- 1Department of Neurosurgery, Huashan Hospital, Shanghai Medical College, Fudan University, Shanghai, China
- 2National Center for Neurological Disorders, Shanghai, China
- 3Neurosurgical Institute of Fudan University, Shanghai, China
- 4Shanghai Clinical Medical Center of Neurosurgery, Shanghai, China
- 5Shanghai Key Laboratory of Brain Function Restoration and Neural Regeneration, Shanghai, China
- 6Research Units of New Technologies of Micro-Endoscopy Combination in Skull Base Surgery, Chinese Academy of Medical Sciences (CAMS), Shanghai, China
- 7Institute of Neuroscience, National Yang Ming Chiao Tung University, Hsinchu, Taiwan
- 8Department of Neurosurgery, Neurological Institute, Taipei Veterans General Hospital, Taipei, Taiwan
- 9Magnetic Resonance (MR) Collaboration, Siemens Healthineers Ltd., Shanghai, China
- 10Department of Neurosurgery, National Regional Medical Center, Fudan University Huashan Hospital, Fuzhou, Fujian, China
Cerebral neoplasms like gliomas may cause intracranial pressure increasing, neural tract deviation, infiltration, or destruction in peritumoral areas, leading to neuro-functional deficits. Novel tracking technology, such as DTI, can objectively reveal and visualize three-dimensional white matter trajectories; in combination with intraoperative navigation, it can help achieve maximum resection whilst minimizing neurological deficit. Since the reconstruction of DTI raw data largely relies on the technical engineering and anatomical experience of the operator; it is time-consuming and prone to operator-induced bias. Here, we develop new user-friendly software to automatically segment and reconstruct functionally active areas to facilitate precise surgery. In this pilot trial, we used an in-house developed software (DiffusionGo) specially designed for neurosurgeons, which integrated a reliable diffusion-weighted image (DWI) preprocessing pipeline that embedded several functionalities from software packages of FSL, MRtrix3, and ANTs. The preprocessing pipeline is as follows: 1. DWI denoising, 2. Gibbs-ringing removing, 3. Susceptibility distortion correction (process if opposite polarity data were acquired), 4. Eddy current and motion correction, and 5. Bias correction. Then, this fully automatic multiple assigned criteria algorithms for fiber tracking were used to achieve easy modeling and assist precision surgery. We demonstrated the application with three language-related cases in three different centers, including a left frontal, a left temporal, and a left frontal-temporal glioma, to achieve a favorable surgical outcome with language function preservation or recovery. The DTI tracking result using DiffusionGo showed robust consistency with direct cortical stimulation (DCS) finding. We believe that this fully automatic processing pipeline provides the neurosurgeon with a solution that may reduce time costs and operating errors and improve care quality and surgical procedure quality across different neurosurgical centers.
1 Introduction
Glioma is the most common malignant intradural tumor, with a new incidence of about 4 per 100,000 people worldwide every year (1). Surgery is the first-line treatment for debulking tumors and obtaining tissues for pathology analysis. It has widely been agreed that the extent of tumor removal is positively correlated with patient survival and that residues surrounding the tumor margin always lead to early recurrence (2, 3). However, glioma grows infiltratively along fiber tracts, making it difficult to determine the tumor boundary only according to surgeons’ experiences. Extended resection may impair eloquent brain areas and cause functional disorders such as hemiplegia and aphasia. Therefore, precise tracing of tumor boundary is the key to balancing the survival and quality of life of glioma patients (4).
Diffusion tensor imaging (DTI) is a noninvasive technique that can probe the molecular diffusivity of water within the white matter to reflect the intravoxel architecture by measuring the water self-diffusion tensor (5, 6). Linking the anisotropic orientation determined by the principal eigenvector of the tensor has been widely applied to map neuronal tracts (7, 8). This method has been used to reveal and visualize three-dimensional white matter trajectories and provides crucial information to neurosurgeons for neurosurgical planning and navigation (9, 10). Thus, DTI tractography has been considered routine for many neurosurgical procedures.
Many imaging techniques and surgical adjuncts, such as integrated neuro-navigation with DTI or blood oxygen level-dependent functional magnetic resonance imaging (BOLD-fMRI), have been developed to delineate the tumor margin and protect eloquent areas to avoid increasing postoperative deficits during aggressive tumor resection (11). Currently, these techniques are routinely applied in many neurosurgical procedures for cortical eloquence and white matter assessment. However, the complexity of imaging processing will increase the clinical burden, and insufficient experience in imaging processing in some clinical centers may misguide the surgical procedure, leading to consequent complications. Therefore, a simple, automatic, less time-consuming, high-accuracy imaging processing and easy-to use software is needed to reduce the clinical burden for the neurosurgeon and narrow the gap between different clinical centers.
Imaging quality and preprocessing procedures are crucial to obtain robust and reliable tractography for neurosurgery. Despite having a few helpful workstations provided by the magnetic resonance imaging (MRI) machine vendors and powerful software (such as MRtrix3, FSL, DSI-studio, 3D-Slicer, etc.) used for reconstructing neural tractography, complicated processing procedures, and reconstructing reliability have hindered its clinical applications, especially for neurosurgery (12). A well-trained technician or surgeon must integrate different software programs for surgical planning based on prior anatomical knowledge, which is time-consuming and prone to operator-induced bias (13). Thus, an automatic imaging processing pipeline and fiber tractography segmentation tool are necessary.
Here, we develop an in-house software, “DiffusionGo” that integrates a fully automatic preprocessing pipeline for diffusion MRI data and a boosted tractography algorithm to achieve efficient and reliable modeling. In this pilot trial, the surgical plan of three language-related cases from multicenter, including one left frontal-temporal-insular glioma, one left temporal glioma, and one left frontal-insular glioma, was reconstructed by DiffusionGo, and favorable surgical outcomes with language function preservation was achieved.
2 Materials and methods
2.1 Study design and patient recruitment
This was a multicenter, observational, prospective pilot trial. We report three patients with preoperatively imaging-diagnosed gliomas who underwent surgical resection at Huashan Hospital (Shanghai, China), Taipei Veterans General Hospital (Taipei, Taiwan), and the First Affiliated Hospital of Fujian Medical University (Fujian, China) between December 2020 and March 2022. We collected clinical, imaging, treatment, and outcome data. This study was approved by the local Hospital Ethics Committee (Approval No. KY2021-452 and KY2019-008) and was conducted under the Declaration of Helsinki. All patients were verbally informed, and the signature of a specific informed consent was obtained.
