk is used as an exposure and COVID-19 severity, hospitalization, or susceptibility is used as an outcome (16, 17). Based on genetically predicted COVID-19 severity, hospitalization, and susceptibility risks, we estimate the causal effects of COVID-19 on GBM. MR analysis is based on three critical assumptions: (i) There is a strong association between exposure and IVs; (ii) Confounders should not affect IVs due to exposure and outcome; and (iii) Only exposure mediates IV-outcome associations.
2.2 Data sources and instruments selection
We obtained summary-level GWAS data for the datasets of genetically predicted COVID-19 risk (38,984 cases and 1,644,784 control individuals for SARS-CoV-2 infection, 8,316 cases and 1,549,095 control individuals for COVID-19 hospitalization, and 4,792 cases and 1,054,664 control individuals for COVID-19 severity) (18). Participants in this GWAS were from the COVID-19 Host Genetics Initiative, which is publicly available (19). Those with very severe respiratory confirmed COVID-19 were defined as those hospitalized for lab confirmed SARS-CoV-2 infection and dying or requiring respiratory support (20). GBM is defined as glioblastoma (World Health Organization grade IV). GBM genotyping data were derived from a meta-analysis of GWAS, which included 6183 cases and 18169 controls (21). We provided comprehensive details of the genotyping and quality control of COVID-19 and GBM’s GWASs (Supplementary Table 1). We used 1000-Genome imputed GWAS data from the European GWAS (22). The mean sample size was larger for COVID-19 and GBM traits, so the genome-wide significance threshold (p < 5×10–9) was used to avoid selecting false positive instruments. In the European 1000G reference panel, SNPs with the lowest p-values were retained as independent SNPs after pruning all SNPs in linkage disequilibrium (LD; r2 < 0.0001) (23).. We cross-checked the Phenoscanner database (http://www.phenoscanner.medschl.cam.ac.uk/) to identify SNPs associated with the exposure that could potentially be linked to confounding variables or outcomes. We calculated the F-statistic for each SNP to evaluate the strength of the IVs (24, 25). Instruments with an F-statistic below 10 are considered weak (26). Supplementary Table 2 showed the characteristics of all the SNPs included in our analysis.
2.3 Statistical analysis
Several MR analytical methods were used to assess the causal effects and evaluate the potential pleiotropic effects of genetic variants. The main analysis was conducted using inverse-variance weighted (IVW) regression, which assumes no directional pleiotropic effects of individual instrumental variables (27). Weighted median and MR-Egger regression methods were used in complementary analyses (28). Additionally, we conducted sensitivity analyses using the Mendelian Randomization Pleiotropy RESidual Sum and Outlier (MR-PRESSO) global test, MR-Egger intercept, and leave-one-out sensitivity analysis. The sensitivity analysis was tested using a leave-one-out sensitivity analysis by removing each SNP from the analysis and re-estimating its causal effect (29). The horizontal pleiotropy of IVs was assessed by MR-PRESSO and MR-Egger intercept methods (p< 0.05 was considered significant) (30). SNPs with outliers are investigated in the MR-PRESSO global test (31). To test heterogeneity, IVW, and MR-Egger in Cochran’s Q statistic were used (p< 0.05 was considered significant) (32, 33). If the results of the IVW method are significant (p< 0.05), and no pleiotropy and heterogeneity were found, even if the results of other methods were not significant, as long as the beta values of other methods were in the same direction, they could be considered as positive results (34). In R (version 4.2.1), MRPRESSO (version 1.0) and TwoSampleMR (version 0.5.6) were used for the analyses.
3 Results
A comparison of MR estimates obtained from different methods of determining whether COVID-19 causes GBM is presented in Table 1. The IVW analysis revealed that the genetically determined COVID-19 hospitalized patients were at higher risk of developing GBM than the general population (OR = 1.202, 95% CI = 1.035–1.395, p = 0.016) (Figure 2). According to the MR-Egger intercept, there was no evidence of horizontal pleiotropy (p=0.918). Additionally, there was no obvious heterogeneity (all p-values were >0.05). In leave-one-out analysis, the effect of COVID‐19 SNPs on GBM was robust. For sensitivity analysis, leave-one-out studies were used and showed no influence (Figure 3). Furthermore, no significant association was found between severe COVID-19, SARS-CoV-2 infection, and the risk of GBM.
