Commentary: Genomic, epigenomic, and transcriptomic signatures of prostate cancer between African American and European American patients
- 1Department of Biochemistry and Molecular Medicine, USC Norris Comprehensive Cancer Center, Keck School of Medicine of USC, Los Angeles, CA, United States
- 2CaRE2 Program, Florida-California Health Equity Center, Los Angeles, CA, United States
- 3Department of Epidemiology and Biostatistics, College of Pharmacy and Pharmaceutical Sciences, Institute of Public Health, Florida A&M University, Tallahassee, FL, United States
- 4Department of Pathology, Immunology, and Laboratory Medicine, University of Florida College of Medicine, Gainesville, FL, United States
Prostate cancer is the second most common cancer in men in the United States, and racial disparities are greatly observed in the disease. Specifically, African American (AA) patients have 60% higher incidence and mortality rates, in addition to higher grade and stage prostate tumors, than European American (EA) patients. In order to narrow the gap between clinical outcomes for these two populations, genetic and molecular signatures contributing to this disparity have been characterized. Over the past decade, profiles of prostate tumor samples from different ethnic groups have been developed using molecular and functional assays coupled with next generation sequencing or microarrays. Comparative genome-wide analyses of genomic, epigenomic, and transcriptomic profiles from prostate tumor samples have uncovered potential race-specific mutations, copy number alterations, DNA methylation, and gene expression patterns. In this study, we reviewed over 20 published studies that examined the aforementioned molecular contributions to racial disparities in AA and EA prostate cancer patients. The reviewed genomic studies revealed mutations, deletions, amplifications, duplications, or fusion genes differentially enriched in AA patients relative to EA patients. Commonly reported genomic alterations included mutations or copy number alterations of FOXA1, KMT2D, SPOP, MYC, PTEN, TP53, ZFHX3, and the TMPRSS2-ERG fusion. The reviewed epigenomic studies identified that CpG sites near the promoters of PMEPA1, RARB, SNRPN, and TIMP3 genes were differentially methylated between AA and EA patients. Lastly, the reviewed transcriptomic studies identified genes (e.g. CCL4, CHRM3, CRYBB2, CXCR4, GALR1, GSTM3, SPINK1) and signaling pathways dysregulated between AA and EA patients. The most frequently found dysregulated pathways were involved in immune and inflammatory responses and neuroactive ligand signaling. Overall, we observed that the genomic, epigenomic, and transcriptomic alterations evaluated between AA and EA prostate cancer patients varied between studies, highlighting the impact of using different methods and sample sizes. The reported genomic, epigenomic, and transcriptomic alterations do not only uncover molecular mechanisms of tumorigenesis but also provide researchers and clinicians valuable resources to identify novel biomarkers and treatment modalities to improve the disparity of clinical outcomes between AA and EA patients.
1 Introduction
Prostate cancer is the second most common cancer in men with one of the highest incidence rates in the United States (1). It has the second highest mortality rate relative to other malignancies, but the severity of clinical outcomes is reported to vary by race and ethnicity (1). Specifically, African American (AA) men have the highest prostate cancer incidence rate amongst racial or ethnic groups in the United States (2). For example, 1 in 6 AA men are diagnosed with prostate cancer in their lifetime, compared to 1 in 8 European American (EA) men, and the incidence is nearly 60% higher for AA men (2). Moreover, AA prostate cancer patients present with higher grade and stage tumors and have a nearly 2-fold higher mortality rate when compared to EA prostate cancer patients (2). Specifically, AA men have a prostate cancer mortality rate of 37.4 per 100,000 in the period of 2014-2018 versus 19.3 per 100,000 among white non-Hispanic men (3). Furthermore, prostate cancer has a higher growth and metastatic transformation rate for AA men compared to EA men (4). It has been reported that low-grade prostate cancer cells grow and spread more quickly in AA than men of other races (5).
This discrepancy between clinical outcomes appears to be attributable to socioeconomic factors that may cause barriers to medical access, diagnosis, and treatment among AA men (6). For example, AA men experience substandard testing of prostate specific antigen (PSA) relative to their EA counterparts, leading to limited access to early detection of prostate cancer (7–9). In addition to socioeconomic factors, biological factors may further widen the gap between clinical outcomes for AA men relative to EA counterparts. For example, Cheng et al. reported that AA men were more likely to develop prostate cancer relative to their EA counterparts in California after correcting for socioeconomic status (10). Another study emphasizes that the investigation of race-specific biological differences needs to be viewed through a multifactorial lens because factors such as environment, social status, and genetic inheritance can lead to different mechanisms of prostate tumorigenesis in a combinatorial manner (11). Currently, there is lack of comprehensive understanding on the different biological contributions to prostate tumorigenesis between AA and EA patients.
To elucidate possible biological determinants of racial disparities in prostate cancer, prostate tumor cells and tissues from AA and EA patients were obtained through various methods (e.g. transrectal ultrasound (TRUS)-guided biopsy, transperineal biopsy, transurethral resection, prostatectomy, radical prostatectomy), and genetic and molecular assays have been performed with the obtained samples. Over the past two decades after next generation sequencing (NGS) technologies were introduced, prostate tumor samples have been studied using genetic and molecular assays that are coupled with sequencing. Using NGS and microarray techniques that can evaluate genome-wide signals at once, many studies have characterized genetic (e.g. mutations, copy number alterations, fusions) and molecular features (e.g. epigenetic alterations, gene expression changes) of prostate tumors through the lens of AA and EA patient outcome disparities.
Here, we have listed findings from over 20 published studies that profiled the genomes, epigenomes, and transcriptomes of prostate tumor tissues of AA and EA patients. Studies were identified by inputting the following key words into the PubMed search query: “African-American” AND “prostate cancer”. For each category (genome, epigenome, transcriptome), search terms were changed to DNA, methylation, and RNA, respectively. Studies were deemed to be within the scope of this review if they were written in the English language and performed using prostate tumor tissue samples from AA and EA patients, clearly reporting the cohort sizes and methods used. Moreover, we only included studies that directly compared genetic, epigenetic, or transcriptomic profiles of prostate tumor tissues between AA and EA patients (Tables 1–3). These studies uncovered potential race-specific mutations, copy number alterations, fusions, and aberrant DNA methylation and gene expression patterns, using varied methods of DNA, DNA methylation, and RNA analysis, respectively (Figure 1). This review, which details a bigger picture of prostate cancer biological signatures, will provide clinicians and researchers with a better understanding of molecular mechanisms of prostate tumorigenesis and facilitate the development of potential biomarkers and treatment modalities to narrow the gap between AA and EA patient outcomes.
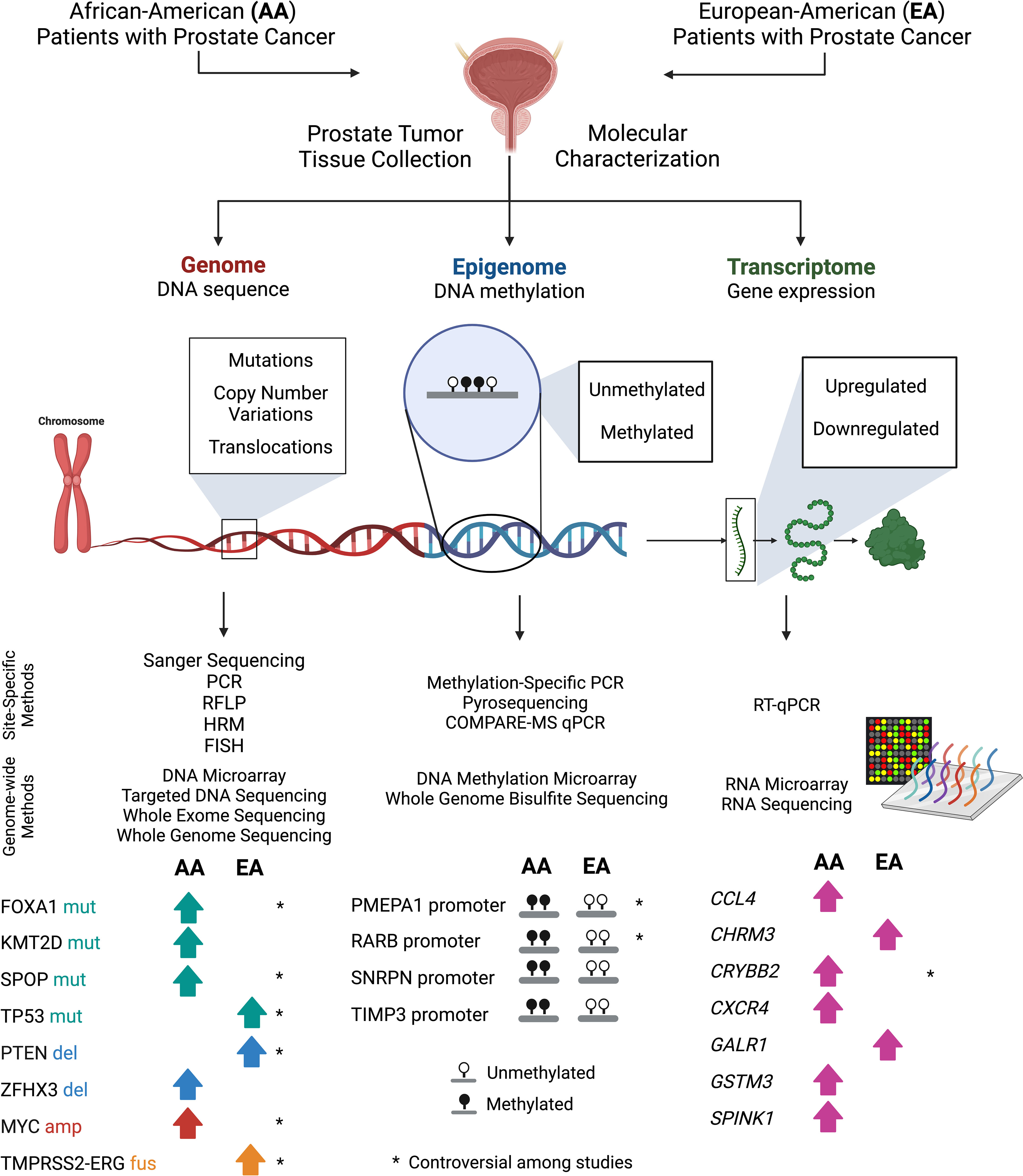
Figure 1 Approaches to compare molecular genetic signatures in prostate tumor tissue samples obtained from AA and EA patients. In reviewed studies, prostate tumor tissue samples from both EA and AA patients were collected by either biopsy or prostatectomy. DNA or RNA material was extracted from aforementioned samples and then prepared for molecular characterization at the genome, epigenome, and transcriptome levels. Studies profiled the signatures of prostate tumor tissues using site-specific techniques and genome-wide utilized high-throughput hybridization (microarray) and next-generation sequencing (NGS) technologies. Subsequently, AA and EA patient sample data was compared to uncover potential molecular differences between the two subpopulations. Key molecular differences found by several studies and discussed in this review were included. Comparisons that were not found to have consistent data across studies (or controversial) was denoted with an asterisk (*). PCR, Polymerase chain reaction; RFLP, Restriction fragment length polymorphisms; HRM, High resolution melting analysis; FISH, Fluorescence in situ hybridization; and COMPARE-MS, Combination of methylated-DNA precipitation and methylation-sensitive restriction enzymes assay.
2 Genomic alterations linked to African American prostate cancer patients
2.1 Methods to profile genomic alterations in cancer
Cancers develop due to the accumulation of genetic alterations such as mutations, amplifications, deletions, and fusions. Deletions and inactivating (loss-of-function) mutations are often found at tumor suppressor genes to dysregulate cell division. Conversely, amplifications and activating mutations are found at oncogenes to increase cancer cell proliferation and survival. Moreover, genetic alterations are observed at non-coding regions such as regulatory regions to activate or inactivate genes involved in carcinogenesis.