2.2 Imaging acquisition
As a pilot trial, three typical cases from three different centers, respectively, with completed MRI and clinical assessment, were described here. The MRI images of the first case were acquired on a 3.0 Tesla MRI scanner (Magnetom Verio; Siemens, Erlangen, Germany) with a 12-channel head coil at Huashan Hospital, Shanghai, China, including high-resolution three-dimensional T1-weighted images (T1WI, TR = 1630 ms; TE = 2.9 ms; flip angle = 9°; field of view (FOV) = 172 x 250 x 176 mm3; voxel size = 1 x 1 x 1 mm3), diffusion-weighted images (DWI, TR = 6500 ms; TE = 95 ms; FOV = 220 x 220 x 140 mm3; voxel size = 2 x 2 x 2 mm3, 30 directions of b value = 1000 s/mm2; average = 2).
The MRI images of the second case were acquired on a 3.0 Tesla MRI scanner (Siemens Magnetom Tim Trio, Erlangen, Germany) at National Yang Ming Chiao Tung University, Taipei, Taiwan, using a 12-channel head array coil. High-resolution T1W images were acquired using a 3D magnetization-prepared rapid gradient echo sequence (MPRAGE, TR/TE = 2530/3.5 ms; TI = 1100 ms; FOV = 256 mm; voxel size = 1 x1 x 1 mm3; flip angle = 7°) for image segmentation, registration, and brain mask extraction. Multishelled, multiband DWIs were acquired using a single-shot spin-echo planar imaging sequence (monopolar scheme; TR = 3525 ms; TE = 109.2 ms; FOV = 240 x 240 x 144 mm3; voxel size: 2 × 2 × 2 mm3; multiband factor = 3; phase encoding: anterior to posterior) with two b-values of 1000 s/mm2 (30 diffusion directions) and 3000 s/mm2 (60 diffusion directions), in which b0 images were interleaved in every six volumes. Data with the same DWI protocol using an opposite polarity (phase encoding from posterior to anterior) were also acquired for three B0 images.
The MRI images of the third case were acquired on a 3.0 Tesla MRI scanner (Siemens Magnetom Prisma, Erlangen, Germany) at the First Affiliated Hospital of Fujian Medical University, Fuzhou, Fujian, China, using a 64-channel head array coil. High-resolution T1W images with a 3D MPRAGE sequence (TR/TE = 2300/2.32 ms; TI = 946 ms; FOV = 240 mm; voxel size = 0.94 x0.94 x 0.90 mm3; flip angle = 8) and DWI with a single shot spin-echo planar imaging sequence (monopolar scheme; TR = 3700 ms; TE = 92 ms; FOV = 220 x 220 x 130 mm3; voxel size: 1.72 × 1.72 × 5.2 mm3 with 20 diffusion directions and b-values of 1000 s/mm2) were acquired.
2.3 Automatic bundle-specific neuro-fiber tractography by DiffusionGo
DiffusionGo integrates a reliable preprocessing pipeline with a fully automatic multiple assigned criteria algorithm for bundle-specific tractography using DTI data based on anatomical connectivity (14). First, structural (typo) images were coregistered with DWI by using Advanced Normalization Tools (ANTs, http://stnava.github.io/ANTs/). All DWIs underwent diffusion preprocessing pipeline and DTI model fitting with MRtrix3 (https://www.mrtrix.org) (15) and FSL (https://fsl.fmrib.ox.ac.uk/fsl/fslwiki) (16): 1. DWI denoising (17–19), 2. Gibbs-ringing removing (20), 3. Susceptibility distortion correction (process if opposite polarity data were acquired), 4. Eddy current and motion correction (21), 5. Bias correction (22), and 6. DTI fitting. A patent-protected multiple assigned criteria (MAC) algorithm (14) for fiber tracking was used. The motor pathway (corticospinal tract, CST), language pathway (arcuate fasciculus, AF, superior longitudinal fasciculus, SLF, frontal aslant tract, FAT, inferior longitudinal fasciculus, ILF, inferior fronto-occipital fasciculus, IFOF, and uncinate fasciculus, UF), and visual pathway (optic radiation, OR) were segmented automatically. The potential false-positive tracts were identified and manually removed by experiences neurosurgeon. The workflow is demonstrated in Figure 1. Validation results for the DiffusionGo automatic fiber tractography are summarised in the Supplementary Material.
