- 1Division of Epidemiology & Clinical Research, Department of Pediatrics, University of Minnesota, Minneapolis, MN, United States
- 2Bioinformatics and Computational Biology, University of Minnesota, Minneapolis, MN, United States
- 3Macalester College, St. Paul, MN, United States
- 4Masonic Cancer Center, University of Minnesota, Minneapolis, MN, United States
- 5Pediatric Hematology and Oncology, Department of Pediatrics, University of Minnesota, Minneapolis, MN, United States
- 6Brain Tumor Program, University of Minnesota, Minneapolis, MN, United States
- 7Department of Pediatrics, Division of Hematology-Oncology, Baylor College of Medicine, Houston, TX, United States
- 8Dan L. Duncan Comprehensive Cancer Center, Baylor College of Medicine, Houston, TX, United States
- 9Cancer and Hematology Center, Texas Children’s Hospital, Houston, TX, United States
- 10Department of Pediatrics, University of Minnesota, Minneapolis, MN, United States
- 11Department of Genetics, Cell Biology and Development, University of Minnesota School of Medicine, Minneapolis, MN, United States
- 12Center for Genome Engineering, University of Minnesota, Minneapolis, MN, United States
Background: Medulloblastoma, the most common malignant pediatric brain tumor, displays marked sex differences in prevalence of the four main molecular subgroups: SHH, WNT, Group 3 and Group 4. Males are more frequently diagnosed with SHH, Group 3 and 4 tumors, which have worse prognoses than WNT tumors. Little is known about sex differences in methylation profiles within subgroups.
Methods: Using publicly available methylation data (Illumina HumanMethylation450K array), we compared beta values for males versus females. Differentially methylated positions (DMP) by sex within medulloblastoma subgroups were identified on the autosomes. DMPs were mapped to genes and Reactome pathway analysis was run by subgroup. Kaplan-Meier survival curves (Log-Rank p-values) were assessed for each sex within subgroup. MethylCIBERSORT was used to investigate the tumor microenvironment using deconvolution to estimate the abundances of immune cell types using DNA methylation data.
Results: There were statistically significant differences in sex by medulloblastoma subgroups (chi-squared p-value=0.00004): Group 3 (n=144; 65% male), Group 4 (n=326; 67% male), SHH (n=223; 57% male) and WNT (n=70; 41% male). Females had worse survival than males for SHH (p-value=0.02). DMPs by sex were identified within subgroups: SHH (n=131), Group 4 (n=29), Group 3 (n=19), and WNT (n=16) and validated in an independent dataset. Unsupervised hierarchical clustering showed that sex-DMPs in SHH did not correlate with other tumor attributes. Ten genes with sex DMPs (RFTN1, C1orf103, FKBP1B, COL25A1, NPDC1, B3GNT1, FOXN3, RNASEH2C, TLE1, and PHF17) were shared across subgroups. Significant pathways (p<0.05) associated with DMPs were identified for SHH (n=22) and Group 4 (n=4) and included signaling pathways for RET proto-oncogene, advanced glycosylation end product receptor, regulation of KIT, neurotrophic receptors, NOTCH, and TGF-β. In SHH, we identified DMPs in four genes (CDK6, COL25A1, MMP16, PRIM2) that encode proteins which are the target of therapies in clinical trials for other cancers. There were few sex differences in immune cell composition within tumor subgroups.
Conclusion: There are sexually dimorphic methylation profiles for SHH medulloblastoma where survival differences were observed. Sex-specific therapies in medulloblastoma may impact outcomes.
1 Introduction
Medulloblastoma is the most common malignant pediatric brain tumor (1) affecting 400 United States (US) children each year (2). Medulloblastoma is comprised of four main molecular subgroups (3) that are prognostic with sonic hedgehog (SHH), Group 3 and Group 4 tumors associated with worse prognoses than wingless (WNT) tumors (4). We have shown there to be a male predominance in medulloblastoma incidence and risk in the US and around the globe in population-based studies (5–7). Unfortunately, with the recent use of these subgroups for prognosis they are almost entirely lacking in population-based and registry studies. As such, we must rely on clinical studies to understand sex differences in outcomes. From clinical studies, there are documented differences in the distribution of medulloblastoma subgroups by sex, with males more frequently diagnosed with the high-risk Groups 3 and 4 subgroups (8). While there are differences in survival between subgroups, little is known about sex differences in survival within subgroups. Further, there is no work examining sex differences in the genomic landscape of medulloblastoma, which may have significant implications for treatment and outcomes.
Sex differences in brain tumor development and progression are multifactorial and depend on the sex chromosome complement (9), immune regulation (10), and intrinsic differences in methylation that begin at conception through the life course (11). Based on the male excess in brain tumor diagnoses at all ages, it is unlikely that sex hormones are a main driving force mechanistically (12). Sex differences in epigenetics as measured by methylation have been documented throughout various organ systems in the body including the brain (13). DNA hypomethylation is often associated with carcinogenesis. Therefore, sex differences in methylation patterns could impact medulloblastoma formation and growth (13). Further, as methylation plays an integral role in brain tumor development and progression, it is important to identify sex differences in methylation profiles, which may help us understand etiology of the disease and identify potential therapeutic targets. Therefore, using publicly available medulloblastoma methylation data (14, 15), we aimed to identify sex differences in methylation profiles within subgroups.
2 Materials and methods
2.1 Data source
Using publicly available DNA methylation data collected by Cavalli et al. (2017) (14), 763 primary medulloblastoma samples were considered in our main analysis. Briefly, patient samples were collected from a number of treatment institutions including The Hospital for Sick Children, Children’s Hospital of Philadelphia, the Mayo Clinic and others (14). Patient samples were only included in the initial study if their medulloblastoma diagnosis required surgical resection. Flash frozen tissues were obtained, DNA from these samples was extracted, and methylation values were determined using Illumina Infinium HumanMethylation450 BeadChips.
2.2 Survival analyses
Chi-squared tests were performed to identify sex differences in the distribution of medulloblastoma subgroups. Fisher’s Exact tests (p<0.05) were performed to test for subgroup-specific sex differences in the distribution of selected covariates including age at diagnosis (years; 0-<5, 5-<10, 10-<15, 15-<20, ≥20), histology (classic, desmoplastic, large-cell anaplastic, medulloblastoma with extensive nodularity), metastasis (yes, no), and vital status (dead, alive). Kaplan-Meier survival curves were constructed and Log-Rank p-values were utilized to compare 12.5-year overall survival differences between sexes. Figures were created using survminor (R v4.0.2). Cox proportional hazards models were used to estimate hazard ratios (HR) and 95% confidence intervals (95% CI) as the measure of association between sex and death within each subgroup adjusting for covariates listed above (SAS v9.4). No violation of the proportional hazard’s assumption was detected as determined by entering a sex and time interaction term in the model.
2.3 Quality control and sex prediction
The R minfi package (Bioconductor v.3.12) (16) was used to convert the original methylation array experiment to an RGChannel Set object and perform quality control. The RGChannel Set contains the raw intensities of each probe. To detect and remove probes with unreliable signals, a function producing a detection p-value for each probe in every sample was run. Probes that returned p-values above 0.01 were removed from the analysis (n=44,069). The RGChannel Set was processed before differential methylation analysis by undergoing functional normalization, thus transforming it into a Genomic Ratio Set. Functional normalization was used as it is commonly applied to datasets with different tissues and cell types. Probes containing single nucleotide polymorphisms (SNPs) at either the CpG interrogation and/or at the single nucleotide extension were removed (n=60,291). Similarly, we removed non-specific, cross-reactive probes previously found to hybridize to autosomal and sex chromosomes by Chen et al. (2013) (n=106,931) (17).
Sex of each patient’s tissue was predicted by observing the median intensity of the X and Y chromosome probes (getSex() minfi). Any samples with discordant sex from the clinical information and predicted sex from the methylation data were dropped from the analysis (n=55). The sex chromosomes were excluded from all differentially methylated position analyses.
2.4 Differential methylation and mapping of differentially methylated positions to genes
After removal of the aforementioned probes, beta values, a standard estimate of the percentage methylation as a ratio of methylation probe fluorescent signal intensity to total probe signal (Beta = Meth/(Meth + Unmeth + offset) of the remaining probes were retrieved using the minfi function getBeta on the functionally normalized Genomic Ratio Set. The bumphunter package was used to run a multivariate model to examine differentially methylated regions, or “bumps”, by sex and subgroup, including adjustment for age at diagnosis (years; 0-<5, 5-<10, 10-<15, 15-<20, ≥20). A cut-off value of 0.05 was used in the model and 500 permutations were run. Differentially methylated positions (DMPs) between sex (male-female) were identified using the lmFit function in custom R code, with adjustment for age at diagnosis as in the DMR analysis. Differential methylation analysis by sex was done within each medulloblastoma subgroup (SHH, Group 3, Group 4 and WNT) and subsequently within each SHH subtype (SHH_alpha, SHH_beta, SHH_gamma, SHH_delta) as defined in Cavalli et al. (2017) (14). Significant DMP’s (Benjamini Hochberg adjusted p-value <0.05) within each subgroup were mapped to their nearest genes using the gene annotations from the 450k probe annotation information for genome hg19 (Supplemental Tables 1–5). Samples missing age data were excluded from this analysis (n=34). Additional healthy adult cerebellum samples detailed in the CNV analysis below were processed in the same manner to identify sex-DMPs in non-diseased tissues. Heatmaps were created using ComplexHeatmap and the Venn diagram of overlapping genes in subgroups was created using Venny (18).