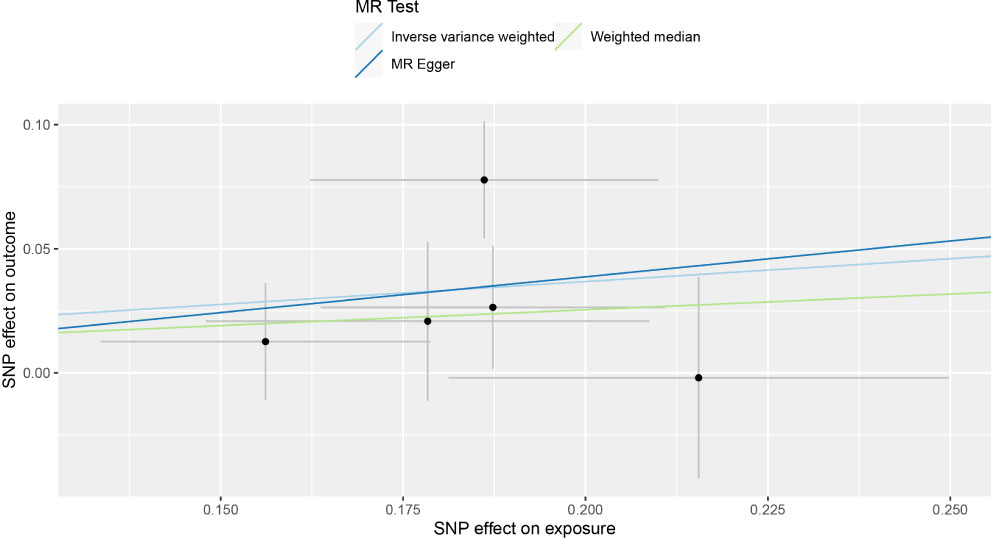
Figure 2 Individual estimates about the putative causal effect of COVID‐19 hospitalization on the risk of GBM.
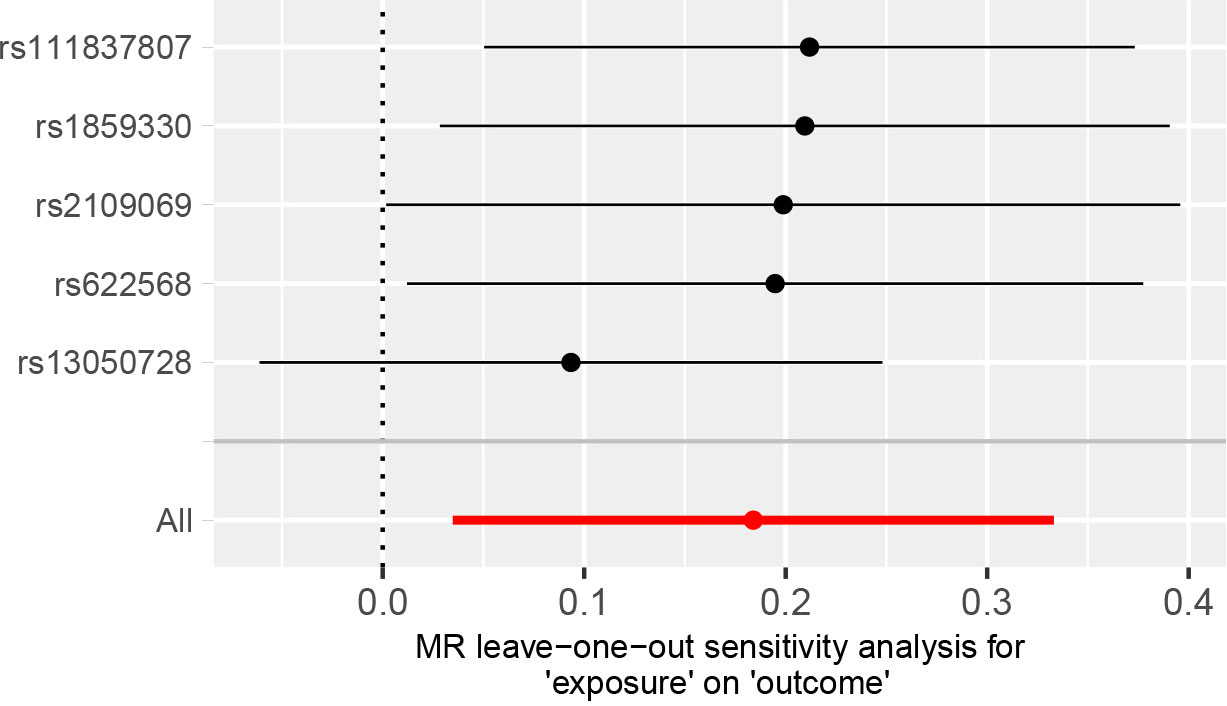
Figure 3 MR leave‐one‐out sensitivity analysis for the effect of COVID‐19 hospitalization SNPs on the risk of GBM.
