- 1Institute of Medical Technology, Peking University Health Science Center, Beijing, China
- 2Key Laboratory of Carcinogenesis and Translational Research (Ministry of Education/Beijing), Department of Radiation Oncology, Peking University Cancer Hospital & Institute, Beijing, China
- 3Centre for Medical Image Computing, Department of Medical Physics and Biomedical Engineering, University College London, London, United Kingdom
- 4School of Basic Medical Sciences, Peking University Health Science Center, Beijing, China
- 5School of Electronics Engineering and Computer Science, Peking University, Beijing, China
Purpose: To propose and evaluate a comprehensive modeling approach combing radiomics, dosiomics and clinical components, for more accurate prediction of locoregional recurrence risk after radiotherapy for patients with locoregionally advanced HPSCC.
Materials and methods: Clinical data of 77 HPSCC patients were retrospectively investigated, whose median follow-up duration was 23.27 (4.83-81.40) months. From the planning CT and dose distribution, 1321 radiomics and dosiomics features were extracted respectively from planning gross tumor volume (PGTV) region each patient. After stability test, feature dimension was further reduced by Principal Component Analysis (PCA), yielding Radiomic and Dosiomic Principal Components (RPCs and DPCs) respectively. Multiple Cox regression models were constructed using various combinations of RPC, DPC and clinical variables as the predictors. Akaike information criterion (AIC) and C-index were used to evaluate the performance of Cox regression models.
Results: PCA was performed on 338 radiomic and 873 dosiomic features that were tested as stable (ICC1 > 0.7 and ICC2 > 0.95), yielding 5 RPCs and DPCs respectively. Three comprehensive features (RPC0, P<0.01, DPC0, P<0.01 and DPC3, P<0.05) were found to be significant in the individual Radiomic or Dosiomic Cox regression models. The model combining the above features and clinical variable (total stage IVB) provided best risk stratification of locoregional recurrence (C-index, 0.815; 95%CI, 0.770-0.859) and prevailing balance between predictive accuracy and complexity (AIC, 143.65) than any other investigated models using either single factors or two combined components.
Conclusion: This study provided quantitative tools and additional evidence for the personalized treatment selection and protocol optimization for HPSCC, a relatively rare cancer. By combining complementary information from radiomics, dosiomics, and clinical variables, the proposed comprehensive model provided more accurate prediction of locoregional recurrence risk after radiotherapy.
1 Introduction
Compared with invasive surgery, organ-preserving options such as definitive radiotherapy (RT) are more commonly used for patients with previously untreated, newly diagnosed locoregionally advanced hypopharyngeal squamous carcinoma (HPSCC), achieving comparable if not better long-term survival (1–3). However, due to advanced-stage disease, poor performance status, comorbidities, alcohol abuse, and nutritional problems, the 5-year survival rates of patients with HPSCC were only no greater than 40% as reported in various studies (4, 5). Furthermore, the prognosis was also poor, ascribable to the tumor heterogeneity and large outcome uncertainties after standard treatment (6). To improve the prognosis, personalized risk prediction is clinically desirable to support patient-specific selection and optimization of treatment protocols for better tumor control (7, 8), however, existing clinical experience is very subjective and unstable based on conventional parameters such as smoking, drinking, T stage, and lymph node metastasis etc. (9).
By analyzing high dimensional image features that are invisible to radiologists based on multi-modality images such as MRI (10), PET-CT (11) and CT (12), Radiomics has been demonstrated as a promising approach to stratify patients of various risks due to tumor heterogeneity. Unlike the dominant dependence of chemotherapy outcome on the biological varieties as represented by Radiomics features (12), the radiation dose is another key determinative factor of treatment effectiveness for HPSCC patients receiving radiotherapy. The radiation dose distribution is optimized based on patient-specific characteristics such as the shape, volume and location of the tumor and adjacent organs. However, conventional dose-volume-histograms (DVHs) provided limited dosimetric statistics without spatial information, and the predictive accuracy is unsatisfactory (13). Using similar methods as that of Radiomics, Dosiomics analyzes the spatial features of the 3-dimentional patient-specific dose distribution, hence provides better prediction of radiation-induced results (13, 14).
Considering the therapeutic responses of HPSCC patients treated with radiotherapy depend on both tumor heterogeneity and dosimetric variables, this work hypothesized that combined signatures using both Radiomics and Dosiomics features may have more robust statistical correlation with locoregional recurrence of patients with locoregionally advanced HPSCC treated with radiotherapy, potentially supporting more quantitative and personalized clinical decision making such as strategy selection and protocol optimization, which has not been reported in the literature before and is the purpose of this study.
2 Materials and methods
2.1 Patients
The data of 77 patients pathologically confirmed with HPSCC at our center between October 2011 and July 2020 were retrospectively investigated. The inclusion criteria were (1): lesion located at hypopharynx and pathologically diagnosed squamous cell carcinoma ; (2) administration and completion of laryngeal-preservation treatments: induction chemotherapy (IC)+radical RT or IC+chemoradiation; (3) no postpone, intervention or discontinuation during RT. Data with violation were excluded. All patients underwent disease staging using the American Joint Committee on Cancer Staging System, Eighth Edition (15).
2.2 Image acquisition and target delineation
All patients were immobilized in the supine position using thermoplastic head-neck-shoulder masks. Planning CT with intravenous contrast was acquired for structure delineation on a SIEMENS Sensation Open CT scanner using the following protocols: tube voltage 120kV; tube current 320mAs; reconstruction thickness 3 mm; matrix 512 × 512. Target and organs-at-risk (OARs) delineation was manually performed by experienced radiation oncologists according to NCCN and RTOG guidelines respectively (16, 17). All delineations were double checked and approved by senior radiation oncologists per our clinical protocols.
Intensity-modulated radiation therapy (IMRT) or volumetric modulated arc therapy (VMAT) plans were optimized to deliver a prescription dose of 60 Gy to at least 95% of the planning target volume (PTV) in 33 fractions. The dose limitations to OARs were in accordance with RTOG 0615 protocols (18). Simultaneous-integrated-boost (SIB) technique was used to deliver 70 Gy to at least 95% of the planning gross tumor volume (PGTV) in the same plan. All patients were treated with one fraction daily, 5 days per week.
2.3 Follow-up protocol and definition of failures
The follow-up was performed at 1 month after the completion of RT, every 3 months during the first 2 years, every 6 months between the third and fifth year, and annually thereafter. At the time of the last follow-up assessment, failure patterns were classified as local, regional, or distant respectively. Local failure was defined as failure of the primary tumor to the treatment. Regional failure was defined as the recurrence in the regional lymph nodes. Distant failure was defined as the appearance of a tumor at any site representing hematogenous dissemination.
