- Breast Cancer Center, Shandong Cancer Hospital and Institute, Shandong First Medical University and Shandong Academy of Medical Sciences, Jinan, China
Objectives: The purpose of this study was to determine the independent risk factors for bone metastasis in breast cancer and to establish a nomogram to predict the risk of bone metastasis in early stages through clinicopathological characteristics and hematological parameters.
Methods: We selected 1042 patients with breast cancer from the database of Shandong Cancer Hospital for retrospective analysis, and determined independent risk factors for bone metastatic breast cancer (BMBC). A BMBC nomogram based on clinicopathological characteristics and hematological parameters was constructed using logistic regression analysis. The performance of the nomograph was evaluated using the receiver operating characteristic (ROC) and calibration curves. The clinical effect of risk stratification was tested using Kaplan-Meier analysis.
Results: BMBC patients were found to be at risk for eight independent risk factors based on multivariate analysis: age at diagnosis, lymphovascular invasion, pathological stage, pathological node stage, molecular subtype, platelet count/lymphocyte count, platelet count * neutrophil count/lymphocyte count ratio, Systemic Immunological Inflammation Index, and radiotherapy. The prediction accuracy of the BMBC nomogram was good. In the training set, the area under the ROC curve (AUC) was 0.909, and in the validation set, it was 0.926, which proved that our model had good calibration. The risk stratification system can analyze the risk of relapse in individuals into high- and low-risk groups.
Conclusion: The proposed nomogram may predict the possibility of breast cancer bone metastasis, which will help clinicians optimize metastatic breast cancer treatment strategies and monitoring plans to provide patients with better treatment.
1 Introduction
Breast cancer (BC) is the most common malignancy and the leading cause of cancer-related deaths worldwide in women (1). Despite remarkable developments in the systemic treatment of BC, it remains an incurable disease at advanced stages, and the clinical symptoms of metastatic breast cancer (MBC), which exhibit a high degree of heterogeneity, are extremely diverse. The most frequent metastatic location of BC is the bone. Seventy percent of patients with stage IV BC have bone metastases (2), and 17–37% of patients have only bone metastasis (3–5). Patients with bone metastatic breast cancer (BMBC) have an average overall survival of 40 months (6), a 3-year survival rate of 25%, and a 5-year survival rate of 13% (7). Furthermore, adverse skeletal-related events such as hypercalcemia, nerve root or spinal cord compression, fractures, and pain, are frequently caused by bone metastases, and they have a significant negative impact on BC (6).
Distant metastasis-free survival (DMFS) may be affected by clinicopathological factors, including lymphovascular invasion (LVI), Ki-67 expression, human epidermal growth factor receptor 2 (HER-2) status, estrogen (ER) or progesterone (PR) status, lymph node stage, and tumor size. Moreover, hematological indicators, such as platelet count/lymphocyte count ratio (PLR), neutrophil count/lymphocyte count ratio (NLR), and monocyte count/lymphocyte count ratio (MLR), have sufficient prognostic value for the recurrence of several cancers, including gastric cancer (8–10). The efficacy of treatment, and treatment biomarkers (circulating immune cells) have been discussed in previous research. Moreover, prognosis, and survival from cancer have been studied (11–13). Furthermore, the Glasgow Prognostic Score and Systemic Immunological Inflammation Index (SII), which represent the patient’s immune status and degree of inflammation, have been proposed as predictive tools in patients with cancer (14, 15). In clinical practice, it is generally believed that an increase in inflammatory markers in the systemic circulation is a prognostic indicator for many cancers (16).
Currently, the independent risk factors for bone metastatic breast cancer (BMBC) are inconsistent, and a dedicated prediction tool for BMBC is lacking. A nomogram is a reliable and convenient prognostic tool, and it is widely used in oncology prediction because of its incorporation of quantitative analysis of risk variables (17–19). However, since the prognostic nomograms of patients with MBC were created using mostly the Surveillance, Epidemiology, and End Results (SEER) database, the possibility of extending these nomograms to the Chinese population is unclear (20, 21). Chinese women have various clinicopathological and ethnic characteristics and health insurance plans. Thus, the accurate prediction of DMFS in Chinese patients with BC may improve their monitoring and treatment options. This study had two purposes: first, to identify factors associated with bone metastasis of breast cancer; and second, to establish a nomogram predicting bone metastasis of early breast cancer through clinicopathological features and hematological parameters.
2 Methods
2.1 Patient population
From 2010 to 2020, we retrospectively evaluated the relationship between hematological parameters, clinicopathological characteristics, and MBC of BC patients at the Shandong Cancer Hospital. We adopted the following inclusion criteria: (1) female patients with BC, (2) the person receiving the operation; (3) patients with available follow-up information; (4) no preoperative chemotherapy or radiotherapy (5) no blood transfusion or other anti-cancer treatment before blood examination; and (6) complete analysis of hematological parameters from admission to the day before surgery. The exclusion criteria were as follows: (1) bilateral primary BC, (2) patients with primary or occult BC (T0), (3) incomplete medical records, (4) patients with distant metastasis at the first visit, and (5) patients with autoimmune diseases or aggressive viral infections (such as HIV or hepatitis). A total of 1042 patients were included in the study, and 730 were randomly assigned to the training set and 312 to the validation set.
