- 1Department of Health Science, Doctoral School of Health Science, University of Pécs, Pécs, Hungary
- 2Department of Public Health Medicine, Doctoral School of Clinical Medicine, University of Pécs Medical School, Pécs, Hungary
- 3Department of Clinical Epidemiology, the Fourth Affiliated Hospital of China Medical University, Shenyang, China
- 4Faculty of Engineering and Information Technology, University of Pécs, Pécs, Hungary
- 5Department of Health Statistics, School of Public Health, China Medical University, Shenyang, China
Background: Single nucleotide polymorphisms (SNPs) interfere with the function of certain genes and thus may influence the probability of skin cancer. The correlation between SNPs and skin cancer (SC) lacks statistical power, however. Therefore, the purpose of this study was to identify the gene polymorphisms involved in skin cancer susceptibility using network meta-analysis and to determine the relationship between SNPs and SC risk.
Methods: PubMed, Embase, and Web of Science were searched for articles including “SNP” and different types of SC as keywords between January 2005 and May 2022. The Newcastle-Ottawa Scale was used to assess bias judgments. The odds ratio (ORs) and their 95% confidence intervals (CIs) were determined to estimate heterogeneity within and between studies. Meta-analysis and network meta-analysis were carried out to identify the SNPs associated with SC. The P-score of each SNP was compared to obtain the rank of probability. Subgroup analyses were performed by cancer type.
Results: A total of 275 SNPs from 59 studies were included in the study. Two subgroup SNP networks using the allele model and dominant model were analyzed. The alternative alleles of rs2228570 (FokI) and rs13181 (ERCC2) were the first-ranking SNPs in both subgroups one and two of the allele model, respectively. The homozygous dominant genotype and heterozygous genotype of rs475007 in subgroup one and the homozygous recessive genotype of rs238406 in subgroup two were most likely to be associated with skin cancer based on the dominant model.
Conclusions: According to the allele model, SNPs FokI rs2228570 and ERCC2 rs13181 and, according to the dominant model, SNPs MMP1 rs475007 and ERCC2 rs238406 are closely linked to SC risk.
1 Introduction
The incidence of skin cancer has increased significantly since the 1970s, mainly due to lifestyle changes, including sun-seeking behavior and the thinning of the ozone layer (1). Skin cancers include cutaneous melanoma (CM) and nonmelanoma skin cancer (NMSC), with growing incidence rates for both cancer types (2). They are all caused by the abnormal growth of skin cells, especially those exposed to the sun. Non-melanoma skin cancer is the most common cancer among white-skinned people, and thus it is a significant cause of morbidity (3). Melanoma is less common; however, its prognosis is poorer, resulting in higher mortality rates (4). Approximately 1.2 million new NMSC (4) and nearly 300,000 new CM cases were diagnosed worldwide with 57,043 deaths from CM in 2020 (4). Timely diagnosis is crucial for reducing mortality from malignant melanoma and also has additional health and economic benefits (5). Since early detection of skin cancer is often limited, identifying suitable markers for its detection is of the utmost importance (6). Therefore, certain new genetic loci were investigated as possible markers for identifying SC risk (7).
Single nucleotide polymorphisms (SNPs) are genetic variations caused by point mutations. The allelic distribution of SNPs may interfere with the function of genes and then influence the probability of certain diseases (8, 9), which has led to SNPs being investigated as possible biological markers. Various SNPs have been shown to be associated with pigmentation, nevi, hair, skin color, and skin cancer. The SNPs of the BRAF and NRAS genes, for example, have been found to be commonly mutated oncogenes in CM (10). Furthermore, similarly to the interactions between genetics and the environment, the number and frequency of SNPs also affect the characteristics of their related genes as well as the development of their related disease phenotypes (11, 12).
Network meta-analysis (NMA), and in particular, Bayesian network meta-analysis, analyzes the direct and indirect evidence from multiple comparisons of tests within and between studies (13), making it possible to investigate the interactions between multiple comparisons of SNP tests.
Therefore, the aim of our study was to identify and compare the single nucleotide polymorphisms predominantly involved in skin cancer susceptibility by conducting a network meta-analysis.
2 Method
2.1 Search strategy and selection criteria
We searched the PubMed, Embase, and Web of Science electronic databases from their starting dates to May 2022 to identify relevant studies. The search strategy is shown in detail in Supplementary Presentation 1. We required the articles to include the following keywords: case–control, single nucleotide polymorphism (SNP), and study skin cancer (SC), cutaneous melanoma (CM), non-melanoma (NM), squamous cell carcinoma (SCC), or basal cell carcinoma (BCC). Inclusion and exclusion criteria are presented in Supplementary Presentation 2. The study was designed and performed in accordance with the PRISMA guidelines (Figure 1).
2.2 Data abstraction and bias assessment
Two researchers (LZ and YS) independently screened the titles and abstracts of the search results and extracted the following information from the included articles: authors’ names, year of publication, population of country and ethnicity, genotyping method, case and control numbers, control’s source, case–control match, cancer type, gene, SNP, and allele frequency.
Subsequently, we applied the Newcastle-Ottawa Scale (NOS) score for case-control studies to evaluate the quality and risk of bias of the included studies (Figure 2) (14). According to the NOS, article quality is assessed through eight questions from the Selection dimension (case definition, case selection, control definition, and control selection), the Comparability dimension (comparability of cases and controls), and the Exposure dimension (exposure ascertainment, case and control ascertainment, and non-response rate). Excepting “Comparability” with two stars, other items can each be given one star. Hence, a study can be awarded a maximum of nine stars and will be excluded if it receives fewer than five stars. Discrepancies were resolved by consensus between the reviewing authors.
2.3 Data synthesis and statistical analysis
Alleles are represented differently in different genomes. Therefore, for clarity, all reference alleles were represented by “A” in this study, and the corresponding alternative alleles were represented by “B.” Hence, for genotype, “AA” meant the homozygous dominant genotype, “AB” meant the heterozygote genotype, and “BB” meant the homozygous recessive genotype. The allele model (A vs. B) was employed to explore dominance. Furthermore, the dominant model (AA + AB vs. BB) and the recessive model (AA vs. AB + BB) were used for investigating the association between different genotypes and phenotypes (15).
A bivariate random effect model was performed for the meta-analysis of the comparative studies. Odds ratios (ORs) and their 95% confidence intervals (CIs) were used for estimating heterogeneity within and between studies. Then, pooled sensitivity (Se), specificity (Sp), positive likelihood ratio (LR), negative likelihood ratio (LR), diagnostic OR (DOR), and area under the summary receiver operating characteristic curve (AUROC) were calculated for each genotyping. The pooled AUROC was used as an indicator for testing to examine the diagnostic accuracy of each genotyping. The statistical heterogeneity between each study was assessed using the inconsistency index I-square. Additionally, meta-regression analysis was performed based on cancer type to assess heterogeneity. Cancer types included: CM, NMSC, and SC (including both CM and NMSC).
Next, Bayesian network meta-analysis (NMA) was used to clarify the relationships between the SNPs and skin cancer according to the allele model (A vs. B) and the dominant model (AA + AB vs. BB). The fixed-effects model that had four chains, 1,000 burn-ins, 200,000 iterations, and a thinning interval of 10 was selected for the MCMC simulation (16). The Gelman–Rubin plot and potential scale reduction factor (PSRF) were used for assessing convergence. Net splitting was carried out to check the consistency of the networks, and the effect estimate table was employed for estimating all SNP comparisons. Then, the overall ranks of SNPs were estimated by P-scores that were equivalent to the surface under the cumulative ranking curve (SUCRA) (17). SNPs with the highest P-scores were the most related to skin cancer.
P <0.05 was statistically significant. RStudio software and StataSE 16.0 software were used for calculations and plotting. The software packages used in the study are listed in Supplementary Presentation 2.
3 Results
3.1 Literature search results
The literature search initially identified 3,575 studies from PubMed, Embase, and Web of Science. The search ended on 2 May 2022. As Figure 1 shows, we recorded screening 3,387 studies based on titles and abstracts, and 368 studies were obtained for full-text screening. At last, 59 studies met the inclusion criteria and were included in our network meta-analysis after being excluded from 232 full-text manuscripts due to background, unusable data, and bias reasons. One article was excluded due to bias, as explained below.
