- The Innovation Center, Beijing StoneWise Technology Co Ltd., Beijing, China
Tumor cells can result from gene mutations and over-expression. Synthetic lethality (SL) offers a desirable setting where cancer cells bearing one mutated gene of an SL gene pair can be specifically targeted by disrupting the function of the other genes, while leaving wide-type normal cells unharmed. Paralogs, a set of homologous genes that have diverged from each other as a consequence of gene duplication, make the concept of SL feasible as the loss of one gene does not affect the cell’s survival. Furthermore, homozygous loss of paralogs in tumor cells is more frequent than singletons, making them ideal SL targets. Although high-throughput CRISPR-Cas9 screenings have uncovered numerous paralog-based SL pairs, the unclear mechanisms of targeting these gene pairs and the difficulty in finding specific inhibitors that exclusively target a single but not both paralogs hinder further clinical development. Here, we review the potential mechanisms of paralog-based SL given their function and genetic combination, and discuss the challenge and application prospects of paralog-based SL in cancer therapeutic discovery.
1 Introduction
Gene duplication is a common phenomenon in cellular evolution, serving as a primary method for the creation of novel genes (1). Paralogs are genes that originate from gene duplication events (2). These duplicated genes can be retained through mechanisms such as “neo-functionalization”, “sub-functionalization”, “dosage amplification” and “back-up compensation,” or become non-functional through a process called “non-functionalization” (1). Despite various evolutionary patterns, many paralogs retain a degree of functional redundancy, which may lead to back-up compensation and confer genetic robustness and adaptive advantages (3–5). For example, it has been observed in both budding yeast and human cells that the loss of function of paralogs is less detrimental than that of singletons (3, 6). The compensation mechanism of paralogs shows greater phenotypic plasticity in various environmental stress conditions. The redundant copies can be free from the constraints of natural selection and obtain “forbidden mutations” that allow the development of new or more specialized functions (1). An example of this is the MSN2-MSN4 paralogs in yeast. Following a gene duplication event, the expression of MSN2 became highly stable and less responsive to environmental changes (low-noise-based expression), while the expression of MSN4 became more dynamic and had random variations (noise). This provided yeast with the opportunity to evolve phenotype-adaptive expression tuning (7).
Homozygous paralog loss occurs more frequently than singletons in cancer (8), suggesting that tumor cells that can tolerate gene loss were selected. However, this also makes the tumors vulnerable as only one of the paralogs is retained (8). Synthetic lethality (SL), a promising strategy for precision cancer medicine (9), uses drugs to target the genes with complementary functions in a tumor that has acquired a certain mutation. This approach results in the complete disruption of the targeted genes’ functions and a subsequent defect in tumor cell viability, while normal cells with the un-mutated gene remain unaffected (Figure 1). The concept of SL overcomes some limitations faced by traditional precision medicine such as a small number of targets and undruggable genes (10). Poly (ADP-ribose) polymerase (PARP) inhibitors developed based on the SL interaction between PARP and BRCA have provided substantial clinical benefit to patients (11–16), and demonstrated the feasibility of SL for precision oncology. The application of SL in precision cancer medicine is facilitated by the presence of many paralog buffer systems in cells and the specific mutations that occur in tumor cells. Therefore, the paralog vulnerability of tumor cells can be leveraged to specifically target the functionally compensating genes corresponding to the mutated paralog genes, thus achieving the goal of selectively eliminating tumor cells.
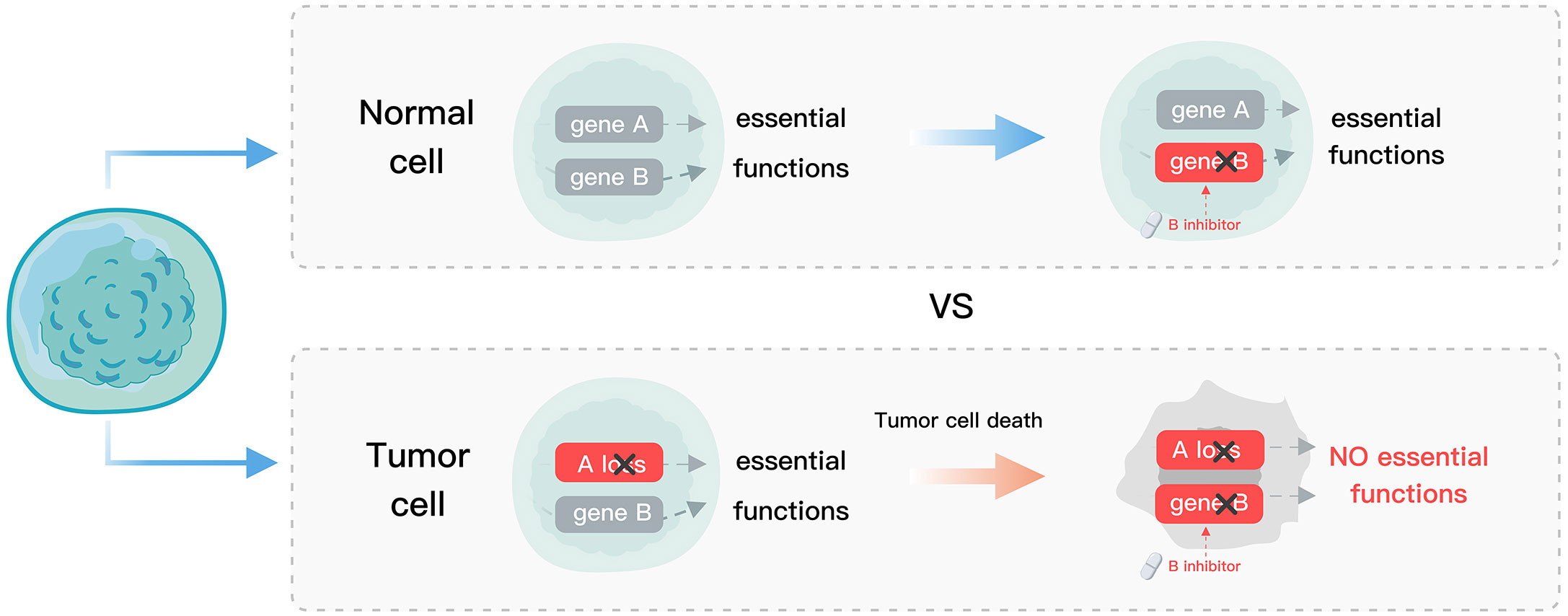
Figure 1 Schematic diagram of SL. Normal cells, devoid of gene A mutations, exhibit tolerance to gene B suppression, whereas tumor cells with gene A loss undergo gene B suppression, leading to cell death.
Approximately 70.5% of the 19,430 protein-coding genes in the human genome have one or more paralogs (17). Despite the extensive screening of over 700 cancer cell lines using genome-wide CRISPR-Cas9 and RNA interference (RNAi) libraries (DepMap portal, https://depmap.org/portal/), new targets related to the most common genetic drivers have not yet been discovered (18). This is likely contributed by the functional redundancies among paralogous genes, which can mask the dependencies to single-gene perturbation. Thus, paralogs with functional buffering are not only promising candidates for SL, but abnormalities in paralog members can also serve as crucial biomarkers for identifying context-specific SL targets. This review will systematically explore paralog-based SL interactions according to their various functional categories and practical applications. Additionally, we will propose a new paralog-based SL genetic combination and its underlying mechanism. Finally, we will discuss the challenges and prospects of paralog-based SL in drug discovery and development.
2 Functional categories of paralog-based SL
The effectiveness of paralog-based SL often relies on whether the complex and the pathway they belong to have essential functions (10). Mapping the functions of paralog-based SL pairs can serve as a reliable starting point for further research. Current confirmed essential cellular functions of SL pairs include gene expression regulation, the cell cycle, DNA damage repair, energy metabolism and material transport. We summarized in Table 1 paralog-based SL interactions that have been validated using low-throughput experiments.
2.1 Regulation of gene expression
Epigenetic factors play a crucial role in the regulation of transcription and expression of many fundamental genes, and have demonstrated potential as therapeutic targets in the field of SL. Mutations in genes encoding subunits of the SWI/SNF chromatin remodeling complex are commonly found in over 20% of known human cancers and are thought to promote tumorigenesis by disrupting transcriptional homeostasis (50, 51). The ARID1A-ARID1B, SMARCA2-SMARCA4, and SMARCC1-SMARCC2, subunits of the SWI/SNF complex, have been proven to have SL interactions (19, 20, 22, 43, 52). CREBBP-EP300 can increase the accessibility of gene transcription (21, 53). Co-deletion of them triggers SL in certain cancers (23–25). Histone deacetylases (HDACs) alter chromatin structures to modulate transcription levels of nearby genes and lead to the down-regulation of cell cycle regulators and tumor suppressors (54). Loss of HDAC2 produces SL effects in HDAC1 hemizygous deletion cells (26). In addition to epigenetic factors, the cohesin complex regulates gene expression by forming a DNA ring, and its members STAG1 and STAG2 have a strong SL interaction (27, 30, 55–57). The RNA-binding proteins RBM26 and RBM27 play a critical role in mRNA processing, and their simultaneous depletion leads to a synergistic reduction in cell viability (44, 58). Sterol regulatory element binding transcription factors SREBF1 and SREBF2 show a strong reciprocal SL interaction (45). RPP25 is a component of the Th/To complex that processes a variety of RNAs (59). Its low expression makes cells sensitive to RPP25L loss (42, 60). DDX5 and DDX17 are members of the DEAD box family, primarily involved in transcription and splicing processes. They have been identified as an SL gene pair through high-throughput screening (46). CSTF2 and CSTF2T are involved in the mRNA cleavage and polyadenylation (61). The knockout of CSTF2 deletes tumor cells with homozygous CSTF2T deletions (31, 42). The core members of the exon junction complex, MAGOH and MAGOHB, are essential for mRNA processing, and their combination knockdown results in lethality (32, 62).