With the same approach, we predicted the association between genetically predicted GBM and COVID-19 risk. All MR methods did not indicate an association between genetically predicted GBM and SARS-CoV-2 infection, COVID-19 hospitalization, or severity. MR, heterogeneity, pleiotropy, and sensitivity analyses of all methods associated with genetically predicted GBM and COVID-19 risk are summarized in Table 2.
4 Discussion
According to epidemiological studies, cancer is an independent adverse prognostic factor for COVID-19 (3). Due to a higher incidence of GBM in the elderly population, frequent hospitalizations, and treatment-related immunosuppression, COVID-19 is a pandemic that affects many patients with GBM (35, 36). The treatment of patients with high-grade gliomas has already been recommended by several expert groups (6, 37). Some preliminary cross-sectional studies have also supported the hypothesis that patients with GBM are biologically vulnerable to COVID-19 (38, 39). Methodological biases and unmeasured confounders prevented the causality of the association from being established. We investigated the causal relationship between GBM and COVID-19 susceptibility, hospitalization, and severity using public GWAS data.
The results of our MR analyses showed that genetically predicted COVID-19 hospitalization risk significantly increased the risk of GBM in the European population (OR = 1.202, 95% CI = 1.035–1.395, p = 0.016). The following mechanism might explain our results. As a receptor for human Coronavirus-229E (40), Alanyl aminopeptidase (ANPEP) plays an important role in the entry of SARS-CoV-2 into cells (41, 42). Based on its co-expression with ACE2, glutamyl aminopeptidase (ENPEP) has been identified as a candidate co-receptor for SARS-CoV-2 (42, 43). COVID-19 infection results in increased distribution of ENPEP and ANPEP in endothelial cells of the blood-brain barrier, providing the place for SARS-CoV-2 cell entry into the brain. Six receptors were analyzed for survival in GBM cells in a study, and it was found that ANPEP and ENPEP have a beneficial effect on survival. This increases the susceptibility of SARS-CoV-2 to GBM (44).
Healthy human lungs contain large amounts of 27-hydroxycholesterol (27-OHC) produced by macrophages in the alveoli (45). 27-OHC prevents the virus’s lipid envelope from fusing with the host cell membrane, making it difficult for the virus to enter the cell (46). Concentrations of 27-OHC will increase during the presence of COVID-19 infection in lung tissue and blood (47). At the same time, 27-OHC can also promote the growth of glioblastoma tumor cells in vitro by stimulating cell division, promoting cell migration and invasion (48). High levels of oxysterols found in glioblastoma tumors isolated from patients were associated with a poorer prognosis. Moreover, the findings confirmed that COVID-19 promotes the malignant behavior of GBM cells. Additionally, there is a possibility that COVID-19-associated coagulopathy could affect long-term tumor behavior and disease progression in GBM in a manner that has not yet been recognized.
