- 1Department of Anesthesiology, The Fourth hospital of Hebei Medical University, Shijiazhuang, Hebei, China
- 2Department of Anesthesiology, Zhuji People’s Hospital, Shaoxing, Zhejiang, China
Background: The prediction model of postoperative pneumonia (POP) after lung cancer surgery is still scarce.
Methods: Retrospective analysis of patients with lung cancer who underwent surgery at The Fourth Hospital of Hebei Medical University from September 2019 to March 2020 was performed. All patients were randomly divided into two groups, training cohort and validation cohort at the ratio of 7:3. The nomogram was formulated based on the results of multivariable logistic regression analysis and clinically important factors associated with POP. Concordance index (C-index), receiver operating characteristic (ROC) curve, calibration curve, Hosmer-Lemeshow goodness-of-fit test and decision curve analysis (DCA) were used to evaluate the predictive performance of the nomogram.
Results: A total of 1252 patients with lung cancer was enrolled, including 877 cases in the training cohort and 375 cases in the validation cohort. POP was found in 201 of 877 patients (22.9%) and 89 of 375 patients (23.7%) in the training and validation cohorts, respectively. The model consisted of six variables, including smoking, diabetes mellitus, history of preoperative chemotherapy, thoracotomy, ASA grade and surgery time. The C-index from AUC was 0.717 (95%CI:0.677-0.758) in the training cohort and 0.726 (95%CI:0.661-0.790) in the validation cohort. The calibration curves showed the model had good agreement. The result of DCA showed that the model had good clinical benefits.
Conclusion: This proposed nomogram could predict the risk of POP in patients with lung cancer surgery in advance, which can help clinician make reasonable preventive and treatment measures.
Introduction
As the Global Cancer Statistics reported in 2020, lung cancer has become the second most common cancer and the highest rate of cancer-related death (1). The treatments of lung cancer mainly include radiotherapy, chemotherapy, surgery, targeted therapy, immunotherapy. However, surgical resection is still an effective and safe intervention for patients with lung cancer.
Unfortunately, the postoperative pulmonary complications (PPCs) of lung cancer surgery are still common problems and major challenges for patient recovery. And postoperative pneumonia (POP) has become the most common PPCs (2, 3). Several studies have found that the rate of POP in lung cancer patients is about 2%-25% (4–7). And POP could significantly prolong the length of hospitalization, increase hospitalization expense, and even increase perioperative mortality (4, 8–10). Thus, early identification of risk factors associated with POP among patients with lung cancer could be beneficial to forecast the risk of POP in advance.
Potential risk factors for POP existed throughout the whole perioperative period (11). Preoperative factors mainly include elderly, smoking, pulmonary function, comorbidities and nutritional status (12, 13). Intraoperative factors include surgery types, duration of surgery, anesthesia types and ventilation mode (6, 14). Postoperative factors include acute pain and other complications (15, 16). A retrospective observational cohort (n=7479) found that elderly, preoperative pulmonary infection, atrial fibrillation, obesity, and alcohol might be associated with POP among lung cancer patients (10). Deguchi et al. (5) found preoperative asthma might also be independently associated with POP in lung cancer patients. And Yendamuri et al. (17) found age >75 years, male, thoracotomy, COPD and American Society of Anesthesiologists (ASA) ≥III might be potential risk factors after analyzing a range of patients (n=12562) who underwent pulmonary lobectomy. However, most of the present studies on POP in lung cancer patients had only identified risk factors for POP, studies about prediction models for POP were very limited.
Although there had a model to predict POP for elderly patients who underwent video-assisted thoracoscopic surgery for lung cancer, the data was collected from 2012 to 2019 and other variables associating with POP such as anesthesia types, anesthetics and surgery types were scarce (4). Thus, the development of a model after fully considering the preoperative and intraoperative variables to forecast the risk of POP in advance would be beneficial to patients.
Therefore, the purpose of this study was to develop and validate a model to predict POP in patients undergoing lung cancer surgery and investigate risk factors for POP so that reasonable preventive and treatment measures could be made earlier.
Methods
Study design
This is a retrospective cohort study, which has been approved by Clinical Research Ethics Committee of the Fourth Hospital of Hebei Medical University (No:2022KS024), Shijiazhuang, Hebei Province, China (Chairperson Prof Hongtao He) on 28 July 2022. The informed consent was exempted with the approval of the local ethics committee. All patients were randomly separated into training and validation cohorts at the ratio of 7:3 which was similar to other studies (18–20). The training cohort was conducted to develop prediction model, while both training and validation cohorts were used to verify the predictive ability of the model.
