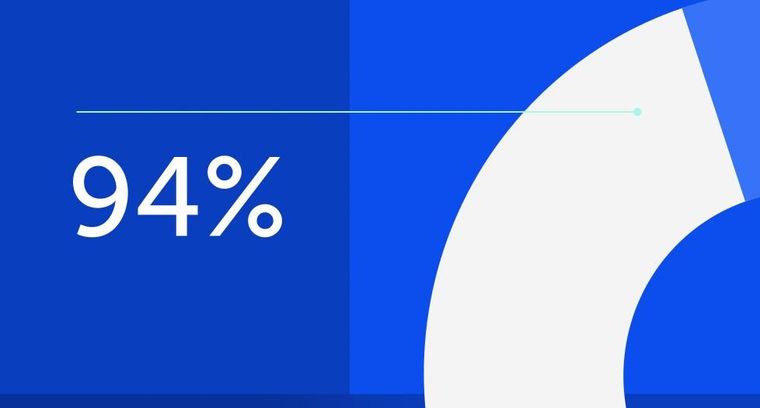
94% of researchers rate our articles as excellent or good
Learn more about the work of our research integrity team to safeguard the quality of each article we publish.
Find out more
ORIGINAL RESEARCH article
Front. Oncol., 13 March 2023
Sec. Thoracic Oncology
Volume 13 - 2023 | https://doi.org/10.3389/fonc.2023.1092478
This article is part of the Research TopicInnovative Strategies and New Insights for the Treatment of Stage III Non-Small Cell Lung CancerView all 10 articles
Objective: To compare the performance of three machine learning algorithms with the tumor, node, and metastasis (TNM) staging system in survival prediction and validate the individual adjuvant treatment recommendations plan based on the optimal model.
Methods: In this study, we trained three machine learning madel and validated 3 machine learning survival models-deep learning neural network, random forest and cox proportional hazard model- using the data of patients with stage-al3 NSCLC patients who received resection surgery from the National Cancer Institute Surveillance, Epidemiology, and End Results (SEER) database from 2012 to 2017,the performance of survival predication from all machine learning models were assessed using a concordance index (c-index) and the averaged c-index is utilized for cross-validation. The optimal model was externally validated in an independent cohort from Shaanxi Provincial People’s Hospital. Then we compare the performance of the optimal model and TNM staging system. Finally, we developed a Cloud-based recommendation system for adjuvant therapy to visualize survival curve of each treatment plan and deployed on the internet.
Results: A total of 4617 patients were included in this study. The deep learning network performed more stably and accurately in predicting stage-iii NSCLC resected patients survival than the random survival forest and Cox proportional hazard model on the internal test dataset (C-index=0.834 vs. 0.678 vs. 0.640) and better than TNM staging system (C-index=0.820 vs. 0.650) in the external validation. The individual patient who follow the reference from recommendation system had superior survival compared to those who did not. The predicted 5-year-survival curve for each adjuvant treatment plan could be accessed in the recommender system via the browser.
Conclusion: Deep learning model has several advantages over linear model and random forest model in prognostic predication and treatment recommendations. This novel analytical approach may provide accurate predication on individual survival and treatment recommendations for resected Stage-iii NSCLC patients.
