- 1Division of Hematology and Oncology, Department of Medicine, Case Western Reserve University, Cleveland, OH, United States
- 2Immune Oncology Program, Case Comprehensive Cancer Center, Cleveland, OH, United States
- 3Adult Hematologic Malignancies & Stem Cell Transplant Section, Seidman Cancer Center, University Hospitals Cleveland Medical Center, Cleveland, OH, United States
Pathways that govern cellular bioenergetics are deregulated in tumor cells and represent a hallmark of cancer. Tumor cells have the capacity to reprogram pathways that control nutrient acquisition, anabolism and catabolism to enhance their growth and survival. Tumorigenesis requires the autonomous reprogramming of key metabolic pathways that obtain, generate and produce metabolites from a nutrient-deprived tumor microenvironment to meet the increased bioenergetic demands of cancer cells. Intra- and extracellular factors also have a profound effect on gene expression to drive metabolic pathway reprogramming in not only cancer cells but also surrounding cell types that contribute to anti-tumor immunity. Despite a vast amount of genetic and histologic heterogeneity within and between cancer types, a finite set of pathways are commonly deregulated to support anabolism, catabolism and redox balance. Multiple myeloma (MM) is the second most common hematologic malignancy in adults and remains incurable in the vast majority of patients. Genetic events and the hypoxic bone marrow milieu deregulate glycolysis, glutaminolysis and fatty acid synthesis in MM cells to promote their proliferation, survival, metastasis, drug resistance and evasion of immunosurveillance. Here, we discuss mechanisms that disrupt metabolic pathways in MM cells to support the development of therapeutic resistance and thwart the effects of anti-myeloma immunity. A better understanding of the events that reprogram metabolism in myeloma and immune cells may reveal unforeseen vulnerabilities and advance the rational design of drug cocktails that improve patient survival.
1 Introduction
The hallmarks of cancer constitute an organizing principle to rationalize the complexities of neoplastic disease (1–4). Six biological capabilities - sustaining proliferative signaling, evading growth suppressors, resisting cell death, enabling replicative immortality, inducing angiogenesis, and activating invasion and metastasis acquired during the multistep development of human tumors - were initially identified as the hallmarks of human cancers (1). Genomic instability underlies these features that promote genetic diversity and intratumoral heterogeneity. Recently, reprogramming of energy metabolism and evading immune destruction have also been recognized as cancer hallmarks (2–4). Cancer cells sustain prodigious anabolic requirements that exceed those of neighboring somatic cells. Metabolic pathways in cancer cells are reprogrammed to achieve the required bioenergetic, biosynthetic and redox demands. Reprogramming of energy metabolism is also required to support continuous cell growth and proliferation, replacing the metabolic program that operates in most healthy tissues and fuels physiological operations within cancer cells (4–6). Tumorigenesis stems from the direct and indirect consequences of oncogenic mutations to reprogram key metabolic pathways (4, 7–12). Cancer-associated metabolic reprogramming also alters the level of key intracellular and extracellular metabolites (2, 11, 12).
Tumors display an added dimension of complexity since they contain a repertoire of recruited, ostensibly normal cells that contribute to the acquisition of hallmark traits by creating the tumor microenvironment (TME) (7–15). The TME is comprised of heterogeneous and interactive cell types including cancer cells and cancer stem cells surrounded by a multitude of recruited stromal cell and immune cell types. Cellular metabolism is reprogrammed in cancer cells by tumor-intrinsic and extrinsic factors. Cancer cells proliferate within the tumor permissive bone marrow (BM) and are surrounded by a complex environment that consists of cellular and acellular components, e.g., blood and lymph vessels, fibroblasts, endothelial cells, numerous immune cell types, osteoblasts, osteoclasts, pericytes, platelets, hematopoietic stem cells and other cell types. In addition, cancer cells are also influenced by surrounding cytokines, extracellular vesicles, cartilage, fat, bone and the extracellular matrix these reside within the BM milieu (10–15).
Oncometabolites are metabolites that aberrantly accumulate from distorted metabolism and are considered novel pathognomonic hallmarks in certain human cancers, e.g., glioma, leukemia, neuroendocrine tumors, and renal cancer (16–19). Oncometabolites have been shown to play a pivotal role in neoplastic transformation, cancer metabolism, and the development of therapeutic resistance. As a consequence of gain-of-function mutations and loss of tumor suppressors, oncometabolites accumulate within cancer cells and within the TME. For example, mutations in isocitrate dehydrogenase 1 and 2 (IDH1/2) occur in a subset of acute myeloid leukemia (AML) patients and IDH2 mutant leukemic cells produce elevated levels of the oncometabolite d-2-hydroxyglutarate (D2-HG) (17, 18). D2-HG is a structural homolog and antagonist of the Krebs cycle intermediate α-ketoglutarate (α-KG) that disrupts the Krebs cycle leading to metabolic and epigenetic derangements. D2-HG changes the catalytic activity of α-ketoglutarate–dependent dioxygenases leading to genome-wide histone and DNA methylation alterations (16–19).
2 Linking altered cellular metabolism to multiple myeloma
Multiple myeloma (MM) is a cancer of terminally-differentiated plasma cells (PCs) that accumulate and proliferate predominantly within the tumor permissive BM microenvironment (20–25). PCs are primary effectors of humoral immunity and function as antibody-producing factories that secrete vast amount of immunoglobulins. PC proliferation within the BM leads to increased production and circulation of the monoclonal (M-spike) protein in serum and/or urine (6, 26). Cardinal clinical features of MM include anemia, hypercalcemia, renal impairment and myeloma-related bone lesions (6, 20–22). The clinical course of nearly all MM patients is characterized by cycles of continuously shortening periods of remission followed by relapse. The prevalence of obesity, cardiovascular disease and diabetes increases with age and elderly patients diagnosed with MM generally present with these concurrent co-morbidities (6, 27–32). The prognosis of MM patients has significantly improved over the past two decades, primarily due to the incorporation of novel agents developed based upon the biology of disease (20, 22, 33). MM cells synthesize and secrete vast amounts of protein, especially immunoglobulins, and have adapted to withstand an enhanced capacity for unfolded polypeptides. Hence, MM cells are exquisitely sensitive to drugs that disrupt protein homeostasis, e.g., proteasome inhibitors (PIs). Although PIs represent a highly effective anti-myeloma therapy and transformed the management of MM, drug resistance inevitably emerges through compensatory protein clearance mechanisms, e.g., the aggresome+autophagy pathway (34). Genome-wide profiling identified individual microRNAs (miRs), e.g., miR-29b, that were differentially expressed in bortezomib-resistant MM cells compared to drug-naive cells. The highly distinct function and specialized habitat of MM cells shapes the circuitry of intracellular pathways that contribute to drug resistance (35).
Genomic, proteomic and metabolic changes in myeloma cells stimulates their clonal evolution and expansion that eventually leads to the emergence of drug resistant clones that are responsible for disease relapse (36–38). Altered cellular metabolism also reduces the anti-myeloma effect of standard-of-care agents, e.g., PIs and immunomodulatory drugs (IMiDs). Metabolic changes within the TME further decreases the beneficial anti-myeloma effects of PIs and IMiDs, monoclonal antibodies and cellular immunotherapies (14, 15, 23, 36). Despite the development of novel anti-myeloma drugs over the past two decades, disease heterogeneity, high-risk disease, early relapse and treatment resistance remain challenges (14, 20, 22, 24, 33). Moreover, subclonal heterogeneity of PCs evolves alongside disease progression through the selection of genetically and metabolically adapted subclones (37, 38). Importantly, the incidence of MM is associated with metabolic syndrome and inflammatory cytokines, while the anti-diabetic agent metformin that lowers blood glucose levels and statins, which lower the level of low-density lipoprotein (LDL) cholesterol, are positive prognostic factors in patients diagnosed with MM (39–42).
3 Metabolic pathways altered in multiple myeloma
MM cells employ specialized metabolic programs that differ from neighboring, untransformed somatic cells to sustain their extraordinary anabolic and catabolic needs (6, 42–45). Features of altered metabolism in MM include deregulated uptake and metabolism of glucose and amino acids especially glutamine, capacity to acquire scarce nutrients, enhanced glycolytic and tricarboxylic acid (TCA) cycle intermediates, elevated nicotinamide adenine dinucleotide phosphate (NADPH) production and elevated level of fatty acid (FA) synthesis.
3.1 Glucose metabolism
The glycolytic enzyme hexokinase II (HKII) is overexpressed in MM cells relative to PCs from healthy donors (46). Ikeda et al. found that hypoxia-inducible HKII impaired glycolysis and contributed to autophagy activation as well as the acquisition of an anti-apoptotic phenotype in myeloma cells. To detect candidate genes crucial for the acquisition of hypoxia-inducible autophagy, a comprehensive expression analysis was performed using MM patient samples incubated under normoxia or hypoxia. Hypoxic stress upregulated glycolytic genes (PFKFB4, ENO2, ALDOC, PFKFB3, HK2, PFKP, GPI, PGK1, LDHA, ALDOA, ENO1, PKM, and GAPDH) including HKII in samples obtained from MM patients (46). These results suggest that hypoxia-drive event may permit myeloma cells to metabolize glucose in an energetically favorable multi-step process. Antisense oligonucleotide (ASO) directed against HKII (HII-ASO1) suppressed HKII expression in MM cell cultures and in MM patient tumor cells xenografted into murine models (47). HKII-ASO1 shows selective HKII inhibition to support the clinical development of this approach. Aerobic glycolysis also activates the TCA cycle to produce NADPH and glutathione (GSH) which reduces oxidative stress. Since oxidative damage is essential for bortezomib-mediated cytotoxicity, drug resistance may be accompanied by increased tolerance towards oxidative insults. Soriano et al. showed that PI-adapted myeloma cells tolerate subtotal proteasome inhibition owing to metabolic adaptations that favor the generation of NADPH reducing equivalents, supported by oxidative glycolysis (48).
Lactate dehydrogenase A (LDHA) expression is increased in relapsed MM patients to suggest that glucose metabolism is enhanced (49). Proliferator-activator receptor-γ coactivator-1β (PGC-1β) and LDHA are highly expressed in MM cells and LDHA is upregulated by PGC-1β through the PGC-1β/RXRβ axis by acting on the LDHA promoter. Overexpression of PGC-1β or LDHA potentiated glycolysis metabolism and increased cell proliferation and tumor growth. Conversely, knockdown of either PGC-1β or LDHA suppressed glycolysis, increased reactive oxygen species (ROS) formation and apoptosis, suppressed tumor growth and enhanced mouse survival. Liu et al. investigated whether excess glucose induced hypoxia-inducible factor-1α (HIF-1α) and stimulated glucose metabolism and cell migration in pancreatic cancer cells (50). The authors studied wild-type (WT) MiaPaCa2 pancreatic cancer cells and a MiaPaCa2 subline transfected with an HIF-1α-specific small interfering (siRNA). Excess glucose stimulated the migration of WT and siRNA-treated MiaPaCa2 cells grown under normoxia and hypoxia, while glucose stimulated cell migration independent of HIF-1α. These studies indicated that excess glucose increases HIF-1α and ATP in hypoxic WT-MiaPaCa2 cells. Extracellular glucose levels and hypoxia regulate glucose metabolism independent of HIF-1α while glucose stimulates cell migration through HIF-1α-dependent and independent mechanisms.