2.2 Clinical variables
The following clinical and pathological information were obtained from medical records: surgery, age at diagnosis, LVI, body mass index (BMI), pathological tumor grade, pathological (pT) stage (T1, T2, T3), pathological node (pN) stage (N0, N1, N2, N3), ER status, PR status, HER2 status, molecular subtype (Luminal A, Luminal B(HER2-), Luminal B(HER2+), HER2-enriched, TNBC), clinical treatment, timing of bone metastases, number of bone metastasis sites and follow-up. Oligometastases of breast cancer bone metastases are usually defined as the number of metastatic sites ≤3 and no distant visceral metastases. Platelet, neutrophil, monocyte, and lymphocyte counts are examples of obtained hematological parameters. The correlation of hematological indicators was described using the following terminology: NLR, MLR, PLR, SII, and lymphocyte count/monocyte count ratio (LMR). These parameters were taken from the last hematological examination before surgery. Molecular subtype was performed according to the 2021 St. Gallen Breast Cancer Guidelines. We obtained postoperative pathological specimens from the patients and performed immunohistochemical analysis. All analyses were performed in the ancillary department of our institution.
2.3 Statistical analysis
The ideal cut off levels for NLR, MLR, PLR, SII, and LMR were identified using ROC curve analysis. A ratio of 7:3 was used to divide all patients into training and verification sets. In univariate analysis, categorical variables were compared using the Pearson chi-square test and Fisher’s exact probability test, while quantitative data with normal or abnormal distributions were subjected to the t-test or Wilcoxon rank test. Logistic regression was used to perform a multivariate analysis, and factors with statistical significance (P< 0.05) were considered. The prediction model was then created using binary logistic regression and significant variables from multivariate analysis. In order to identify independent risk factors for BMBC, a nomogram of BMBC was established based on multivariate logistic regression (P< 0.05). ROC curves were used to assess the area under the curve (AUC) of the nomogram. An AUC value closer to 1 indicates more accurate risk prediction. Calibration curves of the prediction model were drawn to assess the compatibility between predicted BMBC probability and observation probability. BMBC probability is displayed on the y-axis