Stage-iii non-small cell lung cancer (NSCLC) accounts for about 1/4 to 1/3 of total lung cancer and is a very heterogeneous disease with a discouraging clinical prognosis, the 5-year survival rate of NSCLC is only 15%-40% (1). For operable stage-iii lung cancer patients, surgery-based comprehensive treatment is recommended. However, even after radical tumor resection, there is still a high risk of recurrence and metastasis, so adjuvant therapy after surgery is required to improve long-term survival probability. Postoperative adjuvant therapy mainly includes adjuvant chemotherapy, radiotherapy and targeted therapy. Among them, adjuvant targeting is mainly aimed at the EGFR-amplified non-small cell lung cancer patients. Targeted therapy can improve its prognosis, but the proportion of this population is relatively low, only 9% of the total non-small cell lung cancer patients (2). For the vast majority of patients with EGFR-negative stage-iii lung cancer, studies have shown that postoperative chemotherapy (POCT) can improve the 5-year survival rate by 5% (3). Other researches confirm that the value of postoperative radiotherapy for high-risk subgroups (4–6), While the results of the meta-analysis in 1998 determines that postoperative adjuvant radiotherapy is not recommended for patients with stage I-IIIB (N0-N1) (7). In addition, the 2020 Lung ART study suggests that adjuvant radiotherapy is not recommended for patients with N2 after lung cancer surgery (8). Therefore, whether postoperative radiotherapy has a beneficial effect on overall survival (OS) is controversial. In the current clinical practice, the formulation and implementation of adjuvant chemotherapy and radiotherapy treatment plans are mainly based on the TNM staging system. Therefore, there are two drawbacks. The first defect is that only three clinical indicators of patients T, N, and M are considered to guide the clinical treatment of patients while ignoring other important characteristics of patients such as physiological characteristics (age, gender) and Other important clinical characteristics (surgical method, primary tumor location, tumor grade, number of positive lymph nodes (LNs), number of LNs examined, and adjuvant therapy methods). Secondly, the TNM staging system is used for risk stratification of the population, and cannot work as a tool to provide prognosis prediction for individual patients. Therefore, it cannot meet the need to improve patient prognosis. Today, with today’s increasingly perfect electronic medical record system, deep learning has been widely used in the medical field to predict the survival rate of cancer patients, which performs better than the traditional cox regression method (9–17). In this experiment, we trained a deep learning model based on a large amount of clinical data and developed a patient-oriented assistant utilizing this model. A recommendation system for radiotherapy and chemotherapy can be accessed through the Internet to provide patients with reference opinions for postoperative radiotherapy and chemotherapy regimens Figure 1.
Regarding the training cohort, We selected 4517 medical cases from Database: Incidence - SEER Research Plus Data, 18 Registries, Nov 2019 Sub (2000–2017) - Linked To County Attributes - Total U.S., 1969-2018 Counties, National Cancer Institute,DCCPS, Surveillance Research Program, released April 2021, based on the November 2019 submission. We included Data records if they meet the criterion (1), patients pathologically diagnosed between January 2012 and December 2017 with primary stage-teriii non-small cell lung cancer (NSCLC) and (2) the existence of one malignant lesion. On the contrary, We excluded clinical cases according to the standard (1), patients whose regional lymph nodes performed during the initial work-up or first course of therapy are unknown or missing. Then we choose the features relevant to the OS (overall survival) of the NSCLC, including demographic information (Age and Sex) and NSCLC-cancer-related characteristics (TNM stage, histology type, primary site, tumor size, regional node number examined, regional node positive number and laterality of the tumor), and treatment details(surgery of primary site, radiation, and chemotherapy), The outcome is the patient survival time and death indicator. As for the cohort for external validation of the model, the inclusion criteria and exclusion criteria are consistent with the training group, So we randomly collected 100 stage-iii non-small cell lung cancer patients who underwent surgery (Lobectomy WITH mediastinal lymph node dissection and Pneumonectomy) from January 2012 to December 2017 in Shaanxi Provincial People’s Hospital, China.
The training data and the testing data are stored in CSV files. Both datasets contain two types of variables in the covariates, numerical variable and categorical variable. In the dataset, we have 3 numerical variable fields, including regional node positive number, regional node number examined and tumor size as well as other 10 categorical variable features. In order to avoid the evaluation problems via using label encoding conversion to categorical, we converse the 10 categorical features by utilizing one hot encoding to identify the different categorical values in the feature in a binary fashion. To illustrate, Regarding feature surgery on the primary site, before conversion, this field contains two values encoded for two surgery types (Lobectomy WITH mediastinal lymph node dissection, Pneumonectomy WITH mediastinal lymph node dissection). After transformation, the very field will be replaced by two surgery types, the value of the two features could only be 0 or 1 to identify the specific surgery type. In addition, as for feature tumor size, in the training set the unit is millimeter while in the testing dataset, the unit is centimeter. So we divide the value in the training set by 10 to make the unit the same. Finally, we perform normalization in order to accelerate the training process.
In this section, we created three machine learning models to perform the survival analysis to select the optimal one.