2.4 Extraction and selection of radiomic and dosiomic features
The flowchart of building the statistical analysis model is shown in Figure 1 Using PGTV as the volume-of-interest (VOI), radiomic and dosiomic features were extracted from the planning CT images and dose maps respectively using the third-party python library (19) (https://pyradiomics.readthedocs.io). Basic radiomic features included 18 first-order features, 14 shape-and-volume features and 75 texture features. To expand the feature pool, Log of Gaussian filter and wavelet transform were applied on the planning CT images respectively. The aforementioned texture features were then recalculated, generating 1209 more features. In this study, BiorSplines (bior6.8) was used as the main wavelet function (20). Dosiomic features were extracted using the same procedures from the dose maps.
Consistent with other studies (21, 22), this study evaluated the stability of the extracted radiomic features by applying Guassian noise and random ROI boundary perturbance to the original CT images and VOI boundaries respectively. Three levels of standard variations of the Gaussian noise and two levels of the boundary perturbance distances were used, i.e., 10, 50, 100 HU and 0.5, 1 pixel pitch unit respectively. The intraclass correlation coefficient (ICC) (23) was used as an indicator of feature stability between different groups, which was calculated on the original and the counterpart images respectively, using the definitions of ICC (1,1) () and ICC (2,1) () respectively. was used to indicate interrater reliability of the features and was used to indicate non-independence of the features respectively (24). and were calculated as Eq. (1) and Eq. (2):
MSR means mean square for rows, MSW means mean square for residual sources of variance and k means the number of raters respectively.
Consistent with the literature, features with > 0.7 and > 0.95 were considered as reproducible (25) in the existence of image noise and segmentation errors respectively. As the main influential factor of dosiomic feature robustness, only perturbation of ROI boundary was used to assess the stability of dosiomic features in this study, in accordance with Francesco’s method (26).
Amongst the screened stable features, the most clinically relevant features were further selected using univariate analysis, according to their capability of stratifying the patients into high and low locoregional recurrence risk rates. Log-rank test (27) was used to examine the statistical significance of the inter-group differences between the survival curves of the two groups. Only significant features with p-values<0.05 (statistically significant) were used for further analysis.
2.5 Modeling and statistical analysis
Dimensionality reduction of the feature space was performed using Principal Component Analysis (PCA). The first five principal components of radiomic and dosiomic features were used as independent variables of two multivariate Cox regression models respectively, noted as the Radiomic Principal Component (RPC) Model and Dosiomic Principal Component (DPC) Model respectively. A multivariate Cox regression model was also constructed on conventional clinical parameters, noted as the Clinical Model. Kaplan-Meier survival analysis and the log-rank test were used to evaluate the predictive capability of each significant Principal Component and clinical variable respectively.
In addition, the principal components with significant hazard ratio (p< 0.05) were combined with clinical parameters to construct another three comprehensive multivariate Cox regression models, named as Radiomics+Clinical Model, Dosiomics+Clinical Model, and Radiomics+Dosiomics+Clinical Model respectively. The performance of these comprehensive models were evaluated with the partial Akaike information criterion (AIC) (28) and concordance index (C-index) (29) respectively. Defined as , AIC was proposed to balance the tradeoff between the ability to fit and the simplicity of the model (30), where k means the number of estimated parameters and means the likelihood function which was maximum. Lower AIC values indicate better models with less complexity. The C-index was used to evaluate discriminative performance of each model. Defined as Eq. (3):
C-index (31) presents the proportion of concordant data pairs, where is a pair of event; means event n’s observation time; means event n’s risk; means when event j’s risk is higher than event i, event j’s observation time is earlier than event i; means event j happened in . As a predictive marker and a time-to-event response variable, C-Index values closer to 1 suggest better model performance.
The statistical analysis was conducted using the following packages of R and Python respectively: the survival package (R) and the lifeline package (Python) were used to execute Kaplan–Meier analysis, build the Cox proportional risk models, and calculate the C-index respectively. Local regional recurrence-free survival (LRRFS), metastasis-free survival (MFS), progression-free survival (PFS), and overall survival (OS) rates were estimated using the Kaplan–Meier method and measured from the first day of treatment to the date of the event. All statistical tests were two-sided, and p values< 0.05 were considered as significant.
3 Results
3.1 Patient demography and treatment outcomes
The characteristics of the 77 patients included in this study are presented in Table 1.
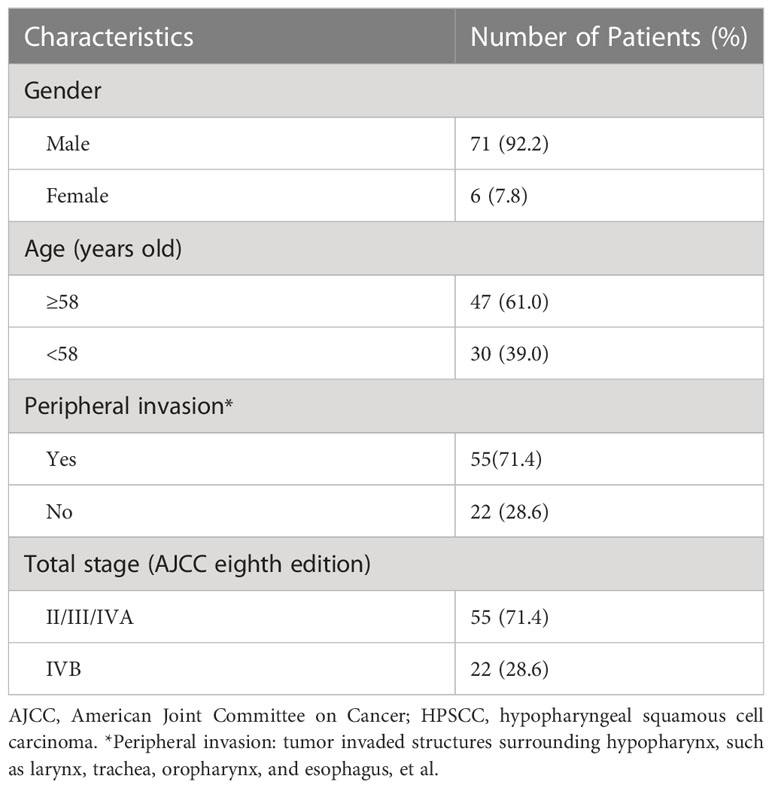
Table 1 Clinical characteristics of the 77 patients with locoregionally advanced HPSCC involved in this study.
The median follow-up duration was 23.27 (range, 4.83–81.40) months. During the study period, 29 patients experienced disease progression, and 30 patients died due to tumor progression (19), local hypopharyngeal haemorrhage (4), infection (5), car accident (1) and secondary primary cancer (1), respectively. The median PFS and OS estimates were 47.07 months and 36.07 months, respectively. Three-year LRRFS, MFS, PFS, and OS rates were 70.6%, 81.7%, 58.3%, and 48.9%, respectively. Of the 29 patients experienced treatment failure at their last follow-up visit, 13, 2, 9, 3, 0, 1 and 1 patients presented with local only, regional only, distant only, local-regional, regional-distant, local-distant, and local-regional-distant failure, respectively.