2.2 Cell-cycle and DNA damage repair
PARP inhibitors are currently approved for treating advanced ovarian and breast cancers that are caused by mutations in the BRCA1/2 genes and are used as second-line therapy (63). Successfully leveraging the SL interaction between PARP and BRCA has led to a focus on identifying genes involved in the cell cycle and DNA damage repair as potential SL targets. It is worth noting that genes in this category tend to have multiple functions. For example, in addition to regulating gene expression, the cohesin complex also has a canonical cell-cycle-associated function (28). Co-inactivation of its subunits STAG1 and STAG2 can lead to loss of sister chromatid cohesion and cell death. Mutation in STAG2 also causes replication fork stall and collapse, making corresponding tumor cells more sensitive to certain chemotherapy and inhibitors targeting DNA double-strand break (DSB) repair genes (27, 57, 64). The nuclear serine-threonine kinase VRK1, which plays a role in regulating the cell cycle and DNA damage repair has been found to have an SL interaction with VRK2 (33, 34). Perhaps due to more buffer systems in the cell cycle and DNA repair regulation, the discovery of SL within paralog families in these processes is well below the expectation. However, more SL interactions between paralog members and other genes have been discovered and verified, such as TLK1/TLK2 and PARP (65), SMARCA4 and CDK4/6 (66). In addition, CDK1, CDK2, CDK12, and CDK17 all have been confirmed to have SL interaction with other genes (9, 67). Further insights into the cyclin-dependent kinases-based SL interactions can be obtained by consulting the review by Li et al. (68).
2.3 Energy metabolism and material transport
Tumorigenesis is heavily influenced by metabolic responses, as cancer cells have a high bioenergetic demand but also are restricted by limited nutrient availability in the tumor microenvironment (69). The loss of the gene encoding metabolic enzymes in cancer cells leads to a dependence on their paralog or redundant metabolic pathway, resulting in the SL phenomenon. For example, with the loss of enolase ENO1, cancer cells are abnormally sensitive to the repression of its redundant gene ENO2 (35, 70). Selective inhibition of ENO2 by either genetic or pharmacological means can inhibit proliferation and trigger apoptosis in ENO1-deficient glioma cells (71). Similarly, loss of mitochondrial malic enzymes ME3 leads to cell death in ME2-mutated pancreatic ductal adenocarcinoma cells (36). Depletion of phosphatidylserine synthase PTDSS1 specifically suppressed growth in PTDSS2-deficient cancer cell lines (47). L-lactate dehydrogenase LDHA and LDHB are essential for the Warburg effect. Inhibition of both LDHA and LDHB could be therapeutically effective (44, 72). Dual inhibition of lactate transporters SLC16A1 and SLC16A3 (44, 48), or combination of SLC16A1-SLC16A4 with metformin (73) leads to cancer cell death.
SL interactions also occur in the transport process, which is crucial for the transfer of energy and materials. For example, the VPS4A and VPS4B, ATPases of the endosomal sorting complex (ESCRT), a polyprotein complex that plays a vital role in reversing membrane remodeling, have been confirmed as an SL gene pair both in vitro and in vivo (37, 38, 42). Another transport paralog gene pair NXT1 and NXT2 regulates the export of mRNA from the nucleus and is known to have an SL interaction (39).
2.4 Other functions
This category includes functions with fewer reported SL interactions. For instance, the simultaneous inactivation of phosphatase DUSP4 and DUSP6 selectively impairs the growth of cells with mutations in NRAS or BRAF by hyperactivating MAPK signaling (18). BCL2L1 and BCL2L2 are a pair of SL genes that are apoptosis-related paralogs, initially identified through dual-gene knockout screening (74, 75). Inhibiting the ubiquitin UBC in tumors of UBB silencing led to tumor regression (40). DDX3X and DDX3Y are DEAD-box RNA helicase that regulates translation and exhibits SL interaction (42, 76). FAM50A and FAM50B, with an unclear function, also exhibit SL interaction (42, 77).
3 Clinical development of SL-targeted drugs
It was first shown in 2005 that SL genes can be a selective and effective target for precision cancer treatment. Ashworth and Helleday demonstrated that inhibition of PARP inhibitors selectively kills BRCA1/2-deficient tumor cells, revealing an SL interaction between PARP and BRAC1/2 (12, 13). More clinical and mechanistic details related to BRCA-deficient tumors can refer to the review article by Patel et al. (63). Since then, multiple PARP inhibitors including olaparib, niraparib, rucaparib, talazoparib have been approved for multiple cancers, and many other SL-targeted drugs have been tested in clinical trials. The SL partners of genes including TP53, KRAS, and MYC have been targeted (10). Inhibitors of ATR, WEE1, CHK1, and mTOR, the SL partners of tumor suppressor gene TP53, all showed efficacy in clinical development (10, 78, 79). There is even a large pool of SL genes in preclinical developments including p38MAPK/MK2, PLK1, PIP4K2B, HK2, PDGFR, and PLA2G16 (80). In addition, the SL partners of oncogene KRAS in clinical development are combinatorial targets such as TBK1-MEK, AKT-MEK, and CDK4-MEK (81), and those of MYC are ATR, AURKB, and CDK9 (9, 82).
Although a pair of paralog genes provide a highly straightforward SL interaction by virtue of their similar functions, it is worth noting that drug development from paralog-based SL is still scarce in clinical trials (ClinicalTrials.gov) and cancer therapies (83). The slow development of these paralog-specific inhibitors might be resulting from the difficulty to balance efficacy and toxicity in the complex environment of a tumor, which requires a highly specific inhibitor to spare the non-target paralog despite the nearly identical gene or protein sequence homologies (84). Similarly, the SL interaction between paralogs is seemingly hampered by the lack of suitably selective inhibitors. However, some promising solutions are under development. For example, protein crystal structures can reveal structural differences that may be exploited to confer paralog selectivity for small-molecule ligands (57). PROTACs (PROteolysis TArgeting Chimeras) are hetero-bifunctional chimeric molecules consisting of one protein-binding ligand, one E3 ubiquitin ligase recruiting moiety, and a linker to connect them. PROTACs can pull a targeted protein to an E3 ubiquitin ligase, leading to their ubiquitination and degradation (85). This approach has shown promise in discriminating between similar paralogous genes and offers some opportunities for therapeutic development. For example, the PROTAC degrader PP-C8 confers specificity for CDK12 over CDK13 (86). VHL-recruiting PROTAC ACBI2 selectively degrades SMARCA2 over SMARCA4 (87). Moreover, Antisense oligonucleotides (ASOs) are short nucleic acid fragments that uniquely hybridize with complementary pre-mRNAs or mRNAs to modulate their functions (88, 89), making them a powerful tool for selectively targeting paralog members. For instance, highly selective ASOs have been developed for HK2 inhibition (90) and TYK2 inhibition (91), without affecting the expression level of other paralogs. In addition, for paralog members such as DDX3Y, whose abnormal expression can be specifically recognized by immune cells in leukemic stem cells, immune treatment is a possibility (92). Although selective targeting paralog-based SL interaction for tumor cells has shown a nice clinical value, it is worth noting to consider the protein expression of its paralog in other normal tissues. For example, ENO1 is the only isoform expressed in red blood cells, so pan-enolase inhibition can lead to anemia (71). This suggests that determining the expression subtypes in normal tissues is imperative to prevent damage to certain normal cells.
Sex paralogs provide a new opportunity for male tumors. Some paralogous genes are located between sex chromosomes. The prevalent loss of chromosome Y in male cancer patients makes depletion of the chrX-encoded paralog attractive as a therapeutic strategy. For example, chrX- and chrY-encoded paralogs including DDX3X-DDX3Y, ZFX-ZFY, and EIF1AX-EIF1AY have been proven to buffer for each other’s loss and dependent on chrX-encoded paralog in cancers with loss of chrY (42). Accumulating evidence suggests that targeting the DDX3X-DDX3Y paralogs may be an effective treatment strategy (41, 42, 76, 93). Specifically, inhibiting DDX3X in male patients with loss of chromosome Y, or targeting DDX3Y in male tumors with co-occurring DDX3X and MYC mutations, could hold promise for eliminating cancerous cells. For example, male lymphomas rely on functional compensation by DDX3Y for rescue, and inhibitors blocking DDX3Y can eliminate cancerous B cells (41). Moreover, since DDX3Y protein is not expressed in normal adult cells, the toxicity of therapeutic DDX3Y inhibition would be low. Although some inhibitors have shown some anti-tumor effects, the development of a specific inhibitor is still challenging and requires a deeper understanding of underlying mechanisms.
4 Genetic combination and mechanism of paralog-based SL
Paralog-based SL can generally be divided into four types: 1) SL interactions within the paralog family (Figure 2A); 2) SL interactions between paralog members and other genes (Figure 2B); 3) SL interactions between a specific paralog member and other genes (Figure 2C); and 4) SL interactions between paralog members and other factors (Figure 2D). The first type typically involves paralog members that play essential roles in a pathway or complex, such as the VPS4A and VPS4B ATPases in ESCRT. The second type involves genes that may exhibit complementary roles such as the BRCA and PARP genes. The third type can be further divided into two categories, one where SL interaction is formed between a gene and its paralog upstream regulatory genes, and the other where it is between functionally non-overlapping parts of paralogs and a functionally compensating gene. The fourth type is similar to the second one, but the targets of drugs and the cellular injury are often not specific, so the relationship cannot be specifically determined.