3.2 Characteristics and bias of enrolled studies
Table 1 summarizes the main characteristics of 60 studies that were published between 2005 and 2022. Studies investigating Caucasian or Mongoloid ethnicities were included. Figure 2 shows the quality assessment of enrolled studies using the NOS risk bias tool. Any studies with NOS scores lower than five stars were excluded. Finally, 59 articles were included in the systematic review and meta-analysis.
3.3 Pairwise meta-analysis
A direct meta-analysis was performed to determine the correlation between 275 SNPs and SC risk (Supplementary Table 1). A total of 72 SNPs from 47 studies were closely associated with SC in the studies using the allele model (A vs. B), while a significant association was found for 52 SNPs from 31 studies using the dominant model (AA + AB vs. BB). Furthermore, based on the recessive model (AA vs. AB + BB), 77 SNPs from 35 studies were related to SC. As depicted in Supplementary Image 1, the detected SNPs were analyzed further for diagnostic accuracy.
Table 2 shows the evaluation of the diagnostic performance of the pooled SNPs for SC. According to SUCRA, the allele model can be employed for exploring dominance. Then, we chose the dominant model as the genotyping model for diagnosing SC.
3.4 The allele model (A vs. B)
The associations between the 72 SNPs and SC susceptibility are shown in Supplementary Table 2. In the allele model, the reference alleles of rs16891982 (G vs. C, combined OR [cOR] = 2.74, 95% CI [2.20, 3.40]), rs885479 (G vs. A, cOR = 1.46, 95% CI [1.06, 2.01]), rs1544410 (G vs. A, cOR = 1.19, 95% CI [1.06, 1.34]), rs731236 (T vs. C, cOR = 1.11, 95% CI [1.00, 1.23]), and the alternative alleles of rs25487 (G vs. A, cOR = 0.92, 95% CI [0.85, 0.99]), rs4911414 (G vs. T, cOR = 0.85, 95% CI [0.75, 0.96]), rs1695 (W vs. M, cOR = 0.79, 95% CI [0.65, 0.95]), and rs2228570 (wild-type allele vs. mutant allele, cOR = 0.79, 95% CI [0.71, 0.88]) were related significantly to SC in at least two of the studies. The pooled P-value for all SNPs was less than 0.05.
3.5 The dominant model (AA + AB vs. BB)
Supplementary Table 3 summarizes the 52 SNPs’ cOR for SC according to the dominant model. The results show that those who were homozygous dominant and heterozygous genotypes: rs16891982 (GG + GC vs. CC, cOR = 3.72, 95% CI [1.66, 8.35]), rs494379 (TT + TC vs. CC, cOR = 2.62, 95% CI [1.96, 3.49]), rs514921 (AA + AG vs. GG, cOR = 2.14, 95% CI [1.67, 2.75]), rs1144393 (AA + AG vs. GG, cOR = 1.48, 95% CI [1.19, 1.84]), rs11615 (AA + AG vs. GG, cOR = 1.41, 95% CI [1.02, 1.95]), and rs498186 (TT + TG vs. GG, cOR = 1.35, 95% CI [1.10, 1.65]) had a higher risk for developing SC, than those who were homozygous recessive genotypes. In contrast, individuals with the homozygous recessive genotypes of rs25487 (GG + GA vs. AA, cOR = 0.85, 95% CI [0.72, 1.00]) and rs1805007 (CC + CT vs. TT, cOR = 0.42, 95% CI [0.19, 0.91]) were significantly associated with increased susceptibility to SC.
3.6 Subgroup analysis
A covariate regression analysis was performed for each of the three genotypes. The results showed that there was no statistical difference (all P-values > 0.05) among CM, NMSC, and SC (including both CM and NMSC) (Table 3).
3.7 Network evidence
3.7.1 The allele model
The network plot depicts the rough comparison of each pair of SNPs (Figure 3). A node indicates an SNP, and its size represents the number of studies. The connections between the nodes represent a pair of comparisons, and their thickness represents the number of direct comparisons. As is evident from Figure 3A, there were three subgroups without any connections. Also, to avoid redundancy, the network of SNPs from one study was deleted from our study. Thus, the NMA of the allele model was divided into two groups: subgroup one (including rs1544410, rs2228570, and rs731236), and subgroup two (including rs1042522, rs1136410, rs11615, rs13181, rs1695, rs1799793, rs1805006, rs1805007, rs1805008, rs25487, rs25489, rs4911414, and rs885479) (Figure 3B).
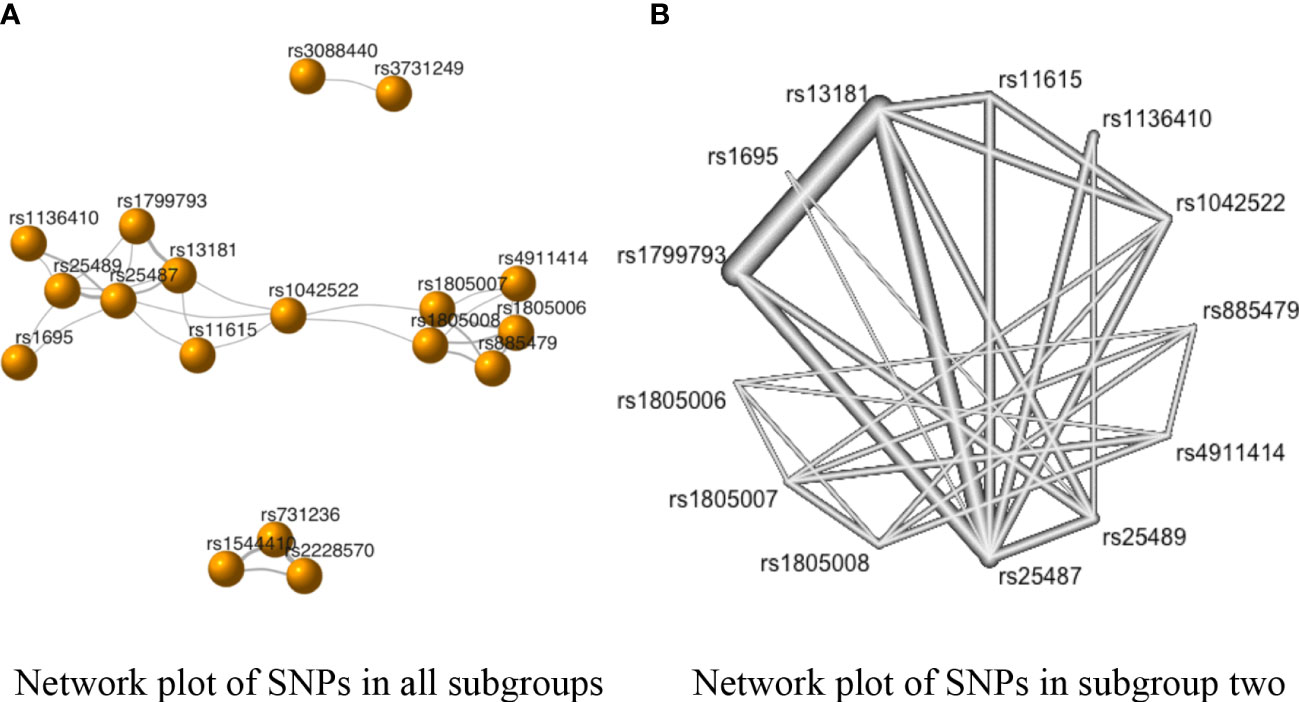
Figure 3 The network evidence plots of single nucleotide polymorphisms (SNPs) in the allele model (A vs. B). (A) Network plot of SNPs in all subgroups in the allele model; (B) Network plot of SNPs in subgroup two of the allele model.
