- 1Institute of Traditional Chinese Medicine in Oncology, Longhua Hospital, Shanghai University of Traditional Chinese Medicine, Shanghai, China
- 2Department of Oncology, Longhua Hospital, Shanghai University of Traditional Chinese Medicine, Shanghai, China
- 3Department of Neurology, Longhua Hospital, Shanghai University of Traditional Chinese Medicine, Shanghai, China
- 4Department of Traditional Chinese Medicine, Ruijin Hospital, Shanghai Jiao Tong University School of Medicine, Shanghai, China
Background: Solanum nigrum L. (SNL) (Longkui) is a Chinese herb that can be used to treat colon cancer. The present study explored the components and mechanisms of SNL in treating colon cancer by using network pharmacology and molecular docking.
Methods: The components of SNL were collected from the TCMSP, ETCM, HERB, and NPASS databases. Meanwhile, the target proteins of these ingredients were collected/predicted by the TCMSP, SEA, SwissTargetPrediction, and the STITCH databases colon cancer-related target genes were identified from TCGA and GTEx databases. The interaction networks were established via Cytoscape 3.7.2. Gene Ontology and KEGG pathways were enriched by using the David 6.8 online tool. Finally, the binding of key components and targets was verified by molecular docking, and the cellular thermal shift assay (CETSA) was used to detect the efficiency of apigenin and kaempferol binding to the AURKB protein in CT26 cells.
Results: A total of 37 SNL components, 796 SNL targets, 5,356 colon cancer genes, and 241 shared targets of SNL and colon cancer were identified. A total of 43 key targets were obtained through topology analysis. These key targets are involved in multiple biological processes, such as signal transduction and response to drug and protein phosphorylation. At the same time, 104 signaling pathways, such as pathways in cancer, human cytomegalovirus infection, and PI3K-Akt signaling pathway, are also involved. The binding of the four key components (i.e., quercetin, apigenin, kaempferol, and luteolin) and the key targets was verified by molecular docking. The CETSA results showed that apigenin and kaempferol were able to bind to the AURKB protein to exert anti-CRC effects.
Conclusions: Quercetin, apigenin, kaempferol, and luteolin are the main components of SNL in treating colon cancer. SNL regulates multiple bioprocesses via signaling pathways, such as pathways in cancer, PI3K-Akt, and cell cycle signaling pathways.
1 Introduction
Colorectal cancer (CRC), including colon and rectal cancers, is a common malignant tumor that threatens human health within a global scope. Its incidence rate ranks top three among all malignant tumors, accounting for 10.0% of the incidence rate of all cancers, with a mortality rate of 9.4% (1). CRC can be treated with surgery, chemotherapy, radiotherapy, targeted therapy and immunotherapy. However, the effect of the treatment is always unsatisfactory. As an important treatment method for CRC, traditional Chinese medicine can inhibit cell proliferation; induce apoptosis, autophagy, and cell senescence; relieve patients’ symptoms; improve their quality of life; alleviate the toxic and side effects of chemoradiotherapy; repress metastasis and recurrence; and enhance the long-term treatment effect (2, 3).
Solanum nigrum L. (SNL) (Longkui) is a traditional Chinese medicine that is commonly applied to cancer treatment. SNL can inhibit the proliferation and metastatic potential of RKO CRC cells (4). SNL also induces autophagy and enhances the cytotoxicity of chemotherapy in CRC (5). With cytotoxic activity for MCF-7 cells in human breast cancer, SNL can inhibit cell migration and regulate multiple gene expressions (6). In oral cancer, SNL extracts can activate caspase-9 and caspase-3 and induce mitochondria pathway apoptosis by boosting the production of reactive oxygen species (ROS). Moreover, they can repress cell proliferation through the downregulation of cyclin-dependent kinase 1 (CDK1) and cyclin B1 (7). When applied to treat prostate cancer, SNL can arrest the cell cycle in the G2/M phase and induce cell apoptosis (8). However, the effective components of SNL and its anticancer mechanism remain to be further investigated.
Network pharmacology, an emerging discipline in recent years, can expound the relationships among “drug–gene–disease” from the aspects of the system to reveal the active components and action mechanisms of drugs (9). Chinese herbal medicinal components are complicated with diversified action characteristics and involve multiple target genes and signaling pathways. Hence, they are suitable for network pharmacology studies. In this study, the active components, targets, and related pathways of SNL in the treatment of colon cancer were explored, the “compound–target–pathway” network was established through the network pharmacology method, the key components and targets of SNL that acted on colon cancer were obtained through network topology analysis and annotated by Gene Ontology (GO) and pathway enrichment, and the binding between key components of SNL and targets was also evaluated via molecular docking, expecting to provide a scientific basis for the study and application of SNL.
2 Materials and methods
2.1 Identification of components of SNL
The SNL components were retrieved from the following databases: the Traditional Chinese Medicine Systems Pharmacology Database and Analysis Platform (TCMSP, http://tcmspw.com/tcmsp.php) (10), The Encyclopedia of Traditional Chinese Medicine (ETCM, http://www.tcmip.cn/ETCM/) (11), the Natural Product Activity and Species Source Database (NPASS, http://bidd.group/NPASS/index.php) (12), SymMap (http://www.symmap.org/) (13), and HERB (http://herb.ac.cn/) (14). The compound properties were inquired from the TCMSP (oral bioavailability (OB) ≥ 20%, drug-likeness (DL) ≥ 0.10, https://old.tcmsp-e.com/load_intro.php?id=29) or ETCM (drug-likeness weight (DW) ≥ 0.49).
2.2 Screening and prediction of compound-related targets
The target genes were retrieved from the TCMSP and ETCM. The target genes in the SNL components were also predicted using Similarity Ensemble Approach (SEA, https://sea.bkslab.org/) (15), STITCH (http://stitch.embl.de/) (16), and SwissTargetPrediction (http://swisstargetprediction.ch/) (17) based on the chemical similarity and pharmacophore model. The SMILE number of compounds was retrieved from PubChem (https://pubchem.ncbi.nlm.nih.gov/) and submitted into the SEA, STITCH, and SwissTargetPrediction databases. Next, the targets in active SNL components were predicted by taking Max Tc ≥ 0.4, confidence score ≥ 0.4, and probability value ≥ 0.5 as the criteria, and the gene name was converted into its official gene symbol.
2.3 Collection of colon cancer-related protein targets
The Gene Expression Profiling Interactive Analysis (GEPIA2, http://gepia2.cancer-pku.cn/#index) online tool (18) was used to identify the differentially expressed genes (fold change (FC) ≥ 2, q-value < 0.01) of colon adenocarcinoma (COAD) in The Cancer Genome Atlas (TCGA) and Genotype-Tissue Expression (GTEx) databases.
2.4 Network construction and topological analysis
The intersection set between SNL and COAD target genes was acquired using the Venn online tool (https://www.omicshare.com/tools/Home/Soft/venn), and overlapped targets were uploaded to the STRING database (https://string-db.org/) (19), the protein–protein network (PPI) was constructed, and the targets that satisfied the confidence score of ≥ 0.7 were screened out. Interaction network and topological analysis were conducted via Cytoscape 3.7.2 (20), including (1) the compound-target network of SNL, (2) SNL compound–target–pathway network, (3) the compound-overlapped target network, and (4) the compound–key target networks. In the compound-overlapped target network, the target genes that satisfied the degree centrality (DC) ≥ 2 × median DC, betweenness centrality (BC) ≥ median BC, and closeness centrality (CC) ≥ median CC were screened out as the key targets of SNL acted upon COAD.
2.5 GO and pathway enrichment analysis
The DAVID 6.8 database (https://david.ncifcrf.gov/) is an online tool for genetic function annotation (21). The key targets of SNL acted upon COAD were imported into the DAVID 6.8 database, the species were restricted to “Homo sapiens”, and the name of each target gene was converted into their official gene symbols, followed by GO (22) and Kyoto Encyclopedia of Genes and Genomes (KEGG) pathway (23–25) enrichment analysis, and visualized in online tools (http://www.bioinformatics.com.cn/, http://vip.sangerbox.com/home.html) (26).
2.6 Molecular docking
The protein structures were inquired about in the Protein Data Bank (PDB) (https://www.rcsb.org/) database, and the file in PDB format was downloaded (27). After the deletion of the water molecule, macromolecular ligand, and symmetric chain in PyMOL v.3.8 software, the file was saved in the PDB format (28). Operations such as hydrogenation, charge calculation, and addition of atom type were implemented for this protein file using AutoDockTools (29), and then it was saved in PDBQT format as the receptor. The SDF files of SNL compounds were retrieved from the PubChem database and optimized in the Chem3D 15.1 module of ChemOffice software, and then the SDF format was converted into mol2 format. Next, the root of the ligand was detected, and its rotatable bond was selected in AutoDockTools and exported in PDBQT format as the ligand. The affinity score was obtained by molecular docking in AutoDockTools software, and the binding site was visualized via the PLIP website (https://projects.biotec.tu-dresden.de/plip-web/plip/) (30) and PyMOL v.3.8 software. The active components with an affinity score ≤ −5 kJ/mol were selected as the criteria for effective binding.