Participants
We retrospectively analyzed patients who underwent thoracic surgery from September 2019 to March 2020 at the Fourth Hospital of Hebei Medical University. The inclusion criteria were patients aged ≥ 18 years with pathology diagnosis of lung cancer and underwent surgery. Patients were excluded if they met one or more of following criteria: 1) preoperative pneumonia diagnosed by computed tomography (CT), 2) bilateral pulmonary resection, 3) reoperation within 30 days, 4) admitted to ICU after surgery, 5) missing data.
Perioperative management
All patients received general anesthesia, either alone or in combined with regional nerve block (including paravertebral nerve block, epidural anesthesia, and intercostal nerve block.) according to the type of surgery. Patients underwent lobectomy or sublobectomy according to surgeon’s comprehensive evaluation based on patient’s condition.
Anesthesia induction used propofol and/or etomidate, sufentanil, and rocuronium or cisatracurium. Anesthesia maintenance used sevoflurane or propofol combined with remifentanil or sufentanil. Rocuronium or cisatracurium was used to maintain muscle relaxation. Supplemental drugs such as flurbiprofen axetil were administered when necessary. The aim was to maintain BIS 40-60, blood pressure within 20% of baseline, and temperature 36-37°C.
Double-lumen endotracheal tube of sizes Ch33-39 was used for lung isolation according to patient height. The ventilation mode was volume control mode with 6-8 ml/kg of tidal volume (TV) during two-lung ventilation and 5-6 ml/kg during one-lung ventilation (OLA), and 0-5 cmH2O of positive end-expiratory pressure (PEEP), and 12-20 breaths/min of respiratory rates. The aim was to maintain PETCO2 35-45 mmHg and SpO2 ≥92%. At the end of anesthesia, neostigmine was used to antagonize muscular relaxant before extubation.
Fluid infusion was administrated with crystalloid at a rate of 4–6 mL/kg-1h-1. Colloids or blood product was used according to anesthesiologist’s comprehensive evaluation based on patient’s condition. Patient-controlled intravenous analgesia was used after surgery for postoperative analgesia to maintain numeric rating scales (NRS) ≤ 3 scores.
Data collection
We collected following variables, including: 1) basic demographics such as age, sex, history of smoking, body mass index (BMI), preoperative chemotherapy, history of lung surgery; 2) preoperative comorbidities containing hypertension, chronic obstructive pulmonary disease (COPD), asthma, diabetes, coronary heart disease, arrhythmia; 3) preoperative laboratory testing including Hemoglobin, serum albumin, Serum glucose; 4) preoperative pulmonary function including forced vital capacity rate of one second(FEV1/FVC), diffusion capacity for carbon monoxide of the lung(DLCO); 5) surgery related characteristics including surgery types, surgery extent, surgery sides, duration of surgery; 6) anesthesia related characteristics including ASA grade, anesthesia types, use of flurbiprofen axetil, use of colloid, allogenic blood transfusion, Input per unit of time (ml·kg-1·h-1). Smoking was defined as smoking index ≥ 400. Duration of surgery was defined as the time interval between skin incision and suture. Input per unit of time was equal to total input divided by duration of surgery and actual weight.
Diagnosis of pneumonia
POP was occurred during hospitalization, which defined as follows (21): patient has received antibiotics for a suspected respiratory infection and met one or more of the following criteria: 1) new or changed sputum, 2) new or changed lung opacities, 3) fewer (>38.3°C), 4) white blood cell count >12×109/L.
Statistical analysis
The normal distribution data were present as mean ± standard deviation (SD) and compared by the independent sample t-test, while the non-normal distribution data were present as median(Q1, Q3) and compared by the Wilcoxon test. And the categorical data were present as number and percentages, and compared by the Chi-square test.
The Least Absolute Shrinkage and Selection Operator (LASSO) logistic regression with 5-fold cross-validation was used to adjust the parameter lambda to screen the variables. And the lambda corresponding to the minimum mean square error was used for selecting variables. The multivariate logistic regression analysis was used to analyze characteristic variables selected by LASSO regression to explore the independent risk factors associated with POP. A nomogram was built according to the independent risk factors and clinically important factors associated with POP.
The AUROC and C index were used to measure the discrimination ability according to the data from training and validation cohorts. And the calibration ability was measured by the calibration curve and Hosmer-Lemeshow goodness-of-fit test. The clinical benefit was measured by the decision curve analysis (DCA). Statistical analysis was performed with R software (version 3.5.3; https://www.R-project.org). A p<0.05 with two sides was considered statistical significance.