The SNPs rs731236 vs. rs2228570 had the strongest negative correlation with SC risk in subgroup one (standardized mean differences (SMD) of OR = −0.08, 95% CI [−0.18, 0.02]) (Supplementary Table 4). However, the P-values of the correlations between the SNPs in subgroup one were above 0.05 (Supplementary Table 4). Similarly, as shown in Figure 3 and Supplementary Table 4, the comparison with the highest direct pooled effect size in subgroup two was rs4911414 vs rs1805006 (SMD of OR = −2.94, 95% CI [−2.48, −3.40]), followed by comparison rs13181 vs. rs25489 (SMD of OR = −2.35, 95% CI [−2.54, −2.16]).
Additionally, in subgroup two, the direct and indirect evidence showed negative correlations in the comparisons of rs1042522 vs. rs25487, rs1136410 vs. rs25489, rs11615 vs. rs13181, rs11615 vs. rs25487, rs13181 vs. rs1799793, rs13181 vs. rs25487, rs1805007 vs. rs1805006, and rs1805007 vs. rs885479 (Table 2 in Supplementary Table 4). However, since the indirect evidence proportion of each comparison (i.e., the mean path length of each estimated comparison) was less than 2 (78), each of the above-mentioned comparisons followed the direction of direct evidence (Supplementary Image 2).
To select the SNPs with the highest chance of a significant association with skin cancer, the P-scores were ranked, as shown in Table 4. The SNP rs2228570 (P-score = 0.85) ranked first in subgroup one in the allele model, and the SNP rs13181 had the highest P-score in subgroup two (P-score = 0.94).
3.7.2 The dominant model
In Figure 4A, only two subgroups met the requirements for the NMA. Subgroup one included rs1051121, rs11225426, rs1144393, rs1729376, rs2071230, rs2071231, rs3213460, rs470215, rs470358, rs475007, rs491152, rs494379, rs498186, rs5031036, rs514921, rs71250626, rs7945189, and rs996999 (Figure 4B), while subgroup two included rs1051740, rs11615, rs2228001, rs238406, rs25487, rs25489, rs3212948, and rs3212950 (Figure 4C).
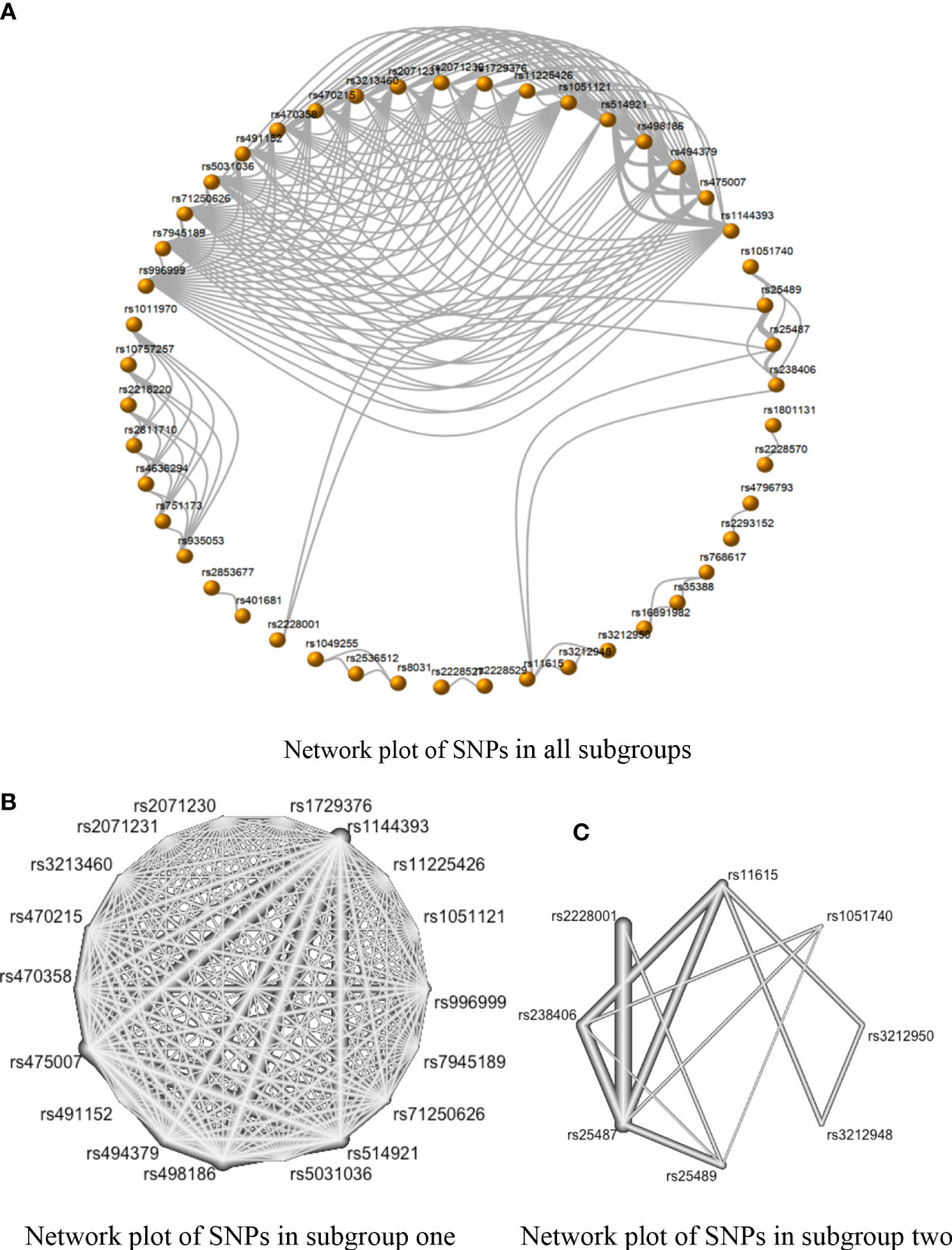
Figure 4 The network evidence plots of single nucleotide polymorphisms (SNPs) in the dominant model (AA + AB vs. BB). (A) Network map with 47 SNPs of all subgroups in the dominant model; (B) Network map of subgroup one with 18 SNPs in the dominant model; and (C) Network map of Subgroup two with eight SNPs in the dominant model.
There was no inconsistency between the direct and indirect evidence in Group 1. The strongest positive correlations in this subgroup were the comparison of rs475007 vs. rs1729376 and the comparison of rs475007 vs. rs2071231 (both SMDs of network OR = 4.23, 95% CI [2.19, 6.25]). These were followed by the rs475007 vs rs491152 comparison, which SNPs were negatively correlated with SC risk (SMD of network OR = −4.21, 95% CI [−6.24, −2.18]). The comparison of rs494379 and rs514921 showed the strongest indirect correlation (SMD of indirect OR = 11.52, 95% CI [−9.40, 32.44]) (Supplementary Image 3–5).
Inconsistencies between direct and indirect evidence were found in the comparison of rs2228001 vs. rs25487 and the comparison of rs2228001 vs. rs25489 in subgroup two (Figure 4 in Figure 5). Both rs25487 and rs25489 were negatively correlated with rs2228001 after performing network analysis (Figure 5). Figure 5 also demonstrates that the rs238406 vs. rs25489 comparison had the strongest association (SMD of network OR = −2.17, 95% CI [−2.72, −1.61]).
As shown in Table 5, rs475007 has the highest P-score (0.97) in subgroup one, and rs238406 has the highest P-score (0.97) in subgroup two. Therefore, the top five SNPs most likely associated with skin cancer are, in descending order, in subgroup one: rs475007, rs470358, rs498186, rs1144393, and rs470215, and in subgroup two: rs238406, rs2228001, rs25487, rs11615, and rs3212950.
4 Discussion
Based on direct comparisons from pairwise meta-analysis and added indirect comparisons, our study employed network meta-analysis to compare the associations between single-nucleotide polymorphisms and skin cancer using the allele model and the dominant model. Our network meta-analysis identified two subgroups in each genetic model, respectively. We ranked SNPs based on their P-scores to select the most appropriate SNPs. Our results showed that the minor alleles (T) of rs2228570 (FokI) and (C) of rs13181 (ERCC2) were the highest-ranking SNPs in both subgroups one and two of the allele model. On the other hand, using the dominant model, the homozygous dominant genotype and heterozygote genotype (AA + AT) of rs475007 in subgroup one and the homozygous dominant genotype (AA) of rs238406 in subgroup two were most likely to be associated with skin cancer.