2.5 Reactome pathway analysis
Reactome pathway analysis was used to identify biologic pathways over-represented by genes that had significant DMPs by sex for each medulloblastoma subgroup (p-value <0.05; date accessed: 10/15/2022). Pathways composed of ≥2 genes that had a significant p-value were included herein. IPA BioProfiler was used to identify chemotherapies available in clinical trials for other human cancers for the genes that contained DMPs by sex (date accessed: 2/24/2021).
2.6 Immune cell profiling based on methylation values
The MethylCIBERSORT R package (version 0.2.0) (19) and CIBERSORTx (https://cibersortx.stanford.edu/) (20) were used to investigate the tumor microenvironment using deconvolution to estimate the abundances of immune cell types using DNA methylation data. The Stromal_v2 reference from MethylCIBERSORT was used as the methylation signature matrix file. Input matrices of beta values for the reference probes in the signature matrix were created as percentages and uploaded to CIBERSORTx with the signature matrix. CIBERSORTx was run in absolute mode using 1,000 permutations without quantile normalization.
2.7 Copy number variation analysis
CNV analysis was performed using the conumee R package on raw methylation data (IDAT files) from the Illumina 450k methylation arrays to confirm that differentially methylated regions or positions were not copy number driven. [http://bioconductor.org/packages/conumee/] Conumee requires control data for analysis. Therefore, we downloaded a publicly available dataset that measured DNA methylation using Illumina’s 450K array in non-demented control brain tissue of the cerebellum (n=179, https://www.ncbi.nlm.nih.gov/geo/query/acc.cgi?acc=GSE134379). Conumee normalizes the combined intensity values of the methylated and unmethylated probes of each CpG site using these controls (representing genomes with no copy number alterations). Surrounding probes are then combined to create bins of a minimum size and probe number (default values and conumee exclude_regions data were used) prior to segmentation into clusters of the same state of variation in the number of copies via the circular binary segmentation algorithm. Segment tables were created for all samples and segment means for male versus female samples within subgroups at each DMP/gene/chromosome region of interest were calculated using pairwise Wilcoxon Rank Sum test.
2.8 Validation analysis
DMP analysis was validated in an independent cohort using publicly available DNA methylation data collected by Schwalbe et al. (2017) (15). This set consisted of 428 clinically annotated primary medulloblastoma samples. These tumor samples were part of a UK Children’s Cancer and Leukaemia Group (CCLG) study with approval from Newcastle North Tyneside Research Ethics committee (heretofore referred to as the Newcastle cohort). The Newcastle patient samples were also tested using Illumina HumanMethylation 450 BeadChips. Quality control and sex prediction were performed as described above. Samples were removed that had lower median intensities and did not cluster using minfi plotQC (badSampleCutoff=10.5, n=21). Additionally, 36 samples were removed as they were discordant between reported and predicted sex. As subgroup classification data was not available for the Newcastle cohort, the R package MethPed was used to perform classification (R package version 1.24.0.) (21). Ten additional samples were removed based on classification to categories outside of medulloblastoma by this algorithm, leaving a total of 361 samples used in the DMP validation analysis (Table 1A). Survival data, metastasis, and vital status were unavailable for the Newcastle cohort.
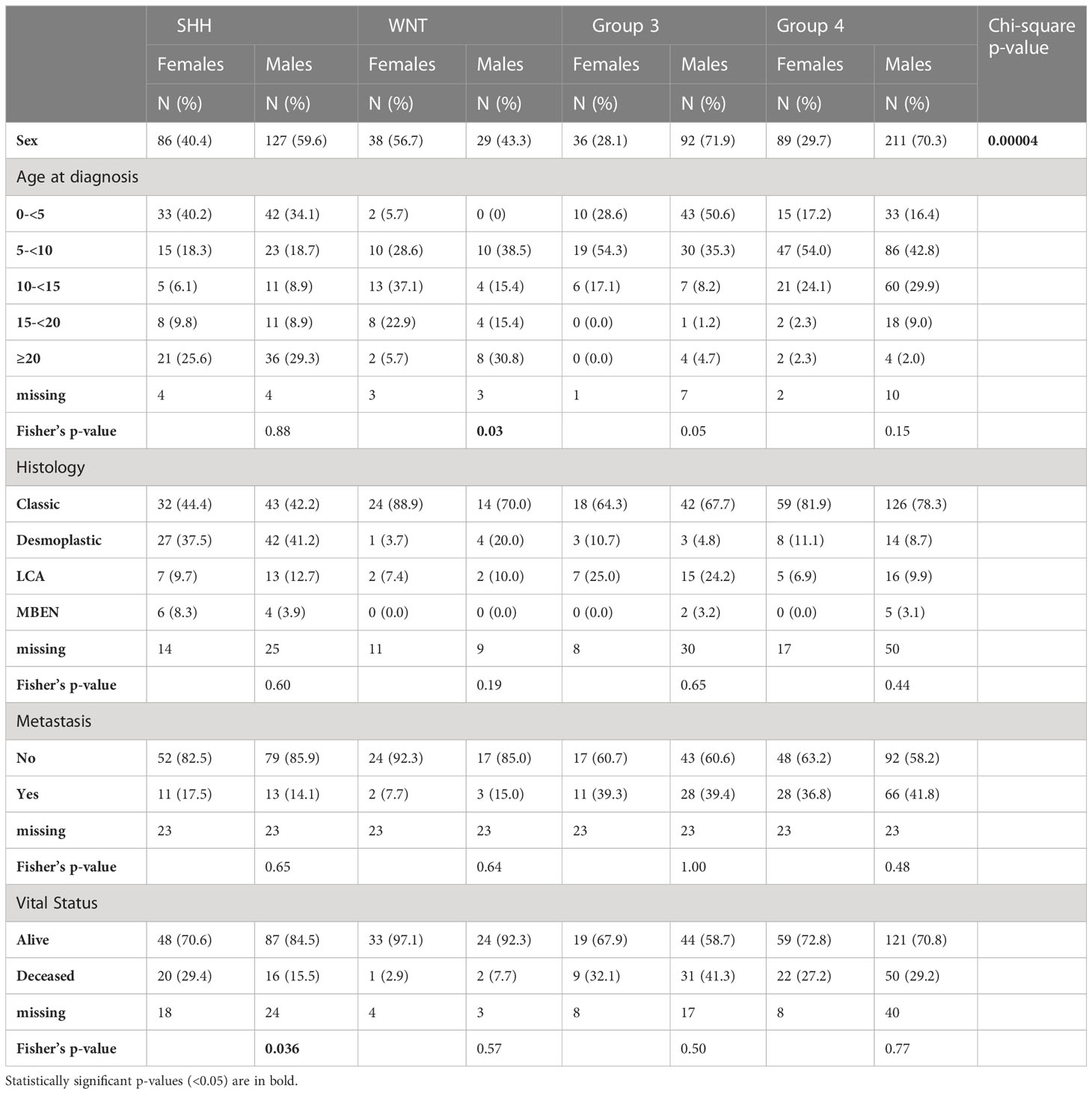
Table 1A Cavalli case demographics and clinical characteristics stratified by sex and medulloblastoma subgroup.
3 Results
There were 708 cases included in this analysis: SHH (N=213, 59.6% male), WNT (N= 67, 43.3% male), Group 3 (N= 128, 71.9% male), and Group 4 (N=300, 70.3% male) (Table 1B). We observed significant 12.5-year overall survival differences by sex in the SHH subgroup (Figure 1A) non-significant differences were observed in other subgroups, (Figures 1B–D) such that females had lower survival than males (Log-Rank p=0.016). Using Cox proportional hazards models adjusted for age at diagnosis, histology, and metastasis, SHH females had nearly three times the risk of death compared to males (hazard ratio: 2.89, 95% CI: 1.29-6.24). There were no significant differences in survival between males and females in the other three subgroups.