of the calibration curve, while e x-axis of the calibration curve shows the BMBC probability predicted by the training and verification sets. R4.2.2 and SPSS version 26.0 (SPSS, Chicago, Illinois, USA) were used to analyze the data (R Statistical Computation Project, www.r-Project.org). Finally, a risk classification model was developed based on the overall nomogram score of each patient. To categorize the patients into low- and high-risk groups, we used the nomogram’s median risk score as the dividing line. BMBC probabilities for various risk categories were compared using the Kaplan-Meier method. P< 0.05 was set as the threshold for statistical significance.
3 Results
3.1 Patient characteristics
A total of 1043 women with BC between 2010 and 2020 were enrolled (training set, 730 patients; validation set, 312 patients). A median follow-up time of 73 months was observed in the training set and 71 months in the validation set. As shown in Table 1, the training and validation sets have the following baseline clinical characteristics. In the training set, the median age at diagnosis was 45 years (range, 18–76 years), and the median BMI was 24.02 (range 13.93 to 34.77). Overall, 668 (91.51%) patients underwent modified radical mastectomy, and 62 (8.49%) underwent breast-conserving surgery. Vascular tumor thrombus infiltration was observed in 75 (10.27%) patients. In the T stage, 461 (63.15%), 247 (33.84%), and 22 (3.01%) cases corresponded to T1, T2, and T3, respectively. In the N stage, N0, N1, N2, and N3 occurred in 449 (61.51%), 179 (24.52%), 52 (7.12%), and 50 (6.85%) cases, respectively. Regarding molecular subtype, LuminalB(HER2-)patients accounted for the highest proportion (40.82%), followed by LuminalA patients (27.67%), LuminalB (HER2+) patients (14.11%), TNBC patients (9.45%) and HER2-enriched patients (7.95%). ROC and cutoff values for MLR, LMR, SII, NLR, and PLR in patients with BC after surgery are presented in Figure 1 and Table 2. The best cutoff point was used for subsequent statistical analysis.
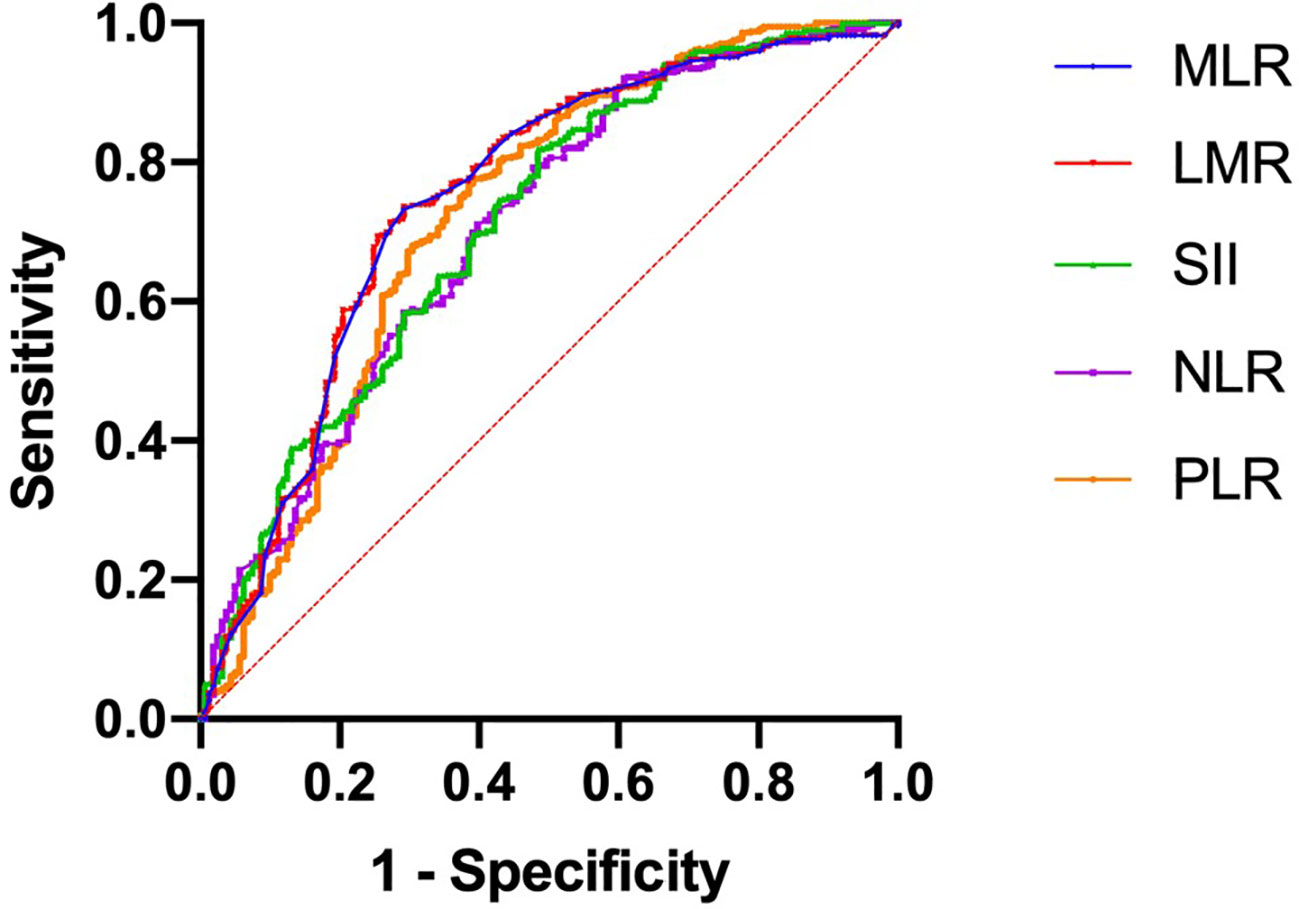
Figure 1 Receiver operating characteristic curves were generated to evaluate the cutoff value of the hematological parameters.
3.2 Univariate and multivariate analysis