The single-nucleotide polymorphism rs2228570 (FokI) is in the vitamin D receptor (VDR) gene. It is one of the common human VDR SNPs along with rs1544410 (BsmI), rs7975232 (ApaI), and rs731236 (TaqI). Vitamin D is metabolized to vitamin D: 1,25(OH)2D3.1 in response to ultraviolet B (UVB) radiation. This metabolite is the ligand of the VDR, which in turn initiates a series of biological responses in bone metabolism, immunity, cell proliferation, and differentiation by binding to vitamin D response elements in DNA (79). Hence, rs2228570 has not only been associated with various skin diseases, such as chronic spontaneous urticaria (CSU) (80), atopic dermatitis (AD) (81), and leprosy (82), but has also been linked to an increased incidence risk and worse prognosis of different cancers, such as breast cancer (83), ovarian cancer (84), gastric cancer (85), hepatocellular carcinoma (86), papillary thyroid cancer (87), pancreatic cancer (88), and melanoma. Our results are consistent with previous studies using assay methods (89). For instance, the study results of Zeljic et al., who used the assay method, showed that the homozygous recessive genotype of rs2228570 was related to increased melanoma risk compared to the homozygous dominant genotype in the Caucasian population (89). However, no association was observed between rs2228570 and melanoma in this investigation using the biosystem assay method (90).
SNPs rs13181 and rs238406 ranked first and second in subgroups two in both the allele and dominant models. Both SNPs are in the ERCC2 (formerly called XPD) gene. The ERCC2 polymorphisms have ATP-dependent DNA helicase activity, which may impact DNA repair functions. Deficiency of ERCC2 has been reported to lead to xeroderma pigmentosum (XP), trichothiodystrophy (TTD), and Cockayne’s syndrome (CS) (91). This observation may explain why rs13181 and rs238406 were found to be linked to cancers such as lung cancer (92), cervical cancer (93), breast cancer, squamous cell carcinomas of the head and neck (94), and bladder cancer (95). In line with these findings, our results showed that the alternative allele (C) of rs13181 and the homozygous recessive genotype (AA) of rs238406 were significantly associated with SC risk. The study by Kertatbs et al. reported a high frequency of the wild-type allele of rs13181 in advanced melanoma (96). However, an investigation using the microarray chip method, including 1,391 NMSC cases and 2,586 cancer-free controls, did not find significantly increased risks of NMSC for the homozygous dominant genotype of rs13181 (97). Furthermore, a meta-analysis found that the homozygous recessive genotype (AA) of rs238406 was positively associated with an increased risk of cancer of the nervous system, the digestive tract, the genito-urinary system, and the respiratory system, but not basal cell cancer (98).
Matrix metalloproteinases (MMPs) are a family of proteolytic enzymes that are involved in cell mobility, proliferation, differentiation, and apoptosis by degrading extracellular proteins (99). MMP1, a secreted enzyme that cleaves fibrillar collagen, has been linked to cancer by promoting cancer cell proliferation, tumor angiogenesis, and vasculogenesis (100). In the dominant model of our research, all the SNPs in subgroup one were located in the MMP1 gene. The SNP most likely to be associated with SC was rs475007. Furthermore, the homozygous recessive genotype of rs475007 was found to decrease the risk of skin cancer. Similar results were found in Liu’s study, which reported that patients with homozygous dominant genotype and heterozygote genotype for the reference allele of rs475007 were more likely to have larger skin tumors (101).
5 Limitation
Due to technical differences and differences in sensitivity, our analysis only included studies that used the PCR genotypic detection method and excluded microarray detection or genome-wide association studies (GWAS). However, GWAS allows for much larger sample sizes than PCR studies. Additionally, due to the limitations of the RStudio and StataSE software and the complexity of multi-arm studies, SNPs only reported in one single article were not included in the final network meta-analysis.
6 Future prospective
Our article indicated that people with mutations in the genes FokI (rs2228570), ERCC2 (rs13181), MMP1 (rs475007), and ERCC2 (rs238406) were more likely to have skin cancer. Dysplastic nevi (also called atypical moles) are precursors and risk factors for malignant melanoma (102). However, it is difficult to distinguish them from melanomas because of overlapping features and a lack of predictive markers (103). Thus, our results may provide a possibility for the early detection of asymptomatic skin cancer if routine genetic screening is implemented in the general population in the future. Additionally, the results of our study may also provide valuable information for decision-making when determining the best mode of therapy for SC in a patient. For instance, since FokI is a vitamin D receptor gene and vitamin D is considered to be a protective factor in certain cancers, such as skin cancer (104, 105), supplementation with vitamin D may be used as adjuvant therapy in cancer patients. Therefore, identification of SC patients with FokI gene (rs2228570) mutations is important, since these patients would not benefit from adjuvant Vitamin D therapy.
In addition, we obtained direct and indirect evidence between the SNP pairs through network analysis, which proposed the possibility of hitherto unexplored relationships between certain gene mutations. For example, ERCC2 gene mutations have been shown to indirectly increase the risk of SC (106, 107), and the Melanocortin receptor 1 (MC1R), which encodes melanocyte-stimulating hormone (MSH) receptors, has also been shown to be a risk factor for skin cancer (108). However, surprisingly, indirect evidence from our network meta-analysis showed that ERCC2 (rs13181) was negatively related to MC1R (1805006, 1805007, 1805008, and rs885479) (Supplementary Table 4). Therefore, the relationship between ERCC2 and MC1R necessitates further research to determine their roles in SC development.
Finally, as an added scientific value, we applied an innovative research design by performing a network analysis of case–control studies, thus providing a fresh perspective on the NMA method. Our analysis implies that all studies involving genetically-related diseases, whether cohort or case–control studies, can be used to build a network in the meta-analysis, which may then provide valuable information for the diseases’ early detection, diagnosis, staging, treatment, and prognosis.
Data availability statement
The raw data supporting the conclusions of this article will be made available by the authors, without undue reservation.
Author contributions
Conceptualization: LZ, HL, and IK. Methodology: LZ and HL. Data curation: LZ, YS, HA, and JZ. Statistical analysis: LZ and HL. Writing (original draft preparation): LZ and IK. Review and editing: ÉP, JM, YS, HA, JZ, HL, and IK. Visualization: ZL. Supervision: HL and IK. Project administration: LZ and IK. All authors listed have made a substantial, direct, and intellectual contribution to the work and approved it for publication.
Funding
This research did not receive any specific grant from funding agencies in the public, commercial, or not-for-profit sectors. But the Stipendium Hungaricum and China Scholarship Council (CSC) funded the author’s doctorate.
Acknowledgments
We would also like to show our huge thanks to Qimeng Zhang for her guidance in methodology and analysis software code that greatly improved the analysis method.
Conflict of interest
The authors declare that the research was conducted in the absence of any commercial or financial relationships that could be construed as a potential conflict of interest.
Publisher’s note
All claims expressed in this article are solely those of the authors and do not necessarily represent those of their affiliated organizations, or those of the publisher, the editors and the reviewers. Any product that may be evaluated in this article, or claim that may be made by its manufacturer, is not guaranteed or endorsed by the publisher.
Supplementary material
The Supplementary Material for this article can be found online at: https://www.frontiersin.org/articles/10.3389/fonc.2023.1094309/full#supplementary-material
References
1. Helping people reduce their risks of skin cancer and cataract. Available at: https://www.who.int/news/item/22-07-2002-helping-people-reduce-their-risks-of-skin-cancer-and-cataract (Accessed August 28 2022).
2. Leiter U, Eigentler T, Garbe C. “Epidemiology of skin cancer”. In: Reichrath J, editor. Sunlight, vitamin d and skin cancer. New York, NY: Springer (2014). p. 120–40. doi: 10.1007/978-1-4939-0437-2_7
3. Skin cancer statistics (2018). World Cancer Research Fund. Available at: https://www.wcrf.org/dietandcancer/cancer-trends/skin-cancer-statistics (Accessed January 6, 2020).