According to our MR analyses, genetically predicted GBM risk is not associated with COVID-19 susceptibility, hospitalization, or severity in the European population. This differs from the findings of several preliminary cross-sectional studies (5, 49). Examples of possible confounding factors include the following: the severe lymphopenia often associated with disease or treatment (e.g., alkylating agents like nitrosourea and temozolomide), a frequent presence of neurological deficits resulting in a loss of autonomy in daily living activities and an increased risk of thromboembolic events, an increase in infection susceptibility as a result of chronic use of steroids to treat brain edema, and finally comorbidities and frailties associated with aging. Additionally, since most patients with GBM are hospitalized patients, the detection rate of COVID-19 in this situation may be higher than that in the non-hospitalized population. As a result, the COVID-19 incidence can appear to be higher in cancerous populations when the detection rate is confused with the actual incidence.
Our study has significant clinical implications. First, it has not been clear in the past whether COVID-19 increases the risk of GBM in patients without prior malignancies. In this study, we used Mendelian randomization to reveal that COVID−19 hospitalization increases the risk of developing glioblastoma. This suggests that cancer development is one such foreseeable COVID-19 sequelae since chronic inflammation is long-established to be a fertile ground for oncogenesis, especially for hospitalized patient. Better prevention of COVID-19 and possibly better evidence-based treatment of COVID-19 is warranted in these patients. Second, in our study, genetically predicted GBM risk is not associated with COVID-19 susceptibility, hospitalization, or severity in the European population. This will help us better allocate medical resources.
Our study, however, has several limitations. First, the results of other MR methods showed a consistent but nonsignificant direction. The best results would be obtained if all three methods were significant. The IVW approach, however, is statistically significantly more powerful than the other MR approaches, including MR-Egger (50). The requirement for MR approaches to follow a consistent beta direction has also been strengthened by research. We used this requirement in our study as well (51, 52). In addition, although our data did not show that a genetic predisposition to GBM is associated with COVID-19 susceptibility or severity, it is not appropriate for patients to assume that these patients can be treated at the same level as the general population for medical surveillance management. The third issue is that the sample is of mixed European ancestry. More studies should be carried out on other ethnic groups or immigrants to prove that the same relationship exists. Fourth, we don’t know what percentage of COVID-19 patients already have symptoms that may be related to glioblastoma. We are also unable to analyze the period after COVID-19 when risk arises, and the information provided for control groups remains incomplete.
In conclusion, even though our analysis suggests a causal link between genetically increased COVID-19 and increased risk of GBM, further studies are needed to determine the mechanism behind this association. To optimize the allocation of healthcare resources, it is crucial to identify those who are susceptible to SARS-CoV-2 and those who are prone to severe illness (53).
Data availability statement
Human data used in this study are publicly available. The database of Genotypes and Phenotypes (dbGaP) contains genotype data from the GWAS for the Glioma International Case-Control Consortium Study under accession phs001319.v1.p1. GWAS Data on COVID-19 is available for download from the website: COVID19-hg GWAS meta-analyses round 5 (covid19hg.org).
Author contributions
SW and JD drafted the article and contributed to its editing and revision. A bioinformatic analysis was conducted by HX, HZ, MQZ, and WY. JX, YP, MGZ, ZX, ZJ, KW, YW, YM and HC was responsible for the collation and analysis of the data. The manuscript was substantively edited by JD, and all authors have approved the final version.
Funding
This study was supported by the Digital Medicine and Additive Manufacturing Engineering Technology Research Center of Jiangmen Engineering Technology Research Center.
Acknowledgments
We thank Melin BS et al. for uploading the GBM Public GWAS, and Andrea Ganna et al. for founding the COVID-19 Host Genetics Project.
Conflict of interest
The authors declare that the research was conducted in the absence of any commercial or financial relationships that could be construed as a potential conflict of interest.
The reviewer, FL, declared a shared parent affiliation with the authors SW, JX, HZ and MQZ to the handling editor at the time of review.
Publisher’s note
All claims expressed in this article are solely those of the authors and do not necessarily represent those of their affiliated organizations, or those of the publisher, the editors and the reviewers. Any product that may be evaluated in this article, or claim that may be made by its manufacturer, is not guaranteed or endorsed by the publisher.