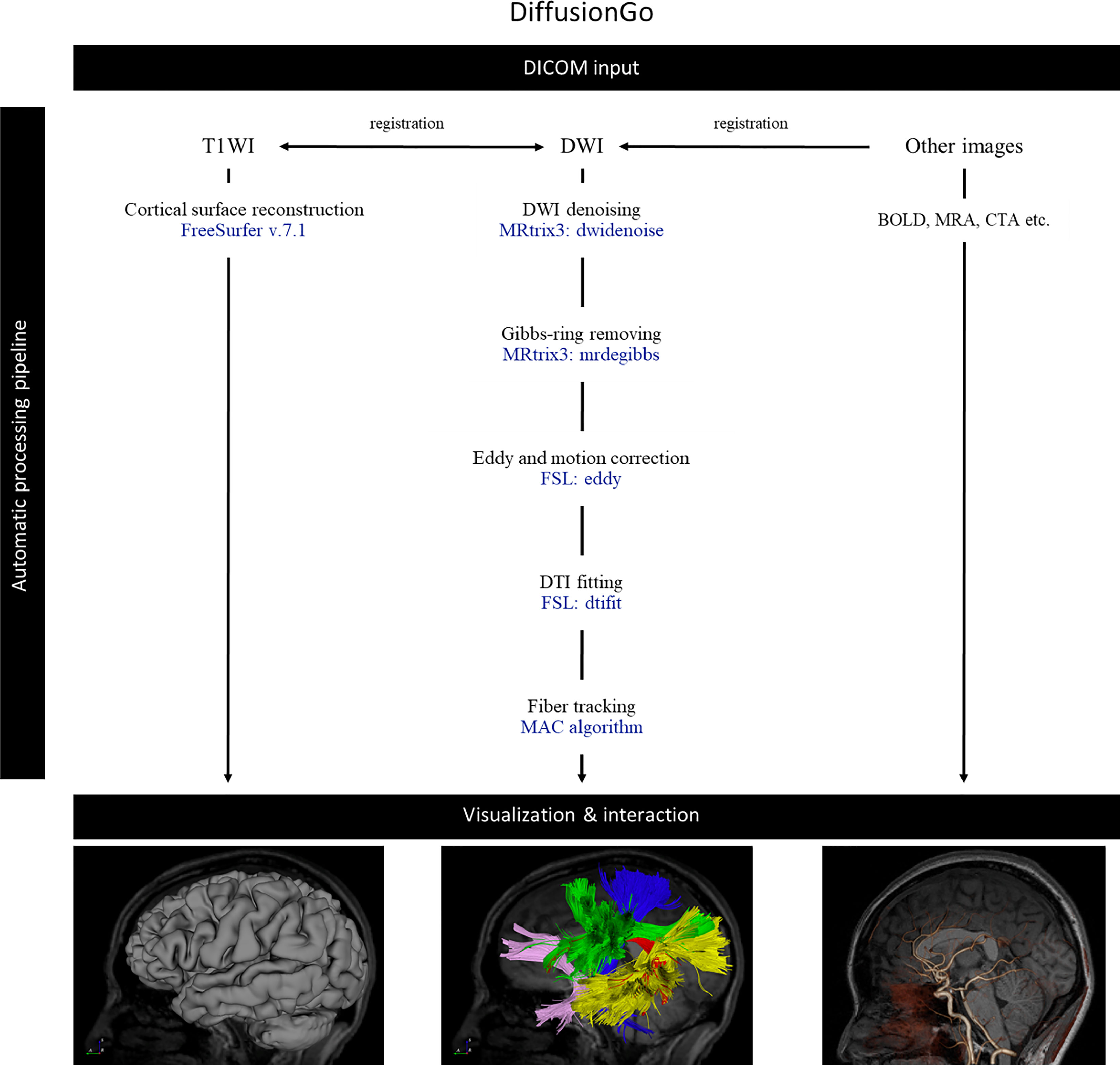
Figure 1 The automatic pipeline of DiffusionGo. MAC, multiple assigned criteria; DICOM, digital imaging and communications in medicine; DSA, digital subtraction angiography; DWI, diffusion weighted image; DTI, diffusion tensor imaging; BOLD, blood oxygen level-dependent.
2.4 Three-dimensional visualization
The cortical surface was reconstructed by FreeSurfer (version 7.1, https://surfer.nmr.mgh.harvard.edu) (23) and integrated into DiffusionGo with DTI tractography to build 3D model visualization.
3 Case study
Here, three exemplary cases from three different neurosurgical centers were demonstrated respectively. The first case was a 42-year-old woman with left frontal-temporal-insular lobe astrocytoma in Huashan Hospital. The lesion was about 79mm in diameter with high signal in T2 and not enhanced after contrast; and had a close relationship to the speech output language area with high surgical risk. Considering the tumor might not be highly aggressive and malignant and was more sensitive to subsequent radiotherapy and chemotherapy, we focused more on functional protection to maintain a relatively high living quality for the patient. DTI fiber tractography and conventional MRI sequences were integrated with DiffusionGo for surgical planning (Figure 2). Multimodality-guided awake surgery under electrophysiology monitoring for language function mapping and preservation was used. Speech arrest was defined as discontinuing number counting without simultaneous motor response by direct cortical stimulation (DCS). In the language mapping phase, we found that the eloquent area of speech arrest was located in the classical Broca’s area as the terminal territory of our reconstructed AF. The surgery was conducted under awake surgery, and the tumor subtotally resected. There was no language dysfunction during the whole procedure. The patients was finally diagnosed as astrocytoma, WHO grade 2, IDH mutant. For this case, the automatic algorithm was used to reconstruct the AF and SLF-II, which were considered the major white matter tracts adjacent to the lesion.
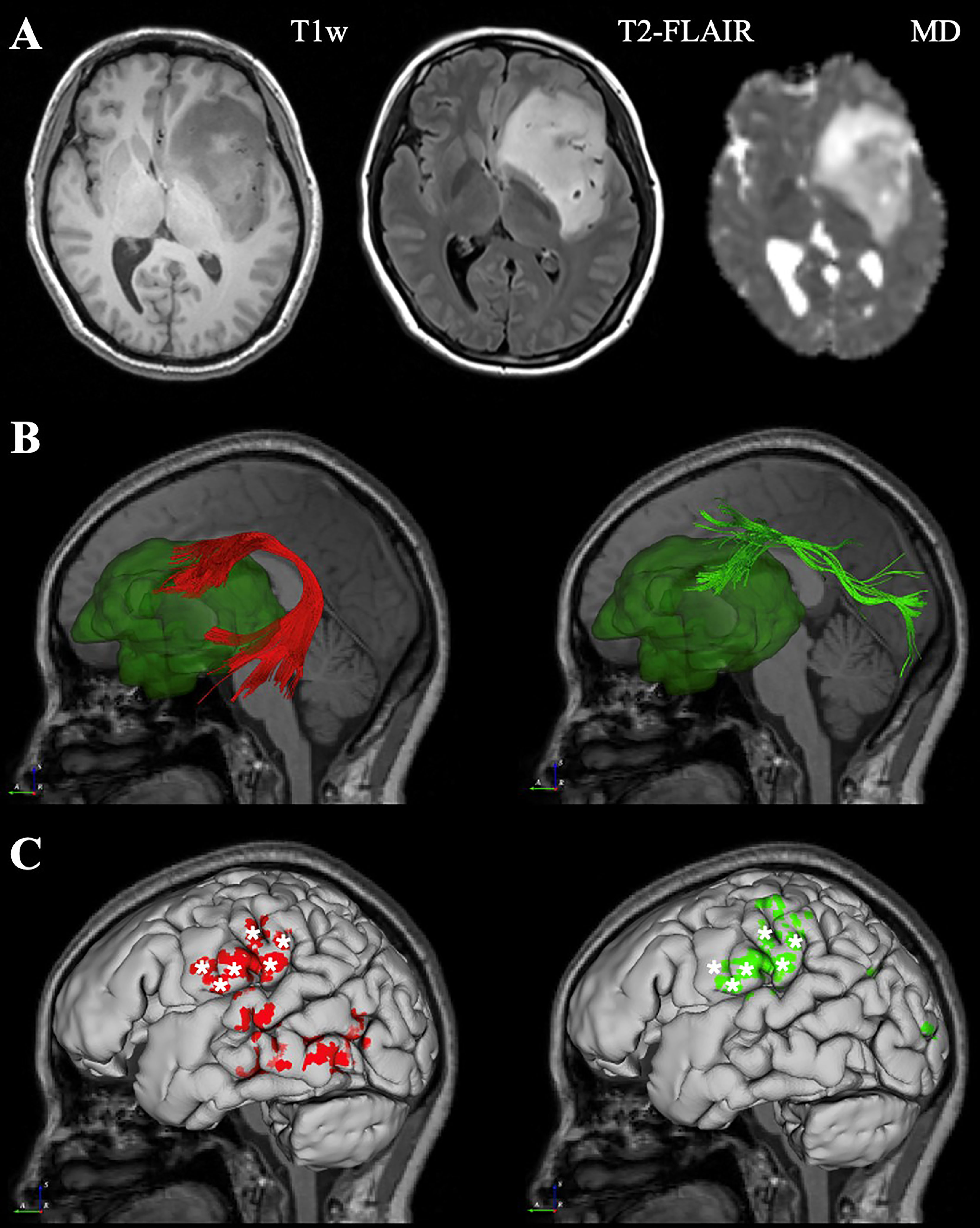
