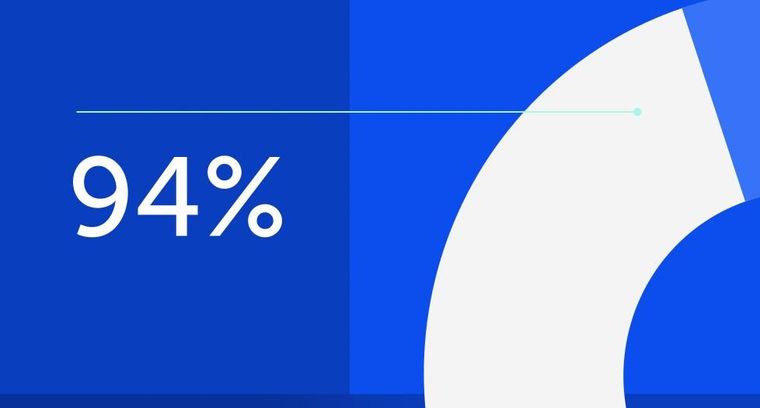
94% of researchers rate our articles as excellent or good
Learn more about the work of our research integrity team to safeguard the quality of each article we publish.
Find out more
PERSPECTIVE article
Front. Oncol., 23 June 2023
Sec. Surgical Oncology
Volume 13 - 2023 | https://doi.org/10.3389/fonc.2023.1063937
This article is part of the Research TopicInvestigations into the Potential Benefits of Artificial Intelligence and Deep Learning to Surgical OncologistsView all 10 articles
Glioblastoma a deadly brain cancer that is nearly universally fatal. Accurate prognostication and the successful application of emerging precision medicine in glioblastoma relies upon the resolution and exactitude of classification. We discuss limitations of our current classification systems and their inability to capture the full heterogeneity of the disease. We review the various layers of data that are available to substratify glioblastoma and we discuss how artificial intelligence and machine learning tools provide the opportunity to organize and integrate this data in a nuanced way. In doing so there is the potential to generate clinically relevant disease sub-stratifications, which could help predict neuro-oncological patient outcomes with greater certainty. We discuss limitations of this approach and how these might be overcome. The development of a comprehensive unified classification of glioblastoma would be a major advance in the field. This will require the fusion of advances in understanding glioblastoma biology with technological innovation in data processing and organization.
Gliomas represent the most common primary brain cancer. They have distinct biological features and clinical behavior, and account for nearly 80% of the malignant brain tumors in adults (1, 2). The commonest subtype of glioma is glioblastoma a deadly brain cancer that is nearly universally fatal. Understanding of natural history, accurate prognostication, therapeutic efficacy, and the successful application of emerging precision medicine in glioblastoma relies upon the resolution and exactitude of classification. The WHO classification of Central Nervous System tumors began in 1970 (3). The first edition was largely based on anatomical and histological findings. Many of the major shifts in neuro-oncology and glioblastoma understanding over the intervening years have been represented in the subsequent WHO classification updates and the associated cIMPACT-NOW statements (4). A major conceptual leap was made in 2012 with the recognition of key subclassification of glioblastoma based on IDH mutation status (10.1038/nature10860.). This single mutation cleaved glioblastoma into two major subtypes with differing etiology, therapeutic vulnerability, and prognosis. In 2021 the significance of this stratification became codified by separating glioblastoma (IDH wild type) fully from grade 4 diffuse astrocytoma with IDH mutation (5).
In addition to the formal WHO classification there have been a multitude of differing stratifications of glioblastoma categorizations based on transcriptional profiles. A major development was in 2006 when Phillips et al. published a transcriptional classification of high-grade glioblastoma (6). This was advanced in 2010 when Verhaak et al. used data derived from The Cancer Genome Atlas to sub-stratify into 4 subgroups; proneural, mesenchymal, classical, and neural (7, 8). These were reported to have differing prognosis and treatment vulnerabilities. Further modifications and refinements to transcriptional groups, including single-cell profiling of both the tumor cells and microenvironmental components such as the neuro-immune niche have since been proposed by several groups including Neftel et al (9) and Richards et al (10). Another layer of complexity was added by epigenetic DNA-methylation profiling. This has already had significant clinical impact in supporting diagnosis and risk stratification (11, 12). Single-cell level profiling is not limited to transcriptional RNA profiling, it can also be applied across a range of biological analytic technologies including proteomic analysis - this opens up unparalleled levels of biological data.