We developed a deep learning model based on DeepSurv to predict personal hazard rate according to the patient’s current clinical condition. From the input to output, the patient’s baseline data is the input to the neural network, followed by the fully-connected hidden layers of nodes as well as a drop layer after each hidden layer. The output of the network is the hazard rate. Regarding the activation function of each node, in order to overcome the problem of vanishing gradients, we select ReLU to add nonlinearity to the model which could help the model learn the complex relationship between covariates and the hazard rate. As for the loss function, we train the model to minimize the average negative log partial likelihood with regularization:
where θ is the weight of every node in the network, is the number of dead patients and λ is the l2 regularization parameter, is the predicated hazard rate. we use Adam for the gradient descent algorithm to update the parameter of the model for lots of epochs, because Adam is more efficient when working with problems involving high dimensional data and requiring less memory for optimization process compared with SGD method (18). We utilize random Search to optimize the hyper-parameters because compared to Grid Search, Random Search could try more cases for important hyper-parameters. In the experiment, we perform this on the log space of the learning rate in [0.00001, 0.1], the dropout rate in [0.2-0.5], the number of hidden layers in [1, 7] and the number of nodes in each hidden layer in [5,90].
We also trained a random forest model, this model is reliable because it forces each split to consider only a subset of the predictors. In this study, Random Search is still used to tune the number of the comprising trees in [100,300], the minimum number of samples required to split an internal node in [2,50] as well as the minimum number of samples required to be at a leaf node in [1,20].
Lastly, we trained the Penalized Cox Proportional hazard model with the same loss function as the deep learning model. we tuned the hyperparameter by using Random Search Method, specifically, the penalizer in [0.001,1] and the learning rate in [0.001,1].
The concordance index(C-index) is used to measure the performance of the model. The C-index is the ratio of pairs of patients ordered correctly to all pairs. Thus the higher C-index, the better performance of the model. In the study, The 4517 SEER data records were divided into two groups, 3534(80%) records were used for training while 883(20%) records were treated as the validation set. The five-fold cross-validation was performed to tune the hyper-parameters of each model and select the best model for survival prediction. Additionally, external validation was performed on the selected optimal model and TNM staging system and compare the generalizability of the two models. Eventually, we performed the attribution analysis for the deep learning survival model by the integrated gradients (19) method based on the testing dataset to rank the clinical feature importance.
The deep learning algorithm could recommend treatment for patients according to their current clinical conditions (20). we could load the model and set the input according to the patient’s demographic feature(age and gender), Surgery Type(lobectomy and pneumonectomy), Type(histology type and laterality) and the stage information of NSCLC(TNM, the number of the examined regional node, the number of the positive regional node and the tumor size). As for Adjuvant therapy, we predict the hazard rate under four adjuvant therapy treatments (with radiation and chemotherapy, with radiation and without chemotherapy, without radiation and with chemotherapy, and without radiation and chemotherapy). Then we could get the four cumulative hazard functions under each adjuvant therapy treatment and finally derive the four 5-year survival functions after negating and exponentiating the cumulative hazard function. In this application, we develop the backend code to calculate the four 5-year adjuvant therapy survival functions and implement the UI code to display the predicated survival functions in the line race chart.
The three models are trained with Python v 3.9, PyTorch v 1.11.0 is used to train the deep learning algorithm and PySurvival v 0.1.2 is utilized to train the random survival forest and penalized cox proportional hazard model. The Front UI of the adjuvant therapy recommender system is developed with Vue.js javascript framework and a Material Design component framework called Vuetify. The backend code of the web application is implemented by the Django REST framework. The recommender system is deployed on Tencent Cloud, which could be accessed through a web browser.
Based on the inclusion criteria, we include 4617 stage-iii NSCLC patients who received Surgeries (Lobectomy and Pneumonectomy with mediastinal lymph node dissection) in this study. The 4517 patients out of 4617 are extracted from the SEER database and used as a training set while the other 100 patients are from China Database for model testing. The baseline medical characteristics of the two cohorts are shown in Table 1. From the AJCC TNM staging system’s perspective, all patients in the training set and the testing set are stage-iii NSCLC patients. In the SEER cohort, most patients’ histology type is Adenocarcinoma, which takes 44.28%. The next one is Squamous cell carcinoma, which takes 23.27%. Regarding the Received surgeries, 85.51% of patients received Lobectomy WITH mediastinal lymph node dissection while the rest (14.48%) accepted Pneumonectomy WITH mediastinal lymph node dissection for treatment. Concerning Adjuvant treatment, 74.12% of patients accepted chemotherapy and about 41.88% received beam radiation. On the contrary, in the test cohort, most patients received Lobectomy WITH mediastinal lymph node dissection, the two leading histology types are Squamous cell carcinoma and Adenocarcinoma, respectively 46% and 43% of the population. As for Adjuvant treatment, 1/3 received beam radiation and almost everyone received chemotherapy.