Figure 2 Representive imaging of a patient with left frontal-temporal lobe astrocytoma. Case 1, a 42-year-old woman with left frontal-temporal lobe astrocytoma (WHO grade II) in MR images (A). The relationship between language-related fiber tracts (AF in red and SLF-II in green color) and tumor (dark green color) were shown in (B). The cortical termination of each tract was projected on the cortical surface (C). The eloquent area of intro-operative speech arrest (DCS) was marked with stars. (MD, Mean Diffusivity).
The second case was diagnosed and treated at Taipei Veterans General Hospital. This 41-year-old woman suffered from intermittent headaches, which progressed gradually. In addition, she could not write or read words that she knew. Ignoring the objects on her right side was also noted. She went to the clinic, and MRI of the brain showed a heterogeneous mass, 55 mm in diameter, over the left temporal lobe with mild perifocal edema. DTI fiber tractography of the language-related pathways (AF, and SLF-II) was reconstructed and displayed in DiffusionGo. Superior displacement of the left Wernicke’s area was identified with intact AF projecting to the left premotor and left Broca areas (Figure 3). After a complete survey, since the patient could not endure an awake surgery, tumor removal was performed under general anesthesia without any neurological deficits postoperatively. MRI of the brain revealed total gross removal without residual tumor. Unfortunately, glioblastoma was diagnosed.
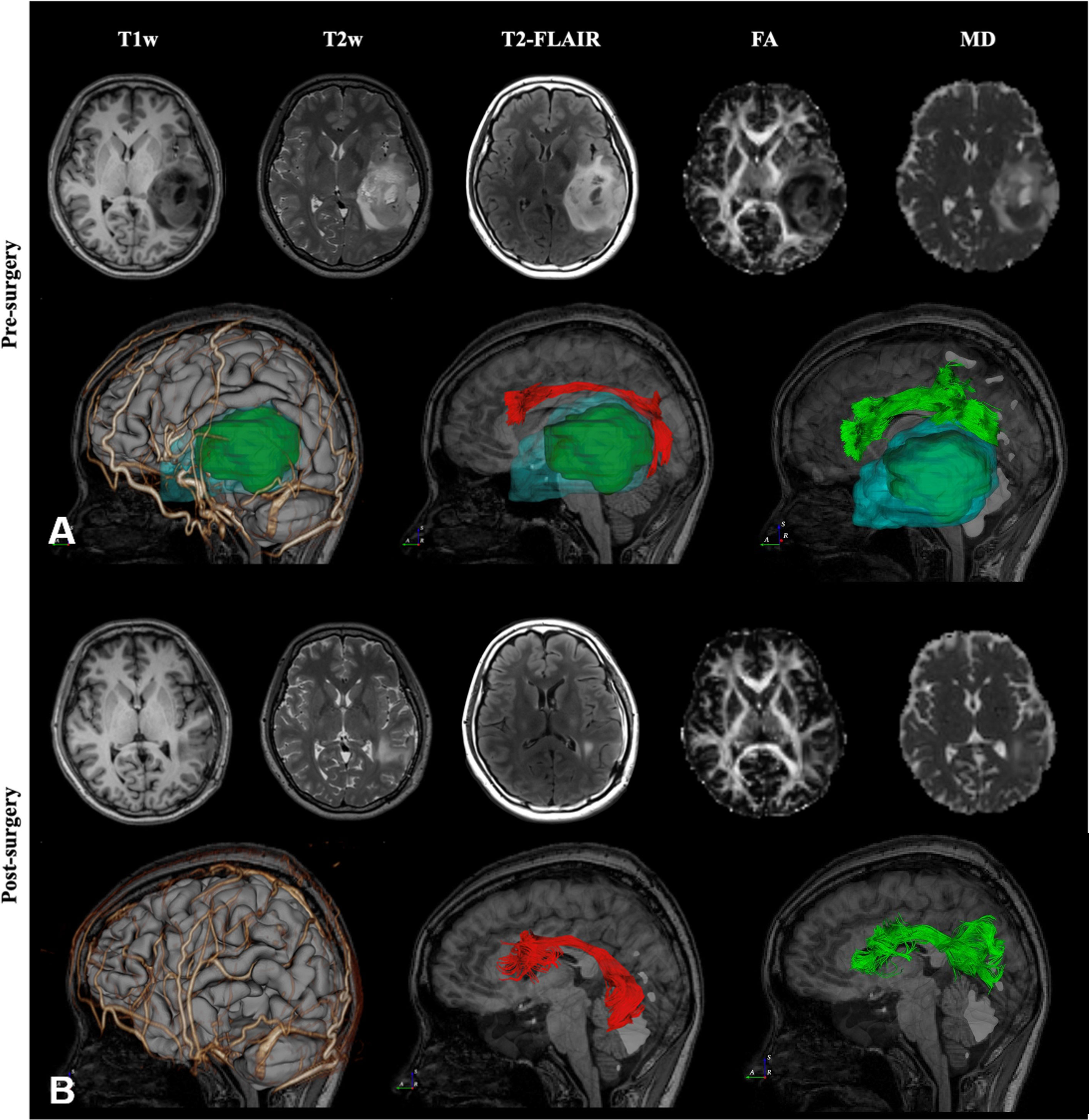
Figure 3 Preoperative and post-operative imaging of a patient with left temporal lobe astrocytoma. Case 2, A 41-year-old woman with temporal lobe glioblastoma over the left temporal lobe with mild perifocal edema was reconstructed and displayed in DiffusionGo (A). The AF (red) and SLF-II (green) were automatically reconstructed and integrated with the cortical surface (gray), arteries and veins (gold), tumor (dark green), and peritumoral edema (light blue with translucent). Superior displacement of the left Wernicke’s area was identified with intact AF projecting to the left premotor and left Broca’s areas. After a complete survey, since the patient could not endure an awake surgery, tumor removal was performed under general anesthesia without any neurological deficits postoperatively. Two months later, MRI of the brain revealed total gross removal without residual tumor, and DTI showed preservation of the AF (red) and SLF-II (green) (B). (FA, Fractional Anisotropy).