2.7 Cytological experiment
Mouse colon cancer CT26 cells were obtained from the Cell Bank of Type Culture Collection of the Chinese Academy of Sciences (Shanghai, China) and cultured in RPMI1640 medium (Gibco, Grand Island, NY, USA) containing 10% fetal bovine serum (Gibco, Grand Island, NY, USA) in an incubator at 37°C with 5% CO2 and saturated humidity. Logarithmic growth stage cells were used in the experiment. Cellular thermal shift assay (CETSA) was used to detect the binding efficiency of drugs to the corresponding target proteins in CT26 cells (31, 32). CT26 cells were seeded in 10 cm culture dishes with a density of 5 × 105 cells/ml. CT26 cells were collected 24 h later and washed with cold PBS three times. Cells were resuspended in RIPA lysate buffer containing protease inhibitor cocktail (Beyotime, Shanghai, China). Cell lysates were prepared by centrifugation at 12,000 rpm for 15 min at 4°C, and supernatants were collected. The supernatant was divided into three equal fractions and treated with DMSO, 100 μM Apigenin (APExBIO, Houston, USA), and 100 μM Kaempferol (APExBIO, Houston, USA), respectively. After incubating at room temperature for 1 h, the three parts were divided into nine parts (60 µl each) and heated at different temperatures (50, 55, 60, 65, 70, 75, 80, and 85°C and one aliquot kept at room temperature as control) for 3 min, followed by cooling at room temperature for 3 min. The heated lysates were centrifuged at 15,000 rpm for 20 min at 4°C, and the precipitate and soluble fraction were separated in an ice bath. The supernatant was transferred to a new centrifuge tube, analyzed by SDS-PAGE, and Western blot analysis was performed using aurora kinase B (AURKB; 1:1,000; Beyotime, Shanghai, China).
2.8 Statistical analysis
The data were expressed as mean ± standard deviation (SD). A two independent sample t-test was used when the data satisfy normality distribution and homogeneity of variance. t’-test was used when the data satisfy normality distribution but not homogeneity of variance. Non-parametric rank sum test was used for non-normally distributed data. Statistically significant differences were considered at p < 0.05.
3 Results
3.1 Active components of SNL
A total of 39 SNL components were acquired from the TCMSP database, 35 from ETCM, 114 from HERB, and 60 from NPASS. Repeated components were deleted and subjected to the OB and DL/DW screening, and then 37 effective compounds were obtained. The targets were collected and predicted in the TCMSP, STITCH, SwissTargetPrediction, and SEA databases, and 796 targets were acquired in total (Table 1; Figure 1). The components that involve over 100 target genes included quercetin, kaempferol, apigenin, and solasodine.
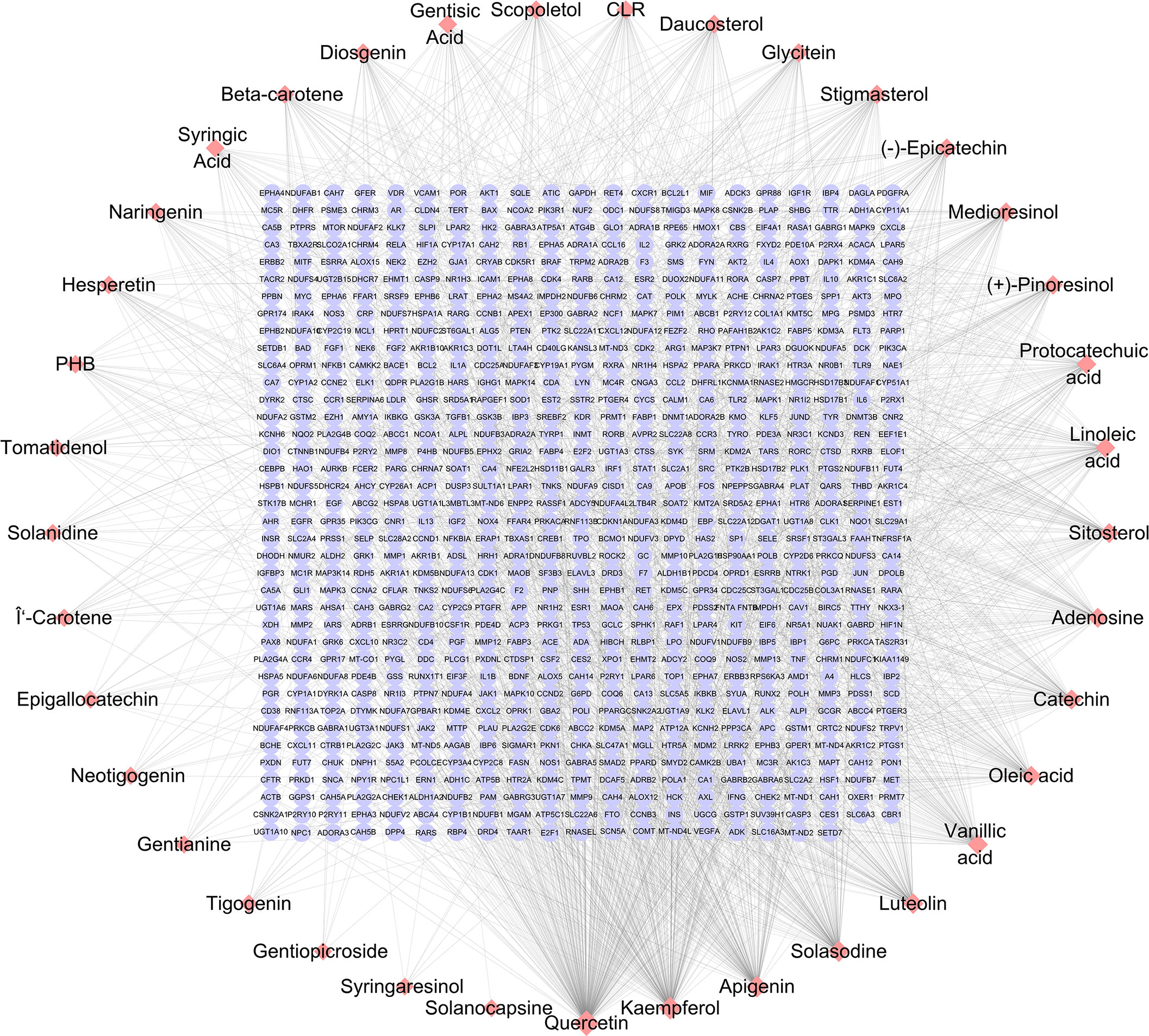
Figure 1 SNL component–target network. The networks were generated by Cytoscape 3.7.2 software. Pink diamond and violet round nodes represent the compounds in SNL and the potential targets of SNL, respectively. Topology analysis shows that the node size is proportional to the degree of centrality.
3.2 GO and pathway enrichment analysis of SNL targets
The GO and KEGG enrichment analyses (Figures 2A, B) were performed using the DAVID 6.8 online tool. The results showed that the targets of SNL mainly existed in cell regions, such as the plasma membrane, cytoplasm, nucleus, membrane, mitochondrion, endoplasmic reticulum, and mitochondrial inner membrane, with their molecular functions involving the binding to protein, ATP, zinc ion, enzyme, and sequence-specific DNA. Meanwhile, they were correlated with the activity of transcription factors and protein kinases and took a part in bioprocesses, such as signal transduction, oxidation–reduction process, protein phosphorylation, drug response, inflammatory response, gene expression, cell proliferation, and apoptotic processes (Figure 2A). According to the KEGG pathway analysis results, a total of 148 pathways were affected by the active components of SNL (p < 0.05), and those ranking in the top 12 (gene number ≥ 50) included metabolic pathways, pathways in cancer, neuroactive ligand–receptor interaction, PI3K-Akt signaling pathway, non-alcoholic fatty liver disease (NAFLD), Alzheimer’s disease, hepatitis B, MAPK signaling pathway, HTLV-I infection, Huntington’s disease, Parkinson’s disease, and Ras signaling pathway (Figures 2B, 3).
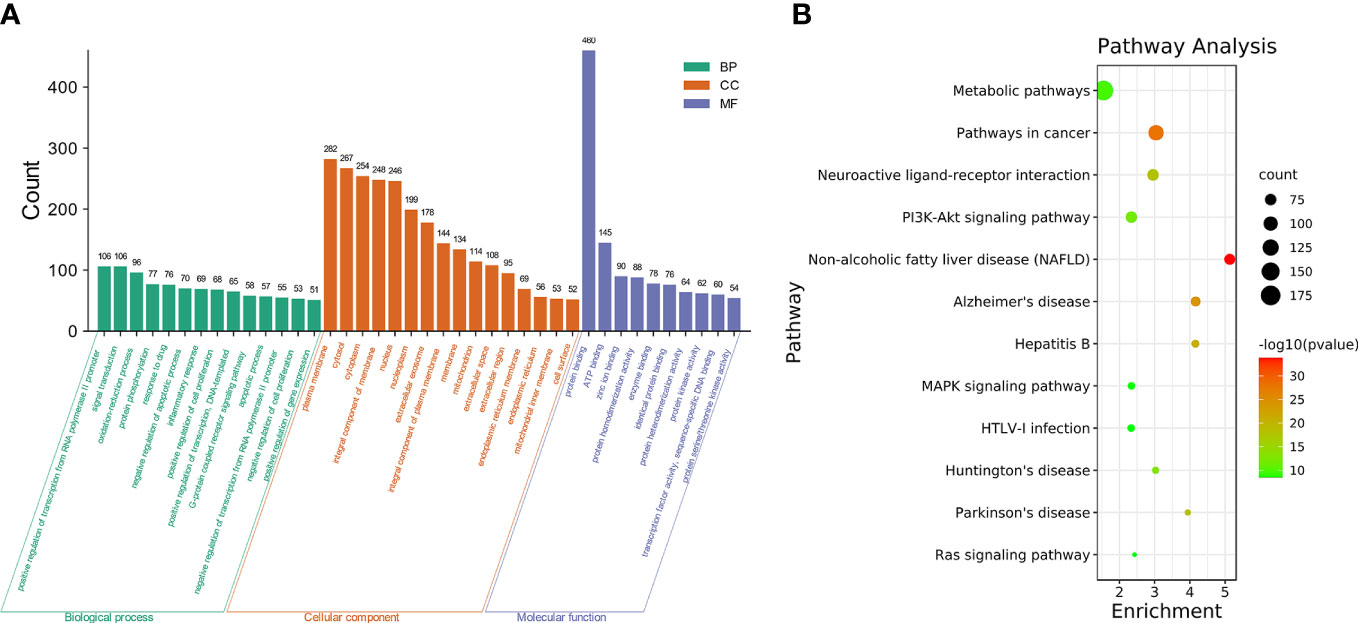
Figure 2 GO and pathway enrichment of SNL targets. (A) GO enrichment of SNL targets (count number ≥ 50). (B) KEGG pathway enrichment analysis of SNL targets (count number ≥ 50).