To identify genetic alterations in cancer cells, polymerase chain reaction (PCR)-based assays, DNA sequencing and hybridization technology have been applied. Classical techniques that have been utilized to parse out short sequences include Sanger sequencing, pyrosequencing, PCR-restriction fragment length polymorphisms (PCR-RFLP) analysis, and High-Resolution Melting (HRM) analysis (33–37). An example of a classical hybridization technique is Fluorescence in situ hybridization (FISH), which utilizes a fluorescently labeled probe targeting a specific sequence to detect copy number variations (CNV). The major drawback of all of the above-mentioned methods is that they are limited to specific regions within the genome. To find high-throughput genetic alterations across the genome, DNA microarray-based and NGS techniques were developed (38, 39). DNA microarray technology determines the number of copies of particular genomic regions through sequence-primer hybridization (40, 41). CNV microarrays such as OncoScan can characterize CNVs in 900 cancer genes and 300kb region outside of the cancer genes throughout the genome, based on hybridization (42).
Two main NGS applications have allowed for high-throughput detection of genetic alterations in tumors: targeted DNA sequencing, and whole genome sequencing (WGS) (43, 44). While WGS determines the sequence of the entire genome, targeted DNA sequencing methods such as hybridization capture NGS and amplicon-based NGS sequence specific DNA fragments (44, 45). One major application for targeted DNA-sequencing is the targeting of exons throughout the genome, also known as whole exome sequencing (WES). Overall, DNA microarrays, WES, and WGS have been essential to elucidating cancer-associated mutations and focal copy number alterations, and large-scale chromosomal copy number alterations.
2.2 Genomic alterations frequently observed in prostate tumors
Prostate tumors often harbor inactivating, or loss of function (LOF), mutations or deletions in tumor suppressor genes involved in pathways regulating cell cycle, DNA repair, and transcriptional regulation. Genetic alterations identified at the greatest frequency in prostate cancer related to cell cycle include mutations at TP53, RB1, PTEN, and deletions at chromosome 10q containing PTEN (46–48). Several studies state that ZFHX3, which functions to inhibit prostate carcinogenesis by suppressing MYC overexpression, is correlated to improved patient survival (49). It is reported that ZFHX3 is deleted or contains a LOF mutation in prostate tumors (50). Notable tumor suppressor genes involved in transcriptional regulation that are frequently deleted or mutated in prostate tumors include SPOP, MED12, CHD1, and ZNF292 (51).
The aforementioned genetic alterations are somatically acquired during tumorigenesis. Germline mutations including BRCA1, BRCA2, HOXB13, CHEK2, and ATM mutations have been associated with hereditary prostate cancer (52). One of the most common mutations is in BRCA2 (47, 52). BRCA1/2, known as tumor suppressor genes, mediate double-strand break DNA repair (53). BRCA1/2 mutations are not only associated with an increased risk of prostate cancer, but also with an aggressive prostate cancer phenotype, such as higher grade, advantaged stage, and poor survival (54, 55). HOXB13 is important in prostate development and this mutation was observed in 0.7-1.4% of prostate cancers, and 6% of early-onset prostate cancer (56). HOXB13 mutations are also associated with an increased hereditary prostate cancer risk (57, 58). Lu et al. found that the HOXB13 mutation disrupts the interaction between HOXB13 and histone deacetylase 3 (HDAC3), an epigenetic modifier (59).
Genetic alterations such as fusions, amplifications, translocations, and gain-of function (GOF) mutations are found in oncogenes in prostate cancer. As reported in various studies, androgen receptor (AR) has been increasingly implicated in prostate cancer (60). AR is a key transcription factor playing a critical role in prostate cancer initiation and progression. It leads to prostate cancer cell proliferation by mediating transcription of pro-mitotic genes (61, 62). Androgen binding induces a conformational change, resulting in its nuclear translocation (63, 64). Subsequently, AR binds at specific genomic regions and activates transcription of its numerous target genes (64).
Since up to 90% of prostate cancer is dependent on androgens at diagnosis, androgen signaling has been considered a pivotal therapeutic target. Androgen deprivation therapy (ADT), either alone or in combination with chemotherapy, is the mainstay of initial treatment for advanced, high-grade and stage, prostate cancer, albeit many patients eventually will develop progressive disease referred to as castration-resistant prostate cancer (CRPC) (64–66). AR genetic alterations including mutations and amplifications are observed in prostate cancer as well as alternative splicing events. There are four domains of AR: amino-terminal transcriptional domain (NTD), DNA-binding domain (DBD), hinge region, and a carboxy-terminal ligand-binding domain (LBD) (66). Splicing variants encode truncated AR forms, such as AR-V7, lacking the LBD and thus making the protein constitutively active regardless of the presence of androgens (67). Interestingly, AR mutations and amplification events are nearly exclusively found in metastatic prostate tumor samples, but not in primary tumor samples. AR mutation burden usually increases with tumor stage, and alterations oftentimes involve missense mutations in the LBD (68). In this way, there is less specificity of the domain overall, as AR activation can be induced by various ligands beyond its non-pathological activators (68, 69).
Downstream and upstream effectors of AR are also found to be altered in primary tumor samples (47). The most frequent gene rearrangements found in primary prostate tumors are those involving TMPRSS2 (Transmembrane Serine Protease 2) and members of the ETS (erythroblast transformation specific transcription factor) family transcription factors. The ETS family includes ERG, ETV1, ETV4, and FLI1 which can all form fusions with TMPRSS2. In the Cancer Genome Atlas (TCGA) prostate adenocarcinoma data, 53% of tumors were found to have ETS family gene fusions. The ETS family gene members involved in the fusions, the most common being ERG (46%), were found to be mutually exclusive. The promoter of TMPRSS2-ERG gene is reported to be bound by AR. Interestingly, AR genetic aberrations and the fusion proteins under its regulation are seen at variable relative levels. Moreover, most of the TMPRSS2-ETS fusion positive tumors also contained PTEN deletions (47).
The next most common genetic alterations found in prostate tumors include mutations in SPOP, FOXA1, and IDH1. Interestingly, SPOP and FOXA1 mutant tumors were found to be both mutually exclusive with TMPRSS2-ETS fusions and had higher AR transcriptional activity (47). Lastly, the presence of amplifications, insertions, and deletions, also known as copy number alterations (CNAs), have been shown to be directly correlated to disease severity. For example, CHD1 deletions and SPOP mutations frequently co-occur in prostate tumors (47). Prostate tumors with whole chromosomal arm gains or losses are associated with high grade, Gleason score, and PSA levels (47). Common amplifications span the oncogenes MYC (8q24.21), CCND1 (11q13.2), FCFR (12p11.21), and NSD3 (8p11.23) and common deletions span tumor suppressor genes PTEN (10q23), TP53 (17p13.1), CDKN1B (12p13.1), MAP3K1 (5q11.2 & 6q.12-22), FANCD2 (3p26), SPOPL (2q22.1), and FOXP1/RYBP/SHQ1 (3p13) (47). Interestingly, aggressive prostate tumors with greater mutational burden or with higher CNA frequencies have more mutations at KMT2D, TP53, and KDM6A and a higher frequency of MYC amplifications (47).
2.3 Genomic alterations differentially identified in AA and EA prostate cancer patients
Due to the reported outcome disparities between AA and EA men with prostate cancer, many studies have sought to evaluate potential genetic alteration differences between tumors obtained from these two subgroups. When we searched for studies that performed their own DNA-sequencing (WGS and WES) or genetic alteration analysis in prostate tumor tissue samples from AA and EA, we found six studies that fulfilled inclusion criteria (12–17) (Table 1). All analyzed data were based on self-identified race, in lieu of comparing samples using ancestry-specific analysis. Sample sizes for participated AA and EA prostate cancer patients varied across studies, as well as methodologies.
Among somatic mutations commonly found in prostate cancer, TP53, PTEN, and ZFHX3 mutations affect cell cycle and growth. As previously mentioned, TP53 loss of function mutations are relatively common in prostate tumor samples. Two studies, Lindquist et al. and Liu et al., which evaluated DNA with WGS and targeted exome sequencing, respectively, reported that AA patients had relatively less TP53 inactivating mutations than EA patients (15, 16). Another two studies, Koga et al. and Liu et al., which used WES and PCR, respectively, found that there was no significant difference in TP53 mutation frequency between AA and EA (13, 17). The correlation between PTEN loss and race is largely unclear. The frequency of PTEN loss was not found to be significantly different between AA or EA prostate cancer patients by Khani et al. (12) and and Liu et al. (16); the two studies used FISH and the Affymetrix OncoScan FFPE SNP CNV microarray to assess CNV status, respectively (12, 16). However, two other studies reported that PTEN loss was less frequent in AA patient tumors using WGS and WES, respectively (13, 15). The ZFHX3 gene has been found to be more frequently deleted or contain a LOF mutation in AA patients relative to EA across several studies (13, 16). This distinction can prove important to the treatment of AA prostate cancer since functional ZFHX3 is necessary for effective ESR2 (aka ERß) agonist treatment (49).
Genetic alteration frequency of two genes, SPOP and KMT2D, which regulate the transcriptional process were investigated between AA and EA across multiple studies. Results describing the frequency of SPOP mutations in both AA and EA tumor samples varied. For example, Koga et al. (13) found a higher frequency of SPOP mutations in AA compared to EA when considering all tumors, regardless of primary or metastatic, whereas Liu et al. (16) found that the frequency of SPOP mutations was lower in AA patients. Additionally, two studies found no differences in mutation frequency between AA and EA (12, 15). KMT2D mutations, which are associated with more aggressive disease, were found at a greater frequency in AA patients in multiple studies (13, 16).
Genetic alterations in genes related to androgen signaling (AR and FOXA1) were also evaluated by multiple studies. The study by Koochekpour et al, which used PCR analysis, found that AR mutations were more frequent in AA prostate cancer patient samples than EA (14), but two other studies found no difference in mutation frequency (13, 16). FOXA1 was found to have a greater mutation frequency in AA patients (15), whereas another study found it not to be statistically significantly different (13). MYC amplification was a point of contention between studies. Lindquist et al. reported that AA patients were less likely to have MYC amplification (15), whereas other studies reported that AA patients were more likely to have MYC amplification (13, 16).
Fusion events were found in many prostate tumors, but these findings were observed in datasets overwhelmingly obtained from EA patient populations, such as the TCGA dataset. Multiple studies found that TMPRSS2-ERG fusions were less prevalent in AA patients relative to EA patients (13, 15, 16, 70, 71). Although the absence of these fusions has not been associated with a worse prognosis, the significant difference in prevalence demonstrates the need to evaluate previously established genetic biomarkers of disease in AA patient populations (72). Interestingly, a novel gene fusion, CDC27-OAT, was shown to be either specific or more common in AA patients (15).
Other genetic alterations not previously associated with prostate cancer were found to be significantly different between AA that EA patient samples. Lindquist et al. described that those frequent mutations in two genes (MUC3A and PRIM2) were found more commonly in AA tumor samples, which could be potentially carcinogenic (15). These genes encode proteins involved in cell growth and survival, and DNA replication, respectively. The study by Koga et al. identified a novel deletion spanning ETV3 (1q23.1), another ETS transcription factor, in AA tumor samples (13).
Overall, most of these studies addressing racial differences did not have consistent results for genes related to prostate carcinogenesis although ZFHX3 and KMT2D alterations were found in multiple studies at a greater frequency in AA patients and were associated with increased disease severity. It is possible that a subset of genetic alterations may at least in part contribute to the clinical disparities seen between AA and EA prostate cancer patients. It is therefore important to fully interrogate more samples to understand the contribution of genomic difference to the severity of disease using DNA-sequencing techniques.