The third case was recently conducted at the First Affiliated Hospital of Fujian Medical University. This 41-year-old female suffered from recurrent seizures attack for 4 years. Conventional MR indicates a left frontal-insular lesion of 3.8 cm, with high signal in T2WI and not enhanced after contrast. Preoperative DTI showed the AF and SLF were located below and behind the tumor, respectively (Figure 4). Considering the close relationship between the lesion and Broca’s region, multimodality-guided awake surgery under electrophysiology monitoring for language function mapping and preservation was conducted. After craniotomy, in the language mapping phase using DCS, we found that the eloquent area of speech arrest was located in the terminal territory of our reconstructed AF and SLF. The surgery was conducted under awake surgery. Since there was no clear boundary between the tumor and the eloquent area and SLF behind the tumor, a subtotal resection of tumor was achieved. There was no language dysfunction during the whole procedure. The pathology showed anaplastic astrocytoma, and the patient was discharged for adjuvant radiotherapy.
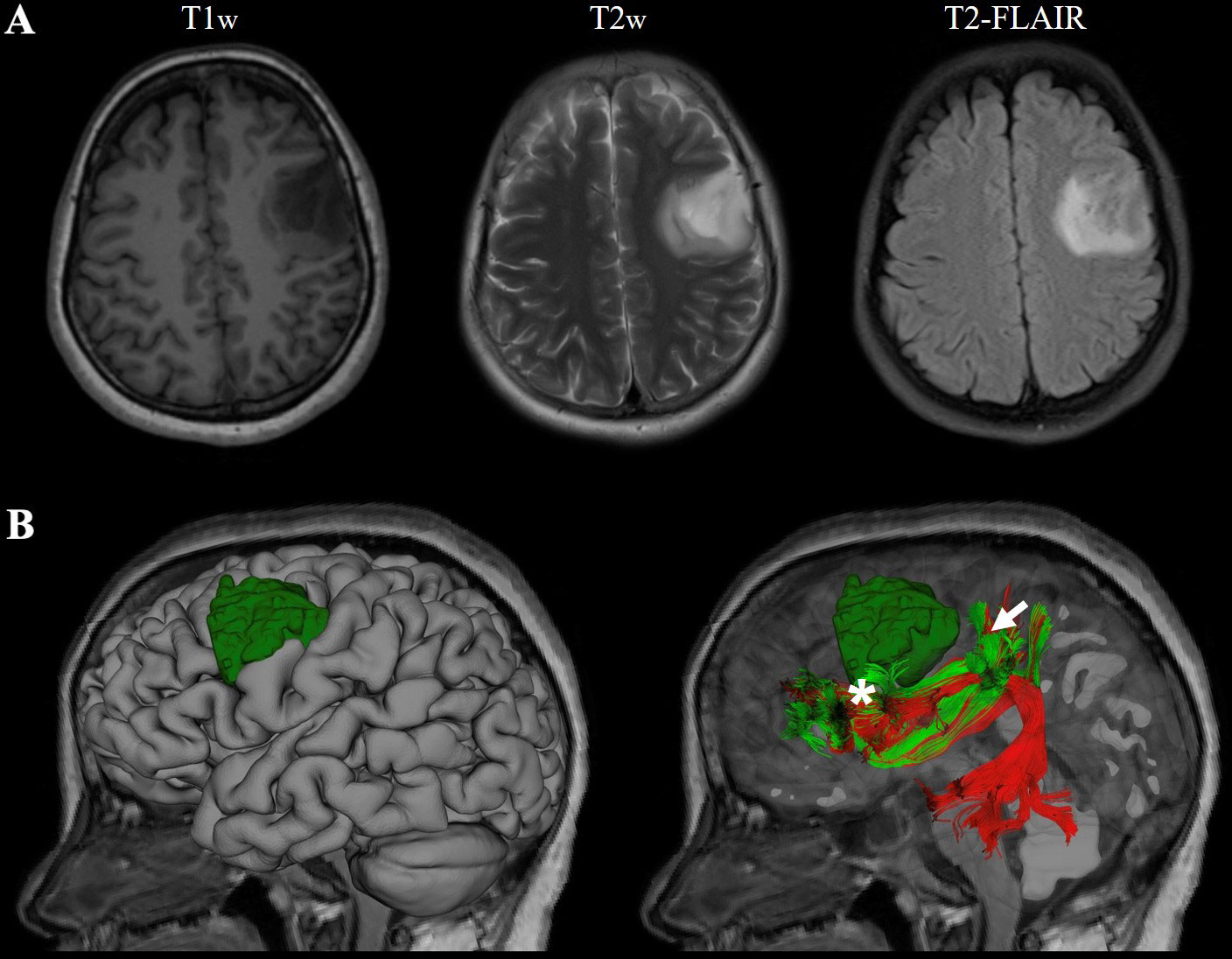
Figure 4 Representive imaging of a patient with left frontal lobe astrocytoma. Case 3, a 41-year-old female with left frontal lobe astrocytoma (WHO grade III) in MR images (A). The relationship between language-related fiber tracts (AF in red and SLF-II in green color) and tumor (dark green) were shown in (B); the eloquent area of intro-operative speech arrest (DCS) was marked with a star(Projecting terminal territory of AF) and an arrow (Projecting terminal territory of SLF-II).