Results
Participants
We initially screened 1651 patients who underwent thoracic surgery from September 2019 to March 2020 (Figure 1). Of these, 233 patients were benign mass and the rest of 1418 patients were included in the study. After data collection, 166 patients were removed from the final analysis: 98 patients had preoperative pneumonia confirmed by CT; 31 patients required bilateral pulmonary resection; 13 patients required reoperation within 30 days; 14 patients admitted to ICU after surgery; 10 patients had missing data. Finally, a total of 1252 patients were admitted into the present study. The training cohort had 877 patients who were aged 60.0 ± 9.4 years. The validation cohort consisted of 375 patients who were aged 59.5 ± 9.7 years. The incidence of POP was 22.9% in the training cohort, 23.7% in the validation cohort and 23.2% among all patients. All variables expect for Hemoglobin (P<0.05) were no statistically significant differences between two groups (other P > 0.05) (Table 1).
Development of prediction model
The protential risk factors of POP were selected by LASSO regression (Figure 2). The coefficients of relatively irrelevant variables were minimized to 0 and subsequently were excluded according to the value of lambda. The LASSO regression showed the optimal value of lambda was 0.015 (Table 2). And 12 non-zero representative variables were remained, including age, history of smoking, diabetes, preoperative chemotherapy, FEV1/FVC, DLCO, surgery type, ASA grade, use of flurbiprofen axetil, use of colloid, input per unit of time and duration of surgery (Table 2).
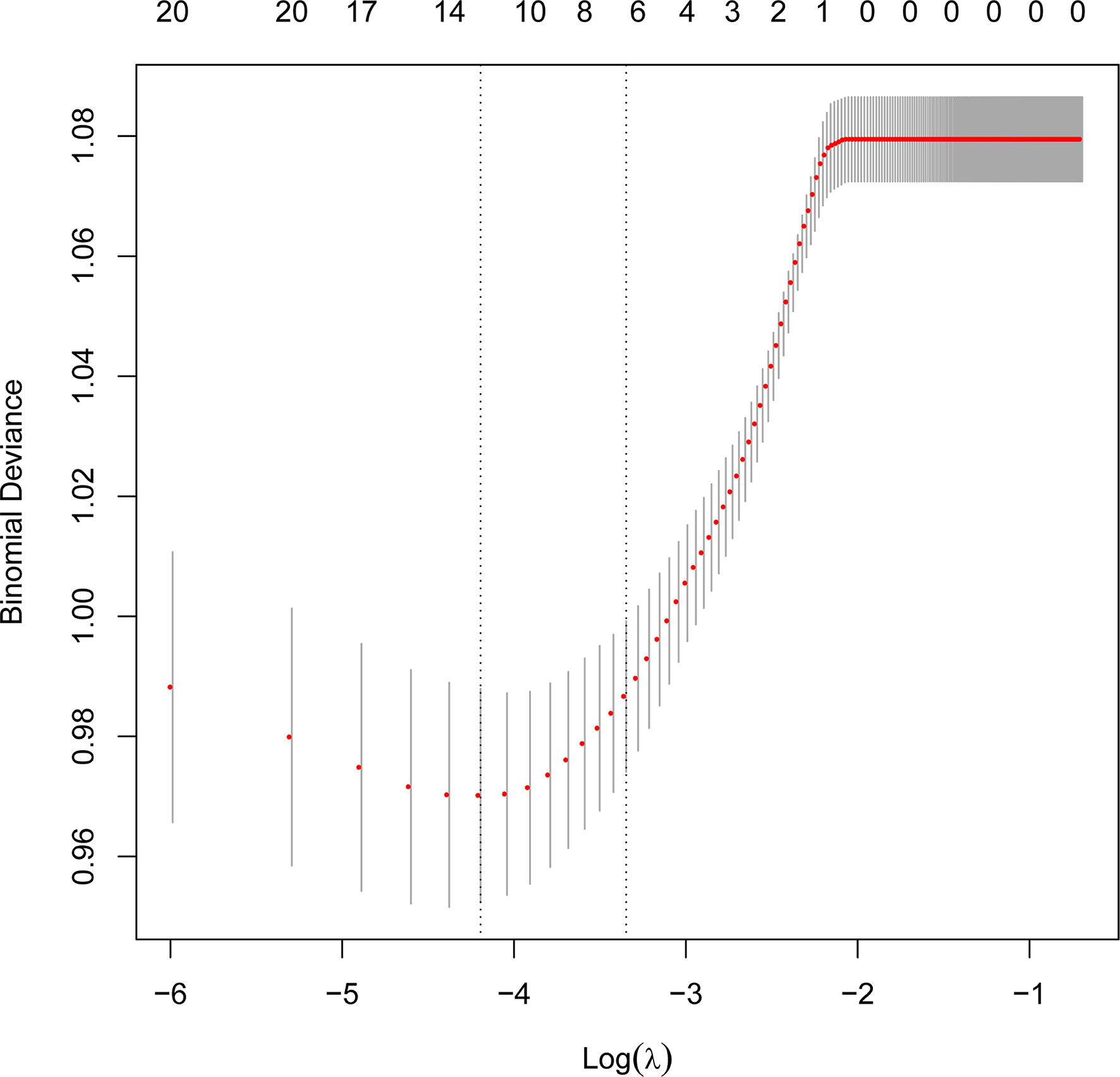
Figure 2 Perioperative variables selection using the Least Absolute Shrinkage and Selection Operator (LASSO) regression.