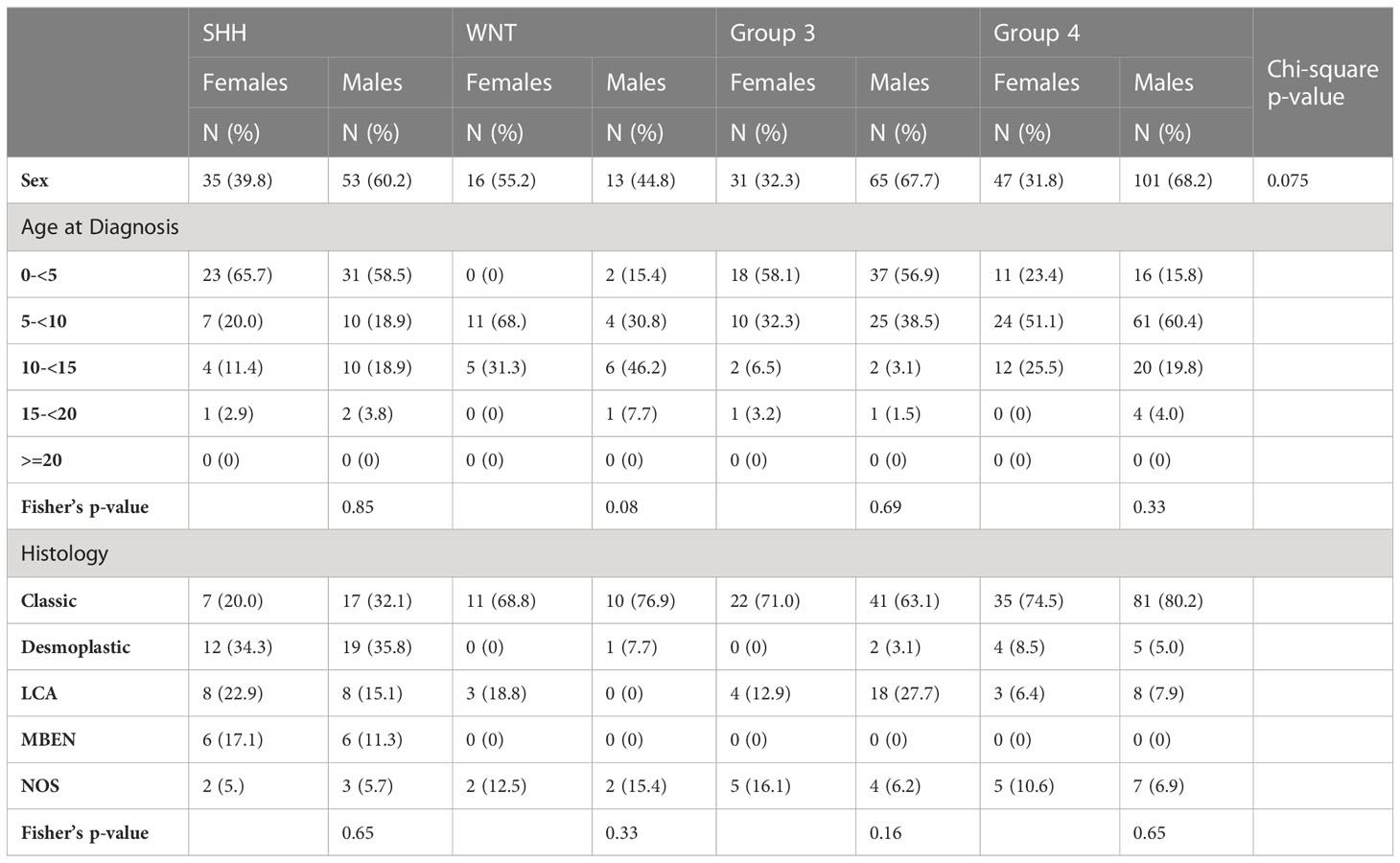
Table 1B Newcastle case demographics and clinical characteristics stratified by sex and medulloblastoma subgroup.
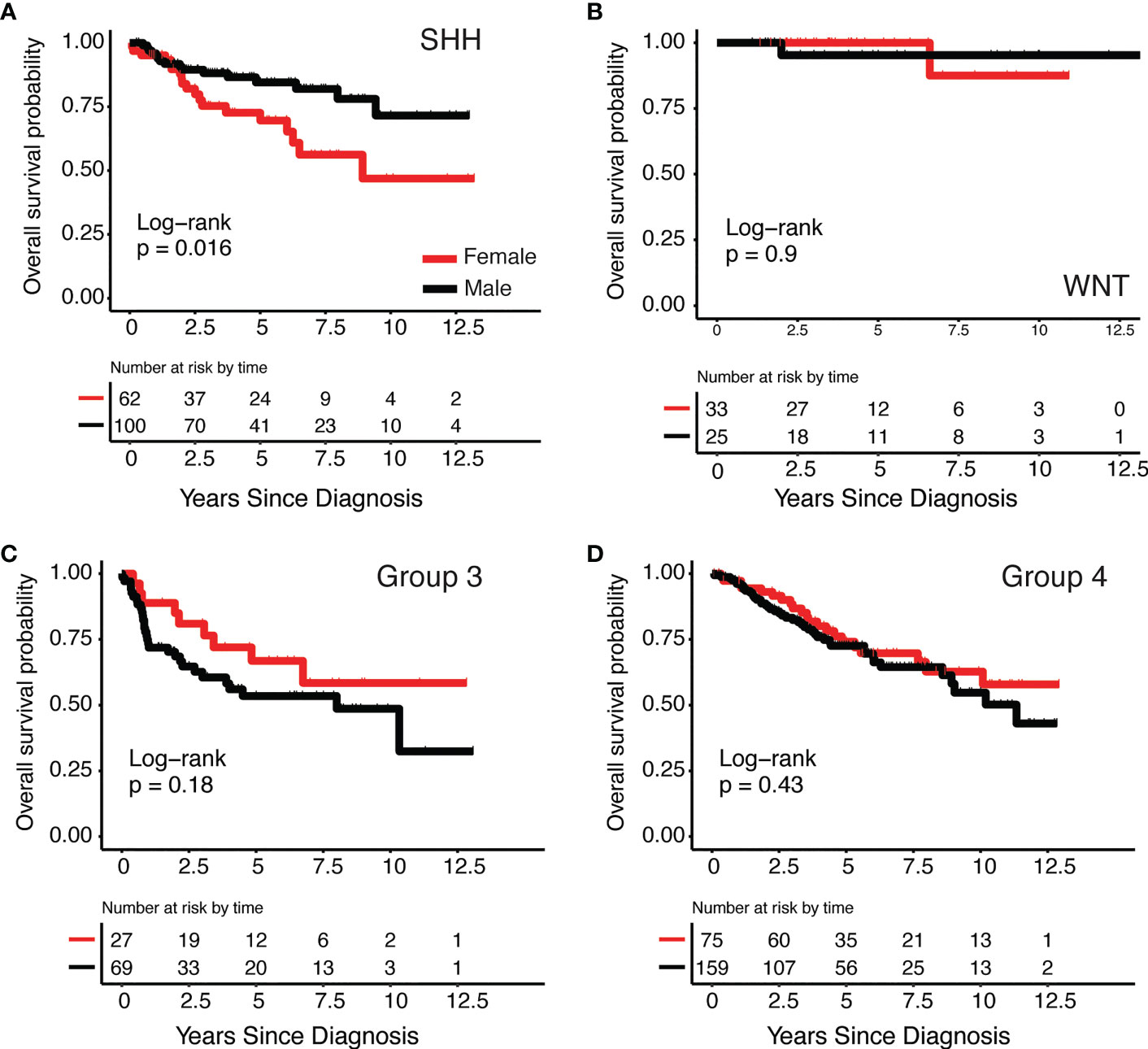
Figure 1 Kaplan-Meier survival curves by medulloblastoma subgroup and sex (A) SHH, (B) WNT, (C) Group 3 and (D) Group 4.
We used the bumphunter package to run a multivariate model to examine sex differences globally by sex and subgroup and found 12 statistically significant DMPs (Supplemental Table 1). After finding this small number of DMPs globally, we then investigated subgroup-specific differentially methylated positions by analysis within each subgroup of tumor as medulloblastoma subgroups are molecularly and prognostically distinct (4). We observed statistically significant sex differences in DNA methylation within each subgroup (Supplemental Tables 2–5). SHH had the highest number of DMPs by sex (n=131), followed by Group 4 (n=29), Group 3 (n=19), and WNT (n=16). Ten genes had statistically significant DMPs by sex in all subgroups: RFTN1, C1orf103, FKBP1B, COL25A1, NPDC1, B3GNT1, FOXN3, RNASEH2C, TLE1, and PHF17. We performed unsupervised hierarchical clustering using significant DMPs by sex within each subgroup (Figures 2A–D). Clustering of samples using these DMPs was independent of other clinically relevant characteristics such as histology and vital status. The SHH group showed the strongest clustering by sex-DMPs (Figure 2A).