The Warburg effect describes an increase in the rate of glucose uptake and preferential production of lactate, even in the presence of oxygen (51–53). Further evidence that Warburg’s
experiments on tumor tissue in vitro were valid in vivo was demonstrated in experiments on surviving tumor tissue and replicated in tumor-bearing animals (54, 55). The effect is clinically utilized in 18F-fluorodeoxyglucose (18F-FDG) positron emission tomography (PET) scans as sensitive diagnostic and prognostic tools (56, 57). Glucose is transported across the cell membrane through glucose transporters (GLUTs) through a facilitated diffusion mechanism (58–61). Owing to its elevated glycolytic gene profile, MM cells have been shown to be dependent on glycolysis and, therefore, susceptible to glycolysis inhibitors, e.g., GLUT inhibitors (58). Of the 14 GLUT subtypes, GLUT1 overexpression is most associated with poor clinical outcomes in cancer cell lines and cancer patients (44, 47, 58, 61). In MM cells, GLUT1 upregulation increases glucose uptake and enhances susceptible to GLUT1 inhibitors (61). MM cells are also dependent on GLUT4 for glucose uptake, survival, and elevated expression of the anti-apoptotic protein Mcl-1, that has been associated with tumorigenesis, poor prognosis, and drug resistance (58).
Upregulation of the GLUT membrane transporters, e.g., GLUT1 GLUT4, GLUT8 and GLUT11, increases the level of glycolytic metabolites in MM cells. The Federal Drug Administration (FDA)-approved HIV protease inhibitor ritonavir demonstrates an off-target inhibitory effect on GLUT4 as well as a dose-dependent inhibitory effect on glucose uptake and proliferation in L363 and KMS11 cells (62). However, a subset of MM cells survive glucose deprivation or ritonavir treatment, possibly through mitochondrial oxidative phosphorylation (OXPHOS). Targeting the mitochondrial complex I using the FDA-approved anti-diabetes drug metformin combined with ritonavir induced apoptosis in primary MM cells. The PI3K/AKT pathway, through mTOR-dependent activity, is linked to increased glucose metabolism and may explain the elevated levels of glycolytic intermediates seen in MM cells (63–66). The combination also suppressed AKT and mTORC1 phosphorylation and downregulated Mcl-1 expression (62).
LDH, which converts pyruvate and NADH to lactate and NAD+, is elevated in ~10% of patients with newly-diagnosed, symptomatic MM (67). HIF-1α is upregulated in drug resistant MM cells and leads to enhanced lactate production and the accumulation of glycolytic metabolites (68). HIF-1α upregulation is associated with metastasis, unfavorable prognosis, and reduced OS in cancer patients (68, 69). Since bortezomib decreases HKII activity in MM cells grown under hypoxic conditions and loss of HKII decreases LDHA activity, targeting LDHA could enhance effects of bortezomib (45). Indeed, inhibition of HIF-1α and LDHA have been shown to restore sensitivity to bortezomib and melphalan in MM cells (45). FX11 is a selective and potent LDHA inhibitor which reduces ATP levels by inducing oxidative stress and ROS production (70) (Table 1). PX-478 selectively inhibits HIF-1α to suppress cell migration, angiogenesis and drug resistance (71). Pyruvate kinase M2 (PKM2) regulates glycolysis and promotes tumor cell survival and proliferation (86). Never in mitosis gene A (NIMA)-related kinase 2 [NEK2] increases the PKM2/PKM1 ratio by splicing PKM to promote enhanced glycolysis that drives oncogenesis (54).
The HK isoform HKII is the rate-limiting step in aerobic glycolysis and is overexpressed in many cancers including MM (87, 88). Vincristine and bortezomib suppressed GLUT-1 and HK expression to induce apoptosis in MM cells (73), while WZb117 and phloretin inhibited GLUT-1 activity to decrease glucose uptake with synergistic anti-tumor effects in leukemia, lung, colon and breast cancers (74, 89, 90). Under hypoxic conditions, phloretin enhanced the effects of daunorubicin and overcame hypoxia-conferred drug resistance (91). Targeting glucose consumption through enzymatic regulators and transporters could serve as an effective anti-myeloma therapy.
3.2 Amino acid metabolism
Glutamine is an abundant amino acid crucial for cell proliferation, differentiation, apoptosis, and cytokine production (92). Glutamine is needed in MM cells for nucleic acid biosynthesis, to generate energy in the TCA cycle and to support increased amino acid and FA synthesis. MM cells are particularly dependent on extracellular glutamine since they exhibit high glutaminase levels and low glutamine synthetase expression. Glutamine depletion prevents MM growth and enhances sensitivity to anti-myeloma agents (77, 79, 84, 93–95). The histidine/large neutral amino acid transporter LAT1 (SLC7A5) glutamine transporter is overexpressed in MM cells and is associated with reduced overall survival (OS) (84). MM cells primarily rely upon the alanine, serine, cysteine transporter 2 (ASCT2/SLC1A5) and glutamine transporters for glutamine uptake. Targeting glutamine transporters, specifically ASCT2 inhibitors combined with the PI carfilzomib induced proteotoxic stress and ROS generation (81, 83). The need for extracellular glutamine makes glutamine transporters interesting targets for MM therapy.
Glutamine serves as an important energy source for cancer cells and glutamine deficiency or the glutaminase inhibitor benzophenanthridinone 968 induces apoptosis in MM cells (13, 78, 82). Benzophenanthridinone 968 effectively inhibits glutaminase and this inhibition induces apoptosis in MM cell lines (MMCLs) and patient primary tumor cells. Elevated expression of the glutamine transporters SNAT1, ASCT2 and LAT1, makes these an attractive target for anti-myeloma therapy (6). The prognostic significance of LAT1 in MM was investigated by immunohistochemistry to monitor the expression of LAT1 and its functional subunit, 4Fc heavy chain (CD98), on tumor cells in 100 newly diagnosed MM (NDMM) patients (84). LAT1 overexpression was associated with high proliferation and poor prognosis in NDMM patients. LAT1 may be a promising pathological marker to identify high-risk MM.
3.3 Fatty acid metabolism
A lipid profiling study uncovered large differences in lipid composition as well as amino acid and energy profiles from NDMM, relapsed and/or refractory (RRMM), monoclonal gammopathy of unknown significance (MGUS) and healthy controls (96). The metabolomic profile was quite different between that observed with samples from healthy controls compared to that of samples from patients with either MGUS, NDMM or RRMM. Significant alterations in amino acid, lipid, and energy metabolism were observed between the different patient groups. Eight metabolites, i.e., free carnitine, acetylcarnitine, glutamate, asymmetric dimethylarginine and phosphatidylcholine species, differed between MGUS and NDMM patients, supporting the notion that metabolic changes occur during myelomagenesis. A second lipidomics study revealed upregulation of ceramides and phosphatidylethanolamines (PEs) and downregulation of phosphatidylcholines, sphingomyelin and one species of PE in MM patients (97). Increased sphingomyelinase expression in primary patient samples was found and inhibition of sphingomyelinase by GW4869 further increased bortezomib and melphalan-mediated cell death (80). Treatment of MM cells with ixazomib led to the accumulation of lipids. Pre-treatment of MM cells with docosahexaenoic acid (DHA) or eicosapentaenoic acid (EHA) also increased the sensitivity to bortezomib by altering the GSH metabolic pathway (98). Tirado-Velez et al. tested the hypothesis that inhibition of β-oxidation and de novo FA synthesis would reduce cell proliferation in myeloma cells (85). The authors found that the RPMI-8226, NCI-H929 and U-266B1 cells displayed increased FA oxidation (FAO) and elevated expression of FA synthase (FAS). Inhibition of FAO by etomoxir and FAS by orlistat inhibited β-oxidation and de novo FA synthesis without significantly altering glucose metabolism. These effects were associated with cell cycle arrest in G0/G1 and reduced cell proliferation (43, 85). Etomoxir-mediated inhibition of FAO modestly increased the amount of lactate generated without altering glucose metabolism, to suggest that the inhibition of FAO in myeloma cells did not result in an adaptive mechanism to sustain energy homeostasis. FAS was elevated in ~70% of MM patients compared to healthy volunteers and inhibition of FAS by cerulenin promoted apoptosis (99). MMCLs and primary MM cells overexpress FAS to promote their survival and proliferation. MM patients have been reported to exhibit greater levels of saturated FAs and n-6 polyunsaturated FAs (PUFA), compared to healthy controls. Acetyl-CoA synthetase 2 (ACSS2) is overexpressed in MM cells derived from obese patients and contributes to myeloma progression (100). ACSS2 interacts with the oncoprotein interferon-regulated factor 4 (IRF4), and enhances IRF4 stability and IRF4-mediated gene transcription through activation of acetylation. The importance of ACSS2 overexpression in myeloma was confirmed by finding that an ACSS2 inhibitor reduced myeloma growth in vitro and in a diet-induced obese mouse model. The findings demonstrated a key impact for obesity-induced ACSS2 on myeloma progression and could be important for other obesity-related malignancies. Glioma cells were incubated with tetradecylthioacetic acid (T11111141), which cannot be β-oxidized, and the oxidizable FA palmitic acid (PA), in the presence of l-carnitine and the carnitine palmitoyltransferase inhibitors etomoxir and aminocarnitine. l-carnitine partially abolished PA-mediated growth reduction of glioma cells, whereas etomoxir and aminocarnitine enhanced the anti-proliferative effect of PA (101). Similarly, Samudio et al. demonstrated that inhibition of FAO with etomoxir or ranolazine reduced the proliferation and sensitized human leukemia cells to ABT-737-induced apoptosis (102). The conventional view has been that cancer cells predominately produce ATP by glycolysis, rather than by oxidation of energy-providing substrates. Mitochondrial uncoupling, i.e., continued reduction of oxygen without ATP synthesis, may obviate the ability of oxygen to inhibit glycolysis and promote the preference for glycolysis by shifting from pyruvate oxidation to FAO.