On the multivariate logistic regression analysis, there were five variables independently associated with POP, including diabetes (OR=1.838; 95%CI:1.110-3.001); preoperative chemotherapy (OR=3.997; 95%CI:2.014-8.093); Thoracotomy (OR=1.891; 95%CI:1.126-3.138); ASA grade (OR=1.760; 95%CI:1.105-2.780); and duration of surgery (OR=1.486; 95%CI:1.268-1.750) (Table 3).
Although multivariate logistic regression analysis showed smoking was not an independent factor, the coefficient of smoking was larger according to LASSO regression. We thought smoking might influence the incidence of POP. So we used five independent risk factors and smoking to draw a nomogram to develop a POP prediction model. The dynamic nomogram of POP is available online (https://lungcancersurgery.shinyapps.io/DynNomapp/). The code of dynamic nomogram is presented in Supplement Material.
Validation of prediction model
In the present, the uncorrected C index was 0.717 (95%CI:0.677-0.758) and bootstrap-corrected C index was 0.710 in the training cohort, while the uncorrected C index was 0.726 (95%CI: 0.661-0.790) and bootstrap-corrected C index was 0.709 in the validation cohort (Figure 3). These results showed the nomogram had good accuracy in distinguishing patients with and without POP. Besides, the calibration curve showed good consistency on the presence of POP between prediction by the nomogram and results of actual clinical data (Figure 4), which demonstrated by Hosmer-Lemeshow goodness-of-fit test both in the training and validation cohorts (both P >0.05). At the same time, the decision curve analysis showed a positive net benefit when the predicted probability threshold is 0%-80%, indicating this nomogram had good clinical benefit (Figure 5).
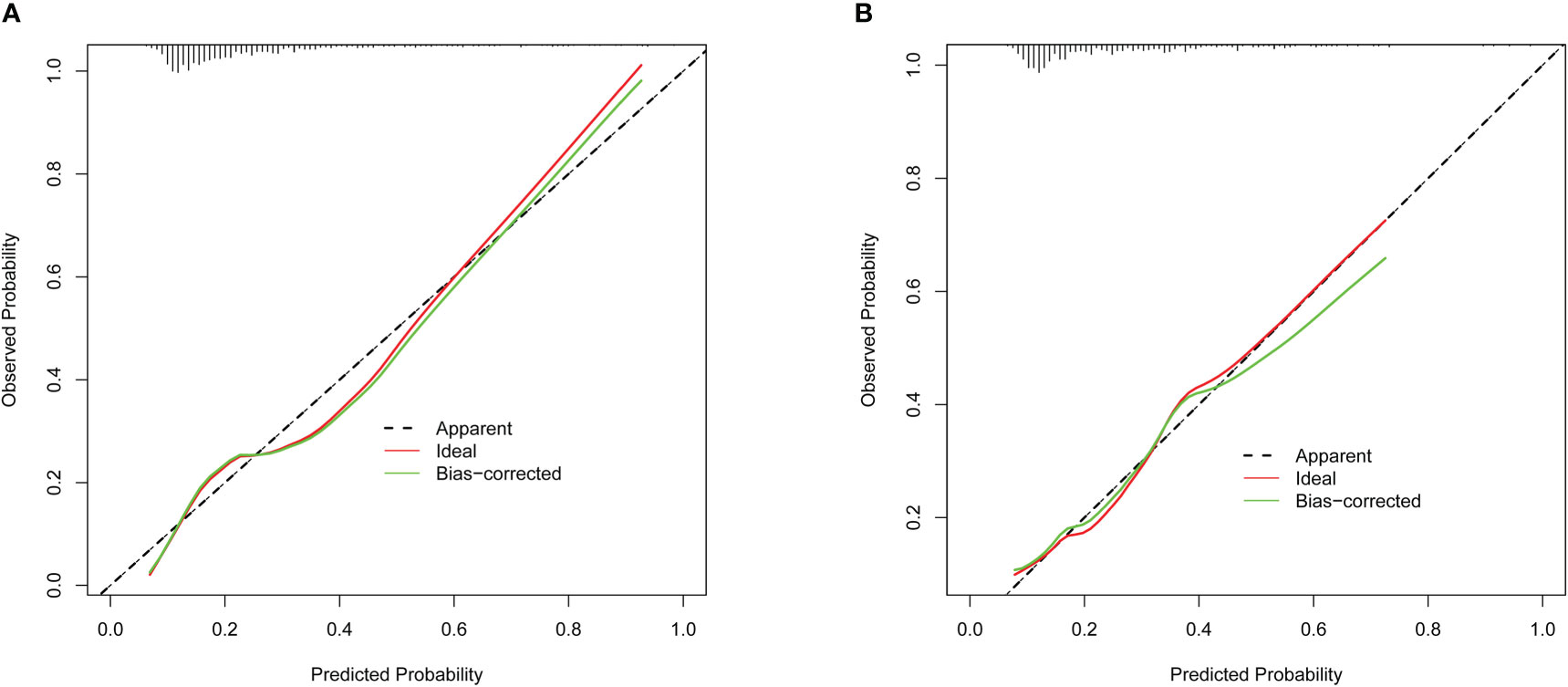
Figure 4 The calibration curve of POP risk nomogram. (A) Calibration curve in the training cohort (n = 877). (B) Calibration curve in the validation cohort (n = 375).
