- Department of Pediatrics, The First Affiliated Hospital, Sun Yat-sen University, Guangzhou, Guangdong, China
Introduction: Arsenic trioxide (ATO) is a promising anticancer drug for hematological malignancy. Given the dramatic efficacy of acute promyelocytic leukemia (APL), ATO has been utilized in other types of cancers, including solid tumors. Unfortunately, the results were not comparable with the effects on APL, and the resistance mechanism has not been clarified yet. This study intends to identify relevant genes and pathways affecting ATO drug sensitivity through genome-wide CRISPR-Cas9 knockdown screening to provide a panoramic view for further study of ATO targets and improved clinical outcomes.
Methods: A genome-wide CRISPR-Cas9 knockdown screening system was constructed for ATO screening. The screening results were processed with MAGeCK, and the results were subjected to pathway enrichment analysis using WebGestalt and KOBAS. We also performed protein-protein interaction (PPI) network analysis using String and Cytoscape, followed by expression profiling and survival curve analysis of critical genes. Virtual screening was used to recognize drugs that may interact with the hub gene.
Results: We applied enrichment analysis and identified vital ATO-related pathways such as metabolism, chemokines and cytokines production and signaling, and immune system responses. In addition, we identified KEAP1 as the top gene relating to ATO resistance. We found that KEAP1 expression was higher in the pan-cancer, including ALL, than in normal tissue. Patients with acute myeloid leukemia (AML) with higher KEAP1 expression had worse overall survival (OS). A virtual screen showed that etoposide and eltrombopag could bind to KEAP1 and potentially interact with ATO.
Discussion: ATO is a multi-target anticancer drug, and the key pathways regulating its sensitivity include oxidative stress, metabolism, chemokines and cytokines, and the immune system. KEAP1 is the most critical gene regulating ATO drug sensitivity, which is related to AML prognosis and may bind to some clinical drugs leading to an interaction with ATO. These integrated results provided new insights into the pharmacological mechanism of ATO and potentiate for further applications in cancer treatments.
1 Introduction
Arsenic trioxide (ATO) is an established agent in treating acute promyelocytic leukemia (APL), a hematological malignancy with the unexpected fusion promyelocytic leukemia-retinoic acid receptor α (PML-RARa) as molecular characterization (1). ATO degrades the existing PML-RARa and triggers cell death by apoptosis (2) and autophagy (3–6). Another target, Fms-like tyrosine kinase 3 gene internal tandem duplication (FLT3-ITD), has been confirmed to be downregulated by ATO, resulting in autophagic degradation and cell cytotoxicity (7–9). Apart from targeting these abnormal fusions or mutated proteins, ATO has been proven with a pro-oxidant effect (10) in treating cancer cells and can rescue structural p53 mutations (11), providing a promising anticancer therapy in other hematological diseased and solid tumors. However, the current trials revealed that ATO was less effective in other types of cancers compared with its above 90% cure rate in APL (12–22). This unsatisfactory result may be related to the insufficient concentration in solid tumor sites and the primary or acquired resistance to ATO. The solutions for the former problem mainly focus on improving the drug delivery system by nanoparticles from exogenous materials (23, 24) or biomimetic nanocarriers such as ferritin (25). As for the resistance caused by genetic events, most studies have recognized PML mutations as a primary resistant mechanism (1, 26), and treatments targeting PML-RARa fusion protein showed a synergistic antileukemic effect in combination with ATO (27, 28). The epigenetic changes induced by ATO also made them valuable targets for overcoming resistance (29). Unfortunately, these results cannot explain the resistance in other cancers which do not harbor such mutations. Furthermore, the current combination methods of ATO concentrate more on regulating the reactive oxygen species (ROS) contents to overcome resistance with limited effect (26, 30–33). Therefore, a more systematic landscape to describe genetic events and identify potential targets or entrances of ATO is required to gain a fully comprehensive understanding of the pharmacology of ATO and further overcome resistance.
Genome-wide CRISPR-Cas9 screening is a revolutionary method appearing in biomedicine in the last decade. Based on the CRISPR-Cas9 system, this screening system effectively creates a genome-wide knockout cell library, and the cells are further challenged with certain drugs, toxins, or virals. The depleted genes will contribute to a specific phenotype, such as cell death or survival, and thus are selected as essential targets of chemical and biological agents. Taking advantage of its high throughput (nearly 20,000 genes at one time) and unbiased nature, the CRISPR-Cas9 screening has emerged as a handy technique for identifying the genes or pathways relevant to the sensitivity or resistance of existing treatments. The determinants of cell response to a list of anticancer drugs have been identified successfully through this method, including traditional chemo agents [Ara-C (34), etoposide (35), lenalidomide (36), and cisplatin (37), e.g.] or small molecular-targeted drugs such as vemurafenib (38–40), panobinostat (41), alisertib (42), ceralasertib (43), olaparib (44–46), selumetinib (47), and trametinib (47).
The Kelch-like ECH-associated protein 1 (KEAP1) is the central cellular defense module against oxidative stresses. As a cysteine thiol-rich sensor of redox stress, KEAP1 binds to and represses NF-E2-related factor 2 (NRF2) through the ubiquitin-26S proteasome pathway in quiescent but releases it on exposure to stress. NRF2 then induces the transcription of several cytoprotective genes to defend against redox insults such as ROS (48). Notably, the production of ROS contributes to the cytotoxicity of cancer cells, indicating the significant role KEAP1 plays in therapeutic resistance. The loss-of-function mutations in KEAP1 confer radiation resistance in non-small cell lung cancer (NSCLC) (49, 50). Several studies have confirmed that the KEAP1–NRF2 complex renders chemoresistance in treatments for colorectal cancer, esophageal squamous cancer, and pancreatic cancer (51–53). The underlying mechanism of resistance relates to the robust production of detoxification molecules, including NAD(P)H quinone dehydrogenase 1 (NQO1), glutathione-S-transferase (GST), and glutathione (GSH), which conjugate chemo agents like cisplatin and then excreted (54, 55). Therefore, KEAP1 is a crucial regulator in cell response to cancer therapy.
In this study, we performed a genome-wide CRISPR-Cas9 loss-of-function screening on ATO to identify the essential genes and pathways in cell response to ATO. The enriched screening results showed that the genes related to metabolism, chemokines and cytokines production and signaling, and immune system responses might contribute to cell death induced by ATO in addition to the effect of ROS. These findings provided a thorough understanding of mechanistic effects and a broader view of the potential application of ATO.
2 Materials and methods
2.1 Cells and plasmids
The 293T and HAP1 cells used in screening were kept in our laboratory. Dulbecco’s Modified Eagle Medium (DMEM, HyClone) was used for 293T maintenance, and Iscove’s Modified Dulbecco’s Medium (IMDM, HyClone) for HAP1 cells. The mediums were supplemented with 10% fetal bovine serum (FBS, NEWZERUM) and 1% penicillin/streptomycin (HyClone). All cells were maintained in a humidified, 5% CO2 incubator at 37°C. The plasmids psPAX2 (RRID: Addgene_12260) and pMD2.G (RRID: Addgene_12259) were obtained via Addgene. Human Brunello CRISPR knockout pooled library was a gift generously provided by David Root and John Doench (RRID: Addgene_73179) (40).
2.2 Genome-wide CRISPR-Cas9 screening
We followed the protocol published by Zhang Feng et al. (56) to conduct the experiments. We utilized the sgRNA library from David Root and John Doench (40), the Brunello library. The plasmid library contains 76,441 gRNAs targeting 19,114 human genes. We used 293T cells, helper plasmids (psPAX2 and pMD2.G), and the plasmid library to generate a lentivirus pool. The culture media of HAP1 cells were replaced with fresh media containing 8 μg/ml polybrene (Beyotime) before lentivirus transfection. To maintain the representativity of the library, we transfected the HAP1 cells at a representation of 500 cells per sgRNA. The multiplicity of infection (MOI) was 0.3 in this study to ensure that each cell obtained one sgRNA. Cells were then cultured under 1 μg/ml puromycin (Beyotime) for 7 days and split into two groups with ATO (2 μM, SLPHARM) or vehicle (DMSO) treatment for 14 days. After propagating to identify sgRNAs enriched or depleted, the genomic DNAs of harvested cells were extracted by TIANamp Genomic DNA Kit (#DP304, TIANGEN) and PCR amplified. The PCR fragments were gel-purified utilizing E.Z.N.A.®Gel Extraction Kit (#D2500, OMEGA) and sequenced by Novogene (Beijing, China). The genes enriched or depleted were identified by the model-based analysis of genome-wide CRISPR-Cas9 knockout (MAGeCK) software (https://sourceforge.net/projects/mageck/) with default parameters. The MAGeCK-RRA algorithm counted each gene’s Robust Rank Aggregation (RRA) score. Essential genes were identified at p < 0.05 and |log2 fold change| ≥1 by comparing ATO-treated sgRNA with vehicle-treated sgRNA. Sequencing data are deposited at Gene Expression Omnibus (GSE218982).
2.3 Functional enrichment analysis
WEB-based GEne SeT AnaLysis Toolkit (WebGestalt, v.2019, RRID: SCR_006786) is a widely used functional enrichment analysis web tool (57–60). In this study, WebGestalt was used to conduct Gene Ontology (GO) enrichment analysis (daily build accessed on 01/14/2019), including biological process (BP), cellular component (CC), and molecular function (MF) categories. KEGG Orthology Based Annotation System (KOBAS, v.3.0, RRID: SCR_006350) is a web server for gene functional annotation and enrichment (61). The enrichment analysis is based on the ORA (overrepresentation analysis) method, a simple and frequently used gene set enrichment method using the Hypergeometric test and Fisher’s exact test. False discovery rate (FDR) correction (Q-value) was performed by Benjamini and Hochberg method. In this study, we used KOBAS and chose three databases, namely, REACTOME (62), Protein ANalysis THrough Evolutionary Relationships (PANTHER) (63, 64), and Kyoto Encyclopedia of Genes and Genomes (KEGG) (65), for cell informative pathway enrichments. The cutoff value was indicated in figure legends.