In univariate analysis, age at diagnosis, LVI, grade, pT stage, pN stage, molecular subtype, PLR, NLR, SII, LMR, MLR, and radiotherapy were correlated with BMBC. In multivariate logistic regression analysis, age at diagnosis, LVI, pT stage, pN stage, molecular subtype, PLR, SII and radiotherapy were found to be independent predictors. These variables were used to construct the nomogram. The results of the univariate and multivariate analysis are shown in Table 3.
3.3 Nomogram development and validation
In the nomogram, the scores for each factor on the coordinate axis were added to obtain the total BMBC recurrence risk score (Figure 2). In our study, age, N stage, and T stage had the greatest impact on the prediction results, followed by radiotherapy, molecular subtype, LVI, PLR and SII. The nomogram of BMBC shows excellent prediction accuracy. We validated our model using calibration curves, and both the training set (Figure 3A) and the verification set (Figure 3B) show that our model has good calibration. Subsequently, we drew the ROC curve of the prediction probability and calculated the AUC values of the development and verification groups. The AUC values in the training (Figure 3C) and verification (Figure 3D) sets were 0.909 and 0.926, respectively. Thus, the nomogram predictions and actual BMBC are in good agreement. According to the nomogram, all patients were divided into two risk levels: a low-risk group (≤95.9), and a high-risk group (>95.9). In addition, Kaplan Meier analysis showed that risk stratification based on nomograms could accurately distinguish BMBC with different DMFSs. The low-risk patients had significantly better DMFS than the high-risk patients (Figure 4). Even when stratified analyzed for different subtypes of patients, the Kaplan-Meier curve showed significant differences in metastasis rates between the high-risk and low-risk groups (Figure 5).
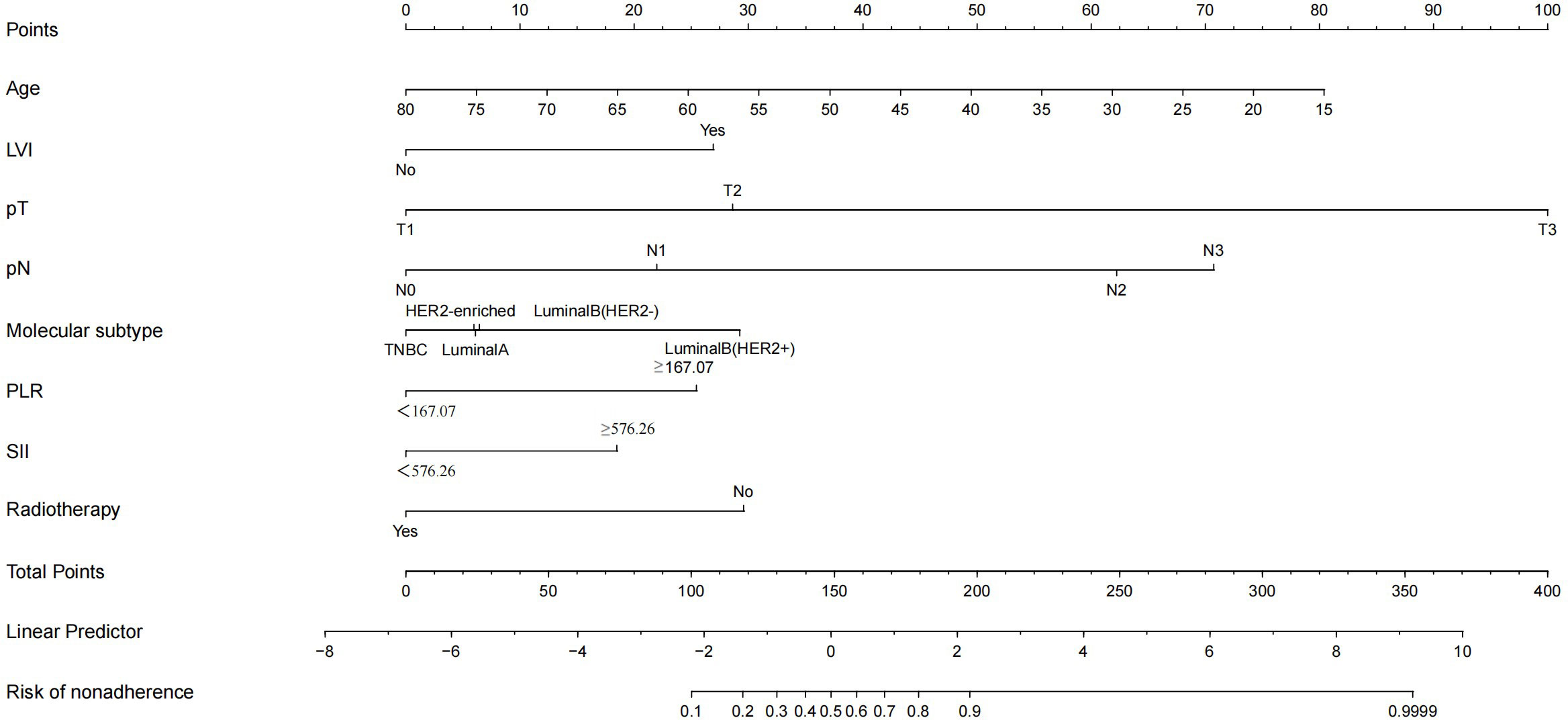
Figure 2 Nomogram to predict the probability of bone metastasis in a patient with non-metastatic breast cancer. Draw a line from each variable’s position to the point axis; calculate the points of different variables and add them to generate a total score, and convert them into BMBC prediction probability according to the nomogram.
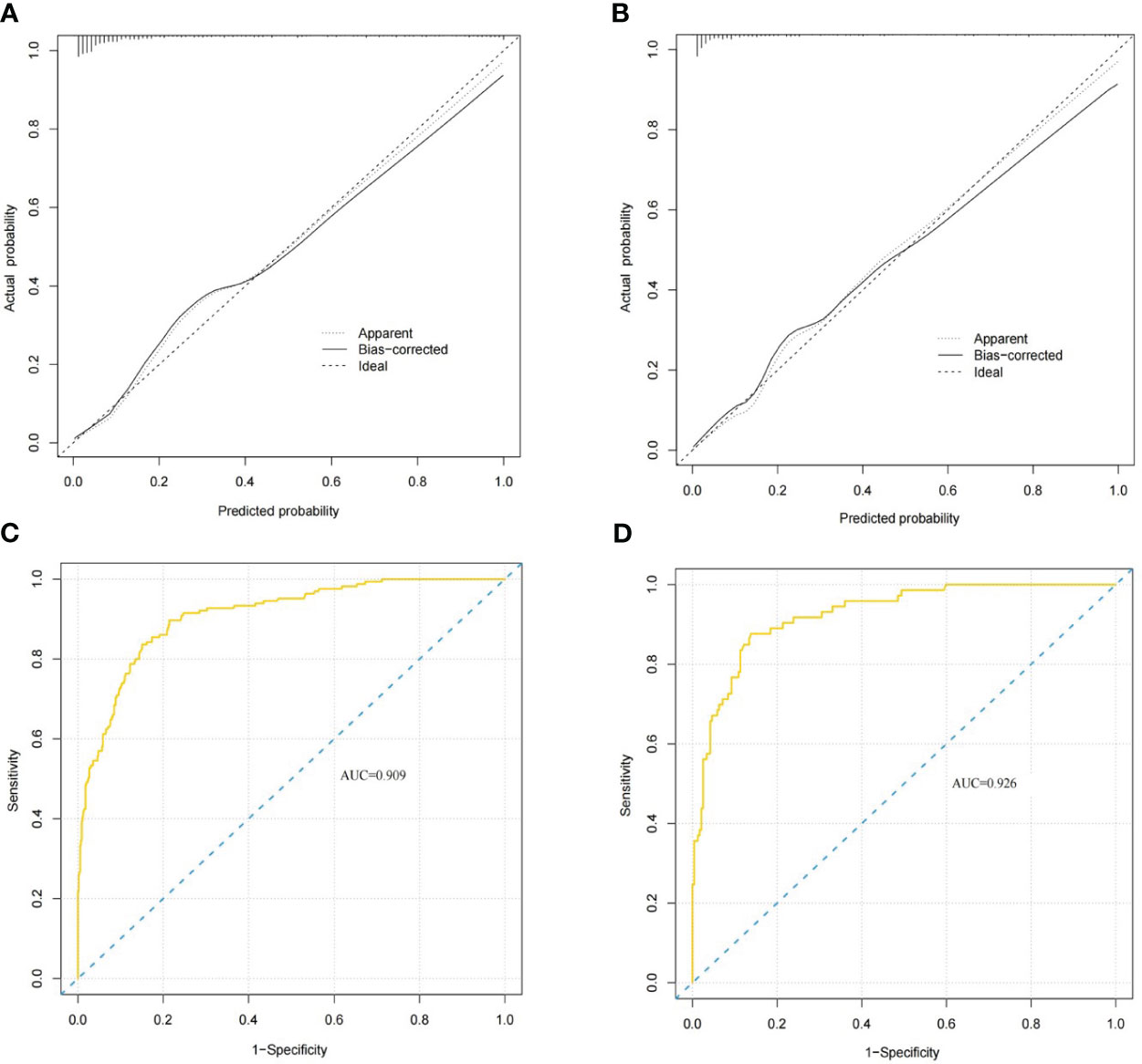
Figure 3 BMBC nomogram evaluation (A–D). Nomogram calibration curves for the training set (A) and validation set (B). Receiver operating characteristic curves for the training set (C) and validation set nomograms (D). The areas under the curves for the training cohort and the validation cohort were 0.909 and 0.926, respectively.