Discussion
This study developed and validated a nomogram to accurately forecast the risk of POP in patients who underwent lung cancer surgery. This model included six preoperative and intraoperative variables, including smoking, diabetes, preoperative chemotherapy, surgery types, ASA grade and duration of surgery, which predicted well as demonstrated by the uncorrected C index values of 0.717 and 0.726 in the training and validation cohorts. At the same time, the calibration curves showed good consistency between prediction and actual observation, respectively, and the decision curve analysis indicated this nomogram had good clinical benefit.
LASSO regression is a common method for variable selection in fitting high-dimensional generalized linear and has been widely used in clinical research (22, 23). The LASSO method selects variables via minimizing the coefficients of relatively irrelevant variables to 0 and subsequently removing these variables by constructing a penalty function, which effectively avoids the overfitting and makes the model more refined (24, 25). So we used the LASSO regression for variable selection in this study.
The nomogram we used is a superior visual tool and has been widely used in the clinical practice, which has several advantages. Firstly, the nomogram could transform predictive model into a single estimate of probability according to patient’s characteristics, making model simple to understand (26). Secondly, the scoring system has high precision and good stability characteristics in predicting results (27, 28). Therefore, we used nomogram to build the visual model to help clinicians to stratify patients and develop individual clinical treatment strategies according to patient’s conditions.
Evaluating the characteristics of the predictive model from multiple perspectives and selecting the optimal model could help promotion and application of the model (29). The calibration ability is model’s capability to demonstrate the consistency between the actual observed and the prediction by the model, which is one of the best indicators to reflect predictive performance of the model (29). Therefore, we used calibration curve and Hosmer-Lemeshow goodness-of-fit test to evaluate calibration ability of this model. And good agreements between prediction and actual observation were supported by Hosmer-Lemeshow goodness-of-fit test. However, good calibration couldn’t perfectly distinguish patients with or without POP. The ROC curve and C index had certain advantages in measuring discrimination ability of the model (30). And the results showed that the model could distinguish patients with and without POP. Furthermore, we used the decision curve analysis and net benefit to evaluate the clinical benefit of the model (31). The results suggested that using this model to assist clinical treatment strategies might help improve patient prognosis.
In the POP risk estimation nomogram, preoperative chemotherapy, thoracotomy, ASA and duration of surgery have been confirmed to increase the risk of POP (17, 32, 33). This study showed that above factors were also independent risk factors associated with POP in lung cancer patients. In addition, we illustrated that diabetes was associated with POP in patients with lung cancer surgery.
In previous reports, diabetes was associated with POP after surgery (34). The incidence of POP in type 2 diabetes mellitus patients was 21% higher than non-diabetic patients (35). In the present study, we found the risk of POP was higher in diabetes patients (OR=1.838; 95%CI:1.110-3.001) after lung cancer surgery. The potential mechanisms were diabetes could destroy innate immunity in pulmonary, impair pulmonary function and reduce cardiorespiratory fitness making patients more susceptible to infections (36).
However, this study still has some limitations. At first, the bias of patient selection could not be entirely avoided because it was a single-center retrospective study. However, we screened patients through strict inclusion and exclusion criteria, which could reduce population homogeneity to some extent. Secondly, the definition of POP in our study might be generic and sensitive to diagnose pneumonia, which made the incidence of POP a little higher than other studies. However, we used the same criteria in diagnosing pneumonia in our study which could make result reliable to some extent. Thirdly, intraoperative respiratory parameters, such as tidal volume, minute ventilation, PEEP were not included in our study because these variables could not be collected. However, some studies had found that intraoperative ventilation strategy might not be associated with postoperative pulmonary complications (37, 38). Finally, some postoperative variables, such as postoperative pain, postoperative aerosolized inhalation might influence the rate of POP among patients after lung cancer surgery, but these variables were not analyzed in the study because we aimed at predicting POP through preoperative and intraoperative variables rather than postoperative variables.