3.2 Statistical analysis and model evaluation
For each patient, 1321 radiomic features and 1321 dosiomic features were extracted from the planning CT images and dose distributions respectively, from which 338 radiomic features and 873 dosiomic features were identified as stable and reproducible (>0.7, >0.95). After PCA, 5 radiomic principal components and 5 dosiomic principal components were obtained respectively. Table 2 shows the hazard ratio (HR) and p-values of each principal component and the AIC of Cox regression, for the Clinical Model, Radiomic Principal Components (RPC) Model and Dosiomic Principal Components (DPC) Model respectively. One clinical variable, one RPC and two DPC had significant hazard ratios in Cox regression (p-value< 0.05) respectively.
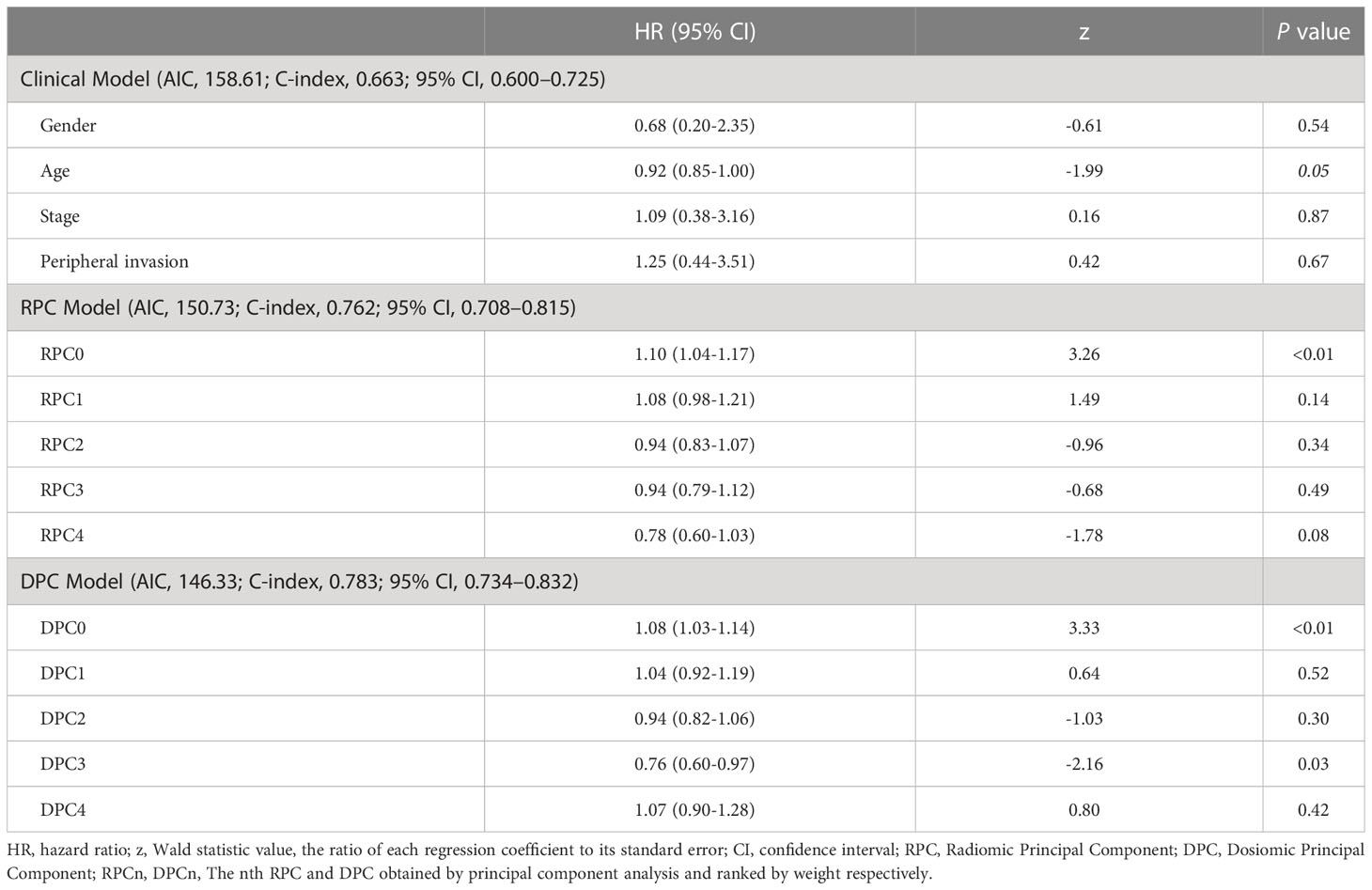
Table 2 Multivariate Cox Regression Analysis for the Clinical Model, Radiomic Principal Components (RPC) Model and Dosiomic Principal Components (DPC) Model respectively.
For the combined models, the results of Multivariate Cox Regression Analysis are presented in Table 3 for the Radiomic+Clinical Model, Dosiomic+Clinical Model and Radiomic+Dosiomic+Clinical Model respectively.
