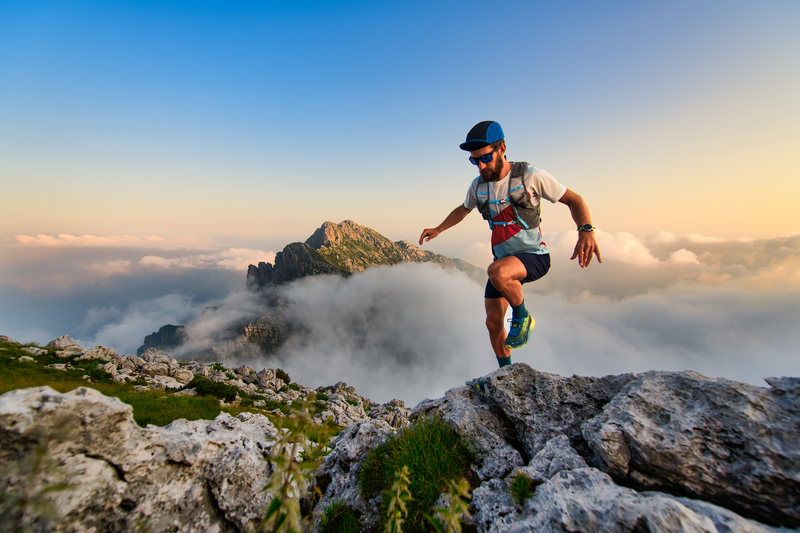
95% of researchers rate our articles as excellent or good
Learn more about the work of our research integrity team to safeguard the quality of each article we publish.
Find out more
MINI REVIEW article
Front. Oncol. , 17 February 2023
Sec. Cancer Imaging and Image-directed Interventions
Volume 13 - 2023 | https://doi.org/10.3389/fonc.2023.1134109
This article is part of the Research Topic Differentiating Brain Cancers and Glioblastoma through Imaging Methodologies View all 5 articles
MRI plays an important role in the evaluation of glioblastoma, both at initial diagnosis and follow up after treatment. Quantitative analysis via radiomics can augment the interpretation of MRI in terms of providing insights regarding the differential diagnosis, genotype, treatment response, and prognosis. The various MRI radiomic features of glioblastoma are reviewed in this article.
MRI is routinely used to formulate a differential diagnosis at the initial presentation of patients with glioblastoma, help guide treatment planning, and follow up treatment response. However, the interpretation of MRI findings in these situations can be challenging. With respect to oncologic imaging, radiomics can provide useful insights regarding tumor characteristics. Specifically, radiomics involves extracting quantitative information from the images, such as texture features, often using artificial intelligence. Reproducible radiomic features of particular tumor types can serve as imaging biomarkers. In this article, various types of radiomic analysis pertaining to glioblastoma depicted on MRI are reviewed.
Glioblastoma is a heterogeneous neoplasm both in imaging appearance and internal genotype. Tissue biopsy from one part of the tumor may not reflect histology in another (1). While this may not have much practical implication beyond explaining treatment failure now, it certainly will in the future. The heterogeneity of glioblastoma is a topic of significant research, as identification of its variable internal constituents will be vital in development of effective therapeutics. This article reviews the role that quantitative MRI may have through the use of radiomics for characterizing glioblastoma heterogeneity and differentiating glioblastoma from other entities.
The appearance of glioblastoma on MRI can sometimes be difficult to distinguish from other neoplasms and even non-neoplastic conditions, such as lower-grade gliomas, primary CNS lymphoma, tumefactive multiple sclerosis (MS), and various infections. Even using advanced MRI techniques such as arterial spin labeling, magnetic resonance spectroscopy (MRS), and perfusion imaging, tissue sampling is typically performed for definitive diagnosis.
Through the computational analysis of MRI at the pixel level, radiomics may help establish a certain diagnosis. Radiomics uses statistical methods to extract useful information from medical images that is otherwise generally beyond human perception. This information, called features, comes in two varieties: semantic and agnostic. Semantic features are the bread and butter of the radiologist. They are common descriptors of radiologic findings such as size and morphology, which guide management, and which have been correlated to gene expression (2, 3). Agnostic features are aptly named—they are esoteric mathematical derivations that reflect patterns beyond human perception. As previously mentioned, features are prone to variation, highlighting the need for standardization in order to identify stable ones (4). However, many features are also useless, being redundant or irrelevant, which contribute only noise to a model. Through a series of filtration methods, which are well-described by Papanikolaou and colleagues, features can be curated to form a radiomics model (5). However, the model must still be externally validated and statistically correlated to a clinical endpoint. It must be reproducible and answer a clinical question. Only then can it be a useful signature.