4 Oncogenic MYC and myeloma metabolism
Transcription factors of the MYC family are deregulated in up to 70% of all human cancers and MYC deregulation is a determinant of myeloma progression (103–105). Oncogenic levels of MYC regulate almost every aspect of cellular metabolism. MYC plays a key role in the regulation of aerobic glycolysis and activates glycolytic genes not only by transcription, but also through alternative splicing. In addition, enhanced MYC expression upregulates the level of glutamine transporters and suppresses inhibition of glutaminolysis (77, 94, 106). Glutamine depletion led to the rapid loss of the MYC protein, independent of MYC transcription and post-translational modifications. However, MYC loss was dependent on proteasomal activity and this loss was paralleled by an equally rapid induction of apoptosis (106). MYC transcription is upregulated in certain MM cells, especially during later stages of disease. The estimated 24-month progression-free survival was found to be significantly shorter in patients with intermediate to high MYC expression compared with patients with low MYC expression (107). However, this did not translate into a significant difference in OS. Somewhat different results were presented by Chng et al. which indicated that patients with MYC-expressing tumors had a significantly shorter OS (105). Chng et al. further reported that nearly all tumors with RAS mutations expressed a MYC activation signature. MYC activation, assessed by gene expression signature or immunohistochemistry was associated with hyperdiploid MM, and shorter survival even in tumors non-proliferative.
5 Impact of the hypoxic microenvironment on metabolism in myeloma cells
MM cells are exposed to different levels of oxygen and nutrients leading to metabolically heterogeneous phenotypes that differentially respond to therapeutic intervention (108–112). Hypoxia-inducible factors (HIFs), e.g., HIF-1α, are stabilized (108, 111, 113) within the TME and HIF-1α activation intensifies conversion of pyruvate into lactate instead of the oxidation of pyruvate in mitochondria. HIF-1α is also essential in regulating vascular endothelial growth factor (VEGF) which is associated with a poor prognosis in MM (114). HIF-1α was reported to be increased in MM as compared to controls (115, 116). The expression of HIF-1α was also correlated with serum levels of VEGF, basic fibroblast growth factor (bFGF) and angiopoietin-2 (Ang-2) (117–125). Gene expression datasets indicated that HIF-1α and HIF-2α were enriched in cells from NDMM patients compared to those from healthy donors (45, 126, 127). IMiDs treatment has been shown to decrease HIF-1α expression within the BM indicating that HIF-1α could also serve as a target in MM (128).
The TME consumes vast amounts of oxygen that is required for aerobic glycolysis within tumor cells (129) (Figure 1). Hypoxia increases anaerobic glycolysis by activating HIF (130) and hypoxia-induced LDHA and HKII promote PI-resistance in MM cells (45). It was also shown that activation of miR-210 due to hypoxia significantly reduced tumor susceptibility to CD8+ cytotoxic T-lymphocytes (CTLs) by downregulating PTPN1, HOXA1, and TP53I11 in melanoma and lung cancer cells (131). Hypoxia inducible miR-210 significantly downregulated PTPN1 and TP53I11 in MMCLs (132). Moreover, the HIF-inducible factor adrenomedullin is released from MM cells and stimulates vascular endothelial cells to express the angiogenic receptors CRLR and RAMP2 to promote angiogenesis (133). HIF-1α regulates interleukin (IL)-32 release from myeloma cells that is taken up by osteoclasts (134). The hypoxia-inducible p38-cyclic adenosine monophosphate (AMP) response element-binding protein (CREB)-Dickkopf-related protein 1 (DKK1) axis and upregulation of the HIF-1α-inducible MM SET domain-containing histone methyltransferase (MMSET) suppress osteoblastic bone formation (135). Taken together, hypoxic stress creates a favorable environment for myeloma survival by regulating chemotaxis, stimulating osteoclasts and endothelial cells, and inhibiting osteoblasts (Figure 1).
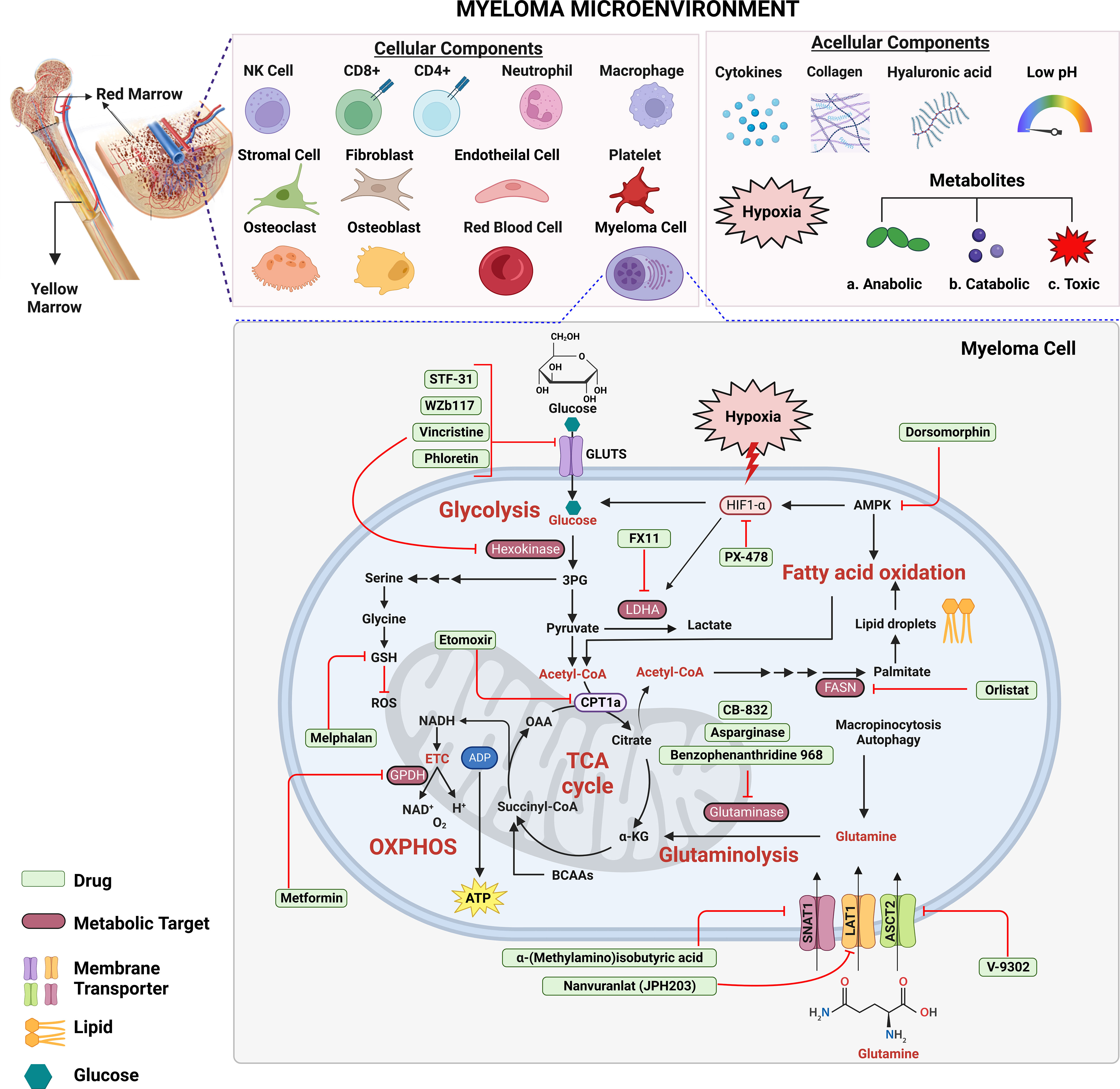
Figure 1 Targeting metabolic energy supply chains to enhance anti-myeloma therapy. Multiple myeloma cells use glucose as a primary source of energy followed by glutamine and fatty acids. Within cytosol, glucose is metabolized via glycolysis into two molecules of pyruvate and adenosine triphosphate (ATP) each. Next, pyruvate is transported across the mitochondrial matrix and is oxidized via TCA cycle to acetyl-CoA. Glutamine is transported across the membrane via transporters where it is metabolized into α-ketoglutarate (α-KG) via glutaminolysis. Oxidation of fatty acids results in breakdown of fatty acids into acetyl-CoA units. Which supplies energy to other tissues when glycogen stores are depleted. Each metabolic step releases energy in the form of electrons which are accepted by the electron transport chain to generate even more ATP through oxidative phosphorylation (OXPHOS). Metabolism targeting drugs (green) inhibit the key metabolic steps in glycolysis, TCA cycle, fatty acid synthesis, OXPHOS and glutaminolysis. Figure 1 is an original image created with Biorender.com, Toronto, Canada.
Bortezomib inhibits HIF-1α at the transcriptional level which in turn impairs recruitment of the coactivator CBP/p300 (136). The effects of PIs are attenuated within the hypoxic TME possibly due to reduced endoplasmic reticulum (ER) stress. In addition, the degradation of unfolded proteins normally mediated by proteasomes may be alternatively removed by autophagy.
HIF-inducible HKII activates autophagy by inhibiting mammalian target of rapamycin (mTOR) signaling (137). HKII is a promising therapeutic target and HKII inhibitors may increase the efficacy of anti-myeloma agents.
6 Metabolic alterations that alter anti-myeloma immunity
MM cells remodel the BM milieu to reshape the TME and negatively impact effectors of anti-tumor immunity (138–140). Deregulated tumor metabolism impairs the functional capacity of neighboring immune cells and compromises their differentiation (141–147). Adaptations within the TME create a competition for nutrients required by myeloma cells with their neighboring non-tumor cells. MM cells outcompete neighboring cells for nutrients to enhance tumor growth and impair anti-tumor immunity. Further dissecting the metabolic requirements of tumor and non-tumor cells in the TME may enhance immunotherapeutic responses. In addition, a disrupted vasculature deprives the TME of adequate blood supply and enhances competition between tumors and infiltrating immune cells (148). In MM, CD4+ and CD8+ T-cells form the primary immune defense, however, tumor-induced remodeling of the TME is unfavorable to T-cells due to nutrient deprivation, acidosis, and the accumulation of toxic metabolites (146) (Figure 1). The hypoxic microenvironment also upregulates PD-L1 expression on tumor cells through HIF-1α and a hypoxia response element (HRE) (149, 150). In MM, PD-L1 expression has been shown to be upregulated on malignant PCs (151, 152). NK cells from MM patients express PD-1, in contrast to NK cells from healthy individuals, which suppresses NK cell cytotoxicity (153). Immune cells take up and utilize amino acids, e.g., l-arginine, a non-essential amino acid present in macrophages and DCs, and lipids that are necessary for functional activity (154–157). As a product of aerobic or anaerobic glycolysis in tumors, lactic acid induces VEGF expression and M2-like polarization of tumor-associated macrophages (158). Tumor secretion of lactate also promotes overexpression of arginase I isoform in macrophages and is associated with immunosuppression. Lactate is not only a secondary product of cancer metabolism, but also promotes immune evasion through various mechanisms (159–161). Adenosine, and other products of cancer cell metabolism, interfere with the antitumor effect of infiltrating T-cells (162, 163). Tryptophan metabolites, especially kynurenine generated through indoleamine 2,3-dioxygenase (IDO1), have been shown to modulate T-cell activity (141–144). Kynurenine, produced by both IDO-1 and tryptophan-2,3-dioxygenase-2 (TDO-2), upregulated the PD-1 co-inhibitory pathway on activated CD8+ T-cells in vitro compared with vehicle-treated cells (140). Since tryptophan catabolites suppress immunity, blocking tryptophan catabolism with IDO inhibitors is a potential anticancer strategy (164). Targeting the tryptophan catabolic kynurenine pathway using immune-based approaches has been shown to enhance antitumor immunity and cytotoxicity in MM (165).
