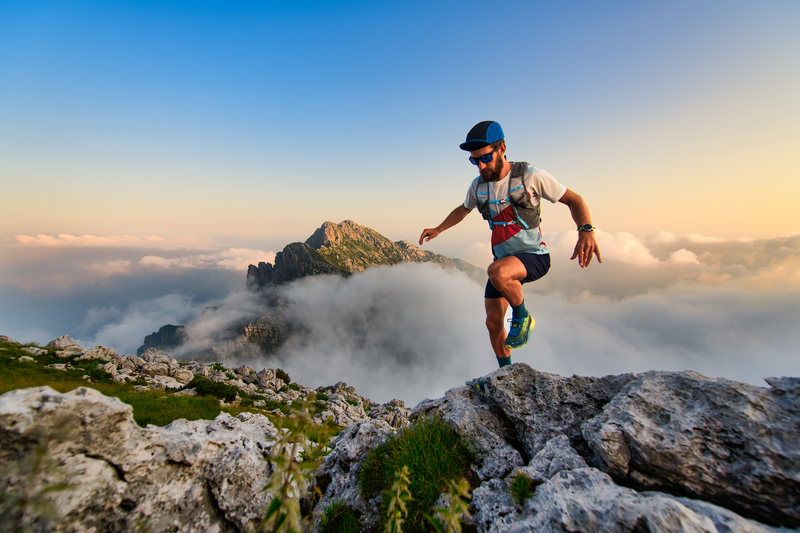
94% of researchers rate our articles as excellent or good
Learn more about the work of our research integrity team to safeguard the quality of each article we publish.
Find out more
REVIEW article
Front. Oncol. , 25 May 2023
Sec. Gastrointestinal Cancers: Gastric and Esophageal Cancers
Volume 13 - 2023 | https://doi.org/10.3389/fonc.2023.1074268
This article is part of the Research Topic Biomarkers, Functional Mechanisms, and Therapeutic Potentials in Gastrointestinal Cancers View all 40 articles
Gastric cancer is one of the most serious malignant tumor and threatens the health of people worldwide. Its heterogeneity leaves many clinical problems unsolved. To treat it effectively, we need to explore its heterogeneity. Single-cell transcriptome sequencing, or single-cell RNA sequencing (scRNA-seq), reveals the complex biological composition and molecular characteristics of gastric cancer at the level of individual cells, which provides a new perspective for understanding the heterogeneity of gastric cancer. In this review, we first introduce the current procedure of scRNA-seq, and discuss the advantages and limitations of scRNA-seq. We then elaborate on the research carried out with scRNA-seq in gastric cancer in recent years, and describe how it reveals cell heterogeneity, the tumor microenvironment, oncogenesis and metastasis, as well as drug response in to gastric cancer, to facilitate early diagnosis, individualized therapy, and prognosis evaluation.
Gastric cancer is a type of malignant tumor of epithelial origin and ranks fourth among all types of cancer worldwide (1). Due to its insidious early symptoms, most patients were diagnosed at an advanced stage, and the five-year survival rate is still less than 5% for patients with distant metastasis (2). Current treatment options, such as surgery, radiotherapy, chemotherapy, and targeted therapy, have achieved some clinical benefits, but still suffer from recurrence, metastasis, and drug resistance (3). Researchers are increasingly focusing on tumor heterogeneity in addressing these questions (4). In terms of histology, genomics, epigenetics, and other aspects, gastric cancer is increasingly recognized as one of the most heterogeneous tumor types (5). Identifying the intrinsic characteristics of gastric cancer tumors in different patients can help to pave the way for personalized therapy. However, several existing classifications, including Lauren’s (6), The Cancer Genome Atlas (TCGA) (7), and Asian Cancer Research Group (ACRG) (8), have contributed to the advancement of understanding gastric cancer, but due to numerous complex factors, it is still not enough to classify and treat all patients precisely. Single-cell transcriptome sequencing represents a new approach for studying the heterogeneity of gastric cancer.
Single-cell transcriptome sequencing, or single-cell RNA sequencing (scRNA-seq), has emerged as one of the technologies for next-generation sequencing (9). Rather than traditional bulk RNA sequencing (RNA-seq), which averages the gene expression levels of all cells, scRNA-seq transcripts of each cell, enable unprecedented recognition of the gene expression profiles of individual cells. In addition to discovering differences in cellular composition and characteristics, certain rare cell populations that are obscured by bulk RNA-seq are identified by using scRNA-seq (10). At the same time, by examining the tumor microenvironment at the level of individual cell, scRNA-seq has helped identify the valuable role of non-tumor cells in tumor development (11). Moreover, metastatic samples have been used to identify intrinsic features associated with metastatic for targeted therapy (12, 13). Analyzing pre- and post-treatment samples assists in discovering intrinsic mechanisms affecting drug response and paving the way for individualized therapy (14, 15). As scRNA-seq technology develops and costs go down, an increasing number of studies are being conducted on the technique. Currently, studies are being conducted on this technology in a number of tumor types, including gastric cancer, melanoma (16), lung cancer (17), liver cancer (18), and pancreatic cancer (19).
Here, we review and summarize the current basic procedure of scRNA-seq and analyze its advantages and limitations. We then present new insights into scRNA-seq in gastric cancer research, and discuss its challenges and application prospects based on the latest research results. Meanwhile, it is expected to provide some help in the diagnosis, treatment, and prognosis evaluation of gastric cancer.
The scRNA-seq procedure consists of the following steps: single cell isolation, library construction, sequencing, and data analysis. Figure 1 shows an overview procedure of scRNA-seq.
Samples of gastric cancer are typically obtained through endoscopic or surgical resection. After mechanical fragmentation of the tissue, enzymatic digestion was performed to obtain a single cell suspension (20). The enzymatic digestion time must be controlled. A short digestion time leads to incomplete digestion, resulting in cell clumps and doublet. Overly long digestion times can damage cells. To ensure that the analysis of sequencing results is not affected by altered cell state, it is important to evaluate the activity of single cell suspensions before sequencing, for example, by using trypan blue stain to determine cell viability. Moreover, specific cell types can be enriched and screened. Fluorescence-activated cell sorting (FACS) allows for the identification of fluorescently labeled cell-specific marker genes, which can be used for screening and enrichment of specific types of cells (20). Additionally, to prevent experimental failures caused by cell viability issues, sample processing and sequencing should be completed in a short period of time to minimize damage to cell viability.
RNA was obtained from cell lysis, followed by reverse transcription to obtain cDNA. The amplification and fragmentation of reverse transcription products are followed by ligating adapters to form a cDNA library (21). The library is constructed, and then is sequenced. Current sequencing methods can be divided into microwell-based methods, such as Smart-Seq2 (22), and droplet-based methods (23, 24), such as 10X Genomics (25). The following is a description of the most commonly used methods, Smart-Seq2 and 10X Genomics. Smart-Seq2 improves the capture of shorter transcripts and identifies transcriptional modifications, such as gene shearing and allelic expression, when full-length transcripts are sequenced (24), but the number of cells processed is limited. The 10X Genomics system uses microfluidics-based approaches to sequence genes by the 5’ or 3’ ends, which can process up to thousands of single cells per second, enabling faster sequences of samples with larger number of cells (26). It is more suitable to sequence samples with a larger number of cells. Unique molecular identifiers (UMIs), which are short barcodes attached to transcripts by 10X Genomics before amplification, allow 10X Genomics to avoid counting the same reverse transcription products twice. The expression level can be more accurately evaluated by reducing quantitative bias during amplification with UMIs in the 10X Genomics method (24, 27).