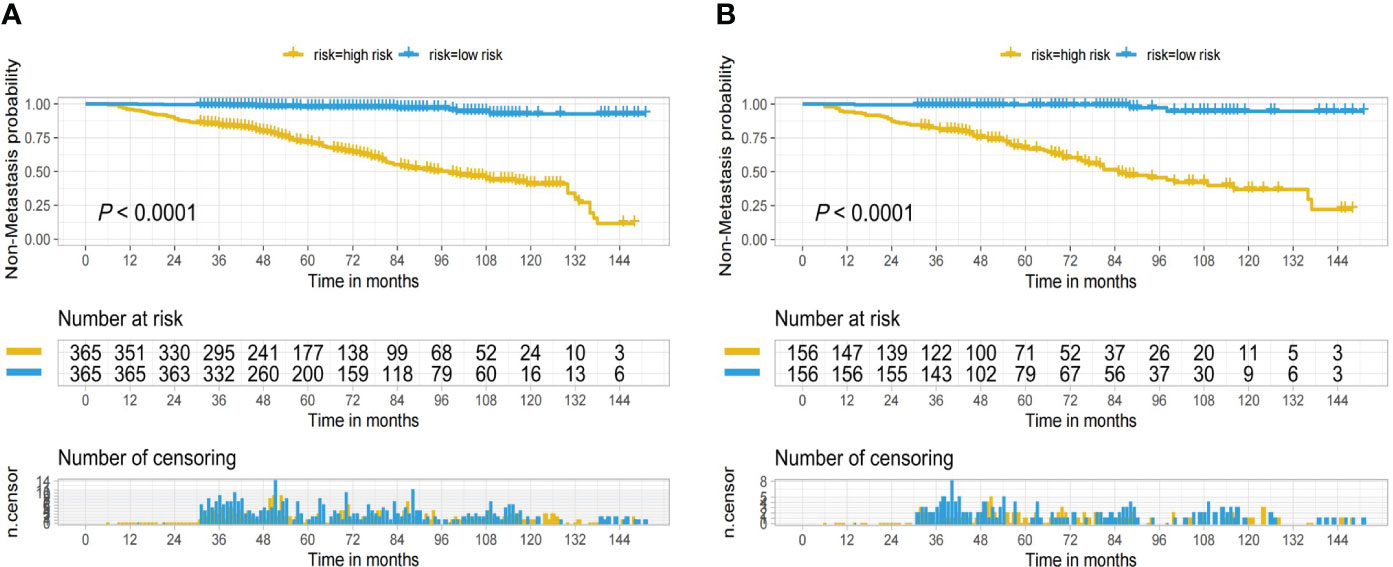
Figure 4 Kaplan-Meier curves for low and high risk bone metastatic cancer breast based on the nomogram of the training set (A) and validation set (B).
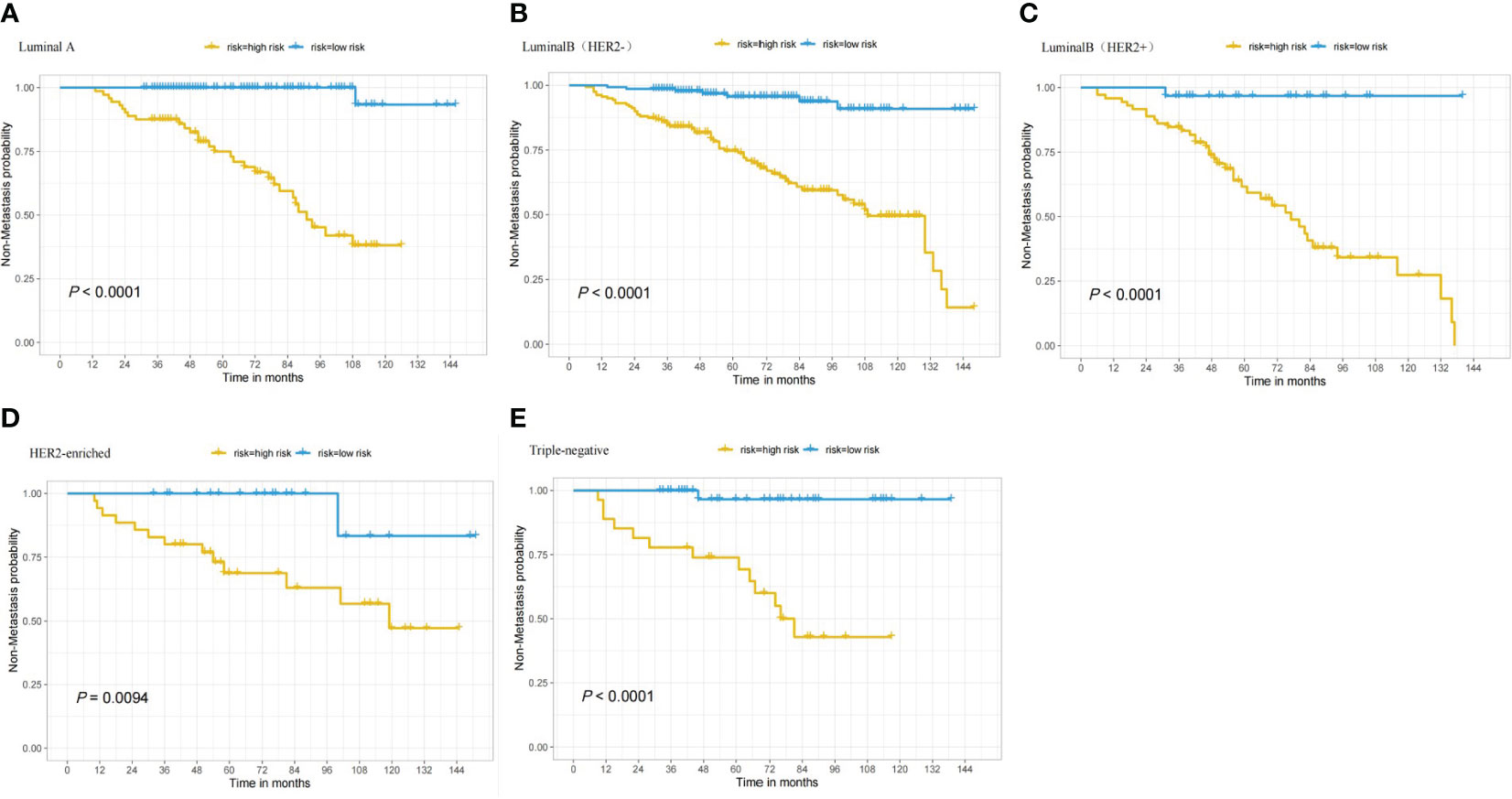
Figure 5 Based on patients with various nomogram subtypes, the Kaplan-Meier curve shows low and high risk BMBC. (A) DMFS in patients who have LuminalA tumors; (B) DMFS in patients who have LuminalB(HER2-) tumors; (C) DMFS in patients who have LuminalB(HER2+) tumors; (D) DMFS in patients who have HER2-enriched tumors; and (E) DMFS in patients who have TNBC tumors.
4 Discussion
This study aimed to identify risk factors associated with BC with bone metastasis and establish a nomogram predicting bone metastasis of early BC through clinicopathological features and hematological parameters. We found that age at diagnosis, LVI, grade, pT stage, pN stage, molecular subtype, PLR, NLR, SII, LMR, MLR, and radiotherapy were correlated with BMBC. Furthermore, age, LVI, pT stage, pN stage, molecular subtype, PLR, SII and radiotherapy were found to be independent predictors of BMBC by multivariate regression analysis. Although some tools, such as the AJCC-TNM staging system, can be used to predict the prognosis of patients with BC, they ignore some important variables, such as hematological indicators (22). In addition, the AJCC-TNM staging system was developed using data obtained from Western women who differ from Chinese women in terms of their biological characteristics. Therefore, it is necessary to develop a more comprehensive model that can better predict individualized patient outcomes to improve the predictive power of BMBC.
Through multivariate analysis, we identified independent risk factors for patients with BMBC. Previous studies have also shown that age, tumor size, tumor grade, and molecular subtype may be risk factors for MBC, which is consistent with the results of our study (21, 23–25). Our study indicates that pN stage, which reflects the status of the axillary lymph nodes, is an important independent risk factor for BMBC with a significant prognostic impact. Patients with advanced pN stage may still have a risk of persistent residual cancer cells after systemic treatment, and the tumor has the characteristics of adjacent lymph node metastasis, resulting in a higher risk of recurrence and metastasis. In our study, LuminalB (HER2+) patients were more likely to develop bone metastasis. On the one hand, the incidence of bone metastases in HR+ patients was higher than