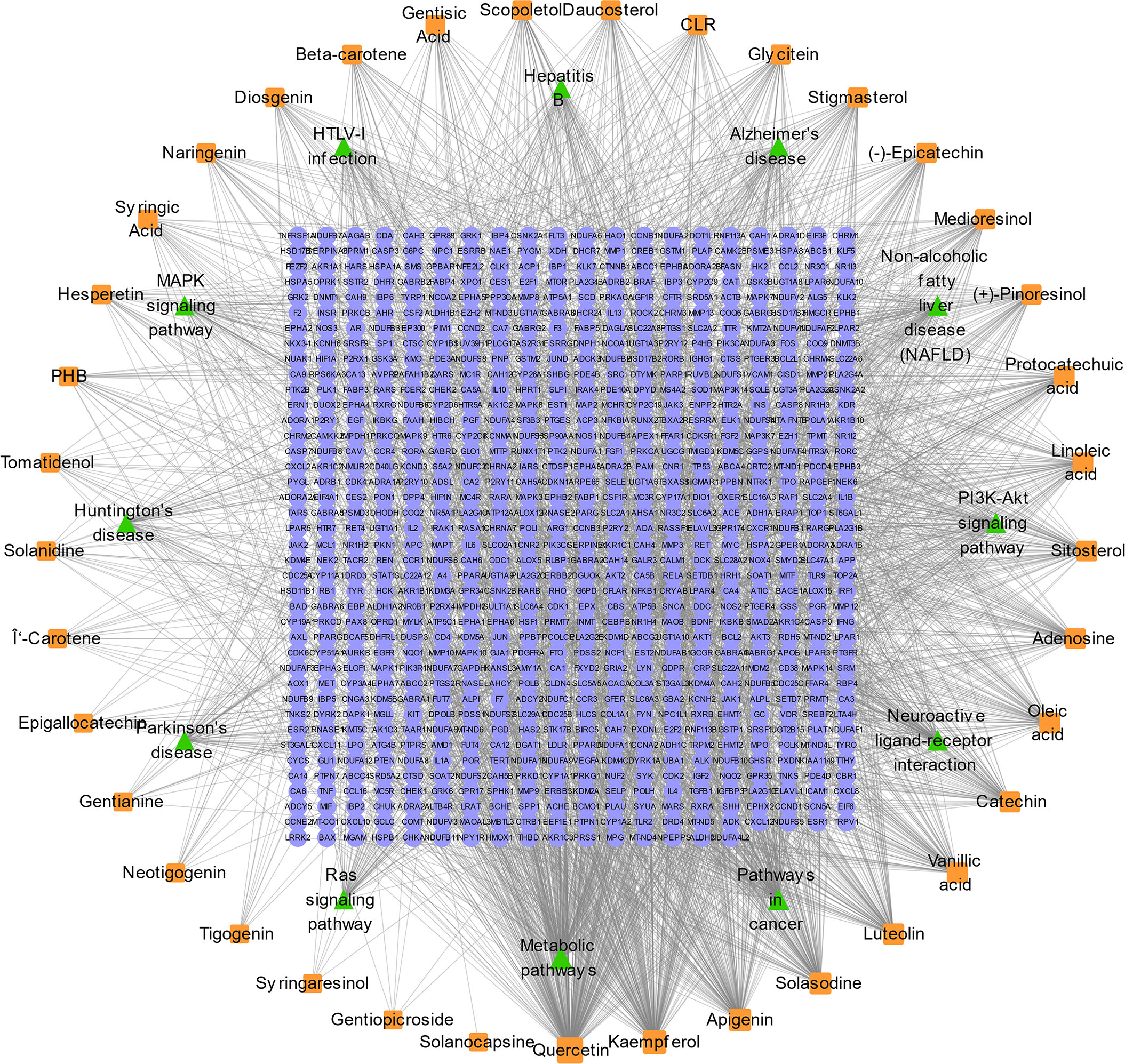
Figure 3 SNL compound–target–pathway network. SNL compound–target–pathway (count number ≥ 50) networks were generated by Cytoscape 3.7.2 software. Light blue ellipse, orange round, and green triangle nodes stand for SNL targets, SNL compounds, and pathways, respectively.
3.3 Identification of SNL targets against COAD
The GEPIA2 online tool screen showed 5,356 genes (i.e., 2,682 upregulated genes and 2,674 downregulated genes) differentially expressed in COAD (Figure 4A; Table 2). The Venn analysis showed 241 overlapped targets of SNL and COAD (Figure 4B). Through the PPI analysis in the STRING database, 215 targets showed high interactions (confidence score ≥ 0.7). A compound-overlapped target network, which consisted of 215 nodes and 930 edges, and the node size was in direct proportion to the degree of centrality, was constructed via Cytoscape 3.7.2. By the topological analysis of this network, 43 key targets and 254 interactions were acquired, and all the targets presented high interactions (confidence score ≥ 0.7) (Figure 4C; Table 3). The key targets related to the main SNL components are listed in Table 4, where the components with a number of key targets of ≥ 5 were quercetin, apigenin, kaempferol, luteolin, adenosine, solasodine, sitosterol, and linoleic acid.
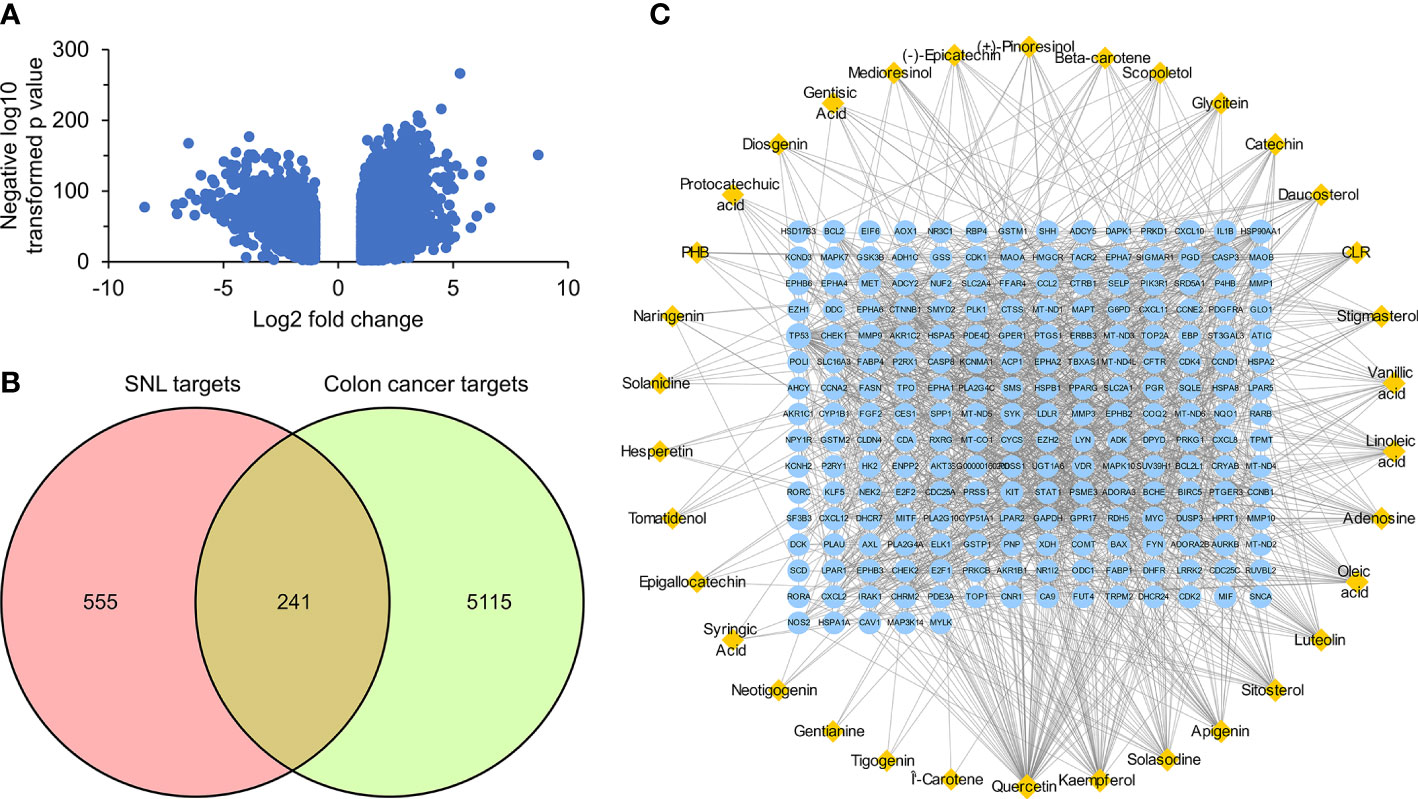
Figure 4 Overlapped targets of SNL and colon cancer. (A) Differently expressed genes in colon cancer were identified by the GEPIA2 online tool from TCGA and GTEx databases and expressed as volcano plots. (B) Shared targets of SNL and colon cancer. (C) SNL compound-shared target networks. Topology analysis shows that the node size is proportional to the degree of centrality.