In data analysis, raw expression matrixes are processed, as well as downstream analysis. The processing of the original expression matrix, includes cell filtering, normalization, dimensionality reduction, clustering, and data integration (28). Data must be preprocessed. The use of cell filtering can reduce the interference caused by low-quality cells. Examples include poorly active cells that express high levels of mitochondrial genes and the artifacts caused by doublet. Dimensional catastrophe can be alleviated through dimension reduction. Using clustering, cells with similar characteristics were grouped together for annotation and further analysis. As single-cell sequencing involves batch effects and technical noise, integration is essential to reduce them so that they can be distinguished from biological differences.
Annotation of cells is typically performed before downstream analysis. Cell annotation in data analysis is a challenging task. The large number of cells make it difficult to annotate cells one by one. The current cluster-based cell annotation method assumes that all cells within a cluster are of the same type. However, annotation of new cell types will be difficult due to the lack of a uniform standard. Fortunately, the Human Cell Atlas provides a powerful guide for determining cell types (29). Additionally, cell marker genes reported in the available literature and machine learning methods can also be used to identify cell types (30).
The downstream analysis consists of a variety of procedures, such as cell abundance, differentiation gene expression, trajectory analysis, copy number variation (CNV) analysis, cell-cell interaction, and transcription factor analysis (31). For the cell ratio, the composition varies among patients at the level of single cells, demonstrating heterogeneity between them. Analysis of the differentiation gene expression and functional enrichments across each cell subpopulation showed their cell states and functions (32). Furthermore, some analysis tools can be used to perform various types of analyses. With tools such as Monocle (33) and Slingshot (34), trajectory analysis can demonstrate the dynamic process of cellular states, such as plasma cells, which have different stages of maturation in gastric cancer (35). Biological mechanisms underlie the transformation process and can be traced to cellular characteristics. InferCNV (36) and copyKAT (37) analyses of copy number variation can provide insights into chromosomal variation and help to determine the malignancy of tumor cells (16). Based on the expression levels of ligands and receptors, CellChat (38) and cellphonedb (39), for example, can assess in the construction of intercellular communication, which is a great resource to understand the complex interactions between cells and the role they play. Taking an example, the stromal cells in diffuse gastric cancer interact more frequently with other cell types (40), which may play an important role. By screening transcription factors and rebuilding gene regulatory networks, SCENIC (41) can enhance our understanding of tumor molecular regulation mechanisms at the level of individual cells.
ScRNA-seq profiles the transcriptome at the level of single cell, uses a variety of analytical methods, and is exceptionally advantageous for identifying gastric cancer heterogeneity. The sequencing depth of scRNA-seq, however, limits it to detecting only genes with relatively high expression abundance. Increased sequencing depth will allow more genes to be detected, but the ensuing increase in data volume and technical noise will make the algorithm more challenging (42). Although improvements to single cell technology are still needed, the constant development of single-cell RNA sequencing is expected to resolve the aforementioned issues.
Increasingly, scRNA-seq is being used in gastric cancer studies due to its advantages and its achievements in other tumor studies. Samples for scRNA-seq studies of gastric cancer are currently available from a variety of sexes, disease stages, pathological types, and molecular subtypes. Table 1 shows a summary of single-cell sequencing data currently available for gastric cancer research.
In normal gastric mucosa, there are a variety of cells, including pit cells, enteroendocrine cells, parietal cells, neck cells, chief cells, goblet cells, stem cells, immune cells and stromal cells (59). More complexity will be found in gastric cancer tissues (60). ScRNA-seq identifies the transcriptome at the level of single cell and has unrivaled advantages for uncovering cell heterogeneity, which provides new insights into discovering new biomarkers, diversity of tumors, and lineage compositions. According to the Correa hypothesis, gastric cancer arises from chronic atrophic gastritis, intestinal metaplasia (IM), and ultimately gastric cancer (61). Based on the Correa hypothesis, a study of gastric lesions at different stages found that a subpopulation of secretory pro-genitor markers goblet cells expresses HES6 during intestinal metaplasia. A population of these cells may represent goblet cells at an early stage of differentiation, and HES6 will help identify precancerous lesions in high-risk patients. For early gastric cancer (EGC), the discovery of two new specific markers, SLC11A2 and KLK7, provides clues for the early detection of EGC (43). Gastric cancer cells can also be heterogeneous. The scRNA-seq discrimination of gastric cancer cells is expected to lead to the stratification of patients based on the characteristics of cancer cells. In the study by Zhang et al, all malignant cells were divided into five groups: C1 is low differentiation cells mainly from diffuse gastric cancer (DGC) samples, C2 is high differentiation cells mainly from intestinal gastric cancer (IGC) samples, C3 is a mixed type with medium differentiation cells between C1 and C2, C4 is an entity fundic gland-type GA (GA-FG-CCP), and C5 is Epstein-Barr virus-infected type. Subgroups vary in their degree of differentiation, which correlates with the prognosis of patients (44). Since sample sizes are limited, scRNA-seq-based stratification of gastric cancer remains to be confirmed in large-scale sequencing data and evaluated for clinical value in guiding treatment and prognosis of patients.
In addition, there is heterogeneity within subtypes of gastric cancer. Based on cell state and characteristics, scRNA-seq provides new insight into gastric cancer development mechanisms in transcriptional dynamics. Lauren classifications divide gastric cancers into intestinal, diffuse, and mixed types (62). A Lauren classification-based study compared the trajectories of epithelial cells in gastric cancers of the intestinal type and diffuse type, and showed the different carcinogenesis mechanisms. IGC shows dynamic changes from non-malignant to tumor cells, with IGC marker genes such as MUC13 and CDH17 expressing higher levels over time, and the risk of tumorigenesis increased accordingly, supporting the Correa hypothesis. In contrast, in DGC, the expression of markers genes of IM and IGC does not change with stage that according to cell lineage, and has no relationship with the pathological classification. The carcinogenic mechanism of DGC is different from IGC (45). In specific types of gastric cancer, transcriptional dynamics differed as well. In a study of hepatoid adenocarcinoma of the stomach (HAS), all epithelial cells from HAS expressed cancer-related genes, and showed a significant copy number of variations from common gastric cancer. Cancer cells of HAS may have been derived from pluripotent precursor cells, which then differentiated into corresponding epithelial cell lineages such as adenocarcinoma and hepatocyte-like components (46). Moreover, in animal models, scRNA-seq is also applicable. Hereditary diffuse gastric cancer (HDGC) is a cancer syndrome caused by inactivating germline mutations in CDH1, but its mechanism remains unclear. In a mouse organoid model of HDGC, deregulation of developmental transcriptional programs was found at the early stage of CDH1 deletion that are associated with differentiation of gastric squamous epithelial cells in mouse (47). All these studies demonstrate that scRNA-seq provides a powerful tool for research on the transcriptional dynamics and lineage compositions of gastric cancer.