7 Conclusions
A century after Warburg discovered that tumor cells switch from mitochondrial respiration to glycolysis to generate energy, even under aerobic conditions, cancer metabolism remains perplexing. Myeloma cells exhibit a metabolic phenotype characterized by enhanced glycolytic flux for ATP production, glucose to lactate conversion and reduced mitochondrial OXPHOS (166–168). In contrast to healthy, differentiated cells, which rely on mitochondrial OXPHOS to generate energy, cancer cells rely on aerobic glycolysis. The switch to aerobic glycolysis may represent an adaptation to facilitate the uptake of nucleotides, amino acids, and lipids required for replication (169–171). Reprogramming of the metabolic pathways that contribute to tumor growth has exposed molecular vulnerabilities and actionable targets that can be exploited (Table 1). Warburg described aerobic, not anaerobic, glycolysis and therefore there must exist factors other than HIF1-α which elicit the Warburg effect. In addition, HIF1-α is not expressed in MM cells unless grown under hypoxic conditions. Recent work by Abdollahi et al. (172, 173) demonstrated a role for PRL-3 in the induction of glucose uptake and enhanced glycolysis. Importantly, this effect was not mediated through HIF1-α, c-Myc or AMPK, but rather through STAT1 and STAT2. In hypoxia there was synergy between HIF1-α and PRL-3 in promoting glycolysis. Contrary to HIF1-α, PRL-3 does not seem to reduce OXPHOS, and recent research has shown that many hematological cancers do not downregulate OXPHOS activity (174).
Proteasomes are central to the protein degradation machinery of eukaryotes (175, 176). Healthy and transformed cells depend on proteasomes to control the level of proteins linked to metabolism, survival and proliferation (177). Based upon these findings, over the past two decades PIs have emerged as a transformative anti-myeloma therapy that has improved patient OS and quality-of-life. Proteasome abundance and catalytic activity is controlled at the level of assembly and is finely tuned by adaptations in cellular metabolism (177). Sequencing of PCs from NDMM patients has shown that MM is frequently dominated by RAS (43% of patients) and nuclear factor kappa B (NF-κB) pathway (17%) mutations (178). Malignant PCs undergo extensive metabolic reprogramming during myelomagenesis that is enhanced by KRAS, NRAS, and BRAF-activating mutations to elevate proteasomal capacity and reduce ER stress (179). Ras and related proteins are mutated or deregulated in many solid tumors, but PIs are ineffective against these cancers (180). Future studies are needed to decipher how solid tumors reprogram cell metabolism to evade the cytotoxic effect of PIs. Novel agents and drug delivery systems that target cancer metabolism may broaden the therapeutic impact of PIs in rationally-designed drug cocktails that improve patient survival.
Author contributions
JD, PR, and KG developed the concept, wrote, edited, made substantial contributions, and approved the final version of the manuscript.
Funding
Research was supported by NIH R01 (5R01AI139141 to JJD), University Hospitals Cleveland Medical Center/Seidman Cancer Center, the Case Comprehensive Cancer Center and the Vinney Foundation Award (to JJD).
Acknowledgments
The authors thank Berkha Rani, MBBS, for critical reading of the manuscript.
Conflict of interest
The authors declare that the research was conducted in the absence of any commercial or financial relationships that could be construed as a potential conflict of interest.
Publisher’s note
All claims expressed in this article are solely those of the authors and do not necessarily represent those of their affiliated organizations, or those of the publisher, the editors and the reviewers. Any product that may be evaluated in this article, or claim that may be made by its manufacturer, is not guaranteed or endorsed by the publisher.
Glossary
References
1. Hanahan D, Weinberg RA. The hallmarks of cancer. Cell (2000) 100(1):57–70. doi: 10.1016/s0092-8674(00)81683-9
2. Hanahan D, Weinberg RA. Hallmarks of cancer: the next generation. Cell (2011) 144(5):646–74. doi: 10.1016/j.cell.2011.02.013
3. Pavlova NN, Thompson CB. The emerging hallmarks of cancer metabolism. Cell Metab (2016) 23(1):27–47. doi: 10.1016/j.cmet.2015.12.006
4. Hanahan D. Hallmarks of cancer: new dimensions. Cancer Discovery (2022) 12(1):31–46. doi: 10.1158/2159-8290.CD-21-1059
5. Zheng J. Energy metabolism of cancer: glycolysis versus oxidative phosphorylation (Review). Oncol Lett (2012) 4(6):1151–7. doi: 10.3892/ol.2012.928
6. Gavriatopoulou M, Paschou SA, Ntanasis-Stathopoulos I, Dimopoulos MA. Metabolic disorders in multiple myeloma. Int J Mol Sci (2021) 22(21). doi: 10.3390/ijms222111430
7. Dey P, Kimmelman AC, DePinho RA. Metabolic codependencies in the tumor microenvironment. Cancer Discovery (2021) 11(5):1067–81. doi: 10.1158/2159-8290.CD-20-1211
8. Alfarouk KO, Shayoub ME, Muddathir AK, Elhassan GO, Bashir AH. Evolution of tumor metabolism might reflect carcinogenesis as a reverse evolution process (Dismantling of multicellularity). Cancers (Basel) (2011) 3(3):3002–17. doi: 10.3390/cancers3033002
9. Elia I, Haigis MC. Metabolites and the tumour microenvironment: from cellular mechanisms to systemic metabolism. Nat Metab (2021) 3(1):21–32. doi: 10.1038/s42255-020-00317-z
10. Martinez-Reyes I, Chandel NS. Cancer metabolism: looking forward. Nat Rev Cancer (2021) 21(10):669–80. doi: 10.1038/s41568-021-00378-6
11. Chen C, Wang Z, Qin Y. Connections between metabolism and epigenetics: mechanisms and novel anti-cancer strategy. Front Pharmacol (2022) 13:935536. doi: 10.3389/fphar.2022.935536
12. Ge T, Gu X, Jia R, Ge S, Chai P, Zhuang A, et al. Crosstalk between metabolic reprogramming and epigenetics in cancer: updates on mechanisms and therapeutic opportunities. Cancer Commun (Lond) (2022) 42(11):1049–82. doi: 10.1002/cac2.12374
13. Altman BJ, Stine ZE, Dang CV. From Krebs to clinic: glutamine metabolism to cancer therapy. Nat Rev Cancer (2016) 16(10):619–34. doi: 10.1038/nrc.2016.71
14. Rana PS, Murphy EV, Kort J, Driscoll JJ. Road testing new car design strategies in multiple myeloma. Front Immunol (2022) 13:957157. doi: 10.3389/fimmu.2022.957157
15. Swamydas M, Murphy EV, Ignatz-Hoover JJ, Malek E, Driscoll JJ. Deciphering mechanisms of immune escape to inform immunotherapeutic strategies in multiple myeloma. J Hematol Oncol (2022) 15(1):17. doi: 10.1186/s13045-022-01234-2
16. Liu Y, Yang C. Oncometabolites in cancer: current understanding and challenges. Cancer Res (2021) 81(11):2820–3. doi: 10.1158/0008-5472.CAN-20-3730
17. Xu W, Yang H, Liu Y, Yang Y, Wang P, Kim SH, et al. Oncometabolite 2-hydroxyglutarate is a competitive inhibitor of alpha-Ketoglutarate-Dependent dioxygenases. Cancer Cell (2011) 19(1):17–30. doi: 10.1016/j.ccr.2010.12.014
18. Han S, Liu Y, Cai SJ, Qian M, Ding J, Larion M, et al. Idh mutation in glioma: molecular mechanisms and potential therapeutic targets. Br J Cancer (2020) 122(11):1580–9. doi: 10.1038/s41416-020-0814-x
19. Nowicki S, Gottlieb E. Oncometabolites: tailoring our genes. FEBS J (2015) 282(15):2796–805. doi: 10.1111/febs.13295
20. van de Donk N, Pawlyn C, Yong KL. Multiple myeloma. Lancet (2021) 397(10272):410–27. doi: 10.1016/S0140-6736(21)00135-5
21. Cowan AJ, Allen C, Barac A, Basaleem H, Bensenor I, Curado MP, et al. Global burden of multiple myeloma: a systematic analysis for the global burden of disease study 2016. JAMA Oncol (2018) 4(9):1221–7. doi: 10.1001/jamaoncol.2018.2128
22. Anderson KC. Progress and paradigms in multiple myeloma. Clin Cancer Res (2016) 22(22):5419–27. doi: 10.1158/1078-0432.CCR-16-0625
23. Ignatz-Hoover JJ, Driscoll JJ. Therapeutics to harness the immune microenvironment in multiple myeloma. Cancer Drug Resist (2022) 5(3):647–61. doi: 10.20517/cdr.2022.23
24. Mimura N, Hideshima T, Anderson KC. Novel therapeutic strategies for multiple myeloma. Exp Hematol (2015) 43(8):732–41. doi: 10.1016/j.exphem.2015.04.010
25. Ignatz-Hoover JJ, Murphy EV, Driscoll JJ. Targeting proteasomes in cancer and infectious disease: a parallel strategy to treat malignancies and microbes. Front Cell Infect Microbiol (2022) 12:925804. doi: 10.3389/fcimb.2022.925804
26. Fairfield H, Falank C, Avery L, Reagan MR. Multiple myeloma in the marrow: pathogenesis and treatments. Ann N Y Acad Sci (2016) 1364(1):32–51. doi: 10.1111/nyas.13038
27. Hales CM, Fryar CD, Carroll MD, Freedman DS, Ogden CL. Trends in obesity and severe obesity prevalence in us youth and adults by sex and age, 2007-2008 to 2015-2016. JAMA (2018) 319(16):1723–5. doi: 10.1001/jama.2018.3060
28. Felix-Redondo FJ, Grau M, Fernandez-Berges D. Cholesterol and cardiovascular disease in the elderly. Facts Gaps Aging Dis (2013) 4(3):154–69.