3.4 GO enrichment analysis of SNL key targets
GO and pathway enrichment analyses of key targets were carried out via DAVID6.8 online tool. The GO analysis manifested that these targets mainly existed in regions, such as the nucleus, cytoplasm, cytosol, nucleoplasm, plasma membrane, extracellular region, perinuclear region of cytoplasm, centrosome, an integral component of the plasma membrane, and chromatin (Figure 5A). They could also bind to molecules, such as protein, ATP, protein kinase, enzyme, transcription factor, and receptor, which were related to the activity of protein kinase and tyrosine/serine/threonine kinase (Figure 5B). Furthermore, they participated in various bioprocesses, such as signal transduction, response to drug, protein phosphorylation, positive regulation of gene expression, G-protein coupled receptor signaling pathway, regulation of transcription from RNA polymerase II promoter, negative regulation of the apoptotic process, positive regulation of transcription, DNA-templated, and response to xenobiotic stimulus (Figure 5C).
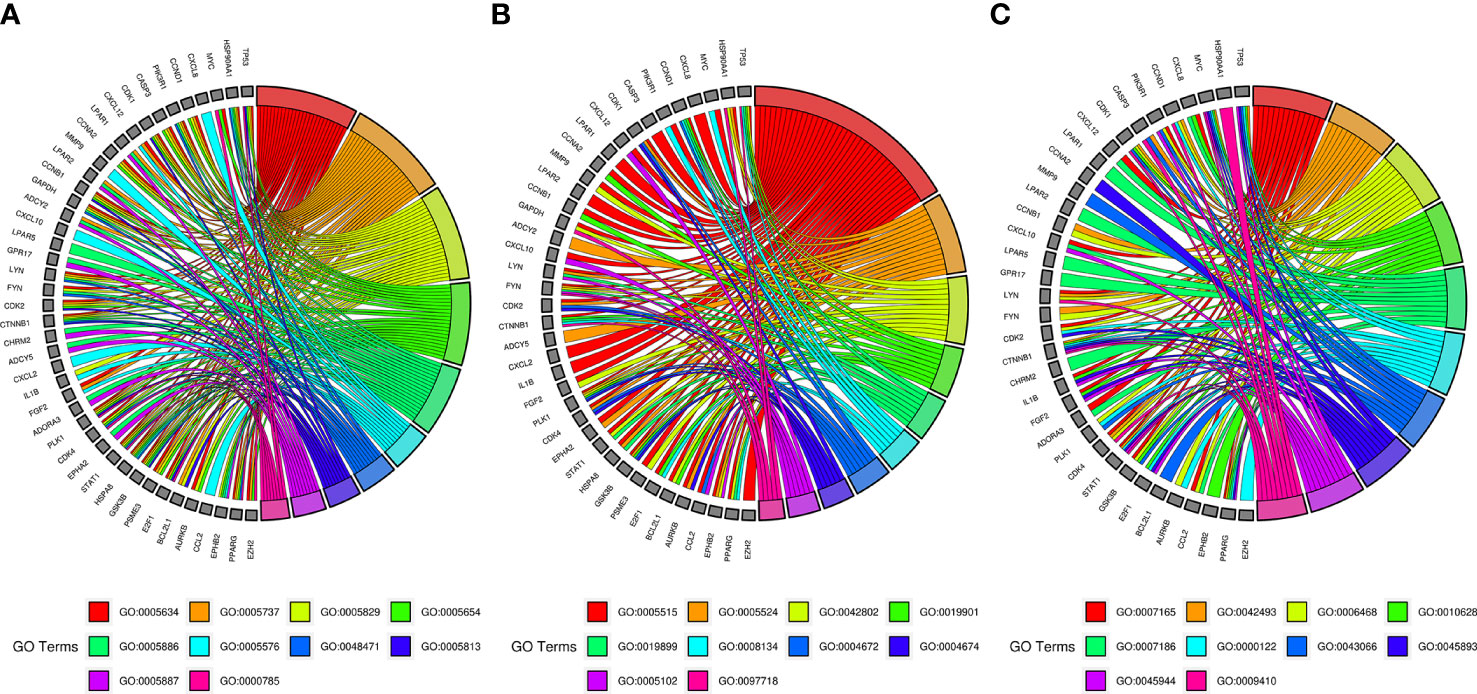
Figure 5 GO enrichment analysis of SNL key targets. (A) Cellular component enrichment. GO:0005634, nucleus; GO:0005737, cytoplasm; GO:0005829, cytosol; GO:0005654, nucleoplasm; GO:0005886, plasma membrane; GO:0005576, extracellular region; GO:0048471, perinuclear region of cytoplasm; GO:0005813, centrosome; GO:0005887, integral component of plasma membrane; GO:0000785, chromatin. (B) Molecular function enrichment. GO:0005515, protein binding; GO:0005524, ATP binding; GO:0042802, identical protein binding; GO:0019901, protein kinase binding; GO:0019899, enzyme binding; GO:0008134, transcription factor binding; GO:0004672, protein kinase activity; GO:0004674, protein serine/threonine kinase activity; GO:0005102, receptor binding; GO:0097718, disordered domain-specific binding. (C) Biological process enrichment. GO:0007165, signal transduction; GO:0042493, response to drug; GO:0006468, protein phosphorylation; GO:0010628, positive regulation of gene expression; GO:0007186, G-protein-coupled receptor signaling pathway; GO:0000122, negative regulation of transcription from RNA polymerase II promoter; GO:0043066, negative regulation of apoptotic process; GO:0045893, positive regulation of transcription, DNA templated; GO:0045944, positive regulation of transcription from RNA polymerase II promoter; GO:0009410, response to a xenobiotic stimulus.
3.5 Pathway enrichment analysis of SNL key targets
According to the pathway enrichment analysis, 104 pathways, including pathways in cancer, human cytomegalovirus infection, PI3K-Akt signaling pathway, Kaposi sarcoma-associated herpesvirus infection, lipid and atherosclerosis, hepatitis C, Epstein–Barr virus infection, cell cycle, measles, cellular senescence, chemokine signaling pathway, and human T-cell leukemia virus 1 infection, were affected by key targets (p < 0.05) (Figures 6A, B). The key targets distributed in pathways in cancer are displayed in Figure 7.
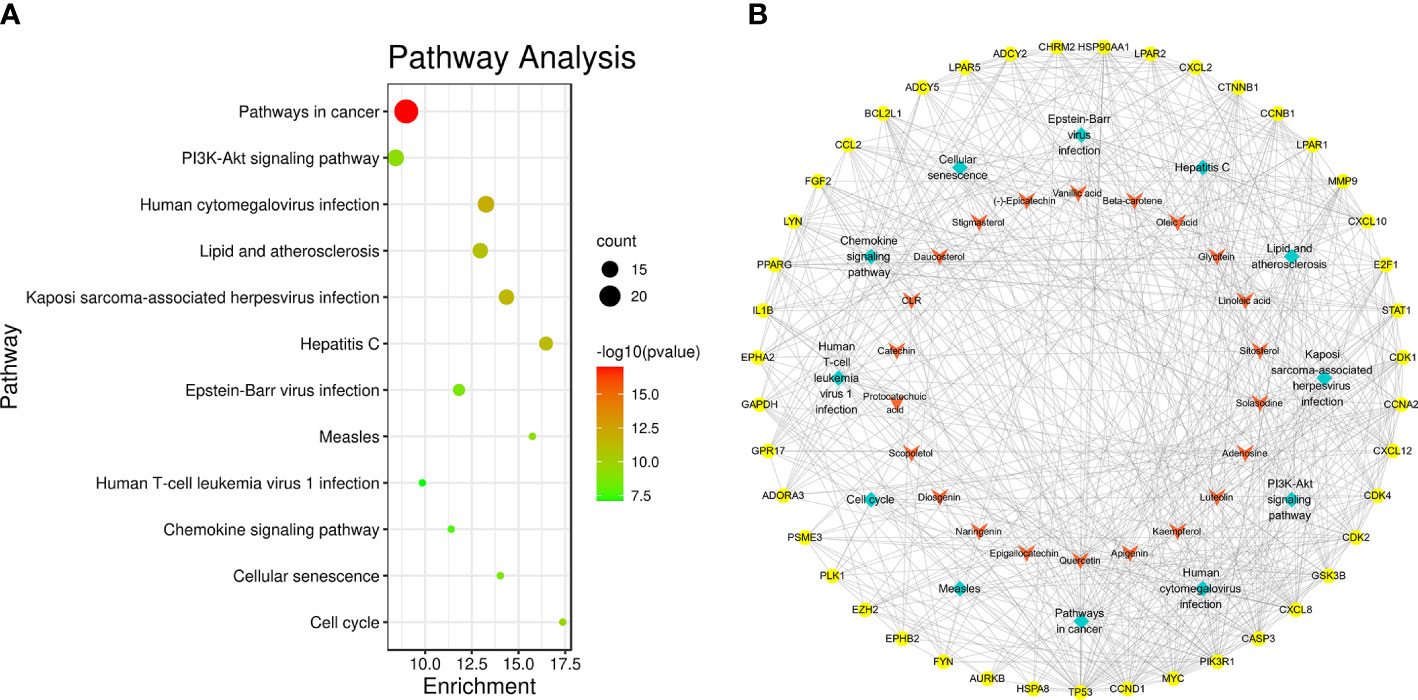
Figure 6 Pathway enrichment analysis of SNL key targets. (A) KEGG pathway enrichment. (B) Network of the compound–key target–pathways. Yellow octagon, red V, and cyan diamond nodes stand for key targets, compounds, and pathways, respectively.