Furthermore, new cell types were identified in large-scale scRNA-seq data. As an example, new cell types that express both endothelial cells and fibroblasts marker genes may be undergoing endothelial-mesenchymal transition (EMT) (35). A variety of gastric cancer cell lines also showed multiple clusters with differing characteristics (48). In part, this may explain why experimental reproducibility differs among cell lines.
In summary, gastric cancer shows cell heterogeneity. ScRNA-seq is a powerful tool for understanding the transcriptional dynamics and lineage compositions of gastric cancer.
All cells and their secretory products, along with the extracellular matrix, make up the tumor microenvironment (63). Immune cells and stromal cells contribute significantly to tumor invasion, metastasis, and drug resistance as non-tumor cells in the microenvironment (64). While bulk RNA-seq can be evaluated for immune infiltration with deconvolution, some limitations remain (65). In microenvironments, scRNA-seq can distinguish cell types at the level of single cell, and it has great advantages for studying the “dialog” and regulatory mechanisms between cells. As a major component of the tumor microenvironment, immune cells take part in tumor immunity response processes. In a study of ascites from patients with advanced gastric cancer (AGC), macrophages in GC malignant ascites have non-inflammatory characteristics compared with in vitro macrophage transcripts. Cancer cells interact with tumor-associated macrophages (TAMs) via IL1B-IL1R2 ligands and receptors, thereby inhibiting inflammatory signaling, and promoting tumor growth (49). Furthermore, tumors contain tertiary lymphatic structures (TLSs), which function as organized aggregates of immune cells, involved in the immune response process in tumors (66). A study found that tissues with mature tertiary lymphatic structures (mTLSs) contain more types of immune cells (67). This may result in more aggressive antitumor responses. However, previous studies have shown that regulatory T cells (Tregs) inhibit antitumor responses (68). ScRNA-seq also shows that immunosuppression is associated with increased Tregs in tumor microenvironment of gastric cancer (69). In another study, tumor microenvironment containing TNFR2-positive Tregs was associated with poor prognosis (50). An assessment of Tregs levels may provide prognostic information. There is a need to pay more attention to the interaction between tumor cells and immune cells, which will facilitate the development of new immunotherapy strategies. A study by Kumar et al. found that KLF2 expressed in epithelial cells was associated with the recruitment of plasma cells in IGC (35). KLF2 may be a potential therapeutic target for recruiting plasma cells in DGC based on their role in gastric cancer (70).
In the microenvironment, stromal cells produce cytokines and chemokines, which affect tumor growth and invasion by promoting extracellular matrix formation and angiogenesis (60). ScRNA-seq analysis also confirmed the significance of stromal cells in tumors. In DGC studies conducted at different sampling depths, there may be a relationship between cell types and the depth level of tumors. Deeper layers were enriched with endothelial, fibroblast, and myeloid cells. In comparison with superficial tumor layers, deeper layers have a more intense cell-cell communication (40). An analysis of the microenvironment of cascade changes in gastric cancer also confirmed the importance of these cells (71). It is notable that the deep enrichment of CCL2-expressing stromal cells in DGC correlates with its invasive ability (40). CCL2 may be a potential target for DGC interference. A study by Kim et al. differentiated tumor-associated fibroblasts (CAFs) into three types based on gene expression profiles: inflammatory (iCAFs), myofibroblastic (myCAFs), and intermediate (inCAFs). One of them, iCAFs, is closely related to GC invasion and promotes the stemness of tumor cells, which can identify patients at high-risk of GC (45). A comprehensive study of the diversity of gastric cancer fibroblasts would benefit from this research. Furthermore, a large-scale sequencing study revealed that INHBA is a positive regulator of FAP in the fibroblast population, and that high expression of these genes in CAFs is associated with poor prognosis (45). As seen in the microenvironment of tumors, stromal cells are also diverse, and appear to be potential therapeutic targets and prognostic indicators.
To conclude, scRNA-seq offers new options for understanding the heterogeneity of tumor microenvironment, which will provide more opportunities for targeted therapies of gastric cancer.
In terms of the mechanism of gastric carcinogenesis, there are still some controversies. Research suggests that long-term chronic inflammation induces intestinal metaplasia as a precursor to gastric cancer (72). Several studies have shown that the abnormal differentiation of chief cells will transform into neck cells, and the consequent development of spasmolytic polypeptide-expressing metaplasia (SPEM), which is associated with dysplasia and cancer (73). Using scRNA-seq, further evidence is presented for the role of SPEM in gastric cancer development. In a study by Zhang et al., cells expressing MUC6, TFF2, CD44, SOX9, and major histocompatibility complex class II genes were classified as SPEM cells. The results confirm SPEM at the level of single cell, suggesting a differentiation pathway between the chief cells and the neck cells, which in turn gives rise to SPEM cells (44). However, despite this, SPEM cells are not clearly defined. With the addition of scRNA-seq recognition, the definition of SPEM has been expanded. A study by Bockerstett et al. examined SPEM in a mouse model showed that the definition of SPEM should include TFFF2+Muc6+GIFF- metaplastic cells without mature principal cell transcripts, which may result in the same cancer risks as standard SPEM. Considering the complexity of the pathological mechanisms, the authors also examined the levels of SPEM transcript in drug-induced acute gastritis and chronic inflammation gastritis mouse models. The results show that SPEM cells are transcriptome-conserved across the two gastritis models (74). Another subsequent study by the same group revealed Gastrokine-3 (Gkn3) expression in the gastric body of chronic atrophic gastritis patients but not in healthy people. Both mouse models and human tissues have demonstrated the specificity of Gkn3 for SPEM recognition (75). Therefore, in chronic atrophic gastritis, identifying SPEM cells should include GKN3-positive cells in the gastric body, so that we can identify SPEM more accurately. SPEM is a type of metaplasia which associated with a risk of cancer, and its reversal may reduce that risk. A previous study confirmed the necessity of IL13 in metaplasia of chief cells (76). Using scRNA-seq, researchers found that mouse with gastritis with loss of IL4/IL13 signaling did not upregulate SPEM transcript levels. Following this treatment, SPEM was development were significantly reduced and reversed in mice with autoimmune gastritis treated with an IL13 antibody (77). It is possible to prevent and/or reverse atrophic gastritis to metaplasia through the inhibition of IL13 and its receptors. To reverse the metaplastic state and prevent the occurrence of gastric cancer is of great significance.