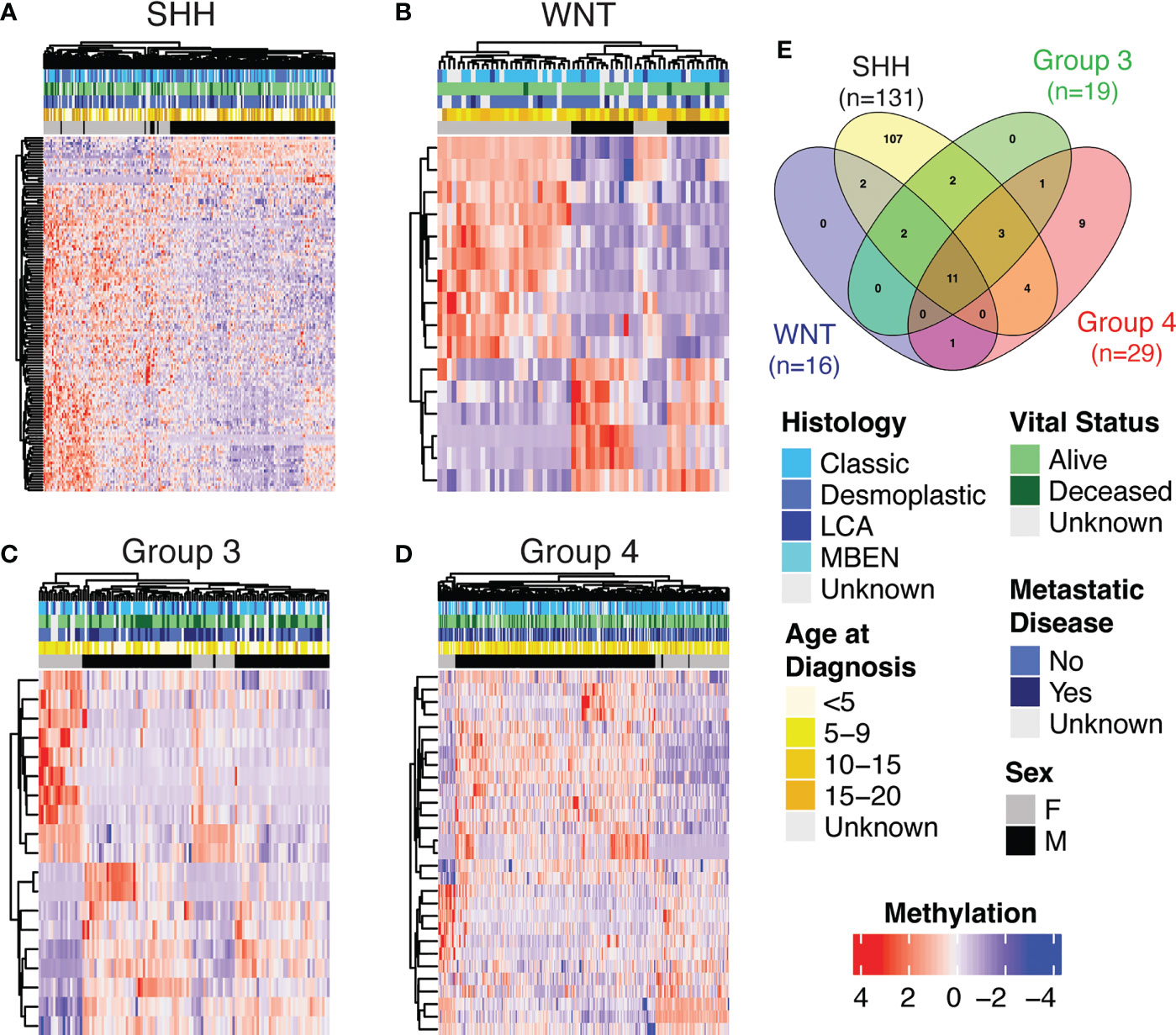
Figure 2 Heatmap showing methylation levels (row-scaled β-values) of statistically significantly differentially methylated positions (DMPs) by sex (adjusted p<0.05) from the autosomes in Cavalli cohort within subgroup (A) SHH, (B) WNT, (C) Group 3 and (D) Group 4. (E) The number of genes that contained a DMP by sex for each subgroup and the overlap of those gene sets.
CNV analysis was performed to confirm that differentially methylated regions or positions were not copy number driven (Supplemental Tables 2–5). Less than ten percent of sex-DMPs in each subgroup were statistically significantly different when we intersected CNV segments with DMP locations and compared mean values for males versus females (SHH=9.9%, Group 4 = 3.5%, Group3 = 0%, WNT=0%). The same approach confirms that in the SHH subgroup samples there is no statistically different level of amplification at GLI2, MYCN, and TP53 or 14q and 17p chromosome arm loss between sexes (results not shown).
To validate our sex-DMPs, we performed subgroup analysis on the validation set arising from the Newcastle cases, which resulted in a smaller, but consistent group of statistically significant sex-DMPs in each subgroup. Again, SHH had the highest number of DMPs by sex (n=11), followed by Group 4 (n=5), Group 3 (n=4), and WNT (n=2) (Supplemental Tables 6–9). All but one of these subgroup sex-DMPs, the gene LTK in the WNT subgroup, were already found in the corresponding Cavalli subgroup analysis. One gene, RFTN1, was a statistically significant DMP by sex in all subgroups. Unsupervised hierarchical clustering of subgroup samples using significant sex-DMPs demonstrates that the clustering is independent of other clinically relevant characteristics, specifically age at diagnosis and histology, and the SHH group shows the strongest clustering by sex (Supplementary Figure 1).
Statistically significant pathways resulting from the Cavalli sex-DMPs for each subgroup are presented in Table 2A. To explore tumor specific pathways, we removed genes associated with sex-DMPs that overlap with those found in the control cerebellum brain samples (Supplementary Table 10) and repeated the pathway analysis (Table 2B). No pathways were identified in WNT or Group 3 in either analysis. The top two pathways in Group 4 (both overall and tumor only sex-DMPs) were activation of HOX genes during differentiation and anterior HOX genes in hindbrain development during early embryogenesis. Both are also found in the top pathways of SHH using all sex-DMPs. Tumor specific sex-DMP pathways in SHH included G alpha (s) signaling events, diseases associated with N-glycosylation of proteins, telomere C-strand (lagging strand) synthesis, and interleukin-1 signaling. The top four pathways in this SHH tumor-specific pathway analysis overlaped with the overall sex-DMP pathway analysis. The top pathway in the non-tumor-specific sex-DMPs of SHH is YAP1- and WWTR1 (TAZ)-stimulated gene expression. Other top pathways identified in SHH include signaling and loss of function of TGF-β receptor in cancer, SOS-mediated signaling, signal attenuation, signaling in RET, and advanced glycosylation end product receptor. Using the IPA BioProfiler, we identified four genes that encode proteins that are the target of therapies approved or in clinical trials for other human cancers that contained sex-DMPs including CDK6, COL25A1, MMP16, PRIM2 in SHH and COL25A1 in WNT and Group 4.
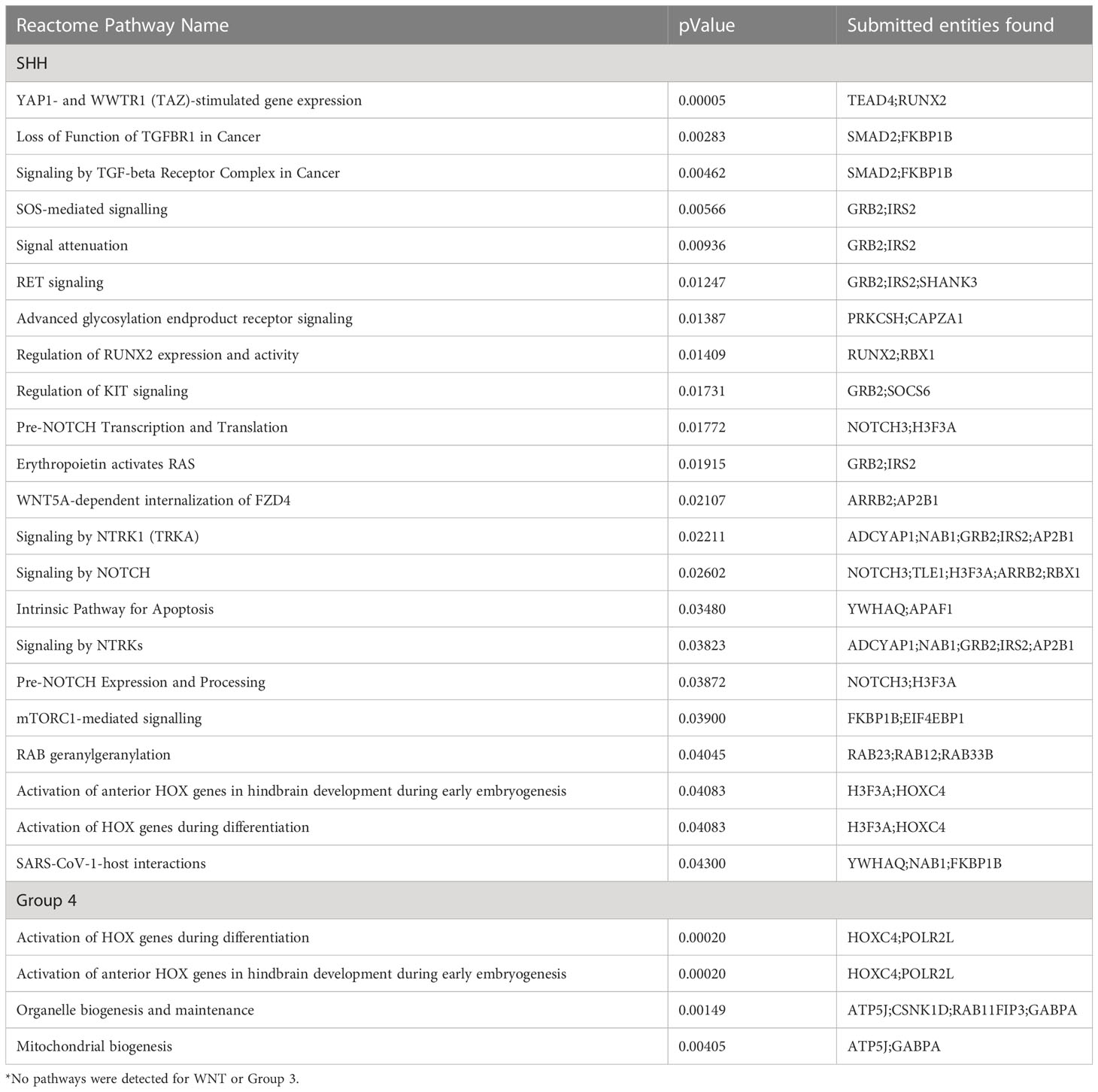
Table 2A Reactome pathway analysis for enriched pathways (p<0.05) comprised of at least two genes with significant differences in methylation by sex for each medulloblastoma subgroup*.