4. Cancer (IARC) TIA for r on . Global Cancer Observatory. Available at: https://gco.iarc.fr/ (Accessed August 28, 2022).
5. Kornek T, Augustin M. Skin cancer prevention. JDDG: J der Deutschen Dermatolog Gesellschaft (2013) 11:283–98. doi: 10.1111/ddg.12066
6. Najmi M, Brown AE, Harrington SR, Farris D, Sepulveda S, Nelson KC. A systematic review and synthesis of qualitative and quantitative studies evaluating provider, patient, and health care system-related barriers to diagnostic skin cancer examinations. Arch Dermatol Res (2022) 314:329–40. doi: 10.1007/s00403-021-02224-z
7. Carrera C, Marghoob AA. Discriminating nevi from melanomas: Clues and pitfalls. Dermatol Clin (2016) 34:395–409. doi: 10.1016/j.det.2016.05.003
8. Park J-H, Gail MH, Weinberg CR, Carroll RJ, Chung CC, Wang Z, et al. Distribution of allele frequencies and effect sizes and their interrelationships for common genetic susceptibility variants. Proc Natl Acad Sci (2011) 108:18026–31. doi: 10.1073/pnas.1114759108
9. Myles S, Davison D, Barrett J, Stoneking M, Timpson N. Worldwide population differentiation at disease-associated SNPs. BMC Med Genomics (2008) 1:22. doi: 10.1186/1755-8794-1-22
10. Thomas NE, Edmiston SN, Orlow I, Kanetsky PA, Luo L, Gibbs DC, et al. Inherited genetic variants associated with melanoma BRAF/NRAS subtypes. J Invest Dermatol (2018) 138:2398–404. doi: 10.1016/j.jid.2018.04.025
11. Lee K-Y, Leung K-S, Ma SL, So HC, Huang D, Tang NL-S, et al. Genome-wide search for SNP interactions in GWAS data: Algorithm, feasibility, replication using schizophrenia datasets. Front Genet (2020) 11:1003. doi: 10.3389/fgene.2020.01003
12. Morris RW, Kaplan NL. On the advantage of haplotype analysis in the presence of multiple disease susceptibility alleles. Genet Epidemiol (2002) 23:221–33. doi: 10.1002/gepi.10200
13. Mills EJ, Thorlund K, Ioannidis JPA. Demystifying trial networks and network meta-analysis. BMJ (2013) 346:f2914. doi: 10.1136/bmj.f2914
14. Ottawa Hospital Research Institute. Available at: http://www.ohri.ca/programs/clinical_epidemiology/oxford.asp (Accessed April 26, 2022)
15. Zhao F, Song M, Wang Y, Wang W. Genetic model. J Cell Mol Med (2016) 20:765. doi: 10.1111/jcmm.12751
16. Hu D, O’Connor AM, Wang C, Sargeant JM, Winder CB. How to conduct a Bayesian network meta-analysis. Front Veterin Sci (2020) 7:271. doi: 10.3389/fvets.2020.00271
17. Rücker G, Schwarzer G. Ranking treatments in frequentist network meta-analysis works without resampling methods. BMC Med Res Method (2015) 15:58. doi: 10.1186/s12874-015-0060-8
18. Jannot A-S, Meziani R, Bertrand G, Gérard B, Descamps V, Archimbaud A, et al. Allele variations in the OCA2 gene (pink-eyed-dilution locus) are associated with genetic susceptibility to melanoma. Eur J Hum Genet (2005) 13:913–20. doi: 10.1038/sj.ejhg.5201415
19. Vogel U, Olsen A, Wallin H, Overvad K, Tjønneland A, Nexø BA. Effect of polymorphisms in XPD, RAI, ASE-1 and ERCC1 on the risk of basal cell carcinoma among caucasians after age 50. Cancer Detect Prev (2005) 29:209–14. doi: 10.1016/j.cdp.2005.01.001
20. Li C, Hu Z, Liu Z, Wang L-E, Strom SS, Gershenwald JE, et al. Polymorphisms in the DNA repair genes XPC, XPD, and XPG and risk of cutaneous melanoma: A case-control analysis. Cancer Epidemiol Biomarkers Prev (2006) 15:2526–32. doi: 10.1158/1055-9965.EPI-06-0672
21. Li C, Liu Z, Wang L-E, Strom SS, Lee JE, Gershenwald JE, et al. Genetic variants of the ADPRT, XRCC1 and APE1 genes and risk of cutaneous melanoma. Carcinogenesis (2006) 27:1894–901. doi: 10.1093/carcin/bgl042
22. Wilkening S, Hemminki K, Rudnai P, Gurzau E, Koppova K, Försti A, et al. No association between MDM2 SNP309 promoter polymorphism and basal cell carcinoma of the skin. Br J Dermatol (2007) 157:375–7. doi: 10.1111/j.1365-2133.2007.07994.x
23. Meyer P, Stapelmann H, Frank B, Varon R, Burwinkel B, Schmitt C, et al. Molecular genetic analysis of NBS1 in German melanoma patients. Melanoma Res (2007) 17:109–16. doi: 10.1097/CMR.0b013e3280dec638
24. Applebaum KM, Karagas MR, Hunter DJ, Catalano PJ, Byler SH, Morris S, et al. Polymorphisms in nucleotide excision repair genes, arsenic exposure, and non-melanoma skin cancer in new Hampshire. Environ Health Perspect (2007) 115:1231. doi: 10.1289/ehp.10096
25. Povey JE, Darakhshan F, Robertson K, Bisset Y, Mekky M, Rees J, et al. DNA Repair gene polymorphisms and genetic predisposition to cutaneous melanoma. Carcinogenesis (2007) 28:1087–93. doi: 10.1093/carcin/bgl257
26. Pjanova D, Engele L, Randerson-Moor JA, Harland M, Bishop DT, Newton Bishop JA, et al. CDKN2A and CDK4 variants in Latvian melanoma patients: Analysis of a clinic-based population. Melanoma Res (2007) 17:185–91. doi: 10.1097/CMR.0b013e328014a2cd
27. Li C, Hu Z, Liu Z, Wang L-E, Gershenwald JE, Lee JE, et al. Polymorphisms of the neuronal and inducible nitric oxide synthase genes and the risk of cutaneous melanoma. Cancer (2007) 109:1570–8. doi: 10.1002/cncr.22582
28. Li C, Liu Z, Wang LE, Gershenwald JE, Lee JE, Prieto VG, et al. Haplotype and genotypes of the VDR gene and cutaneous melanoma risk in non-Hispanic whites in Texas: A case–control study. Int J Cancer (2008) 122:2077–84. doi: 10.1002/ijc.23357
29. Fernandez LP, Milne RL, Pita G, Avilés JA, Lázaro P, Benítez J, et al. SLC45A2: a novel malignant melanoma-associated gene. Hum Mutat (2008) 29:1161–7. doi: 10.1002/humu.20804
30. Guedj M, Bourillon A, Combadières C, Rodero M, Dieudé P, Descamps V, et al. Variants of the MATP/SLC45A2 gene are protective for melanoma in the French population. Hum Mutat (2008) 29:1154–60. doi: 10.1002/humu.20823
31. Nan H, Qureshi AA, Hunter DJ, Han J. A functional SNP in the MDM2 promoter, pigmentary phenotypes, and risk of skin cancer. Cancer Causes Control (2009) 20:171–9. doi: 10.1007/s10552-008-9231-9
32. Schoof N, von Bonin F, König IR, Mössner R, Krüger U, Reich K, et al. Distal and proximal interleukin (IL)-10 promoter polymorphisms associated with risk of cutaneous melanoma development: A case–control study. Genes Immun (2009) 10:586–90. doi: 10.1038/gene.2009.40
33. Figl A, Scherer D, Nagore E, Bermejo JL, Botella-Estrada R, Gast A, et al. Single-nucleotide polymorphisms in DNA-repair genes and cutaneous melanoma. Mutat Res/Gen Toxicol Environ Mutagen (2010) 702:8–16. doi: 10.1016/j.mrgentox.2010.06.011
34. Capasso M, Ayala F, Avvisati RA, Russo R, Gambale A, Mozzillo N, et al. MDM2 SNP309 and p53 Arg72Pro in cutaneous melanoma: Association between SNP309 GG genotype and tumor breslow thickness. J Hum Genet (2010) 55:518–24. doi: 10.1038/jhg.2010.62
35. Debniak T, Jakubowska A, Serrano-Fernández P, Kurzawski G, Cybulski C, Chauhan SR, et al. Association of MMP8 gene variation with an increased risk of malignant melanoma. Melanoma Res (2011) 21:464–8. doi: 10.1097/CMR.0b013e3283485fdd