Clonal evolution suggests that tumor progression is also a dynamic process, and each stage has its own characteristics (78). Whether a tumor is progressing, metastasizing, or spreading to different organs, it exhibits inherent characteristics. Using scRNA-seq to analyze gastric cancer at different stages can reveal the characteristics of changes related to its development and outcome, which are critical for discovering new blocking targets and assessing prognosis. According to the findings of a study of peritoneal carcinoma (PC) in gastric adenocarcinoma patients, PC malignant cells are highly heterogeneous among patients, with most clustering by patient. Depending on the lineage status of malignant PC cells, different oncogenic pathways are involved in patient survival. Those tumor cells with gastric characteristics had shorter survival times and were predominantly enriched in oncogenic pathways, while those with enterocyte characteristics had longer survival times and were predominantly enriched in immune pathways (51). Hence, PCs with a variety of cellular characteristics may be useful in determining survival prognosis. An analysis of gastric cancer lymph node metastasis revealed the presence of ERBB2, CLDN11 and CDK12, as markers of lymph node metastasis, which may contribute to lymph node metastasis in gastric cancer (52). Aside from the peritoneum and lymph nodes, the liver, lung, and ovary are common metastatic organs for advanced gastric cancer. According to Jiang et al., a study of metastatic foci from different organs of gastric cancer found that malignant cells have invasion features, organ metastasis tendency, tumor stem cell phenotypes, and dormancy-like characteristics (53). These characteristics of malignant cells may be associated with metastatic propensity and recurrence. Together, scRNA-seq demonstrates the heterogeneity of metastases from gastric cancer.
Cells found in the circulatory system of tumor patients are known as circulating tumor cells (CTCs), which are thought to be associated with distant metastases (79). In a scRNA-seq study of gastric cancer CTCs, mesenchymal genes such as ZEB2 and SERPINE1 were highly expressed, possibly suggesting that they underwent EMT (54). Heterogeneity in gastric cancer has been further explored in these studies. In addition, tumor stem cells have also been implicated as a cause of progression, metastasis, and recurrence in gastric cancer (80). The use of scRNA-seq has provided new insights into cancer stem cells in some studies, including bladder cancer and liver tumors (81, 82). Using scRNA-seq, more evidence will be provide for cancer metastasis in gastric cancer stem cells.
In summary, scRNA-seq offers more possibilities for studying gastric cancer development and metastasis.
For patients with advanced gastric cancer, effective treatment is particularly crucial given the low survival rates. A main treatment option for advanced patients is chemotherapy, and patients respond to drugs differently due to tumor heterogeneity (83). It is possible to discover potential mechanisms that affect the drug response through scRNA-seq by detecting changes in cells before and after drug administration. This has important implications for making “cold tumors” hot tumors. A study on neoadjuvant chemotherapy found that chemotherapy could remodel the microenvironment. As compared with the pre-treatment samples, the post-treatment samples showed impaired immune cells, but increased endothelial and fibroblast cells. The T cells demonstrated lower cytotoxicity and proliferation characteristics than the pre-treatment tumors, along with downregulation of immune pathways, and the angiogenesis pathway is activated in tumor cells and endothelial cells (55). Research on advanced gastric cancer has also shown immune remodeling during chemotherapy. It was found that pro-inflammatory genes and MHC class I antigen-presenting genes decreased after chemotherapy, as well as the expression of M2-type macrophage-related genes, indicating that macrophages after chemotherapy transformed from M2 to M1 cells. LAG3 was expressed by T cells in non-responders patients, which may be associated with drug resistance (56). It was also shown that immune remodeling occurs during the early process of chemotherapy in gastric cancer. Despite the small sample size, this study provides a good foundation for predicting how individual patients will respond to chemotherapy. A greater understanding of drug resistance requires the study of larger-scale data and the advancement of molecular mechanism research.
An emerging cancer treatment strategy involves immunotherapy. Several immune checkpoint blockade treatments have been approved as third-line therapies for advanced gastric cancer due to their favorable trends in patients, including pembrolizumab, nivolumab, avelumab, durvalumab, and atezolizumab (3). In a large-scale clinical trial of PD-L1 blockade therapy in metastatic gastric cancer, EBV-positive patients showed a good clinical response to PD-L1 blockade therapy. A better response to drugs is also observed in patients with microsatellite instability-high (MSI-H) (84). Although PD-L1 blockade treatment has shown promising results in clinical trials, some patients still do not respond and develop drug resistance. The scRNA-seq approach offers a new opportunity to discover potential mechanisms of drug resistance. According to a study of patients with MSI-H treated with pembrolizumab, responders had higher levels of T cells and NK cells than non-responders. The number of T cells and NK cells decreased in non-responders after two cycles of treatment, whereas stromal cells increased. In further analysis, a further study of T cell subsets revealed a significant increase in exhausted CD8+ T cells (57). Thus, failure to mobilize the immune system is associated with the lack of response to pembrolizumab in non-responders with MSI-H gastric cancer. The discovery of how to activate the immune response of non-responders will have revolutionary significance for tumor treatment.
Clinical problems associated with drug resistance can be challenging. Testing drug sensitivity and selecting more sensitive antitumor drugs for the treatment of gastric cancer patients is of great significance in improving therapeutic benefit. Cells cultured in two dimensions have certain limitations regarding reflecting cancer heterogeneity and the microenvironment. The emergence of the single-cell patient derived organoids (PDOs) transcriptome not only compensates for the deficiencies of traditional cell models, but also provides good results for drug sensitivity tests of gastric cancer, which is a very promising tool for drug sensitivity testing (85, 86).
In conclusion, scRNA-seq may offer an opportunity to find the best treatment combinations based on their intrinsic characteristics of their drug responses. It has the potential to be used for predicting drug sensitivity and screening drugs in the future.
Gastric cancer is a malignant tumor of the digestive system whose heterogeneity has a significant impact on the clinical outcome and prognosis of patients. With single-cell RNA sequencing, we have gained a deeper understanding of the complexity and diversity of gastric cancer. It is capable of revealing cell heterogeneity, the tumor microenvironment, oncogenesis and metastasis, as well as drug response in an unparalleled manner (Figure 2). Even though scRNA-seq is powerful, it can be improved. For instance, not all samples can be processed in an optimal timeframe after being collected. The sequencing results obtained from some frozen samples revealed that the poor cell activity invariably affected the subsequent analysis. Fortunately, cell fixation and preservation methods can now be used in library construction for frozen tissues (87, 88). By using single-nucleus transcriptome sequencing (snRNA-seq), libraries can be constructed on frozen tissues and sequencing results are more consistent than scRNA-seq (89, 90). Some analytical techniques still have limitations. The method of estimating copy number variation based on genetic variation, for instance, is not entirely accurate. Single-cell DNA sequencing retains its irreplaceable advantages in determining copy number variations (48). Furthermore, the emergence of spatial transcriptomes can compensate for the loss of spatial information caused by dissociation of single cell (91).
Figure 2 Single-cell RNA sequencing reveals gastric cancer in four aspects: cell heterogeneity, tumor microenvironment, oncogenesis and metastasis, and drug response. Created with Biorender.com.
In future studies, it is anticipated that different types of gastric cancer will be investigated using scRNA-seq in terms of their molecular characteristics, carcinogenesis mechanism, immune response, and drug response. As a result, biomarkers and therapeutic targets for various types of gastric cancer will be discovered, which will facilitate more precise treatment. New biomarkers are expected to become indicators for monitoring, and the discovery of new targets offers more options for precisely treating gastric cancer. Moreover, instead of conventional sequencing analysis, scRNA-seq will probe deeper into the molecular mechanism of gastric cancer. Further molecular and cellular verification is required for results of scRNA-seq in gastric cancer. As an example, the key ligand receptor pairs uncovered through cell communication analysis, how they work, and whether they can be used to treat cancer. New perspective on the molecular mechanisms of gastric cancer can be gained through scRNA-seq. With the improvement of scRNA-seq and the universal of analysis technology, we will see scRNA-seq sequencing used more as part of research evidence, in determining molecular characteristics and lineage relationship of cell populations (92), and verifying gene expression in specific types of cells (93, 94). Additionally, multiomics combined analysis at the level of single cell will reveal the occurrence and evolution of gastric cancer through transcriptomics, genomics, proteomics, and epigenetics. It will be more feasible to support this with bioinformatics analysis techniques (95).