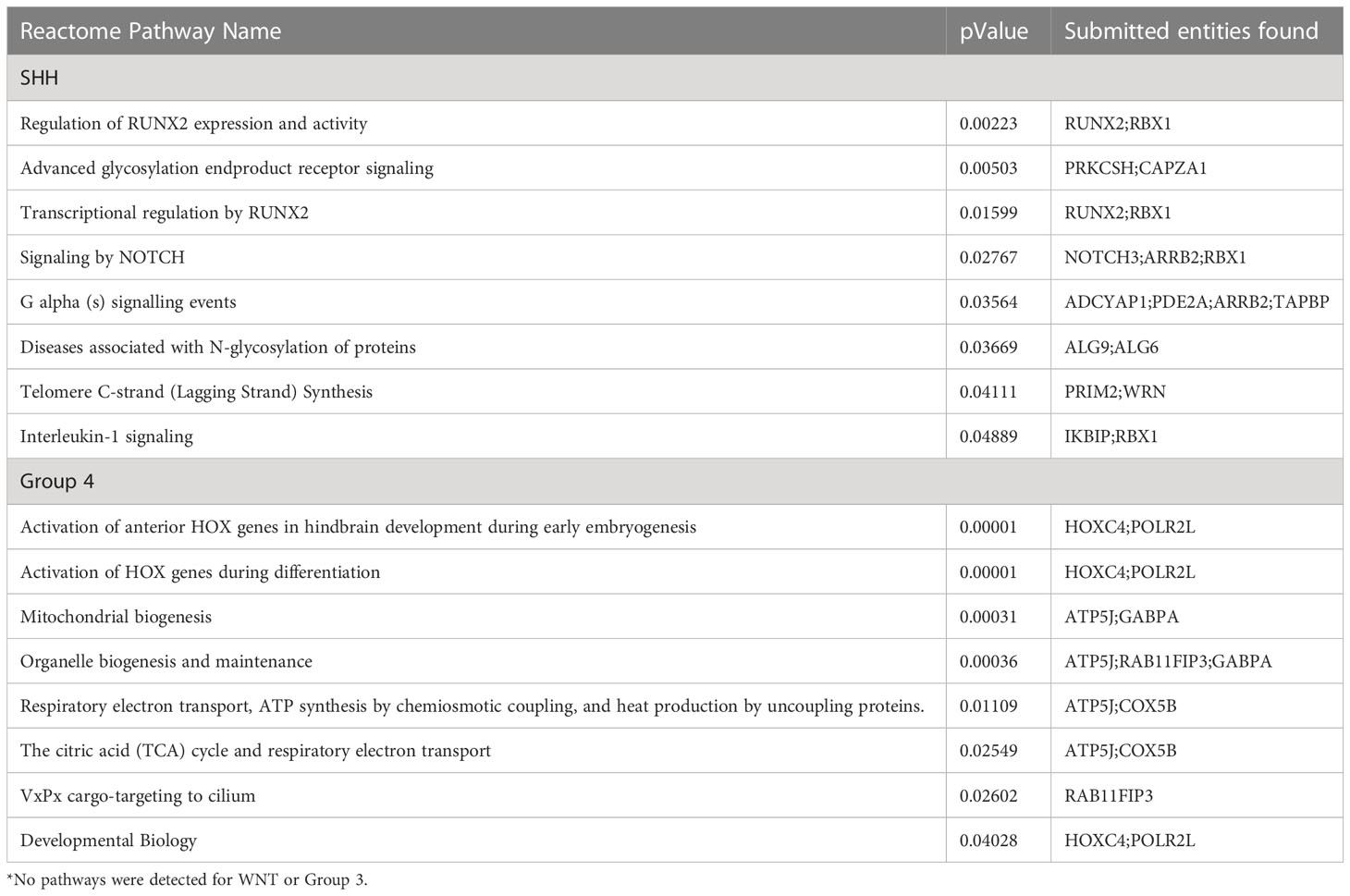
Table 2B Reactome pathway analysis for enriched pathways (p<0.05) comprised of at least two genes with significant differences in methylation by sex for each medulloblastoma subgroup (without overlap with non-tumor cerebellum sex-DMPs) *.
As the SHH subgroup had significant overall survival differences by sex, we explored sex-DMPs in the four clinically and cytogenetically distinct SHH subtype groups: SHH alpha (N = 59, 61.0% male), SHH beta (N = 32, 46.9% male), SHH gamma (N = 45, 55.6% male), and SHH delta (N = 69, 68.1% male). Although smaller sample sizes in these subtypes limited our ability to find methylation differences due to sex, we observed that SHH delta subtype had the largest number of sex-DMPs within SHH delta subtypes (n=38). SHH delta likely drove many of the differences by sex found in SHH overall (Supplementary Tables 11–14; Supplementary Figure 2).
Due to the complex nature of the tumor microenvironment and role of the immune system in tumor development, medulloblastoma subgroup and sex differences in tumor immune cell composition were assessed using MethylCIBERSORT. Comparisons of medulloblastoma subgroups overall for each immune cell type assessed in the MethylCIBERSORT deconvolution pipeline show statistically significant differences in distribution between at least two subgroups in each cell type (all p<0.0001, Figure 3A) though the absolute scores of these cell types were low overall. Within subgroup comparisons of males versus females cell type composition showed statistically significant differences in regulatory T cells in Group 3 (Figure 3B). No other subgroups displayed statistically significant sex differences in immune cell types identified in MethylCIBERSORT (Figure 3B).
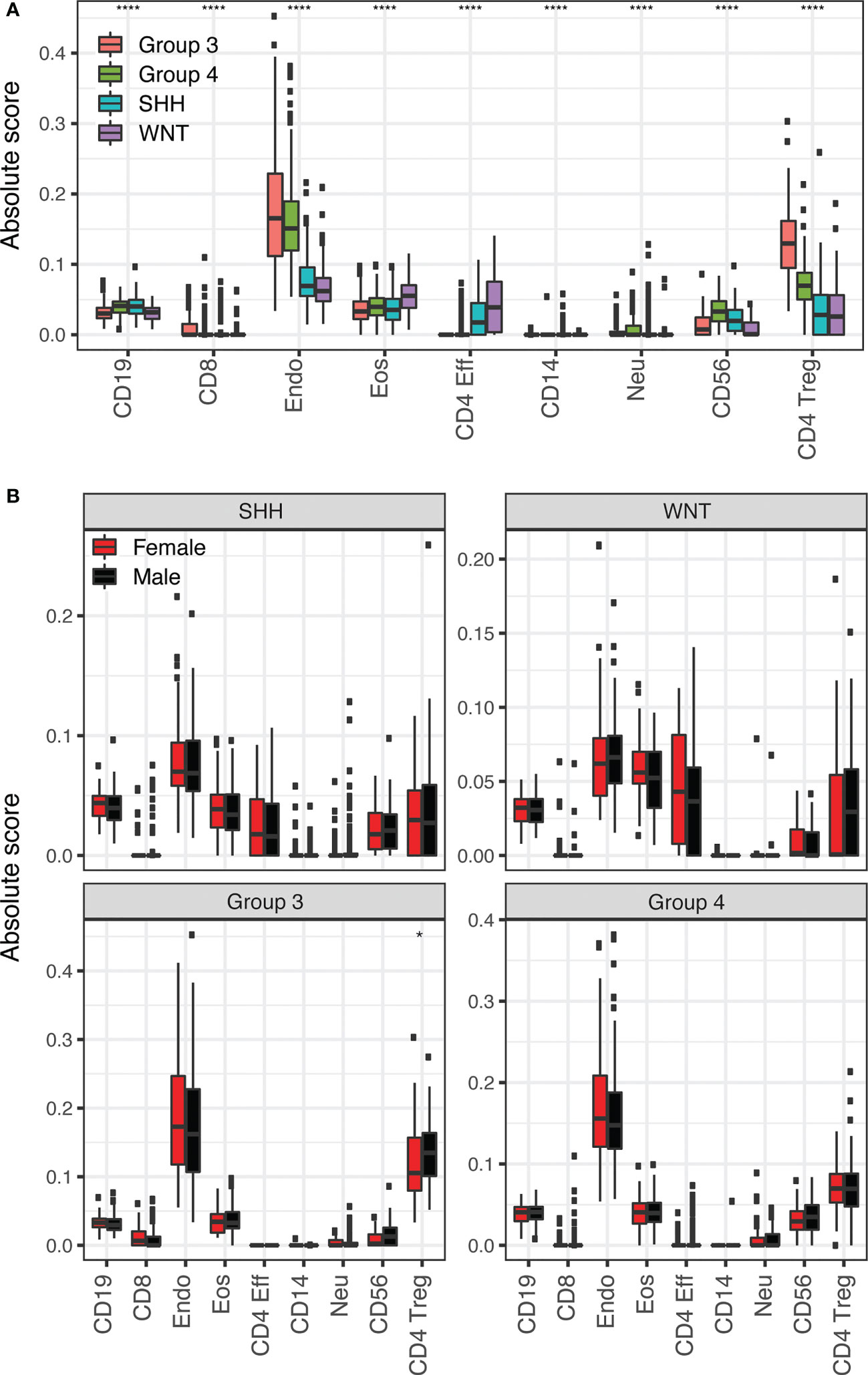
Figure 3 (A) Application of the MethylCIBERSORT stromal signature matrix to deconvolution of 708 medulloblastoma 450K methylation arrays using CIBERSORT. Boxplots compare medulloblastoma subgroup means for each cell type. Significance is calculated using pairwise Wilcoxon Rank Sum test with p-values (*p <= 0.05; ****: p <= 0.0001). The symbol is shown for the highest significance between two different subgroups for each cell type. (B) Boxplots comparing female (red) and male (black) means for each cell type in each medulloblastoma subgroup as labeled.