29. Geiss LS, Wang J, Cheng YJ, Thompson TJ, Barker L, Li Y, et al. Prevalence and incidence trends for diagnosed diabetes among adults aged 20 to 79 years, united states, 1980-2012. JAMA (2014) 312(12):1218–26. doi: 10.1001/jama.2014.11494
30. Fotiou D, Ntanasis-Stathopoulos I, Gavriatopoulou M, Dimopoulos MA. Multiple myeloma: current and future management in the aging population. Maturitas (2020) 138:8–13. doi: 10.1016/j.maturitas.2020.04.015
31. Gavriatopoulou M, Fotiou D, Koloventzou U, Roussou M, Migkou M, Ntanasis-Stathopoulos I, et al. Vulnerability variables among octogenerian myeloma patients: a single-center analysis of 110 patients. Leuk Lymphoma (2019) 60(3):619–28. doi: 10.1080/10428194.2018.1509323
32. Gavriatopoulou M, Fotiou D, Ntanasis-Stathopoulos I, Kastritis E, Terpos E, Dimopoulos MA. How I treat elderly patients with plasma cell dyscrasias. Aging (Albany NY) (2018) 10(12):4248–68. doi: 10.18632/aging.101707
33. Driscoll JJ, Brailey M. Emerging small molecule approaches to enhance the antimyeloma benefit of proteasome inhibitors. Cancer Metast Rev (2017) 36(4):585–98. doi: 10.1007/s10555-017-9698-5
34. Jagannathan S, Vad N, Vallabhapurapu S, Vallabhapurapu S, Anderson KC, Driscoll JJ. Mir-29b replacement inhibits proteasomes and disrupts Aggresome+Autophagosome formation to enhance the antimyeloma benefit of bortezomib. Leukemia (2015) 29(3):727–38. doi: 10.1038/leu.2014.279
35. Obeng EA, Carlson LM, Gutman DM, Harrington WJ Jr., Lee KP, Boise LH. Proteasome inhibitors induce a terminal unfolded protein response in multiple myeloma cells. Blood (2006) 107(12):4907–16. doi: 10.1182/blood-2005-08-3531
36. Schwestermann J, Besse A, Driessen C, Besse L. Contribution of the tumor microenvironment to metabolic changes triggering resistance of multiple myeloma to proteasome inhibitors. Front Oncol (2022) 12:899272. doi: 10.3389/fonc.2022.899272
37. Bolli N, Avet-Loiseau H, Wedge DC, Van Loo P, Alexandrov LB, Martincorena I, et al. Heterogeneity of genomic evolution and mutational profiles in multiple myeloma. Nat Commun (2014) 5:2997. doi: 10.1038/ncomms3997
38. Keats JJ, Chesi M, Egan JB, Garbitt VM, Palmer SE, Braggio E, et al. Clonal competition with alternating dominance in multiple myeloma. Blood (2012) 120(5):1067–76. doi: 10.1182/blood-2012-01-405985
39. Sanfilippo KM, Keller J, Gage BF, Luo S, Wang TF, Moskowitz G, et al. Statins are associated with reduced mortality in multiple myeloma. J Clin Oncol (2016) 34(33):4008–14. doi: 10.1200/JCO.2016.68.3482
40. Song Y, Chen S, Xiang W, Xiao M, Xiao H. The mechanism of treatment of multiple myeloma with metformin by way of metabolism. Arch Med Sci (2021) 17(4):1056–63. doi: 10.5114/aoms.2020.101305
41. Wu W, Merriman K, Nabaah A, Seval N, Seval D, Lin H, et al. The association of diabetes and anti-diabetic medications with clinical outcomes in multiple myeloma. Br J Cancer (2014) 111(3):628–36. doi: 10.1038/bjc.2014.307
42. Roman-Trufero M, Auner HW, Edwards CM. Multiple myeloma metabolism - a treasure trove of therapeutic targets? Front Immunol (2022) 13:897862. doi: 10.3389/fimmu.2022.897862
43. Rizzieri D, Paul B, Kang Y. Metabolic alterations and the potential for targeting metabolic pathways in the treatment of multiple myeloma. J Cancer Metastasis Treat (2019) 5. doi: 10.20517/2394-4722.2019.05
44. Porporato PE, Dhup S, Dadhich RK, Copetti T, Sonveaux P. Anticancer targets in the glycolytic metabolism of tumors: a comprehensive review. Front Pharmacol (2011) 2:49. doi: 10.3389/fphar.2011.00049
45. Maiso P, Huynh D, Moschetta M, Sacco A, Aljawai Y, Mishima Y, et al. Metabolic signature identifies novel targets for drug resistance in multiple myeloma. Cancer Res (2015) 75(10):2071–82. doi: 10.1158/0008-5472.CAN-14-3400
46. Ikeda S, Abe F, Matsuda Y, Kitadate A, Takahashi N, Tagawa H. Hypoxia-inducible hexokinase-2 enhances anti-apoptotic function Via activating autophagy in multiple myeloma. Cancer Sci (2020) 111(11):4088–101. doi: 10.1111/cas.14614
47. Xu S, Zhou T, Doh HM, Trinh KR, Catapang A, Lee JT, et al. An Hk2 antisense oligonucleotide induces synthetic lethality in Hk1(-)Hk2(+) multiple myeloma. Cancer Res (2019) 79(10):2748–60. doi: 10.1158/0008-5472.CAN-18-2799
48. Soriano GP, Besse L, Li N, Kraus M, Besse A, Meeuwenoord N, et al. Proteasome inhibitor-adapted myeloma cells are largely independent from proteasome activity and show complex proteomic changes, in particular in redox and energy metabolism. Leukemia (2016) 30(11):2198–207. doi: 10.1038/leu.2016.102
49. Zhang H, Li L, Chen Q, Li M, Feng J, Sun Y, et al. Pgc1beta regulates multiple myeloma tumor growth through ldha-mediated glycolytic metabolism. Mol Oncol (2018) 12(9):1579–95. doi: 10.1002/1878-0261.12363
50. Liu Z, Jia X, Duan Y, Xiao H, Sundqvist KG, Permert J, et al. Excess glucose induces hypoxia-inducible factor-1alpha in pancreatic cancer cells and stimulates glucose metabolism and cell migration. Cancer Biol Ther (2013) 14(5):428–35. doi: 10.4161/cbt.23786
51. Warburg O. On the origin of cancer cells. Science (1956) 123(3191):309–14. doi: 10.1126/science.123.3191.309
52. Cori CF, Cori GT. The carbohydrate metabolism of tumors ii. changes in the sugar, lactic acid, and Co2-combining power of blood passing through a tumor. J Biol Chem (1925) 65:397–405.
53. Vander Heiden MG, Cantley LC, Thompson CB. Understanding the warburg effect: the metabolic requirements of cell proliferation. Science (2009) 324(5930):1029–33. doi: 10.1126/science.1160809
54. Gu Z, Xia J, Xu H, Frech I, Tricot G, Zhan F. Nek2 promotes aerobic glycolysis in multiple myeloma through regulating splicing of pyruvate kinase. J Hematol Oncol (2017) 10(1):17. doi: 10.1186/s13045-017-0392-4
55. Walters DK, Arendt BK, Jelinek DF. Cd147 regulates the expression of Mct1 and lactate export in multiple myeloma cells. Cell Cycle (2013) 12(19):3175–83. doi: 10.4161/cc.26193
56. Cavo M, Terpos E, Nanni C, Moreau P, Lentzsch S, Zweegman S, et al. Role of (18)F-fdg Pet/Ct in the diagnosis and management of multiple myeloma and other plasma cell disorders: a consensus statement by the international myeloma working group. Lancet Oncol (2017) 18(4):e206–e17. doi: 10.1016/S1470-2045(17)30189-4
57. Takahashi MES, Mosci C, Souza EM, Brunetto SQ, Etchebehere E, Santos AO, et al. Proposal for a quantitative (18)F-fdg Pet/Ct metabolic parameter to assess the intensity of bone involvement in multiple myeloma. Sci Rep (2019) 9(1):16429. doi: 10.1038/s41598-019-52740-2
58. McBrayer SK, Cheng JC, Singhal S, Krett NL, Rosen ST, Shanmugam M. Multiple myeloma exhibits novel dependence on Glut4, Glut8, and Glut11: implications for glucose transporter-directed therapy. Blood (2012) 119(20):4686–97. doi: 10.1182/blood-2011-09-377846
59. Mueckler M, Thorens B. The Slc2 (Glut) family of membrane transporters. Mol Aspects Med (2013) 34(2-3):121–38. doi: 10.1016/j.mam.2012.07.001
60. D'Souza L, Bhattacharya D. Plasma cells: you are what you eat. Immunol Rev (2019) 288(1):161–77. doi: 10.1111/imr.12732
61. Matsumoto T, Jimi S, Migita K, Takamatsu Y, Hara S. Inhibition of glucose transporter 1 induces apoptosis and sensitizes multiple myeloma cells to conventional chemotherapeutic agents. Leuk Res (2016) 41:103–10. doi: 10.1016/j.leukres.2015.12.008
62. Dalva-Aydemir S, Bajpai R, Martinez M, Adekola KU, Kandela I, Wei C, et al. Targeting the metabolic plasticity of multiple myeloma with fda-approved ritonavir and metformin. Clin Cancer Res (2015) 21(5):1161–71. doi: 10.1158/1078-0432.CCR-14-1088
63. Rodon J, Dienstmann R, Serra V, Tabernero J. Development of Pi3k inhibitors: lessons learned from early clinical trials. Nat Rev Clin Oncol (2013) 10(3):143–53. doi: 10.1038/nrclinonc.2013.10
64. Hennessy BT, Smith DL, Ram PT, Lu Y, Mills GB. Exploiting the Pi3k/Akt pathway for cancer drug discovery. Nat Rev Drug Discovery (2005) 4(12):988–1004. doi: 10.1038/nrd1902
65. Parsons R. Human cancer, pten and the pi-3 kinase pathway. Semin Cell Dev Biol (2004) 15(2):171–6. doi: 10.1016/j.semcdb.2003.12.021
66. Lee JY, Engelman JA, Cantley LC. Biochemistry. Pi3k Charges Ahead Sci (2007) 317(5835):206–7. doi: 10.1126/science.1146073
67. Dimopoulos MA, Kastritis E, Roussou M, Gkotzamanidou M, Migkou M, Gavriatopoulou M, et al. Elevated serum lactate dehydrogenase (Ldh) should be included among the variables which define high risk multiple myeloma. Blood (2010) 116(21):2969–. doi: 10.1182/blood.V116.21.2969.2969
68. Theodoropoulos VE, Lazaris A, Sofras F, Gerzelis I, Tsoukala V, Ghikonti I, et al. Hypoxia-inducible factor 1 alpha expression correlates with angiogenesis and unfavorable prognosis in bladder cancer. Eur Urol (2004) 46(2):200–8. doi: 10.1016/j.eururo.2004.04.008
69. Baba Y, Nosho K, Shima K, Irahara N, Chan AT, Meyerhardt JA, et al. Hif1a overexpression is associated with poor prognosis in a cohort of 731 colorectal cancers. Am J Pathol (2010) 176(5):2292–301. doi: 10.2353/ajpath.2010.090972
70. Rellinger EJ, Craig BT, Alvarez AL, Dusek HL, Kim KW, Qiao J, et al. Fx11 inhibits aerobic glycolysis and growth of neuroblastoma cells. Surgery (2017) 161(3):747–52. doi: 10.1016/j.surg.2016.09.009
71. Muz B, Wasden K, Alhallak K, Jeske A, Azab F, Sun J, et al. Inhibition of hif-1a by px-478 normalizes blood vessels, improves drug delivery and suppresses progression and dissemination in multiple myeloma. Blood (2020) 136(Supplement 1):3. doi: 10.1182/blood-2020-142154
72. Vyas AK, Koster JC, Tzekov A, Hruz PW. Effects of the hiv protease inhibitor ritonavir on Glut4 knock-out mice. J Biol Chem (2010) 285(47):36395–400. doi: 10.1074/jbc.M110.176321
73. Demel HR, Feuerecker B, Piontek G, Seidl C, Blechert B, Pickhard A, et al. Effects of topoisomerase inhibitors that induce DNA damage response on glucose metabolism and Pi3k/Akt/Mtor signaling in multiple myeloma cells. Am J Cancer Res (2015) 5(5):1649–64.