Finally, although scRNA-seq will take some time to become widely used in clinics for a variety of objective reasons, it is extremely promising. In addition to providing more opportunities for gastric cancer research, scRNA-seq will help solve many clinical challenges, advancing the processions of personalized medicine.
GD substantially contributed to the concept and outline of the work. GD, YC, XZ, SCL, and SL drafted revised the review. ZY and ML revised the review. All authors contributed to the article and approved the submitted version.
This work was supported by the National Natural Science Foundation of China (82101504) and Sichuan Youth Science and Technology Innovation Research Team (2021JDTD0003).
The authors declare that the research was conducted in the absence of any commercial or financial relationships that could be construed as a potential conflict of interest.
All claims expressed in this article are solely those of the authors and do not necessarily represent those of their affiliated organizations, or those of the publisher, the editors and the reviewers. Any product that may be evaluated in this article, or claim that may be made by its manufacturer, is not guaranteed or endorsed by the publisher.
1. Sung H, Ferlay J, Siegel RL, Laversanne M, Soerjomataram I, Jemal A, et al. Global cancer statistics 2020: GLOBOCAN estimates of incidence and mortality worldwide for 36 cancers in 185 countries. CA Cancer J Clin (2021) 71(3):209–49. doi: 10.3322/caac.21660
2. Thrift AP, El-Serag HB. Burden of gastric cancer. Clin Gastroenterol Hepatol (2020) 18(3):534–42. doi: 10.1016/j.cgh.2019.07.045
3. Smyth EC, Nilsson M, Grabsch HI, van Grieken NCT, Lordick F. Gastric cancer. Lancet (2020) 396(10251):635–48. doi: 10.1016/S0140-6736(20)31288-5
4. Swanton C. Intratumor heterogeneity: evolution through space and time. Cancer Res (2012) 72(19):4875–82. doi: 10.1158/0008-5472.CAN-12-2217
5. Hudler P. Challenges of deciphering gastric cancer heterogeneity. World J Gastroenterol (2015) 21(37):10510–27. doi: 10.3748/wjg.v21.i37.10510
6. Lauren P. The two histological main types of gastric carcinoma: diffuse and so-called intestinal-type carcinoma. an attempt at a histo-clinical classification. Acta Pathologica Microbiologica Scandinavica (1965) 64:31–49. doi: 10.1111/apm.1965.64.1.31
7. Bass AJ, Thorsson V, Shmulevich I, Reynolds SM, Miller M, et al. Cancer Genome Atlas Research Network, Comprehensive molecular characterization of gastric adenocarcinoma. Nature (2014) 513(7517):202–9. doi: 10.1038/nature13480
8. Cristescu R, Lee J, Nebozhyn M, Kim KM, Ting JC, Wong SS, et al. Molecular analysis of gastric cancer identifies subtypes associated with distinct clinical outcomes. Nat Med (2015) 21(5):449–56. doi: 10.1038/nm.3850
9. Yohe S, Thyagarajan B. Review of clinical next-generation sequencing. Arch Pathol Lab Med (2017) 141(11):1544–57. doi: 10.5858/arpa.2016-0501-RA
10. Baslan T, Hicks J. Unravelling biology and shifting paradigms in cancer with single-cell sequencing. Nat Rev Cancer (2017) 17(9):557–69. doi: 10.1038/nrc.2017.58
11. Guruprasad P, Lee YG, Kim KH, Ruella M. The current landscape of single-cell transcriptomics for cancer immunotherapy. J Exp Med (2021) 218(1):e20201574. doi: 10.1084/jem.20201574
12. Puram SV, Tirosh I, Parikh AS, Patel AP, Yizhak K, Gillespie S, et al. Single-cell transcriptomic analysis of primary and metastatic tumor ecosystems in head and neck cancer. Cell (2017) 171(7):1611–24.e24. doi: 10.1016/j.cell.2017.10.044
13. Chen YC, Sahoo S, Brien R, Jung S, Humphries B, Lee W, et al. Single-cell RNA-sequencing of migratory breast cancer cells: discovering genes associated with cancer metastasis. Analyst (2019) 144(24):7296–309. doi: 10.1039/C9AN01358J
14. Kim C, Gao R, Sei E, Brandt R, Hartman J, Hatschek T, et al. Chemoresistance evolution in triple-negative breast cancer delineated by single-cell sequencing. Cell (2018) 173(4):879–93.e13. doi: 10.1016/j.cell.2018.03.041
15. Kim M, Min YK, Jang J, Park H, Lee S, Lee CH. Single-cell RNA sequencing reveals distinct cellular factors for response to immunotherapy targeting CD73 and PD-1 in colorectal cancer. J Immunother Cancer (2021) 9(7):e002503. doi: 10.1136/jitc-2021-002503
16. Tirosh I, Izar B, Prakadan SM, Wadsworth MH 2nd, Treacy D, Trombetta JJ, et al. Dissecting the multicellular ecosystem of metastatic melanoma by single-cell RNA-seq. Science (2016) 352(6282):189–96. doi: 10.1126/science.aad0501
17. Guo X, Zhang Y, Zheng L, Zheng C, Song J, Zhang Q, et al. Global characterization of T cells in non-small-cell lung cancer by single-cell sequencing. Nat Med (2018) 24(7):978–85. doi: 10.1038/s41591-018-0045-3
18. Ma L, Hernandez MO, Zhao Y, Mehta M, Tran B, Kelly M, et al. Tumor cell biodiversity drives microenvironmental reprogramming in liver cancer. Cancer Cell (2019) 36(4):418–30 e6. doi: 10.1016/j.ccell.2019.08.007
19. Elyada E, Bolisetty M, Laise P, Flynn WF, Courtois ET, Burkhart RA, et al. Cross-species single-cell analysis of pancreatic ductal adenocarcinoma reveals antigen-presenting cancer-associated fibroblasts. Cancer Discov (2019) 9(8):1102–23. doi: 10.1158/2159-8290.CD-19-0094
20. Gross A, Schoendube J, Zimmermann S, Steeb M, Zengerle R, Koltay P. Technologies for single-cell isolation. Int J Mol Sci (2015) 16(8):16897–919. doi: 10.3390/ijms160816897
21. Grun D, van Oudenaarden A. Design and analysis of single-cell sequencing experiments. Cell (2015) 163(4):799–810. doi: 10.1016/j.cell.2015.10.039
22. Picelli S, Bjorklund AK, Faridani OR, Sagasser S, Winberg G, Sandberg R. Smart-seq2 for sensitive full-length transcriptome profiling in single cells. Nat Methods (2013) 10(11):1096–8. doi: 10.1038/nmeth.2639
23. Mazutis L, Gilbert J, Ung WL, Weitz DA, Griffiths AD, Heyman JA. Single-cell analysis and sorting using droplet-based microfluidics. Nat Protoc (2013) 8(5):870–91. doi: 10.1038/nprot.2013.046