4 Discussion
From 708 primary medulloblastoma samples, we identified statistically significant sex differences in survival in the SHH subgroup, with females demonstrating worse long-term survival than males. There were no survival differences between sexes in the Group 3, Group 4, or WNT subgroups. We identified sex differences in methylation within the four subgroups and SHH had the highest number of sex-DMPs (n=131), followed by Group 4 (n=29), Group 3 (n=19), and WNT (n=16). In our validation cohort, sex-DMPs were identified in smaller numbers, but were largely the same as those seen in the Cavalli data further strengthening the evidence of sex differences in methylation in medulloblastoma. Unsupervised hierarchical clustering based on sex-DMPs did not appear to be driven by any other clinically-relevant factors. The strongest sex driven clustering was observed in the SHH subgroup, which comprise approximately 30% of medulloblastomas in general (1, 4). These findings suggest there are true sex differences in DMPs within each subgroup that are independent of important clinical factors.
After mapping the sex-DMPs to the nearest gene, there were ten genes shared between all four subgroups. Nine of these ten genes were also found as sex-DMPs in the healthy adult cerebellum tissue analysis, suggesting more global sex differences in brain methylation that may be not disease- or age- specific. The genes identified in all four subgroups were RFTN1, C1orf103, FKBP1B, COL25A1, NPDC1, B3GNT1, FOXN3, RNASEH2C, TLE1, and PHF17. Of these, RFTN1, COL25A1, TLE1, and RNASEH2C have been found to have sex differences in methylation in leukocytes (22), which are known to impact tumor maintenance (23) and be regulated by sex hormones (24, 25). Remaining genes from this list are involved in various neuron-related processes, such as FKBP1B and neuronal aging (26), NPDC1 and neuronal differentiation (27), and FOXN3 and neuronal activation (28). Sex differences in brain development have been reported extensively in the psychiatric literature such that males not only have larger brain volumes by approximately 10% (12, 29) they also have a larger volume of gray matter (30). Gray matter is composed of various neuronal cell types (30) and may contribute to the sex differences in brain tumor incidence we see in populations as well as the sex differences we observed herein. We did not find that sex-DMPs corresponded to numerous sex differences in CNVs again suggesting a true role for sex-DMPs in medulloblastoma. Collectively, these findings suggest that sexually dimorphic epigenetic regulation of these genes may underlie medulloblastoma etiology more broadly and may operate through leukocyte-mediated mechanisms and neuronal development.
In our analysis, we also identified biologic pathways from the lists of sex-DMPs that may be sexually dimorphic in medulloblastomas. The top pathway in SHH is YAP1- and WWTR1 (TAZ)-stimulated gene expression. YAP1 and WWTR1 are both transcriptional co-activators regulated via HIPPO signaling with transcriptional targets crucial to cell proliferation and apoptosis. HIPPO signaling has previously been associated with pediatric cancers, including a known interaction with Sonic Hedgehog that upregulates the nuclear localization of YAP (31). Several top pathways identified using only tumor specific sex-DMPs in SHH were also found in the top SHH pathways using all sex-DMPs, including regulation of RUNX2 expression and activity. RUNX2 has been previously implicated in SHH tumorigenesis (32). Additional pathways from the tumor-specific sex-DMPs in SHH include G α (s) signaling events and diseases associated with N-glycosylation of proteins. The G α (s) signaling pathway can suppress SHH tumorigenesis through negative regulation of the Hippo pathway. The top two pathways in Group 4 are activation of HOX genes during differentiation and anterior HOX genes in hindbrain development during early embryogenesis, and both are also found in the top pathways of SHH. HOX genes are critical to embryonic development, with their expression accompanied by specific epigenetic states with noted changes to DNA methylation in other brain tumors (33). Other top pathways identified in SHH include signaling and loss of function of TGF-β receptor in cancer, SOS-mediated signaling, signal attenuation, and signaling in RET and advanced glycosylation end product receptor. TGF-β secretion has been documented in medulloblastomas and TGF-β pathway activity is potentially a predictor of survival in SHH-driven medulloblastomas (34). We then went on to identify four genes from our IPA BioProfiler analysis that had current targeted therapies approved for use in other cancers: CDK6, COL25A1, MMP16, PRIM2, which highlights the potential sex-specific utility of these genes and their encoded proteins as therapeutic targets for future study.
Population-based studies show incidence rates for medulloblastoma vary by sex both within the United States and around the world, with males more frequently diagnosed and male-to-female incidence rate ratios ranging between 1.4-2.2 (5, 6, 24); however, these studies often lack modern subgroup classifications. Based on findings from clinical-based studies, male-to-female ratios differ between molecular subgroups, with WNT and SHH groups showing approximately equal male and female distributions, but Group 3 and Group 4 medulloblastoma comprised with about twice as many males (11, 35). In our study, we observed an excess of males in SHH medulloblastoma rather than a 1:1 distribution by sex. Characterizing the sex ratio in population-based studies with genomic samples is critical.
Ten-year survival rates by sex in medulloblastoma are similar among all ages in studies, including our previous publication (24, 36), while five-year survival rates in children aged 0-19 indicate a lower risk of death in females than males (HR: 0.79) (37). Group 3 and 4 subgroup tumors are often found to have poorer survival than WNT tumors across the age-spectrum (35) yet we did not observe survival differences by sex in our study within these groups. Conversely, we observed that females in our study had worse survival than males for SHH tumors, which have an approximate 5-year survival of 50-75% depending on TP53 mutation status (1, 38). Unfortunately, we could not evaluate TP53 mutation status in our SHH tumors as we were unable to match samples to their mutation status based on the publicly available data (14), but there was no indication of sex differences in TP53 CNV in our analyses. However, we did observe that SHH females had a 5-year survival of approximately 60% in our study suggesting the association between TP53 mutation status and sex should be evaluated in future studies. Age-stratified analyses among SHH tumors where TP53 mutation is thought to occur more commonly in children (8) were non-informative in our analysis likely due to sample size limitations (results not shown). The male excess in brain tumor incidence is not confined to medulloblastoma. There is a male excess in gliomas (39) and significantly decreased overall survival in males for recurrent gliomas (40). Notably, Johansen et al. (2020) found genome-wide differences in DNA methylation by sex, with distinct patterns in glioma molecular subgroups as we have observed in medulloblastoma (41).
Sex differences in brain tumor genomics and epigenetics have been discussed extensively by Rubin and colleagues (11, 12). Sex can influence tumorigenesis through the sex chromosome complement, direct hormone action, and epigenetic disparities (11). Given that the molecular subgroups of medulloblastoma are suspected to arise from different cells of origin (1), it is reasonable to hypothesize that distinct mechanisms of carcinogenesis and/or progenitor cell types are more susceptible to the impact of sex. In SHH medulloblastoma, the cell of origin is hypothesized to be the cerebellar granule neuron precursor that may be particularly susceptible to TP53 mutagenesis (1, 42). In our study, we observed the highest number of sex-DMPs in SHH in which females had worse outcomes than males. Whether these sex differences in epigenetics of SHH are a coincidental product of the cell of origin or themselves drive outcomes remains to be investigated in other studies. During early development, sex hormones enact vast changes in epigenetics that determine sexual phenotypes (11). Using in vitro and in vivo models of medulloblastoma where sex of the host and tumor is known may help to further uncover sexually dimorphic biologic mechanisms of medulloblastoma development.
Though we have a large sample size and a validation cohort with which we conducted sex-stratified analyses within medulloblastoma subgroups, our study is not without limitations. While medulloblastoma is the most common malignant brain tumor in children less than 19 years of age (1), the parent study was not conducted exclusively in children and limiting it to children would have greatly diminished our sample size. It may be that pediatric and young adult medulloblastomas have different sex-specific methylation profiles and this should be investigated in appropriate studies in the future as there are endogenous changes that occur between childhood and adulthood that may impact tumor etiology. While the parent study had various clinical data, including survival, we are lacking risk factor data such as birth characteristics and other exposures such as radiation (43), which are hypothesized to impact medulloblastoma risk. We cannot rule out the possibility that the lower survival reported here in females versus males in SHH tumors may be due to subsequent cancers or long-term adverse events rather than tumor progression, as this data is also lacking. This study did contain gene expression data from microarrays, but there were few sex differences in gene expression (results not shown) identified in our initial analyses. RNA sequencing data with greater breadth and depth of gene coverage may allow for the identification of gene expression differences that could further help identify biologic mechanisms underlying sex differences in methylation and medulloblastoma tumorigenesis as we have reported previously in osteosarcoma (44). Additionally, single cell RNA sequencing and tumor microdissection might further highlight sex differences in medulloblastoma genomics as has been observed in adult glioblastomas where sex differences were found to be dependent on the sex chromosome composition of the tumor rather than the host (45). Sex differences in treatment received or response to therapy (46) may underlie the observed sex differences, particularly in SHH medulloblastoma, but this information was not available for evaluation herein.