Technologies in spatial-omics enable a greater understanding of cellular organizations and interactions within a tissue of interest. This is particularly of interest in cancer biology and can be applied at microscopic and super-resolution levels across the full range of spectral wavelengths and including spectroscopy data (13–16).
However, histology, selective mutations, transcriptional profiles and epigenetic changes do not tell the full story of glioblastoma diversity. One of the major barriers to successful new therapies in glioblastoma is considered the intra- and inter- heterogeneity of the tumors and this extends beyond these molecular sources of variability. In addition to transcriptional and epigenetic variability, anatomical location and structural features – including presence of cysts, degree of necrosis, proliferation indices etc (17), variability in radiomic findings (18), whole genome genetic/mutational characteristics (potentially including variability in extra-chromosomal sites) (19, 20) metabolic and lipidomic (21) and proteomics (22) can all be used to codify glioblastoma.
Integrating all these variables into a unified classification which reflects the diversity of glioblastoma states and in a clinically relevant manner, represents a daunting task (see Figure 1). Without this nuanced lamination we continue to risk masking the efficacy of new therapies by disease heterogeneity leading to variability of response. Likewise, our ability to accurately provide disease prognostication will remain limited.
Figure 1 Example of multisource data input and integration for deep learning guided classification of glioblastoma.
Artificial intelligence (AI) tools provide the opportunity to organize and integrate these factors to generate clinically relevant disease sub-stratifications, which would help predict neuro-oncological patient outcomes with greater certainty. With enough data, DL (deep learning) methods based on neural networks have emerged as a leading approach for capturing highly informative features in oncology datasets. Using these tools, rapid progress has been made in each of the modalities described above. However, unanswered questions remain about how multimodal data can be integrated and a unified classification model be built.
A key requirement of multimodal integration is that each data source complements the others, enhancing information content beyond the scope of any single modality. For example, radiological data on macroscopic tumor morphology, as well as molecular and histological data, describe disease from different perspectives and scales. Each data source in a unified model should be at least partially orthogonal to the next.
While multimodal patient stratification methods have been developed for cancer patients in general, these mainly rely on multi-omic (multi-dimensional genomic data) in the absence of radiological or clinical information (23), and there currently exist few examples which utilize multimodal strategies for glioma patients specifically. Among these, there have been single-center studies which stratify glioma patients using multiparametric MRI, molecular and transcriptome information using kernel based learning (24), and deep learning approaches to predict survival which integrate both histological and genomic (but not radiological) information based on gliomas from The Cancer Genome Atlas (25, 26). These studies suggest that multimodal integration improves patient stratification and outcome prediction over unimodal methods.
Given its purported success, what is limiting this type of work? Many major limitations are simply related to the lack of availability of large, annotated datasets with multimodal information streams, which are sufficiently rich and class-balanced that the breadth of glioma heterogeneity can be encompassed. Other limitations pertain to how individual data modalities should be fused. It is unclear whether raw data should be concatenated from the start and used to train a single model. Or, alternatively, a composite model should be built from learned features, that are each derived from multiple single modality models (27).
In this regard, novel dimension-reduction and clustering methods (28), alongside other techniques which appropriately weigh will help in leveraging the vast amount of collected multimodal parameters for each patient and help prevent overfitting (29, 30). Finally, interpretation of deep learning models is notoriously difficult, and if clinicians want to understand how a unified model relates to the disease process, methods to make such models explainable are urgently needed.
Only by developing a comprehensive unified classification of glioblastoma can we optimize our prognostication and maximize the chance of precision therapies being successful. A system that allows integration of ever-increasing complexity and nuance will allow flexibility and adaption to new discoveries and therapies. Given the multiple layers of data involved in glioblastoma biology and their deep complexity and inter-related influence the consolidation and organization into a utilizable structure will require novel approaches. The application of artificial intelligence and deep machine learning in oncology is expanding at an explosive rate with numerous potential applications (31, 32). These technologies will be instrumental in achieving this final goal of a single unified classification of glioblastoma heterogeneity.
The original contributions presented in the study are included in the article/supplementary material. Further inquiries can be directed to the corresponding author.