2.4 Construction of the protein–protein network
The Search Tool for the Retrieval of Interacting Genes/Proteins (STRING, v.11.5, RRID: SCR_005223) database (66) and Cytoscape (v.3.9.1, RRID: SCR_003032) software (67) were used to establish and visualize a protein–protein interaction (PPI) network of depleted and enriched genes identified by the genome-wide CRISPR-Cas9 screening of ATO. The plugin Molecular COmplex DEtection (MCODE) (68) in Cytoscape was applied to calculate the degree of each protein and recognized the key modules and hub genes with default parameters (degree cutoff at 2, node score cutoff at 0.2, K-core at 2, and max depth at 100). The hub genes of the top cluster identified by MCODE were then analyzed by the plugin Cluego (69) and CluePedia (70) for REACTOME pathway enrichment (25.05.2022 version).
2.5 Gene expression profiles, correlation analysis, and survival analysis
TNMplot (https://tnmplot.com/analysis) is a web analysis tool focused on differential gene expression in tumor, normal, and metastatic tissues (71). This database utilizes RNA-seq and clinical data from GTex, TCGA, and TARGET databases to analyze gene expression comparison in a selected tumor or pan-cancer. This study applied this tool to investigate the KEAP1 gene expression in acute lymphoblastic leukemia (ALL) compared with normal tissues utilizing TARGET (https://ocg.cancer.gov/programs/target), a database with comprehensive molecular characterization and clinical prognosis of childhood cancers. We also used TNMplot to generate the KEAP1 gene expression profiles in pan-cancer.
GEPIA2 (RRID: SCR_018294) is a newly developed interactive web server for analyzing the RNA-seq data of samples from the GTex and TCGA projects and is widely used for patient survival analysis (72). This research used this web tool to identify whether the KEAP1 gene expression was related to prognosis in acute myeloid leukemia (AML) patients.
The half maximal inhibitory concentration (IC50) values of ATO in different cell lines were collected from our former study (8) and Cellminer (NCI-60 project) (73). The KEAP1 expression per kilobase per million reads log2(FPKM + 1) values were collected from the NCI-60 project (74) and Depmap expression public 22Q4 (https://depmap.org/portal/). The correlation analyses were performed using Pearson correlation.
2.6 Virtual screening
A total of 2,506 FDA-approved drugs from DRUGBANK (RRID: SCR_002700) were involved in the virtual screening. The ligand database was energy minimized using MMFF force field (steepest descent) in the open-source OpenBabel software package (http://openbabel.org/) and saved in mol2 format. The compound library is then pre-processed and saved in pdbqt format using prepare_ligand4.py from AutoDock tools (https://ccsb.scripps.edu/mgltools/).
The crystal structure of the human KEAP1 BTB domain and Kelch domain was obtained from RCSB PDB (RRID: SCR_012820, https://www.rcsb.org, PDB ID: 7EXI, 6TYM) (75). Proteins were pre-processed in PyMol (http://pymol.org/2/, RRID: SCR_000305) (76) to remove waters and ligands and in AutoDock tools to add polar hydrogens, charges by Kollman charge, and saved in pdbqt format. The grid box of the KEAP1 kelch domain was defined by ligand in 6TYM. The binding pocket of the KEAP1 BTB domain was predicted by DeepSite (https://www.playmolecule.com/deepsite/). The grid box was defined by employing AutoDock tools’ Grid setting feature.
We used Smina for molecular docking, an open-source molecular docking software based on AutoDock Vina (https://vina.scripps.edu) (77–79). Molecular docking parameters in this study are as follows: exhaustiveness = 8, num_modes = 10, energy_range = 3, min_rmsd_filter = 1. After the complete execution of docking, the top 20 minimized affinity ligands were obtained. Subsequently, the neural-network-based scoring function (NNScore2) of those 20 ligands was calculated, and the top 10 compounds for each domain with predictive IC50 value were selected as potential candidates targeting KEAP1 (80). We used PyMol to visualize the docking position.
2.7 Data visualization and statistical analysis
Figures 1B, 2B, and 4A were plotted by ImageGP (http://www.ehbio.com/ImageGP/index.php/Home) (81). The other figures were visualized as indicated in figure captions. The methods for the significance test were described in related texts or figure legends.
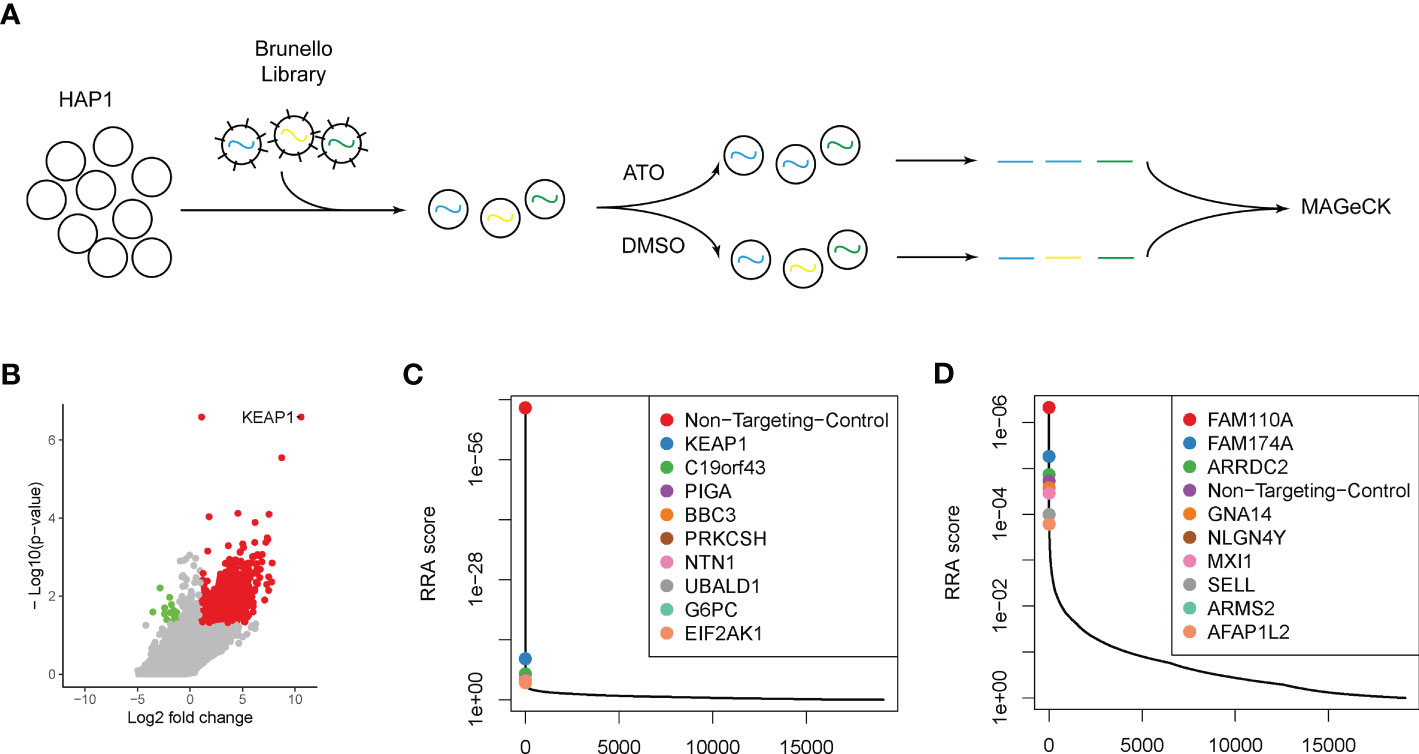
Figure 1 Genome-wide CRISPR-Cas9 screening identified key modulators attributing to ATO sensitivity. (A) The schematic outline of the genome-wide CRISPR-Cas9 screening. (B) The volcano plot of genes significantly enriched (red dots) or depleted (green dots) in ATO treatment groups compared with vehicle. The cutoff threshold was |log2 fold change| ≥1 and P < 0.05. (C) The positively selected genes in ATO treatment groups compared with vehicle. (D) The negatively selected genes in ATO treatment groups compared with vehicle.
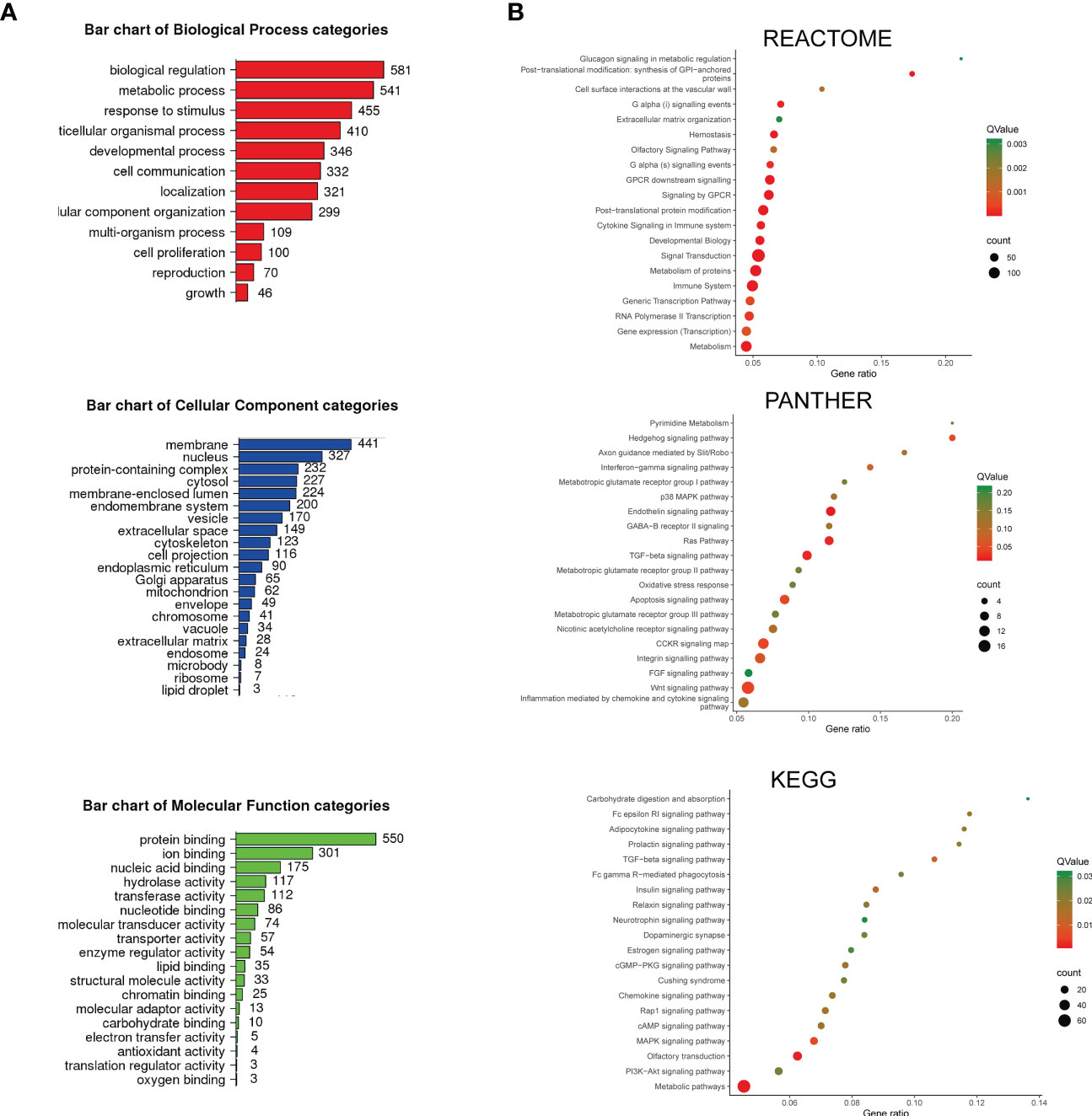
Figure 2 The enrichment analysis of depleted and enriched genes was identified by screening. (A) The bar charts of Gene Ontology (GO) enriched terms. The enrichment analysis was performed and plotted by WebGestalt. The top most relevant GO terms with an adjusted P < 0.05 were shown in the charts. (B) Pathway enrichment analysis. The enrichment analysis was performed by KOBAS. REACTOME, PANTHER, and KEGG-enriched terms were presented separately. The color demonstrated the Q-value of each pathway, and the size of the dots demonstrated the count of genes related to the pathway.