Supplementary material
The Supplementary Material for this article can be found online at: https://www.frontiersin.org/articles/10.3389/fonc.2023.1185466/full#supplementary-material
References
1. Ismail II, Salama S. Association of CNS demyelination and COVID-19 infection: an updated systematic review. J Neurol (2022) 269(2):541–76. doi: 10.1007/s00415-021-10752-x
2. Huang C, Wang Y, Li X, Ren L, Zhao J, Hu Y, et al. Clinical features of patients infected with 2019 novel coronavirus in Wuhan, China. Lancet (2020) 395(10223):497–506. doi: 10.1016/s0140-6736(20)30183-5
3. Zhang H, Han H, He T, Labbe KE, Hernandez AV, Chen H, et al. Clinical characteristics and outcomes of COVID-19-infected cancer patients: A systematic review and meta-analysis. J Natl Cancer Inst (2021) 113(4):371–80. doi: 10.1093/jnci/djaa168
4. Tian Y, Qiu X, Wang C, Zhao J, Jiang X, Niu W, et al. Cancer associates with risk and severe events of COVID-19: A systematic review and meta-analysis. Int J Cancer (2021) 148(2):363–74. doi: 10.1002/ijc.33213
5. Liang W, Guan W, Chen R, Wang W, Li J, Xu K, et al. Cancer patients in SARS-CoV-2 infection: a nationwide analysis in China. Lancet Oncol (2020) 21(3):335–7. doi: 10.1016/s1470-2045(20)30096-6
6. Mohile NA, Blakeley JO, Gatson NTN, Hottinger AF, Lassman AB, Ney DE, et al. Urgent considerations for the neuro-oncologic treatment of patients with gliomas during the COVID-19 pandemic. Neuro Oncol (2020) 22(7):912–7. doi: 10.1093/neuonc/noaa090
7. Paules CI, Marston HD, Fauci AS. Coronavirus infections-more than just the common cold. Jama (2020) 323(8):707–8. doi: 10.1001/jama.2020.0757
8. Khan I, Hatiboglu MA. Can COVID-19 induce glioma tumorogenesis through binding cell receptors? Med Hypotheses (2020) 144:110009. doi: 10.1016/j.mehy.2020.110009
9. Coolen T, Lolli V, Sadeghi N, Rovai A, Trotta N, Taccone FS, et al. Early postmortem brain MRI findings in COVID-19 non-survivors. Neurology (2020) 95(14):e2016–27. doi: 10.1212/wnl.0000000000010116
10. Reichard RR, Kashani KB, Boire NA, Constantopoulos E, Guo Y, Lucchinetti CF. Neuropathology of COVID-19: a spectrum of vascular and acute disseminated encephalomyelitis (ADEM)-like pathology. Acta Neuropathol (2020) 140(1):1–6. doi: 10.1007/s00401-020-02166-2
11. Zlotnik Y, Gadoth A, Abu-Salameh I, Horev A, Novoa R, Ifergane G. Case report: anti-LGI1 encephalitis following COVID-19 vaccination. Front Immunol (2021) 12:813487. doi: 10.3389/fimmu.2021.813487
12. Liu X, Guo C, Leng T, Fan Z, Mai J, Chen J, et al. Disease, Differential regulation of H3K9/H3K14 acetylation by small molecules drives neuron-fate-induction of glioma cell. Cell Death Dis (2023) 14(2):142. doi: 10.1038/s41419-023-05611-8
13. Zhong S, Ren J-X, Yu Z-P, Peng Y-D, Yu C-W, Deng D, et al. Predicting glioblastoma molecular subtypes and prognosis with a multimodal model integrating convolutional neural network, radiomics, and semantics. J Neurosurg. (2022) 1–10. doi: 10.3171/2022.10.JNS22801
14. Hartwig FP, Borges MC, Horta BL, Bowden J, Davey Smith G. Inflammatory biomarkers and risk of schizophrenia: A 2-sample mendelian randomization study. JAMA Psychiatry (2017) 74(12):1226–33. doi: 10.1001/jamapsychiatry.2017.3191
15. Sekula P, Del Greco MF, Pattaro C, Köttgen A. Mendelian randomization as an approach to assess causality using observational data. J Am Soc Nephrol (2016) 27(11):3253–65. doi: 10.1681/asn.2016010098