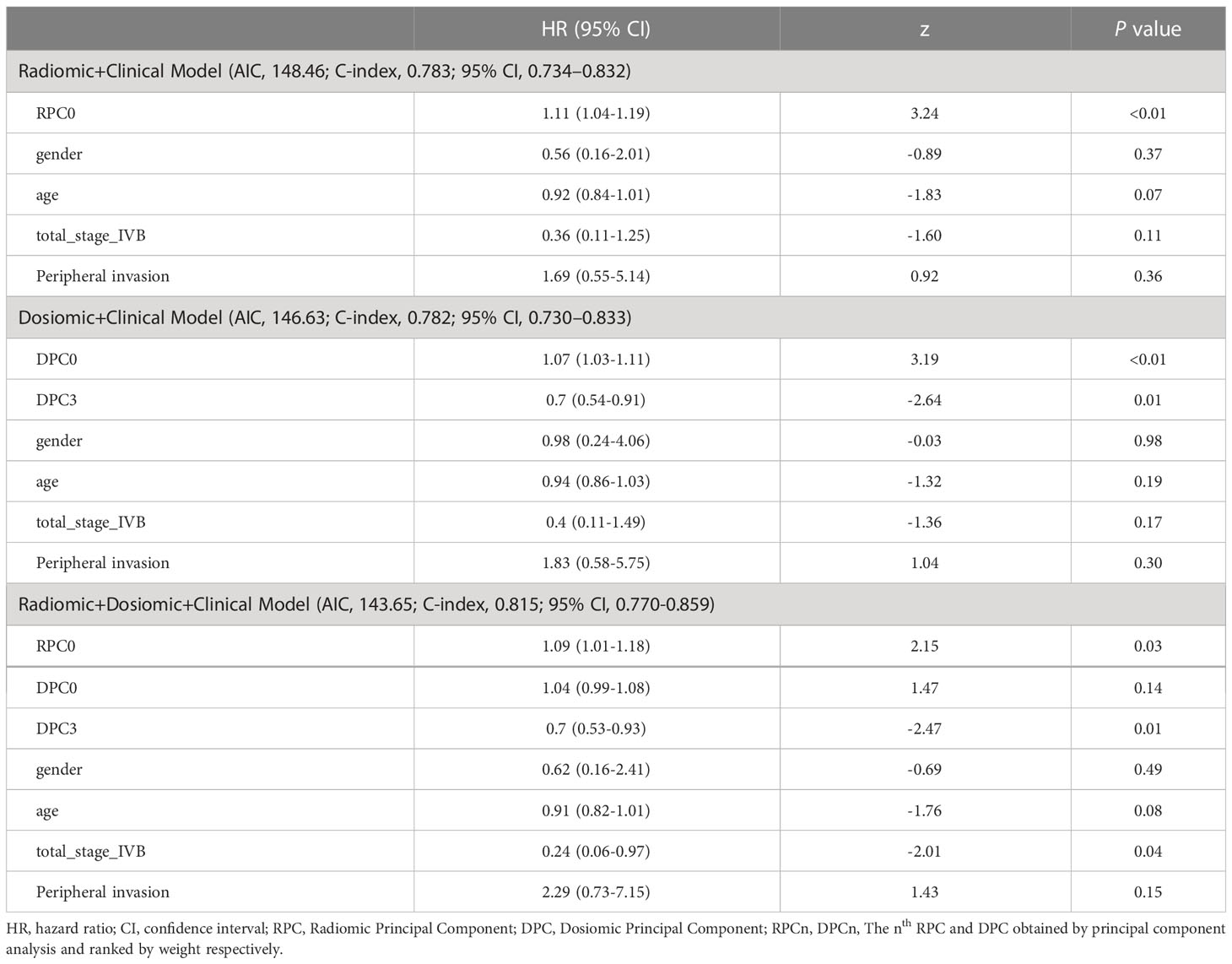
Table 3 Multivariate Cox Regression Analysis for the Radiomic+Clinical Model, Dosiomic+Clinical Model and Radiomic+Dosiomic+Clinical Model respectively.
Figure 2 displays the survival curves of high-risk and low-risk locoregionally advanced patient groups stratified by significant Radiomic and Dosiomic principal components respectively.
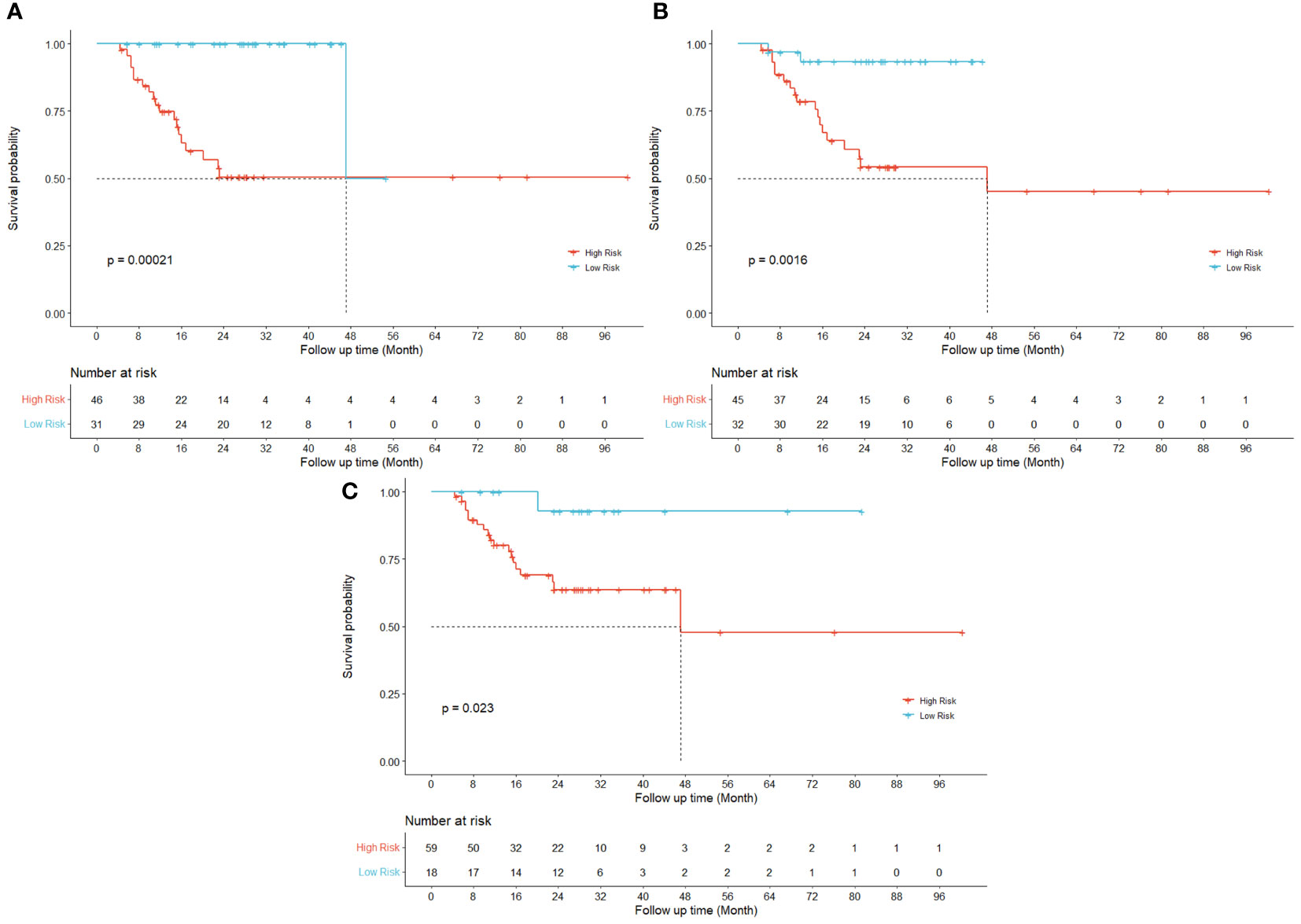
Figure 2 Kaplan-Meier curves for LRRFS. Univariate results are shown for three different principal components in radiomic ((A) RPC0) and dosiomic ((B) DPC0; (C) DPC3) features respectively.