3 Epigenomic alterations linked to African American prostate cancer patients
3.1 Methods to profile epigenomic alterations in cancer
Epigenetic alterations change the chromatin state and structure to regulate gene expression without changes in DNA sequence in cancer cells. Chromatin state and structure are important for the maintenance of cell states. Nucleosome positioning, histone modifications as well as DNA methylation define the status and identity of each cell, and they are maintained throughout the cell cycle (73–77). Non-coding RNAs (ncRNAs) are also reported to regulate gene expression and chromatin state to control cell proliferation and differentiation (78). Among those epigenetic alterations, DNA methylation analysis is used most often because DNA samples obtained for the DNA methylation analysis are easily isolated and stored from diverse tissue types. Additionally, DNA methylation analysis can be performed with a small amount of DNA (79).
Classical DNA methylation methodologies include methylation-specific PCR (MSP), pyrosequencing, and Luminometric Methylation Assay (LUMA). MSP involves PCR at a specific CpG site of-interest using site-specific primers, one for methylated CpG and the other for unmethylated CpG detection (80). Although this method interrogates the DNA methylation status at a specific site of interest, the drawback is that only one or two CpG sites can be assessed at a time. Additionally, MSP is difficult to perform in regions that are not CpG islands (81). Pyrosequencing detects DNA methylation levels of CpG sites in a PCR product (82). The advantages of this method are that it is time-efficient, quantitative, and can detect even small differences in methylation (83). However, primer design is difficult, and only a short region can be analyzed (83).
To profile DNA methylation at multiple sites at once, multiple techniques were developed. LUMA technology, which was based on the combined methylation-sensitive restriction enzyme DNA cleavage and pyrosequencing-based polymerase extension, was one of the earliest developed methods (84). A combined method such as Combination of methylated-DNA precipitation and methylation-sensitive restriction enzymes (COMPARE-MS) assay, which uses PCR products of DNA first digested by methylation-sensitive restriction enzymes then precipitated by methyl-binding domain polypeptides, was also developed to detect CpG island DNA methylation (85). However, these assays are only capable of detecting differences in DNA methylation within methylation-sensitive restriction enzyme cut sites, which are not uniformly distributed in the genome. Therefore, these assays cannot exhaust all of the CpG sites in the genome.
To better profile DNA methylation sites, genome-wide, high-throughput techniques coupled with NGS and microarrays have been developed. Methyl-CpG-binding domain sequencing (MBD-seq) and methylated DNA immunoprecipitation sequencing (MeDIP-seq) are based on affinity purification using antibodies (86). Genomic DNA is prepared, sheared, denatured, and then immunoprecipitated. Pull down of methylated DNA is possible by using MBD-seq or MeDIP-seq methodologies (81). MBD proteins bind to double-strand methylated DNA using the methyl-binding domain while MeDIP uses a 5-methylcytosine monoclonal antibody against single-strand DNA (87). Both techniques are cost-effective, are capable of distinguishing between 5mC and 5hmC, induce no mutations, and provide no limitations to enzyme recognition sites. However, both methods are biased toward hypermethylated regions (86). MBD methods tend to be more sensitive to enrichment of CpG islands compared to MeDIP, while MeDIP provides relatively superior profiling of enrichment regions with lower CpG density (86).
Subsequent technologies using bisulfite conversion coupled with hybridization (i.e. DNA methylation microarrays) or sequencing (i.e. whole genome bisulfite sequencing) have been developed to provide insight as to the DNA methylation state of regions of-interest simultaneously and globally. The DNA methylation arrays that are most used were developed by Illumina. The Illumina methylation assay uses BeadChip to generate a genome-wide methylation profile. Similar to pyrosequencing, this method quantifies methylation levels at individual CpG loci within the genome (88). The bisulfite-converted DNA is amplified, fragmented, and hybridized to probes on the microarray, providing targeted-enrichment of methylated regions (89). The main advantages of the DNA methylation microarray method include cost-effectiveness, time-efficiency, and the low DNA input required (51, 89, 90). The initial Infinium Human Methylation27 (HM27) BeadChip contains 27,578 CpG sites. Later, Illumina developed the Infinium Human Methylation450 (HM450) BeadChip assay which interrogates 482,421 CpG sites, including 90% of the sites on the HM27 (89). More recently, the Infinium Methylation EPIC (EPIC) BeadChip has been developed, containing over 850,000 CpG sites including promoters, enhancers, and open chromatin regions (91).
Whole genome methylation sequencing utilizes NGS techniques to obtain DNA methylation data that can include all CpG sites within the genome at single nucleotide resolution (76). Whole genome bisulfite sequencing (WGBS) allows the identification of methylation state at almost every CpG site in the genome, providing highly integrated single base resolution DNA methylation patterning (92). However, this method is high cost, unable to distinguish between 5mC and 5-Hydroxymethylcytosine (5hmC) and causes substantial DNA degradation after bisulfite treatment (86). Reduced representation bisulfite sequencing (RRBS) applies WGBS techniques to specific regions of interest. Because only a fraction of the genome is sequenced, RRBS is highly sensitive and cost-effective compared to WGBS (86).
DNA methylation changes reported by these techniques in cancer results in the silencing of tumor suppressor genes or activation of oncogenes. Compared to normal cells, the promoter regions of tumor suppressors are hypermethylated whereas the promoter regions of oncogenes are hypomethylated (93). Moreover, cancer-specific enhancer regions are hypomethylated (94, 95). Furthermore, long-range hypomethylated regions such as partially methylated domains are detected in cancer cells (96). The hypomethylation and hypermethylation of specific CpG sites in cancer allows for the understanding of molecular mechanisms of dysregulated of tumor suppressors and oncogenes (97).
3.2 Heterogeneous DNA methylation patterns among prostate tumors
To understand and characterize DNA methylation states of prostate tumor samples relative to normal prostate tissue, several DNA methylation studies have been performed (47, 92, 98–125). For example, in a review written by Lam et al. (99), six genes (GSTP1, APC, RARB, PITX2, CCND2, and PTGS2) along with their corresponding CpG sites were identified as having prognostic importance across several prostate cancer studies (99–125). Of the six genes, GSTP1, APC, RARB, CCND2, and PTGS2 were tumor suppressors identified as having been hypermethylated in prostate tumor tissues. PITX2, an oncogene that also bears importance in lung adenocarcinoma, was identified as being hypomethylated in prostate cancer.
Moreover, TCGA, which profiled DNA methylation levels of over 300 prostate tumor tissues using Illumina HM450 arrays, revealed heterogeneous DNA methylation patterns amongst 8 prostate tumor molecular subtypes including: TMPRSS2-ERG fusion, TMPRSS2-ETV1 fusion, TMPRSS2-ETV4 fusion, TMPRSS2-FLI1 fusion, SPOP mutation, FOXA1 mutation, IDH1 mutation, and tumors without genetic alterations (47). For example, IDH1 mutant tumors exhibited heavy hypermethylation throughout the genome. SPOP and FOXA1 mutant tumors had similar DNA hypermethylation patterns with a moderate number of hypermethylated loci. Compared to SPOP and FOXA1 mutant tumors, two-thirds of ERG fusion-positive tumors had relatively low hypermethylated loci. However, one-third of ERG fusion-positive tumors had distinct hypermethylation patterns, which include more than twice the number of hypermethylated loci than the remaining two-thirds of the ERG fusion-positive tumors. DNA methylation patterns of ETV1 and ETV4 fusion-positive tumors were distinct from ERG fusion-positive tumors while FLI1 fusion-positive tumors exhibited similar hypermethylation pattern as the latter two-thirds of the ERG fusion-positive tumors, having moderate hypermethylated loci.
Over 150 epigenetically silenced genes in prostate tumors were also identified by TCGA. For example, SHF, FAXDC2, GSTP1, ZNF154, and KLF8 genes had hypermethylated promoters and silenced gene expression across the prostate tumor samples compared to normal prostate samples. In ETS fusion-positive tumors, STAT6 was found to be epigenetically silenced, whereas this silencing was not found in prostate tumors with mutations in SPOP and IDH1. Unlike other tumor types, SPOP mutant tumors had epigenetically silenced HEXA (47).
3.3 Differentially methylated regions detected between AA and EA prostate cancer patients
To characterize epigenomic alterations in prostate tumors from AA patients, differential DNA methylation analyses between benign and malignant prostate tissues obtained from AA patients have been performed by multiple research groups. We found seven studies (18–24), of which six investigated DNA methylation signatures at specific genes, and two performed global epigenome analysis (Table 2).
For example, Barry et al. investigated DNA methylation levels at the MYC locus (6 CpG sites from exon 3 to the 3′ UTR) in AA and EA prostate cancer patients using pyrosequencing (18). They determined that AA patient samples were relatively hypomethylated at exon 3 of the MYC gene, a site that is associated with a higher Gleason score, and therefore severity of disease (18). They showed that DNA methylation level at one of the examined CpG sites is more strongly associated with Gleason score in prostate tumors from AA patients than EA patients. However, subsequent RNA-sequencing data analysis indicated that MYC expression was not significantly different regardless of the DNA methylation status at MYC region; the study suggested ncRNA expression to be responsible for the difference (18).
Tang et al. reported that hypermethylation of RARB (aka RARß2) was significantly associated with a higher risk of prostate cancer in AA men but not in EA men, but this study was not included since it only evaluated cancer risk according to methylation within benign prostate tissue (126). Woodson et al. studied DNA methylation of a set of genes (GSTP1, RASSF1A, RARB, CD44, EDNRB, CDH1, ANXA2, and CAV1) that were previously implicated in prostate tumorigenesis in AA patient samples and reported that GSTP1, RASSF1A, and RARB were hypermethylated and CDH1, EDNRB, and CD44 were hypomethylated in tumor samples (24). This group compared the data to EA patients and found no significant differences in overall DNA methylation status for all aforementioned genes, but found CD44 hypermethylation twice as prevalent in AA men (24). Another study by Kwabi-Addo et al. selected epigenetically altered genes in prostate cancer and found AR, GSTP1, RARB, SPARC, TIMP3, and NKX2-5 to be hypermethylated in AA patients (21). Although TIMP3 was identified by Lam et al. to be hypermethylated in prostate cancer, it was determined to have no prognostic utility. Two of the aforementioned genes, SPARC and NKX2-5, have been shown to be hypermethylated in prostate cancer (110, 112). When comparing these results with EA samples, Kwabi-Abbo et al. found that there was statistically significant differential methylation solely in the TIMP3 and NKX2-5 genes (21).
GSTP1 was also found to be hypermethylated in AA patients by Sharad et al. (23). However, it was not found to be differentially methylated between AA and EA patients. Another study, which investigated the DNA methylation of GSTP1 across different ethnic groups, also found it universally hypermethylated in prostate cancer, but not significantly different in DNA methylation levels between ethnic groups (20). Sharad et al. also found that the PMEPA1 gene was found to be significantly more hypermethylated in EA prostate cancer patients compared to AA prostate cancer patients (23). This gene encodes an androgen-sensitive ligase binding protein that is now known to maintain AR protein levels in prostate tissues (23).
Unlike the aforementioned studies that characterized the DNA methylation levels of specific genes of interest, Devaney et al. performed Illumina 450K methylation arrays in AA (7 normal and 3 cancer) and EA (8 normal and 3 cancer) prostate tumor tissues (19). They identified 25 promoter-associated CpG sites that were differentially methylated by race. The most significantly differentially methylated genes were MST1R, ABCG5, and SNRPN (19). It is important to note that this study had a very small sample size, which may limit statistical power in identifying statistically significantly differentially DNA methylation sites from the array.