74. Ojelabi OA, Lloyd KP, Simon AH, De Zutter JK, Carruthers A. Wzb117 (2-Fluoro-6-(M-Hydroxybenzoyloxy) phenyl m-hydroxybenzoate) inhibits Glut1-mediated sugar transport by binding reversibly at the exofacial sugar binding site. J Biol Chem (2016) 291(52):26762–72. doi: 10.1074/jbc.M116.759175
75. Liu Y, Cao Y, Zhang W, Bergmeier S, Qian Y, Akbar H, et al. A small-molecule inhibitor of glucose transporter 1 downregulates glycolysis, induces cell-cycle arrest, and inhibits cancer cell growth in vitro and in vivo. Mol Cancer Ther (2012) 11(8):1672–82. doi: 10.1158/1535-7163.MCT-12-0131
76. Semenza GL. Hif-1 mediates metabolic responses to intratumoral hypoxia and oncogenic mutations. J Clin Invest (2013) 123(9):3664–71. doi: 10.1172/JCI67230
77. Effenberger M, Bommert KS, Kunz V, Kruk J, Leich E, Rudelius M, et al. Glutaminase inhibition in multiple myeloma induces apoptosis Via myc degradation. Oncotarget (2017) 8(49):85858–67. doi: 10.18632/oncotarget.20691
78. Chen L, Cui H. Targeting glutamine induces apoptosis: a cancer therapy approach. Int J Mol Sci (2015) 16(9):22830–55. doi: 10.3390/ijms160922830
79. Thompson RM, Dytfeld D, Reyes L, Robinson RM, Smith B, Manevich Y, et al. Glutaminase inhibitor Cb-839 synergizes with carfilzomib in resistant multiple myeloma cells. Oncotarget (2017) 8(22):35863–76. doi: 10.18632/oncotarget.16262
80. Faict S, Oudaert I, D'Auria L, Dehairs J, Maes K, Vlummens P, et al. The transfer of sphingomyelinase contributes to drug resistance in multiple myeloma. Cancers (Basel) (2019) 11(12). doi: 10.3390/cancers11121823
81. Bolzoni M, Chiu M, Accardi F, Vescovini R, Airoldi I, Storti P, et al. Dependence on glutamine uptake and glutamine addiction characterize myeloma cells: a new attractive target. Blood (2016) 128(5):667–79. doi: 10.1182/blood-2016-01-690743
82. Pochini L, Scalise M, Galluccio M, Indiveri C. Membrane transporters for the special amino acid glutamine: Structure/Function relationships and relevance to human health. Front Chem (2014) 2:61. doi: 10.3389/fchem.2014.00061
83. Prelowska MK, Mehlich D, Ugurlu MT, Kedzierska H, Cwiek A, Kosnik A, et al. Inhibition of the l-glutamine transporter Asct2 sensitizes plasma cell myeloma cells to proteasome inhibitors. Cancer Lett (2021) 507:13–25. doi: 10.1016/j.canlet.2021.02.020
84. Isoda A, Kaira K, Iwashina M, Oriuchi N, Tominaga H, Nagamori S, et al. Expression of l-type amino acid transporter 1 (Lat1) as a prognostic and therapeutic indicator in multiple myeloma. Cancer Sci (2014) 105(11):1496–502. doi: 10.1111/cas.12529
85. Tirado-Velez JM, Joumady I, Saez-Benito A, Cozar-Castellano I, Perdomo G. Inhibition of fatty acid metabolism reduces human myeloma cells proliferation. PloS One (2012) 7(9):e46484. doi: 10.1371/journal.pone.0046484
86. Christofk HR, Vander Heiden MG, Harris MH, Ramanathan A, Gerszten RE, Wei R, et al. The M2 splice isoform of pyruvate kinase is important for cancer metabolism and tumour growth. Nature (2008) 452(7184):230–3. doi: 10.1038/nature06734
87. Liu Y, Wu K, Shi L, Xiang F, Tao K, Wang G. Prognostic significance of the metabolic marker hexokinase-2 in various solid tumors: a meta-analysis. PloS One (2016) 11(11):e0166230. doi: 10.1371/journal.pone.0166230
88. Nakano A, Miki H, Nakamura S, Harada T, Oda A, Amou H, et al. Up-regulation of hexokinaseii in myeloma cells: targeting myeloma cells with 3-bromopyruvate. J Bioenerg Biomembr (2012) 44(1):31–8. doi: 10.1007/s10863-012-9412-9
89. Nelson JA, Falk RE. The efficacy of phloridzin and phloretin on tumor cell growth. Anticancer Res (1993) 13(6A):2287–92.
90. Kobori M, Shinmoto H, Tsushida T, Shinohara K. Phloretin-induced apoptosis in B16 melanoma 4a5 cells by inhibition of glucose transmembrane transport. Cancer Lett (1997) 119(2):207–12. doi: 10.1016/s0304-3835(97)00271-1
91. Cao X, Fang L, Gibbs S, Huang Y, Dai Z, Wen P, et al. Glucose uptake inhibitor sensitizes cancer cells to daunorubicin and overcomes drug resistance in hypoxia. Cancer Chemother Pharmacol (2007) 59(4):495–505. doi: 10.1007/s00280-006-0291-9
92. Yuneva M, Zamboni N, Oefner P, Sachidanandam R, Lazebnik Y. Deficiency in glutamine but not glucose induces myc-dependent apoptosis in human cells. J Cell Biol (2007) 178(1):93–105. doi: 10.1083/jcb.200703099
93. Bajpai R, Matulis SM, Wei C, Nooka AK, Von Hollen HE, Lonial S, et al. Targeting glutamine metabolism in multiple myeloma enhances bim binding to bcl-2 eliciting synthetic lethality to venetoclax. Oncogene (2016) 35(30):3955–64. doi: 10.1038/onc.2015.464
94. Wise DR, DeBerardinis RJ, Mancuso A, Sayed N, Zhang XY, Pfeiffer HK, et al. Myc regulates a transcriptional program that stimulates mitochondrial glutaminolysis and leads to glutamine addiction. Proc Natl Acad Sci U.S.A. (2008) 105(48):18782–7. doi: 10.1073/pnas.0810199105
95. Gonsalves WI, Ramakrishnan V, Hitosugi T, Ghosh T, Jevremovic D, Dutta T, et al. Glutamine-derived 2-hydroxyglutarate is associated with disease progression in plasma cell malignancies. JCI Insight (2018) 3(1). doi: 10.1172/jci.insight.94543
96. Steiner N, Muller U, Hajek R, Sevcikova S, Borjan B, Johrer K, et al. The metabolomic plasma profile of myeloma patients is considerably different from healthy subjects and reveals potential new therapeutic targets. PloS One (2018) 13(8):e0202045. doi: 10.1371/journal.pone.0202045
97. Jurczyszyn A, Czepiel J, Gdula-Argasinska J, Pasko P, Czapkiewicz A, Librowski T, et al. Plasma fatty acid profile in multiple myeloma patients. Leuk Res (2015) 39(4):400–5. doi: 10.1016/j.leukres.2014.12.010
98. Chen J, Zaal EA, Berkers CR, Ruijtenbeek R, Garssen J, Redegeld FA. Omega-3 fatty acids dha and epa reduce bortezomib resistance in multiple myeloma cells by promoting glutathione degradation. Cells-Basel (2021) 10(9). doi: 10.3390/cells10092287
99. Wang WQ, Zhao XY, Wang HY, Liang Y. Increased fatty acid synthase as a potential therapeutic target in multiple myeloma. J Zhejiang Univ Sci B (2008) 9(6):441–7. doi: 10.1631/jzus.B0740640
100. Li Z, Liu H, He J, Wang Z, Yin Z, You G, et al. Acetyl-coa synthetase 2: a critical linkage in obesity-induced tumorigenesis in myeloma. Cell Metab (2021) 33(1):78–93 e7. doi: 10.1016/j.cmet.2020.12.011
101. Berge K, Tronstad KJ, Bohov P, Madsen L, Berge RK. Impact of mitochondrial beta-oxidation in fatty acid-mediated inhibition of glioma cell proliferation. J Lipid Res (2003) 44(1):118–27. doi: 10.1194/jlr.m200312-jlr200
102. Samudio I, Harmancey R, Fiegl M, Kantarjian H, Konopleva M, Korchin B, et al. Pharmacologic inhibition of fatty acid oxidation sensitizes human leukemia cells to apoptosis induction. J Clin Invest (2010) 120(1):142–56. doi: 10.1172/JCI38942
103. Terunuma A, Putluri N, Mishra P, Mathe EA, Dorsey TH, Yi M, et al. Myc-driven accumulation of 2-hydroxyglutarate is associated with breast cancer prognosis. J Clin Invest (2014) 124(1):398–412. doi: 10.1172/JCI71180
104. Xiao R, Cerny J, Devitt K, Dresser K, Nath R, Ramanathan M, et al. Myc protein expression is detected in plasma cell myeloma but not in monoclonal gammopathy of undetermined significance (Mgus). Am J Surg Pathol (2014) 38(6):776–83. doi: 10.1097/PAS.0000000000000213
105. Shi Y, Sun F, Cheng Y, Holmes B, Dhakal B, Gera JF, et al. Critical role for cap-independent c-myc translation in progression of multiple myeloma. Mol Cancer Ther (2022) 21(4):502–10. doi: 10.1158/1535-7163.MCT-21-0016
106. Tambay V, Raymond VA, Bilodeau M. Myc rules: leading glutamine metabolism toward a distinct cancer cell phenotype. Cancers (Basel) (2021) 13(17). doi: 10.3390/cancers13174484
107. Chng WJ, Huang GF, Chung TH, Ng SB, Gonzalez-Paz N, Troska-Price T, et al. Clinical and biological implications of myc activation: a common difference between mgus and newly diagnosed multiple myeloma. Leukemia (2011) 25(6):1026–35. doi: 10.1038/leu.2011.53
108. Ratcliffe PJ. Oxygen sensing and hypoxia signalling pathways in animals: the implications of physiology for cancer. J Physiol (2013) 591(8):2027–42. doi: 10.1113/jphysiol.2013.251470
109. Lomas OC, Tahri S, Ghobrial IM. The microenvironment in myeloma. Curr Opin Oncol (2020) 32(2):170–5. doi: 10.1097/CCO.0000000000000615
110. de Jong MME, Kellermayer Z, Papazian N, Tahri S, Hofste Op Bruinink D, Hoogenboezem R, et al. The multiple myeloma microenvironment is defined by an inflammatory stromal cell landscape. Nat Immunol (2021) 22(6):769–80. doi: 10.1038/s41590-021-00931-3
111. Hayashi Y, Yokota A, Harada H, Huang G. Hypoxia/Pseudohypoxia-mediated activation of hypoxia-inducible factor-1alpha in cancer. Cancer Sci (2019) 110(5):1510–7. doi: 10.1111/cas.13990
112. Borsi E, Perrone G, Terragna C, Martello M, Dico AF, Solaini G, et al. Hypoxia inducible factor-1 alpha as a therapeutic target in multiple myeloma. Oncotarget (2014) 5(7):1779–92. doi: 10.18632/oncotarget.1736
113. Semenza GL. Hypoxia-inducible factors in physiology and medicine. Cell (2012) 148(3):399–408. doi: 10.1016/j.cell.2012.01.021
114. Kim JW, Gao P, Liu YC, Semenza GL, Dang CV. Hypoxia-inducible factor 1 and dysregulated c-myc cooperatively induce vascular endothelial growth factor and metabolic switches hexokinase 2 and pyruvate dehydrogenase kinase 1. Mol Cell Biol (2007) 27(21):7381–93. doi: 10.1128/MCB.00440-07
115. Bhaskar A, Tiwary BN. Hypoxia inducible factor-1 alpha and multiple myeloma. Int J Adv Res (Indore) (2016) 4(1):706–15.