16. Zhang Z, Wang S, Ren F, Yang L, Xie H, Pan L, et al. Inflammatory factors and risk of meningiomas: a bidirectional mendelian-randomization study. Front Neurosci (2023) 17:1186312. doi: 10.3389/fnins.2023.1186312
17. Wang S, Pan L, Wu R, Shao Y, Xue M, Zhu H, et al. Oily fish and raw vegetable consumption can decrease the risk of AQP4-positive neuromyelitis optica spectrum disorders: a Mendelian-randomization study. Sci Rep (2023) 13(1):9372. doi: 10.1038/s41598-023-36372-1
18. A first update on mapping the human genetic architecture of COVID-19. Nature (2022) 608(7921):E1–e10. doi: 10.1038/s41586-022-04826-7
19. The COVID-19 Host Genetics Initiative, a global initiative to elucidate the role of host genetic factors in susceptibility and severity of the SARS-CoV-2 virus pandemic. Eur J Hum Genet (2020) 28(6):715–8. doi: 10.1038/s41431-020-0636-6
20. Yoshikawa M, Asaba K, Nakayama T. Estimating causal effects of genetically predicted type 2 diabetes on COVID-19 in the East Asian population. Front Endocrinol (Lausanne) (2022) 13:1014882. doi: 10.3389/fendo.2022.1014882
21. Melin BS, Barnholtz-Sloan JS, Wrensch MR, Johansen C, Il'yasova D, Kinnersley B, et al. Genome-wide association study of glioma subtypes identifies specific differences in genetic susceptibility to glioblastoma and non-glioblastoma tumors. Nat Genet (2017) 49(5):789–94. doi: 10.1038/ng.3823
22. Bentham J, Morris DL, Graham DSC, Pinder CL, Tombleson P, Behrens TW, et al. Genetic association analyses implicate aberrant regulation of innate and adaptive immunity genes in the pathogenesis of systemic lupus erythematosus. Nat Genet (2015) 47(12):1457–64. doi: 10.1038/ng.3434
23. Georgakis MK, de Lemos JA, Ayers C, Wang B, Björkbacka H, Pana TA, et al. Association of circulating monocyte chemoattractant protein-1 levels with cardiovascular mortality: A meta-analysis of population-based studies. JAMA Cardiol (2021) 6(5):587–92. doi: 10.1001/jamacardio.2020.5392
24. Shim H, Chasman DI, Smith JD, Mora S, Ridker PM, Nickerson DA, et al. A multivariate genome-wide association analysis of 10 LDL subfractions, and their response to statin treatment, in 1868 Caucasians. PloS One (2015) 10(4):e0120758. doi: 10.1371/journal.pone.0120758
25. Yarmolinsky J, Bonilla C, Haycock PC, Langdon RJQ, Lotta LA, Langenberg C, et al. Circulating selenium and prostate cancer risk: A mendelian randomization analysis. J Natl Cancer Inst (2018) 110(9):1035–8. doi: 10.1093/jnci/djy081
26. Burgess S, Small DS, Thompson SG. A review of instrumental variable estimators for Mendelian randomization. Stat Methods Med Res (2017) 26(5):2333–55. doi: 10.1177/0962280215597579
27. Burgess S, Scott RA, Timpson NJ, Davey Smith G, Thompson SG. Using published data in Mendelian randomization: a blueprint for efficient identification of causal risk factors. Eur J Epidemiol (2015) 30(7):543–52. doi: 10.1007/s10654-015-0011-z
28. Larsson SC, Scott RA, Traylor M, Langenberg CC, Hindy G, Melander O, et al. Type 2 diabetes, glucose, insulin, BMI, and ischemic stroke subtypes: Mendelian randomization study. Neurology (2017) 89(5):454–60. doi: 10.1212/wnl.0000000000004173
29. Burgess S, Davey Smith G, Davies NM, Dudbridge F, Gill D, Glymour MM, et al. Guidelines for performing Mendelian randomization investigations. Wellcome Open Res (2019) 4. doi: 10.12688/wellcomeopenres.15555.2