Most radiomics studies are retrospective, which is useful for building and testing models; however, they are limited in their clinical application. Regardless, there have been promising advances in delineating glioblastomas from other disease processes. For example, Conte and colleagues showed that 100 primary semantic and agnostic features based on T2-weighted images were able to delineate glioblastoma from tumefactive MS with a 0.83 accuracy and 0.86 area under the ROC curve for the best set of hyperparameters (6). Another retrospective study utilized multiparametric MRI sequences, including T1, T2, and postcontrast imaging in order to delineate glioblastoma from primary CNS lymphoma. In this study, regions encompassing the solid tumor component as well as the tumor and the peritumoral edema were selected. Of the 127 total features available, just 15 were selected, which still rendered an area under the curve of 0.979, a sensitivity of 0.938, and a specificity of 0.944 (7).
A study by Kunimatsu et al. compared the T1 postcontrast textural features of glioblastoma to primary CNS lymphoma. Their principal component analysis revealed that only four of these features—entropy, median, run length non-uniformity, and run percentage—were the predominant features of the dataset, which could explain the variance of the feature set (8). In doing so, this study suggested specific features for future analysis that may be helpful in delineating the two disease processes and address the problem of overfitting.
Another potential scenario with important clinical management implications is differentiating glioblastoma versus a solitary intracranial metastasis, which can also demonstrate edema, heterogeneity, and ring-enhancing features identical to glioblastoma. It is here too that radiomics has shown significant promise. Many of these models utilize similar textural analysis algorithms to accomplish the task (9–11). Indeed, a 2022 meta-analysis involving seventeen studies encompassing 1,717 total patients has shown the pooled sensitivity and specificity of radiomics in differentiating glioblastoma from metastasis are both 84 percent (12). In addition to MRI, more recent studies have shown that multimodality, integrative models have improved diagnostic accuracy. Zhang and colleagues showed that an integrated model including contrast-enhanced T1, T2-weighted images, diffusion-weighted images, ADC maps, and 18F-FDG PET obtained areas under the curve of 0.98 and 0.93, sensitivities of 0.925 and 0.835, and specificities of 0.987 and 0.849 for the training and validation sets (13).
Given that MRI has excellent tissue contrast and can provide diverse information about water diffusivity, blood flow, the state of the blood brain barrier, and cellularity, it follows that radiomic analysis of these data could provide further valuable information. Multiple studies have shown promise in differentiating low-grade gliomas from high-grade ones using textural analysis (14–17). Others have shown that radiomic phenotyping of glioblastoma improved survival prediction (18, 19). Yet others have demonstrated potential use in predicting treatment outcomes and assessing pseudoprogression in high-grade gliomas (20, 21). These are remarkable results considering that the analyzed features are mostly beyond human perception.
Surgery, temozolomide, and radiotherapy are the current standard of care for treating patients with glioblastoma. Unfortunately, the mean survival time of these patients remains about 15 months. There are multiple ideas as to why glioblastoma is so lethal and resilient. One contributing factor may be the cancer stem cell, which facilitates a mesenchymal transformation that allows the tumor to better infiltrate the brain. These cancer stem cells may also adapt to temozolomide therapy directly. The second idea is the tumor microenvironment. Glioblastoma is comprised of approximately 40% non-malignant cells, which act as a support network. These cells are immunosuppressive and generate a vascular-proliferative environment to help the tumor grow. Another consideration is the heterogeneity of the tumor in general. The phenotypes and genotypes of glioblastomas vary throughout their architecture and change as the disease progresses and as treatments are applied. Radiomics may play an important role here in characterizing the tumor both phenotypically and ultimately genotypically (radiogenomics), which would permit a tailored and dynamic therapeutic response. However, first biomarkers must be identified.