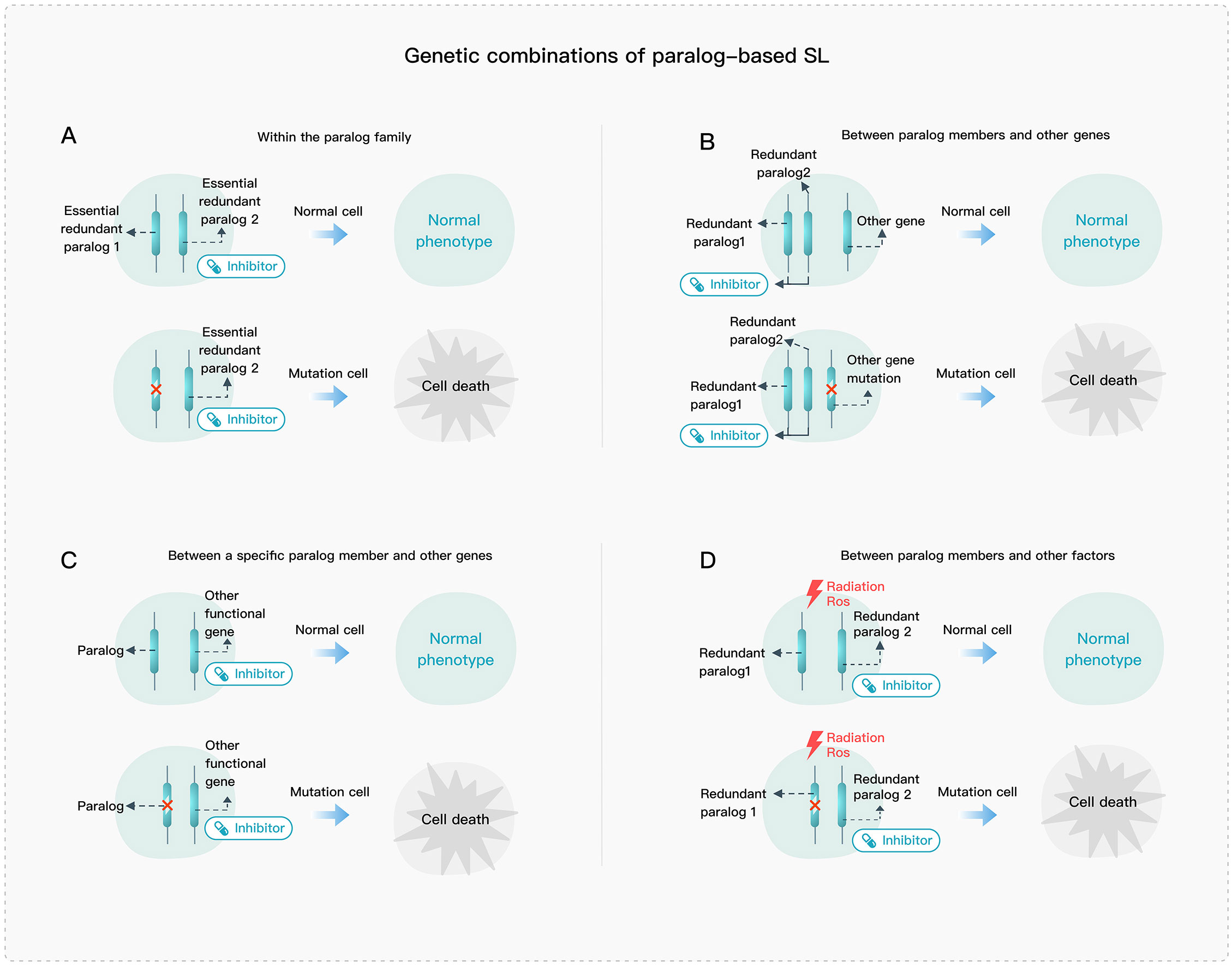
Figure 2 Genetic combination of paralog-based SL. (A) SL interactions within the paralog family. (B) SL interactions between paralog members and other genes. (C) SL interactions between a specific paralog member and other genes. (D) SL interactions between paralog members and other factors.
4.1 SL interactions within paralog families
4.1.1 “Destabilization” of the core complexes
The loss of paralog members involved in a core protein complex can lead to critical dysfunction and cellular damage (Figure 3A). Mechanistically, protein complex structures and stoichiometry will be abnormal once there is a complete absence of mutually exclusive paralog members, which then triggers post-translational regulation of other members of the protein complex (94, 95). For example, in the SWI/SNF complex, the double deletion of ARID1A and ARID1B leads to the structural disruption of the complex (19), and when both SMARCC1 and SMARCC2 are deleted, the complex is almost completely destabilized (43, 96). Additionally, targeting HDAC1/2 in the NuRD complex, can lead to the selective degradation of essential subunits and impair transcriptional control (26). Another mechanism of SL may arise from altered chromatin interactions, as seen with the single deletion of STAG1 and STAG2, which can alter the distribution of cohesin complexes and cause changes in DNA-DNA loop formation and chromatin accessibility and interactions (Figure 3A’) (29, 97–99).
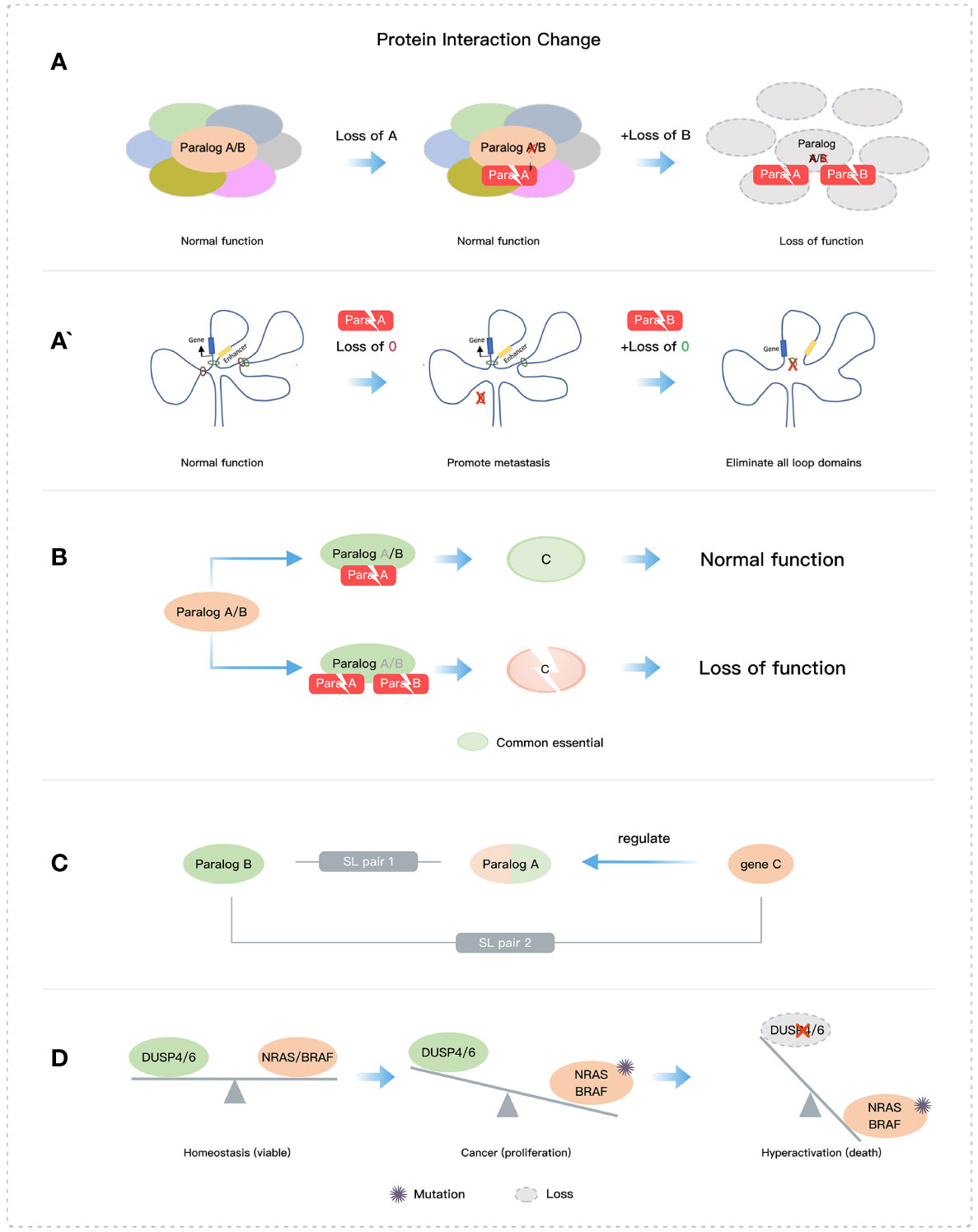
Figure 3 Some mechanisms of paralog-based SL. (A) Loss of core structures. (B) Loss of essential protein function. (C) The SL interaction of gene and its paralog or the regulator of its paralog. (D) Imbalance of two functions.
4.1.2 Core protein abnormalities
Disrupting the stability and/or function of essential proteins by deleting paralogs is an effective mechanism for SL (Figures 2A and 3B) (26). An example of paralog-based SL pairs with this mechanism is NXT1-NXT2, which regulates the stability of the essential protein NXF1. NXT1 or NXT2 forms heterodimers with NXF1 and constitutes the main mechanism for mRNA nuclear export. When both are absent, NXF1 is destabilized and rapidly degraded. The absence of NXF1 causes dysregulation of mRNA export from the nucleus to the cytoplasm and leads to abnormal cell growth or death (39, 100). Additionally, the exogenous expression of NXF1 can effectively restore mRNA export, and its protein stability is directly influenced by the presence of NXT1 or NXT2 (39, 101). Moreover, the deletion of both VRK1 and VRK2 reduces the essential protein BAF activity, leading to abnormal nuclear morphology, G2/M phase arrest, DNA damage, and eventually apoptosis (33, 34). The mechanism underlying the SL pair CREBBP and EP300 involves the abrogation of MYC expression (23, 24).
4.2 SL interactions between paralogs and other genes
The functional redundancy of paralogs hides some key SL targets, which highlights the existence of SL interactions between multiple paralog members and other genes (Figure 2B). BRCA1/2 proteins play a crucial role in DSB repair mediated by the homologous recombination (HR) pathway and have an indispensable contribution to maintaining genomic stability (63, 102). The PARP family has 17 members, among which PARP1 and PARP2 function as DNA damage sensing and transducing enzymes. PARP1 regulates cell proliferation and differentiation by repairing DNA single-strand break and DSB involved in the HR pathway, nucleotide excision repair, and base excision repair (10, 63, 103). PARP1/2 inhibitors are used as an SL therapy for BRCA-mutated cancers, although PARP1 and PARP2 can compensate for the deletion of each other in DNA repair. In the future, the SL relationships involving paralogs between compensatory pathways still need to be further clarified. Additionally, cells with NRAS or BRAF mutations are selectively impaired by the dual inactivation of DUSP4 and DUSP6 due to hyperactivation of the MAPK signaling pathway (18) (Figure 3D). This SL mechanism is not entirely dependent on paralogs but rather involves the balance of two functions (phosphorylation and dephosphorylation). Moreover, the SWI/SNF complex and the PRC2 complex containing EZH2 methyltransferase have been shown antagonistic activity in gene transcription (104, 105), of which EZH2 has an obvious anti-tumor effect on cell lines and xenografts with concurrent loss of SMARCA2 and SMARCA4 (106, 107). Dual loss of SMARCA4 and SMARCA2 also impacts tumor cell growth in PAX3:FOXO1+ARMS (108).