30. Aung N, Khanji MY, Munroe PB, Petersen SE. Causal inference for genetic obesity, cardiometabolic profile and COVID-19 susceptibility: A mendelian randomization study. Front Genet (2020) 11:586308. doi: 10.3389/fgene.2020.586308
31. Verbanck M, Chen CY, Neale B, Do R. Detection of widespread horizontal pleiotropy in causal relationships inferred from Mendelian randomization between complex traits and diseases. Nat Genet (2018) 50(5):693–8. doi: 10.1038/s41588-018-0099-7
32. Greco MF, Minelli C, Sheehan NA, Thompson JR. Detecting pleiotropy in Mendelian randomisation studies with summary data and a continuous outcome. Stat Med (2015) 34(21):2926–40. doi: 10.1002/sim.6522
33. Liu G, Zhang S, Cai Z, Ma G, Zhang L, Jiang Y, et al. PICALM gene rs3851179 polymorphism contributes to Alzheimer's disease in an Asian population. Neuromol Med (2013) 15(2):384–8. doi: 10.1007/s12017-013-8225-2
34. Chen X, Hong X, Gao W, Luo S, Cai J, Liu G, et al. Causal relationship between physical activity, leisure sedentary behaviors and COVID-19 risk: a Mendelian randomization study. J Transl Med (2022) 20(1):216. doi: 10.1186/s12967-022-03407-6
35. Ostrom QT, Cioffi G, Gittleman H, Patil N, Waite K, Kruchko C, et al. CBTRUS statistical report: primary brain and other central nervous system tumors diagnosed in the United States in 2012-2016. Neuro Oncol (2019) 21(Suppl 5):v1–v100. doi: 10.1093/neuonc/noz150
36. Gatson NTN, Barnholtz-Sloan J, Drappatz J, Henriksson R, Hottinger AF, Hinoul P, et al. Tumor treating fields for glioblastoma therapy during the COVID-19 pandemic. Front Oncol (2021) 11:679702. doi: 10.3389/fonc.2021.679702
37. Bernhardt D, Wick W, Weiss SE, Sahgal A, Lo SS, Suh JH, et al. Neuro-oncology management during the COVID-19 pandemic with a focus on WHO grade III and IV gliomas. Neuro Oncol (2020) 22(7):928–35. doi: 10.1093/neuonc/noaa113
38. Dai M, Liu D, Liu M, Zhou F, Li G, Chen Z, et al. Patients with cancer appear more vulnerable to SARS-coV-2: A multicenter study during the COVID-19 outbreak. Cancer Discovery (2020) 10(6):783–91. doi: 10.1158/2159-8290.Cd-20-0422
39. Moujaess E, Kourie HR, Ghosn M. Cancer patients and research during COVID-19 pandemic: A systematic review of current evidence. Crit Rev Oncol Hematol (2020) 150:102972. doi: 10.1016/j.critrevonc.2020.102972
40. Millet JK, Jaimes JA, Whittaker GR. Molecular diversity of coronavirus host cell entry receptors. FEMS Microbiol Rev (2021) 45(3). doi: 10.1093/femsre/fuaa057
41. Tang D, Comish P, Kang R. The hallmarks of COVID-19 disease. PloS Pathog (2020) 16(5):e1008536. doi: 10.1371/journal.ppat.1008536
42. Qi F, Qian S, Zhang S, Zhang Z. Single cell RNA sequencing of 13 human tissues identify cell types and receptors of human coronaviruses. Biochem Biophys Res Commun (2020) 526(1):135–40. doi: 10.1016/j.bbrc.2020.03.044
43. Tripathi SC, Deshmukh V, Creighton CJ, Patil A. Renal carcinoma is associated with increased risk of coronavirus infections. Front Mol Biosci (2020) 7:579422. doi: 10.3389/fmolb.2020.579422
44. Chen A, Zhao W, Li X, Sun G, Ma Z, Peng L, et al. Comprehensive oncogenic features of coronavirus receptors in glioblastoma multiforme. Front Immunol (2022) 13:840785. doi: 10.3389/fimmu.2022.840785