There are several critical mutations and molecular alterations in glioblastomas, which may be actionable biomarkers of the disease and are the subjects of intense research. The first of these is mutation of the epidermal growth factor receptor (EGFR), which is seen in about 60% of primary glioblastomas and results in a more aggressive tumor phenotype (2). In a 2017 study, Zinn and colleagues quantified the mutually exclusive and significant (false discovery rate 0.05) MRI texture features of EGFR, TP53, and PTEN mutations (22). They also analyzed the upregulated genes that were unique to each mutation versus their wildtype comparisons. The biofunctions of the mutated gene clusters were linked to predominant functions, showing TP-53-angiogenesis, PTEN-invasion, and EGFR-immune response. Consensus clustering demonstrated similar correlation matrices of the radiomic features of mutants versus wildtype mutations and the corresponding gene expressions. These findings suggesting a link between radiomic texture features and biologic function and could prove important in treatment planning, though specific compounds targeting EGFR have not yet proven effective. Early tyrosine kinase inhibitors targeting EGFR have shown little clinical efficacy in glioblastoma patients, though research is ongoing, including preclinical trials in third generation EGFR irreversible inhibitors (23, 24).
Another mutation significant to the pathogenesis of gliomas, including glioblastoma, is isocitrate dehydrogenase-1 (IDH-1) on chromosome 2. Glioblastoma can arise de novo or develop from lower grade gliomas through sequential mutations (25). Wildtype glioblastoma is far more common than mutational, and is considered the more aggressive form, which responds poorly to current therapies and has a dismal five-year prognosis. Glioblastomas with IDH-1 mutations account for 73% of tumors and are reported to have a slightly better prognosis due to their enhanced response to radiotherapy and temozolomide (26). Regardless, IDH-1 mutants and wildtype tumors have different metabolic profiles, suggesting that each type may have unique therapeutic vulnerabilities (27). One such potential vulnerability is seen in the IDH1R132H mutational subtype, which has significantly improved prognosis versus other gliomas, and overexpresses microRNA mIR-128. This microRNA suppresses glioma cell proliferation in vitro, possibly accounting for the survival benefit in vivo, and making it a potential therapeutic target (28). Therefore, imaging differentiation of this mutation remains a valuable goal. Multiple studies have had varying degrees of success in the use of radiomics for identifying IDH mutations (29–31). Of further note, IDH-1 mutation also has other diagnostic implications, as IDH-1 mutants produce 2-Hydroxyglutarate, which can be detected on MRS (32).
O6-methylguanine–DNA methyltransferase (MGMT) is a DNA-repair enzyme that repairs damage caused by alkylating agents. Production of this enzyme is inhibited by methylation of its promoter region; therefore, methylation status can predict a patient’s response to temozolomide therapy (33). Indeed, MGMT methylation status is the dominant predictor of increased survival in patients with glioblastoma, even showing significant value in patients with unresectable disease (34). Consequently, utilizing MRI to assess this gene’s methylation status would have prognostic and treatment value. Sasaki and colleagues used T1 precontrast, T1 postcontrast, and T2-weighted images to analyze the radiomics features of 201 preoperative patients with glioblastoma. Despite being able to stratify low and high-risk patients based on 22 features correlated with prognosis, this study did not display sufficient accuracy for the prediction of MGMT methylation status (35). A similar study by Chen et al. (though focused on diffuse gliomas) was able to predict methylation status of the tumors utilizing deep learning and features extracted from postcontrast T1 and ADC imaging (36).
Complete surgical excision of glioblastomas is extremely rare and they almost invariably recur, often in the surgical bed. Therefore, routine monitoring of patients is essential. Unfortunately, it can be difficult to differentiate true disease progression from radiation-associated pseudo-progression on routine MR imaging and its negative predictive value is not sufficient for clinical use (37). This is a significant problem as pseudo-progression occurs in up to a third of patients according to a 2017 meta-analysis (38). Advanced MR techniques, to include perfusion-weighted imaging and MRS have proven useful in supplementing routine sequences with a combined accuracy of about 90 percent (39). Indeed, a recent meta-analysis of 30 studies showed that dynamic susceptibility contrast (DSC) MRI and DWI had the best sensitivity and specificity in differentiating true progression from pseudoprogression in high-grade gliomas (40). Radiomics again may prove useful in this setting. Two studies have investigated algorithmic assessment of routine MR images (41, 42). These showed diagnostic accuracies of around 70%, performing better than the neuroradiologists. Similarly, another study that integrated Ktrans and relative cerebral blood volume metrics from PWI sequences attained an accuracy of about 91% (43).