Conclusion
This study developed and validated a predictive model representing by the nomogram to quantify the risk of POP in patients with lung cancer surgery. This model showed good discrimination ability, calibration ability and clinical benefit which could help make better prevention and individual treatment strategies in advance.
Data availability statement
The code of dynamic nomogram in the study is included in the article/Supplementary Material. Further inquiries can be directed to the corresponding author.
Ethics statement
The study involving human participants was reviewed and approved by Ethics Committee of The Fourth hospital of Hebei Medical University. Written informed consent for participation was not required for this study in accordance with the national legislation and the institutional requirements.
Author contributions
JF: This author helped in data acquisition, data analysis, and manuscript drafting. LW: This author helped in data acquisition, and data analysis. XQ: This author helped in data acquisition, and data analysis. JS: This author helped in data acquisition. RX: This author helped in data acquisition. H-QJ: This author helped in concept and design, data analysis, data interpretation, revision of the manuscript, and final approval of submission. All authors contributed to the article and approved the submitted version.
Conflict of interest
The authors declare that the research was conducted in the absence of any commercial or financial relationships that could be construed as a potential conflict of interest.
Publisher’s note
All claims expressed in this article are solely those of the authors and do not necessarily represent those of their affiliated organizations, or those of the publisher, the editors and the reviewers. Any product that may be evaluated in this article, or claim that may be made by its manufacturer, is not guaranteed or endorsed by the publisher.
Supplementary material
The Supplementary Material for this article can be found online at: https://www.frontiersin.org/articles/10.3389/fonc.2023.1114302/full#supplementary-material
Abbreviations
PPCs, postoperative pulmonary complications; POP, postoperative pneumonia; LASSO, least absolute shrinkage and selection operator; ROC, receiver operating characteristic; C index, concordance index; DCA, decision curve analysis; ASA, American Society of Anesthesiologists; TV, tidal volume; PEEP, positive end-expiratory pressure; BMI, body mass index; COPD, chronic obstructive pulmonary disease; FEV1/FVC, forced vital capacity rate of one second; DLCO, diffusion capacity for carbon monoxide of the lung; VATS, Video-assisted Thoracoscopic Surgery; SD, standard deviation; GA, general anesthesia; RA, regional nerve block; OR, odds ratio; CI, confidence interval; OLV, one-lung ventilation.
References
1. Sung H, Ferlay J, Siegel RL, Laversanne M, Soerjomataram I, Jemal A, et al. Global cancer statistics 2020: Globocan estimates of incidence and mortality worldwide for 36 cancers in 185 countries. CA Cancer J Clin (2021) 71:209–49. doi: 10.3322/caac.21660
2. Choi JW, Jeong H, Ahn HJ, Yang M, Kim JA, Kim DK, et al. The impact of pulmonary function tests on early postoperative complications in open lung resection surgery: An observational cohort study. Sci Rep (2022) 12:1277. doi: 10.1038/s41598-022-05279-8
3. Chang S, Zhou K, Wang Y, Lai Y, Che G. Prognostic value of preoperative peak expiratory flow to predict postoperative pulmonary complications in surgical lung cancer patients. Front Oncol (2021) 11:782774. doi: 10.3389/fonc.2021.782774
4. Song Y, Liu J, Lei M, Wang Y, Fu Q, Wang B, et al. An external-validated algorithm to predict postoperative pneumonia among elderly patients with lung cancer after video-assisted thoracoscopic surgery. Front Oncol (2021) 11:777564. doi: 10.3389/fonc.2021.777564
5. Motono N, Ishikawa M, Iwai S, Iijima Y, Usuda K, Uramoto H. Individualization of risk factors for postoperative complication after lung cancer surgery: A retrospective study. BMC Surg (2021) 21:311. doi: 10.1186/s12893-021-01305-0
6. Yang R, Du C, Xu J, Yao L, Zhang S, Wu Y. Excessive intravenous crystalloid infusion after video-assisted thoracoscopic surgery lobectomy is associated with postoperative pneumonia. J Cardiothorac Surg (2019) 14:209. doi: 10.1186/s13019-019-1024-6