Rubicz et al. also performed Illumina HM450 methylation arrays in AA patients, subsequently with RNA-seq (22). They compared DNA methylation levels with EA from Fred Hutch Cancer Research Center (FHCRC) prostate cancer studies (127, 128). They found hypermethylation of STOX7, SNRPN, TIMP3, and PMEPA1 in AA patients, which were subsequently found to be differentially methylated between AA and EA (22). The hypermethylation of TIMP3 and PMEPA1 were found by several other studies (20, 21, 23, 24, 126). However, SNRPN hypermethylation did not correspond to lower expression in this study, unlike the findings by Devaney et al. (19). Additionally, they were able to identify differentially methylated regions between AA prostate cancer patients with and without prostate cancer recurrence in the gene bodies and promoter regions of genes involved in specific tumorigenic biological processes such as protein kinase activity and metal ion binding activity. For example, CDKL2, FOXA2, NEUROG1, and GCK were found to be hypermethylated at promoter regions with corresponding decreased transcription levels in AA prostate cancer patients with recurrence compared to patients without recurrence (22).
The lack of consistency in the findings between studies could be attributed to insufficient sample sizes, differences of profiling methods, the heterogeneous nature of the subpopulation itself, or non-biological factors. Overall, this shows the necessity for further whole genome methylation sequencing analysis of AA patient prostate cancer tissue samples.
4 Transcriptomic alterations linked to African American prostate cancer patients
4.1 Methods to profile gene expression in cancer
Genomic and epigenomic alterations in tumors lead to the reprogramming of gene expression patterns. Profiling gene expression is crucial to understand carcinogenesis and identify therapeutic targets. For example, amplification of the MYC oncogene leads to its overexpression, which in turn activates its target genes, promoting cell proliferation. Moreover, some tumor cells can be targeted directly based on the overexpression of certain transmembrane proteins. For instance, prostate specific membrane antigen (PSMA), which is upregulated in many metastatic prostate tumors, can be targeted with its radiolabeled inhibitor and used for imaging modalities or radiotherapy (129, 130). By comparing gene expression patterns, transcriptional networks and signaling pathways altered in tumors can be also revealed.
In order to better develop the global gene expression profiles of cancer cells, researchers have utilized mRNA quantification techniques such as Reverse Transcriptase Quantitative Polymerase Chain Reaction (RT-qPCR), RNA microarrays, and RNA-sequencing (131, 132). All techniques utilize the conversion of RNA transcripts into cDNA through reverse transcription, but RT-qPCR is limited due to its probing of only known gene regions. Both RNA microarrays and RNA-sequencing are instead capable of profiling entire transcriptomes. However, these two platforms differ in their benefits and limitations. Microarrays allow for the expression profiling of thousands of transcripts through cDNA hybridization using probes simultaneously. This methodology can only interrogate expression of known transcripts and selected exons. It also has a lack of specificity, or high background noise due to cross-hybridization of multiple transcripts to the same probe (132, 133). Examples of microarrays commonly used include the GeneChip Human 525 Exon 1.0 ST, which can interrogate over 1 million exon clusters, with four probes per exon on average. Another commonly used microarray with a smaller breadth of capabilities is the GeneChip HG-U133A 2.0, which interrogates 14,500 well-known genes.
RNA-sequencing instead uses NGS to fully catalog the transcriptome of a sample through sequencing of cDNA transcripts, regardless of whether they are known transcripts or not (134). RNA-sequencing allows for the characterization of transcriptomic features such as alternative splicing events and antisense transcripts (134). The most popular RNA-sequencing technique involves the capture of mRNA based on the presence of polyA tails at the 3’ end (polyA RNA-seq); an example of this technique is the Illumina TruSeq RNA library preparation assay (135). Another type of RNA-sequencing (total RNA-seq) is to capture the total RNA after depleting ribosomal RNA, which profiles RNA transcript levels of coding genes, noncoding regions, and small RNAs (136). The major limitation of this method is that it is more expensive than microarray technology. Additionally, RNA-seq libraries are relatively difficult to prepare and analyze compared to RNA microarrays. Overall, when studying global transcriptomic features of tumor cells and tissues, RNA microarrays allow for the consistent generation of tumor expression profiles, and RNA-sequencing technology allows for the discovery of novel transcriptomic events and the quantification of their overall expression.
4.2 Genes and signaling pathways dysregulated in prostate cancer
RNA-sequencing and microarray technologies have been used to analyze the transcriptomes of prostate carcinomas to better understand the gene expression patterns that are specific to the disease and contribute to tumorigenesis. Many of these expression changes are consequences of genomic or epigenomic alterations, which are used to categorize the molecular subtypes mentioned above. Gene expression changes found in prostate cancer can be understood through their effects on AR signaling (137). AR is essential for the normal growth and development of the prostate gland. Individuals with defective AR signaling do not experience prostate enlargement, and inhibiting AR activity results in reduced prostate size and symptoms (137). During prostate tumor cell transformation and carcinogenesis, AR activity is dysregulated, and androgen dependence and sensitivity is altered. For example, when AR transcriptional activity has been characterized in primary prostate tumors by the expression pattern of the AR gene itself as well as the 20 previously described AR target genes, tumors with SPOP or FOXA1 mutations have the highest AR transcriptional activity (47). This pattern is supported by the fact that SPOP mutant has been shown to induce AR signaling (138). FOXA1 mutations in prostate cancer cells are reported to reduce the number of AR binding sites but replaces AR function by increasing the activities of AR target genes (139) AR coactivators and FOXA1 are known to interact with AR by binding to numerous enhancers to increase AR signaling (77). However, AR activity varies among tumors with fusion involving an AR-controlled ETS gene (i.e. TMPRSS2-ERG, TMPRSS2-ETV1, TMPRSS2-ETV4, TMPRSS2-FLI1 fusions) (47). TMPRSS2-ETS fusion gene is reported to be regulated by AR, but it is highly expressed in both primary and metastatic prostate tumors, independent of AR transcription levels (47). Mechanistic models have been proposed regarding AR transcription and signaling, but ultimately the exact mechanisms that result in the formation of molecular subtypes throughout tumorigenesis is unknown (134).
AR signaling alterations are found to be associated with metastatic prostate tumors. For example, the expression of AR-V7 transcript variant that lacks LBD in metastatic prostate tumors is associated with resistance to hormone therapy (140). AR-V7 expression was not associated with the molecular subtypes of primary prostate tumors and AR transcriptional activity, however (47). AR expression in metastatic prostate cancer is also reported to altered by epigenetic changes such as enhancer and chromatin interaction changes (141). Additionally, AR expression is affected by changes in ncRNA transcripts, and subsequently affects the expression of other ncRNA transcripts (69).
There are additional genes and signaling pathways reported to be altered in prostate cancer. For example, prostate tumor samples with SPOP mutations have been associated with the overexpression of SPINK1 through activating the mitogen-activated protein kinase (MAPK) pathway (142). Other significant pathways found to be upregulated in prostate tumors are the phosphatidylinositol-3-kinase (PI3K)/Akt/mammalian target of rapamycin (mTOR), Ras/MAPK, DNA repair, and receptor tyrosine kinase pathways (143, 144).
4.3 Genes differentially expressed between AA and EA prostate cancer patients
Characterizing the genes and pathways linked to AA prostate tumor samples will allow researchers to better understand differences in tumor progression and treatment response between subgroups. When we searched studies that generated RNA-sequencing or microarray data from prostate tissue samples, and directly compared gene expression profiles between AA and EA prostate cancer patients, we found eight different studies (25–32) (Table 3).
The largest study among the 8 studies was performed by Rayford et al., which analyzed the gene expression patterns between 596 AA and 556 EA prostate cancer patients using the Human Exon 1.0 ST microarrays, as well as TCGA RNA-seq data (30). Major findings included the significant association of SPINK1 overexpression in AA patients relative to EA patients, and lack of TMPRSS2-ERG fusions (30). SPINK1 was also found to be overexpressed at the protein level more frequently in AA patient samples in the study by Khani et al. (12). The lack of TMPRSS2-ERG fusions in AA patients was also noted in patient samples analyzed by Echevarria et al. (25). Indeed, five (BCL6, EMP1, MYADM, SRGN, and TIMP3) of the six differentially expressed genes (APOD, BCL6, EMP1, MYADM, SRGN, and TIMP3) that Echevarria et al. suggested to be useful as AA-specific prostate cancer biomarkers were primarily present in the TMPRSS2-ETS fusion negative tumor samples (25).
Additionally, Rayford et al. found that the CRYBB2 and GSTM3 genes were upregulated in AA prostate cancer patients than EA patients (30). These genes were also reported to be upregulated in TCGA prostate cancer AA patients. CRYBB2 specifically was found by two studies to be overexpressed in AA men (28, 30) while another study indicated that it was underexpressed in AA men relative to EA men prostate tissue samples (29). Rayford et al. also reported that the biological pathways upregulated in AA patients were related to inflammatory response (e.g. IL33, IFNG, CCL4, CD3, ICOSLG), whereas the biological pathways upregulated in EA patients were related to DNA repair (e.g. MSH2, MSH6), metabolism, cell proliferation, and cell cycle (30). Inflammatory and immune pathway dysregulation was noted by other studies as well. A study by Hardiman et al. in 2016, which performed RNA-sequencing in 10 AA and 17 EA prostate cancer patients, observed that multiple immune and inflammatory pathways (e.g. IL2RG, CD1C, CD207, CCL4, CCL8, CXCR4) were upregulated in AA patients relative to EA patients (26). A study by Rahmatpanah et al., which analyzed RNA-seq data obtained from 15 AA and 30 EA prostate tumors, observed an upregulation of inflammatory and immune pathways (e.g. CXCL10, CXCL2, HLA-A, CCL2) (29). Using the Affymetrix GeneChip HU-U133A 2.0 array, Wallace et al. observed upregulation in the inflammatory pathway (e.g. CXCR4, CCL5, CCR7) in tumor samples from 33 AA patients relative to 36 EA patients as well (32).
Nagaya et al. performed RNA-sequencing in prostate tumors from 31 AA and 30 EA men and found 45 differentially expressed genes (28). The identified genes were not involved in the inflammatory and immune pathway. The most notable findings in this study were that four of the 45 differentially expressed genes were found to be in the neuroactive ligand pathway: GALR1, CHRM3, NPFFR1, and S1PR3 (28). S1PR3 expression level was higher in AA than EA prostate cancer patients whereas GALR1, CHRM3, and NPFFR1 expression level was lower in AA than EA prostate cancer patients (28). Expression changes found for NPFFR1 and S1PR3 genes were not reported by other studies (25–32). However, GALR1 was also found to be downregulated in AA patient samples compared to EA patient samples in one other study (27) and CHRM3 was found to be downregulated in two other studies (25, 30). Hardiman et al., which performed RNA-seq in prostate tumors from 33 AA and 27 EA men, reported downregulation of GALR1 in AA patients relative to EA patients, and reported differential expression of other genes in the neuroactive ligand pathway (27). For example, GABRP, ADORA2B, TACR1, TAAR1, and GABRQ were modestly downregulated in AA compared to EA, and AGTR1, F2RL2, NPY4R and GRIN3A were upregulated in AA compared to EA (27). Rahmatpanah et al. found that GRIN3A was also downregulated in AA relative to EA patients (29). The involvement of aberrant neuroactive ligand signaling pathway has been reported in other cancer types (145, 146).