in HR−patients, which is consistent with previous studies by Shi and Xiao et al. (26, 27). On the other hand, the distant metastasis-free interval was shorter in breast cancer HER2+ patients. LuminalB (HER2+)tumors have a better prognosis than other subtypes (27), and we found that patients with these subtypes were more prone to bone metastases. In addition, independent risk factors for BMBC, such as young age (23, 24), advanced T stage (24, 25), positive LVI (28), and no radiation therapy (29), were consistent with those reported for other breast cancers.
With the development of research on the tumor microenvironment, an important intermediary exists between the inflammatory response and cancer progression (30, 31). Studies have shown that massive release of inflammatory factors in the circulatory system adversely affects the prognosis of patients with BC (32). Systemic inflammatory factors increase cancer cell invasion and proliferation, thereby promoting tumor growth and progression. We further analyzed the correlations between PLR, NLR, SII, LMR, MLR, and BMBC. The results showed that the incidence of bone metastases was higher in patients with high SII and PLR than in those with low SII and PLR. The data validated the value of the SII and PLR as BMBC prognostic biomarkers, and this was consistent with the results of previous studies (33, 34). Moreover, changes in inflammatory factors can promote tumor growth and metastasis (35, 36). During tumor progression, platelets promote angiogenesis, mainly by adhering to tumor vessels and releasing granules containing platelet-derived endothelial growth factor (37). In the local tumor inflammatory environment, greater infiltration of neutrophils and platelets and less infiltration of lymphocytes can be observed. Lymphocytes contribute to the destruction of residual malignant tumor cells and associated micrometastases in host cells, and play an important role in the immune regulation of host cells (38). Studies have shown that the markers we used such as SII and PLR may be considered risk factors for BMBC. The SII takes into account the combined effects of platelet, neutrophil, and lymphocyte counts. In a study of patients with BC, Zhang et al. found that an elevated SII predicted lower survival outcomes and was correlated with clinicopathological features indicative of tumor progression (39). Another meta-analysis of the prognostic value of PLR in BC showed that PLR is an effective prognostic biomarker (33). This is consistent with our study findings.