Figure 3 displays the survival curves stratified by conventional clinical variables such as gender (a), peripheral invasion (b), total stage (c) and age (d) respectively.
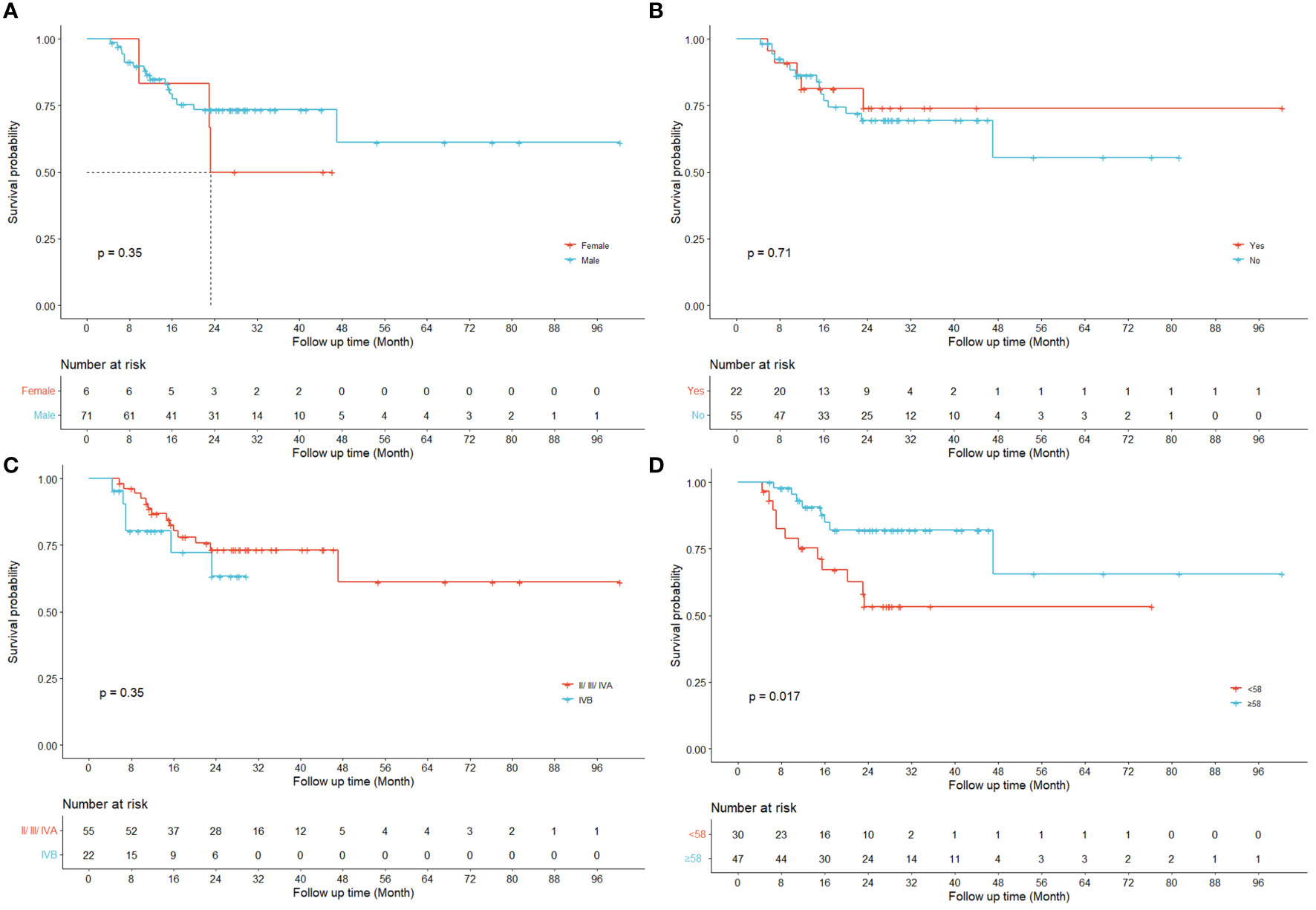
Figure 3 Kaplan-Meier curves for LRRFS. Univariate results are shown for four different clinical variables respectively ((A) gender; (B) peripheral invasion; (C) total stage IVB; (D) age).
4 Discussion
HPSCC is a relatively rare but aggressive malignancy accounting for 5%-10% of head and neck cancer, with high incidence of recurrence and low survival rates (32). There is no sufficient data and evidence to guide precision medicine and prognosis for HPSCC patients. Several clinical prediction models for HPSCC have been published previously (33–35). A reported clinical prediction model for survival in hypopharynx cancer consisted of gender, subsite, TNM classification, Adult Comorbidity Evaluation-27 score (ACE27), body mass index (BMI), hemoglobin, albumin, and leukocyte count. Of these, TNM classification, ACE27, BMI, hemoglobin, and albumin had independent significant associations with survival. But the TNM classification might vary by investigator bias. ACE27 score incorporated 27 ailments which could have mutual effect. Hemoglobin, albumin and leukocyte counts were all baseline data at one time point, and there were 29%, 12% and 2% missing data in the peripheral blood value albumin, leukocyte counts and hemoglobin respectively. All these factors may increase uncertainties of this prediction model (34). The effectiveness and consistency should be further improved in a patient-specific way to assist clinical decision making and treatment protocol optimization.
Previous studies have demonstrated the prognostic values of radiomics features in predicting the risk of HPSCC patients treated with chemoradiation (12, 36) and their key findings were echoed and reconfirmed by our study as shown in the Kaplan-Meier curves for LRRFS (RPC0) in Figure 2A. In addition to radiomics, our work also revealed that the principal components of dosiomic features (DPC0 and DPC3) could also stratify patients with different risk (log-rank P<0.05), as shown in Figures 2B, C. Table 2 suggested that dosiomics principal components (C-index=0.783, 95% CI, 0.734–0.832) achieved better predictive performance than that of radiomics (C-index=0.762, 95% CI, 0.708–0.815), if used alone. In contrast, the prognostic performance of conventional clinical variables was less satisfactory (C-index=0.663, 95% CI, 0.600–0.725), consistent with the Kaplan-Meier curves in Figure 3. The AIC of RPC Model (150.73) and DPC Model (146.33) were also better than that of Clinical Model (158.61), suggesting better balance was achieved between reducing model regression error and complexity by the former two models.