36. Rizzato C, Canzian F, Rudnai P, Gurzau E, Stein A, Koppova K, et al. Interaction between functional polymorphic variants in cytokine genes, established risk factors and susceptibility to basal cell carcinoma of skin. Carcinogenesis (2011) 32:1849–54. doi: 10.1093/carcin/bgr197
37. Rizzato C, Scherer D, Rudnai P, Gurzau E, Koppova K, Hemminki K, et al. POMC and TP53 genetic variability and risk of basal cell carcinoma of skin: Interaction between host and genetic factors. J Dermatol Sci (2011) 63:47–54. doi: 10.1016/j.jdermsci.2011.03.006
38. Lesiak A, Norval M, Wodz-Naskiewicz K, Pawliczak R, Rogowski-Tylman M, Sysa-Jedrzejowska A, et al. An enhanced risk of basal cell carcinoma is associated with particular polymorphisms in the VDR and MTHFR genes. Exp Dermatol (2011) 20:800–4. doi: 10.1111/j.1600-0625.2011.01328.x
39. Wang L-E, Huang Y, Yin M, Gershenwald JE, Prieto VG, Lee JE, et al. Promoter polymorphisms in matrix metallopeptidase 1 and risk of cutaneous melanoma. Eur J Cancer (Oxford England : 1990) (2011) 47:107. doi: 10.1016/j.ejca.2010.06.129
40. Almquist LM, Karagas MR, Christensen BC, Welsh MM, Perry AE, Storm CA, et al. The role of TP53 and MDM2 polymorphisms in TP53 mutagenesis and risk of non-melanoma skin cancer. Carcinogenesis (2011) 32:327–30. doi: 10.1093/carcin/bgq256
41. Ibarrola-Villava M, Martin-Gonzalez M, Lazaro P, Pizarro A, Lluch A, Ribas G. Role of glutathione s-transferases in melanoma susceptibility: Association with GSTP1 rs1695 polymorphism. Br J Dermatol (2012) 166:1176–83. doi: 10.1111/j.1365-2133.2012.10831.x
42. Helsing P, Nymoen DA, Rootwelt H, Vårdal M, Akslen LA, Molven A, et al. MC1R, ASIP, TYR, and TYRP1 gene variants in a population-based series of multiple primary melanomas. Genes Chromosomes Cancer (2012) 51:654–61. doi: 10.1002/gcc.21952
43. Santonocito C, Scapaticci M, Penitente R, Paradisi A, Capizzi R, Lanza-Silveri S, et al. Polymorphisms in base excision DNA repair genes and association with melanoma risk in a pilot study on central-south Italian population. Clinica Chimica Acta (2012) 413:1519–24. doi: 10.1016/j.cca.2012.06.013
44. Cocoş R, Schipor S, Nicolae I, Thomescu C, Raicu F. Role of COX-2 activity and CRP levels in patients with non-melanoma skin cancer. –765G>C PTGS2 polymorphism and NMSC risk. Arch Dermatol Res (2012) 304:335–42. doi: 10.1007/s00403-011-1194-0
45. Gao R, Reece KM, Sissung T, Fu SH, Venzon DJ, Reed E, et al. Are race-specific ERCC1 haplotypes in melanoma cases versus controls related to the predictive and prognostic value of ERCC1 N118N? BMJ Open (2013) 3:e002030. doi: 10.1136/bmjopen-2012-002030
46. Oliveira C, Rinck-Junior JA, Lourenço GJ, Moraes AM, Lima CSP. Assessment of the XPC (A2920C), XPF (T30028C), TP53 (Arg72Pro) and GSTP1 (Ile105Val) polymorphisms in the risk of cutaneous melanoma. J Cancer Res Clin Oncol (2013) 139:1199–206. doi: 10.1007/s00432-013-1430-4
47. Maccioni L, Rachakonda PS, Scherer D, Bermejo JL, Planelles D, Requena C, et al. Variants at chromosome 20 (ASIP locus) and melanoma risk. Int J Cancer (2013) 132:42–54. doi: 10.1002/ijc.27648
48. Peña-Chilet M, Blanquer-Maceiras M, Ibarrola-Villava M, Martinez-Cadenas C, Martin-Gonzalez M, Gomez-Fernandez C, et al. Genetic variants in PARP1 (rs3219090) and IRF4 (rs12203592) genes associated with melanoma susceptibility in a Spanish population. BMC Cancer (2013) 13:160. doi: 10.1186/1471-2407-13-160
49. Peña-Chilet M, Ibarrola-Villava M, Martin-González M, Feito M, Gomez-Fernandez C, Planelles D, et al. rs12512631 on the group specific complement (Vitamin d-binding protein GC) implicated in melanoma susceptibility. PloS One (2013) 8:e59607. doi: 10.1371/journal.pone.0059607
50. Maccioni L, Rachakonda PS, Bermejo JL, Planelles D, Requena C, Hemminki K, et al. Variants at the 9p21 locus and melanoma risk. BMC Cancer (2013) 13:325. doi: 10.1186/1471-2407-13-325
51. Francisco G, Gonçalves FT, Luiz OC, Saito RF, Toledo RA, Sekiya T, et al. Polymorphisms in the p27kip-1 and prohibitin genes denote novel genes associated with melanoma risk in Brazil, a high ultraviolet index region. Melanoma Res (2013) 23:231–6. doi: 10.1097/CMR.0b013e3283612483
52. Yamashita J, Iwakiri T, Fukushima S, Jinnin M, Miyashita A, Hamasaki T, et al. The rs2910164 G>C polymorphism in microRNA-146a is associated with the incidence of malignant melanoma. Melanoma Res (2013) 23:13. doi: 10.1097/CMR.0b013e32835c5b30
53. Córdoba-Lanús E, Hernández-Jiménez JG, Medina-Coello C, Espinoza-Jiménez A, González A, Rodríguez-Pérez M-C, et al. MC1R gene variants and sporadic malignant melanoma susceptibility in the canary islands population. Arch Dermatol Res (2014) 306:51–8. doi: 10.1007/s00403-013-1420-z
54. Gomez-Lira M, Ferronato S, Malerba G, Santinami M, Maurichi A, Sangalli A, et al. Association of promoter polymorphism –765G>C in the PTGS2 gene with malignant melanoma in Italian patients and its correlation to gene expression in dermal fibroblasts. Exp Dermatol (2014) 23:766–8. doi: 10.1111/exd.12522
55. Oliveira C, Lourenço GJ, Rinck-Junior JA, Cintra ML, Moraes AM, Lima CSP. Association between genetic polymorphisms in apoptosis-related genes and risk of cutaneous melanoma in women and men. J Dermatol Sci (2014) 74:135–41. doi: 10.1016/j.jdermsci.2013.12.010
56. Thunell LK, Bivik C, Wäster P, Fredrikson M, Stjernström A, Synnerstad I, et al. MDM2 SNP309 promoter polymorphism confers risk for hereditary melanoma. Melanoma Res (2014) 24:190–7. doi: 10.1097/CMR.0000000000000063
57. Llorca-Cardeñosa MJ, Peña-Chilet M, Mayor M, Gomez-Fernandez C, Casado B, Martin-Gonzalez M, et al. Long telomere length and a TERT-CLPTM1 locus polymorphism association with melanoma risk. Eur J Cancer (2014) 50:3168–77. doi: 10.1016/j.ejca.2014.09.017
58. Hsu L-I, Wu M-M, Wang Y-H, Lee C-Y, Yang T-Y, Hsiao B-Y, et al. Association of environmental arsenic exposure, genetic polymorphisms of susceptible genes, and skin cancers in Taiwan. BioMed Res Int (2015) 2015:e892579. doi: 10.1155/2015/892579
59. Russo I, Cona C, Saponeri A, Bassetto F, Baldo V, Alaibac M. Association between toll-like receptor 7 Gln11Leu single-nucleotide polymorphism and basal cell carcinoma. Biomed Rep (2016) 4:459. doi: 10.3892/br.2016.597
60. Elefanti L, Sacco G, Stagni C, Rastrelli M, Menin C, Russo I, et al. TLR7 Gln11Leu single nucleotide polymorphism and susceptibility to cutaneous melanoma. Oncol Lett (2016) 12:275–80. doi: 10.3892/ol.2016.4584
61. Mukhammadiyeva GF, Karimov DO, Bakirov AB, Karimova LK. TP53 gene polymorphisms and occupational skin cancer risks for workers of glass fiber manufacture. Iranian J Public Health (2017) 46:1495.