After the process of random search, we finally settled down on the hyperparameter of the deep learning model, the model consists of 2 hidden layers, from input to output, including 60, 43 neurons in each layer with a dropping out unit between each layer. we improve neural network generalization by setting the learning rate to 0.001 and 0.5 as the dropout rate to avoid overfitting. Figure 2 shows the training loss curves of the survival network. At the beginning of the training process, the loss of the validation and training set decreases continually. After 331 epochs of parameter optimization, the loss of the validation set begins at 3.6936 and stops decreasing at 3.1753 while the training loss continues to decrease from 3.3844 started at 3.8446. Then we terminate the optimization to avoid overfitting and save the model for test.
Figure 2 Diagram of the traing loss and the validation loss in the optimization procedure. The x-axis represents the number of epoch, and the y-axis represents value of loss function. The orange line is the validation loss function and the blue one represents the training loss function.
In the random survival forest, We set the number of the estimating trees to 959, the minimum number of samples required to split an internal node to 10 and the minimum number of samples required to be at a leaf node to 15. In the Penalized Cox Proportional hazard model, we configure the penalizer to 0.005 and the learning rate to 0.01
Then we perform 5-fold cross-validation to select the optimal model for survival prediction. Figure 3 displays the exact value and the line chart of each model in every fold validation, the deep learning model shows a more stable and exceptional performance on the concordance index compared to the other two models. The mean of the concordance index of the deep learning algorithm is 0.843, which is much higher than the random forest (0.678) and cox proportional hazard model (0.678) (Table 2). Based on the result of cross-validation, deep learning is selected to compare the TNM staging system on external validation. The performance of the deep learning model is better (0.82 vs 0.65)
Figure 3 The concordance index of three models for 5 fold cross validation. The x-axis represents the number of fold, and the y-axis represents value of concordance index for each model.
Table 2 Performance of the survival models to predict hazard rate of the stage-III NSCLC patient received resection surgery.
As for the feature importance for the network, from the Figure 4 we can observe four of the top important features: regional positive nodes (0.6634), regional examied nodes (-0.7648), tumor size (-0.5633) and Age(-0.4633). In terms of least important features, we observe that the surgery on the primary site (0.0632) is voted to be least significant based on attribution algorithm. The absolute value for attribution scores of other features is greater than 0.1 and less than 0.5.
Figure 4 The attribution score of all input features in the deep learning model. The x-axis represents the name of the input features, and the y-axis represents value of attribution score for each feature.
Then we perform 5-fold cross-validation to select the optimal model for survival prediction. Figure 3 displays the exact value and the line chart of each model in every fold validation, the deep learning model shows a more stable and exceptional performance on the concordance index compared to the other two models. The mean of the concordance index of the deep learning algorithm is 0.843, which is much higher than the random forest (0.678) and cox proportional hazard model (0.678) (Table 2). Based on the result of cross-validation, deep learning is selected to compare the TNM staging system on external validation. The performance of the deep learning model is better (0.82 vs 0.65)
Since the deep learning model has better performance than the TNM staging system, we could not only predict the survival function of the current patient but also offer an adjuvant therapy reference to the oncology doctor based on prediction over different therapy treatment plans. Thus we deployed the recommender system to the Internet, which could be accessed with a browser in [http://1.15.80.136/nsclc/], input the current clinical status, including Demographic, surgery type, cancer type and stage information, of one patient, and click the submit button (Figure 5).
Then the browser will redirect to the result page (Figure 6), and we could see four 5-year predicted survival curves for each treatment plan. Based on the plot, the predicted optimal treatment plan is only receiving beam radiation for adjuvant treatment, whose survival probability is highest in the next 60 months.
Thus, the specialist could get the reference for adjuvant treatment plan decision-making. Code related to this application can be found at https://github.com/snowflake-Zhao/nsclc.
This study provides a model that is more accurate than the TNM staging system to predict the prognosis of the stage-iii received resection NSCLC cancer patients in 5 years. Additionally, the deep learning survival model is more precise and stable than the random survival forest and cox proportional model to predict the hazard rate of the stage-III resectable NSCLC cancer patients. This demonstrated our first goal that the deep learning approach is more reliable than TNM in predicting the hazard rate. Driven by the desire to resolve the controversy on devising adjuvant treatment plans for stage-iii received resection NSCLC cancer patients, we did solve this problem by developing a recommender system based on the externally validated deep learning model. To our best knowledge, this is the first recommender system to provide adjuvant treatment plans reference for stage-iii NSCLC cancer patients who received resection.