7. Schussler O, Alifano M, Dermine H, Strano S, Casetta A, Sepulveda S, et al. Postoperative pneumonia after major lung resection. Am J Respir Crit Care Med (2006) 173:1161–9. doi: 10.1164/rccm.200510-1556OC
8. Fujishima S, Tsujimoto H, Nagata K, Sugasawa H, Nomura S, Ito N, et al. Postoperative pneumonia causes the loss of skeletal muscle volume and poor prognosis in patients undergoing esophagectomy for esophageal cancer. Gen Thorac Cardiovasc Surg (2021) 69:84–90. doi: 10.1007/s11748-020-01482-4
9. Zhang D, Zhuo H, Yang G, Huang H, Li C, Wang X, et al. Postoperative pneumonia after craniotomy: Incidence, risk factors and prediction with a nomogram. J Hosp Infect (2020) 105:167–75. doi: 10.1016/j.jhin.2020.03.015
10. Simonsen DF, Sogaard M, Bozi I, Horsburgh CR, Thomsen RW. Risk factors for postoperative pneumonia after lung cancer surgery and impact of pneumonia on survival. Respir Med (2015) 109:1340–6. doi: 10.1016/j.rmed.2015.07.008
11. Walder B, Story DA. Postoperative pneumonia: Can this important complication be predicted and anticipated? Eur J Anaesthesiol. (2019) 36:87–9. doi: 10.1097/EJA.0000000000000922
12. Maruyama S, Okamura A, Ishizuka N, Kanie Y, Sakamoto K, Fujiwara D, et al. Airflow limitation predicts postoperative pneumonia after esophagectomy. World J Surg (2021) 45:2492–500. doi: 10.1007/s00268-021-06148-7
13. Liu GW, Sui XZ, Wang SD, Zhao H, Wang J. Identifying patients at higher risk of pneumonia after lung resection. J Thorac Dis (2017) 9:1289–94. doi: 10.21037/jtd.2017.04.42
14. Deng QW, Tan WC, Zhao BC, Wen SH, Shen JT, Xu M. Intraoperative ventilation strategies to prevent postoperative pulmonary complications: A network meta-analysis of randomised controlled trials. Br J Anaesth. (2020) 124:324–35. doi: 10.1016/j.bja.2019.10.024
15. Popping DM, Elia N, Van Aken HK, Marret E, Schug SA, Kranke P, et al. Impact of epidural analgesia on mortality and morbidity after surgery: Systematic review and meta-analysis of randomized controlled trials. Ann Surg (2014) 259:1056–67. doi: 10.1097/SLA.0000000000000237
16. Lee JY, Jin SM, Lee CH, Lee BJ, Kang CH, Yim JJ, et al. Risk factors of postoperative pneumonia after lung cancer surgery. J Kor Med Sci (2011) 26:979–84. doi: 10.3346/jkms.2011.26.8.979
17. Yendamuri S, Battoo A, Attwood K, Dhillon SS, Dy GK, Hennon M, et al. Concomitant mediastinoscopy increases the risk of postoperative pneumonia after pulmonary lobectomy. Ann Surg Oncol (2018) 25:1269–76. doi: 10.1245/s10434-018-6397-6
18. Huang L, Lin W, Xie D, Yu Y, Cao H, Liao G, et al. Development and validation of a preoperative ct-based radiomic nomogram to predict pathology invasiveness in patients with a solitary pulmonary nodule: A machine learning approach, multicenter, diagnostic study. Eur Radiol (2022) 32:1983–96. doi: 10.1007/s00330-021-08268-z
19. Yang S, Zhang Q, Xu Y, Chen F, Shen F, Zhang Q, et al. Development and validation of nomogram prediction model for postoperative sleep disturbance in patients undergoing non-cardiac surgery: A prospective cohort study. Nat Sci Sleep. (2021) 13:1473–83. doi: 10.2147/NSS.S319339
20. Wang D, Chen X, Wu J, Le S, Xie F, Li X, et al. Development and validation of nomogram models for postoperative pneumonia in adult patients undergoing elective cardiac surgery. Front Cardiovasc Med (2021) 8:750828. doi: 10.3389/fcvm.2021.750828
21. Mazo V, Sabate S, Canet J, Gallart L, de Abreu MG, Belda J, et al. Prospective external validation of a predictive score for postoperative pulmonary complications. Anesthesiology (2014) 121:219–31. doi: 10.1097/ALN.0000000000000334
22. Li J, Liang K, Song X. Logistic regression with adaptive sparse group lasso penalty and its application in acute leukemia diagnosis. Comput Biol Med (2022) 141:105154. doi: 10.1016/j.compbiomed.2021.105154
23. Tang G, Qi L, Sun Z, Liu J, Lv Z, Chen L, et al. Evaluation and analysis of incidence and risk factors of lower extremity venous thrombosis after urologic surgeries: A prospective two-center cohort study using lasso-logistic regression. Int J Surg (2021) 89:105948. doi: 10.1016/j.ijsu.2021.105948
24. Tibshirani R. The lasso method for variable selection in the cox model. Stat Med (1997) 16:385–95. doi: 10.1002/(sici)1097-0258(19970228)16:4<385::aid-sim380>3.0.co;2-3