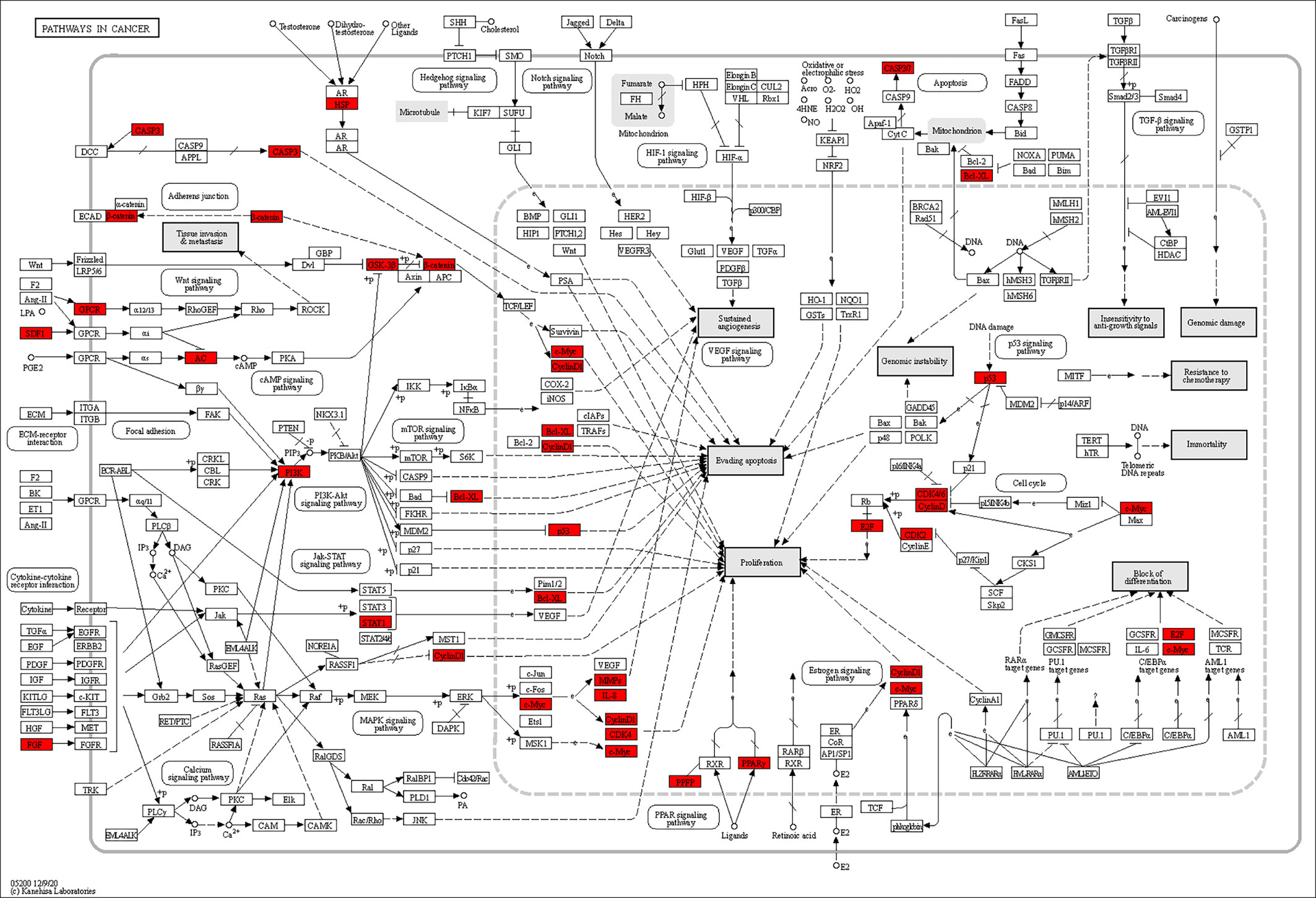
Figure 7 Distribution of key targets in pathways in cancer. The red rectangles stand for the key targets. (KEGG Copyright Permission 230270).
3.6 Molecular docking
Quercetin, apigenin, kaempferol, and luteolin were selected and docked with the corresponding key targets by using the AutoDockTools software. The binding sites between compounds and key targets were visualized using the PLIP website and PyMOL v.3.8 software. The lower the binding energy between ligand and receptor, the stabler their binding conformation would be. By screening according to the criterion of affinity score ≤ −5 kJ/mol, four active components (i.e., quercetin, apigenin, kaempferol, and luteolin) could stably bind to the corresponding key targets. Quercetin and CASP3 formed two hydrogen bonds through ASP-2 and SER-251. Apigenin and AURKB formed two hydrogen bonds through HIS-192 and GLY-193. Linoleic acid and CCNA2 formed a hydrogen bond through PRO-155. Kaempferol and AURKB formed two hydrogen bonds through LEU-83 and PHE-219. All of them form two hydrogen bonds each, except for linoleic acid and CCNA2, which form one hydrogen bond. The findings above indicated that quercetin, apigenin, and kaempferol are probably the core active components of SNL (Figure 8).
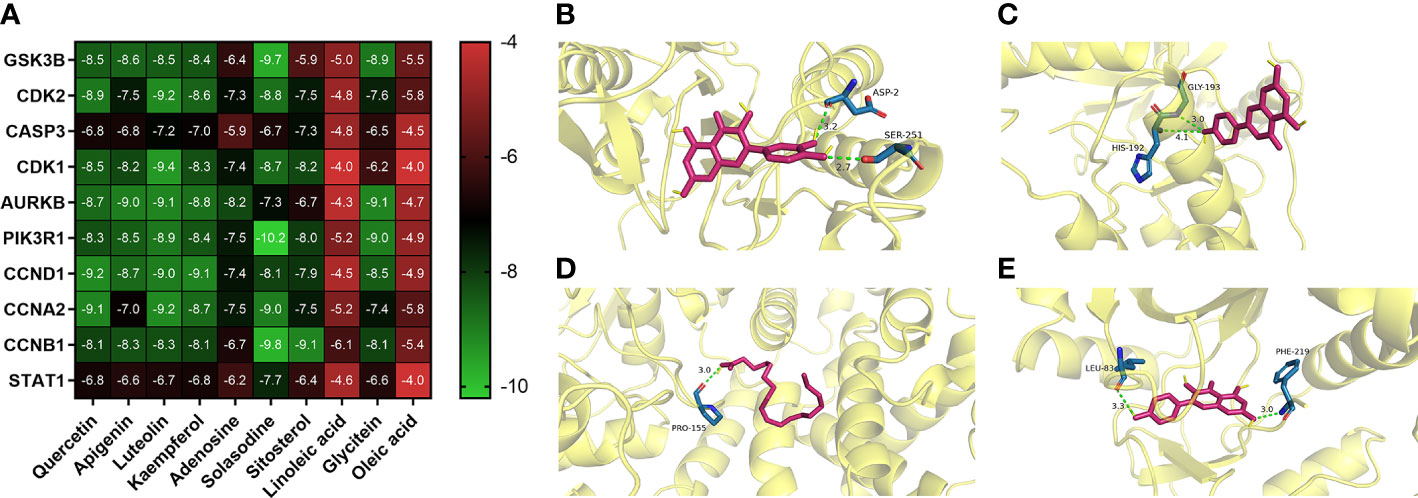
Figure 8 Representative molecular docking. (A) Binding affinity score of key compounds and key targets. (B) Quercetin binding with CASP3. (C) Apigenin binding with AURKB. (D) Linoleic acid binding with CCNA2. (E) Kaempferol binding with AURKB.
3.7 CETSA
The stability of compound-induced target proteins was examined by CETSA, and the interaction of apigenin and kaempferol with AURKB in CT26 cells was determined by a western blot assay. As shown in Figure 9, the expression of the AURKB protein decreased continuously with the increase in temperature. The heat stability of the AURKB protein in CT26 cells was significantly higher than that in the control group under the intervention of 100 μM apigenin and 100 μM kaempferol. Taken together, these biophysical binding characteristics suggested that AURKB may be a direct target of apigenin and kaempferol.
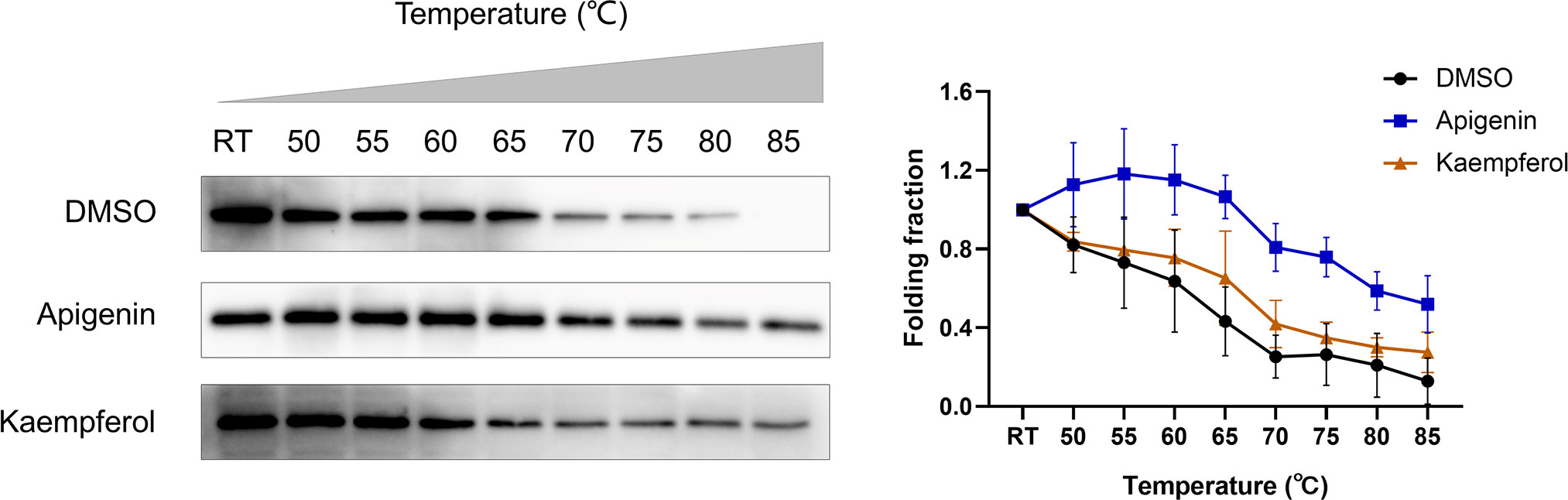
Figure 9 Cellular thermal shift assay (CETSA). CETSA was performed in CT26 cells treated with apigenin (100 μM), kaempferol (100 μM), or DMSO control. The effect of apigenin and kaempferol on AURKB protein stability was evaluated by Western blot assay. RT, room temperature.