Another pathway found to be differentially expressed between AA and EA prostate cancer patients across studies was the PI3K-Akt pathway, which is involved in cell survival, growth, and proliferation. For example, Rahmatpanah et al. found that genes involved in this pathway such as PIK3CA were overexpressed in AA samples relative to EA samples (29). Hardiman et al. also reported that PI3K pathway is altered and that some genes involved in the PIK3-Akt pathway were significantly upregulated (THBS4, CREB3L1, TNN, COL4A4, COL4A3, COL2A1, FGF12, MYC, and GNG13) and downregulated (SGK1, ANGPT2, FGF11, IL4, IL6, ANGPT4, THBS2, FLT4, NTRK2, PIK3R6, LAMA5, and MET) between AA and EA (27). However, these genes were not found to be differentially regulated in the Rahmatpanah study (29).
The Ras/MAPK pathway has also been implicated in prostate cancer. One study by Timofeeva et al. showed the overexpression of SOS1, an activator of this pathway, in prostate cancer tissues compared to normal prostate tissues at both mRNA and protein levels (31). The researchers showed that SOS1 expression was two-fold higher in AA men relative to EA men with prostate cancer (31). Interestingly, its overexpression was correlated to higher Gleason score, indicating that SOS1 overexpression could be a biomarker for AA disease severity (31).
Although the above eight studies revealed genes that are differentially expressed between AA and EA, these data have not entirely elucidated differences in prostate tumorigenesis in AA versus EA patients. Wallace et al., for example, compared their differentially expressed genes between AA and EA to the top 80 differentially expressed genes in prostate cancer (tumor vs. normal) and found no overlap (32). It is possible, however, that the differentially expressed genes classically found in prostate cancer were not studied with a large amount of AA patient samples, or that there are relatively less significant race-specific biological factors contributing to the progression of disease.
5 Discussion
In this study, we reviewed more than 20 studies that analyzed the genomes, epigenomes, and transcriptomes of prostate tumor tissue samples from AA and EA patients. Although there were several AA-specific alterations reported in more than one study, many of the findings reported by multiple research groups were contradictory to others. Another recent review on prostate tumor genomics also reported that there was no clear association between specific genetic changes and race (147). This could be due to the large variance in sample sizes of both EA and AA groups as the size of samples is crucial for getting statistically significant findings. To further evaluate the biological contributions, if any, to the clinical disparities that disproportionately affect AA men with prostate cancer, there needs to be a greater number of AA-specific studies that take account of genome-wide genomic, epigenomic, and transcriptomic approaches. For example, although there were a significant number of genes that are differentially expressed between normal prostate and prostate cancer, those genes were characterized in patient cohorts that were largely composed of EA men. Additionally, there are very few epigenetic studies relative to the number of genetic and transcriptomic studies. Evaluating prostate cancer in AA men with a whole genome approach with equally large sample sizes could possibly allow researchers to elucidate AA-specific biomarkers. It is recently reported that the RESPOND study (Research on Prostate Cancer in Men of African Ancestry: Defining the Roles of Genetics, Tumor Markers, and Social Stress) will characterize molecular genetic signatures from 10,000 AA prostate cancer patients.
Additionally, the methods by which the prostate tissue samples are obtained can bias study results. Prostate tissue for these studies was obtained from patients by different kinds of methods (e.g. biopsy versus prostatectomy, fresh versus frozen versus formalin fixed paraffin embedded tissue (FFPE), etc), which may lead to results that are not comparable. For example, the treatment of tissue samples ex vivo (e.g. FFPE) may affect tissue conditions. Moreover, the prostate tumor tissue samples themselves can be heterogeneous within the given subpopulation. For instance, tumor stage, cellularity, and microenvironment can affect genomic, epigenomic, and transcriptomic signals. In addition, there is substantial molecular heterogeneity among prostate tumors. Distinct genetic and molecular signatures have found to define molecular subgroups of prostate cancer. Considering the greater relative incidence and mortality that is suffered by AA men with prostate cancer, it is important that we further characterize prostate cancer molecular subgroups with appropriate AA patient representation.
Lastly, most studies rely on ethnicity information inferred from self-reported ancestry. It has been recently shown by Schumacher et al. that, upon analyzing the GENIE 8.0 registry, genetic mutational frequency differences determined across patients of varying self-reported race were either insignificant or in non-clinically actionable regions as of present, but this study was not included in our analysis due to unclear cohort sizes (148). It is important to confirm and measure ethnicity information from samples using genetic ancestry informative marker data that quantify the high heterogeneity among subpopulations.
In conclusion, to better understand racial disparities in prostate cancer, molecular genetic signatures that are differentially associated between AA and EA patients were characterized using various techniques. By reviewing over 20 published studies, we revealed that heterogeneous genomic, epigenomic, and transcriptomic alterations are found between AA and EA prostate cancer patients. However, as results are controversial across different studies, additional large-scale investigations that take into account of potential confounding factors are greatly needed. Elucidating molecular mechanisms of tumorigenesis associated with tumor subgroups will provide valuable resources to identify novel biomarkers and treatment modalities to improve the disparity of clinical outcomes between AA and EA patients.
Author contributions
CS and SKR wrote the initial draft and finalized the manuscript. CS, AH, and SKR collected the data and prepared the figure and tables. SKR conceptualized and supervised the manuscript. All authors contributed to the article and approved the submitted version.
Funding
This work was supported by the National Institutes of Health under award numbers R21HG011506, R21CA260082, R21CA264637, U54CA233396, U54CA233444, and U54CA233465, which support the Florida-California Cancer Research, Education and Engagement (CaRE2) Health Equity Center. This work was also supported in part by W81XWH-21-1-0805 grant from the Department of Defense, USC Keck School of Medicine (KSOM) Dean’s pilot funding program, and the pilot grant from USC Center for Genetic Epidemiology.
Acknowledgments
We thank other Rhie lab members for providing insightful suggestions.
Conflict of interest
The authors declare that the research was conducted in the absence of any commercial or financial relationships that could be construed as a potential conflict of interest.
Publisher’s note
All claims expressed in this article are solely those of the authors and do not necessarily represent those of their affiliated organizations, or those of the publisher, the editors and the reviewers. Any product that may be evaluated in this article, or claim that may be made by its manufacturer, is not guaranteed or endorsed by the publisher.
References
1. Siegel RL, Miller KD, Fuchs HE, Jemal A. Cancer statistics, 2022. CA Cancer J Clin (2022) 72(1):7–33. doi: 10.3322/caac.21708
2. Giaquinto AN, Miller KD, Tossas KY, Winn RA, Jemal A, Siegel RL. Cancer statistics for African American/Black people 2022. CA: A Cancer J Clin (2022) 72(3):202–29. doi: 10.3322/caac.21718
3. Johnson JR, Woods-Burnham L, Hooker SE, Batai K, Kittles RA. Genetic contributions to prostate cancer disparities in men of West African descent. Front Oncol (2021) 11:770500. doi: 10.3389/fonc.2021.770500
4. Powell IJ, Bock CH, Ruterbusch JJ, Sakr W. Evidence supports a faster growth rate and/or earlier transformation to clinically significant prostate cancer in black than in white American men, and influences racial progression and mortality disparity. J Urol. (2010) 183(5):1792–6. doi: 10.1016/j.juro.2010.01.015
5. Mahal BA, Berman RA, Taplin ME, Huang FW. Prostate cancer–specific mortality across Gleason scores in black vs nonblack men. JAMA. (2018) 320(23):2479–81. doi: 10.1001/jama.2018.11716
6. Nyame YA, Cooperberg MR, Cumberbatch MG, Eggener SE, Etzioni R, Gomez SL, et al. Deconstructing, addressing, and eliminating racial and ethnic inequities in prostate cancer care. Eur Urology. (2022) 82(4):341–51. doi: 10.1016/j.eururo.2022.03.007
7. Dess RT, Hartman HE, Mahal BA, Soni PD, Jackson WC, Cooperberg MR, et al. Association of black race with prostate cancer–specific and other-cause mortality. JAMA Oncol (2019) 5(7):975–83. doi: 10.1001/jamaoncol.2019.0826
8. Kensler KH, Pernar CH, Mahal BA, Nguyen PL, Trinh QD, Kibel AS, et al. Racial and ethnic variation in PSA testing and prostate cancer incidence following the 2012 USPSTF recommendation. J Natl Cancer Inst (2020) 113(6):719–26. doi: 10.1093/jnci/djaa171
9. Ellis L, Canchola AJ, Spiegel D, Ladabaum U, Haile R, Gomez SL. Racial and ethnic disparities in cancer survival: The contribution of tumor, sociodemographic, institutional, and neighborhood characteristics. J Clin Oncol (2018) 36(1):25–33. doi: 10.1200/JCO.2017.74.2049
10. Cheng I, Witte JS, McClure LA, Shema SJ, Cockburn MG, John EM, et al. Socioeconomic status and prostate cancer incidence and mortality rates among the diverse population of California. Cancer Causes Control. (2009) 20(8):1431–40. doi: 10.1007/s10552-009-9369-0
11. Nelson WG, Brawley OW, Isaacs WB, Platz EA, Yegnasubramanian S, Sfanos KS, et al. Health inequity drives disease biology to create disparities in prostate cancer outcomes. J Clin Invest. (2022) 132(3):e155031. doi: 10.1172/JCI155031
12. Khani F, Mosquera JM, Park K, Blattner M, O’Reilly C, MacDonald TY, et al. Evidence for molecular differences in prostate cancer between African American and Caucasian men. Clin Cancer Res (2014) 20(18):4925–34. doi: 10.1158/1078-0432.CCR-13-2265
13. Koga Y, Song H, Chalmers ZR, Newberg J, Kim E, Carrot-Zhang J, et al. Genomic profiling of prostate cancers from men with African and European ancestry. Clin Cancer Res (2020) 26(17):4651–60. doi: 10.1158/1078-0432.CCR-19-4112
14. Koochekpour S, Buckles E, Shourideh M, Hu S, Chandra D, Zabaleta J, et al. Androgen receptor mutations and polymorphisms in African American prostate cancer. Int J Biol Sci (2014) 10(6):643–51. doi: 10.7150/ijbs.8974
15. Lindquist KJ, Paris PL, Hoffmann TJ, Cardin NJ, Kazma R, Mefford JA, et al. Mutational landscape of aggressive prostate tumors in African American men. Cancer Res (2016) 76(7):1860–8. doi: 10.1158/0008-5472.CAN-15-1787
16. Liu W, Zheng SL, Na R, Wei L, Sun J, Gallagher J, et al. Distinct genomic alterations in prostate tumors derived from African American men. Mol Cancer Res (2020) 18(12):1815–24. doi: 10.1158/1541-7786.MCR-20-0648
17. Liu W, Zhang Y, Wei S, Bae S, Yang WH, Smith GJ, et al. A CD24-p53 axis contributes to African-American prostate cancer disparities. Prostate. (2020) 80(8):609–18. doi: 10.1002/pros.23973
18. Barry KH, Mohanty K, Erickson PA, Wang D, Shi J, Rose G, et al. MYC DNA methylation in prostate tumor tissue is associated with Gleason score. Genes (Basel). (2020) 12(1):12. doi: 10.3390/genes12010012
19. Devaney J, Wang S, Furbert-Harris P, Apprey V, Ittmann M, Wang BD, et al. Genome-wide differentially methylated genes in prostate cancer tissues from African-American and Caucasian men. Epigenetics. (2015) 10(4):319–28. doi: 10.1080/15592294.2015.1022019
20. Enokida H, Shiina H, Urakami S, Igawa M, Ogishima T, Pookot D, et al. Ethnic group-related differences in CpG hypermethylation of the GSTP1 gene promoter among African-American, Caucasian and Asian patients with prostate cancer. Int J Cancer. (2005) 116(2):174–81. doi: 10.1002/ijc.21017
21. Kwabi-Addo B, Wang S, Chung W, Jelinek J, Patierno SR, Wang BD, et al. Identification of differentially methylated genes in normal prostate tissues from African American and Caucasian men. Clin Cancer Res (2010) 16(14):3539–47. doi: 10.1158/1078-0432.CCR-09-3342
22. Rubicz R, Zhao S, Geybels M, Wright JL, Kolb S, Klotzle B, et al. DNA Methylation profiles in African American prostate cancer patients in relation to disease progression. Genomics. (2019) 111(1):10–6. doi: 10.1016/j.ygeno.2016.02.004
23. Sharad S, Ravindranath L, Haffner MC, Li H, Yan W, Sesterhenn IA, et al. Methylation of the PMEPA1 gene, a negative regulator of the androgen receptor in prostate cancer. Epigenetics. (2014) 9(6):918–27. doi: 10.4161/epi.28710
24. Woodson K, Hanson J, Tangrea J. A survey of gene-specific methylation in human prostate cancer among black and white men. Cancer Letters. (2004) 205(2):181–8. doi: 10.1016/j.canlet.2003.11.027
25. Echevarria MI, Awasthi S, Cheng CH, Berglund AE, Rounbehler RJ, Gerke TA, et al. African American Specific gene panel predictive of poor prostate cancer outcome. J Urology. (2019) 202(2):247–55. doi: 10.1097/JU.0000000000000193
26. Hardiman G, Savage SJ, Hazard ES, Wilson RC, Courtney SM, Smith MT, et al. Systems analysis of the prostate transcriptome in African–American men compared with European–American men. Pharmacogenomics. (2016) 17(10):1129–43. doi: 10.2217/pgs-2016-0025
27. Hardiman G, Savage SJ, Hazard ES, da Silveira WA, Morgan R, Harris A, et al. A systems approach to interrogate gene expression patterns in African American men presenting with clinically localized prostate cancer. Cancers (Basel). (2021) 13(20):5143. doi: 10.3390/cancers13205143
28. Nagaya N, Rosenfeld J, Lee GT, Kim IY. RNA-Seq profile of African American men with a clinically localized prostate cancer. Prostate Int (2021) 9(3):125–31. doi: 10.1016/j.prnil.2020.11.002
29. Rahmatpanah F, Robles GD, Lilly M, Keane T, Kumar V, Mercola D, et al. RNA Expression differences in prostate tumors and tumor-adjacent stroma between black and white americans. Oncotarget. (2021) 12(15):1457–69. doi: 10.18632/oncotarget.28024
30. Rayford W, Beksac AT, Alger J, Alshalalfa M, Ahmed M, Khan I, et al. Comparative analysis of 1152 African-American and European-American men with prostate cancer identifies distinct genomic and immunological differences. Commun Biol (2021) 4(1):670. doi: 10.1038/s42003-021-02140-y
31. Timofeeva OA, Zhang X, Ressom HW, Varghese RS, Kallakury BVS, Wang K, et al. Enhanced expression of SOS1 is detected in prostate cancer epithelial cells from African American men. Int J Oncol (2009) 35(4):751–60.