There are unique challenges in the application of radiomics to MRI. Unlike CT where attenuation in Hounsfield Units is directly correlated to tissue density, the signal obtained from MRI is unitless and can variably reflect tissue properties. MRI field strength, pulse sequences, as well as manufacturer differences have been shown to affect radiomics features (44, 45). Motion artifact on MRI can also be significant as the sequences take longer to obtain. Additionally, magnetic field inhomogeneity from intrinsic and extrinsic sources can affect image acquisition. Consequently, there is a greater potential for imaging variability in MRI than CT, which could affect the robustness of imaging features. Various computational postprocessing techniques can be used to normalize MRI imaging data. These techniques have been shown to improve the prognostic value and stability of radiomics features in the setting of glioblastoma, though only 8% (113/1404) remained stable in a multicenter study (46).
Radiomics is also prone to multiple forms of error intrinsic to high level statistical analysis. Specifically, feature-based radiomics requires the selection and analysis of a region-of-interest. This is not only prone to human variability if it is not assisted by artificial intelligence, but it may also result in overfitting, which occurs if excessive high-dimensional data is applied to a small cohort. The result is a model that is “self-assessing” in that it corresponds to a single set of data but is otherwise useless in predicting outcomes elsewhere. Indeed, a 2022 study by Gidwani and colleagues revealed two marked shortcomings in contemporary radiomics literature: improper data partitioning and unproductive feature association. The first resulted in data leakage during the training, validation, and/or testing phases of these studies. The second resulted in feature self-association and overfitting. When these errors were applied to randomly generated features based on real-world data sets, they discovered a spurious combined 1.4 factor performance boost to radiomics models, indicating random chance after correction (47). Consequently, future radiomics studies must be large and multicenter with careful attention to features selection and data partitioning in order to mitigate this issue and create reproducible models (48). In deep learning radiomics, also known as deep radiomics, convoluted neural networks (CNNs) or autoencoders search for relevant patterns/features in a dataset without image segmentation. Therefore, the dataset must be large to prevent overfitting, though transfer learning in which a neural network is trained on an outside dataset, can be used to mitigate this shortcoming (49). Unfortunately, deep learning also has the “black box” shortcoming in which the AI presents information that is uninterpretable, which cannot be linked to findings of clinical relevance.
As mentioned, radiomics thrives or fails based on the reproducibility of its features. Certainly, when the success of the analysis is incumbent upon data reviewed at the pixel level, it is essential that imaging acquisition, segmentation, and analysis techniques are standardized. Unfortunately, most studies to his point have been small and retrospective, which have been valuable in early proof-of-concept of the field but insufficient for clinical validation. As a result, more recent attempts at reference value standardization have been undertaken. The Image Biomarker Standardization Initiative (IBSI) is one such example in which multiple teams established reference values for 169 radiomics features utilizing a phantom and publicly available lung cancer imaging. These values were then validated on multimodality imaging of soft tissue sarcomas with 167 of the features demonstrating good or excellent reproducibility (50).
Certainly, radiomics does have significant challenges. But such an emerging technology could prove invaluable to the field of neurooncology. The concept of a virtual biopsy is alluring, especially with the heterogeneity of glioblastoma. Indeed, as contemporary molecular genetics works to elucidate gene expression in glioblastomas and reveal potential sites for targeted therapy, it is essential that medical imaging synchronously works to reveal these biomarkers. Radiogenomics in this case would accomplish two tasks. The first, of course, to better characterize the glioblastoma. The second would be to link the radiomics features to a biologic basis. Liu and colleagues accomplished this in patients with low-grade gliomas, showing that T2-weighted radiomics features of progression-free survival were significantly associated with apoptosis, immune response, cell proliferation, and vascular development (51). A 2020 paper by Franco and colleagues outlined a future study that aims to use machine learning to analyze magnetic resonance spectroscopy (MRS) metabolite profiles in order to predict tumor types (52). Thus, by linking the abstract semantic features of radiomics to a tangible biologic basis, such endeavors could in turn improve understanding and acceptance of the technology. Nonetheless, radiomics remains a largely experimental field that has not been routinely adopted for clinical use. This is due to difficulty reproducing results and a lack of understanding between the radiomics signatures and the underlying biology, which undermines trust (53).
Radiomics is an evolving field. It is currently in its discovery phase with a preponderance of retrospective studies serving as proof-of-concept. As it stands now, radiomics has extraordinary potential to revolutionize diagnostic medicine. From the macro level of differentiating tumor types and various non-oncogenic pathologies to the micro level of tumor histology down to possibly radiogenomics, the use of computers to supplement the human eye will grow.