4.3 SL interaction between paralog single member and other genes/factors
Despite the redundancy of most paralog members, paralogs can acquire some non-overlapping functions through sub-functionalization and neo-functionalization. This can also lead to SL interactions with other genes (Figure 2C). For example, PRMT5 and PRMT9 are both type II arginine methyltransferases (109), but PRMT5 has a wider range of no redundancy functions compared with PRMT9 (110) and is a perceived SL target that can selectively kill tumor cells with MTAP deficiency (111). Similarly, despite the functional redundancy between SREBF1 and SREBF2, it was observed that SREBF2 had a significant negative genetic interaction with FASN while SREBF1 did not, indicating that they may have different functions that do not overlap (45). Also, CDK1 rather than CDK4/6 or CDK2 is selectively lethal in MYC-dependent cancers (112).
Genes can also form an SL interaction with upstream regulatory genes of their paralogs (Figure 3C). For example, BET inhibitors targeting BRD2 can downregulate the ARID1B expression, which indirectly triggers the SL interaction between ARID1A and ARID1B (113). Additionally, paralogs can also have SL effects in conjunction with other factors (Figure 2D), such as STAG2 mutations and DSB repair genes which increase sensitivity to cytotoxic chemotherapeutics and PARP or ATR inhibitors (27, 64).
5 Mechanism and influencing factors of paralog regulation
Notably, not all paralogs are capable of functionally compensating. Functional divergence is a major limiting factor for their ability to compensate for their function (Figure 4A). The neo-functionalization suggests that the duplicate gene has developed a new or more specialized function, and the complete sub-functionalization of paralog genes suggests that the duplicate genes have completely split their functions from those of the ancestral gene. Therefore, the aforementioned two cases have the greatest theoretical functional divergence, and there is almost no functional compensation relationship among the paralogs in these cases. In contrast, the structural and functional entanglement in sub-functionalization often have partial functional overlap, that is, there is partial functional compensation (114). In addition to the partitioning of different biochemical functions, sub-functionalization also includes the partition of its expression and dosage sub-functionalization (1). Back-up compensation and dosage amplification involve fewer functional divergences, and the paralogs from this evolutionary route often obtain a selective advantage (115, 116). Theoretically, this case can provide complete compensation for missing members of paralogs.
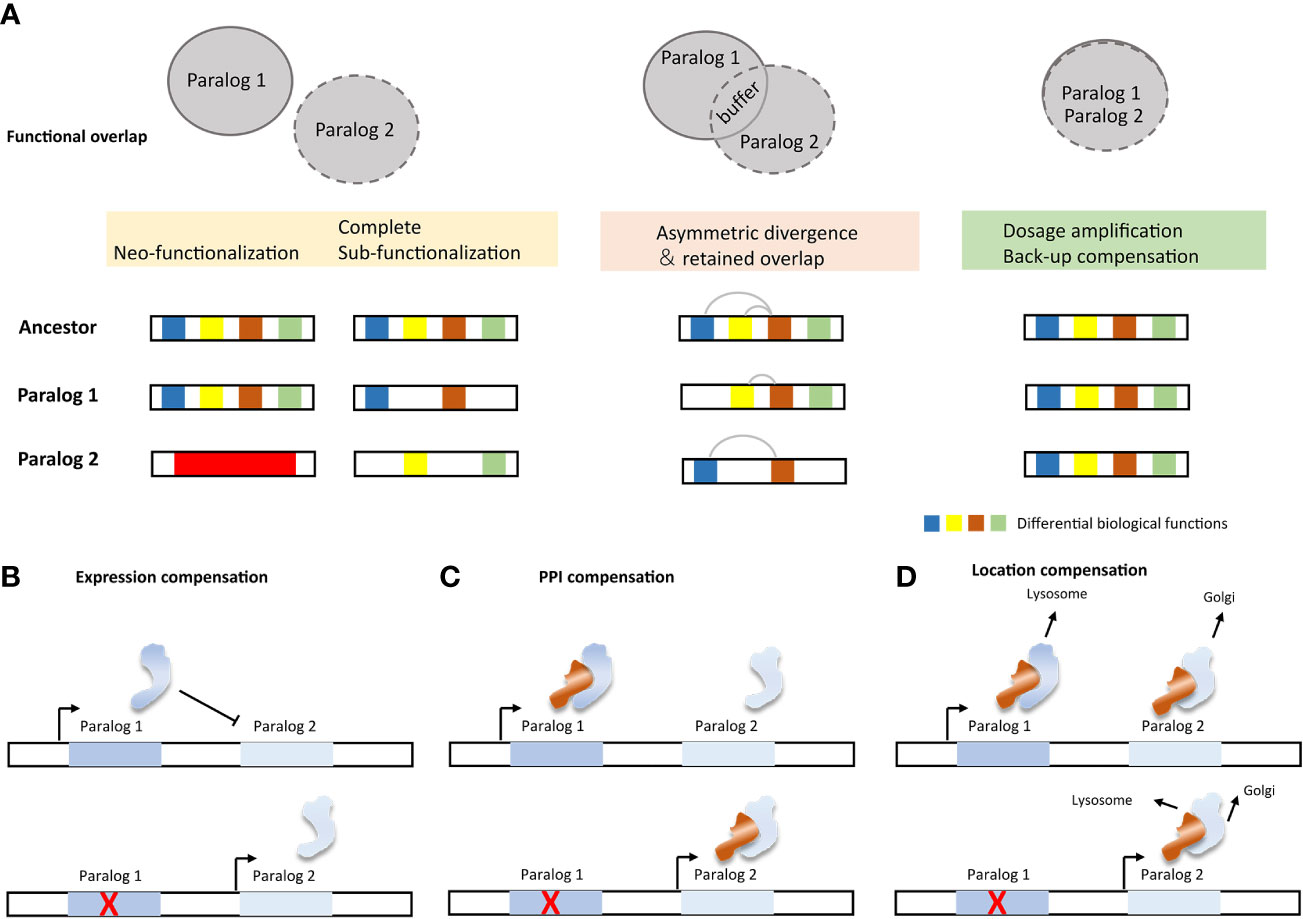
Figure 4 Impact factors and molecular mechanisms of paralog compensation. (A) Functional divergence and compensation capabilities of paralogs. (B) Active paralogous compensation forms (117).
The buffering effect of paralog can be subdivided into different mechanisms, passive paralogous compensation (analogous to “haplosufficient”) and active compensation (Figure 4B). Active compensation includes expression compensation, protein-protein interaction (PPI) compensation, and location compensation (117). Change in expression is the most easily monitored compensation method. However, the mode of action after up-regulation of expression still needs further confirmation. Paralogs involved in PPI are more likely to be subject to post-translational regulation (95). Compensation from PPI therefore often requires quantification of protein product rather than merely detection of changes in expression. Despite the limited reports on the regulation of SL paralog targets, evidence still suggests that multiple levels of regulation exist. For example, cancer cell lines with low UBB expression show elevated UBC levels, and inhibiting UBC induces UBB expression (118). In cells lacking Cohesin-SA2, the protein level of cohesin-SA1 increases, which changes the composition of the cohesin complex (29). Inactivation of DUSP4 leads to upregulation of DUSP6 in melanoma cells, likely through a transcriptional process (119). SMARCA4 inactivation leads to greater incorporation of the SMARCA2 subunit into the SWI/SNF complex (22).
From an alternate point of view, the paralog interaction relationship can be used as an argument for compensation ability. For example, the protective redundancy of paralogs partly depends on their independent functions. A large fraction of paralogous proteins may establish functional interdependence by heteromerization (physically interacting with each other), which reduces the ability of paralogous genes to compensate for each other’s loss (6). This indirect effect could stem from heteromeric paralogs having a larger number of PPI partners than non-heteromeric ones, thus loss-of-function (LOF) has a stronger effect on heteromeric ones and deal a greater degree of damage to the organism. Another research indicated that paralog pairs involved in protein complexes are more likely to show SL interactions (120). These findings are consistent with the SL interactions observed between mutually exclusive paralogs in the SWI/SNF complex (19, 22). In addition, from a dynamic evolution perspective, paralogs with earlier origins, and originating from whole-genome duplication are more likely to show SL interactions (6, 120).
6 Approaches for mining paralog-based SL pairs
Some SL pairs could be uncovered using genome-wide single gene perturbation (RNAi or CRISPR) combined with background abnormality in cancer cell lines (25, 26, 33, 37, 42, 45, 52). However, due to the functional redundancy, it is difficult to directly identify many essential paralogs for given cells. Instead, computational methods and systematic analysis across multiple cell lines will be feasible. Moreover, combinatorial screening methods are efficient at uncovering a more comprehensive set of paralog-based SL interactions. In addition, the mechanism of paralog-based SL interactions with other genes (Figure 2B) suggests that SL often involves not only two paralogs but also additional context-dependent genes. In this case, combinatorial paralog screening will be more effective. Therefore, experimental and computational approaches are complementary to each other.
6.1 Experimental approaches
To identify numerous and reliable paralog-based SL pairs, researchers have utilized combinatorial screening approaches (18, 44, 60, 77, 83, 121). However, due to the vast library size, they have to filter the paralogs based on various criteria such as sequence identity (60, 77, 83), paralog family size (44, 77), a single common orthologue in either Caenorhabditis elegans or Drosophila melanogaster (77), expression (60), gene essentiality (60, 77), and enzymatic paralogous genes (18). Several combinatorial screening tools are available in the paralog field and are continuously evolving. For instance, Parrish et al. and Thompson et al. utilized the relatively traditional Streptococcus pyogenes Cas9 enzyme system and focused on their interested paralog combinations (77, 83). To avoid the interference between the single-guide RNAs (sgRNAs) and increase the efficiency of combinatorial screening, Najm et al. expanded Streptococcus pyogenes Cas9 to orthogonal Cas9 enzymes from Staphylococcus aureus and Streptococcus pyogenes (75). Dede et al. took advantage of CRISPR/enCas12a to synthesize specific guide pairs in a single oligo and applied the enCas12a multiplex knockout system to identify paralog-based SL pairs (60). Gonatopoulos-Pournatzis et al. developed a hybrid Cas9-Cas12a enzyme from Streptococcus pyogenes and Lachnospiraceae bacterium to further improve efficiency (44). Many paralog-based SL interactions uncovered by these combinatorial screen approaches, and some new paralog-based SL pairs such as FAM50A-FAM50B (60, 77) and DUSP4-DUSP6 (18) have been confirmed in low-throughput experiments.