To the best of our knowledge, this study is the first to simultaneously compare clinicopathological features and inflammatory markers of BC (PLR, NLR, SII, LMR, and MLR) and establish a BMBC nomogram that includes SII and PLR to predict the incidence of bone metastases in patients. The nomogram we developed showed a satisfactory predictive effect. Unlike the TNM staging system model, in which the C-indices range from 0.678 to 0.775 (23–25), our model achieves more accurate predictions and is economical and convenient. However, this study has some limitations. It is a single-center retrospective study; thus, there are some uncertain biases, and the study has not been validated in other centers or databases. Therefore, the influencing factors and prediction models of BMBC require further verification. Despite these limitations, we found that age at diagnosis, LVI, pT stage, pN stage, molecular subtype, PLR, SII, and radiotherapy were significantly associated with BMBC. Furthermore, young age, positive LVI, late pT stage, late pN stage, LuminalB (HER2+) subtype, high PLR, high SII, and no radiotherapy were predisposing factors for the incidence of bone metastases. These findings can be used to identify high-risk patients to improve follow-up plans by raising early suspicion of relapse, in addition to helping clinicians optimize BMBC treatment strategies and surveillance plans to provide better treatment for patients.
Data availability statement
The raw data supporting the conclusions of this article will be made available by the authors, without undue reservation.
Ethics statement
Written informed consent was obtained from the individual(s) for the publication of any potentially identifiable images or data included in this article.
Author contributions
ZY designed this research. ZT and CL conducted analyses of the statistics and drafted the manuscript. XW, HS, and PZ carried out collection of data and processed the figures or tables. All of the authors reviewed the manuscript. All authors contributed to the article and approved the submitted version.
Funding
The only funds used were those provided by the authors’ institution.
Conflict of interest
The authors declare that the research was conducted in the absence of any commercial or financial relationships that could be construed as a potential conflict of interest.
Publisher’s note
All claims expressed in this article are solely those of the authors and do not necessarily represent those of their affiliated organizations, or those of the publisher, the editors and the reviewers. Any product that may be evaluated in this article, or claim that may be made by its manufacturer, is not guaranteed or endorsed by the publisher.
Abbreviations
BMBC, bone metastatic cancer breast; DMFS, distant metastasis-free survival.
References
1. Bray F. Global cancer statistics 2018: GLOBOCAN estimates of incidence and mortality worldwide for 36 cancers in 185 countries. CA Cancer J Clin (2018) 68(6):394–424. doi: 10.3322/caac.21492
2. Ibrahim T. A new emergency in oncology: bone metastases in breast cancer patients (Review). Oncol Lett (2013) 6(2):306–10. doi: 10.3892/ol.2013.1372
3. Sherry MM. Metastatic breast cancer confined to the skeletal system. an indolent disease. Am J Med (1986) 81(3):381–6. doi: 10.1016/0002-9343(86)90286-x
4. Scheid V. Clinical course of breast cancer patients with osseous metastasis treated with combination chemotherapy. Cancer (1986) 58(12):2589–93. doi: 10.1002/1097-0142(19861215)58:12<2589::aid-cncr2820581206>3.0.co;2-o
5. Plunkett TA. Risk of complications from bone metastases in breast cancer. implications management. Eur J Cancer (2000) 36(4):476–82. doi: 10.1016/s0959-8049(99)00331-7
6. Kuchuk I. Incidence, consequences and treatment of bone metastases in breast cancer patients-experience from a single cancer centre. J Bone Oncol (2013) 2(4):137–44. doi: 10.1016/j.jbo.2013.09.001
7. Svensson E. Survival after bone metastasis by primary cancer type: a Danish population-based cohort study. BMJ Open (2017) 7(9):e016022. doi: 10.1136/bmjopen-2017-016022
8. Elyasinia F. Neutrophil-lymphocyte ratio in different stages of breast cancer. Acta Med Iran (2017) 55(4):228–32.
9. Ma JY. Prognostic significance of the lymphocyte-to-Monocyte ratio in bladder cancer undergoing radical cystectomy: a meta-analysis of 5638 individuals. Dis Markers (2019) 2019:7593560. doi: 10.1155/2019/7593560
10. Chen L. Peripheral venous blood platelet-to-Lymphocyte ratio (PLR) for predicting the survival of patients with gastric cancer treated with SOX or XELOX regimen neoadjuvant chemotherapy. Technol Cancer Res Treat (2019) 18:1533033819829485. doi: 10.1177/1533033819829485
11. Karin M. NF-kappaB: linking inflammation and immunity to cancer development and progression. Nat Rev Immunol (2005) 5(10):749–59. doi: 10.1038/nri1703
12. McMillan DC. The systemic inflammation-based Glasgow prognostic score: a decade of experience in patients with cancer. Cancer Treat Rev (2013) 39(5):534–40. doi: 10.1016/j.ctrv.2012.08.003
13. Saito K. C-reactive protein as a biomarker for urological cancers. Nat Rev Urol (2011) 8(12):659–66. doi: 10.1038/nrurol.2011.145
14. Leitch EF. Comparison of the prognostic value of selected markers of the systemic inflammatory response in patients with colorectal cancer. Br J Cancer (2007) 97(9):1266–70. doi: 10.1038/sj.bjc.6604027