4 Discussion
Sensorimotor and language eloquence are considered higher brain function, and even mild impairment causes poor outcomes (24). Unlike the sensorimotor area, the eloquent language area is more diverse, and currently, researchers have revealed that it is quite different from classical canonical classification (25, 26). Classic language eloquent models posited that motor and sensory language cortex existed in Broca’s area (including pars triangularis and pars opercularis) and Wernicke’s area, respectively (26). However, cortical maps generated with intraoperative direct cortical stimulation (DCS) data revealed extraordinary variability in language localization in the dominant hemisphere, and the eloquent language areas are quite different from the classical canonical models (27). The expression tasks are best mapped in the frontal lobe, with 100% of sensitivity and 66% of specificity with a 5-mm resolution (28). Tate et al. found that speech arrest regions (or the speech output region) seemed to be localized in the ventral premotor cortex rather than the classical Broca’s area (29). According to Wu et al. study, most Chinese speech arrest areas were located at specific language production sites, which 50% positive sites in the ventral precentral gyrus, 28% in the pars opercularis and pars triangularis. Additionally, the left middle frontal gyrus (Brodmann’s areas 6/9) was found to be unique for Chinese production. Moreover, Chinese speakers’ inferior ventral precentral gyrus (Brodmann’s area 6) was used more often than English speakers (30). Therefore, the combinational speech arrest map can be divided into four clusters: Cluster 1 was mainly located in the ventral precentral gyrus and the pars opercularis, which contained the peak of speech arrest in the ventral precentral gyrus; Cluster 2 was in the ventral and dorsal precentral gyrus; Cluster 3 was in the supplementary motor area; Cluster 4 was in the posterior superior temporal gyrus and supramarginal gyrus (31).
The white matter tracts transmit information between different cortical regions, and their connectivity enables the central nervous system to function normally. Hence, the idea of “eloquent areas” should be expanded to include deep structures rather than a purely cortical concept. BOLD-fMRI and DTI tractography have been routinely applied in many neurosurgical procedures for cortical eloquence and white matter assessment.
However, conventional DTI tractography methods rely on the technician to manually select the region of interest based on prior knowledge of anatomy, then generate the fibers from there, then clean and refine the bundle obtained. This approach is time-consuming, prone to operator-induced deviation, and without any “gold standard” (32). Therefore, the training of a specialized technician does need a learning curve. Unfortunately, most hospitals in China do not even provide this technician position due to the huge inequalty of medical resources among different neurosurgery centers (33). Although there are several automatic algorithms for whole-brain tractography, tracts derived from these algorithms can deviate significantly from each other, making it difficult to identify the most accurate one (12). A feasible automatic bundle-specific neurofiber tractography algorithm is not yet available.
Given this, we have developed an automatic bundle-specific white matter fiber tracking tool (DiffusionGo) with a fully automatic multiple assigned criteria (MAC) algorithm for bundle-specific neurofiber reconstruction (14) to achieve multimodality modeling and visualization for precision surgical planning with minimal human processing error. Previous bundle-specific tractography studies mostly used manually tractography but faced significant challenges in identifying accurate tracts, especially in cases with apparent lesion effects for neurosurgical implementation (34–36). In contrast, DiffusionGo was developed based on anatomical connectivity from clinical and autopsy data.
Modern neuroscience research has revealed a dorsal language pathway (arcuate fasciculus, AF, and superior longitudinal fasciculus II, SLF-II) using DTI tractography responsible for verbal repetition by integrating sensory-motor information. The SLF/AF system is the most comprehensive association fiber system at the lateral surface, connecting the frontal, temporal, parietal, and occipital lobes. AF was considered to connect the classic Broca’s area and Wernicke’s area. Specifically, the SLF connects the frontal and parietal lobes, allowing communication between the dorsal premotor and prefrontal cortices to the angular gyrus. The SLF also contains frontal-to-parietal connections terminating within the supramarginal gyrus (26).
In these three cases, both AF and SLF were automatically generated using DiffusionGo. In Case 1, the actual speech arrest areas were located at the tract-based cortical termination of SLF-II. Independently, our glioma team’s study of language mapping in glioma surgery showed that in Chinese people the goodness of fit between the terminal territories of the manually tracked AF and SLF and the DSC-mapped eloquent speech output area in the pars opercularis and ventral premotor cortex was 82% and 86%, respectively (25). For Case 2 treated in another institute, although nonawake surgery was performed, the automatic tracked neurofibers still augmented surgical plans, with an exceptionally safe approach design, for removing highly invasive gliomas by analyzing the relationship between the tumor and white matter tracts. Similar results were reported previously (37) and as in Case 3.
The major limitation of this pilot study was its limited sample size and lack of a control group. However, these results did show the promising value of the clinical implementation of DiffusionGO. We are now pursuing a multicenter clinical trial of this automatic DTI tractography pipeline to prove its potential role as an efficient, clinically applicable bundle-specific tractography tool to augment technical equality and improve surgical planning precision across different hospitals in China.
5 Conclusion
We demonstrated the application of DiffusionGo in three language-related cases. The fully automatic processing pipeline may provide the technician or surgeon with a solution to reduce time cost and operating error. We believe that this promising technique can improve care quality and surgical procedure quality across different facilities.
Data availability statement
The raw data supporting the conclusions of this article will be made available by the authors, without undue reservation.
Ethics statement
The studies involving human participants were reviewed and approved by IRB of Huashan Hospital, Fudan University (KY2021-452 and KY2019-008). The patients/participants provided their written informed consent to participate in this study. Written informed consent was obtained from the individual(s) for the publication of any potentially identifiable images or data included in this article.