3 Results
3.1 Genome-wide CRISPR-Cas9 screening of arsenic trioxide in HAP1 cells
To recognize the essential genes and pathways related to the sensitivity or resistance of ATO, we performed a genome-wide CRISPR-Cas9 knockout loss-of-function screen in the HAP1 cell line (Figure 1A). We chose this cell model according to its haploid nature, so that the integrity sister chromatid would not mask the knockout effect. The Brunello sgRNA library has been previously described and targets 19,114 human genes with 76,441 distinct sgRNAs (40). The transduced cell library was then exposed to 2 μM ATO (IC50 of HAP1 cells to ATO) or DMSO as a control group. The genomic DNAs were collected, PCR amplified, and sequenced. Using the MAGeCK tool (82), we examined the data to determine the relative enrichment or depletion of each sgRNA compared with the control groups. We mapped the genes whose deletion resulted in ATO sensitivity (results shown in Supplementary Table S1). Nine hundred eighty-four genes were recognized as contributors to the sensitivity to ATO with |log2 fold change| ≥1 and p < 0.05 (Figure 1B). Among these genes, we identified 970 positively selected genes whose deletion contributed to the resistance to ATO or were potential targets as the entrance of cell responses to ATO (Figure 1B, red dots). The top genes ranked by RRA score were listed and plotted (Figure 1C). These genes included Kelch ECH associating protein 1 (KEAP1), a sensor of oxidative stress, which has been confirmed to contribute to the progression and resistance to chemo- and radiotherapy in many types of cancers (83, 84). BBC3, coding BCL2-binding component involved in p53 signaling apoptosis, was also highly selected. Genes involved in the endoplasmic reticulum (ER) stress were also found in the top-ranked candidates, PRKCSH and EIF2AK1. Other genes positively selected in this study, including C19orf43, PIGA, NTN1, UBALD1, and G6PC, have not yet been related to cell response to ATO. Meanwhile, we recognized 15 negatively selected genes under the treatment of ATO (Figure 1B, green dots). The deletion of this group of genes can improve the sensitivity to ATO and are potential targets in combination with ATO to overcome the resistance to ATO. The top 10 selected genes ranked by RRA score were listed in Figure 1D. The genes involved in cell cycle regulation should be identified in negativity selection, because the deletions of this group of genes were bound to induced cytotoxicity. In this study, we recognized FAM110A, localized to centrosomes and accumulated at the microtubule organization center, and AFAP1L2, regulating the mitotic cell cycle, as cell cycle–related genes in response to ATO. We also identified several genes functioning in cell adhesion. GNA14, encoding a member of the guanine nucleotide binding, or G protein family, was a well-known target in hepatocellular carcinoma and vascular tumors (85, 86). The protein encoded by NLGN4Y (Neuroligin 4 Y-Linked) is presented at the postsynaptic side of the synapse (87). Another vital cell surface adhesion molecule, SELL (Selectin L, or LAM-1), mediated cell adhesion by binding to glycoproteins on neighboring cells in a calcium-dependent manner, a key regulator in viral infection (88–91). In addition, a tumor suppressor negatively regulating MYC function in lung cancer, MXI1, was also identified in the selection (92). The exact function of the other genes in the top rank, FAM174A, ARRDC2, and ARMS2, remained unknown in ATO or cancer research.
3.2 Enrichment analysis of pathways relating to arsenic trioxide sensitivity
To understand the overview mechanism of cell response in sensitivity to ATO, we performed enrichment analysis on the 984 genes recognized with |log2 fold change| ≥1 and P < 0.05 in screening. First, we applied WebGestalt for GO analysis to annotate genes products and characteristics (Figure 2A). GO terms with a P < 0.05 were displayed. The top GO enrichment items were classified into three functional categories, namely, BP (12 items, Figure 2A, red panel), CC (21 items, Figure 2A, blue panel), and MF (18 items, Figure 2A, green panel). These genes were mainly enriched in biological regulation, metabolic process, response to stimulus, multicellular organismal process, and developmental process referring to BP. The most enriched CC annotations included membrane, nucleus, protein-containing complex, cytosol, and membrane-enclosed lumen. As for MF, these genes were significantly involved in protein binding, ion binding, nucleic acid binding, hydrolase activity, and transferase activity.
Next, we utilized KOBAS and performed the pathway enrichment analysis. We chose three databases (REACTOME, PANTHER, and KEGG) for their enriched tactics and annotations (Figure 2B). REACTOME focused on the reactions and grouped entities into a network based on their reaction participation. Most of the enriched pathways in this study were linked with metabolism, including glucagon signaling in metabolic regulation and metabolism of proteins, followed by GPCR downstream signaling events, cytokine signaling, immune system, and transcription events. PANTHER clustered proteins through evolutionary and functional relationships. The enrichment study using this database revealed that the cell response to ATO was significantly relevant to pyrimidine metabolism, Hedgehog signaling pathway, and interferon−gamma signaling pathway. In addition, the pathways involving the metabotropic glutamate receptor groups and a broad spectrum of chemokine and cytokine signaling (p38 MAPK, Ras, TGF−beta, FGF, and Wnt) were also enriched. It should be noted that the oxidative stress response and apoptosis signaling pathway were also included, which were consistent with the top-rank gene result in this study and the known mechanism. KEGG signaling pathway annotations, one of the most frequently used open databases integrating genomic and systemic functional information, were also analyzed. The enriched pathways were associated with a series of metabolism processes, including carbohydrate digestion and absorption, adipocytokine, insulin, estrogen signaling pathways, and Cushing syndrome. The immune-related pathways (Fc epsilon RI signaling, Fc gamma R−mediated phagocytosis, and neurotrophin signaling pathway) and chemokine and cytokine signals (TGF−beta, cGMP−PKG, Rap1, MAPK, and PI3K-Akt) were also included. Therefore, these results demonstrated that the sensitivity of ATO could be affected by varied cell responses relating to metabolism, chemokines and cytokines, and the immune system, indicating that ATO was an anticancer candidate with broad targets and functions.
3.3 Hub genes recognized by a protein–protein interaction network
To understand the relationship between genes recognized in the screening, we performed a PPI network analysis. PPI assessed the physical and functional connections between the proteins of target genes to provide new aspects of the selected genes. The PPI network was illustrated using the STRING website and visualized in Cytoscape software (Figure 3A, left). The stability of the entire network mostly depends on nodes (genes in this context) with a higher degree of connectivity, that is, the core module. To recognize the significant gene modules in this network, we used the plugin MCODE with default parameters and identified a cluster with the highest hub score of 6.24 (Figure 3A, right). This essential module contained 26 hub genes, including important transcription factors (ATF2 and E2F4) and kinases (FLT3 and AKT1). To explore the functional relationships between hub genes, we performed REACTOME pathway enrichment analysis with the plugins Cluego and CluePedia (Figure 3B). The hub genes were mainly enriched in CREB1 phosphorylation by activating Adenylate Cyclase, FOXO-mediated transcription, and RUNX1 interactions. These results revealed that ATO was significantly related to transcription factor activity.
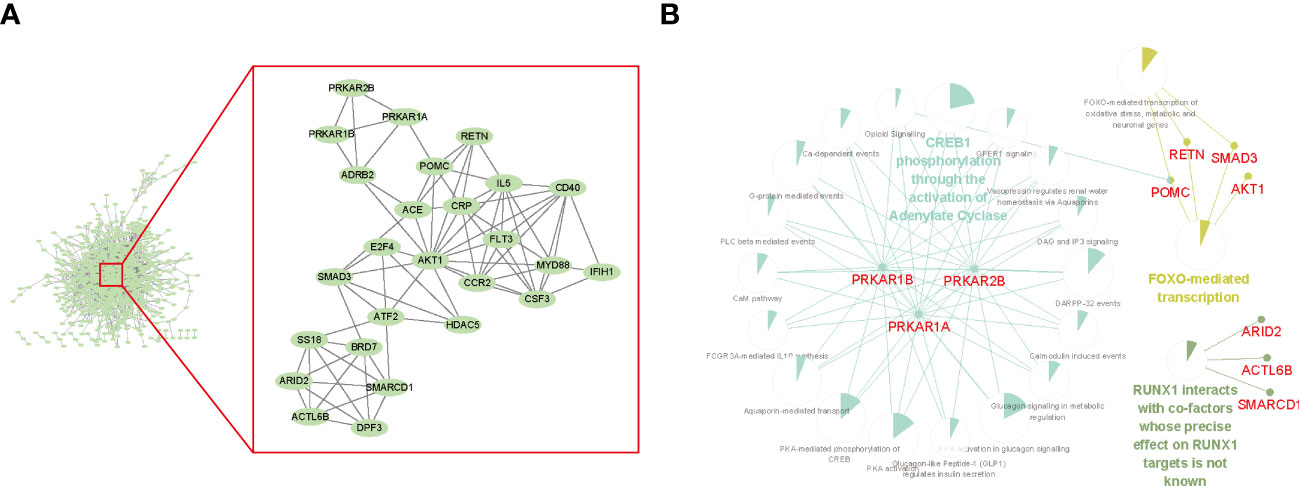
Figure 3 Protein–protein interaction (PPI) network among depleted or enriched genes. (A) The hub genes of the PPI. The PPI network was generated by the STRING database with default settings and was built by Cytoscape. The cluster of hub genes within the red frame was calculated using MCODE in Cytoscape with the default setting. (B) The top enriched REACTOME pathways of the hub genes. The enrichment analysis was performed and visualized by Cytoscape using the plugin Cluego and CluePedia. The sector graphs indicated the portion of related genes compared with all the enriched pathway genes.