32. Wallace TA, Prueitt RL, Yi M, Howe TM, Gillespie JW, Yfantis HG, et al. Tumor immunobiological differences in prostate cancer between African-American and European-American men. Cancer Res (2008) 68(3):927–36. doi: 10.1158/0008-5472.CAN-07-2608
33. Sanger F, Coulson AR. A rapid method for determining sequences in DNA by primed synthesis with DNA polymerase. J Mol Biol (1975) 94(3):441–8. doi: 10.1016/0022-2836(75)90213-2
34. Sanger F, Nicklen S, Coulson AR. DNA Sequencing with chain-terminating inhibitors. Proc Natl Acad Sci U.S.A. (1977) 74(12):5463–7. doi: 10.1073/pnas.74.12.5463
35. Saiki RK, Scharf S, Faloona F, Mullis KB, Horn GT, Erlich HA, et al. Enzymatic amplification of beta-globin genomic sequences and restriction site analysis for diagnosis of sickle cell anemia. Science. (1985) 230(4732):1350–4. doi: 10.1126/science.2999980
36. Narayanan S. Applications of restriction fragment length polymorphism. Ann Clin Lab Sci (1991) 21(4):291–6.
37. Pasay C, Arlian L, Morgan M, Vyszenski-Moher D, Rose A, Holt D, et al. High-resolution melt analysis for the detection of a mutation associated with permethrin resistance in a population of scabies mites. Med Vet Entomol (2008) 22(1):82–8. doi: 10.1111/j.1365-2915.2008.00716.x
38. Goodwin S, McPherson JD, McCombie WR. Coming of age: Ten years of next-generation sequencing technologies. Nat Rev Genet (2016) 17(6):333–51. doi: 10.1038/nrg.2016.49
39. Slatko BE, Gardner AF, Ausubel FM. Overview of next generation sequencing technologies. Curr Protoc Mol Biol (2018) 122(1):e59. doi: 10.1002/cpmb.59
40. Pollack JR, Perou CM, Alizadeh AA, Eisen MB, Pergamenschikov A, Williams CF, et al. Genome-wide analysis of DNA copy-number changes using cDNA microarrays. Nat Genet (1999) 23(1):41–6. doi: 10.1038/12640
41. Bentley DR, Balasubramanian S, Swerdlow HP, Smith GP, Milton J, Brown CG, et al. Accurate whole human genome sequencing using reversible terminator chemistry. Nature. (2008) 456(7218):53–9. doi: 10.1038/nature07517
42. Jung HS, Lefferts JA, Tsongalis GJ. Utilization of the oncoscan microarray assay in cancer diagnostics. Appl Cancer Res (2017) 37(1):1. doi: 10.1186/s41241-016-0007-3
43. Nakagawa H, Fujita M. Whole genome sequencing analysis for cancer genomics and precision medicine. Cancer Sci (2018) 109(3):513–22. doi: 10.1111/cas.13505
44. Hodges E, Xuan Z, Balija V, Kramer M, Molla MN, Smith SW, et al. Genome-wide in situ exon capture for selective resequencing. Nat Genet (2007) 39(12):1522–7. doi: 10.1038/ng.2007.42
45. Samorodnitsky E, Jewell BM, Hagopian R, Miya J, Wing MR, Lyon E, et al. Evaluation of hybridization capture versus amplicon-based methods for whole-exome sequencing. Hum Mutat (2015) 36(9):903–14. doi: 10.1002/humu.22825
46. Taylor BS, Schultz N, Hieronymus H, Gopalan A, Xiao Y, Carver BS, et al. Integrative genomic profiling of human prostate cancer. Cancer Cell (2010) 18(1):11–22. doi: 10.1016/j.ccr.2010.05.026
47. The Cancer Genome Atlas Research Network. The molecular taxonomy of primary prostate cancer. Cell (2015) 163(4):1011–25. doi: 10.1016/j.cell.2015.10.025
48. Rebello RJ, Oing C, Knudsen KE, Loeb S, Johnson DC, Reiter RE, et al. Prostate cancer. Nat Rev Dis Primers. (2021) 7(1):9. doi: 10.1038/s41572-020-00243-0
49. Hu Q, Zhang B, Chen R, Fu C, A J, Fu X, et al. ZFHX3 is indispensable for ERβ to inhibit cell proliferation via MYC downregulation in prostate cancer cells. Oncogenesis. (2019) 8(4):28. doi: 10.1038/s41389-019-0138-y
50. Sun X, Frierson HF, Chen C, Li C, Ran Q, Otto KB, et al. Frequent somatic mutations of the transcription factor ATBF1 in human prostate cancer. Nat Genet (2005) 37(4):407–12. doi: 10.1038/ng1528
51. Barbieri CE, Baca SC, Lawrence MS, Demichelis F, Blattner M, Theurillat JP, et al. Exome sequencing identifies recurrent SPOP, FOXA1 and MED12 mutations in prostate cancer. Nat Genet (2012) 44(6):685–9. doi: 10.1038/ng.2279
52. Pritchard CC, Mateo J, Walsh MF, De Sarkar N, Abida W, Beltran H, et al. Inherited DNA-repair gene mutations in men with metastatic prostate cancer. New Engl J Med (2016) 375(5):443–53. doi: 10.1056/NEJMoa1603144
53. Yoshida K, Miki Y. Role of BRCA1 and BRCA2 as regulators of DNA repair, transcription, and cell cycle in response to DNA damage. Cancer Sci (2004) 95(11):866–71. doi: 10.1111/j.1349-7006.2004.tb02195.x
54. Sokolova A, Cheng H. Germline testing in prostate cancer: When and who to test. Oncol (Williston Park). (2021) 35(10):645–53. doi: 10.46883/ONC.2021.3510.0645
55. Castro E, Goh C, Olmos D, Saunders E, Leongamornlert D, Tymrakiewicz M, et al. Germline BRCA mutations are associated with higher risk of nodal involvement, distant metastasis, and poor survival outcomes in prostate cancer. J Clin Oncol (2013) 31(14):1748–57. doi: 10.1200/JCO.2012.43.1882
56. Vietri MT, D’Elia G, Caliendo G, Resse M, Casamassimi A, Passariello L, et al. Hereditary prostate cancer: Genes related, target therapy and prevention. Int J Mol Sci (2021) 22(7):3753. doi: 10.3390/ijms22073753
57. Ewing CM, Ray AM, Lange EM, Zuhlke KA, Robbins CM, Tembe WD, et al. Germline mutations in HOXB13 and prostate-cancer risk. New Engl J Med (2012) 366(2):141–9. doi: 10.1056/NEJMoa1110000
58. Karlsson R, Aly M, Clements M, Zheng L, Adolfsson J, Xu J, et al. A population-based assessment of germline HOXB13 G84E mutation and prostate cancer risk. Eur Urology. (2014) 65(1):169–76. doi: 10.1016/j.eururo.2012.07.027
59. Lu X, Fong KW, Gritsina G, Wang F, Wang F, Baca SC, Brea LT, et al. HOXB13 suppresses de novo lipogenesis through HDAC3-mediated epigenetic reprogramming in prostate cancer. Nat Genet (2022) 54(5):670–83. doi: 10.1038/s41588-022-01045-8
60. Aurilio G, Cimadamore A, Mazzucchelli R, Lopez-Beltran A, Verri E, Scarpelli M, et al. Androgen receptor signaling pathway in prostate cancer: From genetics to clinical applications. Cells. (2020) 9(12):2653. doi: 10.3390/cells9122653
61. Wang Q, Li W, Zhang Y, Yuan X, Xu K, Yu J, et al. Androgen receptor regulates a distinct transcription program in androgen-independent prostate cancer. Cell. (2009) 138(2):245–56. doi: 10.1016/j.cell.2009.04.056
62. Itkonen H, Mills IG. Chromatin binding by the androgen receptor in prostate cancer. Mol Cell Endocrinology. (2012) 360(1):44–51. doi: 10.1016/j.mce.2011.09.037
63. Marcelli M, Stenoien DL, Szafran AT, Simeoni S, Agoulnik IU, Weigel NL, et al. Quantifying effects of ligands on androgen receptor nuclear translocation, intranuclear dynamics, and solubility. J Cell Biochem (2006) 98(4):770–88. doi: 10.1002/jcb.20593
64. Fitzgerald KA, Evans JC, McCarthy J, Guo J, Prencipe M, Kearney M, et al. The role of transcription factors in prostate cancer and potential for future RNA interference therapy. Expert Opin Ther Targets. (2014) 18(6):633–49. doi: 10.1517/14728222.2014.896904
65. Hankey W, Chen Z, Wang Q. Shaping chromatin states in prostate cancer by pioneer transcription factors. Cancer Res (2020) 80(12):2427–36. doi: 10.1158/0008-5472.CAN-19-3447
66. Fujita K, Nonomura N. Role of androgen receptor in prostate cancer: A review. World J Mens Health (2019) 37(3):288. doi: 10.5534/wjmh.180040
67. Paschalis A, Sharp A, Welti JC, Neeb A, Raj GV, Luo J, et al. Alternative splicing in prostate cancer. Nat Rev Clin Oncol (2018) 15(11):663–75. doi: 10.1038/s41571-018-0085-0
68. Heinlein CA, Chang C. Androgen receptor in prostate cancer. Endocrine Rev (2004) 25(2):276–308. doi: 10.1210/er.2002-0032
69. Taheri M, Khoshbakht T, Jamali E, Kallenbach J, Ghafouri-Fard S, Baniahmad A. Interaction between non-coding RNAs and androgen receptor with an especial focus on prostate cancer. Cells. (2021) 10(11):3198. doi: 10.3390/cells10113198
70. Magi-Galluzzi C, Tsusuki T, Elson P, Simmerman K, LaFargue C, Esgueva R, et al. TMPRSS2-ERG gene fusion prevalence and class are significantly different in prostate cancer of Caucasian, African-American and Japanese patients. Prostate. (2011) 71(5):489–97. doi: 10.1002/pros.21265
71. Xiao Q, Sun Y, Dobi A, Srivastava S, Wang W, Srivastava S, et al. Systematic analysis reveals molecular characteristics of ERG-negative prostate cancer. Sci Rep (2018) 8(1):12868. doi: 10.1038/s41598-018-30325-9
72. Pettersson A, Graff RE, Bauer SR, Pitt MJ, Lis RT, Stack EC, et al. The TMPRSS2:ERG rearrangement, ERG expression, and prostate cancer outcomes: a cohort study and meta-analysis. Cancer Epidemiol Biomarkers Prev (2012) 21(9):1497–509. doi: 10.1158/1055-9965.EPI-12-0042
73. Halsall JA, Andrews S, Krueger F, Rutledge CE, Ficz G, Reik W, et al. Histone modifications form a cell-type-specific chromosomal bar code that persists through the cell cycle. Sci Rep (2021) 11(1):3009. doi: 10.1038/s41598-021-82539-z
75. Pal S, Tyler JK. Epigenetics and aging. Sci Adv (2016) 2(7):e1600584. doi: 10.1126/sciadv.1600584
76. Lee BH, Rhie SK. Molecular and computational approaches to map regulatory elements in 3D chromatin structure. Epigenet Chromatin. (2021) 14:14. doi: 10.1186/s13072-021-00390-y