Ultimately, the computational analysis of MRI via radiomics and artificial intelligence is promising for characterizing glioblastoma, particularly using multimodality combinatorial models. However, radiomics is limited by the lack of standardization, which hinders reproducibility. Therefore, it is imperative that routine techniques in both imaging acquisition and segmentation are established. Radiomics also must explain the biologic underpinnings of its features in order to gain trust and move towards clinical translation (54). Studies such as that of Zinn and colleagues hint at such explanations and add unique value to the credibility of this growing field. Nevertheless, more prospective examinations of radiologic imaging correlation to controlled genetic gain of function are warranted.
The authors are both responsible for drafting and editing the article. All authors contributed to the article and approved the submitted version.
The authors declare that the research was conducted in the absence of any commercial or financial relationships that could be construed as a potential conflict of interest.
All claims expressed in this article are solely those of the authors and do not necessarily represent those of their affiliated organizations, or those of the publisher, the editors and the reviewers. Any product that may be evaluated in this article, or claim that may be made by its manufacturer, is not guaranteed or endorsed by the publisher.
1. Parker N, Hudson A, Khong P, Parkinson JF, Dwight T, Ikin RJ, et al. Intratumoral heterogeneity identified at the epigenetic, genetic and transcriptional level in glioblastoma. Sci Rep (2016) 6(22477). doi: 10.1038/srep22477
2. Diehn M, Nardini C, Wang DS, Kuo MD, McGovern S, Liang Y, et al. Identification of noninvasive imaging surrogates for brain tumor gene-expression modules. PNAS (2008) 105(13):5213–8. doi: 10.1073/pnas.0801279105
3. Hasse A, Dapash M, Jeong Y, Ansari SA, Carroll TJ, Lesniak M, et al. Correlation of post-contrast T1-weighted MRI surface regularity, tumor bulk, and necrotic volume with Ki67 and p53 in glioblastomas. Neuroradiology (2019) 61:861–7. doi: 10.1007/s00234-019-02204-1
4. Zwanenburg A, Leger S, Agolli L, Pilz K, Troost EGC, Richter C, et al. Assessing robustness of radiomic features by image perturbation. Sci Rep (2019) 9(1):614. doi: 10.1038/s41598-018-36938-4
5. Papanikolaou N, Matos C, Koh DM. How to develop a meaningful radiomic signature for clinical use in oncologic patients. Cancer Imag (2020) 20(1):33. doi: 10.1186/s40644-020-00311-4
6. Conte GM, Eckel-Passow JE, Tobin WO, Decker P, Lachance DH, Jenkins RB, et al. (2021). Differentiation of tumefactive multiple sclerosis and glioblastoma using radiomics features extracted from magnetic resonance imaging and machine learning [abstract], in: Proceedings of the AACR Virtual Special Conference on Artificial Intelligence, Diagnosis, and Imaging, Philadelphia (PA): AACR, 2021 Jan 13-14, Vol. 27.
7. Kim Y, Cho HH, Kim ST, Park H, Nam D, Kong DS. Radiomics features to distinguish glioblastoma from primary central nervous system lymphoma on multi-parametric MRI. Neuroradiology (2018) 60(12):1297–305. doi: 10.1007/s00234-018-2091-4
8. Kunimatsu A, Kunimatsu N, Kamiya K, Watadani T, Mori H, Abe O. Comparison between glioblastoma and primary central nervous system lymphoma using MR image-based texture analysis. Magn Reson Med Sci (2018) 17(1):50–7. doi: 10.2463/mrms.mp.2017-0044
9. Artzi M, Bressler I, Bashat DB. Differentiation between glioblastoma, brain metastasis and subtypes using radiomic analysis. J MRI. (2019) 50(2):519.528. doi: 10.1002/jmri.26643
10. Chen C, Ou X, Wang J, Guo W, Ma X. Radiomics-based machine learning in differentiation between glioblastoma and metastatic brain tumors. Front Oncol Sec. Cancer Imaging Image-direct Interventions (2019) 9):806. doi: 10.3389/fonc.2019.00806