As reported, Adeoye J, et al. have trained DeepSurv and RSF (random survival forest) models for predicting the malignant transformation probability of oral leukoplakia and lichenoid lesions with (N=716) patients (21). Their exceptional results suggest a considerable improvement of accuracy for hazard prediction using the deep learning model when it is compared with the Cox proportional hazard model(C-index=0.95 vs 0.83), and RSF’s performance is much better and more stable than that of Cox proportional hazard model(C-index=0.91 vs 0.83) in this task. Our outcome of the experiment is consistent with their conclusion. In another study, Huang C, et al. developed software to select adjuvant radiotherapy and chemotherapy treatment plan according to the corresponding output hazard rate. Our software has two major points different from their product (22). One is the output page for oncology specialists. Their output is just one hazard rate, which is difficult for specialists and patients to understand. On the contrary, we plot the four adjuvant treatments predicted survival curves in 60 months, which is more straightforward for patients and doctors because people could understand their probability of survival for each adjuvant treatment plan in the 5 years. The other point is our software could be accessed directly through the web browser either on mobile phones, iPad or personal computers instead of installed on the personal computer for seeking recommendation guidance, which is not convenient for doctors to use.
In our study, the random survival forest did not perform well as Lin J, et al’s (C-index= 0.678 vs 0.723) (23), I think this is mainly because the two features in the dataset after one hot encoding, the Histologic type and Radiation, generate lots of sparse variables, including Radioactive implants, Signet ring cell carcinoma and so on, which eventually cause harm to the formation of different estimator trees. The result that the deep learning model’s C-index is higher than the Cox Proportional hazard model(C-index= 0.834 vs 0.640) meets our expectations, mainly because deep learning could formulate the complex relationships between clinical baseline characteristics and the patient’s hazard rate, which is more accurate than the linear relationship assumption of the Cox proportional hazard model. Additionally, the deep learning model has superior performance than the TNM staging system(C-index= 0.82 vs 0.65) is expected, because the neural network takes in more clinical features related to the prognosis of the patients, including Histologic type, age, sex, tumor size and many others, than the TNM staging system and the most important features of the network are regional postive nodes,regional examied nodes, tumor size and the Age, which is slightly different from the TNM stage system, even though the T stage value comes from the tumor size, N stage value comes form the regional nodes, we could tell the exact detailed number of the tumor size and the regional positive nodes could help the model to predict the prognoses more clearly than the general value. Besides the trained model could perform personal prognosis prediction while the TNM staging system could only predict the cohort prognosis. Thus, the deep learning model could possibly substitute the TNM staging system in the future if more medical records could be utilized for training.
In the current medical practice, there is a lack of consensus regarding the principles of adjuvant therapy for stage-iii NSCLC patients. For instance, According to the latest version of NCCN Guidelines for NSCLC(Version5.2022), one major controversy is inconsistent results among different randomized controlled trials of stage-III NSCLC (23–26). The one reason for the inconsistent results among different randomized controlled trials is the RCT lacks external validity (27), which means there might be neglected features that are effective for the prognosis. Because the externally validated deep learning model could include lots of features might related to the prognosis and be sensitive to the different inputs, the model could output the hazard risk of the different treatment plans, then the optimal plan could be obtained by comparing the output of different treatments. In our adjuvant recommendation system, we could obtain the reliable and accurate hazard rate for 4 adjuvant treatment plans from the developed externally validated model. To visualize the outcome, after mathematic transformation, the predicated survival curves for 4 treatment plans are displayed on the Web User Interface. Because of the significant prognostic benefit of following the treatment recommendation which clearly outweighs those who don’t, the recommendation system is promising to serve as a dependable tool for decision-making on the adjuvant treatment plan for each stage-iii NSCLC patient.
From the results of our experiment, the deep learning model performs well in the survival analysis task. However, the model is lacking in explainability owing to the high complexity inside the neural network, which is not realistic to explain the process to humans. If we want to extensively apply the deep learning algorithm in the decision-making of the NSCLC, we definitely need to improve the explainability of the model (28–30). we could incorporate the causal inference ideas in designing inherently interpretable models by adding sample reweighting technique into the loss function to compare the performance with our deep learning result in the future (31–34). Even though the SEER database has numerous NSCLC patient’s medical records, the database could record more detailed attributes in three aspects, including 1) resection information in detail, like resection status (R0/R1/R2) 2)detailed information related to beam radiation, for instance, total dose and dose per fraction 3) further information relevant to chemotherapy on drugs and dosage.