To conclude, in our sex-stratified analysis of methylation differences within medulloblastoma subgroups, we identified sex-DMPs that varied by subgroup with SHH having the highest number of DMPs. Interestingly, in this study we only observed sex differences in survival in SHH medulloblastoma where females had worse long-term survival than males. We found 10 genes with DMPs that were conserved across subgroups suggesting a shared genetic background by subgroup may underlie some of the observed sexual dimorphism in medulloblastoma. Pathways identified within subgroups were largely signaling pathways including TGF-β, neurotrophic receptors, and NOTCH, which are known to impact prognosis in medulloblastoma and according to our findings may vary by sex within subgroups (34, 47, 48). Importantly, we identified four genes that housed sex-DMPs that also have chemotherapies available that could be studied in a sex-specific manner to improve outcomes for males and females with medulloblastoma.
Data availability statement
Publicly available datasets were analyzed in this study. This data can be found here: GEO Series GSE85218 - https://www.ncbi.nlm.nih.gov/geo/query/acc.cgi?acc=GSE85218 GEO Series GSE93646 - https://www.ncbi.nlm.nih.gov/geo/query/acc.cgi?acc=GSE93646 GEO Series GSE134379 - https://www.ncbi.nlm.nih.gov/geo/query/acc.cgi?acc=GSE134379.
Ethics statement
As this is publicly available data the study does not require consent for participation.
Author contributions
NS, RM: Data analysis, results interpretation, manuscript drafting and editing. LM: conceptualization of study, study design, data analysis, manuscript editing. LS, DL, CM, TH: conceptualization of study, manuscript drafting and editing. LW: study oversight, conceptualization of study, study design, data analysis, manuscript drafting and editing. All authors contributed to the article and approved the submitted version.
Funding
This work was supported by the American Cancer Society Research Professorship (DAL) and the Children’s Cancer Research Fund (LAW, NS). This research was supported by the National Institutes of Health’s National Center for Advancing Translational Sciences, grants TL1R002493 and UL1TR002494. The content is solely the responsibility of the authors and does not necessarily represent the official views of the National Institutes of Health’s National Center for Advancing Translational Sciences (RMM).
Conflict of interest
DAL is a co-founder and co-owner of NeoClone Biotechnologies, Inc., Discovery Genomics, Inc. recently acquired by Immunsoft, Inc., B-MoGen Biotechnologies, Inc. recently acquired by Bio-Techne corporation, and Luminary Therapeutics, Inc. DAL holds equity in, is a Board of Directors member, and serves as a senior scientific advisor of Recombinetics, a genome-editing company. DAL holds equity in and consults for Styx Biotechnologies Inc. as a member of their scientific advisory board. DAL consults for Genentech, Inc., which is funding some of his research. The business of all these companies is unrelated to the contents of this manuscript.
The remaining authors declare that the research was conducted in the absence of any commercial or financial relationships that could be construed as a potential conflict of interest.
The handling editor SR declared a shared affiliation with the author TH at the time of review.
Publisher’s note
All claims expressed in this article are solely those of the authors and do not necessarily represent those of their affiliated organizations, or those of the publisher, the editors and the reviewers. Any product that may be evaluated in this article, or claim that may be made by its manufacturer, is not guaranteed or endorsed by the publisher.
Supplementary material
The Supplementary Material for this article can be found online at: https://www.frontiersin.org/articles/10.3389/fonc.2023.1113121/full#supplementary-material
Supplementary Figure 1 | Heatmap showing methylation levels (row-scaled β-values) of statistically significantly differentially methylated positions (DMPs) by sex (adjusted p<0.05) from the autosomes in Newcastle cohort within subgroup (A) SHH, (B) WNT, (C) Group 3 and (D) Group 4. (E) The number of genes that contained a DMP by sex for each subgroup and the overlap of those gene sets.
Supplementary Figure 2 | The number of sex-DMPs for each SHH subtype and the overlap of those probes.
Abbreviations
US, United States; SHH, sonic hedgehog; WNT, wingless; HR, hazard ratios; 95% CI, 95% confidence intervals; SNPs, single nucleotide polymorphisms; DMPs, Differentially methylated positions; IPA, Ingenuity Pathway Analysis.
References
1. Ellison D, Eberhart C, Pietsch T, Pfister S. WHO classification of tumours of the central nervous system: Medulloblastoma. 4th Edition. Louis DN, Ohgaki H, Wiestler OD, Cavenee WK, editors. (Lyon, France: International Agency for Research on Cancer) (2016) p. 184–200.
2. United states cancer statistics: 1999 - 2018 incidence, WONDER online database (2020). United States Dep Heal Hum Serv Centers Dis Control Prev Natl Cancer Inst. Available at: https://wonder.cdc.gov/cancer.html (Accessed February 1, 2022).
3. Northcott PA, Buchhalter I, Morrissy AS, Hovestadt V, Weischenfeldt J, Ehrenberger T, et al. The whole-genome landscape of medulloblastoma subtypes. Nature (2017) 547:311–7. doi: 10.1038/nature22973
4. Taylor MD, Northcott PA, Korshunov A, Remke M, Cho YJ, Clifford SC, et al. Molecular subgroups of medulloblastoma: The current consensus. Acta Neuropathol (2012) 123:465–72. doi: 10.1007/s00401-011-0922-z
5. Williams LA, Richardson M, Marcotte EL, Poynter JN, Spector LG. Sex ratio among childhood cancers by single year of age. Pediatr Blood Cancer (2019) 66: e27620. doi: 10.1002/pbc.27620
6. Williams LA, Hubbard AK, Scheurer ME, Spector LG, Poynter JN. Trends in paediatric central nervous system tumour incidence by global region from 1988 to 2012. Int J Epidemiol (2021) 50:116–27. doi: 10.1093/ije/dyaa176
7. Williams LA, Richardson M, Kehm RD, McLaughlin CC, Mueller BA, Chow EJ, et al. The association between sex and most childhood cancers is not mediated by birthweight. Cancer Epidemiol (2018) 57:7–12. doi: 10.1016/j.canep.2018.09.002
8. Juraschka K, Taylor MD. Medulloblastoma in the age of molecular subgroups: A review: JNSPG 75th anniversary invited review article. J Neurosurg Pediatr (2019) 24:353–63. doi: 10.3171/2019.5.PEDS18381
9. Clocchiatti A, Cora E, Zhang Y, Dotto GP. Sexual dimorphism in cancer. Nat Rev Cancer (2016) 16:330–9. doi: 10.1038/nrc.2016.30
10. Klein SL, Flanagan KL. Sex differences in immune responses. Nat Rev Immunol (2016) 16:626–38. doi: 10.1038/nri.2016.90
11. Sun T, Plutynski A, Ward S, Rubin JB. An integrative view on sex differences in brain tumors. Cell Mol Life Sci (2015) 72:3323–42. doi: 10.1007/s00018-015-1930-2
12. Sun T, Warrington NM, Rubin JB. Why does jack, and not jill, break his crown? sex disparity in brain tumors. Biol Sex Differ (2012) 3:3. doi: 10.1186/2042-6410-3-3
13. Rubin JB, Lagas JS, Broestl L, Sponagel J, Rockwell N, Rhee G, et al. Sex differences in cancer mechanisms. Biol Sex Differ (2020) 11:17. doi: 10.1186/s13293-020-00291-x
14. Cavalli FMG, Remke M, Rampasek L, Peacock J, Shih DJH, Luu B, et al. Intertumoral heterogeneity within medulloblastoma subgroups. Cancer Cell (2017) 31:737–754.e6. doi: 10.1016/j.ccell.2017.05.005
15. Schwalbe EC, Lindsey JC, Nakjang S, Crosier S, Smith AJ, Hicks D, et al. Novel molecular subgroups for clinical classification and outcome prediction in childhood medulloblastoma: a cohort study. Lancet Oncol (2017) 18:958–71. doi: 10.1016/S1470-2045(17)30243-7
16. Aryee MJ, Jaffe AE, Corrada-Bravo H, Ladd-Acosta C, Feinberg AP, Hansen KD, et al. Minfi: A flexible and comprehensive bioconductor package for the analysis of infinium DNA methylation microarrays. Bioinformatics (2014) 30:1363–9. doi: 10.1093/bioinformatics/btu049
17. Chen YA, Lemire M, Choufani S, Butcher DT, Grafodatskaya D, Zanke BW, et al. Discovery of cross-reactive probes and polymorphic CpGs in the illumina infinium HumanMethylation450 microarray. Epigenetics (2013) 8:203–9. doi: 10.4161/epi.23470
18. Oliveros JC. An interactive tool for comparing lists with venn’s diagramsVenny (2007–2015). Available at: https://bioinfogp.cnb.csic.es/tools/venny/index.html.