116. Storti P, Bolzoni M, Donofrio G, Airoldi I, Guasco D, Toscani D, et al. Hypoxia-inducible factor (Hif)-1alpha suppression in myeloma cells blocks tumoral growth in vivo inhibiting angiogenesis and bone destruction. Leukemia (2013) 27(8):1697–706. doi: 10.1038/leu.2013.24
117. Vacca A, Scavelli C, Montefusco V, Di Pietro G, Neri A, Mattioli M, et al. Thalidomide downregulates angiogenic genes in bone marrow endothelial cells of patients with active multiple myeloma. J Clin Oncol (2005) 23(23):5334–46. doi: 10.1200/JCO.2005.03.723
118. Dmoszynska A, Bojarska-Junak A, Domanski D, Rolinski J, Hus M, Soroka-Wojtaszko M. Production of proangiogenic cytokines during thalidomide treatment of multiple myeloma. Leuk Lymphoma (2002) 43(2):401–6. doi: 10.1080/10428190290006224
119. Neben K, Moehler T, Egerer G, Kraemer A, Hillengass J, Benner A, et al. High plasma basic fibroblast growth factor concentration is associated with response to thalidomide in progressive multiple myeloma. Clin Cancer Res (2001) 7(9):2675–81.
120. Terpos E, Anargyrou K, Katodritou E, Kastritis E, Papatheodorou A, Christoulas D, et al. Circulating angiopoietin-1 to angiopoietin-2 ratio is an independent prognostic factor for survival in newly diagnosed patients with multiple myeloma who received therapy with novel antimyeloma agents. Int J Cancer (2012) 130(3):735–42. doi: 10.1002/ijc.26062
121. Zhang J, Sattler M, Tonon G, Grabher C, Lababidi S, Zimmerhackl A, et al. Targeting angiogenesis via a c-Myc/Hypoxia-Inducible factor-1alpha-Dependent pathway in multiple myeloma. Cancer Res (2009) 69(12):5082–90. doi: 10.1158/0008-5472.CAN-08-4603
122. Bhaskar A, Gupta R, Kumar L, Sharma A, Sharma MC, Kalaivani M, et al. Circulating endothelial progenitor cells as potential prognostic biomarker in multiple myeloma. Leuk Lymphoma (2012) 53(4):635–40. doi: 10.3109/10428194.2011.628880
123. Zhang H, Vakil V, Braunstein M, Smith EL, Maroney J, Chen L, et al. Circulating endothelial progenitor cells in multiple myeloma: implications and significance. Blood (2005) 105(8):3286–94. doi: 10.1182/blood-2004-06-2101
124. Pour L, Svachova H, Adam Z, Almasi M, Buresova L, Buchler T, et al. Levels of angiogenic factors in patients with multiple myeloma correlate with treatment response. Ann Hematol (2010) 89(4):385–9. doi: 10.1007/s00277-009-0834-3
125. Colla S, Tagliaferri S, Morandi F, Lunghi P, Donofrio G, Martorana D, et al. The new tumor-suppressor gene inhibitor of growth family member 4 (Ing4) regulates the production of proangiogenic molecules by myeloma cells and suppresses hypoxia-inducible factor-1 alpha (Hif-1alpha) activity: involvement in myeloma-induced angiogenesis. Blood (2007) 110(13):4464–75. doi: 10.1182/blood-2007-02-074617
126. Mulligan G, Mitsiades C, Bryant B, Zhan F, Chng WJ, Roels S, et al. Gene expression profiling and correlation with outcome in clinical trials of the proteasome inhibitor bortezomib. Blood (2007) 109(8):3177–88. doi: 10.1182/blood-2006-09-044974
127. Chng WJ, Kumar S, Vanwier S, Ahmann G, Price-Troska T, Henderson K, et al. Molecular dissection of hyperdiploid multiple myeloma by gene expression profiling. Cancer Res (2007) 67(7):2982–9. doi: 10.1158/0008-5472.CAN-06-4046
128. Storti P, Toscani D, Airoldi I, Marchica V, Maiga S, Bolzoni M, et al. The anti-tumoral effect of lenalidomide is increased in vivo by hypoxia-inducible factor (Hif)-1alpha inhibition in myeloma cells. Haematologica (2016) 101(3):e107–10. doi: 10.3324/haematol.2015.133736
129. Noman MZ, Hasmim M, Lequeux A, Xiao M, Duhem C, Chouaib S, et al. Improving cancer immunotherapy by targeting the hypoxic tumor microenvironment: new opportunities and challenges. Cells-Basel (2019) 8(9). doi: 10.3390/cells8091083
130. Chouaib S, Messai Y, Couve S, Escudier B, Hasmim M, Noman MZ. Hypoxia promotes tumor growth in linking angiogenesis to immune escape. Front Immunol (2012) 3:21. doi: 10.3389/fimmu.2012.00021
131. Noman MZ, Buart S, Romero P, Ketari S, Janji B, Mari B, et al. Hypoxia-inducible mir-210 regulates the susceptibility of tumor cells to lysis by cytotoxic T cells. Cancer Res (2012) 72(18):4629–41. doi: 10.1158/0008-5472.CAN-12-1383
132. Ikeda S, Kitadate A, Abe F, Saitoh H, Michishita Y, Hatano Y, et al. Hypoxia-inducible microrna-210 regulates the Dimt1-Irf4 oncogenic axis in multiple myeloma. Cancer Sci (2017) 108(4):641–52. doi: 10.1111/cas.13183
133. Kocemba KA, van Andel H, de Haan-Kramer A, Mahtouk K, Versteeg R, Kersten MJ, et al. The hypoxia target adrenomedullin is aberrantly expressed in multiple myeloma and promotes angiogenesis. Leukemia (2013) 27(8):1729–37. doi: 10.1038/leu.2013.76
134. Zahoor M, Westhrin M, Aass KR, Moen SH, Misund K, Psonka-Antonczyk KM, et al. Hypoxia promotes il-32 expression in myeloma cells, and high expression is associated with poor survival and bone loss. Blood Adv (2017) 1(27):2656–66. doi: 10.1182/bloodadvances.2017010801
135. Xu Y, Guo J, Liu J, Xie Y, Li X, Jiang H, et al. Hypoxia-induced creb cooperates mmset to modify chromatin and promote Dkk1 expression in multiple myeloma. Oncogene (2021) 40(7):1231–41. doi: 10.1038/s41388-020-01590-8
136. Shin DH, Chun YS, Lee DS, Huang LE, Park JW. Bortezomib inhibits tumor adaptation to hypoxia by stimulating the fih-mediated repression of hypoxia-inducible factor-1. Blood (2008) 111(6):3131–6. doi: 10.1182/blood-2007-11-120576
137. Yun Z, Zhichao J, Hao Y, Ou J, Ran Y, Wen D, et al. Targeting autophagy in multiple myeloma. Leuk Res (2017) 59:97–104. doi: 10.1016/j.leukres.2017.06.002
138. Janker L, Mayer RL, Bileck A, Kreutz D, Mader JC, Utpatel K, et al. Metabolic, anti-apoptotic and immune evasion strategies of primary human myeloma cells indicate adaptations to hypoxia. Mol Cell Proteomics (2019) 18(5):936–53. doi: 10.1074/mcp.RA119.001390
139. Rana PS, Soler DC, Kort J, Driscoll JJ. Targeting tgf-B signaling in the multiple myeloma microenvironment: steering cars and T cells in the right direction. Front Cell Dev Biol (2022) 10:1059715. doi: 10.3389/fcell.2022.1059715
140. Leone RD, Powell JD. Metabolism of immune cells in cancer. Nat Rev Cancer (2020) 20(9):516–31. doi: 10.1038/s41568-020-0273-y
141. Liu Y, Liang X, Dong W, Fang Y, Lv J, Zhang T, et al. Tumor-repopulating cells induce pd-1 expression in Cd8(+) T cells by transferring kynurenine and ahr activation. Cancer Cell (2018) 33(3):480–94 e7. doi: 10.1016/j.ccell.2018.02.005
142. Labadie BW, Bao R, Luke JJ. Reimagining ido pathway inhibition in cancer immunotherapy Via downstream focus on the tryptophan-Kynurenine-Aryl hydrocarbon axis. Clin Cancer Res (2019) 25(5):1462–71. doi: 10.1158/1078-0432.CCR-18-2882
143. Zhai L, Spranger S, Binder DC, Gritsina G, Lauing KL, Giles FJ, et al. Molecular pathways: targeting Ido1 and other tryptophan dioxygenases for cancer immunotherapy. Clin Cancer Res (2015) 21(24):5427–33. doi: 10.1158/1078-0432.CCR-15-0420
144. Hornyak L, Dobos N, Koncz G, Karanyi Z, Pall D, Szabo Z, et al. The role of indoleamine-2,3-Dioxygenase in cancer development, diagnostics, and therapy. Front Immunol (2018) 9:151. doi: 10.3389/fimmu.2018.00151
145. Beckermann KE, Hongo R, Ye X, Young K, Carbonell K, Healey DCC, et al. Cd28 costimulation drives tumor-infiltrating T cell glycolysis to promote inflammation. JCI Insight (2020) 5(16). doi: 10.1172/jci.insight.138729
146. El Arfani C, De Veirman K, Maes K, De Bruyne E, Menu E. Metabolic features of multiple myeloma. Int J Mol Sci (2018) 19(4). doi: 10.3390/ijms19041200
147. Luengo A, Gui DY, Vander Heiden MG. Targeting metabolism for cancer therapy. Cell Chem Biol (2017) 24(9):1161–80. doi: 10.1016/j.chembiol.2017.08.028