To our best knowledge, this study is the first to research the performance of a deep learning network and random forest in resected Stage-III NSCLC and obtain satisfactory results in survival prediction. In addition, the recommendation system for adjuvant therapy based on the deep learning model will be likely applied to offer recommendation reference to the specialist in the clinical practice.
The original contributions presented in the study are included in the article/Supplementary Material. Further inquiries can be directed to the corresponding author.
Written informed consent was obtained from the individual(s) for the publication of any potentially identifiable images or data included in this article.
LJ and QZ designed the research. LJ collected the training and testing dataset. QZ trained the models and developed the web-application. LJ and QZ wrote the manuscript. JM, BH, SF and FC edited and critically revised the manuscript in regard to important intellectual content. All authors read and approved the manuscript.
This research was funded by a grant from the Science and Technology Foundation of Shaanxi Province (2022JQ-934 and 2022JQ-862) and the Shaanxi Provincial People’s Hospital (2021JY-07).
The authors declare that the research was conducted in the absence of any commercial or financial relationships that could be construed as a potential conflict of interest.
All claims expressed in this article are solely those of the authors and do not necessarily represent those of their affiliated organizations, or those of the publisher, the editors and the reviewers. Any product that may be evaluated in this article, or claim that may be made by its manufacturer, is not guaranteed or endorsed by the publisher.
The Supplementary Material for this article can be found online at: https://www.frontiersin.org/articles/10.3389/fonc.2023.1092478/full#supplementary-material
1. Putora PM, Leskow P, McDonald F, Batchelor T, Evison M. International guidelines on stage iii n2 nonsmall cell lung cancer: surgery or radiotherapy? ERJ Open Res (2020) 6. doi: 10.1183/23120541.00159-2019
2. Kato S, Okamura R, Mareboina M, Lee S, Goodman A, Patel SP, et al. Revisiting epidermal growth factor receptor (egfr) amplification as a target for anti-egfr therapy: analysis of cell-free circulating 290 tumor dna in patients with advanced malignancies. JCO Precis Oncol (2019) 3:1–14. doi: 10.1200/PO.18.00180
3. Felip E, Altorki N, Zhou C, Csoszi T, Vynnychenko I, Goloborodko O, et al. Adjuvant atezolizumab after adjuvant chemotherapy in resected stage ib–iiia non-small-cell lung cancer (impower010): a randomised, multicentre, open-label, phase 3 trial. Lancet (2021) 398:1344–57. doi: 10.1016/S0140-6736(21)02098-5
4. Deng W, Xu T, Xu Y, Wang Y, Liu X, Zhao Y, et al. Survival patterns for patients with resected n2 non–small cell lung cancer and postoperative radiotherapy: a prognostic scoring model and heat map approach. J Thorac Oncol (2018) 13:1968–74. doi: 10.1016/j.jtho.2018.08.2021
5. Wei S, Xie M, Tian J, Song X, Wu B, Liu L. Propensity score-matching analysis of postoperative radiotherapy for stage iiia-n2 non-small cell lung cancer using the surveillance, epidemiology, and end results database. Radiat Oncol (2017) 12:1–11. doi: 10.1186/s13014-017-0836-6
6. Gao F, Li N, Xu Y, Yang G. Evaluation of postoperative radiotherapy effect on survival of resected stage iii-n2 non-small cell lung cancer patients. Front Oncol (2020) 10:1135. doi: 10.3389/fonc.2020.01135
7. Wang EH, Corso CD, Park HS, Chen AB, Wilson LD, Kim AW, et al. Association between radiation 303 dose and outcomes with postoperative radiotherapy for n0-n1 non–small cell lung cancer. Am J Clin Oncol (2018) 41:152–8. doi: 10.1097/COC.0000000000000245