6.2 Computational approaches
The large-scale loss-of-function screening database the Cancer Dependency Map (DepMap portal, https://depmap.org/portal/) offers abundant single gene perturbation resources. Bioinformatic approaches leveraged these resources to systematically discover cancer-relevant paralog-based SL interactions by correlating genetic biomarkers (gene expression, copy number, mutation, and promoter methylation) with gene dependency (42, 60, 62, 118). For instance, this approach has uncovered paralog-based SL interactions such as UBB-UBC (118), MAGOH-MAGOHB (62), RPP25-RPP25, and DNAJC15-DNAJC19 (42). Moreover, an SL prediction model specifically for paralogs has been developed (49). The authors developed a random forest classifier to predict the robust SL interaction between paralogs by utilizing context-specific paralog SL interactions and 22 features reliable at evolutionary and mechanistic levels. ASF1A-ASF1B and COPS7A-COPS7B were the highly-ranked predicted SL pairs and were further validated by RNAi (49). As more cell lines undergo combinatorial perturbation of paralog pairs, the number of ground-truth positive and negative labels will increase, offering more opportunities for methods such as Network-based and Deep learning methods. Details of these methods can be found in reference in the review papers by Wang et al. (122) and Tang et al. (123).
7 Conclusion and future perspectives
The concept of SL has provided robust and novel strategies for precision cancer therapies. However, the compensatory relationship of functionally redundant genes may obscure potential therapeutic utility. Functional buffers, such as paralogs, are key factors in background dependence and compensation. Here, we reviewed the functions of paralogs in terms of SL interaction, the four genetic combination patterns of paralog-based SL interactions, the mechanisms and the factors affecting the buffering effect of paralogs, and efficient mining methods for SL paralog pairs. It provided a theoretical foundation for the mining of more paralog-based SL targets.
The evolutionary characteristics of tumor initiation and development are closely tied to the robustness provided by buffer systems. Paralog variations in tumor cells provide rich resources for developing SL-based cancer therapies. Thorough characterization of the paralog buffer system can be useful for future tumor research and therapeutic development. This includes understanding the importance of paralog members, predicting functional divergence and buffering effects, and identifying regulatory differences among paralog members. The same paralog SL gene pair can elicit different responses in different cell lines (24) depending on factors such as the deletion of a background gene, the existence of different compensation pathways, and the presence of other non-paralog compensatory genes. Achreja et al. had attempted to identify collateral lethal genes through collateral lethal gene identification via metabolic fluxes (CLIM), opening up a new avenue for finding compensatory genes beyond paralog genes (69, 124). The possible functional divergence of paralog could also be explored for tumor heterogeneity characterization (125–128).
In conclusion, paralog-based SL provides a powerful genetic engine for cancer research and clinical development. Understanding the mechanisms of SL pairs and the characterization of buffer systems in tumors will shed light on future research and development of precision cancer therapy.
Author contributions
YX and YZ initiated the project. XY wrote the manuscript. YZ provided feedback on the manuscript. All authors contributed to the article and approved the submitted version.
Acknowledgments
We thank K.Tian, L.Wei, M.Pu, W.Zheng, G.Peng, S.Li, B.Fu and others from the Innovation Center of StoneWise, AI. Inc. for their helpful discussions and support; We also thank L.Zheng and Q.Tang from the Product Center of StoneWise, AI. Inc. for their assistance in optimizing figures. X.Xiang, S.Guo, Y.Wang, and our colleagues at StoneWise, AI. Inc. for their support and encouragement.
Conflict of interest
Authors YX and YZ are employed by Beijing StoneWise Technology Co Ltd, China.
Publisher’s note
All claims expressed in this article are solely those of the authors and do not necessarily represent those of their affiliated organizations, or those of the publisher, the editors and the reviewers. Any product that may be evaluated in this article, or claim that may be made by its manufacturer, is not guaranteed or endorsed by the publisher.
References
1. Kuzmin E, Taylor JS, Boone C. Retention of duplicated genes in evolution. Trends Genet (2022) 38(1):59–72. doi: 10.1016/j.tig.2021.06.016
2. Zhang J. Evolution by gene duplication: an update. Trends Ecol Evol (2003) 18(6):292–8. doi: 10.1016/S0169-5347(03)00033-8
3. Gu Z, Steinmetz LM, Gu X, Scharfe C, Davis RW, Li WH. Role of duplicate genes in genetic robustness against null mutations. Nature (2003) 421(6918):63–6. doi: 10.1038/nature01198
4. Kamath RS, Fraser AG, Dong Y, Poulin G, Durbin R, Gotta M, et al. Systematic functional analysis of the caenorhabditis elegans genome using RNAi. Nature (2003) 421(6920):231–7. doi: 10.1038/nature01278
5. White JK, Gerdin AK, Karp NA, Ryder E, Buljan M, Bussell JN, et al. Genome-wide generation and systematic phenotyping of knockout mice reveals new roles for many genes. Cell (2013) 154(2):452–64. doi: 10.1016/j.cell.2013.06.022
6. Dandage R, Landry CR. Paralog dependency indirectly affects the robustness of human cells. Mol Syst Biol (2019) 15(9):e8871. doi: 10.15252/msb.20198871
7. Chapal M, Mintzer S, Brodsky S, Carmi M, Barkai N. Resolving noise-control conflict by gene duplication. PloS Biol (2019) 17(11):e3000289. doi: 10.1371/journal.pbio.3000289
8. De Kegel B, Ryan CJ. Paralog dispensability shapes homozygous deletion patterns in tumor genomes. bioRxiv (2022). doi: 10.1101/2022.06.20.496722
9. Topatana W, Juengpanich S, Li S, Cao J, Hu J, Lee J, et al. Advances in synthetic lethality for cancer therapy: cellular mechanism and clinical translation. J Hematol Oncol (2020) 13(1):1–22. doi: 10.1186/s13045-020-00956-5
10. Li S, Topatana W, Juengpanich S, Cao J, Hu J, Zhang B, et al. Development of synthetic lethality in cancer: molecular and cellular classification. Signal Transduct Target Ther (2020) 5(1):241. doi: 10.1038/s41392-020-00358-6
11. Lord CJ, Ashworth A. BRCAness revisited. Nat Rev Cancer (2016) 16(2):110–20. doi: 10.1038/nrc.2015.21
12. Bryant HE, Schultz N, Thomas HD, Parker KM, Flower D, Lopez E, et al. Specific killing of BRCA2-deficient tumours with inhibitors of poly (ADP-ribose) polymerase. Nature (2005) 434(7035):913–7. doi: 10.1038/nature03443
13. Farmer H, McCabe N, Lord CJ, Tutt AN, Johnson DA, Richardson TB, et al. Targeting the DNA repair defect in BRCA mutant cells as a therapeutic strategy. Nature (2005) 434(7035):917–21. doi: 10.1038/nature03445
14. D’Andrea AD. Mechanisms of PARP inhibitor sensitivity and resistance. DNA Repair (2018) 71:172–6. doi: 10.1016/j.dnarep.2018.08.021
15. Ashworth A, Lord CJ. Synthetic lethal therapies for cancer: what’s next after PARP inhibitors? Nat Rev Clin Oncol (2018) 15(9):564–76. doi: 10.1038/s41571-018-0055-6
16. Pilié PG, Tang C, Mills GB, Yap TA. State-of-the-art strategies for targeting the DNA damage response in cancer. Nat Rev Clin Oncol (2019) 16(2):81–104. doi: 10.1038/s41571-018-0114-z
17. Ibn-Salem J, Muro EM, Andrade-Navarro MA. Co-regulation of paralog genes in the three-dimensional chromatin architecture. Nucleic Acids Res (2017) 45(1):81–91. doi: 10.1093/nar/gkw813
18. Ito T, Young MJ, Li R, Jain S, Wernitznig A, Krill-Burger JM, et al. Paralog knockout profiling identifies DUSP4 and DUSP6 as a digenic dependence in MAPK pathway-driven cancers. Nat Genet (2021) 53(12):1664–72. doi: 10.1038/s41588-021-00967-z
19. Helming KC, Wang X, Wilson BG, Vazquez F, Haswell JR, Manchester HE, et al. ARID1B is a specific vulnerability in ARID1A-mutant cancers. Nat Med (2014) 20(3):251–4. doi: 10.1038/nm.3480
20. Oike T, Ogiwara H, Tominaga Y, Ito K, Ando O, Tsuta K, et al. A synthetic lethality–based strategy to treat cancers harboring a genetic deficiency in the chromatin remodeling factor BRG1. Cancer Res (2013) 73(17):5508–18. doi: 10.1158/0008-5472.CAN-12-4593