4 Discussion
SNL is a commonly clinically used anticancer in traditional Chinese medicine. The antitumor effect of SNL is mainly manifested by repressing the proliferation, arresting the cell cycle, inducing cell apoptosis, and inhibiting the cell migration of tumor cells (33–36). However, the active components of SNL and their mechanisms remain unclear. The components and potential targets of SNL were explored through the network pharmacology method. The study results showed that SNL targets existed in multiple cellular areas, involved diversified molecular functions, and were correlated with pathways, such as metabolic pathways, pathways in cancer, neuroactive ligand–receptor interaction, PI3K-Akt signaling pathway, non-alcoholic fatty liver disease (NAFLD), Alzheimer’s disease, hepatitis B, MAPK signaling pathway, HTLV-I infection, Huntington’s disease, Parkinson’s disease, and Ras signaling pathway, suggesting that SNL exerts extensive pharmacological effects in cancers, nervous system diseases, non-alcoholic fatty liver disease, HBV, and HTLV-I virus-related diseases.
The results showed that compounds, such as quercetin, apigenin, kaempferol, luteolin, adenosine, solasodine, sitosterol, and linoleic acid, were the active components of SNL, and quercetin, apigenin, kaempferol, and luteolin were the key components of SNL in treating colon cancer. Quercetin, apigenin, kaempferol, and luteolin are all natural flavonoids that can be used as anticancer agents (37–40). Darband et al. found that quercetin has potential preventive properties in CRC by inhibiting proliferation, metastasis, and angiogenesis, inducing apoptosis, and regulating cell metabolic activities and related signaling pathways (41). In HCT116 cells, apigenin downregulated the expression of Cyclin B1 and its activating partners, Cdc2 and Cdc25c, induced poly (ADP-ribose) polymerase (PARP) cleavage, decreased the expression of procaspase-3, procaspase-8, and procaspase-9, and upregulated the level of LC3-II, suggesting that apigenin could regulate the cell cycle and induce apoptosis in HCT116 cells by inhibiting autophagy (42). Kaempferol has a significant antiproliferative and cytotoxic effect on HCT116, HCT15, and SW480 cells, which could promote the cleavage of PARP in HCT116 and HCT15 cells as well as the activation of caspase-8, caspase-9, and caspase-3, phosphor-p38 MAPK, p53, and p21. These changes were reversed after treatment with the ROS inhibitor NAC, the pan-caspase inhibitor z-vad-fmk, and the p38 MAPK inhibitor SB203580. The above results suggested that p38 phosphorylation and caspase activation mediated by ROS and p53 signaling are closely related to kaempferol-induced apoptosis in CRC cells (43). Luteolin has no effect on the proliferation of CRC cells but could inhibit the migration and invasion of CRC cells by downregulating MMP2, MMP9, and MMP16 in vitro and in vivo. The expression of miR-384 was downregulated and that of pleiotrophin (PTN) was upregulated compared with adjacent normal tissues, suggesting that the antitumor effects of luteolin were partly mediated through the miR-384/PTN axis (44)..
In addition to the four abovementioned key components, other components also had anti-CRC effects. Linoleic acid facilitates CRC cell apoptosis by inducing oxidative stress and mitochondrial dysfunction (45). Sitosterol activated caspase-9 and caspase-3 and boosted the CRC cell apoptosis by inhibiting PI3K/Akt and regulating the expressions of Bcl-xl and Bad (46). Solasodine induced the CRC cells’ apoptosis by regulating AKT/GSK3B/β-catenin signaling pathway and activating the caspase cascade (47). By regulating the IL-6/STAT3 pathway, beta-carotene repressed the polarization of M2 macrophages and inhibited the fibroblasts activated by transforming growth factor-β1 (TGF-β1) to inhibit the invasion and migration and the epithelial–mesenchymal transition of CRC cells, as well as azoxymethane/dextran sodium sulfate-induced CRC formation (48).
In this study, 43 key targets of SNL were found for the treatment of colon cancer. As serine/threonine kinase, AURKB was able to regulate the G2/M phase of the cell cycle in cell mitosis, and its overexpression was related to the CRC prognosis (49). Glycogen synthase kinase 3 beta (GSK3B) is a multifunctional serine/threonine kinase involved in mediating various cellular functions such as proliferation, apoptosis, metabolism, differentiation, and cell motility (50). Rosales-Reynoso et al. discovered that GSK3B may play a role in tumor progression by regulating the Wnt/β-catenin pathway and that GSK3B gene variation is associated with tumor location and tumor-node-metastasis (TNM) stage in CRC patients (51). Caspase-3 (CASP3) is an apoptotic executive protein, which can not only inhibit the invasion and metastasis of CRC cells but also increase the sensitivity of tumor cells to radiotherapy and chemotherapy (52). During cell division, cyclin-dependent kinase 2 (CDK2) is a core cell cycle regulator that is active from the late G1-phase to the S-phase. Overexpression of CDK2 leads to abnormal regulation of the cell cycle, which is directly related to the overproliferation of tumor cells. Thus, CDK2 inhibitors could induce antitumor activities (53, 54). CCND1, a type of cyclin, was correlated with the cell cycle and proliferation. Studies have shown that miR-374a could inhibit the PI3K-Akt pathway by lowering the expression of CCND1 to inhibit the proliferation of CRC cells (55). Transcription 1 (STAT1) can repress miR-181a, thereby further inhibiting the phosphatase and tensin homolog (PTEN)/Akt signal transduction and the proliferation of CRC cells (56). In addition, the molecular docking results showed that the key SNL components, namely, quercetin, apigenin, luteolin, and kaempferol, presented favorable binding activity to the corresponding key targets, such as GSK3B, CDK2, and CASP3. Subsequent CETSA experiments validated these results, showing that AURKB could bind to apigenin and kaempferol and perform the corresponding biological functions.
According to the results of GO and pathway enrichment analysis, the key targets of SNL can bind to protein, ATP, protein kinase, enzyme, transcription factor, and receptor; regulate the activities of tyrosine/serine/threonine kinases; influence pathways in cancer, human cytomegalovirus infection, PI3K-Akt signaling pathway, Kaposi sarcoma-associated herpesvirus infection, lipid and atherosclerosis, hepatitis C, Epstein–Barr virus infection, cell cycle, measles, cellular senescence, chemokine signaling pathway, and human T-cell leukemia virus 1 infection and further took a part in bioprocesses, such as signal transduction, response to the drug, protein phosphorylation, regulation of the apoptotic process, gene expression, and transcription, DNA-templated, and response to a xenobiotic stimulus.
The pathways in cancer and colorectal cancer were the sets of multiple pathways, including MAPK, p53, PI3K-Akt, Wnt, and Jak-STAT signaling pathways. Mitogen-activated protein kinases (MAPKs) are serine-threonine kinases that could link extracellular signals to fundamental cellular processes such as cell growth, proliferation, differentiation, migration, and apoptosis (57). The MAPK pathway was closely related to the adhesion, angiogenesis, invasion, and migration of CRC cells (58). The Janus kinase (JAK)-signal transducer of activators of transcription (STAT) pathway participated in the proliferation, invasion, and migration of tumor cells, whereas miR-198 could inhibit the proliferation and induce the apoptosis of CRC cells by repressing the JAK-STAT signal transduction (59). The phosphatidylinositol-3 kinase (PI3K)/protein kinase B (Akt) signaling pathway, an important tumor cell pathway, participated in gene transcription and translation, and it was related to the cycle, proliferation, apoptosis, and autophagy of CRC cells (60). As a cancer suppressor gene, p53 could regulate the downstream genes and take a part in DNA repair and regulation of the cell cycle and apoptosis (61). Reactivation and restoration of p53 function hold great potential for the treatment of CRC. The Wnt signaling pathway participates in the regulation of cell cycle, proliferation, and apoptosis and has been suggested as a therapeutic target for CRC treatment (62).
5 Conclusion
In conclusion, the present study demonstrated that the active components of SNL for the treatment of colon cancer include quercetin, apigenin, kaempferol, and luteolin, and the effective targets include AURKB, PIK3R1, CDK1, CDK2, GSK3B, CCNA2, CCND1, CCNB1, CASP3, CCNA2, and STAT1. SNL regulates bioprocesses such as signal transduction, response to the drug, protein phosphorylation, gene expression, and apoptotic process via signaling pathways, including pathways in cancer, the PI3K-Akt signaling pathway, cell cycle, cellular senescence, and the chemokine signaling pathway. The CETSA confirmed that apigenin and kaempferol, the active components of SNL in colon cancer treatment, were able to bind to AURKB. The present study provides a basis for the treatment of CRC by SNL from the perspective of network pharmacology. We identified the main components, targets, and signaling pathways of SNL for the treatment of colon cancer and provided new clues for the R&D of anti-CRC drugs.