8. Le Pechoux C, Pourel N, Barlesi F, Lerouge D, Antoni D, Lamezec B, et al. Postoperative radiotherapy versus no postoperative radiotherapy in patients with completely resected non-small-cell lung cancer and proven mediastinal n2 involvement (lung art): an open-label, randomised, phase 3 trial. Lancet 3Oncology (2022) 23:104–14. doi: 10.1016/S1470-2045(21)00606-9
9. Lee C, Light A, Alaa A, Thurtle D, van der Schaar M, Gnanapragasam VJ. Application of a novel machine learning framework for predicting non-metastatic prostate cancer-specific mortality in men 311 using the surveillance, epidemiology, and end results (seer) database. Lancet Digital Health (2021) 3 312:e158–65. doi: 10.1016/S2589-7500(20)30314-9
10. Jones O, Matin R, van der Schaar M, Bhayankaram KP, Ranmuthu C, Islam M, et al. Artificial intelligence and machine learning algorithms for early detection of skin cancer in community and primary care settings: a systematic review. Lancet Digital Health (2022) 4:e466–76. doi: 10.1016/S2589-7500(22)00023-1
11. Lee C, Light A, Saveliev ES, van der Schaar M, Gnanapragasam VJ. Developing machine learning algorithms for dynamic estimation of progression during active surveillance for prostate cancer. NPJ digital Med (2022) 5:1–7. doi: 10.1038/s41746-022-00659-w
12. Zhou H, Cheng C, Lipton ZC, Chen GH, Weiss JC. Predicting mortality risk in viral and unspecified pneumonia to assist clinicians with covid-19 ecmo planning. arXiv preprint arXiv:2006.01898. (2020). doi: 10.48550/arXiv.2006.01898
13. Hu S, Chen GH. Distributionally robust survival analysis: A novel fairness loss without demographics. Mach Learn Health (PMLR) (2022), 62–87. doi: 10.48550/arXiv.2211.10508
14. Chiappetta M, Tabacco D, Iaffaldano AG, Evangelista J, Congedo MT, Sassorossi C, et al. Clinical stage iii nsclc patients treated with neoadjuvant therapy and surgery: The prognostic role of nodal characteristics. Life (2022) 12:1753. doi: 10.3390/life12111753
15. Jagjampi A, Khadirnaikar S, Malik PS, Jain D, N MB, Shukla S. Deepmps: Development and validation of a deep learning model for whole slide image base prognostic prediction of low grade lung adenocarcinoma patients. bioRxiv (2022) 2022:12. doi: 10.1101/2022.12.27.522072
16. Lococo F, Chiappetta M, Evangelista J, Sperduti I, Nachira D, Porziella V, et al. Role of peripheral blood markers for detecting response and predicting prognosis in patients with non-small-cell lung cancer undergoing neoadjuvant therapy and surgery. Lung (2022) 200:393–400. doi: 10.1007/s00408-022-00541-2
17. Yang L, Fan X, Qin W, Xu Y, Zou B, Fan B, et al. A novel deep learning prognostic system improves survival predictions for stage iii non-small cell lung cancer. Cancer Med (2022) 11:4246–55. doi: 10.1002/cam4.4782
18. Kingma DP, Ba J. Adam: A method for stochastic optimization. arXiv preprint arXiv (2014) 1412:6980. doi: 10.48550/arXiv.1412.6980
19. Sundararajan M, Taly A, Yan Q. (2017). Axiomatic attribution for deep networks, in: Proceedings of the 34th International Conference on Machine Learning, 70 ICML’17:3319–28. doi: 10.5555/3305890.3306024
20. Katzman JL, Shaham U, Cloninger A, Bates J, Jiang T, Kluger Y. Deepsurv: personalized treatment recommender system using a cox proportional hazards deep neural network. BMC Med Res Method (2018) 18:1–12. doi: 10.1186/s12874-018-0482-1
21. Adeoye J, Koohi-Moghadam M, Lo AWI, Tsang RKY, Chow VLY, Zheng LW, et al. Deep learning predicts the malignant-transformation-free survival of oral potentially malignant disorders. Cancers (2021) 13:6054. doi: 10.3390/cancers13236054
22. Huang C, Dai Y, Chen Q, Chen H, Lin Y, Wu J, et al. Development and validation of a deep learning model to predict survival of patients with esophageal cancer. Front Oncol (2022) 12. doi: 10.3389/fonc.2022.971190
23. Lin J, Yin M, Liu L, Gao J, Yu C, Liu X, et al. The development of a prediction model based on random survival forest for the postoperative prognosis of pancreatic cancer: A seer-based study. Cancers (2022) 14:4667. doi: 10.3390/cancers14194667