3.4 KEAP1 as a critical modulator in sensitivity to arsenic trioxide
A similar genome-wide CRISPR-Cas9 screening focused on ATO has been performed by Amin Sobh et al. They utilized a different sgRNA library (GeCKO v2 sgRNA library containing 65,383 sgRNA targeting approximately 19,000 human genes) and cell lines [K562, a Chronic Myelogenous Leukemia (CML) cell line derived from human bone marrow] and identified a total of 151 genes with significance (93). We speculated that the essential genes relating to ATO sensitivity should be recognized in these two screens and compared the enriched or depleted genes in our study with the another. The Venn diagram showed that the overlapping genes were KEAP1, ZNF844, SLC22A18AS, CCNL2, and EIF2AK1 (Figure 4A). Among these five genes, KEAP1 was top ranked in both two studies. It seemed that the deletion of KEAP1 significantly damaged the cell death to ATO and may be a key entrance target of ATO to trigger cell responses. We reviewed the counts of sgRNAs targeting KEAP1 in different groups and found that three of four sgRNAs were significantly enriched in ATO-treated groups (Figure 4B). In addition, the IC50 values of ATO were negatively correlated with KEAP1 expression in leukemic cell lines (Figure 4C). These results illustrated that KEAP1 did play a role in cell sensitivity to ATO.
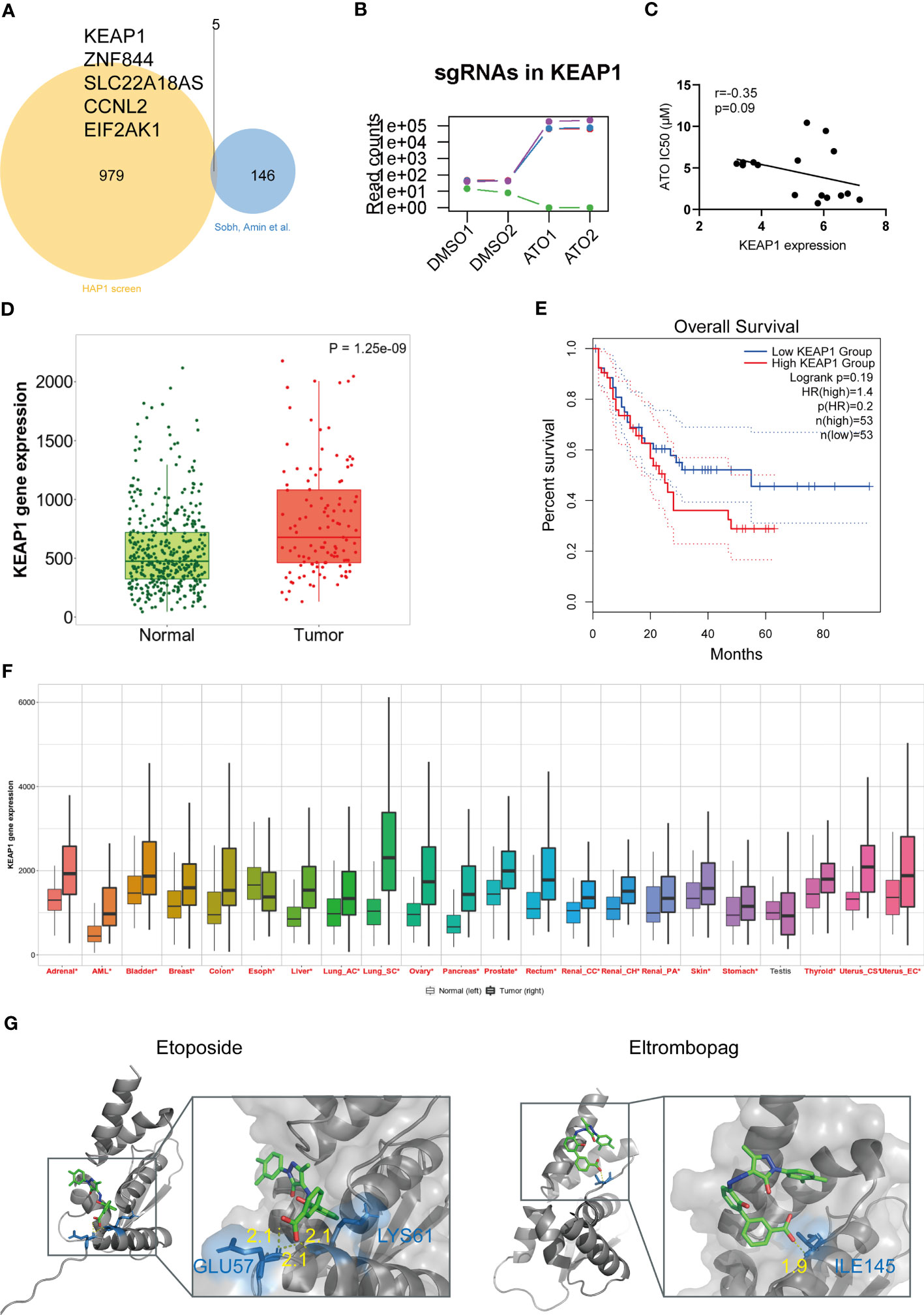
Figure 4 KEAP1 was a vital target of ATO with clinical significance in pan-cancer. (A) The Venn diagram of overlapping genes between two studies plotted by ImageGP. (B) The read counts of sgRNAs targeting KEAP1 in screening. (C) The correlation analysis of KEAP1 gene expression and sensitivity to ATO. Gene expression showed the log2 (FPKM + 1) values. The correlation analyses were performed using Pearson correlation. (D) The box plot derived from KEAP1 expressions data for acute leukemias of ambiguous lineage (ALAL) compared with normal samples from non-cancerous pediatric tissues. The plot was generated by TNMplot. Significant differences were tested by the Mann-Whitney U test. (E) The Kaplan–Meier survival plot comparing overall survival (OS) with high and low KEAP1 expression in AML patients generated using GEPIA2. Significance was tested by log-rank test. (F) The pan-cancer gene expression profiles of KEAP1 generated by TNMplot. Significant differences (P < 0.05) by the Mann-Whitney U test were marked with red*. (G) The docking poses of two drugs. The yellow dot lines indicate hydrogen bonds. The blue sticks represent residues.
We then investigated the clinical prognosis effect of KEAP1. Given that ATO was primarily used in hematological malignance instead of another disease, we first assessed the KEAP1 expressions in leukemia patients from the TARGET database, which contained genetic characteristics and clinical information of pediatric acute ALL patients. We found that the KEAP1 expression was higher in acute leukemias of ambiguous lineage (ALAL) patients compared with normal (p < 0.05) (Figure 4D). However, the expression did not affect the overall survival (OS) or disease-free survival (DFS) (data not shown). In addition, no expression difference was shown in other types of leukemia from TARGET (data not shown). Then, we tested the effect on AML from the TCGA database utilizing GEPIA2. The high expression of KEAP1 predicted poor OS in AML patients (Figure 4E). Finally, we examined the expression in pan-cancer from TCGA and found that all the tumors except for the testis were of higher KEAP1 expression compared with normal tissues (Figure 4F). These results suggested that ATO may be a potential therapy in treating pan-cancer through a mechanism involving KEAP1.
Considering that anticancer therapy was usually performed in combination, we were interested in what drugs may interact with KEAP1 and be a potential sensitizer or inhibitor when combined with ATO. To uncover these drug-gene interactions, we performed virtual screening for KEAP1 and screened for 2,506 FDA-approved drugs from DRUGBANK. The drugs with the highest affinity to the domain of KEAP1 were listed (Table 1). Among these drugs, we were particularly interested in etoposide and eltrombopag. Etoposide (VP-16) is a topoisomerase II inhibitor and an essential chemotherapeutic agent. It is the frontline drug used against several types of cancer, including pediatric ALL (94) and AML (95). Moreover, eltrombopag is a thrombopoietin (TPO) receptor agonist for the treatment of aplastic anemia (96) and chemotherapy-induced thrombocytopenic patients (97, 98). Therefore, these drugs may interfere with the therapeutic effect of ATO during cancer treatment. The docking poses of these two drugs with the KEAP1 BTB domain were shown in Figure 4G. These results indicated a potential interaction with ATO through KEAP1.
4 Discussion
Our work uncovered a vast landscape on which genetic perturbations attributed to the sensitivity to ATO. ATO attenuates the induction of ROS (99). The ability of ATO to promote ROS formation and ER-mitochondria cross-response has been confirmed by a series of experimental evidence in normal cells or cancer cells (100, 101), eventually leading to apoptosis or autophagy. KEAP1, the top-ranked gene in this study, was a key regulator in response to oxidative stress. It interacts with NRF2 and dissociates after sensing redox, leading to the transportation of NRF2 from the cytoplasm to the nucleus. This reaction results in a cascade of events against the redox stress. Our result suggested that KEAP1 is the most vital regulator in response to ATO instead of other genes involved in oxidative reactions. The loss-of-function of KEAP1 causes robust transportation of NRF2 and activates the antioxidant reactions, defending cells against ATO. Previous studies have also clarified that the NRF2-associated activation and downstream GSH biosynthesis accelerated arsenic efflux and ameliorated the cytotoxicity of ATO (83). The exact underlying mechanism remained to be explored. As indicated in this study, KEAP1 was differently expressed in tumors and related to the prognosis of certain types of cancers. It seems that ATO can be potentially applied to other cancers as a candidate therapy.