Data availability statement
The datasets used and/or analyzed during the current study are available from the corresponding author on reasonable request.
Author contributions
Conception and design: JFC and BH. Administrative support: BH. Provision of study materials: JFC and BH. Collection and assembly of data: JFC, SWW, YJQ and MRD. Data analysis and interpretation: JFC. All authors contributed to the article and approved the submitted version.
Funding
This study was supported by the National Natural Science Foundation of China (grant number 82074352) and the Natural Science Foundation of Shanghai Municipality (grant number 20ZR1458700). The funders had no role in study design, data collection and analysis, the decision to publish, or the preparation of the manuscript.
Conflict of interest
The authors declare that the research was conducted in the absence of any commercial or financial relationships that could be construed as a potential conflict of interest.
Publisher’s note
All claims expressed in this article are solely those of the authors and do not necessarily represent those of their affiliated organizations, or those of the publisher, the editors and the reviewers. Any product that may be evaluated in this article, or claim that may be made by its manufacturer, is not guaranteed or endorsed by the publisher.
References
1. Sung H, Ferlay J, Siegel RL, Laversanne M, Soerjomataram I, Jemal A, et al. Global cancer statistics 2020: GLOBOCAN estimates of incidence and mortality worldwide for 36 cancers in 185 countries. CA Cancer J Clin (2021) 71(3):209–49. doi: 10.3322/caac.21660
2. Deng S, Hu B, An HM. Traditional Chinese medicinal syndromes and treatment in colorectal cancer. J Cancer Ther (2012) 3(6A):888–97. doi: 10.4236/jct.2012.326114
3. Wang Y, Liu P, Fang Y, Tian J, Li S, Xu J, et al. The effect of long-term traditional Chinese medicine treatment on survival time of colorectal cancer based on propensity score matching: A retrospective cohort study. Evid Based Complement Alternat Med (2020) 2020:7023420. doi: 10.1155/2020/7023420
4. Hu B, An HM, Shen KP, Shi XF, Deng S, Wei MM. Effect of Solanum nigrum on human colon carcinoma RKO cells. Zhong Yao Cai (2013) 36(6):958–61. doi: 10.13863/j.issn1001-4454.2013.06.034Chinese
5. Tai CJ, Wang CK, Tai CJ, Lin YF, Lin CS, Jian JY, et al. Aqueous extract of Solanum nigrum leaves induces autophagy and enhances cytotoxicity of cisplatin, doxorubicin, docetaxel, and 5-fluorouracil in human colorectal carcinoma cells. Evid Based Complement Alternat Med (2013) 2013:514719. doi: 10.1155/2013/514719
6. Ling B, Xiao S, Yang J, Wei Y, Sakharkar MK, Yang J. Probing the antitumor mechanism of Solanum nigrum l. aqueous extract against human breast cancer MCF7 cells. Bioengineering (Basel) (2019) 6(4):112. doi: 10.3390/bioengineering6040112
7. Uen WC, Lee BH, Shi YC, Wu SC, Tai CJ, Tai CJ. Inhibition of aqueous extracts of Solanum nigrum (AESN) on oral cancer through regulation of mitochondrial fission. J Tradit Complement Med (2017) 8(1):220–5. doi: 10.1016/j.jtcme.2017.05.011
8. Nawab A, Thakur VS, Yunus M, Ali Mahdi A, Gupta S. Selective cell cycle arrest and induction of apoptosis in human prostate cancer cells by a polyphenol-rich extract of Solanum nigrum. Int J Mol Med (2012) 29(2):277–84. doi: 10.3892/ijmm.2011.835
9. Li S, Zhang B. Traditional Chinese medicine network pharmacology: Theory, methodology and application. Chin J Nat Med (2013) 11(2):110–20. doi: 10.1016/S1875-5364(13)60037-0
10. Ru J, Li P, Wang J, Zhou W, Li B, Huang C, et al. TCMSP: a database of systems pharmacology for drug discovery from herbal medicines. J Cheminformatics (2014) 6:13. doi: 10.1186/1758-2946-6-13
11. Xu HY, Zhang YQ, Liu ZM, Chen T, Lv CY, Tang SH, et al. ETCM: An encyclopaedia of traditional Chinese medicine. Nucleic Acids Res (2019) 47(D1):D976–82. doi: 10.1093/nar/gky987
12. Zeng X, Zhang P, He W, Qin C, Chen S, Tao L, et al. NPASS: natural product activity and species source database for natural product research, discovery and tool development. Nucleic Acids Res (2018) 46(D1):D1217–22. doi: 10.1093/nar/gkx1026
13. Wu Y, Zhang F, Yang K, Fang S, Bu D, Li H, et al. SymMap: An integrative database of traditional Chinese medicine enhanced by symptom mapping. Nucleic Acids Res (2019) 47(D1):D1110–7. doi: 10.1093/nar/gky1021
14. Fang S, Dong L, Liu L, Guo J, Zhao L, Zhang J, et al. HERB: a high-throughput experiment- and reference-guided database of traditional Chinese medicine. Nucleic Acids Res (2021) 49(D1):D1197–206. doi: 10.1093/nar/gkaa1063
15. Keiser MJ, Roth BL, Armbruster BN, Ernsberger P, Irwin JJ, Shoichet BK. Relating protein pharmacology by ligand chemistry. Nat Biotechnol (2007) 25(2):197–206. doi: 10.1038/nbt1284
16. Szklarczyk D, Santos A, von Mering C, Jensen LJ, Bork P, Kuhn M. STITCH 5: Augmenting protein-chemical interaction networks with tissue and affinity data. Nucleic Acids Res (2016) 44(D1):D380–4. doi: 10.1093/nar/gkv1277
17. Daina A, Michielin O, Zoete V. SwissTargetPrediction: updated data and new features for efficient prediction of protein targets of small molecules. Nucleic Acids Res (2019) 47(W1):W357–64. doi: 10.1093/nar/gkz382
18. Tang Z, Kang B, Li C, Chen T, Zhang Z. GEPIA2: an enhanced web server for large-scale expression profiling and interactive analysis. Nucleic Acids Res (2019) 47(W1):W556–60. doi: 10.1093/nar/gkz430
19. Szklarczyk D, Gable AL, Lyon D, Junge A, Wyder S, Huerta-Cepas J, et al. STRING v11: Protein-protein association networks with increased coverage, supporting functional discovery in genome-wide experimental datasets. Nucleic Acids Res (2019) 47(D1):D607–13. doi: 10.1093/nar/gky1131
20. Shannon P, Markiel A, Ozier O, Baliga NS, Wang JT, Ramage D, et al. Cytoscape: a software environment for integrated models of biomolecular interaction networks. Genome Res (2003) 13(11):2498–504. doi: 10.1101/gr.1239303
21. Huang DW, Sherman BT, Lempicki RA. Systematic and integrative analysis of large gene lists using DAVID bioinformatics resources. Nat Protoc (2009) 4(1):44–57. doi: 10.1038/nprot.2008.211
22. Ashburner M, Ball CA, Blake JA, Botstein D, Butler H, Cherry JM, et al. Gene ontology: tool for the unification of biology. the gene ontology consortium. Nat Genet (2000) 25(1):25–9. doi: 10.1038/75556
23. Kanehisa M, Goto S. KEGG: kyoto encyclopedia of genes and genomes. Nucleic Acids Res (2000) 28(1):27–30. doi: 10.1093/nar/28.1.27
24. Kanehisa M. Toward understanding the origin and evolution of cellular organisms. Protein Sci (2019) 28(11):1947–51. doi: 10.1002/pro.3715
25. Kanehisa M, Furumichi M, Sato Y, Kawashima M, Ishiguro-Watanabe M. KEGG for taxonomy-based analysis of pathways and genomes. Nucleic Acids Res (2023) 51(D1):D587–92. doi: 10.1093/nar/gkac963
26. Shen W, Song Z, Zhong X, Huang M, Shen D, Gao P, et al. Sangerbox: A comprehensive, interaction-friendly clinical bioinformatics analysis platform. iMeta (2022) 1:e36. doi: 10.1002/imt2.36
27. Burley SK, Berman HM, Bhikadiya C, Bi C, Chen L, Di Costanzo L, et al. RCSB protein data bank: Biological macromolecular structures enabling research and education in fundamental biology, biomedicine, biotechnology and energy. Nucleic Acids Res (2019) 47(D1):D464–74. doi: 10.1093/nar/gky1004
28. Mooers BHM. Shortcuts for faster image creation in PyMOL. Protein Sci (2020) 29(1):268–76. doi: 10.1002/pro.3781
29. Trott O, Olson AJ. AutoDock vina: Improving the speed and accuracy of docking with a new scoring function, efficient optimization, and multithreading. J Comput Chem (2010) 31(2):455–61. doi: 10.1002/jcc.21334
30. Salentin S, Schreiber S, Haupt VJ, Adasme MF, Schroeder M. PLIP: fully automated protein-ligand interaction profiler. Nucleic Acids Res (2015) 43(W1):W443–47. doi: 10.1093/nar/gkv315
31. Martinez Molina D, Jafari R, Ignatushchenko M, Seki T, Larsson EA, Dan C, et al. Monitoring drug target engagement in cells and tissues using the cellular thermal shift assay. Science (2013) 341(6141):84–7. doi: 10.1126/science.1233606
32. Jafari R, Almqvist H, Axelsson H, Ignatushchenko M, Lundbäck T, Nordlund P, et al. The cellular thermal shift assay for evaluating drug target interactions in cells. Nat Protoc (2014) 9(9):2100–22. doi: 10.1038/nprot.2014.138
33. Tuan Anh HL, Tran PT, Thao DT, Trang DT, Dang NH, Van Cuong P, et al. Degalactotigonin, a steroidal glycoside from Solanum nigrum, induces apoptosis and cell cycle arrest via inhibiting the egfr signaling pathways in pancreatic cancer cells. BioMed Res Int (2018) 2018:3120972. doi: 10.1155/2018/3120972
34. Yan X, Li M, Chen L, Peng X, Que ZJ, An HM, et al. α−Solanine inhibits growth and metastatic potential of human colorectal cancer cells. Oncol Rep (2020) 43(5):1387–96. doi: 10.3892/or.2020.7519
35. Wang X, Zou S, Lan YL, Xing JS, Lan XQ, Zhang B. Solasonine inhibits glioma growth through anti-inflammatory pathways. Am J Transl Res (2017) 9(9):3977–989.