77. Rhie SK, Perez AA, Lay FD, Schreiner S, Shi J, Polin J, et al. A high-resolution 3D epigenomic map reveals insights into the creation of the prostate cancer transcriptome. Nat Commun (2019) 10(1):4154. doi: 10.1038/s41467-019-12079-8
78. Bolton EM, Tuzova AV, Walsh AL, Lynch T, Perry AS. Noncoding RNAs in prostate cancer:the long and the short of it. Clin Cancer Res (2014) 20(1):35–43. doi: 10.1158/1078-0432.CCR-13-1989
79. Vaisvila R, Ponnaluri VKC, Sun Z, Langhorst BW, Saleh L, Guan S, et al. Enzymatic methyl sequencing detects DNA methylation at single-base resolution from picograms of DNA. Genome Res (2021) 31(7):1280–9. doi: 10.1101/gr.266551.120
80. Ku JL, Jeon YK, Park JG. Methylation-specific PCR. Methods Mol Biol (2011) 791:23–32. doi: 10.1007/978-1-61779-316-5_3
81. Kurdyukov S, Bullock M. DNA Methylation analysis: Choosing the right method. Biology. (2016) 5(1):3. doi: 10.3390/biology5010003
82. Delaney C, Garg SK, Yung R. Analysis of DNA methylation by pyrosequencing. In: Shaw AC, editor. Immunosenescence. New York, NY: Springer New York (2015). p. 249–64. doi: 10.1007/978-1-4939-2963-4_19
83. Zhao F, Bapat B. Chapter 8 - the role of methylation-specific PCR and associated techniques in clinical diagnostics. In: García-Giménez JL, editor. Epigenetic biomarkers and diagnostics. Boston: Academic Press (2016). p. 155–73. Available at: https://www.sciencedirect.com/science/article/pii/B9780128018996000085.
84. Karimi M, Johansson S, Stach D, Corcoran M, Grandér D, Schalling M, et al. LUMA (LUminometric methylation assay)–a high throughput method to the analysis of genomic DNA methylation. Exp Cell Res (2006) 312(11):1989–95. doi: 10.1016/j.yexcr.2006.03.006
85. Yegnasubramanian S, Lin X, Haffner MC, DeMarzo AM, Nelson WG. Combination of methylated-DNA precipitation and methylation-sensitive restriction enzymes (COMPARE-MS) for the rapid, sensitive and quantitative detection of DNA methylation. Nucleic Acids Res (2006) 34(3):e19. doi: 10.1093/nar/gnj022
86. Yong WS, Hsu FM, Chen PY. Profiling genome-wide DNA methylation. Epigenet Chromatin. (2016) 9:26. doi: 10.1186/s13072-016-0075-3
87. Neary JL, Perez SM, Peterson K, Lodge DJ, Carless MA. Comparative analysis of MBD-seq and MeDIP-seq and estimation of gene expression changes in a rodent model of schizophrenia. Genomics. (2017) 109(3–4):204–13. doi: 10.1016/j.ygeno.2017.03.004
88. Du P, Zhang X, Huang CC, Jafari N, Kibbe WA, Hou L, et al. Comparison of beta-value and m-value methods for quantifying methylation levels by microarray analysis. BMC Bioinf (2010) 11(1):587. doi: 10.1186/1471-2105-11-587
89. Pidsley R, Wong CC Y, Volta M, Lunnon K, Mill J, Schalkwyk LC. A data-driven approach to preprocessing illumina 450K methylation array data. BMC Genomics (2013) 14(1):293. doi: 10.1186/1471-2164-14-293
90. Huang YW, Huang THM, Wang LS. Profiling DNA methylomes from microarray to genome-scale sequencing. Technol Cancer Res Treat (2010) 9(2):139–47. doi: 10.1177/153303461000900203
91. Zhou W, Laird PW, Shen H. Comprehensive characterization, annotation and innovative use of infinium DNA methylation BeadChip probes. Nucleic Acids Res (2017) 45(4):e22. doi: 10.1093/nar/gkw967
92. Sun Z, Cunningham J, Slager S, Kocher JP. Base resolution methylome profiling: considerations in platform selection, data preprocessing and analysis. Epigenomics. (2015) 7(5):813–28. doi: 10.2217/epi.15.21
93. Robertson KD. DNA Methylation and human disease. Nat Rev Genet (2005) 6(8):597–610. doi: 10.1038/nrg1655
94. Mullen DJ, Yan C, Kang DS, Zhou B, Borok Z, Marconett CN, et al. TENET 2.0: Identification of key transcriptional regulators and enhancers in lung adenocarcinoma. PloS Genet (2020) 16(9):e1009023. doi: 10.1371/journal.pgen.1009023
95. Rhie SK, Guo Y, Tak YG, Yao L, Shen H, Coetzee GA, et al. Identification of activated enhancers and linked transcription factors in breast, prostate, and kidney tumors by tracing enhancer networks using epigenetic traits. Epigenet Chromatin. (2016) 9(1):50. doi: 10.1186/s13072-016-0102-4
96. Berman BP, Weisenberger DJ, Aman JF, Hinoue T, Ramjan Z, Liu Y, et al. Regions of focal DNA hypermethylation and long-range hypomethylation in colorectal cancer coincide with nuclear lamina–associated domains. Nat Genet (2011) 44(1):40–6. doi: 10.1038/ng.969
97. Shen H, Laird PW. Interplay between the cancer genome and epigenome. Cell. (2013) 153(1):38–55. doi: 10.1016/j.cell.2013.03.008
98. Zhao SG, Chen WS, Li H, Foye A, Zhang M, Sjöström M, et al. DNA Methylation landscapes in advanced prostate cancer. Nat Genet (2020) 52(8):778–89. doi: 10.1038/s41588-020-0648-8
99. Lam D, Clark S, Stirzaker C, Pidsley R. Advances in prognostic methylation biomarkers for prostate cancer. Cancers (Basel). (2020) 12(10):E2993. doi: 10.3390/cancers12102993
100. Yegnasubramanian S, Kowalski J, Gonzalgo ML, Zahurak M, Piantadosi S, Walsh PC, et al. Hypermethylation of CpG islands in primary and metastatic human prostate cancer. Cancer Res (2004) 64(6):1975–86. doi: 10.1158/0008-5472.CAN-03-3972
101. Rosenbaum E, Hoque MO, Cohen Y, Zahurak M, Eisenberger MA, Epstein JI, et al. Promoter hypermethylation as an independent prognostic factor for relapse in patients with prostate cancer following radical prostatectomy. Clin Cancer Res (2005) 11(23):8321–5. doi: 10.1158/1078-0432.CCR-05-1183
102. Woodson K, O’Reilly KJ, Ward DE, Walter J, Hanson J, Walk EL, et al. CD44 and PTGS2 methylation are independent prognostic markers for biochemical recurrence among prostate cancer patients with clinically localized disease. Epigenetics. (2006) 1(4):183–6. doi: 10.4161/epi.1.4.3530
103. Henrique R, Ribeiro FR, Fonseca D, Hoque MO, Carvalho AL, Costa VL, et al. High promoter methylation levels of APC predict poor prognosis in sextant biopsies from prostate cancer patients. Clin Cancer Res (2007) 13(20):6122–9. doi: 10.1158/1078-0432.CCR-07-1042
104. Ellinger J, Bastian PJ, Jurgan T, Biermann K, Kahl P, Heukamp LC, et al. CpG island hypermethylation at multiple gene sites in diagnosis and prognosis of prostate cancer. Urology. (2008) 71(1):161–7. doi: 10.1016/j.urology.2007.09.056
105. Alumkal JJ, Zhang Z, Humphreys EB, Bennett C, Mangold LA, Carducci MA, et al. Impact of DNA methylation on the identification of aggressive prostate cancer. Urology. (2008) 72(6):1234–9. doi: 10.1016/j.urology.2007.12.060
106. Weiss G, Cottrell S, Distler J, Schatz P, Kristiansen G, Ittmann M, et al. DNA Methylation of the PITX2 gene promoter region is a strong independent prognostic marker of biochemical recurrence in patients with prostate cancer after radical prostatectomy. J Urol. (2009) 181(4):1678–85. doi: 10.1016/j.juro.2008.11.120
107. Vanaja DK, Ehrich M, Van den Boom D, Cheville JC, Karnes RJ, Tindall DJ, et al. Hypermethylation of genes for diagnosis and risk stratification of prostate cancer. Cancer Invest. (2009) 27(5):549–60. doi: 10.1080/07357900802620794
108. Richiardi L, Fiano V, Vizzini L, De Marco L, Delsedime L, Akre O, et al. Promoter methylation in APC, RUNX3, and GSTP1 and mortality in prostate cancer patients. J Clin Oncol (2009) 27(19):3161–8. doi: 10.1200/JCO.2008.18.2485
109. Bañez LL, Sun L, van Leenders GJ, Wheeler TM, Bangma CH, Freedland SJ, et al. Multicenter clinical validation of PITX2 methylation as a prostate specific antigen recurrence predictor in patients with post-radical prostatectomy prostate cancer. J Urol. (2010) 184(1):149–56. doi: 10.1016/j.juro.2010.03.012
110. Vasiljeviš N, Wu K, Brentnall AR, Kim DC, Thorat MA, Kudahetti SC, et al. Absolute quantitation of DNA methylation of 28 candidate genes in prostate cancer using pyrosequencing. Dis Markers. (2011) 30(4):151–61. doi: 10.1155/2011/157829
111. Rosenbaum E, Begum S, Brait M, Zahurak M, Maldonado L, Eisenberger MA, et al. Aim1 promoter hypermethylation as a predictor of decreased risk of recurrence following radical prostatectomy. Prostate. (2012) 72(10):1133–9. doi: 10.1002/pros.22461
112. Vasiljević N, Ahmad AS, Beesley C, Thorat MA, Fisher G, Berney DM, et al. Association between DNA methylation of HSPB1 and death in low Gleason score prostate cancer. Prostate Cancer Prostatic Dis (2013) 16(1):35–40. doi: 10.1038/pcan.2012.47
113. Dietrich D, Hasinger O, Bañez LL, Sun L, van Leenders GJ, Wheeler TM, et al. Development and clinical validation of a real-time PCR assay for PITX2 DNA methylation to predict prostate-specific antigen recurrence in prostate cancer patients following radical prostatectomy. J Mol Diagn. (2013) 15(2):270–9. doi: 10.1016/j.jmoldx.2012.11.002
114. Richiardi L, Fiano V, Grasso C, Zugna D, Delsedime L, Gillio-Tos A, et al. Methylation of APC and GSTP1 in non-neoplastic tissue adjacent to prostate tumour and mortality from prostate cancer. PloS One (2013) 8(7):e68162. doi: 10.1371/journal.pone.0068162
115. Moritz R, Ellinger J, Nuhn P, Haese A, Müller SC, Graefen M, et al. DNA Hypermethylation as a predictor of PSA recurrence in patients with low- and intermediate-grade prostate cancer. Anticancer Res (2013) 33(12):5249–54.