24. Wang X, He Y, Zhang Q, Ren X, Zhang Z. Direct comparative analyses of 10X genomics chromium and smart-seq2. Genomics Proteomics Bioinf (2021) 19(2):253–66. doi: 10.1016/j.gpb.2020.02.005
25. Singh S, Wang L, Schaff DL, Sutcliffe MD, Koeppel AF, Kim J, et al. In situ 10-cell RNA sequencing in tissue and tumor biopsy samples. Sci Rep (2019) 9(1):4836. doi: 10.1038/s41598-019-41235-9
26. Kulkarni A, Anderson AG, Merullo DP, Konopka G. Beyond bulk: a review of single cell transcriptomics methodologies and applications. Curr Opin Biotechnol (2019) 58:129–36. doi: 10.1016/j.copbio.2019.03.001
27. Islam S, Zeisel A, Joost S, La Manno G, Zajac P, Kasper M, et al. Quantitative single-cell RNA-seq with unique molecular identifiers. Nat Methods (2014) 11(2):163–6. doi: 10.1038/nmeth.2772
28. Andrews TS, Kiselev VY, McCarthy D, Hemberg M. Tutorial: guidelines for the computational analysis of single-cell RNA sequencing data. Nat Protoc (2021) 16(1):1–9. doi: 10.1038/s41596-020-00409-w
29. Regev A, Teichmann SA, Lander ES, Amit I, Benoist C, Birney E, et al. The human cell atlas. Elife (2017) 6:e27041. doi: 10.7554/eLife.27041
30. Alquicira-Hernandez J, Sathe A, Ji HP, Nguyen Q, Powell JE. scPred: accurate supervised method for cell-type classification from single-cell RNA-seq data. Genome Biol (2019) 20(1):264. doi: 10.1186/s13059-019-1862-5
31. Luecken MD, Theis FJ. Current best practices in single-cell RNA-seq analysis: a tutorial. Mol Syst Biol (2019) 15(6):e8746. doi: 10.15252/msb.20188746
32. Hänzelmann S, Castelo R, Guinney J. GSVA: gene set variation analysis for microarray and RNA-seq data. BMC Bioinf (2013) 14:7. doi: 10.1186/1471-2105-14-7
33. Qiu X, Mao Q, Tang Y, Wang L, Chawla R, Pliner HA, et al. Reversed graph embedding resolves complex single-cell trajectories. Nat Methods (2017) 14(10):979–82. doi: 10.1038/nmeth.4402
34. Street K, Risso D, Fletcher RB, Das D, Ngai J, Yosef N, et al. Slingshot: cell lineage and pseudotime inference for single-cell transcriptomics. BMC Genomics (2018) 19(1):477. doi: 10.1186/s12864-018-4772-0
35. Kumar V, Ramnarayanan K, Sundar R, Padmanabhan N, Srivastava S, Koiwa M, et al. Single-cell atlas of lineage states, tumor microenvironment, and subtype-specific expression programs in gastric cancer. Cancer Discov (2022) 12(3):670–91. doi: 10.1158/2159-8290.CD-21-0683
36. Patel AP, Tirosh I, Trombetta JJ, Shalek AK, Gillespie SM, Wakimoto H, et al. Single-cell RNA-seq highlights intratumoral heterogeneity in primary glioblastoma. Science (2014) 344(6190):1396–401. doi: 10.1126/science.1254257
37. Gao R, Bai S, Henderson YC, Lin Y, Schalck A, Yan Y, et al. Delineating copy number and clonal substructure in human tumors from single-cell transcriptomes. Nat Biotechnol (2021) 39(5):599–608. doi: 10.1038/s41587-020-00795-2
38. Jin S, Guerrero-Juarez CF, Zhang L, Chang I, Ramos R, Kuan CH, et al. Inference and analysis of cell-cell communication using CellChat. Nat Commun (2021) 12(1):1088. doi: 10.1038/s41467-021-21246-9
39. Efremova M, Vento-Tormo M, Teichmann SA, Vento-Tormo R. CellPhoneDB: inferring cell-cell communication from combined expression of multi-subunit ligand-receptor complexes. Nat Protoc (2020) 15(4):1484–506. doi: 10.1038/s41596-020-0292-x
40. Jeong HY, Ham IH, Lee SH, Ryu D, Son SY, Han SU, et al. Spatially distinct reprogramming of the tumor microenvironment based on tumor invasion in diffuse-type gastric cancers. Clin Cancer Res (2021) 27(23):6529–42. doi: 10.1158/1078-0432.CCR-21-0792
41. Aibar S, Gonzalez-Blas CB, Moerman T, Huynh-Thu VA, Imrichova H, Hulselmans G, et al. SCENIC: single-cell regulatory network inference and clustering. Nat Methods (2017) 14(11):1083–6. doi: 10.1038/nmeth.4463
42. Fan J, Slowikowski K, Zhang F. Single-cell transcriptomics in cancer: computational challenges and opportunities. Exp Mol Med (2020) 52(9):1452–65. doi: 10.1038/s12276-020-0422-0
43. Zhang P, Yang M, Zhang Y, Xiao S, Lai X, Tan A, et al. Dissecting the single-cell transcriptome network underlying gastric premalignant lesions and early gastric cancer. Cell Rep (2019) 27(6):1934–47.e5. doi: 10.1016/j.celrep.2019.04.052
44. Zhang M, Hu S, Min M, Ni Y, Lu Z, Sun X, et al. Dissecting transcriptional heterogeneity in primary gastric adenocarcinoma by single cell RNA sequencing. Gut (2021) 70(3):464–75. doi: 10.1136/gutjnl-2019-320368
45. Kim J, Park C, Kim KH, Kim EH, Kim H, Woo JK, et al. Single-cell analysis of gastric pre-cancerous and cancer lesions reveals cell lineage diversity and intratumoral heterogeneity. NPJ Precis Oncol (2022) 6(1):9. doi: 10.1038/s41698-022-00251-1
46. Liu Z, Wang A, Pu Y, Li Z, Xue R, Zhang C, et al. Genomic and transcriptomic profiling of hepatoid adenocarcinoma of the stomach. Oncogene (2021) 40(38):5705–17. doi: 10.1038/s41388-021-01976-2
47. Dixon K, Brew T, Farnell D, Godwin TD, Cheung S, Chow C, et al. Modelling hereditary diffuse gastric cancer initiation using transgenic mouse-derived gastric organoids and single-cell sequencing. J Pathol (2021) 254(3):254–64. doi: 10.1002/path.5675
48. Andor N, Lau BT, Catalanotti C, Sathe A, Kubit M, Chen J, et al. Joint single cell DNA-seq and RNA-seq of gastric cancer cell lines reveals rules of in vitro evolution. NAR Genom Bioinform (2020) 2(2):lqaa016. doi: 10.1093/nargab/lqaa016
49. Eum HH, Kwon M, Ryu D, Jo A, Chung W, Kim N, et al. Tumor-promoting macrophages prevail in malignant ascites of advanced gastric cancer. Exp Mol Med (2020) 52(12):1976–88. doi: 10.1038/s12276-020-00538-y
50. Qu Y, Wang X, Bai S, Niu L, Zhao G, Yao Y, et al. The effects of TNF-alpha/TNFR2 in regulatory T cells on the microenvironment and progression of gastric cancer. Int J Cancer (2022) 150(8):1373–91. doi: 10.1002/ijc.33873