15. Cupp MA. Neutrophil to lymphocyte ratio and cancer prognosis: an umbrella review of systematic reviews and meta-analyses of observational studies. BMC Med (2020) 18(1):360. doi: 10.1186/s12916-020-01817-1
16. Nøst TH. Systemic inflammation markers and cancer incidence in the UK biobank. Eur J Epidemiol (2021) 36(8):841–8. doi: 10.1007/s10654-021-00752-6
17. Zhang C. Nomogram based on homogeneous and heterogeneous associated factors for predicting bone metastases in patients with different histological types of lung cancer. BMC Cancer (2019) 19(1):238. doi: 10.1186/s12885-019-5445-3
18. Wang X. The incidence, associated factors, and predictive nomogram for early death in stage IV colorectal cancer. Int J Colorectal Dis (2019) 34(7):1189–201. doi: 10.1007/s00384-019-03306-1
19. Xu Y. The nomogram for early death in patients with bone and soft tissue tumors. J Cancer (2020) 11(18):5359–70. doi: 10.7150/jca.46152
20. Li S. Development and validation of a nomogram predicting the overall survival of stage IV breast cancer patients. Cancer Med (2017) 6(11):2586–94. doi: 10.1002/cam4.1224
21. Wang Z. Novel prognostic nomograms for female patients with breast cancer and bone metastasis at presentation. Ann Transl Med (2020) 8(5):197. doi: 10.21037/atm.2020.01.37
22. Plichta JK. Implications for breast cancer restaging based on the 8th edition AJCC staging manual. Ann Surg (2020) 271(1):169–76. doi: 10.1097/SLA.0000000000003071
23. Liu D. Breast subtypes and prognosis of breast cancer patients with initial bone metastasis: a population-based study. Front Oncol (2020) 10:580112. doi: 10.3389/fonc.2020.580112
24. Ye LJ. Nomogram for predicting the risk of bone metastasis in breast cancer: a SEER population-based study. Transl Cancer Res (2020) 9(11):6710–9. doi: 10.21037/tcr-20-2379
25. Hou N. Development and validation of a risk stratification nomogram for predicting prognosis in bone metastatic breast cancer: a population-based study. Med (Baltimore) (2021) 100(6):e24751. doi: 10.1097/MD.0000000000024751
26. Shi D. Predicting the incidence and prognosis of bone metastatic breast cancer: a SEER-based observational study. BioMed Res Int (2020) 2020:1068202. doi: 10.1155/2020/1068202
27. Xiao W. Breast cancer subtypes and the risk of distant metastasis at initial diagnosis: a population-based study. Cancer Manag Res (2018) 10:5329–38. doi: 10.2147/CMAR.S176763
28. Wang Y, Yang Y, Chen Z, Zhu T, Wu J, Su F, et al. Development and validation of a novel nomogram for predicting distant metastasis-free survival among breast cancer patients. Ann Transl Med (2019) 7(20):537. doi: 10.21037/atm.2019.10.10
29. Cardoso F. Early breast cancer: ESMO clinical practice guidelines for diagnosis, treatment and follow-up. Ann Oncol (2019) 30(8):1194–220. doi: 10.1093/annonc/mdz173
30. Grivennikov SI. Immunity, inflammation, and cancer. Cell (2010) 140(6):883–99. doi: 10.1016/j.cell.2010.01.025
31. Hanahan D. Hallmarks of cancer: the next generation. Cell (2011) 144(5):646–74. doi: 10.1016/j.cell.2011.02.013
32. Mills RC 3rd. Breast cancer survivors, common markers of inflammation, and exercise: a narrative review. Breast Cancer (Auckl) (2017) 11:1178223417743976. doi: 10.1177/1178223417743976
33. Zhu Y. Platelet-lymphocyte ratio acts as an indicator of poor prognosis in patients with breast cancer. Oncotarget (2017) 8(1):1023–30. doi: 10.18632/oncotarget.13714
34. Krenn-Pilko S. The elevated preoperative platelet-to-lymphocyte ratio predicts poor prognosis in breast cancer patients. Br J Cancer (2014) 110(10):2524–30. doi: 10.1038/bjc.2014.163
35. Moon G. Prediction of late recurrence in patients with breast cancer: elevated neutrophil to lymphocyte ratio (NLR) at 5 years after diagnosis and late recurrence. Breast Cancer (2020) 27(1):54–61. doi: 10.1007/s12282-019-00994-z
36. Coffelt SB. Neutrophils in cancer: neutral no more. Nat Rev Cancer (2016) 16(7):431–46. doi: 10.1038/nrc.2016.52
37. Franco AT. Platelets at the interface of thrombosis, inflammation, and cancer. Blood (2015) 126(5):582–8. doi: 10.1182/blood-2014-08-531582
38. Wang HF. Progression of different cell ratios in the peripheral blood for breast cancer. Chin J Cancer Prev Treat (2019) 26(08):598–602. doi: 10.16073/j.cnki.cjcpt.2019.08.016
Keywords: bone metastasis, nomogram, predictive model, breast cancer, clinicopathological characteristics, hematological parameters
Citation: Tian Z, Li C, Wang X, Sun H, Zhang P and Yu Z (2023) Prediction of bone metastasis risk of early breast cancer based on nomogram of clinicopathological characteristics and hematological parameters. Front. Oncol. 13:1136198. doi: 10.3389/fonc.2023.1136198
Received: 02 January 2023; Accepted: 03 July 2023;
Published: 14 July 2023.
Edited by:
Hossam Taha Mohamed, October University for Modern Sciences and Arts, EgyptReviewed by:
Bo Chen, The First Affiliated Hospital of China Medical University, ChinaMonica Verrico, Sapienza University of Rome, Italy
Copyright © 2023 Tian, Li, Wang, Sun, Zhang and Yu. This is an open-access article distributed under the terms of the Creative Commons Attribution License (CC BY). The use, distribution or reproduction in other forums is permitted, provided the original author(s) and the copyright owner(s) are credited and that the original publication in this journal is cited, in accordance with accepted academic practice. No use, distribution or reproduction is permitted which does not comply with these terms.
*Correspondence: Zhiyong Yu, ZHJ6aGl5b25neXVAYWxpeXVuLmNvbQ==