25. Tibshirani R. Regression shrinkage and selection via the lasso. J R Stat Soc(Methodological) (1996) 58:267–88. doi: 10.1111/j.2517-6161.1996.tb02080.x
26. Iasonos A, Schrag D, Raj GV, Panageas KS. How to build and interpret a nomogram for cancer prognosis. J Clin Oncol (2008) 26:1364–70. doi: 10.1200/JCO.2007.12.9791
27. Shu ZY, Cui SJ, Zhang YQ, Xu YY, Hung SC, Fu LP, et al. Predicting chronic myocardial ischemia using ccta-based radiomics machine learning nomogram. J Nucl Cardiol (2022) 29:262–74. doi: 10.1007/s12350-020-02204-2
28. Zou W, Xu NL. Development and internal validation of a nomogram used to predict chemotherapy-induced neutropenia in non-small cell lung cancer patients: A retrospective cohort study. Cancer Manag Res (2021) 13:2797–804. doi: 10.2147/CMAR.S302722
29. Steyerberg EW, Vergouwe Y. Towards better clinical prediction models: Seven steps for development and an abcd for validation. Eur Heart J (2014) 35:1925–31. doi: 10.1093/eurheartj/ehu207
30. Carter JV, Pan J, Rai SN, Galandiuk S. Roc-ing along: Evaluation and interpretation of receiver operating characteristic curves. Surgery (2016) 159:1638–45. doi: 10.1016/j.surg.2015.12.029
31. Vickers AJ, Holland F. Decision curve analysis to evaluate the clinical benefit of prediction models. Spine J (2021) 21:1643–8. doi: 10.1016/j.spinee.2021.02.024
32. Ishikawa S, Yamamori I, Takamori S, Kitabatake K, Edamatsu K, Sugano A, et al. Evaluation of effects of perioperative oral care intervention on hospitalization stay and postoperative infection in patients undergoing lung cancer intervention. Support Care Cancer. (2021) 29:135–43. doi: 10.1007/s00520-020-05450-9
33. Shiono S, Yoshida J, Nishimura M, Hagiwara M, Hishida T, Nitadori J, et al. Risk factors of postoperative respiratory infections in lung cancer surgery. J Thorac Oncol (2007) 2:34–8. doi: 10.1097/JTO.0b013e31802bafb6
34. Soutome S, Yanamoto S, Funahara M, Hasegawa T, Komori T, Oho T, et al. Preventive effect on post-operative pneumonia of oral health care among patients who undergo esophageal resection: A multi-center retrospective study. Surg Infect (Larchmt). (2016) 17:479–84. doi: 10.1089/sur.2015.158
35. Lopez-de-Andres A, Perez-Farinos N, de Miguel-Diez J, Hernandez-Barrera V, Jimenez-Trujillo I, Mendez-Bailon M, et al. Type 2 diabetes and postoperative pneumonia: an observational, population-based study using the spanish hospital discharge database, 2001-2015. PloS One (2019) 14:e211230. doi: 10.1371/journal.pone.0211230
36. Ma CM, Liu Q, Li ML, Ji MJ, Zhang JD, Zhang BH, et al. The effects of type 2 diabetes and postoperative pneumonia on the mortality in inpatients with surgery. Diabetes Metab Syndr Obes (2019) 12:2507–13. doi: 10.2147/DMSO.S232039
37. Lim CH, Han JY, Cha SH, Kim YH, Yoo KY, Kim HJ. Effects of high versus low inspiratory oxygen fraction on postoperative clinical outcomes in patients undergoing surgery under general anesthesia: A systematic review and meta-analysis of randomized controlled trials. J Clin Anesth (2021) 75:110461. doi: 10.1016/j.jclinane.2021.110461
Keywords: postoperative pneumonia, nomogram, lung cancer, risk factors, thoracic surgery
Citation: Jin F, Liu W, Qiao X, Shi J, Xin R and Jia H-Q (2023) Nomogram prediction model of postoperative pneumonia in patients with lung cancer: A retrospective cohort study. Front. Oncol. 13:1114302. doi: 10.3389/fonc.2023.1114302
Received: 02 December 2022; Accepted: 06 February 2023;
Published: 23 February 2023.
Edited by:
Mohamed Rahouma, Weill Cornell Medical Center, NewYork-Presbyterian, United StatesReviewed by:
Martin Langer, Emergency NGO Italia, ItalyFederico Tacconi, University of Rome Tor Vergata, Italy
Copyright © 2023 Jin, Liu, Qiao, Shi, Xin and Jia. This is an open-access article distributed under the terms of the Creative Commons Attribution License (CC BY). The use, distribution or reproduction in other forums is permitted, provided the original author(s) and the copyright owner(s) are credited and that the original publication in this journal is cited, in accordance with accepted academic practice. No use, distribution or reproduction is permitted which does not comply with these terms.
*Correspondence: Hui-Qun Jia, bWF6dWl4dWVrZUBzaW5hLmNvbQ==