Compared with the aforementioned single models, the predictive performance of the combined models was improved, as suggested by the lower AIC and higher C-index values shown in Tables 2, 3. Consistent with our hypothesis, the comprehensive model combining the radiomics principal components, dosiomics principal components and conventional clinical variables can best stratify the HPSCC patients with different risks of locoregional recurrence after radiotherapy, as suggested by the largest C-index (0.815) than any other models as shown in Tables 2 and 3. This result is also better than that of the previous models using radiomics alone, achieving C-index between 0.690-0.788 (12, 37, 38). Compared with other comprehensive models such as using clinical features + radiomics features by Boot (39) et al (C-index=0.73), and using CT + FDG-PET by Starke (40) et al (C-index=0.80), this work also achieved better reulsts. The comprehensive model also best balanced the ability to fit and the complexity of the model, as suggested by the lowest AIC value (143.65) in Tables 2, 3. We ascribe these improvements to the complementary incorporation of biological heterogeneity as represented by radiomics, personalized treatment intervention as depicted by dosiomics, and empirical evidence as reflected by clinical variables, which were all determinative factors of HPSCC patient outcomes treated with radiotherapy. Firstly, as reported before, the radiomic features are associated with the cancer microenvironment, genetic characteristics, cell growth and histological grading covering the whole tumor area (41–45), providing influential suggestions to the prognosis from the aspects of patient biology. Secondly, as treatment dose distribution was optimized deliberately based on patient-specific anatomies and target prescription, the high-dimensional dosiomic features characterize the personalized specification of treatment intervention. Thirdly, although less robust, clinical variables such as gender, age, stage and peripheral invasion are most familiar to the oncologists, and are broadly used as rule-of-thumb experience to predict the patient prognosis (46–49). The combined data provide complementary information in the quantitative prediction, which is more accurate than that of single factors.
Regarding accessibility, all the required data for the comprehensive modelling, including the planning CT images, dose distribution of treatment plans and conventional clinical variables are readily available for every patient before the start of radiotherapy, potentially facilitating the clinical application of this approach. It provides prompt suggestions before the start of radiotherapy, enabling possible reconsideration of receiving surgery for patients with high risk of local regional recurrence to achieve longer survival than receiving larynx preservation treatments. For patients that have received larynx preservation treatment but are predicted with high risk of local regional recurrence, our model can support personalized clinical suggestions such as more frequent monitoring and examination after radiotherapy.
Regarding methodologies, this work avoided the interference of collinearity to the radiomic and dosiomic features by using PCA. As reported by Traverso et al. (50), the radiomic features have multicollinearity and are largely dependent on tumor volume. Our approach searched for patterns in the data without assuming any a-priori distribution or condition. This study also avoided the bias from subjective selection of cut-off values, by using Youden index for all continuous variables involved in the Kaplan-Meier analysis, consistent with other researches (51, 52).
Although this work is limited by its retrospective design and the relatively small population due to the low morbidity of HPSCC, it provides additional personalized estimation tools and complementary clinical evidence to stratify patients with various risk of locoregional recurrence after radiotherapy, supporting personalized optimization of precision treatment strategies. For instance, if higher locoregional recurrence risks were predicted for a patient receiving function-preserving radiotherapy, more radical treatment such as total laryngectomy might be considered with multi-disciplinary evaluation.
5 Conclusions
This study demonstrates that comprehensive models combing radiomic, dosiomic and clinical components displayed better predictive values than any single factor for locoregionally advanced HPSCC treated with chemoradiotherapy, potentially supporting more accurate and prompt clinical decision making such as personalized treatment strategy selection and optimization.
Data availability statement
The raw data supporting the conclusions of this article will be made available by the authors, without undue reservation.
Ethics statement
The studies involving human participants were reviewed and approved by Peking University Cancer Hospital (IRB#2019YJZ76). The patients/participants provided their written informed consent to participate in this study.
Author contributions
HL, DZ and YH contributed equally to this work. HL was Responsible for Statistical Analysis (Email: stevendeliu@stu.pku.edu.cn). All authors contributed to the article and approved the submitted version.
Funding
The authors disclosed receipt of the following financial support for the research, authorship, and/or publication of this article: This research was supported by National Natural Science Foundation of China (12275012), Beijing Natural Science Foundation (Z210008); Science Foundation of Peking University Cancer Hospital (2021-1, PY202305, PY202306), Project 2020BD029 supported by PKU-Baidu Fund; Peking University Health Science Center Medical Education Research Funding Project (2020YB34), Peking University Emerging Engineering Interdisciplinary Project/the Fundamental Research Funds for the Central Universities (PKU2022XGK006), National Key R&D Program of China (2019YFF01014405), Open Project funded by Key Laboratory of Carcinogenesis and Translational Research, Ministry of Education/Beijing (2022 Open Project-2) and Inner Mongolia Science & Technology Project Plan (2022YFSH0064).
Acknowledgments
The authors thank Yan Sun, Baomin Zheng, Yichen Pu, Jian Zhang, Zhuolun Liu, Jian Gong, Zhongsu Feng, Fan Jiang, Qiaoqiao Hu, Kaining Yao, Sha Li, Meijiao Wang, Shun Zhou, and Yixiao Du for their assistance.
Conflict of interest
The authors declare that the research was conducted in the absence of any commercial or financial relationships that could be construed as a potential conflict of interest.
Publisher’s note
All claims expressed in this article are solely those of the authors and do not necessarily represent those of their affiliated organizations, or those of the publisher, the editors and the reviewers. Any product that may be evaluated in this article, or claim that may be made by its manufacturer, is not guaranteed or endorsed by the publisher.
References
1. Bar-Ad V, Palmer J, Yang H, Cognetti D, Curry J, Luginbuhl A, et al. Current management of locally advanced head and neck cancer: The combination of chemotherapy with locoregional treatments. Semin Oncol (2014), 41(6):798–806. doi: 10.1053/j.seminoncol.2014.09.018
2. Kuo P, Sosa JA, Burtness BA, Husain ZA, Mehra S, Roman SA, et al. Treatment trends and survival effects of chemotherapy for hypopharyngeal cancer: Analysis of the national cancer data base. Cancer (2016) 122:1853–60. doi: 10.1002/cncr.29962
3. Takes RP, Strojan P, Silver CE, Bradley PJ, Haigentz M, Wolf GT, et al. Current trends in initial management of hypopharyngeal cancer: the declining use of open surgery. Head Neck (2012) 34:270–81. doi: 10.1002/hed.21613