62. Li Y-L, Wei F, Li Y-P, Zhang L-H, Bai Y-Z. A case-control study on association of nucleotide excision repair polymorphisms and its interaction with environment factors with the susceptibility to non-melanoma skin cancer. Oncotarget (2017) 8:80994. doi: 10.18632/oncotarget.20942
63. Burns EM, Guroji P, Ahmad I, Nasr HM, Wang Y, Tamimi IA, et al. Association of vitamin d receptor polymorphisms with the risk of nonmelanoma skin cancer in adults. JAMA Dermatol (2017) 153:983. doi: 10.1001/jamadermatol.2017.1976
64. Sangalli A, Orlandi E, Poli A, Maurichi A, Santinami M, Nicolis M, et al. Sex-specific effect of RNASEL rs486907 and miR-146a rs2910164 polymorphisms’ interaction as a susceptibility factor for melanoma skin cancer. Melanoma Res (2017) 27:309–14. doi: 10.1097/CMR.0000000000000360
65. Motorina AV, Palkina NV, Komina AV, Ruksha TG, Artyukhov IP, Kozlov VV. Genetic analysis of melanocortin 1 receptor red hair color variants in a Russian population of Eastern Siberia. Eur J Cancer Prev (2018) 27:192. doi: 10.1097/CEJ.0000000000000317
66. Gomez GVB, Rinck-Junior JA, Oliveira C, Silva DHL, Mamoni RL, Lourenço GJ, et al. PDCD1 gene polymorphisms as regulators of T-lymphocyte activity in cutaneous melanoma risk and prognosis. Pigment Cell Melanoma Res (2018) 31:308–17. doi: 10.1111/pcmr.12665
67. Yuan T-A, Yourk V, Farhat A, Ziogas A, Meyskens FL, Anton-Culver H, et al. A case-control study of the genetic variability in reactive oxygen species–metabolizing enzymes in melanoma risk. Int J Mol Sci (2018) 19(1):242. doi: 10.3390/ijms19010242
68. Sławińska M, Zabłotna M, Gleń J, Lakomy J, Nowicki RJ, Sobjanek M. STAT3 polymorphisms and IL-6 polymorphism are associated with the risk of basal cell carcinoma in patients from northern Poland. Arch Dermatol Res (2019) 311:697. doi: 10.1007/s00403-019-01952-7
69. Orlandi E, Zanot C, Poli A, Nicolis M, Rodolfo M, Turco A, et al. Lack of association of metastasis-associated lung adenocarcinoma transcript 1 variants with melanoma skin cancer risk. Melanoma Res (2019) 29:660–3. doi: 10.1097/CMR.0000000000000605
70. Ozola A, Ruklisa D, Pjanova D. The complementary effect of rs1042522 in TP53 and rs1805007 in MC1R is associated with an elevated risk of cutaneous melanoma in Latvian population. Oncol Lett (2019) 18:5225. doi: 10.3892/ol.2019.10906
71. Fathi F, Ebrahimi M, Eslami A, Hafezi H, Eskandari N, Motedayyen H. Association of programmed death-1 gene polymorphisms with the risk of basal cell carcinoma. Int J Immunogen (2019) 46:444–50. doi: 10.1111/iji.12447
72. Reis LB, Bakos RM, Vianna FSL, Macedo GS, Jacovas VC, Ribeiro-dos-Santos AM, et al. Skin pigmentation polymorphisms associated with increased risk of melanoma in a case-control sample from southern Brazil. BMC Cancer (2020) 20:1069. doi: 10.1186/s12885-020-07485-x
73. Morgado-Águila C, Rey-Sánchez P, Gil-Fernández G, Costa-Fernández MC, Rodríguez-Velasco FJ. Vitamin d receptor polymorphisms and non-melanoma skin cancer risk: A case-control study. J Clin Med (2020) 9(12):3819. doi: 10.3390/jcm9123819
74. Tovar-Parra JD, Gutiérrez-Castañeda LD, Gil-Quiñones SR, Nova JA, Pulido L. CDKN2A polymorphism in melanoma patients in Colombian population: A case-control study. BioMed Res Int (2020) 2020:7458917. doi: 10.1155/2020/7458917
75. Fathi F, Zamani B, Piroozmand A, Mozafarpoor S, Seyedhashemi E, ArefNezhad R, et al. Programmed cell death 1 (PDCD1) gene haplotypes and susceptibility of patients to basal cell carcinoma. Mol Biol Rep (2021) 48:2047–52. doi: 10.1007/s11033-020-06115-w
76. Aristizabal-Pachon A, Gonzalez-Giraldo Y, Garcia A, Suarez D, Rodriguez A, Gonzalez-Santos J. Association between VDR gene polymorphisms and melanoma susceptibility in a Colombian population. Asian Pac J Cancer Prev (2022) 23:79–85. doi: 10.31557/APJCP.2022.23.1.79
77. Dunjic M, Lukic N, Djordjevic B, Uzelac B, Ostojic N, Supic G. GLI-1 polymorphisms of hedgehog pathway as novel risk and prognostic biomarkers in melanoma patients. Melanoma Res (2022) 32:11–7. doi: 10.1097/CMR.0000000000000789
78. König J, Krahn U, Binder H. Visualizing the flow of evidence in network meta-analysis and characterizing mixed treatment comparisons. Stat Med (2013) 32:5414–29. doi: 10.1002/sim.6001
79. Uitterlinden AG, Fang Y, van Meurs JBJ, Pols HAP, van Leeuwen JPTM. Genetics and biology of vitamin d receptor polymorphisms. Gene (2004) 338:143–56. doi: 10.1016/j.gene.2004.05.014
80. Ma Y, Xiang Z, Yao X, Li C, Wu J, Feng S, et al. Associations between vitamin d receptor gene polymorphisms and chronic spontaneous urticaria in Chinese han population. Postepy Dermatol Alergol (2020) 37:250–4. doi: 10.5114/ada.2020.94843
81. Ou Y, Jiang X, Guan H. Vitamin d receptor gene polymorphisms and risk of atopic dermatitis in Chinese han population. Int J Gen Med (2021) 14:5301–12. doi: 10.2147/IJGM.S326477
82. Pepineli AC, Alves HV, Tiyo BT, Macedo LC, Visentainer L, de Lima Neto QA, et al. Vitamin d receptor gene polymorphisms are associated with leprosy in southern Brazil. Front Immunol (2019) 10:2157. doi: 10.3389/fimmu.2019.02157
83. Kazemi SM, Esmaieli-bandboni A, Veisi Malekshahi Z, Shahbaz Sardood M, Hashemi M, Majidzadeh K, et al. Vitamin d receptor gene polymorphisms and risk of breast cancer in Iranian women. Ann Med Surg (Lond) (2021) 73:103150. doi: 10.1016/j.amsu.2021.103150
84. Hu J, Xu Z, Ye Z, Li J, Hao Z, Wang Y. The association between single nucleotide polymorphisms and ovarian cancer risk: A systematic review and network meta-analysis. Cancer Med. (2002) 12(1):541–56. doi: 10.1002/cam4.4891
85. Hoseinkhani Z, Rastegari-Pouyani M, Tajemiri F, Yari K, Mansouri K. Association of vitamin d receptor polymorphisms (FokI (Rs2228570), ApaI (Rs7975232), BsmI (Rs1544410), and TaqI (Rs731236)) with gastric cancer in a Kurdish population from West of Iran. Rep Biochem Mol Biol (2021) 9:435–41. doi: 10.52547/rbmb.9.4.435
86. Quan Y, Yang J, Qin T, Hu Y. Associations between twelve common gene polymorphisms and susceptibility to hepatocellular carcinoma: Evidence from a meta-analysis. World J Surg Oncol (2019) 17:216. doi: 10.1186/s12957-019-1748-8
87. Beysel S, Eyerci N, Pinarli FA, Apaydin M, Kizilgul M, Caliskan M, et al. VDR gene FokI polymorphism as a poor prognostic factor for papillary thyroid cancer. Tumour Biol (2018) 40. doi: 10.1177/1010428318811766. 1010428318811766.