148. Anderson KG, Stromnes IM, Greenberg PD. Obstacles posed by the tumor microenvironment to T cell activity: a case for synergistic therapies. Cancer Cell (2017) 31(3):311–25. doi: 10.1016/j.ccell.2017.02.008
149. Noman MZ, Desantis G, Janji B, Hasmim M, Karray S, Dessen P, et al. Pd-L1 is a novel direct target of hif-1alpha, and its blockade under hypoxia enhanced mdsc-mediated T cell activation. J Exp Med (2014) 211(5):781–90. doi: 10.1084/jem.20131916
150. Shurin MR, Umansky V. Cross-talk between hif and pd-1/Pd-L1 pathways in carcinogenesis and therapy. J Clin Invest (2022) 132(9). doi: 10.1172/JCI159473
151. Paiva B, Azpilikueta A, Puig N, Ocio EM, Sharma R, Oyajobi BO, et al. Pd-L1/Pd-1 presence in the tumor microenvironment and activity of pd-1 blockade in multiple myeloma. Leukemia (2015) 29(10):2110–3. doi: 10.1038/leu.2015.79
152. Jelinek T, Paiva B, Hajek R. Update on pd-1/Pd-L1 inhibitors in multiple myeloma. Front Immunol (2018) 9:2431. doi: 10.3389/fimmu.2018.02431
153. Benson DM Jr., Bakan CE, Mishra A, Hofmeister CC, Efebera Y, Becknell B, et al. The pd-1/Pd-L1 axis modulates the natural killer cell versus multiple myeloma effect: a therapeutic target for ct-011, a novel monoclonal anti-Pd-1 antibody. Blood (2010) 116(13):2286–94. doi: 10.1182/blood-2010-02-271874
154. Riera-Domingo C, Audige A, Granja S, Cheng WC, Ho PC, Baltazar F, et al. Immunity, hypoxia, and metabolism-the menage a trois of cancer: implications for immunotherapy. Physiol Rev (2020) 100(1):1–102. doi: 10.1152/physrev.00018.2019
155. Li H, van der Leun AM, Yofe I, Lubling Y, Gelbard-Solodkin D, van Akkooi ACJ, et al. Dysfunctional Cd8 T cells form a proliferative, dynamically regulated compartment within human melanoma. Cell (2019) 176(4):775–89 e18. doi: 10.1016/j.cell.2018.11.043
156. van der Windt GJ, O'Sullivan D, Everts B, Huang SC, Buck MD, Curtis JD, et al. Cd8 memory T cells have a bioenergetic advantage that underlies their rapid recall ability. Proc Natl Acad Sci USA (2013) 110(35):14336–41. doi: 10.1073/pnas.1221740110
157. Pan Y, Tian T, Park CO, Lofftus SY, Mei S, Liu X, et al. Survival of tissue-resident memory T cells requires exogenous lipid uptake and metabolism. Nature (2017) 543(7644):252–6. doi: 10.1038/nature21379
158. Colegio OR, Chu NQ, Szabo AL, Chu T, Rhebergen AM, Jairam V, et al. Functional polarization of tumour-associated macrophages by tumour-derived lactic acid. Nature (2014) 513(7519):559–63. doi: 10.1038/nature13490
159. Gajewski TF, Meng Y, Blank C, Brown I, Kacha A, Kline J, et al. Immune resistance orchestrated by the tumor microenvironment. Immunol Rev (2006) 213:131–45. doi: 10.1111/j.1600-065X.2006.00442.x
160. de la Cruz-Lopez KG, Castro-Munoz LJ, Reyes-Hernandez DO, Garcia-Carranca A, Manzo-Merino J. Lactate in the regulation of tumor microenvironment and therapeutic approaches. Front Oncol (2019) 9:1143. doi: 10.3389/fonc.2019.01143
161. Fischer K, Hoffmann P, Voelkl S, Meidenbauer N, Ammer J, Edinger M, et al. Inhibitory effect of tumor cell-derived lactic acid on human T cells. Blood (2007) 109(9):3812–9. doi: 10.1182/blood-2006-07-035972
162. Vigano S, Alatzoglou D, Irving M, Menetrier-Caux C, Caux C, Romero P, et al. Targeting adenosine in cancer immunotherapy to enhance T-cell function. Front Immunol (2019) 10:925. doi: 10.3389/fimmu.2019.00925
163. Mastelic-Gavillet B, Navarro Rodrigo B, Decombaz L, Wang H, Ercolano G, Ahmed R, et al. Adenosine mediates functional and metabolic suppression of peripheral and tumor-infiltrating Cd8(+) T cells. J Immunother Cancer (2019) 7(1):257. doi: 10.1186/s40425-019-0719-5
164. Qin R, Zhao C, Wang CJ, Xu W, Zhao JY, Lin Y, et al. Tryptophan potentiates Cd8(+) T cells against cancer cells by Trip12 tryptophanylation and surface pd-1 downregulation. J Immunother Cancer (2021) 9(7). doi: 10.1136/jitc-2021-002840
165. Ray A, Song Y, Du T, Tai YT, Chauhan D, Anderson KC. Targeting tryptophan catabolic kynurenine pathway enhances antitumor immunity and cytotoxicity in multiple myeloma. Leukemia (2020) 34(2):567–77. doi: 10.1038/s41375-019-0558-x
166. Panchabhai S, Schlam I, Sebastian S, Fonseca R. Pkm2 and other key regulators of warburg effect positively correlate with Cd147 (Emmprin) gene expression and predict survival in multiple myeloma. Leukemia (2017) 31(4):991–4. doi: 10.1038/leu.2016.389
167. Kühnel A, Blau O, Nogai K, Blau IW. The warburg effect in multiple myeloma and its microenvironment. Med Res Arch (2017) 5.
168. Evans LA, Anderson EI, Petterson XM, Kumar S, Gonsalves WI. Disrupting the reverse warburg effect as a therapeutic strategy in multiple myeloma. Blood (2021) 138. doi: 10.1182/blood-2021-147970
170. Liberti MV, Locasale JW. The warburg effect: how does it benefit cancer cells? Trends Biochem Sci (2016) 41(3):211–8. doi: 10.1016/j.tibs.2015.12.001
171. DeBerardinis RJ, Chandel NS. We need to talk about the warburg effect. Nat Metab (2020) 2(2):127–9. doi: 10.1038/s42255-020-0172-2
172. Abdollahi P, Vandsemb EN, Elsaadi S, Rost LM, Yang R, Hjort MA, et al. Phosphatase of regenerating liver-3 regulates cancer cell metabolism in multiple myeloma. FASEB J (2021) 35(3):e21344. doi: 10.1096/fj.202001920RR
173. Vandsemb EN, Rye MB, Steiro IJ, Elsaadi S, Ro TB, Slordahl TS, et al. Prl-3 induces a positive signaling circuit between glycolysis and activation of Stat1/2. FEBS J (2021) 288(23):6700–15. doi: 10.1111/febs.16058
174. Ashton TM, McKenna WG, Kunz-Schughart LA, Higgins GS. Oxidative phosphorylation as an emerging target in cancer therapy. Clin Cancer Res (2018) 24(11):2482–90. doi: 10.1158/1078-0432.CCR-17-3070
175. Driscoll J. The role of the proteasome in cellular protein degradation. Histol Histopathol (1994) 9(1):197–202.
176. Driscoll JJ, Woodle ES. Targeting the Ubiquitin+Proteasome system in solid tumors. Semin Hematol (2012) 49(3):277–83. doi: 10.1053/j.seminhematol.2012.04.002
177. Rousseau A, Bertolotti A. Regulation of proteasome assembly and activity in health and disease. Nat Rev Mol Cell Bio (2018) 19(11):697–712. doi: 10.1038/s41580-018-0040-z
178. Walker BA, Boyle EM, Wardell CP, Murison A, Begum DB, Dahir NM, et al. Mutational spectrum, copy number changes, and outcome: results of a sequencing study of patients with newly diagnosed myeloma. J Clin Oncol (2015) 33(33):3911–20. doi: 10.1200/JCO.2014.59.1503
179. Shirazi F, Jones RJ, Singh RK, Zou J, Kuiatse I, Berkova Z, et al. Activating kras, nras, and braf mutants enhance proteasome capacity and reduce endoplasmic reticulum stress in multiple myeloma. Proc Natl Acad Sci USA (2020) 117(33):20004–14. doi: 10.1073/pnas.2005052117
Keywords: metabolism, multiple myeloma, proteasome inhibitor, oxidative phosphorylation, glycolysis, fatty acid synthesis
Citation: Rana PS, Goparaju K and Driscoll JJ (2023) Shutting off the fuel supply to target metabolic vulnerabilities in multiple myeloma. Front. Oncol. 13:1141851. doi: 10.3389/fonc.2023.1141851
Received: 10 January 2023; Accepted: 18 May 2023;
Published: 08 June 2023.
Edited by:
Manoj Kumar Kashyap, Amity University Gurgaon, IndiaReviewed by:
Magne Børset, Norwegian University of Science and Technology, NorwayMarkus Munder, Johannes Gutenberg University Mainz, Germany
Copyright © 2023 Rana, Goparaju and Driscoll. This is an open-access article distributed under the terms of the Creative Commons Attribution License (CC BY). The use, distribution or reproduction in other forums is permitted, provided the original author(s) and the copyright owner(s) are credited and that the original publication in this journal is cited, in accordance with accepted academic practice. No use, distribution or reproduction is permitted which does not comply with these terms.
*Correspondence: James J. Driscoll, James.driscoll@UHhospitals.org