11. Su CQ, Chen XT, Duan SF, Zhang JX, You YP, Lu SS, et al. A radiomics-based model to differentiate glioblastoma from solitary brain metastases. Clin Radiol (2021) 76(8):629.e11–629.e18. doi: 10.1016/j.crad.2021.04.012
12. Li Y, Liu Y, Liang Y, Wei R, Zhang W, Wang Y, et al. Radiomics can differentiate high-grade glioma from brain metastasis: a systematic review and meta-analysis. Eur Radiol (2022) 32(11):8039–51. doi: 10.1007/s00330-022-08828-x
13. Zhang L, Yao R, Gao J, Tan D, Yang X, Wen M, et al. An integrated radiomics model incorporating diffusion-weighted imaging and 18F-FDG PET imaging improves the performance of differentiating glioblastoma from solitary brain metastases. Front Oncol.Sec. Neuro-Oncol Neurosurg Oncol (2021) 11):732704. doi: 10.3389/fonc.2021.732704
14. Kobayashi K, Miyake M, Takahashi M, Hamamoto R. Observing deep radiomics for the classification of glioma grades. Sci Rep (2021) 11(1):10942. doi: 10.1038/s41598-021-90555-2
15. Tian Q, Yan LF, Zhang X, Zhang X, Hu YC, Yu H, et al. Radiomics strategy for glioma grading using texture features from multiparametric MRI. J Magn Reson Imag (2018) 48(6):1518–28. doi: 10.1002/jmri.26010
16. Xie T, Chen X, Fang J, Kang H, Xue W, Tong H, et al. Textural features of dynamic contrast-enhanced MRI derived model-free and model-based parameter maps in glioma grading. J Magn Reson Imag (2018) 47(4):1099–111. doi: 10.1002/jmri.25835
17. Malik N, Geraghty B, Dasgupta A, Maralani PJ, Sandhu M, Detsky J, et al. MRI Radiomics to differentiate between low grade glioma and glioblastoma peritumoral region. J Neurooncol. (2021) 155(2):181–91. doi: 10.1007/s11060-021-03866-9
18. Bae S, Choi YS, Ahn SS, Chang JH, Kang S. Radiomic MRI phenotyping of glioblastoma: Improving survival prediction. Radiology (2018) 289(3):797–806. doi: 10.1148/radiol.2018180200
19. Yan J, Zhang B, Zhang S, Cheng J, Liu X, Wang W, et al. Quantitative MRI-based radiomics for noninvasively predicting molecular subtypes and survival in glioma patients. NPJ Precis Oncol (2021) 5(1):72. doi: 10.1038/s41698-021-00205-z
20. Kickingereder P, Götz M, Muschelli J, Wick A, Neuberger U, Shinohara RT, et al. Large-Scale radiomic profiling of recurrent glioblastoma identifies an imaging predictor for stratifying anti-angiogenic treatment response. Clin Cancer Res (2016) 22(23):5765–71. doi: 10.1158/1078-0432.CCR-16-0702
21. Mammadov O, Akkurt BH, Musigmann M, Ari AP, Bloemer DA, Kasap DNG, et al. Radiomics for pseudoprogression prediction in high grade gliomas: added value of MR contrast agent. Heliyon (2022) 8(8):e10023. doi: 10.1016/j.heliyon.2022.e10023
22. Zinn P, Singh S, Kotrotsou A, Abrol S, Thomas G, Mosley J, et al. Distinct radiomic phenotypes define glioblastoma TP53-PTEN-EGFR mutational landscape. Neurosurgery (2017) 64(CN_suppl_1):203–10. doi: 10.1093/neuros/nyx316
23. Westphal M, Maire CL, Lamszus K. EGFR as a target for glioblastoma treatment: An unfulfilled promise. CNS Drugs (2017) 31(9):723–35. doi: 10.1007/s40263-017-0456-6
24. Reardon DA, Nabors LB, Mason WP, Perry JR, Shapiro W, Kavan P, et al. BI 1200 36 trial group and the Canadian brain tumour consortium. phase i/randomized phase II study of afatinib, an irreversible ErbB family blocker, with or without protracted temozolomide in adults with recurrent glioblastoma. Neuro Oncol (2015) 17(3):430–9. doi: 10.1093/neuonc/nou160
25. Urbańska K, Sokołowska J, Szmidt M, Sysa P. Glioblastoma multiforme - an overview. Contemp Oncol (Pozn). (2014) 18(5):307–12. doi: 10.5114/wo.2014.40559
26. Han S, Liu Y, Cai SJ, Qian M, Ding J, Larion M, et al. IDH mutation in glioma: molecular mechanisms and potential therapeutic targets. Br J Cancer. (2020) 122(11):1580–9. doi: 10.1038/s41416-020-0814-x