Author contributions
Conceptualization, JS and C-PL. Methodology, YY, TQ, SC and S-PC. Software, Y-HC, Y-CH, Y-TK and K-TK. Validation, GX, YY and ZY. Investigation, YY and TQ. Resources, WZ and C-PL. Data curation, YY and TQ. Writing—original draft preparation, YY and SC. Writing—review and editing, SC, JS and C-PL. Visualization, YY, GX, Y-TK and K-TK. Supervision, JS and C-PL. Funding acquisition, JS. All authors contributed to the article and approved the submitted version.
Funding
This study was supported and granted by the CAMS Innovation Fund for Medical Sciences (CIFMS, 2019-I2M-5-008), Fujian Province Science and Technology Innovation Joint Fund (2021Y9135) and Shanghai Municipal Science and Technology Major Project (No.2018SHZDZX01) and ZJLab and Ministry of Science and Technology (MOST) of Taiwan (MOST 111-2321-B-A49-003).
Conflict of interest
Author Y-HC and Y-CH were employed by the company Siemens Healthineers Ltd.
The remaining authors declare that the research was conducted in the absence of any commercial or financial relationships that could be construed as a potential conflict of interest.
Publisher’s note
All claims expressed in this article are solely those of the authors and do not necessarily represent those of their affiliated organizations, or those of the publisher, the editors and the reviewers. Any product that may be evaluated in this article, or claim that may be made by its manufacturer, is not guaranteed or endorsed by the publisher.
Supplementary material
The Supplementary Material for this article can be found online at: https://www.frontiersin.org/articles/10.3389/fonc.2023.1089923/full#supplementary-material
References
1. Bray F, Ferlay J, Soerjomataram I, Siegel RL, Torre LA, Jemal A. Global cancer statistics 2018: GLOBOCAN estimates of incidence and mortality worldwide for 36 cancers in 185 countries. CA Cancer J Clin (2018) 68:394–424. doi: 10.3322/caac.21492
2. Weller M, van den Bent M, Preusser M, Le Rhun E, JC T, Minniti G, et al. EANO guidelines on the diagnosis and treatment of diffuse gliomas of adulthood. Nat Rev Clin Oncol (2021) 18:170–86. doi: 10.1038/s41571-020-00447-z
3. Molinaro AM, Hervey-Jumper S, Morshed RA, Young J, Han SJ, Chunduru P, et al. Association of maximal extent of resection of contrast-enhanced and non-Contrast-Enhanced tumor with survival within molecular subgroups of patients with newly diagnosed glioblastoma. JAMA Oncol (2020) 6:495–503. doi: 10.1001/jamaoncol.2019.6143
4. Yuan Y, Yu Y, Guo Y, Chu Y, Chang J, Hsu Y, et al. Noninvasive delineation of glioma infiltration with combined 7T chemical exchange saturation transfer imaging and MR spectroscopy: A diagnostic accuracy study. Metabolites (2022) 12. doi: 10.3390/metabo12100901
5. Basser PJ, Mattiello J, LeBihan D. MR diffusion tensor spectroscopy and imaging. Biophys J (1994) 66:259–67. doi: 10.1016/S0006-3495(94)80775-1
6. Basser PJ. Inferring microstructural features and the physiological state of tissues from diffusion-weighted images. NMR BioMed (1995) 8:333–44. doi: 10.1002/nbm.1940080707
7. Mori S, van Zijl PC. Fiber tracking: Principles and strategies - a technical review. NMR BioMed (2002) 15:468–80. doi: 10.1002/nbm.781
8. Mori S, BJ C, VP C, van Zijl PC. Three-dimensional tracking of axonal projections in the brain by magnetic resonance imaging. Ann Neurol (1999) 45:265–9. doi: 10.1002/1531-8249(199902)45:2<265::aid-ana21>3.0.co;2-3
9. Bello L, Gambini A, Castellano A, Carrabba G, Acerbi F, Fava E, et al. Motor and language DTI fiber tracking combined with intraoperative subcortical mapping for surgical removal of gliomas. NEUROIMAGE (2008) 39:369–82. doi: 10.1016/j.neuroimage.2007.08.031
10. Berman JI, Berger MS, Mukherjee P, Henry RG. Diffusion-tensor imaging-guided tracking of fibers of the pyramidal tract combined with intraoperative cortical stimulation mapping in patients with gliomas. J Neurosurg (2004) 101:66–72. doi: 10.3171/jns.2004.101.1.0066
11. Wu JS, Zhang J, Zhuang DX, Yao CJ, Qiu TM, Lu JF, et al. Current status of cerebral glioma surgery in China. Chin Med J (Engl) (2011) 124:2569–77.
12. Maier-Hein KH, Neher PF, Houde JC, Cote MA, Garyfallidis E, Zhong J, et al. The challenge of mapping the human connectome based on diffusion tractography. Nat Commun (2017) 8:1349. doi: 10.1038/s41467-017-01285-x
13. Pujol S, Wells W, Pierpaoli C, Brun C, Gee J, Cheng G, et al. The DTI challenge: Toward standardized evaluation of diffusion tensor imaging tractography for neurosurgery. J Neuroimaging (2015) 25:875–82. doi: 10.1111/jon.12283
14. Lin C-P, Chong S-T, Lo C, Huang C. Method and apparatus of fiber tracking, and non-transitory computer-readable medium thereof. (2019).