45. Kikuchi T, Sugiura H, Koarai A, Ichikawa T, Minakata Y, Matsunaga K, et al. Increase of 27-hydroxycholesterol in the airways of patients with COPD: possible role of 27-hydroxycholesterol in tissue fibrosis. Chest (2012) 142(2):329–37. doi: 10.1378/chest.11-2091
46. Cagno V, Civra A, Rossin D, Calfapietra S, Caccia C, Leoni V, et al. Inhibition of herpes simplex-1 virus replication by 25-hydroxycholesterol and 27-hydroxycholesterol. Redox Biol (2017) 12:522–7. doi: 10.1016/j.redox.2017.03.016
47. Marcello A, Civra A, Milan Bonotto R, Nascimento Alves L, Rajasekharan S, Giacobone C, et al. The cholesterol metabolite 27-hydroxycholesterol inhibits SARS-CoV-2 and is markedly decreased in COVID-19 patients. Redox Biol (2020) 36:101682. doi: 10.1016/j.redox.2020.101682
48. Liu L, Li MY, Xing Y, Wang XY, Wang Y. The oncogenic roles of 27-hydroxycholesterol in glioblastoma. Oncol Lett (2019) 18(4):3623–9. doi: 10.3892/ol.2019.10690
49. Zhang Y, Mao Q, Li Y, Cheng J, Xia Q, Chen G, et al. Cancer and COVID-19 susceptibility and severity: A two-sample mendelian randomization and bioinformatic analysis. Front Cell Dev Biol (2021) 9:759257. doi: 10.3389/fcell.2021.759257
50. Lin Z, Deng Y, Pan W. Combining the strengths of inverse-variance weighting and Egger regression in Mendelian randomization using a mixture of regressions model. PloS Genet (2021) 17(11):e1009922. doi: 10.1371/journal.pgen.1009922
51. Bowden J, Del Greco MF, Minelli C, Davey Smith G, Sheehan NA, Thompson JR. Assessing the suitability of summary data for two-sample Mendelian randomization analyses using MR-Egger regression: the role of the I2 statistic. Int J Epidemiol (2016) 45(6):1961–74. doi: 10.1093/ije/dyw220
52. Venkatesh SS, Ferreira T, Benonisdottir S, Rahmioglu N, Becker CM, Granne I, et al. Obesity and risk of female reproductive conditions: A Mendelian randomisation study. PloS Med (2022) 19(2). doi: 10.1371/journal.pmed.1003679
Keywords: Mendelian randomization, COVID-19, SARS-CoV-2, glioblastoma, genome-wide association study
Citation: Dong J, Wang S, Xie H, Mou Y, Zhu H, Peng Y, Xi J, Zhong M, Xie Z, Jiang Z, Wang K, Chen H, Yang W, Zhu M, Wen Y and Wu Y (2023) COVID−19 hospitalization increases the risk of developing glioblastoma: a bidirectional Mendelian-randomization study. Front. Oncol. 13:1185466. doi: 10.3389/fonc.2023.1185466
Received: 13 March 2023; Accepted: 01 August 2023;
Published: 21 August 2023.
Edited by:
Wei Wang, Massachusetts General Hospital and Harvard Medical School, United StatesReviewed by:
Feng Lin, Jilin University, ChinaJorge Matias-Guiu, Complutense University of Madrid, Spain
Xu-zhao Li, Guizhou University of Traditional Chinese Medicine, China
Copyright © 2023 Dong, Wang, Xie, Mou, Zhu, Peng, Xi, Zhong, Xie, Jiang, Wang, Chen, Yang, Zhu, Wen and Wu. This is an open-access article distributed under the terms of the Creative Commons Attribution License (CC BY). The use, distribution or reproduction in other forums is permitted, provided the original author(s) and the copyright owner(s) are credited and that the original publication in this journal is cited, in accordance with accepted academic practice. No use, distribution or reproduction is permitted which does not comply with these terms.
*Correspondence: Jiajun Dong, ZG9uZ2ppYWp1bjQwOEAxMjYuY29t
†These authors have contributed equally to this work and share first authorship