24. Schaake-Koning C, Van den Bogaert W, Dalesio O, Festen J, Hoogenhout J, van Houtte P, et al. Effects of concomitant cisplatin and radiotherapy on inoperable non-small-cell lung cancer. New Engl J Med (1992) 326:524–30. doi: 10.1056/NEJM199202203260805
25. Dillman RO, Seagren SL, Propert KJ, Guerra J, Eaton WL, Perry MC, et al. A randomized trial of induction chemotherapy plus high-dose radiation versus radiation alone in stage iii non-small-cell lung cancer. New Engl J Med (1990) 323:940–5. doi: 10.1056/NEJM199010043231403
26. Dillman RO, Herndon J, Seagren SL, Eaton WL Jr., Green MR. Improved survival in stage iii non-small-cell lung cancer: seven-year follow-up of cancer and leukemia group b (calgb) 8433 trial. JNCI. J Natl Cancer Institute (1996) 88:1210–5. doi: 10.1093/jnci/88.17.1210
27. Mulder R, Singh AB, Hamilton A, Das P, Outhred T, Morris G, et al. The limitations of using randomised controlled trials as a basis for developing treatment guidelines. Evidence-Based Ment Health (2018) 21:4–6. doi: 10.1136/eb-2017-102701
28. Pearl J. Theoretical impediments to machine learning with seven sparks from the causal revolution. arXiv preprint arXiv (2018) 1801:04016. doi: 10.1145/3159652.3176182
29. Heinze-Deml C, Meinshausen N. Conditional variance penalties and domain shift robustness. arXiv preprint arXiv (2017) 1710:11469. doi: 10.48550/arXiv.1710.11469
30. Athey SC, Bryan KA, Gans JS. (2020). The allocation of decision authority to human and artificial intelligence, in: AEA Papers and Proceedings, American Economic Association 2014 Broadway, Suite 305, Nashville, TN 37203, Vol. 110. pp. 80–4. Available at: https://papers.ssrn.com/sol3/papers.cfm?abstract_id=3517287.
31. Cui P, Athey S. Stable learning establishes some common ground between causal inference and machine learning. Nat Mach Intell (2022) 4:110–5. doi: 10.1038/s42256-022-00445-z
32. Xu R, Cui P, Shen Z, Zhang X, Zhang T. Why stable learning works? a theory of covariate shift generalization. arXiv preprint arXiv:2111.02355 (2021) 2. doi: 10.48550/arXiv.2111.02355
33. Kuang K, Cui P, Athey S, Xiong R, Li B. (2018). Stable prediction across unknown environments, in: Proceedings of the 24th ACM SIGKDD international conference on knowledge discovery & data mining, .pp. 1617–26. doi: 10.1145/3219819.3220082
Keywords: non-small cell lung cancer (NSCLC), stage-III, machine learning, survival predication, treatment recommendation, adjuvant therapy
Citation: Jin L, Zhao Q, Fu S, Cao F, Hou B and Ma J (2023) Development and validation of machine learning models to predict survival of patients with resected stage-III NSCLC. Front. Oncol. 13:1092478. doi: 10.3389/fonc.2023.1092478
Received: 08 November 2022; Accepted: 13 February 2023;
Published: 13 March 2023.
Edited by:
Lal Hussain, University of Azad Jammu and Kashmir, PakistanReviewed by:
Peng-Chan Lin, National Cheng Kung University, TaiwanCopyright © 2023 Jin, Zhao, Fu, Cao, Hou and Ma. This is an open-access article distributed under the terms of the Creative Commons Attribution License (CC BY). The use, distribution or reproduction in other forums is permitted, provided the original author(s) and the copyright owner(s) are credited and that the original publication in this journal is cited, in accordance with accepted academic practice. No use, distribution or reproduction is permitted which does not comply with these terms.
*Correspondence: Jia Ma, bWFqaWExMTEwQHNpbmEuY29t
†These authors have contributed equally to this work
Disclaimer: All claims expressed in this article are solely those of the authors and do not necessarily represent those of their affiliated organizations, or those of the publisher, the editors and the reviewers. Any product that may be evaluated in this article or claim that may be made by its manufacturer is not guaranteed or endorsed by the publisher.
Research integrity at Frontiers
Learn more about the work of our research integrity team to safeguard the quality of each article we publish.