15. Tournier JD, Smith R, Raffelt D, Tabbara R, Dhollander T, Pietsch M, et al. MRtrix3: A fast, flexible and open software framework for medical image processing and visualisation. NEUROIMAGE (2019) 202:116137. doi: 10.1016/j.neuroimage.2019.116137
16. Jenkinson M, Beckmann CF, Behrens TE, Woolrich MW, Smith SM. FSL. NEUROIMAGE (2012) 62:782–90. doi: 10.1016/j.neuroimage.2011.09.015
17. Veraart J, Fieremans E, Novikov DS. Diffusion MRI noise mapping using random matrix theory. Magn Reson Med (2016) 76:1582–93. doi: 10.1002/mrm.26059
18. Cordero-Grande L, Christiaens D, Hutter J, Price AN, Hajnal JV. Complex diffusion-weighted image estimation via matrix recovery under general noise models. NEUROIMAGE (2019) 200:391–404. doi: 10.1016/j.neuroimage.2019.06.039
19. Veraart J, Novikov DS, Christiaens D, Ades-Aron B, Sijbers J, Fieremans E. Denoising of diffusion MRI using random matrix theory. NEUROIMAGE (2016) 142:394–406. doi: 10.1016/j.neuroimage.2016.08.016
20. Kellner E, Dhital B, Kiselev VG, Reisert M. Gibbs-Ringing artifact removal based on local subvoxel-shifts. Magn Reson Med (2016) 76:1574–81. doi: 10.1002/mrm.26054
21. Graham MS, Drobnjak I, Jenkinson M, Zhang H. Quantitative assessment of the susceptibility artefact and its interaction with motion in diffusion MRI. PLoS One (2017) 12:e185647. doi: 10.1371/journal.pone.0185647
22. Tustison NJ, Avants BB, Cook PA, Zheng Y, Egan A, Yushkevich PA, et al. N4ITK: improved N3 bias correction. IEEE Trans Med Imaging (2010) 29:1310–20. doi: 10.1109/TMI.2010.2046908
24. Mascitelli JR, Yoon S, Cole TS, Kim H, Lawton MT. Does eloquence subtype influence outcome following arteriovenous malformation surgery? J Neurosurg (2018) 131:876–83. doi: 10.3171/2018.4.JNS18403
25. Wu J, Lu J, Zhang H, Zhang J, Mao Y, Zhou L. Probabilistic map of language regions: Challenge and implication. BRAIN (2015) 138:e337. doi: 10.1093/brain/awu247
26. Chang EF, Raygor KP, Berger MS. Contemporary model of language organization: An overview for neurosurgeons. J Neurosurg (2015) 122:250–61. doi: 10.3171/2014.10.JNS132647
27. Sanai N, Mirzadeh Z, Berger MS. Functional outcome after language mapping for glioma resection. N Engl J Med (2008) 358:18–27. doi: 10.1056/NEJMoa067819
28. Gamble AJ, Schaffer SG, Nardi DJ, Chalif DJ, Katz J, Dehdashti AR. Awake craniotomy in arteriovenous malformation surgery: The usefulness of cortical and subcortical mapping of language function in selected patients. World Neurosurg (2015) 84:1394–401. doi: 10.1016/j.wneu.2015.06.059
29. Tate MC, Herbet G, Moritz-Gasser S, Tate JE, Duffau H. Probabilistic map of critical functional regions of the human cerebral cortex: Broca's area revisited. BRAIN (2014) 137:2773–82. doi: 10.1093/brain/awu168
30. Wu J, Lu J, Zhang H, Zhang J, Yao C, Zhuang D, et al. Direct evidence from intraoperative electrocortical stimulation indicates shared and distinct speech production center between Chinese and English languages. Hum Brain MAPP (2015) 36:4972–85. doi: 10.1002/hbm.22991
31. Lu J, Zhao Z, Zhang J, Wu B, Zhu Y, Chang EF, et al. Functional maps of direct electrical stimulation-induced speech arrest and anomia: A multicentre retrospective study. BRAIN (2021) 144:2541–53. doi: 10.1093/brain/awab125
32. Rathore RK, Gupta RK, Agarwal S, Trivedi R, Tripathi RP, Awasthi R. Principal eigenvector field segmentation for reproducible diffusion tensor tractography of white matter structures. Magn Reson Imaging (2011) 29:1088–100. doi: 10.1016/j.mri.2011.04.014
33. Shi A, Zhou X, Xie Z, Mou H, Ouyang Q, Wang D. Internet Plus health care's role in reducing the inequality of high-quality medical resources in China. Asia Pacific J Public Health (2021) 33(8):997–8. doi: 10.1177/10105395211044954
34. Nazem-Zadeh MR, Davoodi-Bojd E, Soltanian-Zadeh H. Atlas-based fiber bundle segmentation using principal diffusion directions and spherical harmonic coefficients. NEUROIMAGE (2011) 54(Suppl 1):S146–64. doi: 10.1016/j.neuroimage.2010.09.035
35. Li H, Xue Z, Guo L, Liu T, Hunter J, Wong ST. A hybrid approach to automatic clustering of white matter fibers. NEUROIMAGE (2010) 49:1249–58. doi: 10.1016/j.neuroimage.2009.08.017
36. Fillard P, Descoteaux M, Goh A, Gouttard S, Jeurissen B, Malcolm J, et al. Quantitative evaluation of 10 tractography algorithms on a realistic diffusion MR phantom. NeuroImage (2011) 56:220–34. doi: 10.1016/j.neuroimage.2011.01.032
Keywords: awake neurosurgery, brain mapping, diffusion tensor imaging, functional neuroimaging, white matter tracts fiber tracking software for neurosurgeon
Citation: Yuan Y, Qiu T, Chong ST, Hsu SP-C, Chu Y-H, Hsu Y-C, Xu G, Ko Y-T, Kuo K-T, Yang Z, Zhu W, Lin C-P and Song J (2023) Automatic bundle-specific white matter fiber tracking tool using diffusion tensor imaging data: A pilot trial in the application of language-related glioma resection. Front. Oncol. 13:1089923. doi: 10.3389/fonc.2023.1089923
Received: 04 November 2022; Accepted: 03 March 2023;
Published: 24 March 2023.
Edited by:
Yan Qu, Air Force Military Medical University, ChinaReviewed by:
Laura Mancini, University College London Hospitals NHS Foundation Trust, United KingdomHaining Zhen, Fourth Military Medical University, China
Copyright © 2023 Yuan, Qiu, Chong, Hsu, Chu, Hsu, Xu, Ko, Kuo, Yang, Zhu, Lin and Song. This is an open-access article distributed under the terms of the Creative Commons Attribution License (CC BY). The use, distribution or reproduction in other forums is permitted, provided the original author(s) and the copyright owner(s) are credited and that the original publication in this journal is cited, in accordance with accepted academic practice. No use, distribution or reproduction is permitted which does not comply with these terms.
*Correspondence: Ching-Po Lin, Y2hpbmdwb2xpbkBnbWFpbC5jb20=; Jianping Song, bmV1cm9zdXJnZXJ5c29uZ0Bmb3htYWlsLmNvbQ==
†These authors have contributed equally to this work