21. He ZX, Wei BF, Zhang X, Gong YP, Ma LY, Zhao W. Current development of CBP/p300 inhibitors in the last decade. Eur J Medicinal Chem (2021) 209:112861. doi: 10.1016/j.ejmech.2020.112861
22. Wilson BG, Helming KC, Wang X, Kim Y, Vazquez F, Jagani Z, et al. Residual complexes containing SMARCA2 (BRM) underlie the oncogenic drive of SMARCA4 (BRG1) mutation. Mol Cell Biol (2014) 34(6):1136–44. doi: 10.1128/MCB.01372-13
23. Ogiwara H, Sasaki M, Mitachi T, Oike T, Higuchi S, Tominaga Y, et al. Targeting p300 addiction in CBP-deficient cancers causes synthetic lethality by apoptotic cell death due to abrogation of MYC expression. Cancer Discovery (2016) 6(4):430–45. doi: 10.1158/2159-8290.CD-15-0754
24. Li J, Huang C, Xiong T, Zhuang C, Zhuang C, Li Y, et al. A CRISPR interference of CBP and p300 selectively induced synthetic lethality in bladder cancer cells in vitro. Int J Biol Sci (2019) 15(6):1276. doi: 10.7150/ijbs.32332
25. Nie M, Du L, Ren W, Joung J, Ye X, Shi X, et al. Genome-wide CRISPR screens reveal synthetic lethal interaction between CREBBP and EP300 in diffuse large b-cell lymphoma. Cell Death Dis (2021) 12(5):419. doi: 10.1038/s41419-021-03695-8
26. Zhang Y, Remillard D, Onubogu U, Karakyriakou B, Asiaban JN, Ramos AR, et al. Collateral lethality between HDAC1 and HDAC2 exploits cancer-specific NuRD complex vulnerabilities. bioRxiv (2022). doi: 10.1101/2022.05.30.493851
27. Liu Y, Xu H, van der Jeught K, Li Y, Liu S, Zhang L, et al. Somatic mutation of the cohesin complex subunit confers therapeutic vulnerabilities in cancer. J Clin Invest (2018) 128(7):2951–65. doi: 10.1172/JCI98727
28. Subramaniam A, Sandén C, Moura-Castro L, Žemaitis K, Schmiderer L, Bäckström A, et al. Inducing synthetic lethality for selective targeting of acute myeloid leukemia cells harboring STAG2 mutations. bioRxiv (2022). doi: 10.1101/2022.02.18.479480
29. Adane B, Alexe G, Seong BKA, Lu D, Hwang EE, Hnisz D, et al. STAG2 loss rewires oncogenic and developmental programs to promote metastasis in Ewing sarcoma. Cancer Cell (2021) 39(6):827–844. e10. doi: 10.1016/j.ccell.2021.05.007
30. Van Der Lelij P, Lieb S, Jude J, Wutz G, Santos CP, Falkenberg K, et al. Synthetic lethality between the cohesin subunits STAG1 and STAG2 in diverse cancer contexts. Elife (2017) 6:e26980. doi: 10.7554/eLife.26980
31. Popow J, Wieshofer C, Schlattl A, Woehrle S, Neumüller R, Petronczki MP, et al. Synthetic lethality between CSTF2 and CSTF2T in lung adenocarcinoma and melanoma cell lines. Cancer Res (2018) 78(13_Supplement):4449–9. doi: 10.1158/1538-7445.AM2018-4449
32. Zhou Y, Li Z, Wu X, Tou L, Zheng J, Zhou D. MAGOH/MAGOHB inhibits the tumorigenesis of gastric cancer via inactivation of b-RAF/MEK/ERK signaling. OncoTargets Ther (2020) 13:12723. doi: 10.2147/OTT.S263913
33. So J, Mabe NW, Englinger B, Chow KH, Moyer SM, Yerrum S, et al. VRK1 as a synthetic lethal target in VRK2 promoter-methylated cancers of the nervous system. JCI Insight (2022) 7(19):e158755. doi: 10.1172/jci.insight.158755
34. Shields JA, Meier SR, Bandi M, Mulkearns-Hubert EE, Hajdari N, Ferdinez MD, et al. VRK1 is a synthetic-lethal target in VRK2-deficient glioblastoma. Cancer Res (2022) 82(21):4044–57. doi: 10.1158/0008-5472.CAN-21-4443
35. Muller FL, Colla S, Aquilanti E, Manzo VE, Genovese G, Lee J, et al. Passenger deletions generate therapeutic vulnerabilities in cancer. Nature (2012) 488(7411):337–42. doi: 10.1038/nature11331
36. Dey P, Baddour J, Muller F, Wu CC, Wang H, Liao WT, et al. Genomic deletion of malic enzyme 2 confers collateral lethality in pancreatic cancer. Nature (2017) 542(7639):119–23. doi: 10.1038/nature21052
37. Neggers JE, Paolella BR, Asfaw A, Rothberg MV, Skipper TA, Yang A, et al. Synthetic lethal interaction between the ESCRT paralog enzymes VPS4A and VPS4B in cancers harboring loss of chromosome 18q or 16q. Cell Rep (2020) 33(11):108493. doi: 10.1016/j.celrep.2020.108493
38. Szymańska E, Nowak P, Kolmus K, Cybulska M, Goryca K, Derezińska-Wołek E, et al. Synthetic lethality between VPS4A and VPS4B triggers an inflammatory response in colorectal cancer. EMBO Mol Med (2020) 12(2):e10812. doi: 10.15252/emmm.201910812
39. Malone CF, Dharia NV, Kugener G, Forman AB, Rothberg MV, Abdusamad M, et al. Selective modulation of a pan-essential protein as a therapeutic strategy in cancer selective modulation of a pan-essential protein in cancer. Cancer Discovery (2021) 11(9):2282–99. doi: 10.1158/2159-8290.CD-20-1213
40. Kedves AT, Gleim S, Liang X, Bonal DM, Sigoillot F, Harbinski F, et al. Recurrent ubiquitin b silencing in gynecological cancers establishes dependence on ubiquitin c. J Clin Invest (2017) 127(12):4554–68. doi: 10.1172/JCI92914
41. Gong C, Krupka JA, Gao J, Grigoropoulos NF, Giotopoulos G, Asby R, et al. Sequential inverse dysregulation of the RNA helicases DDX3X and DDX3Y facilitates MYC-driven lymphomagenesis. Mol Cell (2021) 81(19):4059–4075. e11. doi: 10.1016/j.molcel.2021.07.041
42. Köferle A, Schlattl A, Hörmann A, Thatikonda V, Popa A, Spreitzer F, et al. Interrogation of cancer gene dependencies reveals paralog interactions of autosome and sex chromosome-encoded genes. Cell Rep (2022) 39(2):110636. doi: 10.1016/j.celrep.2022.110636
43. Schick S, Rendeiro AF, Runggatscher K, Ringler A, Boidol B, Hinkel M, et al. Systematic characterization of BAF mutations provides insights into intracomplex synthetic lethalities in human cancers. Nat Genet (2019) 51(9):1399–410. doi: 10.1038/s41588-019-0477-9
44. Gonatopoulos-Pournatzis T, Aregger M, Brown KR, Farhangmehr S, Braunschweig U, Ward HN, et al. Genetic interaction mapping and exon-resolution functional genomics with a hybrid Cas9–Cas12a platform. Nat Biotechnol (2020) 38(5):638–48. doi: 10.1038/s41587-020-0437-z
45. Aregger M, Lawson KA, Billmann M, Costanzo M, Tong AH, Chan K, et al. Systematic mapping of genetic interactions for de novo fatty acid synthesis identifies C12orf49 as a regulator of lipid metabolism. Nat Metab (2020) 2(6):499–513. doi: 10.1038/s42255-020-0211-z
46. Lord CJ, Quinn N, Ryan CJ. Integrative analysis of large-scale loss-of-function screens identifies robust cancer-associated genetic interactions. Elife (2020) 9:e58925. doi: 10.7554/eLife.58925.sa2
47. Yoshihama Y, Namiki H, Kato T, Shimazaki N, Takaishi S, Kadoshima-Yamaoka K, et al. Potent and selective PTDSS1 inhibitors induce collateral lethality in cancers with PTDSS2 deletion. Cancer Res (2022) 82(21):4031–43. doi: 10.1158/0008-5472.CAN-22-1006
48. Pemovska T, Bigenzahn JW, Srndic I, Lercher A, Bergthaler A, César-Razquin A, et al. Metabolic drug survey highlights cancer cell dependencies and vulnerabilities. Nat Commun (2021) 12(1):7190. doi: 10.1038/s41467-021-27329-x
49. De Kegel B, Quinn N, Thompson NA, Adams DJ, Ryan CJ. Comprehensive prediction of robust synthetic lethality between paralog pairs in cancer cell lines. Cell Syst (2021) 12(12):1144–1159. e6. doi: 10.1016/j.cels.2021.08.006
50. Ogiwara H, Takahashi K, Sasaki M, Kuroda T, Yoshida H, Watanabe R, et al. Targeting the vulnerability of glutathione metabolism in ARID1A-deficient cancers. Cancer Cell (2019) 35(2):177–190. e8. doi: 10.1016/j.ccell.2018.12.009
51. Kadoch C, Hargreaves DC, Hodges C, Elias L, Ho L, Ranish J, et al. Proteomic and bioinformatic analysis of mammalian SWI/SNF complexes identifies extensive roles in human malignancy. Nat Genet (2013) 45(6):592–601. doi: 10.1038/ng.2628
52. Hoffman GR, Rahal R, Buxton F, Xiang K, McAllister G, Frias E, et al. Functional epigenetics approach identifies BRM/SMARCA2 as a critical synthetic lethal target in BRG1-deficient cancers. Proc Natl Acad Sci (2014) 111(8):3128–33. doi: 10.1073/pnas.1316793111
53. Kadoch C. Lifting up the HAT: synthetic lethal screening reveals a novel vulnerability at the CBP–p300 axis. Cancer Discovery (2016) 6(4):350–2. doi: 10.1158/2159-8290.CD-16-0163
54. Barnaba N, LaRocque JR. Targeting cell cycle regulation via the G2-m checkpoint for synthetic lethality in melanoma. Cell Cycle (2021) 20(11):1041–51. doi: 10.1080/15384101.2021.1922806
55. Ciccarelli FD, Benedetti LG, Cereda M, Monteverde L, Desai NH. Synthetic lethal interaction between the tumour suppressor STAG2 and its paralog STAG1. Oncotarget (2017) 8(23):37619–32. doi: 10.18632/oncotarget.16838