27. Garrett M, Fujii Y, Osaka N, Sasaki A, et al. Emerging roles of wild-type and mutant IDH1 in growth, metabolism and therapeutics of glioma. In: Debinski W, editor. Gliomas [Internet]. Brisbane (AUExon Publications (2021). doi: 10.36255/exonpublications.gliomas.2021.chapter4
28. Nie QM, Lin YY, Yang X, Shen L, Que SL, et al. IDH1R¹³²H decreases the proliferation of U87 glioma cells through upregulation of microRNA-128a. Mol Med Rep (2015) 12(5):6695–701. doi: 10.3892/mmr.2015.4241
29. Macyszyn L, Akbari H, Pisapia JM, Da X, Attiah M, Pigrish V, et al. Imaging patterns predict patient survival and molecular subtype in glioblastoma via machine learning techniques. Neuro Oncol (2016) 18(3):417–25. doi: 10.1093/neuonc/nov127
30. Wu S, Meng J, Yu Q, Li P, Fu S. Radiomics-based machine learning methods for isocitrate dehydrogenase genotype prediction of diffuse gliomas. J Cancer Res Clin Oncol (2019) 145(3):543–50. doi: 10.1007/s00432-018-2787-1
31. Calabrese E, Villanueva-Meyer JE, Cha S. A fully automated artificial intelligence method for non-invasive, imaging-based identification of genetic alterations in glioblastomas. Sci Rep (2020) 10(11852). doi: 10.1038/s41598-020-68857-8
32. Andronesi OC, Kim GS, Gerstner E, Batchelor T, Tzika AA, Fantin VR, et al. Detection of 2-hydroxyglutarate in IDH-mutated glioma patients by in vivo spectral-editing and 2D correlation magnetic resonance spectroscopy. Sci Transl Med (2012) 4(116):116ra4. doi: 10.1126/scitranslmed.3002693
33. Yu W, Zhang L, Wei Q, Shao A. O6-Methylguanine-DNA methyltransferase (MGMT): Challenges and new opportunities in glioma chemotherapy. Front Oncol (2020) 9. doi: 10.3389/fonc.2019.01547
34. Szylberg M, Sokal P, Śledzińska P, Bebyn M, Krajewski S, Szylberg L, et al. MGMT promoter methylation as a prognostic factor in primary glioblastoma: A single-institution observational study. Biomedicines (2022) 10(8):2030. doi: 10.3390/biomedicines10082030
35. Sasaki T, Kinoshita M, Fujita K, Fukai J, Hayashi N, Uematsu Y, et al. Radiomics and MGMT promoter methylation for prognostication of newly diagnosed glioblastoma. Sci Rep (2019) 9(14435). doi: 10.1038/s41598-019-50849-y
36. Chen S, Xu Y, Ye M, Li Y, Sun Y, Liang J, et al. Predicting MGMT promoter methylation in diffuse gliomas using deep learning with radiomics. J Clin Med (2022) 11(12):3445. doi: 10.3390/jcm11123445
37. Young RJ, Gupta A, Shah AD, Graber JJ, Zhang Z, Shi W, et al. Potential utility of conventional MRI signs in diagnosing pseudo-progression in glioblastoma. Neurology (2011) 76(22):1918–24. doi: 10.1212/WNL.0b013e31821d74e7
38. Abbasi AW, Westerlaan HE, Holtman GA, Aden KM, Jan van Laar P, van der Hoorn A, et al. Incidence of tumour progression and pseudoprogression in high-grade gliomas: a systematic review and meta-analysis. Clin Neuroradiol (2018) 28:401–11. doi: 10.1007/s00062-017-0584-x
39. Thust SC, van den Bent MJ, Smits M. Pseudoprogression of brain tumors. JMRI (2018) 48(3):571–89. doi: 10.1002/jmri.26171
40. Taylor C, Ekert JO, Sefcikova V, Fersht N, Samandouras G. Discriminators of pseudoprogression and true progression in high-grade gliomas: A systematic review and meta-analysis. Sci Rep (2022) 12(1):13258. doi: 10.1038/s41598-022-16726-x
41. Bani-Sadr A, Eker OF, Berner LP, Ameli R, Hermier M, Barritault M, et al. Conventional MRI radiomics in patients with suspected early- or pseudo-progression. Neuro-Oncol Advances. (2019) 1(1). doi: 10.1093/noajnl/vdz019
42. Sun YZ, Yan LF, Han Y, Nan HY, Xiao G, Tian Q, et al. Differentiation of pseudoprogression from true progressionin glioblastoma patients after standard treatment: A machine learning strategy combinedwith radiomics features from T1-weighted contrast-enhanced imaging. BMC Med Imag (2021) 21(1):17. doi: 10.1186/s12880-020-00545-5
43. Elshafeey N, Kotrotsou A, Hassan A, Elshafei N, Hassan I, Ahmed S, et al. Multicenter study demonstrates radiomic features derived from magnetic resonance perfusion images identify pseudoprogression in glioblastoma. Nat Commun (2019) 10(3170). doi: 10.1038/s41467-019-11007-0