4. Street W. American Cancer Society. Cancer facts and figures 2021. (America: American Cancer Society). (2021).
5. Street W. American Cancer Society. Cancer facts and figures 2020. (America: American Cancer Society). (2020).
6. Song L, Zhang S, Yu S, Ma F, Wang B, Zhang C, et al. Cellular heterogeneity landscape in laryngeal squamous cell carcinoma. Int J Cancer (2020) 147:2879–90. doi: 10.1002/ijc.33192
7. Department of Veterans Affairs Laryngeal Cancer Study Group. Induction chemotherapy plus radiation compared with surgery plus radiation in patients with advanced laryngeal cancer. N Engl J Med (1991) 324:1685–90. doi: 10.1056/NEJM199106133242402
8. Beitler JJ, Zhang Q, Fu KK, Trotti A, Spencer SA, Jones CU, et al. Final results of local-regional control and late toxicity of RTOG 9003: A randomized trial of altered fractionation radiation for locally advanced head and neck cancer. Int J Radiat Oncol Biol Phys (2014) 89:13–20. doi: 10.1016/j.ijrobp.2013.12.027
9. Eskander A, Mifsud M, Irish J, Gullane P, Gilbert R, Brown D, et al. Overview of surgery for laryngeal and hypopharyngeal cancer in Ontario, 2003-2010. Head Neck (2017) 39:1559–67. doi: 10.1002/hed.24787
10. Yan BC, Li Y, Ma FH, Zhang GF, Feng F, Sun MH, et al. Radiologists with MRI-based radiomics aids to predict the pelvic lymph node metastasis in endometrial cancer: a multicenter study. Eur Radiol (2021) 31:411–22. doi: 10.1007/s00330-020-07099-8
11. Oikonomou A, Khalvati F, Tyrrell PN, Haider MA, Tarique U, Jimenez-Juan L, et al. Radiomics analysis at PET/CT contributes to prognosis of recurrence and survival in lung cancer treated with stereotactic body radiotherapy. Sci Rep (2018) 8:1–11. doi: 10.1038/s41598-018-22357-y
12. Mo X, Wu X, Dong D, Guo B, Liang C, Luo X, et al. Prognostic value of the radiomics-based model in progression-free survival of hypopharyngeal cancer treated with chemoradiation. Eur Radiol (2020) 30:833–43. doi: 10.1007/s00330-019-06452-w
13. Liang B, Yan H, Tian Y, Chen X, Yan L, Zhang T, et al. Dosiomics: Extracting 3D spatial features from dose distribution to predict incidence of radiation pneumonitis. Front Oncol (2019) 9:269. doi: 10.3389/fonc.2019.00269
14. Wu A, Li Y, Qi M, Lu X, Jia Q, Guo F, et al. Dosiomics improves prediction of locoregional recurrence for intensity modulated radiotherapy treated head and neck cancer cases. Oral Oncol (2020) 104:104625. doi: 10.1016/j.oraloncology.2020.104625
15. Amin MB, Greene FL, Edge SB, Compton CC, Gershenwald JE, Brookland RK, et al. The eighth edition AJCC cancer staging manual: Continuing to build a bridge from a population-based to a more “personalized” approach to cancer staging. CA: Cancer J Clin (2017) 67:93–9. doi: 10.3322/caac.21388
16. Grégoire V, Ang K, Budach W, Grau C, Hamoir M, Langendijk JA, et al. Delineation of the neck node levels for head and neck tumors: A 2013 update. DAHANCA, EORTC, HKNPCSG, NCIC CTG, NCRI, RTOG, TROG consensus guidelines. Radiother Oncol (2014) 110:172–81. doi: 10.1016/j.radonc.2013.10.010
17. Network NCC. Nccn clinical practice guidelines in oncology (Nccn guidelines) head and neck cancers version 2.2020. (America: Journal of the National Comprehensive Cancer Network) (2021).
18. Lee NY, Zhang Q, Pfister DG, Kim J, Garden AS, Mechalakos J, et al. Addition of bevacizumab to standard chemoradiation for locoregionally advanced nasopharyngeal carcinoma (RTOG 0615): A phase 2 multi-institutional trial. Lancet Oncol (2012) 13:172–80. doi: 10.1016/S1470-2045(11)70303-5
19. van Griethuysen JJM, Fedorov A, Parmar C, Hosny A, Aucoin N, Narayan V, et al. Computational radiomics system to decode the radiographic phenotype. Cancer Res (2017) 77:e104–7. doi: 10.1158/0008-5472.CAN-17-0339
20. Soufi M, Arimura H, Nagami N. Identification of optimal mother wavelets in survival prediction of lung cancer patients using wavelet decomposition-based radiomic features. Med Phys (2018) 45:5116–28. doi: 10.1002/mp.13202
21. Traverso A, Kazmierski M, Shi Z, Kalendralis P, Welch M, Nissen HD, et al. Stability of radiomic features of apparent diffusion coefficient (ADC) maps for locally advanced rectal cancer in response to image pre-processing. Physica Med (2019) 61:44–51. doi: 10.1016/j.ejmp.2019.04.009
22. Yaşar H, Ceylan M. A new radiomic study on lung CT images of patients with covid-19 using LBP and deep learning (Convolutional neural networks (CNN)). (2020). doi: 10.21203/rs.3.rs-30427/v1
23. Shrout PE, Fleiss JL. Intraclass correlations: Uses in assessing rater reliability. [J]. Psychological bulletin (1979), 86(2):420. doi: 10.1037/0033-2909.86.2.420
24. Panth KM, Leijenaar RTH, Carvalho S, Lieuwes NG, Yaromina A, Dubois L, et al. Is there a causal relationship between genetic changes and radiomics-based image features? an in vivo preclinical experiment with doxycycline inducible GADD34 tumor cells. Radiother Oncol (2015) 116:462–6. doi: 10.1016/j.radonc.2015.06.013
25. LeBreton JM, Senter JL. Answers to 20 questions about interrater reliability and interrater agreement. Organizat Res Methods (2008) 11:815–52. doi: 10.1177/1094428106296642
26. Rizzetto F, Calderoni F, De Mattia C, Defeudis A, Giannini V, Mazzetti S, et al. Impact of inter-reader contouring variability on textural radiomics of colorectal liver metastases. Eur Radiol Exp (2020) 4:62. doi: 10.1186/s41747-020-00189-8
27. Kleinbaum DG, Klein M. Kaplan-Meier Survival curves and the log-rank test. In: Survival analysis. (America: Springer) (2012). p. 55–96.