The other enriched pathways in this study were consistent with the current understanding of sensitivity and resistance to ATO. The metabolic process was enriched in GO and pathway analysis. ATO has been identified with an inhibitory effect on the glycolytic pathway (102). Lower glucose uptake and the distinct metabolical pattern were recognized in ATO-resistant cell lines (33, 103). Abu Bakar Noraini et al. found that ATO altered lipids metabolites, particularly arachidonic acid and docosahexaenoic acid (104). We also linked chemokines and cytokines productions with ATO. The chemokine-induced differentiation syndrome is one of the most common causes of death in APL patients, formerly known as a retinoic acid syndrome (105). Nevertheless, further evidence showed that ATO could induce chemokine production as a single agent (106). Considering that ATO-induced apoptosis is mediated by PI3K/Akt signaling pathway (107–109), which was also identified in our study, the involvement of ATO in regulating chemokine and cytokine may be of wide spectrum and related to chemoattraction and inflammation. Another vital pathway enriched in this study was the immune system. We performed enrichment analysis in three different databases. REACTOME and PANTHER enriched the immune system pathway, and we identified interferon-gamma and CCKR as immune-related signaling pathways. Fc epsilon RI signaling and Fc gamma R−mediated phagocytosis were enriched by KEGG as well. The cross talk between ATO and the immune system has not been clarified yet. Srivastava, Ritesh K et al. reported that ATO regulated macrophage innate immune function via unfolded protein response (UPR) signaling and activating transcription factor 4 (ATF4) (110, 111). ATO also exerts its efficacy on regulatory T cells (112–114). Chen Jinfeng et al. recently demonstrated that ATO was highly immunogenic and increased antigenicity and adjuvanticity after preconditioning ex vivo (115). These corroborated observations shed light on the correlation between ATO and cancer immunity and a promising therapeutic agent for autoimmune diseases (116).
The haploid cells that we used as cell models in this study, HAP1 cells, suit the aim of genetics research. The HAP1 cell line contains only one copy of the genome and shows the unmasked phenotype of different variants. In addition, it has a rapid doubling time and is sensitive to transfection, making it a handy and revolutionary model for gene editing. It has been applied in screens on resistance to a spectrum of anticancer drugs (117–120). The screens in HAP1 cells draw drug-specific conclusions instead of a cell type–specific character, making the interpretation easier in pan-caner. As mentioned, Amin Sobh et al. screened ATO using a different sgRNA library and CML cell lines (93). Although both studies identified that the disruption of KEAP1 markedly increased ATO tolerance, the genes involved in selenocysteine metabolism were recognized and confirmed to be resistant in their study but not ours. These differences may partly be due to the different cell lines and thresholds that we employed.
Our research showed that CRISPR-based functional genomics screening could be utilized to understand the molecular processes influencing sensitivity to ATO. The results may have implications for using ATO in chemotherapy in situations other than treating APL. However, further studies are needed to validate the relevance of this study, especially in cell-specific contexts.
Data availability statement
The datasets presented in this study can be found in online repositories. The names of the repository/repositories and accession number(s) can be found below: https://www.ncbi.nlm.nih.gov/geo/,GSE218982.
Author contributions
J-ZC and Y-LT designed the study. J-ZC performed the experiments. L-NW and X-QL contributed to the study design and scientific discussions. J-ZC drafted the manuscript. X-QL and Y-LT revised the manuscript critically. All authors contributed to the article and approved the submitted version.
Funding
This work was funded by the Science and Technology Planning Project of Guangdong Province, China (Grant No.2021A0505060004), the Basic and Applied Basic Research Project of Guangdong Province, China (Grant No. 2021A1515111169), the Youth Talent Promotion Project of Guangzhou Association for Science and Technology, China (Grant No.X20210201015), the Science and Technology Planning Project of Guangzhou, China (Grant No. 202102020156), and the Grant Award from the Terry Fox Foundation, Canada.
Acknowledgments
Bei Wang, Yu Xu, and Professor Qiao-Ping Wang from the Lab of Metabolism and Aging, School of Pharmaceutical Sciences (Shenzhen), Sun Yat-sen University aided the efforts of the authors.
Conflict of interest
The authors declare that the research was conducted in the absence of any commercial or financial relationships that could be construed as a potential conflict of interest.
Publisher’s note
All claims expressed in this article are solely those of the authors and do not necessarily represent those of their affiliated organizations, or those of the publisher, the editors and the reviewers. Any product that may be evaluated in this article, or claim that may be made by its manufacturer, is not guaranteed or endorsed by the publisher.
Supplementary material
The Supplementary Material for this article can be found online at: https://www.frontiersin.org/articles/10.3389/fonc.2023.1178686/full#supplementary-material
References
1. Noguera NI, Catalano G, Banella C, Divona M, Faraoni I, Ottone T, et al. Acute promyelocytic leukemia: update on the mechanisms of leukemogenesis, resistance and on innovative treatment strategies. Cancers (2019) 11(10):1591. doi: 10.3390/cancers11101591
2. Chen GQ, Zhu J, Shi XG, Ni JH, Zhong HJ, Si GY, et al. In vitro studies on cellular and molecular mechanisms of arsenic trioxide (As2O3) in the treatment of acute promyelocytic leukemia: As2O3 induces NB4 cell apoptosis with downregulation of bcl-2 expression and modulation of PML-RAR alpha/PML proteins. Blood (1996) 88(3):1052–61. doi: 10.1182/blood.V88.3.1052.1052
3. Isakson P, Bjørås M, Bøe SO, Simonsen A. Autophagy contributes to therapy-induced degradation of the PML/RARA oncoprotein. Blood (2010) 116(13):2324–31. doi: 10.1182/blood-2010-01-261040
4. Zhang SP, Niu YN, Yuan N, Zhang AH, Chao D, Xu QP, et al. Role of autophagy in acute myeloid leukemia therapy. Chin J cancer. (2013) 32(3):130–5. doi: 10.5732/cjc.012.10073
5. Moosavi MA, Djavaheri-Mergny M. Autophagy: new insights into mechanisms of action and resistance of treatment in acute promyelocytic leukemia. Int J Mol Sci (2019) 20(14):3559. doi: 10.3390/ijms20143559
6. Yousefnia S. Mechanistic effects of arsenic trioxide on acute promyelocytic leukemia and other types of leukemias. Cell Biol Int (2021) 45(6):1148–57. doi: 10.1002/cbin.11563
7. Liang C, Peng CJ, Wang LN, Li Y, Zheng LM, Fan Z, et al. Arsenic trioxide and all-trans retinoic acid suppress the expression of FLT3-ITD. Leukemia lymphoma. (2020) 61(11):2692–9. doi: 10.1080/10428194.2020.1775212
8. Wang LN, Tang YL, Zhang YC, Zhang ZH, Liu XJ, Ke ZY, et al. Arsenic trioxide and all-trans-retinoic acid selectively exert synergistic cytotoxicity against FLT3-ITD AML cells via co-inhibition of FLT3 signaling pathways. Leukemia lymphoma. (2017) 58(10):2426–38. doi: 10.1080/10428194.2017.1289522
9. Liu XJ, Wang LN, Zhang ZH, Liang C, Li Y, Luo JS, et al. Arsenic trioxide induces autophagic degradation of the FLT3-ITD mutated protein in FLT3-ITD acute myeloid leukemia cells. J Cancer. (2020) 11(12):3476–82. doi: 10.7150/jca.29751
10. Liu T, Sun L, Zhang Y, Wang Y, Zheng J. Imbalanced GSH/ROS and sequential cell death. J Biochem Mol toxicology. (2022) 36(1):e22942. doi: 10.1002/jbt.22942
11. Chen S, Wu JL, Liang Y, Tang YG, Song HX, Wu LL, et al. Arsenic trioxide rescues structural p53 mutations through a cryptic allosteric site. Cancer Cell (2021) 39(2):225–39.e8. doi: 10.1016/j.ccell.2020.11.013
12. Sanz MA, Lo-Coco F. Modern approaches to treating acute promyelocytic leukemia. J Clin oncology: Off J Am Soc Clin Oncol (2011) 29(5):495–503. doi: 10.1200/JCO.2010.32.1067
13. Burnett AK, Russell NH, Hills RK, Bowen D, Kell J, Knapper S, et al. Arsenic trioxide and all-trans retinoic acid treatment for acute promyelocytic leukaemia in all risk groups (AML17): results of a randomised, controlled, phase 3 trial. Lancet Oncol (2015) 16(13):1295–305. doi: 10.1016/S1470-2045(15)00193-X