51. Wang R, Dang M, Harada K, Han G, Wang F, Pool Pizzi M, et al. Single-cell dissection of intratumoral heterogeneity and lineage diversity in metastatic gastric adenocarcinoma. Nat Med (2021) 27(1):141–51. doi: 10.1038/s41591-020-1125-8
52. Wang B, Zhang Y, Qing T, Xing K, Li J, Zhen T, et al. Comprehensive analysis of metastatic gastric cancer tumour cells using single-cell RNA-seq. Sci Rep (2021) 11(1):1141. doi: 10.1038/s41598-020-80881-2
53. Jiang H, Yu D, Yang P, Guo R, Kong M, Gao Y, et al. Revealing the transcriptional heterogeneity of organ-specific metastasis in human gastric cancer using single-cell RNA sequencing. Clin Transl Med (2022) 12(2):e730. doi: 10.1002/ctm2.730
54. Negishi R, Yamakawa H, Kobayashi T, Horikawa M, Shimoyama T, Koizumi F, et al. Transcriptomic profiling of single circulating tumor cells provides insight into human metastatic gastric cancer. Commun Biol (2022) 5(1):20. doi: 10.1038/s42003-021-02937-x
55. Chen Y, Yin J, Zhao L, Zhou G, Dong S, Zhang Y, et al. Reconstruction of the gastric cancer microenvironment after neoadjuvant chemotherapy by longitudinal single-cell sequencing. J Transl Med (2022) 20(1):563. doi: 10.1186/s12967-022-03792-y
56. Kim R, An M, Lee H, Mehta A, Heo YJ, Kim KM, et al. Early tumor-immune microenvironmental remodeling and response to first-line fluoropyrimidine and platinum chemotherapy in advanced gastric cancer. Cancer Discov (2022) 12(4):984–1001. doi: 10.1158/2159-8290.CD-21-0888
57. Kwon M, An M, Klempner SJ, Lee H, Kim KM, Sa JK, et al. Determinants of response and intrinsic resistance to PD-1 blockade in microsatellite instability-high gastric cancer. Cancer Discov (2021) 11(9):2168–85. doi: 10.1158/2159-8290.CD-21-0219
58. Nagaoka K, Shirai M, Taniguchi K, Hosoi A, Sun C, Kobayashi Y, et al. Deep immunophenotyping at the single-cell level identifies a combination of anti-IL-17 and checkpoint blockade as an effective treatment in a preclinical model of data-guided personalized immunotherapy. J Immunother Cancer (2020) 8(2):e001358. doi: 10.1136/jitc-2020-001358
59. Bartfeld S, Koo BK. Adult gastric stem cells and their niches. Wiley Interdiscip Rev Dev Biol (2017) 6(2). doi: 10.1002/wdev.261
60. Maman S, Witz IP. A history of exploring cancer in context. Nat Rev Cancer (2018) 18(6):359–76. doi: 10.1038/s41568-018-0006-7
61. Correa P, Piazuelo MB. The gastric precancerous cascade. J Dig Dis (2012) 13(1):2–9. doi: 10.1111/j.1751-2980.2011.00550.x
62. Jimenez Fonseca P, Carmona-Bayonas A, Hernandez R, Custodio A, Cano JM, Lacalle A, et al. Lauren Subtypes of advanced gastric cancer influence survival and response to chemotherapy: real-world data from the AGAMENON national cancer registry. Br J Cancer (2017) 117(6):775–82. doi: 10.1038/bjc.2017.245
63. Hanahan D, Weinberg RA. Hallmarks of cancer: the next generation. Cell (2011) 144(5):646–74. doi: 10.1016/j.cell.2011.02.013
64. Junttila MR, de Sauvage FJ. Influence of tumour micro-environment heterogeneity on therapeutic response. Nature (2013) 501(7467):346–54. doi: 10.1038/nature12626
65. Newman AM, Steen CB, Liu CL, Gentles AJ, Chaudhuri AA, Scherer F, et al. Determining cell type abundance and expression from bulk tissues with digital cytometry. Nat Biotechnol (2019) 37(7):773–82. doi: 10.1038/s41587-019-0114-2
66. Schumacher TN, Thommen DS. Tertiary lymphoid structures in cancer. Science (2022) 375(6576):eabf9419. doi: 10.1126/science.abf9419
67. Jia L, Wang T, Zhao Y, Zhang S, Ba T, Kuai X, et al. Single-cell profiling of infiltrating b cells and tertiary lymphoid structures in the TME of gastric adenocarcinomas. Oncoimmunology (2021) 10(1):1969767. doi: 10.1080/2162402X.2021.1969767
68. Moreno Ayala MA, Li Z, DuPage M. Treg programming and therapeutic reprogramming in cancer. Immunology (2019) 157(3):198–209. doi: 10.1111/imm.13058
69. Sathe A, Grimes SM, Lau BT, Chen J, Suarez C, Huang RJ, et al. Single-cell genomic characterization reveals the cellular reprogramming of the gastric tumor microenvironment. Clin Cancer Res (2020) 26(11):2640–53. doi: 10.1158/1078-0432.CCR-19-3231
70. Fristedt R, Borg D, Hedner C, Berntsson J, Nodin B, Eberhard J, et al. Prognostic impact of tumour-associated b cells and plasma cells in oesophageal and gastric adenocarcinoma. J Gastrointest Oncol (2016) 7(6):848–59. doi: 10.21037/jgo.2016.11.07
71. Yin H, Guo R, Zhang H, Liu S, Gong Y, Yuan Y. A dynamic transcriptome map of different tissue microenvironment cells identified during gastric cancer development using single-cell RNA sequencing. Front Immunol (2021) 12:728169. doi: 10.3389/fimmu.2021.728169
72. Meyer AR, Goldenring JR. Injury, repair, inflammation and metaplasia in the stomach. J Physiol (2018) 596(17):3861–7. doi: 10.1113/JP275512
73. Goldenring JR, Mills JC. Cellular plasticity, reprogramming, and regeneration: metaplasia in the stomach and beyond. Gastroenterology (2022) 162(2):415–30. doi: 10.1053/j.gastro.2021.10.036
74. Bockerstett KA, Lewis SA, Wolf KJ, Noto CN, Jackson NM, Ford EL, et al. Single-cell transcriptional analyses of spasmolytic polypeptide-expressing metaplasia arising from acute drug injury and chronic inflammation in the stomach. Gut (2020) 69(6):1027–38. doi: 10.1136/gutjnl-2019-318930
75. Bockerstett KA, Lewis SA, Noto CN, Ford EL, Saenz JB, Jackson NM, et al. Single-cell transcriptional analyses identify lineage-specific epithelial responses to inflammation and metaplastic development in the gastric corpus. Gastroenterology (2020) 159(6):2116–29.e4. doi: 10.1053/j.gastro.2020.08.027
76. Petersen CP, Meyer AR, De Salvo C, Choi E, Schlegel C, Petersen A, et al. A signalling cascade of IL-33 to IL-13 regulates metaplasia in the mouse stomach. Gut (2018) 67(5):805–17. doi: 10.1136/gutjnl-2016-312779