19. Chakravarthy A, Furness A, Joshi K, Ghorani E, Ford K, Ward MJ, et al. Pan-cancer deconvolution of tumour composition using DNA methylation. Nat Commun (2018) 9:3220. doi: 10.1038/s41467-018-05570-1
20. Newman AM, Steen CB, Liu CL, Gentles AJ, Chaudhuri AA, Scherer F, et al. Determining cell type abundance and expression from bulk tissues with digital cytometry. Nat Biotechnol (2019) 37:773–82. doi: 10.1038/s41587-019-0114-2
21. Danielsson A, Nemes S, Tisell M, Lannering B, Nordborg C, Sabel M, et al. MethPed: a DNA methylation classifier tool for the identification of pediatric brain tumor subtypes. Clin Epigenet (2015) 7:1–9. doi: 10.1186/s13148-015-0103-3
22. Inoshita M, Numata S, Tajima A, Kinoshita M, Umehara H, Yamamori H, et al. Sex differences of leukocytes DNA methylation adjusted for estimated cellular proportions. Biol Sex Differ (2015) 6:0–6. doi: 10.1186/s13293-015-0029-7
23. Lança T, Silva-Santos B. The split nature of tumor-infiltrating leukocytes: Implications for cancer surveillance and immunotherapy. Oncoimmunology (2012) 1:717–25. doi: 10.4161/onci.20068
24. Khanna V, Achey RL, Ostrom QT, Block-Beach H, Kruchko C, Barnholtz-Sloan JS, et al. Incidence and survival trends for medulloblastomas in the united states from 2001 to 2013. J Neurooncol (2017) 135:433–41. doi: 10.1007/s11060-017-2594-6
25. Brown MA, Su MA. An inconvenient variable: sex hormones and their impact on T cell responses Melissa. J Immunol (2019) 202:1927–33. doi: 10.1126/science.1249098.Sleep
26. Gant JC, Chen KC, Norris CM, Kadish I, Thibault O, Blalock EM, et al. Disrupting function of FK506-binding protein 1b/12.6 induces the Ca 2+-dysregulation aging phenotype in hippocampal neurons. J Neurosci (2011) 31:1693–703. doi: 10.1523/JNEUROSCI.4805-10.2011
27. Dupont E, Sansal I, Evrard C, Rouget P. Developmental pattern of expression of NPDC-1 and its interaction with E2F-1 suggest a role in the control of proliferation and differentiation of neural cells. J Neurosci Res (1998) 51:257–67. doi: 10.1002/(SICI)1097-4547(19980115)51:2<257::AID-JNR14>3.0.CO;2-5
28. Grassi D, Franz H, Vezzali R, Bovio P, Heidrich S, Dehghanian F, et al. Neuronal activity, TGFβ-signaling and unpredictable chronic stress modulate transcription of Gadd45 family members and DNA methylation in the hippocampus. Cereb Cortex (2017) 27:4166–81. doi: 10.1093/cercor/bhx095
29. Ritchie SJ, Cox SR, Shen X, Lombardo MV, Reus LM, Alloza C, et al. Sex differences in the adult human brain: Evidence from 5216 UK biobank participants. Cereb Cortex (2018) 28:2959–75. doi: 10.1093/cercor/bhy109
30. Kaczkurkin AN, Raznahan A, Satterthwaite TD. Sex differences in the developing brain: insights from multimodal neuroimaging. Neuropsychopharmacology (2019) 44:71–85. doi: 10.1038/s41386-018-0111-z
31. Ahmed AA, Mohamed AD, Gener M, Li W, Taboada E. YAP and the hippo pathway in pediatric cancer. Mol Cell Oncol (2017) 4:e1295127. doi: 10.1080/23723556.2017.1295127
32. Matsui Y, Mineharu Y, Noguchi Y, Hattori EY, Kubota H, Hirata M, et al. Chlorambucil-conjugated PI-polyamides (Chb-m’), a transcription inhibitor of RUNX family, has an anti-tumor activity against SHH-type medulloblastoma with p53 mutation. Biochem Biophys Res Commun (2022) 620:150–7. doi: 10.1016/j.bbrc.2022.06.090
33. Gonçalves CS, Le Boiteux E, Arnaud P, Costa BM. HOX gene cluster (de)regulation in brain: from neurodevelopment to malignant glial tumours. Cell Mol Life Sci (2020) 77:3797–821. doi: 10.1007/s00018-020-03508-9
34. Aref D, Moffatt CJ, Agnihotri S, Ramaswamy V, Dubuc AM, Northcott PA, et al. Canonical TGF-beta pathway activity is a predictor of SHH-driven medulloblastoma survival and delineates putative precursors in cerebellar development. Brain Pathol (2013) 23:178–91. doi: 10.1111/j.1750-3639.2012.00631.x
35. Kool M, Korshunov A, Remke M, Jones DTW, Schlanstein M, Northcott PA, et al. Molecular subgroups of medulloblastoma: An international meta-analysis of transcriptome, genetic aberrations, and clinical data of WNT, SHH, group 3, and group 4 medulloblastomas. Acta Neuropathol (2012) 123:473–84. doi: 10.1007/s00401-012-0958-8
36. Williams LA, Spector LG. Survival differences between males and females diagnosed with childhood cancer. JNCI Cancer Spectr (2019) 3:1–11. doi: 10.1093/jncics/pkz032
37. Dressler EV, Dolecek TA, Liu M, Villano JL. Demographics, patterns of care, and survival in pediatric medulloblastoma. J Neurooncol (2017) 132:497–506. doi: 10.1007/s11060-017-2400-5
38. Ramaswamy V, Remke M, Bouffet E, Bailey S, Clifford SC, Doz F, et al. Risk stratification of childhood medulloblastoma in the molecular era: the current consensus. Acta Neuropathol (2016) 131:821–31. doi: 10.1007/s00401-016-1569-6
39. Ostrom QT, Gittleman H, Liao P, Vecchione-Koval T, Wolinsky Y, Kruchko C, et al. CBTRUS statistical report: Primary brain and other central nervous system tumors diagnosed in the united states in 2010-2014. Neuro Oncol (2017) 19:v1–v88. doi: 10.1093/neuonc/nox158
40. Ruden E, Reardon DA, Coan AD, Herndon JE, Hornsby WE, West M, et al. Exercise behavior, functional capacity, and survival in adults with malignant recurrent glioma. J Clin Oncol (2011) 29:2918–23. doi: 10.1200/JCO.2011.34.9852
41. Johansen ML, Stetson LC, Vadmal V, Waite K, Berens ME, Connor JR, et al. Gliomas display distinct sex-based differential methylation patterns based on molecular subtype. Neuro-Oncology Adv (2020) 2:1–12. doi: 10.1093/noajnl/vdaa002
42. Schüller U, Heine VM, Mao J, Kho AT, Dillon AK, Han YG, et al. Acquisition of granule neuron precursor identity is a critical determinant of progenitor cell competence to form shh-induced medulloblastoma. Cancer Cell (2008) 14:123–34. doi: 10.1016/j.ccr.2008.07.005
43. Johnson KJ, Cullen J, Barnholtz-Sloan JS, Ostrom QT, Langer CE, Turner MC, et al. Childhood brain tumor epidemiology: A brain tumor epidemiology consortium review. Cancer Epidemiol Biomarkers Prev (2015) 23:2716–36. doi: 10.1158/1055-9965.EPI-14-0207.Childhood
44. Mills LJ, Spector LG, Largaespada DA, Williams LA. Sex differences in expression of immune elements emerge in children, young adults and mice with osteosarcoma. Biol Sex Differ (2021) 12:1–12. doi: 10.1186/s13293-020-00347-y
45. Sun T, Warrington NM, Luo J, Brooks MD, Dahiya S, Snyder SC, et al. Sexually dimorphic RB inactivation underlies mesenchymal glioblastoma prevalence in males. J Clin Invest (2014) 124:4123–33. doi: 10.1172/JCI71048
46. Smolic M, Bozic I, Omanovic T. 2017 U. Pharmacogenomics: recent progress, sex gender differences, translation into clinical practice, application in pediatrics and future perspectives. Southeaster Eur Med J (2017) 1:108–20. doi: 10.26332/seemedj.v1i1.21
47. Thomaz A, Jaeger M, Brunetto AL, Brunetto AT, Gregianin L, de Farias CB, et al. Neurotrophin signaling in medulloblastoma. Cancers (Basel) (2020) 12:1–22. doi: 10.3390/cancers12092542
Keywords: medulloblastoma, sex differences, methylation, survival, pediatric and young adult cancer
Citation: Moss RM, Sorajja N, Mills LJ, Moertel CL, Hoang TT, Spector LG, Largaespada DA and Williams LA (2023) Sex differences in methylation profiles are apparent in medulloblastoma, particularly among SHH tumors. Front. Oncol. 13:1113121. doi: 10.3389/fonc.2023.1113121
Received: 01 December 2022; Accepted: 15 March 2023;
Published: 24 March 2023.
Edited by:
Syed Ahsan Raza, Department of Medicine, Baylor College of Medicine, United StatesReviewed by:
Sébastien Perreault, CHU Sainte-Justine, CanadaJoseph Louis Lasky, Cure 4 The Kids, United States
Copyright © 2023 Moss, Sorajja, Mills, Moertel, Hoang, Spector, Largaespada and Williams. This is an open-access article distributed under the terms of the Creative Commons Attribution License (CC BY). The use, distribution or reproduction in other forums is permitted, provided the original author(s) and the copyright owner(s) are credited and that the original publication in this journal is cited, in accordance with accepted academic practice. No use, distribution or reproduction is permitted which does not comply with these terms.
*Correspondence: Lindsay A. Williams, bGF3aWxsaWFtc0B1bW4uZWR1