14. Zhu HH, Wu DP, Du X, Zhang X, Liu L, Ma J, et al. Oral arsenic plus retinoic acid versus intravenous arsenic plus retinoic acid for non-high-risk acute promyelocytic leukaemia: a non-inferiority, randomised phase 3 trial. Lancet Oncol (2018) 19(7):871–9. doi: 10.1016/S1470-2045(18)30295-X
15. Iland HJ, Collins M, Bradstock K, Supple SG, Catalano A, Hertzberg M, et al. Use of arsenic trioxide in remission induction and consolidation therapy for acute promyelocytic leukaemia in the Australasian leukaemia and lymphoma group (ALLG) APML4 study: a non-randomised phase 2 trial. Lancet Haematology. (2015) 2(9):e357–66. doi: 10.1016/S2352-3026(15)00115-5
16. Wang HY, Gong S, Li GH, Yao YZ, Zheng YS, Lu XH, et al. An effective and chemotherapy-free strategy of all-trans retinoic acid and arsenic trioxide for acute promyelocytic leukemia in all risk groups (APL15 trial). Blood Cancer J (2022) 12(11):158. doi: 10.1038/s41408-022-00753-y
17. Ma YF, Lu Y, Wu Q, Lou YJ, Yang M, Xu JY, et al. Oral arsenic and retinoic acid for high-risk acute promyelocytic leukemia. J Hematol Oncol (2022) 15(1):148. doi: 10.1186/s13045-022-01368-3
18. Zheng H, Jiang H, Hu S, Liao N, Shen D, Tian X, et al. Arsenic combined with all-trans retinoic acid for pediatric acute promyelocytic leukemia: report from the CCLG-APL2016 protocol study. J Clin oncology: Off J Am Soc Clin Oncol (2021) 39(28):3161–70. doi: 10.1200/JCO.20.03096
19. Yang MH, Wan WQ, Luo JS, Zheng MC, Huang K, Yang LH, et al. Multicenter randomized trial of arsenic trioxide and realgar-indigo naturalis formula in pediatric patients with acute promyelocytic leukemia: interim results of the SCCLG-APL clinical study. Am J hematology. (2018) 93(12):1467–73. doi: 10.1002/ajh.25271
20. Vuky J, Yu R, Schwartz L, Motzer RJ. Phase II trial of arsenic trioxide in patients with metastatic renal cell carcinoma. Investigational New Drugs (2002) 20(3):327–30. doi: 10.1023/A:1016270206374
21. Dilda PJ, Hogg PJ. Arsenical-based cancer drugs. Cancer Treat Rev (2007) 33(6):542–64. doi: 10.1016/j.ctrv.2007.05.001
22. Subbarayan PR, Ardalan B. In the war against solid tumors arsenic trioxide need partners. J Gastrointestinal Cancer. (2014) 45(3):363–71. doi: 10.1007/s12029-014-9617-8
23. Hollow SE, Johnstone TC. Realgar and arsenene nanomaterials as arsenic-based anticancer agents. Curr Opin Chem Biol (2022) 72:102229. doi: 10.1016/j.cbpa.2022.102229
24. Sönksen M, Kerl K, Bunzen H. Current status and future prospects of nanomedicine for arsenic trioxide delivery to solid tumors. Medicinal Res Rev (2022) 42(1):374–98. doi: 10.1002/med.21844
25. Wang C, Zhang W, He Y, Gao Z, Liu L, Yu S, et al. Ferritin-based targeted delivery of arsenic to diverse leukaemia types confers strong anti-leukaemia therapeutic effects. Nat nanotechnology. (2021) 16(12):1413–23. doi: 10.1038/s41565-021-00980-7
26. Sui M, Zhang Z, Zhou J. Inhibition factors of arsenic trioxide therapeutic effects in patients with acute promyelocytic leukemia. Chin Med J (2014) 127(19):3503–6. doi: 10.14348/molcells.2020.2234
27. Maimaitiyiming Y, Wang QQ, Yang C, Ogra Y, Lou Y, Smith CA, et al. Hyperthermia selectively destabilizes oncogenic fusion proteins. Blood Cancer discovery. (2021) 2(4):388–401. doi: 10.1158/2643-3230.BCD-20-0188
28. Dai B, Wang F, Wang Y, Zhu J, Li Y, Zhang T, et al. Targeting HDAC3 to overcome the resistance to ATRA or arsenic in acute promyelocytic leukemia through ubiquitination and degradation of PML-RARα. Cell Death differentiation (2023) 30(5):1320–1333. doi: 10.1038/s41418-023-01139-8
29. Maimaitiyiming Y, Wang QQ, Hsu CH, Naranmandura H. Arsenic induced epigenetic changes and relevance to treatment of acute promyelocytic leukemia and beyond. Toxicol Appl Pharmacol (2020) 406:115212. doi: 10.1016/j.taap.2020.115212
30. Mesbahi Y, Zekri A, Ahmadian S, Alimoghaddam K, Ghavamzadeh A, Ghaffari SH. Targeting of EGFR increase anticancer effects of arsenic trioxide: promising treatment for glioblastoma multiform. Eur J Pharmacol (2018) 820:274–85. doi: 10.1016/j.ejphar.2017.12.041
31. Davison K, Côté S, Mader S, Miller WH. Glutathione depletion overcomes resistance to arsenic trioxide in arsenic-resistant cell lines. Leukemia (2003) 17(5):931–40. doi: 10.1038/sj.leu.2402876
32. Mun YC, Ahn JY, Yoo ES, Lee KE, Nam EM, Huh J, et al. Peroxiredoxin 3 has important roles on arsenic trioxide induced apoptosis in human acute promyelocytic leukemia cell line via hyperoxidation of mitochondrial specific reactive oxygen species. Molecules Cells (2020) 43(9):813–20. doi: 10.14348/molcells.2020.2234
33. Balasundaram N, Ganesan S, Palani HK, Alex AA, David S, Korula A, et al. Metabolic rewiring drives resistance to arsenic trioxide in acute promyelocytic leukemia. Blood (2016) 128(22):3956–. doi: 10.1182/blood.V128.22.3956.3956
34. Kurata M, Rathe SK, Bailey NJ, Aumann NK, Jones JM, Veldhuijzen GW, et al. Using genome-wide CRISPR library screening with library resistant DCK to find new sources of ara-c drug resistance in AML. Sci Rep (2016) 6:36199. doi: 10.1038/srep36199
35. Nguyen NH, Rafiee R, Tagmount A, Sobh A, Loguinov A, de Jesus Sosa AK, et al. Genome-wide CRISPR/Cas9 screen identifies etoposide response modulators associated with outcomes in pediatric AML. Blood Adv (2022) 7(9):1769–1783. doi: 10.1182/blood-2022-159322
36. Sievers QL, Gasser JA, Cowley GS, Fischer ES, Ebert BL. Genome-wide screen identifies cullin-RING ligase machinery required for lenalidomide-dependent CRL4(CRBN) activity. Blood (2018) 132(12):1293–303. doi: 10.1182/blood-2018-01-821769
37. Xu D, Liang SQ, Yang H, Bruggmann R, Berezowska S, Yang Z, et al. CRISPR screening identifies WEE1 as a combination target for standard chemotherapy in malignant pleural mesothelioma. Mol Cancer Ther (2020) 19(2):661–72. doi: 10.1158/1535-7163.MCT-19-0724
38. Shalem O, Sanjana NE, Hartenian E, Shi X, Scott DA, Mikkelson T, et al. Genome-scale CRISPR-Cas9 knockout screening in human cells. Sci (New York NY). (2014) 343(6166):84–7. doi: 10.1126/science.1247005
39. Sanjana NE, Shalem O, Zhang F. Improved vectors and genome-wide libraries for CRISPR screening. Nat Methods (2014) 11(8):783–4. doi: 10.1038/nmeth.3047
40. Doench JG, Fusi N, Sullender M, Hegde M, Vaimberg EW, Donovan KF, et al. Optimized sgRNA design to maximize activity and minimize off-target effects of CRISPR-Cas9. Nat Biotechnol (2016) 34(2):184–91. doi: 10.1038/nbt.3437
41. Jiang C, Qian M, Gocho Y, Yang W, Du G, Shen S, et al. Genome-wide CRISPR/Cas9 screening identifies determinant of panobinostat sensitivity in acute lymphoblastic leukemia. Blood advances. (2022) 6(8):2496–509. doi: 10.1182/bloodadvances.2021006152
42. Chen A, Wen S, Liu F, Zhang Z, Liu M, Wu Y, et al. CRISPR/Cas9 screening identifies a kinetochore-microtubule dependent mechanism for aurora-a inhibitor resistance in breast cancer. Cancer Commun (London England). (2021) 41(2):121–39. doi: 10.1002/cac2.12125
43. Wang C, Wang G, Feng X, Shepherd P, Zhang J, Tang M, et al. Genome-wide CRISPR screens reveal synthetic lethality of RNASEH2 deficiency and ATR inhibition. Oncogene (2019) 38(14):2451–63. doi: 10.1038/s41388-018-0606-4
44. Fang P, De Souza C, Minn K, Chien J. Genome-scale CRISPR knockout screen identifies TIGAR as a modifier of PARP inhibitor sensitivity. Commun Biol (2019) 2:335. doi: 10.1038/s42003-019-0580-6
45. Zimmermann M, Murina O, Reijns MAM, Agathanggelou A, Challis R, Tarnauskaitė Ž, et al. CRISPR screens identify genomic ribonucleotides as a source of PARP-trapping lesions. Nature (2018) 559(7713):285–9. doi: 10.1038/s41586-018-0291-z
46. Clements KE, Schleicher EM, Thakar T, Hale A, Dhoonmoon A, Tolman NJ, et al. Identification of regulators of poly-ADP-ribose polymerase inhibitor response through complementary CRISPR knockout and activation screens. Nat Commun (2020) 11(1):6118. doi: 10.1038/s41467-020-19961-w
47. Šuštić T, van Wageningen S, Bosdriesz E, Reid RJD, Dittmar J, Lieftink C, et al. A role for the unfolded protein response stress sensor ERN1 in regulating the response to MEK inhibitors in KRAS mutant colon cancers. Genome Med (2018) 10(1):90. doi: 10.1186/s13073-018-0600-z
48. Yamamoto M, Kensler TW, Motohashi H. The KEAP1-NRF2 system: a thiol-based sensor-effector apparatus for maintaining redox homeostasis. Physiol Rev (2018) 98(3):1169–203. doi: 10.1152/physrev.00023.2017
49. Singh A, Venkannagari S, Oh KH, Zhang YQ, Rohde JM, Liu L, et al. Small molecule inhibitor of NRF2 selectively intervenes therapeutic resistance in KEAP1-deficient NSCLC tumors. ACS Chem Biol (2016) 11(11):3214–25. doi: 10.1021/acschembio.6b00651