36. Jain R, Sharma A, Gupta S, Sarethy IP, Gabrani R. Solanum nigrum: current perspectives on therapeutic properties. Altern Med Rev (2011) 16(1):78–85.
37. Russo GL, Russo M, Spagnuolo C, Tedesco I, Bilotto S, Iannitti R, et al. Quercetin: a pleiotropic kinase inhibitor against cancer. Cancer Treat Res (2014) 159:185–205. doi: 10.1007/978-3-642-38007-5_11
38. Imran M, Aslam Gondal T, Atif M, Shahbaz M, Batool Qaisarani T, Hanif Mughal M, et al. Apigenin as an anticancer agent. Phytother Res (2020) 34(8):1812–28. doi: 10.1002/ptr.6647
39. Imran M, Salehi B, Sharifi-Rad J, Aslam Gondal T, Saeed F, Imran A, et al. Kaempferol: A key emphasis to its anticancer potential. Molecules (2019) 24(12):2277. doi: 10.3390/molecules24122277
40. Imran M, Rauf A, Abu-Izneid T, Nadeem M, Shariati MA, Khan IA, et al. Luteolin, a flavonoid, as an anticancer agent: A review. BioMed Pharmacother (2019) 112:108612. doi: 10.1016/j.biopha.2019.108612
41. Darband SG, Kaviani M, Yousefi B, Sadighparvar S, Pakdel FG, Attari JA, et al. Quercetin: A functional dietary flavonoid with potential chemo-preventive properties in colorectal cancer. J Cell Physiol (2018) 233(9):6544–60. doi: 10.1002/jcp.26595
42. Lee Y, Sung B, Kang YJ, Kim DH, Jang JY, Hwang SY, et al. Apigenin-induced apoptosis is enhanced by inhibition of autophagy formation in HCT116 human colon cancer cells. Int J Oncol (2014) 44(5):1599–606. doi: 10.3892/ijo.2014.2339
43. Choi JB, Kim JH, Lee H, Pak JN, Shim BS, Kim SH. Reactive oxygen species and p53 mediated activation of p38 and caspases is critically involved in kaempferol induced apoptosis in colorectal cancer cells. J Agric Food Chem (2018) 66(38):9960–7. doi: 10.1021/acs.jafc.8b02656
44. Yao Y, Rao C, Zheng G, Wang S. Luteolin suppresses colorectal cancer cell metastasis via regulation of the miR−384/pleiotrophin axis. Oncol Rep (2019) 42(1):131–41. doi: 10.3892/or.2019.7136
45. Lu X, Yu H, Ma Q, Shen S, Das UN. Linoleic acid suppresses colorectal cancer cell growth by inducing oxidant stress and mitochondrial dysfunction. Lipids Health Dis (2010) 9:106. doi: 10.1186/1476-511X-9-106
46. Ma H, Yu Y, Wang M, Li Z, Xu H, Tian C, et al. Correlation between microbes and colorectal cancer: Tumor apoptosis is induced by sitosterols through promoting gut microbiota to produce short-chain fatty acids. Apoptosis (2019) 24(1-2):168–83. doi: 10.1007/s10495-018-1500-9
47. Zhuang YW, Wu CE, Zhou JY, Chen X, Wu J, Jiang S, et al. Solasodine inhibits human colorectal cancer cells through suppression of the AKT/glycogen synthase kinase-3β/β-catenin pathway. Cancer Sci (2017) 108(11):2248–64. doi: 10.1111/cas.13354
48. Lee NY, Kim Y, Kim YS, Shin JH, Rubin LP, Kim Y. β-carotene exerts anti-colon cancer effects by regulating M2 macrophages and activated fibroblasts. J Nutr Biochem (2020) 82:108402. doi: 10.1016/j.jnutbio.2020.108402
49. Kasap E, Gerceker E, Boyacıoglu SÖ, Yuceyar H, Yıldırm H, Ayhan S, et al. The potential role of the NEK6, AURKA, AURKB, and PAK1 genes in adenomatous colorectal polyps and colorectal adenocarcinoma. Tumour Biol (2016) 37(3):3071–80. doi: 10.1007/s13277-015-4131-6
50. Lin J, Song T, Li C, Mao W. GSK-3β in DNA repair, apoptosis, and resistance of chemotherapy, radiotherapy of cancer. Biochim Biophys Acta Mol Cell Res (2020) 1867(5):118659. doi: 10.1016/j.bbamcr.2020.118659
51. Rosales-Reynoso MA, Zepeda-López P, Saucedo-Sariñana AM, Pineda-Razo TD, Barros-Núñez P, Gallegos-Arreola MP, et al. GSK3β polymorphisms are associated with tumor site and TNM stage in colorectal cancer. Arch Iran Med (2019) 22(8):453–60.
52. Zhou M, Liu X, Li Z, Huang Q, Li F, Li CY. Caspase-3 regulates the migration, invasion and metastasis of colon cancer cells. Int J Cancer (2018) 143(4):921–30. doi: 10.1002/ijc.31374
53. Chohan TA, Qian H, Pan Y, Chen JZ. Cyclin-dependent kinase-2 as a target for cancer therapy: Progress in the development of CDK2 inhibitors as anti-cancer agents. Curr Med Chem (2015) 22(2):237–63. doi: 10.2174/0929867321666141106113633
54. Tadesse S, Anshabo AT, Portman N, Lim E, Tilley W, Caldon CE, et al. Targeting CDK2 in cancer: challenges and opportunities for therapy. Drug Discovery Today (2020) 25(2):406–13. doi: 10.1016/j.drudis.2019.12.001
55. Chen Y, Jiang J, Zhao M, Luo X, Liang Z, Zhen Y, et al. microRNA-374a suppresses colon cancer progression by directly reducing CCND1 to inactivate the PI3K/AKT pathway. Oncotarget (2016) 7(27):41306–19. doi: 10.18632/oncotarget.9320
56. Zhang X, Li X, Tan F, Yu N, Pei H. STAT1 inhibits MiR-181a expression to suppress colorectal cancer cell proliferation through PTEN/Akt. J Cell Biochem (2017) 118(10):3435–43. doi: 10.1002/jcb.26000
57. Dhillon AS, Hagan S, Rath O, Kolch W. MAP kinase signalling pathways in cancer. Oncogene (2007) 26(22):3279–90. doi: 10.1038/sj.onc.1210421
58. Fang JY, Richardson BC. The MAPK signalling pathways and colorectal cancer. Lancet Oncol (2005) 6(5):322–7. doi: 10.1016/S1470-2045(05)70168-6
59. Li LX, Lam IH, Liang FF, Yi SP, Ye LF, Wang JT, et al. MiR-198 affects the proliferation and apoptosis of colorectal cancer through regulation of ADAM28/JAK-STAT signaling pathway. Eur Rev Med Pharmacol Sci (2019) 23(4):1487–93. doi: 10.26355/eurrev_201902_17106
60. Zhang T, Ma Y, Fang J, Liu C, Chen L. A deregulated PI3K-AKT signaling pathway in patients with colorectal cancer. J Gastrointest Cancer (2019) 50(1):35–41. doi: 10.1007/s12029-017-0024-9
61. Slattery ML, Mullany LE, Wolff RK, Sakoda LC, Samowitz WS, Herrick JS. The p53-signaling pathway and colorectal cancer: Interactions between downstream p53 target genes and miRNAs. Genomics (2019) 111(4):762–71. doi: 10.1016/j.ygeno.2018.05.006
Keywords: colon cancer, Solanum nigrum L., network pharmacology, molecular docking, compounds, gene ontology, signal pathway, bioactivity
Citation: Chen JF, Wu SW, Shi ZM, Qu YJ, Ding MR and Hu B (2023) Exploring the components and mechanism of Solanum nigrum L. for colon cancer treatment based on network pharmacology and molecular docking. Front. Oncol. 13:1111799. doi: 10.3389/fonc.2023.1111799
Received: 05 December 2022; Accepted: 17 February 2023;
Published: 08 March 2023.
Edited by:
Hang Ma, University of Rhode Island, United StatesReviewed by:
Harikrishna Reddy Rallabandi, Oklahoma Medical Research Foundation, United StatesXiaomeng Xie, China-US (Henan) Hormel Cancer Institute, China
Copyright © 2023 Chen, Wu, Shi, Qu, Ding and Hu. This is an open-access article distributed under the terms of the Creative Commons Attribution License (CC BY). The use, distribution or reproduction in other forums is permitted, provided the original author(s) and the copyright owner(s) are credited and that the original publication in this journal is cited, in accordance with accepted academic practice. No use, distribution or reproduction is permitted which does not comply with these terms.
*Correspondence: Bing Hu, beearhu@shutcm.edu.cn