77. Noto CN, Hoft SG, Bockerstett KA, Jackson NM, Ford EL, Vest LS, et al. IL13 acts directly on gastric epithelial cells to promote metaplasia development during chronic gastritis. Cell Mol Gastroenterol Hepatol (2022) 13(2):623–42. doi: 10.1016/j.jcmgh.2021.09.012
78. Black JRM, McGranahan N. Genetic and non-genetic clonal diversity in cancer evolution. Nat Rev Cancer (2021) 21(6):379–92. doi: 10.1038/s41568-021-00336-2
79. De Rubis G, Rajeev Krishnan S, Bebawy M. Liquid biopsies in cancer diagnosis, monitoring, and prognosis. Trends Pharmacol Sci (2019) 40(3):172–86. doi: 10.1016/j.tips.2019.01.006
80. Brungs D, Aghmesheh M, Vine KL, Becker TM, Carolan MG, Ranson M. Gastric cancer stem cells: evidence, potential markers, and clinical implications. J Gastroenterol (2016) 51(4):313–26. doi: 10.1007/s00535-015-1125-5
81. Zheng H, Pomyen Y, Hernandez MO, Li C, Livak F, Tang W, et al. Single-cell analysis reveals cancer stem cell heterogeneity in hepatocellular carcinoma. Hepatol (Baltimore Md) (2018) 68(1):127–40. doi: 10.1002/hep.29778
82. Wang H, Mei Y, Luo C, Huang Q, Wang Z, Lu GM, et al. Single-cell analyses reveal mechanisms of cancer stem cell maintenance and epithelial-mesenchymal transition in recurrent bladder cancer. Clin Cancer Res (2021) 27(22):6265–78. doi: 10.1158/1078-0432.CCR-20-4796
83. Carrasco-Garcia E, García-Puga M, Arevalo S, Matheu A. Towards precision medicine: linking genetic and cellular heterogeneity in gastric cancer. Ther Adv Med Oncol (2018) 10:1758835918794628. doi: 10.1177/1758835918794628
84. Kim ST, Cristescu R, Bass AJ, Kim KM, Odegaard JI, Kim K, et al. Comprehensive molecular characterization of clinical responses to PD-1 inhibition in metastatic gastric cancer. Nat Med (2018) 24(9):1449–58. doi: 10.1038/s41591-018-0101-z
85. Gao M, Harper MM, Lin M, Qasem SA, Patel RA, Mardini SH, et al. Development of a single-cell technique to increase yield and use of gastrointestinal cancer organoids for personalized medicine application. J Am Coll Surg (2021) 232(4):504–14. doi: 10.1016/j.jamcollsurg.2020.11.009
86. Chen J, Lau BT, Andor N, Grimes SM, Handy C, Wood-Bouwens C, et al. Single-cell transcriptome analysis identifies distinct cell types and niche signaling in a primary gastric organoid model. Sci Rep (2019) 9(1):4536. doi: 10.1038/s41598-019-40809-x
87. Guillaumet-Adkins A, Rodriguez-Esteban G, Mereu E, Mendez-Lago M, Jaitin DA, Villanueva A, et al. Single-cell transcriptome conservation in cryopreserved cells and tissues. Genome Biol (2017) 18(1):45. doi: 10.1186/s13059-017-1171-9
88. Attar M, Sharma E, Li S, Bryer C, Cubitt L, Broxholme J, et al. A practical solution for preserving single cells for RNA sequencing. Sci Rep (2018) 8(1):2151. doi: 10.1038/s41598-018-20372-7
89. Wu H, Kirita Y, Donnelly EL, Humphreys BD. Advantages of single-nucleus over single-cell RNA sequencing of adult kidney: rare cell types and novel cell states revealed in fibrosis. J Am Soc Nephrol (2019) 30(1):23–32. doi: 10.1681/ASN.2018090912
90. Cavalli M, Diamanti K, Pan G, Spalinskas R, Kumar C, Deshmukh AS, et al. A multi-omics approach to liver diseases: integration of single nuclei transcriptomics with proteomics and HiCap bulk data in human liver. OMICS (2020) 24(4):180–94. doi: 10.1089/omi.2019.0215
91. Ståhl PL, Salmén F, Vickovic S, Lundmark A, Navarro JF, Magnusson J, et al. Visualization and analysis of gene expression in tissue sections by spatial transcriptomics. Science (2016) 353(6294):78–82. doi: 10.1126/science.aaf2403
92. Han S, Fink J, Jorg DJ, Lee E, Yum MK, Chatzeli L, et al. Defining the identity and dynamics of adult gastric isthmus stem cells. Cell Stem Cell (2019) 25(3):342–56 e7. doi: 10.1016/j.stem.2019.07.008
93. Yasuda T, Koiwa M, Yonemura A, Miyake K, Kariya R, Kubota S, et al. Inflammation-driven senescence-associated secretory phenotype in cancer-associated fibroblasts enhances peritoneal dissemination. Cell Rep (2021) 34(8):108779. doi: 10.1016/j.celrep.2021.108779
94. Ajani JA, Xu Y, Huo L, Wang R, Li Y, Wang Y, et al. YAP1 mediates gastric adenocarcinoma peritoneal metastases that are attenuated by YAP1 inhibition. Gut (2021) 70(1):55–66. doi: 10.1136/gutjnl-2019-319748
Keywords: single-cell RNA sequencing, gastric cancer, tumor heterogeneity, oncogenesis, individualized therapy
Citation: Deng G, Zhang X, Chen Y, Liang S, Liu S, Yu Z and Lü M (2023) Single-cell transcriptome sequencing reveals heterogeneity of gastric cancer: progress and prospects. Front. Oncol. 13:1074268. doi: 10.3389/fonc.2023.1074268
Received: 19 October 2022; Accepted: 11 May 2023;
Published: 25 May 2023.
Edited by:
Kui Zhang, The University of Chicago, United StatesReviewed by:
Keisuke Sekine, National Cancer Center Research Institute, JapanCopyright © 2023 Deng, Zhang, Chen, Liang, Liu, Yu and Lü. This is an open-access article distributed under the terms of the Creative Commons Attribution License (CC BY). The use, distribution or reproduction in other forums is permitted, provided the original author(s) and the copyright owner(s) are credited and that the original publication in this journal is cited, in accordance with accepted academic practice. No use, distribution or reproduction is permitted which does not comply with these terms.
*Correspondence: Muhan Lü, bHZtdWhhbkBzd211LmVkdS5jbg==; Zehui Yu, eXV6ZWh1aV9zd211QG91dGxvb2suY29t
Disclaimer: All claims expressed in this article are solely those of the authors and do not necessarily represent those of their affiliated organizations, or those of the publisher, the editors and the reviewers. Any product that may be evaluated in this article or claim that may be made by its manufacturer is not guaranteed or endorsed by the publisher.
Research integrity at Frontiers
Learn more about the work of our research integrity team to safeguard the quality of each article we publish.