56. Bailey ML, Tieu D, Habsid A, Tong AHY, Chan K, Moffat J, et al. Paralogous synthetic lethality underlies genetic dependencies of the cancer-mutated gene STAG2. Life Sci Alliance (2021) 4(11):1–14. doi: 10.26508/lsa.202101083
57. van der Lelij P, Newman JA, Lieb S, Jude J, Katis V, Hoffmann T, et al. STAG1 vulnerabilities for exploiting cohesin synthetic lethality in STAG2-deficient cancers. Life Sci Alliance (2020) 3(7):1–17. doi: 10.26508/lsa.202000725
58. Silla T, Schmid M, Dou Y, Garland W, Milek M, Imami K, et al. The human ZC3H3 and RBM26/27 proteins are critical for PAXT-mediated nuclear RNA decay. Nucleic Acids Res (2020) 48(5):2518–30. doi: 10.1093/nar/gkz1238
59. Xiao D, Wu J, Zhao H, Jiang X, Nie C. RPP25 as a prognostic-related biomarker that correlates with tumor metabolism in glioblastoma. Front Oncol (2022) 11:5393. doi: 10.3389/fonc.2021.714904
60. Dede M, McLaughlin M, Kim E, Hart T. Multiplex enCas12a screens detect functional buffering among paralogs otherwise masked in monogenic Cas9 knockout screens. Genome Biol (2020) 21:1–19. doi: 10.1186/s13059-020-02173-2
61. Xu Y, Yuan F, Sun Q, Zhao L, Hong Y, Tong S, et al. The RNA-binding protein CSTF2 regulates BAD to inhibit apoptosis in glioblastoma. Int J Biol Macromol (2023) 226:915–26. doi: 10.1016/j.ijbiomac.2022.12.044
62. Viswanathan SR, Nogueira MF, Buss CG, Krill-Burger JM, Wawer MJ, Malolepsza E, et al. Genome-scale analysis identifies paralog lethality as a vulnerability of chromosome 1p loss in cancer. Nat Genet (2018) 50(7):937–43. doi: 10.1038/s41588-018-0155-3
63. Patel PS, Algouneh A, Hakem R. Exploiting synthetic lethality to target BRCA1/2-deficient tumors: where we stand. Oncogene (2021) 40(17):3001–14. doi: 10.1038/s41388-021-01744-2
64. Mondal G, Stevers M, Goode B, Ashworth A, Solomon DA. A requirement for STAG2 in replication fork progression creates a targetable synthetic lethality in cohesin-mutant cancers. Nat Commun (2019) 10(1):1686. doi: 10.1038/s41467-019-09659-z
65. Lee SB, Segura-Bayona S, Villamor-Payà M, Saredi G, Todd MA, Attolini CS-O, et al. Tousled-like kinases stabilize replication forks and show synthetic lethality with checkpoint and PARP inhibitors. Sci Adv (2018) 4(8):eaat4985. doi: 10.1126/sciadv.aat4985
66. Xue Y, Meehan B, Macdonald E, Venneti S, Wang XQD, Witkowski L, et al. CDK4/6 inhibitors target SMARCA4-determined cyclin D1 deficiency in hypercalcemic small cell carcinoma of the ovary. Nat Commun (2019) 10(1):558. doi: 10.1038/s41467-018-06958-9
67. Houles T, Lavoie G, Nourreddine S, Cheung W, Vaillancourt-Jean É, Guérin CM, et al. CDK12 is hyperactivated and a synthetic-lethal target in BRAF-mutated melanoma. Nat Commun (2022) 13(1):6457. doi: 10.1038/s41467-022-34179-8
68. Li K, You J, Wu Q, Meng W, He Q, Yang B, et al. Cyclin-dependent kinases-based synthetic lethality: evidence, concept, and strategy. Acta Pharm Sin B (2021) 11(9):2738–48. doi: 10.1016/j.apsb.2021.01.002
69. Achreja A, Yu T, Mittal A, Choppara S, Animasahun O, Nenwani M, et al. Metabolic collateral lethal target identification reveals MTHFD2 paralogue dependency in ovarian cancer. Nat Metab (2022) 4(9):1119–37. doi: 10.1038/s42255-022-00636-3
70. Leonard PG, Satani N, Maxwell D, Lin YH, Hammoudi N, Peng Z, et al. SF2312 is a natural phosphonate inhibitor of enolase. Nat Chem Biol (2016) 12(12):1053–8. doi: 10.1038/nchembio.2195
71. Lin YH, Satani N, Hammoudi N, Yan VC, Barekatain Y, Khadka S, et al. An enolase inhibitor for the targeted treatment of ENO1-deleted cancers. Nat Metab (2020) 2(12):1413–26. doi: 10.1038/s42255-020-00313-3
72. Stine ZE, Schug ZT, Salvino JM, Dang CV. Targeting cancer metabolism in the era of precision oncology. Nat Rev Drug Discovery (2022) 21(2):141–62. doi: 10.1038/s41573-021-00339-6
73. Benjamin D, Robay D, Hindupur SK, Pohlmann J, Colombi M, El-Shemerly MY, et al. Dual inhibition of the lactate transporters MCT1 and MCT4 is synthetic lethal with metformin due to NAD+ depletion in cancer cells. Cell Rep (2018) 25(11):3047–3058. e4. doi: 10.1016/j.celrep.2018.11.043
74. DeWeirdt PC, Sangree AK, Hanna RE, Sanson KR, Hegde M, Strand C, et al. Genetic screens in isogenic mammalian cell lines without single cell cloning. Nat Commun (2020) 11(1):752. doi: 10.1038/s41467-020-14620-6
75. Najm FJ, Strand C, Donovan KF, Hegde M, Sanson KR, Vaimberg EW, et al. Orthologous CRISPR-Cas9 enzymes for combinatorial genetic screens. Nat Biotechnol (2018) 36(2):179–89. doi: 10.1038/nbt.4048
76. Venkataramanan S, Gadek M, Calviello L, Wilkins K, Floor SN. DDX3X and DDX3Y are redundant in protein synthesis. Rna (2021) 27(12):1577–88. doi: 10.1261/rna.078926.121
77. Thompson NA, Ranzani M, van der Weyden L, Iyer V, Offord V, Droop A, et al. Combinatorial CRISPR screen identifies fitness effects of gene paralogues. Nat Commun (2021) 12(1):1302. doi: 10.1038/s41467-021-21478-9
78. Lecona E, Fernandez-Capetillo O. Targeting ATR in cancer. Nat Rev Cancer (2018) 18(9):586–95. doi: 10.1038/s41568-018-0034-3
79. Diab A, Kao M, Kehrli K, Kim HY, Sidorova J, Mendez E. Multiple defects sensitize p53-deficient head and neck cancer cells to the WEE1 kinase inhibition. Mol Cancer Res (2019) 17(5):1115–28. doi: 10.1158/1541-7786.MCR-18-0860
80. Hu J, Cao J, Topatana W, Juengpanich S, Li S, Zhang B, et al. Targeting mutant p53 for cancer therapy: direct and indirect strategies. J Hematol Oncol (2021) 14:1–19. doi: 10.1186/s13045-021-01169-0
81. Liu P, Wang Y, Li X. Targeting the untargetable KRAS in cancer therapy. Acta Pharm Sin B (2019) 9(5):871–9. doi: 10.1016/j.apsb.2019.03.002
82. Thng DKH, Toh TB, Chow EKH. Capitalizing on synthetic lethality of MYC to treat cancer in the digital age. Trends Pharmacol Sci (2021) 42(3):166–82. doi: 10.1016/j.tips.2020.11.014
83. Parrish PC, Thomas JD, Gabel AM, Kamlapurkar S, Bradley RK, Berger AH. Discovery of synthetic lethal and tumor suppressor paralog pairs in the human genome. Cell Rep (2021) 36(9):109597. doi: 10.1016/j.celrep.2021.109597
84. Llombart V, Mansour MR. Therapeutic targeting of “undruggable” MYC. EBioMedicine (2022) 75:103756. doi: 10.1016/j.ebiom.2021.103756
85. Sun X, Gao H, Yang Y, He M, Wu Y, Song Y, et al. PROTACs: great opportunities for academia and industry. Signal Transduct Target Ther (2019) 4(1):64. doi: 10.1038/s41392-019-0101-6
86. Niu T, Li K, Jiang L, Zhou Z, Hong J, Chen X, et al. Noncovalent CDK12/13 dual inhibitors-based PROTACs degrade CDK12-cyclin K complex and induce synthetic lethality with PARP inhibitor. Eur J Medicinal Chem (2022) 228:114012. doi: 10.1016/j.ejmech.2021.114012
87. Kofink C, Trainor N, Mair B, Wöhrle S, Wurm M, Mischerikow N, et al. A selective and orally bioavailable VHL-recruiting PROTAC achieves SMARCA2 degradation in vivo. Nat Commun (2022) 13(1):5969. doi: 10.1038/s41467-022-33430-6
88. Bennett CF. Therapeutic antisense oligonucleotides are coming of age. Annu Rev Med (2019) 70:307–21. doi: 10.1146/annurev-med-041217-010829
89. Shadid M, Badawi M, Abulrob A. Antisense oligonucleotides: absorption, distribution, metabolism, and excretion. Expert Opin Drug Metab Toxicol (2021) 17(11):1281–92. doi: 10.1080/17425255.2021.1992382
90. Xu S, Zhou T, Doh HM, Trinh KR, Catapang A, Lee JT, et al. An HK2 antisense oligonucleotide induces synthetic lethality in HK1– HK2+ multiple MyelomaA precision therapy for HK1– HK2+ multiple myeloma. Cancer Res (2019) 79(10):2748–60. doi: 10.1158/0008-5472.CAN-18-2799
91. Tran NV, Nguyen LTA, Lim KW, Phan AT. Potent and selective knockdown of tyrosine kinase 2 by antisense oligonucleotides. ImmunoHorizons (2021) 5(2):70–80. doi: 10.4049/immunohorizons.2000108
92. Rosinski KV, Fujii N, Mito JK, Koo KK, Xuereb SM, Sala-Torra O, et al. DDX3Y encodes a class I MHC–restricted HY antigen that is expressed in leukemic stem cells. Blood J Am Soc Hematol (2008) 111(9):4817–26. doi: 10.1182/blood-2007-06-096313
93. Lacroix M, Beauchemin H, Möröy T. DDX3: a relevant therapeutic target for lymphoma? Expert Opin Ther Targets (2022) 26(12):1037–40. doi: 10.1080/14728222.2022.2166830
94. Taggart JC, Li GW. Production of protein-complex components is stoichiometric and lacks general feedback regulation in eukaryotes. Cell Syst (2018) 7(6):580–589. e4. doi: 10.1016/j.cels.2018.11.003