116. Vasiljević N, Ahmad AS, Thorat MA, Fisher G, Berney DM, Møller H, et al. DNA Methylation gene-based models indicating independent poor outcome in prostate cancer. BMC Cancer. (2014) 14:655. doi: 10.1186/1471-2407-14-655
117. Vasiljević N, Ahmad AS, Carter PD, Fisher G, Berney DM, Foster CS, et al. DNA Methylation of PITX2 predicts poor survival in men with prostate cancer. biomark Med (2014) 8(9):1143–50. doi: 10.2217/bmm.14.41
118. Maldonado L, Brait M, Loyo M, Sullenberger L, Wang K, Peskoe SB, et al. GSTP1 promoter methylation is associated with recurrence in early stage prostate cancer. J Urol. (2014) 192(5):1542–8. doi: 10.1016/j.juro.2014.04.082
119. Daniunaite K, Jarmalaite S, Kalinauskaite N, Petroska D, Laurinavicius A, Lazutka JR, et al. Prognostic value of RASSF1 promoter methylation in prostate cancer. J Urology. (2014) 192(6):1849–55. doi: 10.1016/j.juro.2014.06.075
120. Litovkin K, Van Eynde A, Joniau S, Lerut E, Laenen A, Gevaert T, et al. DNA Methylation-guided prediction of clinical failure in high-risk prostate cancer. PloS One (2015) 10(6):e0130651. doi: 10.1371/journal.pone.0130651
121. Carozzi F, Tamburrino L, Bisanzi S, Marchiani S, Paglierani M, Di Lollo S, et al. Are biomarkers evaluated in biopsy specimens predictive of prostate cancer aggressiveness? J Cancer Res Clin Oncol (2016) 142(1):201–12. doi: 10.1007/s00432-015-2015-1
122. Rybicki BA, Rundle A, Kryvenko ON, Mitrache N, Do KC, Jankowski M, et al. Methylation in benign prostate and risk of disease progression in men subsequently diagnosed with prostate cancer. Int J Cancer. (2016) 138(12):2884–93. doi: 10.1002/ijc.30038
123. Holmes EE, Goltz D, Sailer V, Jung M, Meller S, Uhl B, et al. PITX3 promoter methylation is a prognostic biomarker for biochemical recurrence-free survival in prostate cancer patients after radical prostatectomy. Clin Epigenetics. (2016) 8:104. doi: 10.1186/s13148-016-0270-x
124. Ahmad AS, Vasiljević N, Carter P, Berney DM, Møller H, Foster CS, et al. A novel DNA methylation score accurately predicts death from prostate cancer in men with low to intermediate clinical risk factors. Oncotarget. (2016) 7(44):71833–40. doi: 10.18632/oncotarget.12377
125. Uhl B, Gevensleben H, Tolkach Y, Sailer V, Majores M, Jung M, et al. PITX2 DNA methylation as biomarker for individualized risk assessment of prostate cancer in core biopsies. J Mol Diagn. (2017) 19(1):107–14. doi: 10.1016/j.jmoldx.2016.08.008
126. Tang D, Kryvenko ON, Mitrache N, Do KC, Jankowski M, Chitale DA, et al. Methylation of the RARB gene increases prostate cancer risk in black americans. J Urol. (2013) 190(1):317–24. doi: 10.1016/j.juro.2013.01.083
127. Stanford JL, Wicklund KG, McKnight B, Daling JR, Brawer MK. Vasectomy and risk of prostate Cancer1. Cancer Epidemiology Biomarkers Prev (1999) 8(10):881–6.
128. Agalliu I, Salinas CA, Hansten PD, Ostrander EA, Stanford JL. Statin use and risk of prostate cancer: Results from a population-based epidemiologic study. Am J Epidemiol. (2008) 168(3):250–60. doi: 10.1093/aje/kwn141
129. Weineisen M, Schottelius M, Simecek J, Baum RP, Yildiz A, Beykan S, et al. 68 Ga- and 177 Lu-labeled PSMA I&T: Optimization of a PSMA-targeted theranostic concept and first proof-of-Concept human studies. J Nucl Med (2015) 56(8):1169–76. doi: 10.2967/jnumed.115.158550
130. Paschalis A, Sheehan B, Riisnaes R, Rodrigues DN, Gurel B, Bertan C, et al. Prostate-specific membrane antigen heterogeneity and DNA repair defects in prostate cancer. Eur Urol. (2019) 76(4):469–78. doi: 10.1016/j.eururo.2019.06.030
131. Stark R, Grzelak M, Hadfield J. RNA Sequencing: the teenage years. Nat Rev Genet (2019) 20(11):631–56. doi: 10.1038/s41576-019-0150-2
132. Wang Z, Gerstein M, Snyder M. RNA-Seq: A revolutionary tool for transcriptomics. Nat Rev Genet (2009) 10(1):57–63. doi: 10.1038/nrg2484
133. Okoniewski MJ, Miller CJ. Hybridization interactions between probesets in short oligo microarrays lead to spurious correlations. BMC Bioinf (2006) 7(1):276. doi: 10.1186/1471-2105-7-276
134. Ozsolak F, Milos PM. RNA Sequencing: Advances, challenges and opportunities. Nat Rev Genet (2011) 12(2):87–98. doi: 10.1038/nrg2934
135. Yang L, Duff MO, Graveley BR, Carmichael GG, Chen LL. Genomewide characterization of non-polyadenylated RNAs. Genome Biol (2011) 12(2):R16. doi: 10.1186/gb-2011-12-2-r16
136. O’Neil D, Glowatz H, Schlumpberger M. Ribosomal RNA depletion for efficient use of RNA-seq capacity. Curr Protoc Mol Biol (2013) 103(1):4. doi: 10.1002/0471142727.mb0419s103
137. Vickman RE, Franco OE, Moline DC, Vander Griend DJ, Thumbikat P, Hayward SW. The role of the androgen receptor in prostate development and benign prostatic hyperplasia: A review. Asian J Urol. (2020) 7(3):191–202. doi: 10.1016/j.ajur.2019.10.003
138. Bernasocchi T, El Tekle G, Bolis M, Mutti A, Vallerga A, Brandt LP, et al. Dual functions of SPOP and ERG dictate androgen therapy responses in prostate cancer. Nat Commun (2021) 12(1):734. doi: 10.1038/s41467-020-20820-x
139. Adams EJ, Karthaus WR, Hoover E, Liu D, Gruet A, Zhang Z, et al. FOXA1 mutations alter pioneering activity, differentiation, and prostate cancer phenotypes. Nature. (2019) 571(7765):408–12. doi: 10.1038/s41586-019-1318-9
140. Antonarakis ES, Lu C, Wang H, Luber B, Nakazawa M, Roeser JC, et al. AR-V7 and resistance to enzalutamide and abiraterone in prostate cancer. N Engl J Med (2014) 371(11):1028–38. doi: 10.1056/NEJMoa1315815
141. Takeda DY, Spisák S, Seo JH, Bell C, O’Connor E, Korthauer K, et al. A somatically acquired enhancer of the androgen receptor is a noncoding driver in advanced prostate cancer. Cell. (2018) 174(2):422. doi: 10.1016/j.cell.2018.05.037
142. Ateeq B, Tomlins SA, Laxman B, Asangani IA, Cao Q, Cao X, et al. Therapeutic targeting of SPINK1-positive prostate cancer. Sci Transl Med (2011) 3(72):72ra17. doi: 10.1126/scitranslmed.3001498
143. Shorning BY, Dass MS, Smalley MJ, Pearson HB. The PI3K-AKT-mTOR pathway and prostate cancer: At the crossroads of AR, MAPK, and WNT signaling. Int J Mol Sci (2020) 21(12):4507. doi: 10.3390/ijms21124507
144. Frank S, Nelson P, Vasioukhin V. Recent advances in prostate cancer research: large-scale genomic analyses reveal novel driver mutations and DNA repair defects. F1000Res (2018) 7(F1000 Faculty Rev):1173. doi: 10.12688/f1000research.14499.1
145. Fang ZQ, Zang WD, Chen R, Ye BW, Wang XW, Yi SH, et al. Gene expression profile and enrichment pathways in different stages of bladder cancer. Genet Mol Res (2013) 12(2):1479–89. doi: 10.4238/2013.May.6.1
146. Liu X, Wang J, Sun G. Identification of key genes and pathways in renal cell carcinoma through expression profiling data. Kidney Blood Press Res (2015) 40(3):288–97. doi: 10.1159/000368504
147. Arenas-Gallo C, Owiredu J, Weinstein I, Lewicki P, Basourakos SP, Vince R, et al. Race and prostate cancer: genomic landscape. Nat Rev Urol. (2022) 19(9):547–61. doi: 10.1038/s41585-022-00622-0
Keywords: prostate cancer, racial disparity, African American (AA), European American (EA), genomics, epigenomics, transcriptomics
Citation: Stevens C, Hightower A, Buxbaum SG, Falzarano SM and Rhie SK (2023) Genomic, epigenomic, and transcriptomic signatures of prostate cancer between African American and European American patients. Front. Oncol. 13:1079037. doi: 10.3389/fonc.2023.1079037
Received: 24 October 2022; Accepted: 10 February 2023;
Published: 28 February 2023.
Edited by:
Masaki Shiota, Kyushu University, JapanReviewed by:
Emilie Lalonde, London Health Sciences Centre, CanadaChristopher M. Heaphy, Boston University, United States
Copyright © 2023 Stevens, Hightower, Buxbaum, Falzarano and Rhie. This is an open-access article distributed under the terms of the Creative Commons Attribution License (CC BY). The use, distribution or reproduction in other forums is permitted, provided the original author(s) and the copyright owner(s) are credited and that the original publication in this journal is cited, in accordance with accepted academic practice. No use, distribution or reproduction is permitted which does not comply with these terms.
*Correspondence: Suhn K. Rhie, cmhpZUB1c2MuZWR1