50. Binkley MS, Jeon YJ, Nesselbush M, Moding EJ, Nabet BY, Almanza D, et al. KEAP1/NFE2L2 mutations predict lung cancer radiation resistance that can be targeted by glutaminase inhibition. Cancer discovery. (2020) 10(12):1826–41. doi: 10.1158/2159-8290.CD-20-0282
51. Zhang Q, Zhang ZY, Du H, Li SZ, Tu R, Jia YF, et al. DUB3 deubiquitinates and stabilizes NRF2 in chemotherapy resistance of colorectal cancer. Cell Death differentiation. (2019) 26(11):2300–13. doi: 10.1038/s41418-019-0303-z
52. Shibata T, Kokubu A, Saito S, Narisawa-Saito M, Sasaki H, Aoyagi K, et al. NRF2 mutation confers malignant potential and resistance to chemoradiation therapy in advanced esophageal squamous cancer. Neoplasia (New York NY). (2011) 13(9):864–73. doi: 10.1593/neo.11750
53. Hong YB, Kang HJ, Kwon SY, Kim HJ, Kwon KY, Cho CH, et al. Nuclear factor (erythroid-derived 2)-like 2 regulates drug resistance in pancreatic cancer cells. Pancreas (2010) 39(4):463–72. doi: 10.1097/MPA.0b013e3181c31314
54. Marullo R, Werner E, Degtyareva N, Moore B, Altavilla G, Ramalingam SS, et al. Cisplatin induces a mitochondrial-ROS response that contributes to cytotoxicity depending on mitochondrial redox status and bioenergetic functions. PloS One (2013) 8(11):e81162. doi: 10.1371/journal.pone.0081162
55. Ishikawa T, Ali-Osman F. Glutathione-associated cis-diamminedichloroplatinum(II) metabolism and ATP-dependent efflux from leukemia cells. molecular characterization of glutathione-platinum complex and its biological significance. J Biol Chem (1993) 268(27):20116–25. doi: 10.1016/S0021-9258(20)80702-9
56. Joung J, Konermann S, Gootenberg JS, Abudayyeh OO, Platt RJ, Brigham MD, et al. Genome-scale CRISPR-Cas9 knockout and transcriptional activation screening. Nat Protoc (2017) 12(4):828–63. doi: 10.1038/nprot.2017.016
57. Liao Y, Wang J, Jaehnig EJ, Shi Z, Zhang B. WebGestalt 2019: gene set analysis toolkit with revamped UIs and APIs. Nucleic Acids Res (2019) 47(W1):W199–w205. doi: 10.1093/nar/gkz401
58. Wang J, Vasaikar S, Shi Z, Greer M, Zhang B. WebGestalt 2017: a more comprehensive, powerful, flexible and interactive gene set enrichment analysis toolkit. Nucleic Acids Res (2017) 45(W1):W130–w7. doi: 10.1093/nar/gkx356
59. Wang J, Duncan D, Shi Z, Zhang B. WEB-based GEne SeT AnaLysis toolkit (WebGestalt): update 2013. Nucleic Acids Res (2013) 41(Web Server issue):W77–83. doi: 10.1093/nar/gkt439
60. Zhang B, Kirov S, Snoddy J. WebGestalt: an integrated system for exploring gene sets in various biological contexts. Nucleic Acids Res (2005) 33(Web Server issue):W741–8. doi: 10.1093/nar/gki475
61. Bu D, Luo H, Huo P, Wang Z, Zhang S, He Z, et al. KOBAS-i: intelligent prioritization and exploratory visualization of biological functions for gene enrichment analysis. Nucleic Acids Res (2021) 49(W1):W317–w25. doi: 10.1093/nar/gkab447
62. Gillespie M, Jassal B, Stephan R, Milacic M, Rothfels K, Senff-Ribeiro A, et al. The reactome pathway knowledgebase 2022. Nucleic Acids Res (2021) 50(D1):D687–D92. doi: 10.1093/nar/gkab1028
63. Mi H, Muruganujan A, Thomas PD. PANTHER in 2013: modeling the evolution of gene function, and other gene attributes, in the context of phylogenetic trees. Nucleic Acids Res (2013) 41(Database issue):D377–86. doi: 10.1093/nar/gks1118
64. Thomas PD, Ebert D, Muruganujan A, Mushayahama T, Albou L-P, Mi H. PANTHER: making genome-scale phylogenetics accessible to all. Protein Sci (2022) 31(1):8–22. doi: 10.1002/pro.4218
65. Kanehisa M, Furumichi M, Sato Y, Kawashima M, Ishiguro-Watanabe M. KEGG for taxonomy-based analysis of pathways and genomes. Nucleic Acids Res (2022) 51(D1):D587–D592. doi: 10.1093/nar/gkac963
66. Szklarczyk D, Gable AL, Lyon D, Junge A, Wyder S, Huerta-Cepas J, et al. STRING v11: protein-protein association networks with increased coverage, supporting functional discovery in genome-wide experimental datasets. Nucleic Acids Res (2019) 47(D1):D607–d13. doi: 10.1093/nar/gky1131
67. Shannon P, Markiel A, Ozier O, Baliga NS, Wang JT, Ramage D, et al. Cytoscape: a software environment for integrated models of biomolecular interaction networks. Genome Res (2003) 13(11):2498–504. doi: 10.1101/gr.1239303
68. Bader GD, Hogue CWV. An automated method for finding molecular complexes in large protein interaction networks. BMC Bioinf (2003) 4(1):2. doi: 10.1186/1471-2105-4-2
69. Bindea G, Mlecnik B, Hackl H, Charoentong P, Tosolini M, Kirilovsky A, et al. ClueGO: a cytoscape plugin to decipher functionally grouped gene ontology and pathway annotation networks. Bioinf (Oxford England). (2009) 25(8):1091–3. doi: 10.1093/bioinformatics/btp101
70. Bindea G, Galon J, Mlecnik B. CluePedia cytoscape plugin: pathway insights using integrated experimental and in silico data. Bioinf (Oxford England). (2013) 29(5):661–3. doi: 10.1093/bioinformatics/btt019
71. Bartha Á, Győrffy B. TNMplot.com: a web tool for the comparison of gene expression in normal, tumor and metastatic tissues. Int J Mol Sci (2021) 22(5):2622. doi: 10.3390/ijms22052622
72. Tang Z, Kang B, Li C, Chen T, Zhang Z. GEPIA2: an enhanced web server for large-scale expression profiling and interactive analysis. Nucleic Acids Res (2019) 47(W1):W556–w60. doi: 10.1093/nar/gkz430
73. Reinhold WC, Sunshine M, Liu H, Varma S, Kohn KW, Morris J, et al. CellMiner: a web-based suite of genomic and pharmacologic tools to explore transcript and drug patterns in the NCI-60 cell line set. Cancer Res (2012) 72(14):3499–511. doi: 10.1158/0008-5472.CAN-12-1370
74. Reinhold WC, Varma S, Sunshine M, Elloumi F, Ofori-Atta K, Lee S, et al. RNA Sequencing of the NCI-60: integration into CellMiner and CellMiner CDB. Cancer Res (2019) 79(13):3514–24. doi: 10.1158/0008-5472.CAN-18-2047
75. Ma B, Lucas B, Capacci A, Lin EY, Jones JH, Dechantsreiter M, et al. Design, synthesis and identification of novel, orally bioavailable non-covalent Nrf2 activators. Bioorganic medicinal Chem letters. (2020) 30(4):126852. doi: 10.1016/j.bmcl.2019.126852
77. Koes DR, Baumgartner MP, Camacho CJ. Lessons learned in empirical scoring with smina from the CSAR 2011 benchmarking exercise. J Chem Inf modeling. (2013) 53(8):1893–904. doi: 10.1021/ci300604z
78. Eberhardt J, Santos-Martins D, Tillack AF, Forli S. AutoDock vina 1.2.0: new docking methods, expanded force field, and Python bindings. J Chem Inf modeling (2021) 61(8):3891–8. doi: 10.1021/acs.jcim.1c00203
79. Trott O, Olson AJ. AutoDock vina: improving the speed and accuracy of docking with a new scoring function, efficient optimization, and multithreading. J Comput Chem (2010) 31(2):455–61. doi: 10.1002/jcc.21334
80. Durrant JD, McCammon JA. NNScore 2.0: a neural-network receptor-ligand scoring function. J Chem Inf modeling (2011) 51(11):2897–903. doi: 10.1021/ci2003889
81. Chen T, Liu Y-X, Huang L. ImageGP: an easy-to-use data visualization web server for scientific researchers. iMeta (2022) 1(1):. doi: 10.1002/imt2.5
82. Li W, Xu H, Xiao T, Cong L, Love MI, Zhang F, et al. MAGeCK enables robust identification of essential genes from genome-scale CRISPR/Cas9 knockout screens. Genome Biol (2014) 15(12):554. doi: 10.1186/s13059-014-0554-4
83. Nishimoto S, Suzuki T, Koike S, Yuan B, Takagi N, Ogasawara Y. Nrf2 activation ameliorates cytotoxic effects of arsenic trioxide in acute promyelocytic leukemia cells through increased glutathione levels and arsenic efflux from cells. Toxicol Appl Pharmacol (2016) 305:161–8. doi: 10.1016/j.taap.2016.06.017
84. Baird L, Kensler TW, Yamamoto M. Novel NRF2-activated cancer treatments utilizing synthetic lethality. IUBMB Life (2022) 74(12):1209–31. doi: 10.1002/iub.2680
85. Ye J, Lin Y, Gao X, Lu L, Huang X, Huang S, et al. Prognosis-related molecular subtypes and immune features associated with hepatocellular carcinoma. Cancers (2022) 14(22):5781. doi: 10.3390/cancers14225721
86. Jansen P, Müller H, Lodde GC, Zaremba A, Möller I, Sucker A, et al. GNA14, GNA11, and GNAQ mutations are frequent in benign but not malignant cutaneous vascular tumors. Front Genet (2021) 12:663272. doi: 10.3389/fgene.2021.663272
87. Nguyen TA, Lehr AW, Roche KW. Neuroligins and neurodevelopmental disorders: X-linked genetics. Front synaptic Neurosci (2020) 12:33. doi: 10.3389/fnsyn.2020.00033
88. Alon R, Chen S, Puri KD, Finger EB, Springer TA. The kinetics of l-selectin tethers and the mechanics of selectin-mediated rolling. J Cell Biol (1997) 138(5):1169–80. doi: 10.1083/jcb.138.5.1169
89. Segura J, He B, Ireland J, Zou Z, Shen T, Roth G, et al. The role of l-selectin in HIV infection. Front Microbiol (2021) 12:725741. doi: 10.3389/fmicb.2021.725741
90. Watany MM, Abdou S, Elkolaly R, Elgharbawy N, Hodeib H. Evaluation of admission levels of p, e and l selectins as predictors for thrombosis in hospitalized COVID-19 patients. Clin Exp Med (2022) 22(4):567–75. doi: 10.1007/s10238-021-00787-9