95. Ishikawa K, Makanae K, Iwasaki S, Ingolia NT, Moriya H. Post-translational dosage compensation buffers genetic perturbations to stoichiometry of protein complexes. PloS Genet (2017) 13(1):e1006554. doi: 10.1371/journal.pgen.1006554
96. Mashtalir N, D’Avino AR, Michel BC, Luo J, Pan J, Otto JE, et al. Modular organization and assembly of SWI/SNF family chromatin remodeling complexes. Cell (2018) 175(5):1272–1288. e20. doi: 10.1016/j.cell.2018.09.032
97. Cuadrado A, Losada A. Specialized functions of cohesins STAG1 and STAG2 in 3D genome architecture. Curr Opin Genet Dev (2020) 61:9–16. doi: 10.1016/j.gde.2020.02.024
98. Viny AD, Bowman RL, Liu Y, Lavallée VP, Eisman SE, Xiao W, et al. Cohesin members Stag1 and Stag2 display distinct roles in chromatin accessibility and topological control of HSC self-renewal and differentiation. Cell Stem Cell (2019) 25(5):682–696. e8. doi: 10.1016/j.stem.2019.08.003
99. Rao SS, Huang SC, St Hilaire BG, Engreitz JM, Perez EM, Kieffer-Kwon K-R, et al. Cohesin loss eliminates all loop domains. Cell (2017) 171(2):305–320. e24. doi: 10.1016/j.cell.2017.09.026
100. Wiegand HL, Coburn GA, Zeng Y, Kang Y, Bogerd HP, Cullen BR. Formation of Tap/NXT1 heterodimers activates tap-dependent nuclear mRNA export by enhancing recruitment to nuclear pore complexes. Mol Cell Biol (2002) 22(1):245–56. doi: 10.1128/MCB.22.1.245-256.2002
101. Herold A, Suyama M, Rodrigues JP, Braun IC, Kutay U, Carmo-Fonseca M, et al. TAP (NXF1) belongs to a multigene family of putative RNA export factors with a conserved modular architecture. Mol Cell Biol (2000) 20(23):8996–9008. doi: 10.1128/MCB.20.23.8996-9008.2000
102. Chen CC, Feng W, Lim PX, Kass EM, Jasin M. Homology-directed repair and the role of BRCA1, BRCA2, and related proteins in genome integrity and cancer. Annu Rev Cancer Biol (2018) 2:313–36. doi: 10.1146/annurev-cancerbio-030617-050502
103. Curtin NJ, Szabo C. Poly (ADP-ribose) polymerase inhibition: past, present and future. Nat Rev Drug Discovery (2020) 19(10):711–36. doi: 10.1038/s41573-020-0076-6
104. Kennison JA, Tamkun JW. Dosage-dependent modifiers of polycomb and antennapedia mutations in drosophila. Proc Natl Acad Sci (1988) 85(21):8136–40. doi: 10.1073/pnas.85.21.8136
105. Bitler BG, Aird KM, Garipov A, Li H, Amatangelo M, Kossenkov AV, et al. Synthetic lethality by targeting EZH2 methyltransferase activity in ARID1A-mutated cancers. Nat Med (2015) 21(3):231–8. doi: 10.1038/nm.3799
106. Januario T, Ye X, Bainer R, Alicke B, Smith T, Haley B, et al. PRC2-mediated repression of SMARCA2 predicts EZH2 inhibitor activity in SWI/SNF mutant tumors. Proc Natl Acad Sci (2017) 114(46):12249–54. doi: 10.1073/pnas.1703966114
107. Chan-Penebre E, Armstrong K, Drew A, Grassian AR, Feldman I, Knutson SK, et al. Selective killing of SMARCA2-and SMARCA4-deficient small cell carcinoma of the ovary, hypercalcemic type cells by inhibition of EZH2: in vitro and in vivo preclinical models. Mol Cancer Ther (2017) 16(5):850–60. doi: 10.1158/1535-7163.MCT-16-0678
108. Bharathy N, Cleary MM, Kim JA, Nagamori K, Crawford KA, Wang E, et al. SMARCA4 biology in alveolar rhabdomyosarcoma. Oncogene (2022) 41(11):1647–56. doi: 10.1038/s41388-022-02205-0
109. Bedford MT, Clarke SG. Protein arginine methylation in mammals: who, what, and why. Mol Cell (2009) 33(1):1–13. doi: 10.1016/j.molcel.2008.12.013
110. Yang Y, Hadjikyriacou A, Xia Z, Gayatri S, Kim D, Zurita-Lopez C, et al. PRMT9 is a type II methyltransferase that methylates the splicing factor SAP145. Nat Commun (2015) 6(1):6428. doi: 10.1038/ncomms7428
111. Marjon K, Kalev P, Marks K. Cancer dependencies: PRMT5 and MAT2A in MTAP/p16-deleted cancers. Annu Rev Cancer Biol (2021) 5:371–90. doi: 10.1146/annurev-cancerbio-030419-033444
112. Kang J, Sergio CM, Sutherland RL, Musgrove EA. Targeting cyclin-dependent kinase 1 (CDK1) but not CDK4/6 or CDK2 is selectively lethal to MYC-dependent human breast cancer cells. BMC Cancer (2014) 14(1):1–13. doi: 10.1186/1471-2407-14-32
113. Berns K, Caumanns JJ, Hijmans EM, Gennissen AM, Severson TM, Evers B, et al. ARID1A mutation sensitizes most ovarian clear cell carcinomas to BET inhibitors. Oncogene (2018) 37(33):4611–25. doi: 10.1038/s41388-018-0300-6
114. Kuzmin E, VanderSluis B, Nguyen Ba AN, Wang W, Koch EN, Usaj M, et al. Exploring whole-genome duplicate gene retention with complex genetic interaction analysis. Science (2020) 368(6498):eaaz5667. doi: 10.1126/science.aaz5667
115. Kondrashov FA, Kondrashov AS. Role of selection in fixation of gene duplications. J Theor Biol (2006) 239(2):141–51. doi: 10.1016/j.jtbi.2005.08.033
116. Kondrashov FA, Rogozin IB, Wolf YI, Koonin EV. Selection in the evolution of gene duplications. Genome Biol (2002) 3(2):1–9. doi: 10.1186/gb-2002-3-2-research0008
117. Diss G, Ascencio D, DeLuna A, Landry CR. Molecular mechanisms of paralogous compensation and the robustness of cellular networks. J Exp Zool Part B: Mol Dev Evol (2014) 322(7):488–99. doi: 10.1002/jez.b.22555
118. Tsherniak A, Vazquez F, Montgomery PG, Weir BA, Kryukov G, Cowley GS, et al. Defining a cancer dependency map. Cell (2017) 170(3):564–576. e16. doi: 10.1016/j.cell.2017.06.010
119. Kamada H, Yasuhira S, Shibazaki M, Amano H, Maesawa C. DUSP4 inactivation leads to reduced extracellular signal-regulated kinase activity through upregulation of DUSP6 in melanoma cells. J Invest Dermatol (2022) 142(9):2499–2507. e6. doi: 10.1016/j.jid.2022.02.007
120. De Kegel B, Ryan CJ. Paralog buffering contributes to the variable essentiality of genes in cancer cell lines. PloS Genet (2019) 15(10):e1008466. doi: 10.1371/journal.pgen.1008466
121. Feng X, Tang M, Dede M, Su D, Pei G, Jiang D, et al. Genome-wide CRISPR screens using isogenic cells reveal vulnerabilities conferred by loss of tumor suppressors. Sci Adv (2022) 8(19):eabm6638. doi: 10.1126/sciadv.abm6638
122. Wang J, Zhang Q, Han J, Zhao Y, Zhao C, Yan B, et al. Computational methods, databases and tools for synthetic lethality prediction. Briefings Bioinf (2022) 23(3):bbac106. doi: 10.1093/bib/bbac106
123. Tang S, Gokbag B, Fan K, Shao S, Huo Y, Wu X, et al. Synthetic lethal gene pairs: experimental approaches and predictive models. Front Genet (2022) 13:961611. doi: 10.3389/fgene.2022.961611
124. Muller FL, Aquilanti EA, DePinho RA. Collateral lethality: a new therapeutic strategy in oncology. Trends Cancer (2015) 1(3):161–73. doi: 10.1016/j.trecan.2015.10.002
125. Gaucher EA, Gu X, Miyamoto MM, Benner SA. Predicting functional divergence in protein evolution by site-specific rate shifts. Trends Biochem Sci (2002) 27(6):315–21. doi: 10.1016/S0968-0004(02)02094-7
126. Nguyen Ba AN, Strome B, Hua JJ, Desmond J, Gagnon-Arsenault I, Weiss EL, et al. Detecting functional divergence after gene duplication through evolutionary changes in posttranslational regulatory sequences. PloS Comput Biol (2014) 10(12):e1003977. doi: 10.1371/journal.pcbi.1003977
127. Pascarelli S, Laurino P. Inter-paralog amino acid inversion events in large phylogenies of duplicated proteins. PloS Comput Biol (2022) 18(4):e1010016. doi: 10.1371/journal.pcbi.1010016
Keywords: cancer therapy, synthetic lethality, paralog, clinical prospect, mechanism
Citation: Xin Y and Zhang Y (2023) Paralog-based synthetic lethality: rationales and applications. Front. Oncol. 13:1168143. doi: 10.3389/fonc.2023.1168143
Received: 17 February 2023; Accepted: 23 May 2023;
Published: 07 June 2023.
Edited by:
Chen Wang, Mayo Clinic, United StatesReviewed by:
Michael Aregger, National Cancer Institute at Frederick (NIH), United StatesTong Wu, Purdue University Indianapolis, United States
Copyright © 2023 Xin and Zhang. This is an open-access article distributed under the terms of the Creative Commons Attribution License (CC BY). The use, distribution or reproduction in other forums is permitted, provided the original author(s) and the copyright owner(s) are credited and that the original publication in this journal is cited, in accordance with accepted academic practice. No use, distribution or reproduction is permitted which does not comply with these terms.
*Correspondence: Yingsheng Zhang, emhhbmd5aW5nc2hlbmdAc3RvbmV3aXNlLmNu
†ORCID: Yingsheng Zhang, orcid.org/0000-0003-2520-3923