28. Sakamoto Y, Ishiguro M, Kitagawa G. Akaike information criterion statistics. Dordrecht Netherlands: D Reidel (1986) 81:26853. doi: 10.1080/01621459.1988.10478680
29. Harrell FE Jr, Lee KL, Califf RM, Pryor DB, Rosati RA. Regression modelling strategies for improved prognostic prediction. Stat Med (1984) 3:143–52. doi: 10.1002/sim.4780030207
30. Kuha J. AIC and BIC: Comparisons of assumptions and performance. Sociological Methods Res (2004) 33:188–229. doi: 10.1177/0049124103262065
31. Xiang A, Lapuerta P, Ryutov A, Buckley J, Azen S. Comparison of the performance of neural network methods and cox regression for censored survival data. Comput Stat Data Anal (2000) 34:243–57. doi: 10.1016/S0167-9473(99)00098-5
32. Bradley PJ. Epidemiology of hypopharyngeal cancer. Hypopharyngeal Cancer (2019) 83:1–14. doi: 10.1159/000492299
33. Visini M, Giger R, Shelan M, Elicin O, Anschuetz L. Predicting factors for oncological and functional outcome in hypopharyngeal cancer. Laryngoscope (2021) 131:E1543–9. doi: 10.1002/lary.29186
34. Arends CR, Petersen JF, van der Noort V, Timmermans AJ, Leemans CR, de Bree R, et al. Optimizing survival predictions of hypopharynx cancer: development of a clinical prediction model. Laryngoscope (2020) 130:2166–72. doi: 10.1002/lary.28345
35. Lin Z, Lin H, Lin C. Dynamic prediction of cancer-specific survival for primary hypopharyngeal squamous cell carcinoma. Int J Clin Oncol (2020) 25:1260–9. doi: 10.1007/s10147-020-01671-4
36. Coroller TP, Grossmann P, Hou Y, Rios Velazquez E, Leijenaar RTH, Hermann G, et al. CT-based radiomic signature predicts distant metastasis in lung adenocarcinoma. Radiother Oncol (2015) 114:345–50. doi: 10.1016/j.radonc.2015.02.015
37. Aerts HJWL, Velazquez ER, Leijenaar RTH, Parmar C, Grossmann P, Carvalho S, et al. Decoding tumour phenotype by noninvasive imaging using a quantitative radiomics approach. Nat Commun (2014) 5:4006. doi: 10.1038/ncomms5006
38. Leger S, Zwanenburg A, Pilz K, Zschaeck S, Zöphel K, Kotzerke J, et al. CT imaging during treatment improves radiomic models for patients with locally advanced head and neck cancer. Radiother Oncol (2019) 130:10–7. doi: 10.1016/j.radonc.2018.07.020
39. Boot PA, Mes SW, de Bloeme CM, Martens RM, Leemans CR, Boellaard R, et al. Magnetic resonance imaging based radiomics prediction of human papillomavirus infection status and overall survival in oropharyngeal squamous cell carcinoma. Oral Oncol (2023) 137:106307. doi: 10.1016/j.oraloncology.2023.106307
40. Starke S, Zwanenburg A, Leger K, Zöphel K, Kotzerke J, Krause M, et al. Longitudinal and multimodal radiomics models for head and neck cancer outcome prediction. Cancers (Basel) (2023) 15:673. doi: 10.3390/cancers15030673
41. Lambin P, Rios-Velazquez E, Leijenaar R, Carvalho S, van Stiphout RGPM, Granton P, et al. Radiomics: Extracting more information from medical images using advanced feature analysis. Eur J Cancer (2012) 48:441–6. doi: 10.1016/j.ejca.2011.11.036
42. Chen S-W, Shen W-C, Lin Y-C, Chen R-Y, Hsieh T-C, Yen K-Y, et al. Correlation of pretreatment 18 f-FDG PET tumor textural features with gene expression in pharyngeal cancer and implications for radiotherapy-based treatment outcomes. Eur J Nucl Med Mol Imaging (2017) 44:567–80. doi: 10.1007/s00259-016-3580-5
43. Leijenaar RT, Carvalho S, Hoebers FJ, Aerts HJ, Van Elmpt WJ, Huang SH, et al. External validation of a prognostic CT-based radiomic signature in oropharyngeal squamous cell carcinoma. Acta Oncol (2015) 54:1423–9. doi: 10.3109/0284186X.2015.1061214
44. Song J, Shi J, Dong D, Fang M, Zhong W, Wang K, et al. A new approach to predict progression-free survival in stage IV EGFR-mutant NSCLC patients with EGFR-TKI therapy. Clin Cancer Res (2018) 24:3583–92. doi: 10.1158/1078-0432.CCR-17-2507
45. Gillies RJ, Kinahan PE, Hricak H. Radiomics: Images are more than pictures, they are data. Radiology (2016) 278:563–77. doi: 10.1148/radiol.2015151169
46. Kim S-Y, Rho Y-S, Choi E-C, Kim M-S, Woo J-H, Lee DH, et al. Clinicopathological factors influencing the outcomes of surgical treatment in patients with T4a hypopharyngeal cancer. BMC Cancer (2017) 17:1–7. doi: 10.1186/s12885-017-3880-6
47. Scherl C, Mantsopoulos K, Semrau S, Fietkau R, Kapsreiter M, Koch M, et al. Management of advanced hypopharyngeal and laryngeal cancer with and without cartilage invasion. Auris Nasus Larynx (2017) 44:333–9. doi: 10.1016/j.anl.2016.08.002
48. Gong EJ, Kim DH, Ahn JY, Choi K-S, Jung KW, Lee JH, et al. Routine endoscopic screening for synchronous esophageal neoplasm in patients with head and neck squamous cell carcinoma: A prospective study. Dis Esophagus (2016) 29:752–9. doi: 10.1111/dote.12404
49. Patel UA, Howell LK. Local response to chemoradiation in T4 larynx cancer with cartilage invasion. Laryngoscope (2011) 121:106–10. doi: 10.1002/lary.21181
50. Traverso A, Kazmierski M, Zhovannik I, Welch M, Wee L, Jaffray D, et al. Machine learning helps identifying volume-confounding effects in radiomics. Physica Med (2020) 71:24–30. doi: 10.1016/j.ejmp.2020.02.010
51. Kremer A, Kremer T, Kristiansen G, Tolkach Y. Where is the limit of prostate cancer biomarker research? systematic investigation of potential prognostic and diagnostic biomarkers. BMC Urol (2019) 19:1–10. doi: 10.1186/s12894-019-0479-z
Keywords: hypopharyngeal squamous cell carcinoma, radiomics, dosiomics, Cox regression, locoregional recurrence
Citation: Liu H, Zhao D, Huang Y, Li C, Dong Z, Tian H, Sun Y, Lu Y, Chen C, Wu H and Zhang Y (2023) Comprehensive prognostic modeling of locoregional recurrence after radiotherapy for patients with locoregionally advanced hypopharyngeal squamous cell carcinoma. Front. Oncol. 13:1129918. doi: 10.3389/fonc.2023.1129918
Received: 22 December 2022; Accepted: 13 March 2023;
Published: 21 March 2023.
Edited by:
Giuseppe Carlo Iorio, University of Turin, ItalyCopyright © 2023 Liu, Zhao, Huang, Li, Dong, Tian, Sun, Lu, Chen, Wu and Zhang. This is an open-access article distributed under the terms of the Creative Commons Attribution License (CC BY). The use, distribution or reproduction in other forums is permitted, provided the original author(s) and the copyright owner(s) are credited and that the original publication in this journal is cited, in accordance with accepted academic practice. No use, distribution or reproduction is permitted which does not comply with these terms.
*Correspondence: Yibao Zhang, zhangyibao@pku.edu.cn
†These authors have contributed equally to this work