91. Bennett TA, Edwards BS, Sklar LA, Rogelj S. Sulfhydryl regulation of l-selectin shedding: phenylarsine oxide promotes activation-independent l-selectin shedding from leukocytes. J Immunol (Baltimore Md: 1950). (2000) 164(8):4120–9. doi: 10.4049/jimmunol.164.8.4120
92. Huang Y, Yang X, Lu Y, Zhao Y, Meng R, Zhang S, et al. UBE2O targets Mxi1 for ubiquitination and degradation to promote lung cancer progression and radioresistance. Cell Death differentiation. (2021) 28(2):671–84. doi: 10.1038/s41418-020-00616-8
93. Sobh A, Loguinov A, Yazici GN, Zeidan RS, Tagmount A, Hejazi NS, et al. Functional profiling identifies determinants of arsenic trioxide cellular toxicity. Toxicological sciences: an Off J Soc Toxicology. (2019) 169(1):108–21. doi: 10.1093/toxsci/kfz024
94. Stutterheim J, Sluis I, Lorenzo Pd, Alten J, Ancliffe P, Attarbaschi A, et al. Clinical implications of minimal residual disease detection in infants with KMT2A-rearranged acute lymphoblastic leukemia treated on the interfant-06 protocol. J Clin Oncol (2021) 39(6):652–62. doi: 10.1200/JCO.20.02333
95. Elsayed AH, Cao X, Mitra AK, Wu H, Raimondi S, Cogle C, et al. Polygenic ara-c response score identifies pediatric patients with acute myeloid leukemia in need of chemotherapy augmentation. J Clin Oncol (2022) 40(7):772–83. doi: 10.1200/JCO.21.01422
96. Peffault de Latour R, Kulasekararaj A, Iacobelli S, Terwel SR, Cook R, Griffin M, et al. Eltrombopag added to immunosuppression in severe aplastic anemia. New Engl J Med (2022) 386(1):11–23. doi: 10.1056/NEJMoa2109965
97. Mittelman M, Platzbecker U, Afanasyev B, Grosicki S, Wong RSM, Anagnostopoulos A, et al. Eltrombopag for advanced myelodysplastic syndromes or acute myeloid leukaemia and severe thrombocytopenia (ASPIRE): a randomised, placebo-controlled, phase 2 trial. Lancet Haematology. (2018) 5(1):e34–43. doi: 10.1016/S2352-3026(17)30228-4
98. Zhu Q, Yang S, Zeng W, Li M, Guan Z, Zhou L, et al. A real-world observation of eltrombopag and recombinant human thrombopoietin (rhTPO) in lymphoma patients with chemotherapy induced thrombocytopenia. Front Oncol (2021) 11:701539. doi: 10.3389/fonc.2021.701539
99. Hoang DH, Buettner R, Valerio M, Ghoda L, Zhang B, Kuo YH, et al. Arsenic trioxide and venetoclax synergize against AML progenitors by ROS induction and inhibition of Nrf2 activation. Int J Mol Sci (2022) 23(12):6568. doi: 10.3390/ijms23126568
100. Cantoni O, Zito E, Guidarelli A, Fiorani M, Ghezzi P. Mitochondrial ROS, ER stress, and Nrf2 crosstalk in the regulation of mitochondrial apoptosis induced by arsenite. Antioxidants (Basel Switzerland) (2022) 11(5):1034. doi: 10.3390/antiox11051034
101. Masciarelli S, Capuano E, Ottone T, Divona M, De Panfilis S, Banella C, et al. Retinoic acid and arsenic trioxide sensitize acute promyelocytic leukemia cells to ER stress. Leukemia (2018) 32(2):285–94. doi: 10.1038/leu.2017.231
102. Zhang T, Lu H, Li W, Hu R, Chen Z. Identification of arsenic direct-binding proteins in acute promyelocytic leukaemia cells. Int J Mol Sci (2015) 16(11):26871–9. doi: 10.3390/ijms161125994
103. Balasundaram N, Ganesan S, Chendamarai E, Palani HK, Venkatraman A, Alex AA, et al. Metabolic adaptation drives arsenic trioxide resistance in acute promyelocytic leukemia. Blood advances. (2022) 6(2):652–63. doi: 10.1182/bloodadvances.2021005300
104. Abu Bakar N, Wan Ibrahim WN, Che Abdullah CA, Ramlan NF, Shaari K, Shohaimi S, et al. Embryonic arsenic exposure triggers long-term behavioral impairment with metabolite alterations in zebrafish. Toxics (2022) 10(9):493. doi: 10.3390/toxics10090493
105. de la Serna J, Montesinos P, Vellenga E, Rayón C, Parody R, León A, et al. Causes and prognostic factors of remission induction failure in patients with acute promyelocytic leukemia treated with all-trans retinoic acid and idarubicin. Blood (2008) 111(7):3395–402. doi: 10.1182/blood-2007-07-100669
106. Luesink M, Pennings JL, Wissink WM, Linssen PC, Muus P, Pfundt R, et al. Chemokine induction by all-trans retinoic acid and arsenic trioxide in acute promyelocytic leukemia: triggering the differentiation syndrome. Blood (2009) 114(27):5512–21. doi: 10.1182/blood-2009-02-204834
107. Bashash D, Delshad M, Riyahi N, Safaroghli-Azar A, Pourbagheri-Sigaroodi A, Momeny M. Inhibition of PI3K signaling pathway enhances the chemosensitivity of APL cells to ATO: proposing novel therapeutic potential for BKM120. Eur J Pharmacol (2018) 841:10–8. doi: 10.1016/j.ejphar.2018.10.007
108. Chen QY, Costa M. PI3K/Akt/mTOR signaling pathway and the biphasic effect of arsenic in carcinogenesis. Mol Pharmacol (2018) 94(1):784–92. doi: 10.1124/mol.118.112268
109. Li Y, Qu X, Qu J, Zhang Y, Liu J, Teng Y, et al. Arsenic trioxide induces apoptosis and G2/M phase arrest by inducing cbl to inhibit PI3K/Akt signaling and thereby regulate p53 activation. Cancer letters. (2009) 284(2):208–15. doi: 10.1016/j.canlet.2009.04.035
110. Srivastava RK, Li C, Chaudhary SC, Ballestas ME, Elmets CA, Robbins DJ, et al. Unfolded protein response (UPR) signaling regulates arsenic trioxide-mediated macrophage innate immune function disruption. Toxicol Appl Pharmacol (2013) 272(3):879–87. doi: 10.1016/j.taap.2013.08.004
111. Srivastava RK, Li C, Wang Y, Weng Z, Elmets CA, Harrod KS, et al. Activating transcription factor 4 underlies the pathogenesis of arsenic trioxide-mediated impairment of macrophage innate immune functions. Toxicol Appl Pharmacol (2016) 308:46–58. doi: 10.1016/j.taap.2016.07.015
112. Gao Q, Jiang J, Chu Z, Lin H, Zhou X, Liang X. Arsenic trioxide inhibits tumor-induced myeloid-derived suppressor cells and enhances T-cell activity. Oncol letters. (2017) 13(4):2141–50. doi: 10.3892/ol.2017.5679
113. Xu W, Li X, Quan L, Yao J, Mu G, Guo J, et al. Arsenic trioxide decreases the amount and inhibits the function of regulatory T cells, which may contribute to its efficacy in the treatment of acute promyelocytic leukemia. Leukemia lymphoma. (2018) 59(3):650–9. doi: 10.1080/10428194.2017.1346253
114. Wang L, Wang R, Fan L, Liang W, Liang K, Xu Y, et al. Arsenic trioxide is an immune adjuvant in liver cancer treatment. Mol Immunol (2017) 81:118–26. doi: 10.1016/j.molimm.2016.12.001
115. Chen J, Jin Z, Zhang S, Zhang X, Li P, Yang H, et al. Arsenic trioxide elicits prophylactic and therapeutic immune responses against solid tumors by inducing necroptosis and ferroptosis. Cell Mol Immunol (2022) 20(1):51–64. doi: 10.1038/s41423-022-00956-0
116. Bobé P, Bonardelle D, Benihoud K, Opolon P, Chelbi-Alix MK. Arsenic trioxide: a promising novel therapeutic agent for lymphoproliferative and autoimmune syndromes in MRL/lpr mice. Blood (2006) 108(13):3967–75. doi: 10.1182/blood-2006-04-020610
117. Kotecki M, Reddy PS, Cochran BH. Isolation and characterization of a near-haploid human cell line. Exp Cell Res (1999) 252(2):273–80. doi: 10.1006/excr.1999.4656
118. Carette JE, Guimaraes CP, Varadarajan M, Park AS, Wuethrich I, Godarova A, et al. Haploid genetic screens in human cells identify host factors used by pathogens. Sci (New York NY). (2009) 326(5957):1231–5. doi: 10.1126/science.1178955
119. Essletzbichler P, Konopka T, Santoro F, Chen D, Gapp BV, Kralovics R, et al. Megabase-scale deletion using CRISPR/Cas9 to generate a fully haploid human cell line. Genome Res (2014) 24(12):2059–65. doi: 10.1101/gr.177220.114
Keywords: arsenic trioxide, CRISPR-Cas9 screening, KEAP1, leukemia, virtual screening
Citation: Chen J-Z, Wang L-N, Luo X-Q and Tang Y-L (2023) The genomic landscape of sensitivity to arsenic trioxide uncovered by genome-wide CRISPR-Cas9 screening. Front. Oncol. 13:1178686. doi: 10.3389/fonc.2023.1178686
Received: 03 March 2023; Accepted: 24 April 2023;
Published: 12 May 2023.
Edited by:
Dongwen Lyu (Lv), The University of Texas Health Science Center at San Antonio, United StatesReviewed by:
Yufeng Xiao, University of Florida, United StatesYasen Maimaitiyiming, Zhejiang University, China
Copyright © 2023 Chen, Wang, Luo and Tang. This is an open-access article distributed under the terms of the Creative Commons Attribution License (CC BY). The use, distribution or reproduction in other forums is permitted, provided the original author(s) and the copyright owner(s) are credited and that the original publication in this journal is cited, in accordance with accepted academic practice. No use, distribution or reproduction is permitted which does not comply with these terms.
*Correspondence: Yan-Lai Tang, dGFuZ3lsYWlAbWFpbC5zeXN1LmVkdS5jbg==