44. Saha A, Yu X, Sahoo D, Mazurowski MA. Effects of MRI scanner parameters on breast cancer radiomics. Expert Syst Applicat (2017) 87:384–91. doi: 10.1016/j.eswa.2017.06.029
45. Lee J, Steinmann A, Ding Y, Lee H, Owens C, Wang J, et al. Radiomics feature robustness as measured using an MRI phantom. Sci Rep (2021) 11(1):3973. doi: 10.1038/s41598-021-83593-3
46. Saltybaeva N, Tanadini-Lang S, Vuong D, Burgermeister S, Mayinger M. Robustness of radiomic features in magnetic resonance imaging for patients with glioblastoma: Multi-center study. Phys Imaging Radiat Oncol (2022) 22:131–6. doi: 10.1016/j.phro.2022.05.006
47. Gidwani M, Chang K, Patel JB, Hoebel KV, Ahmed SR, Singh P, et al. Inconsistent partitioning and unproductive feature associations yield idealized radiomic models. Radiology (2022) 20:220715. doi: 10.1148/radiol.220715
48. Lohmann P, Bousabarah K, Hoevels M. Treuer H.Radiomics in radiation oncology–basics, methods, and limitations. Strahlentherapie und Onkolo (2020) 196:848–55. doi: 10.1007/s00066-020-01663-3
49. Wagner MW, Namdar K, Biswas A, Monah S, Khalvati F, Ertl-Wagner BB. Radiomics, machine learning, and artificial intelligence-what the neuroradiologist needs to know. Neuroradiology (2021) 63(12):1957–67. doi: 10.1007/s00234-021-02813-9
50. Zwanenburg A, Vallières M, Abdalah MA, Aerts HJWL, Andrearczyk V, Apte A, et al. The image biomarker standardization initiative: Standardized quantitative radiomics for high-throughput image-based phenotyping. Radiology (2020) 295(2):328–38. doi: 10.1148/radiol.2020191145
51. Liu X, Li Y, Qian Z, Sun Z, Xu K, Wang K, et al. A radiomic signature as a non-invasive predictor of progression-free survival in patients with lower-grade gliomas. NeuroImage Clin (2018) 20:1070–7. doi: 10.1016/j.nicl.2018.10.014
52. Franco P, Würtemberger U, Dacca K, Hübschle I, Beck J, Schnell O, et al. SPectroscOpic prediction of bRain tumours (SPORT): study protocol of a prospective imaging trial. BMC Med Imag (2020) 20(1):123. doi: 10.1186/s12880-020-00522-y
53. Lohmann P, Franceschi E, Vollmuth P, Dhermain F, Weller M. Radiomics in neuro-oncological clinical trials. Lancet (2022) 4(11):E841–9. doi: 10.1016/S2589-7500(22)00144-3
Keywords: MRI, glioblastoma, radiomic analysis, radiomics, radiology, gliomas
Citation: Hooper GW and Ginat DT (2023) MRI radiomics and potential applications to glioblastoma. Front. Oncol. 13:1134109. doi: 10.3389/fonc.2023.1134109
Received: 29 December 2022; Accepted: 07 February 2023;
Published: 17 February 2023.
Edited by:
Pilar López-Larrubia, Spanish National Research Council (CSIC), SpainReviewed by:
Kathleen Schmainda, Medical College of Wisconsin, United StatesCopyright © 2023 Hooper and Ginat. This is an open-access article distributed under the terms of the Creative Commons Attribution License (CC BY). The use, distribution or reproduction in other forums is permitted, provided the original author(s) and the copyright owner(s) are credited and that the original publication in this journal is cited, in accordance with accepted academic practice. No use, distribution or reproduction is permitted which does not comply with these terms.
*Correspondence: Daniel T. Ginat, Z2luYXRkMDFAZ21haWwuY29t
Disclaimer: All claims expressed in this article are solely those of the authors and do not necessarily represent those of their affiliated organizations, or those of the publisher, the editors and the reviewers. Any product that may be evaluated in this article or claim that may be made by its manufacturer is not guaranteed or endorsed by the publisher.
Research integrity at Frontiers
Learn more about the work of our research integrity team to safeguard the quality of each article we publish.