88. Li L, Wu B, Yang L, Yin G, Wei W, Sui S, et al. Association of vitamin d receptor gene polymorphisms with pancreatic cancer: A pilot study in a north China population. Oncol Lett (2013) 5:1731–5. doi: 10.3892/ol.2013.1215
89. Zeljic K, Kandolf-Sekulovic L, Supic G, Pejovic J, Novakovic M, Mijuskovic Z, et al. Melanoma risk is associated with vitamin d receptor gene polymorphisms. Melanoma Res (2014) 24:273–9. doi: 10.1097/CMR.0000000000000065
90. Barroso E, Fernandez LP, Milne RL, Pita G, Sendagorta E, Floristan U, et al. Genetic analysis of the vitamin d receptor gene in two epithelial cancers: Melanoma and breast cancer case-control studies. BMC Cancer (2008) 8:385. doi: 10.1186/1471-2407-8-385
91. Wang F, Chang D, Hu F, Sui H, Han B, li D, et al. DNA Repair gene XPD polymorphisms and cancer risk: A meta-analysis based on 56 case-control studies. Cancer Epidemiol Biomarkers Prev (2008) 17:507–17. doi: 10.1158/1055-9965.EPI-07-2507
92. Liao Z, Yi M, Li J, Zhang Y. DNA Repair in lung cancer: A large-scale quantitative analysis for polymorphisms in DNA repairing pathway genes and lung cancer susceptibility. Expert Rev Respir Med (2022) 16:997–1010. doi: 10.1080/17476348.2022.2115361
93. Shao X, Yang X, Liu Y, Song Q, Pan X, Chen W, et al. Genetic polymorphisms in DNA repair genes and their association with risk of cervical cancer: A systematic review and meta-analysis. J Obstet Gynaecol Res (2022) 48:2405–18. doi: 10.1111/jog.15325
94. Mitra AK, Singh N, Garg VK, Chaturvedi R, Sharma M, Rath SK. Statistically significant association of the single nucleotide polymorphism (SNP) rs13181 (ERCC2) with predisposition to squamous cell carcinomas of the head and neck (SCCHN) and breast cancer in the north Indian population. J Exp Clin Cancer Res (2009) 28:104. doi: 10.1186/1756-9966-28-104
95. Li S-X, Dai Q-S, Chen S-X, Zhang S-D, Liao X-Y, Deng X, et al. Xeroderma pigmentosum complementation group d (XPD) gene polymorphisms contribute to bladder cancer risk: A meta-analysis. Tumour Biol (2014) 35:3905–15. doi: 10.1007/s13277-013-1519-z
96. Kertat K, Rosdahl I, Sun X-F, Synnerstad I, Zhang H. The Gln/Gln genotype of XPD codon 751 as a genetic marker for melanoma risk and Lys/Gln as an important predictor for melanoma progression: A case control study in the Swedish population. Oncol Rep (2008) 20:179–83.
97. Wheless L, Kistner-Griffin E, Jorgensen TJ, Ruczinski I, Berthier-Schaad Y, Kessing B, et al. A community-based study of nucleotide excision repair polymorphisms in relation to risk of non-melanoma skin cancer. J Invest Dermatol (2012) 132:1354. doi: 10.1038/jid.2012.4
98. Wu H, Li S, Hu X, Qin W, Wang Y, Sun T, et al. Associations of mRNA expression of DNA repair genes and genetic polymorphisms with cancer risk: A bioinformatics analysis and meta-analysis. J Cancer (2019) 10:3593–607. doi: 10.7150/jca.30975
99. Laronha H, Caldeira J. Structure and function of human matrix metalloproteinases. Cells (2020) 9:1076. doi: 10.3390/cells9051076
100. Gialeli C, Theocharis AD, Karamanos NK. Roles of matrix metalloproteinases in cancer progression and their pharmacological targeting. FEBS J (2011) 278:16–27. doi: 10.1111/j.1742-4658.2010.07919.x
101. Liu H, Wei Q, Gershenwald JE, Prieto VG, Lee JE, Duvic M, et al. Influence of single nucleotide polymorphisms in the MMP1 promoter region on cutaneous melanoma progression. Melanoma Res (2012) 22:169–75. doi: 10.1097/CMR.0b013e32834fc46b
102. Bevona C, Goggins W, Quinn T, Fullerton J, Tsao H. Cutaneous melanomas associated with nevi. Arch Dermatol (2003) 139:1620–4. doi: 10.1001/archderm.139.12.1620
103. Sung W-W, Chang C-H. Nevi, dysplastic nevi, and melanoma: Molecular and immune mechanisms involving the progression. Tzu Chi Med J (2021) 34:1–7. doi: 10.4103/tcmj.tcmj_158_20
104. Giammanco M, Di Majo D, La Guardia M, Aiello S, Crescimannno M, Flandina C, et al. Vitamin d in cancer chemoprevention. Pharm Biol (2015) 53:1399–434. doi: 10.3109/13880209.2014.988274
105. Mostafa WZ, Hegazy RA. Vitamin d and the skin: Focus on a complex relationship: A review. J Adv Res (2015) 6:793–804. doi: 10.1016/j.jare.2014.01.011
106. Kraemer KH, DiGiovanna JJ, Tamura D. “Xeroderma pigmentosum”, in: GeneReviews® (1993). Seattle (WA: University of Washington, Seattle. Available at: http://www.ncbi.nlm.nih.gov/books/NBK1397/ (Accessed December 22, 2022).
107. ERCC2 ERCC excision repair 2, TFIIH core complex helicase subunit [Homo sapiens (human)] - gene . NCBI. Available at: https://www.ncbi.nlm.nih.gov/gene/2068 (Accessed August 28, 2022).
Keywords: skin cancer, single-nucleotide polymorphisms, network meta-analysis, systematic review, melanoma
Citation: Zhang L, Pozsgai É, Song Y, Macharia J, Alfatafta H, Zheng J, Li Z, Liu H and Kiss I (2023) The relationship between single nucleotide polymorphisms and skin cancer susceptibility: A systematic review and network meta-analysis. Front. Oncol. 13:1094309. doi: 10.3389/fonc.2023.1094309
Received: 09 November 2022; Accepted: 23 January 2023;
Published: 15 February 2023.
Edited by:
Mauro Alaibac, University of Padua, ItalyReviewed by:
Mangala Hegde, Indian Institute of Technology Guwahati, IndiaLuz Gutierrez-Castañeda, Fundación Universitaria de ciencias de la Salud, Colombia
Copyright © 2023 Zhang, Pozsgai, Song, Macharia, Alfatafta, Zheng, Li, Liu and Kiss. This is an open-access article distributed under the terms of the Creative Commons Attribution License (CC BY). The use, distribution or reproduction in other forums is permitted, provided the original author(s) and the copyright owner(s) are credited and that the original publication in this journal is cited, in accordance with accepted academic practice. No use, distribution or reproduction is permitted which does not comply with these terms.
*Correspondence: István Kiss, istván.kiss@aok.pte.hu; Hongbo Liu, hbliu@cmu.edu.cn
†These authors have contributed equally to this work