CH conceived the manuscript. CH and AP co-wrote and reviewed the manuscript. All authors contributed to the article and approved the submitted version.
CH is supported by a CRUK Pioneer Award, an AMS Starter Grant, a National Brain Appeal Innovation Award, and BRC (UCL/NIHR) funding.
The authors declare that the research was conducted in the absence of any commercial or financial relationships that could be construed as a potential conflict of interest.
All claims expressed in this article are solely those of the authors and do not necessarily represent those of their affiliated organizations, or those of the publisher, the editors and the reviewers. Any product that may be evaluated in this article, or claim that may be made by its manufacturer, is not guaranteed or endorsed by the publisher.
1. Ostrom QT, Gittleman H, Truitt G, Boscia A, Kruchko C, Barnholtz-Sloan JS. CBTRUS statistical report: primary brain and other central nervous system tumors diagnosed in the united states in 2011–2015. Neuro-oncology (2018) 20:iv1–iv86. doi: 10.1093/neuonc/noy131
2. Weller M, Wick W, Aldape K, Brada M, Berger M, Pfister SM, et al. Glioma. Nat Rev Dis Primers (2015) 1:15017. doi: 10.1038/nrdp.2015.17
3. Scheithauer BW. Development of the WHO classification of tumors of the central nervous system: a historical perspective. Brain Pathol (2009) 19:551–64. doi: 10.1111/j.1750-3639.2008.00192.x
4. Gonzalez Castro LN, Wesseling P. The cIMPACT-NOW updates and their significance to current neuro-oncology practice. Neuro-Oncol Practice (2021) 8:4–10. doi: 10.1093/nop/npaa055
5. Komori T. The 2021 WHO classification of tumors, central nervous system tumors: the 10 basic principles. Brain Tumor Pathol (2022) 39(2), 47–50. doi: 10.1007/s10014-022-00428-3
6. Phillips HS, Kharbanda S, Chen R, Forrest WF, Soriano RH, Wu TD, et al. Molecular subclasses of high-grade glioma predict prognosis, delineate a pattern of disease progression, and resemble stages in neurogenesis. Cancer Cell (2006) 9(3):157–73. doi: 10.1016/j.ccr.2006.02.019
7. Verhaak RGW, Hoadley KA, Purdom E, Wang V, Qi Y, Wilkerson MD, et al. Integrated genomic analysis identifies clinically relevant subtypes of glioblastoma characterized by abnormalities in PDGFRA, IDH1, EGFR, and NF1. Cancer Cell (2010) 17(1):98–110. doi: 10.1016/j.ccr.2009.12.020
8. Sidaway P. Glioblastoma subtypes revisited. Nat Rev Clin Oncol (2017) 14:587–7. doi: 10.1038/nrclinonc.2017.122
9. Neftel C, Laffy J, Filbin MG, Hara T, Shore ME, Rahme GJ, et al. An integrative model of cellular states, plasticity, and genetics for glioblastoma. Cell (2019) 178(4):835–849.e21. doi: 10.1016/j.cell.2019.06.024
10. Richards LM, Whitley OKN, MacLeod G, Cavalli FMG, Coutinho FJ, Jaramillo JE, et al. Gradient of developmental and injury response transcriptional states defines functional vulnerabilities underpinning glioblastoma heterogeneity. Nat Cancer (2021) 2(2):157–73. doi: 10.1038/s43018-020-00154-9
11. Jaunmuktane Z, Capper D, Jones DTW, Schrimpf D, Sill M, Dutt M, et al. Methylation array profiling of adult brain tumours: diagnostic outcomes in a large, single centre. Acta Neuropathol Commun (2019) 7(1):24. doi: 10.1186/s40478-019-0668-8
12. Klughammer J, Kiesel B, Roetzer T, Fortelny N, Nemc A, Nenning KH, et al. The DNA methylation landscape of glioblastoma disease progression shows extensive heterogeneity in time and space. Nat Med (2018) 24(10):1611–24. doi: 10.1038/s41591-018-0156-x
13. Dey N, Li S, Bermond K, Heintzman R, Curcio CA, Ach T 3, et al. Multi-modal image fusion for multispectral super-resolution in microscopy. Proc SPIE Int Soc Opt Eng (2019) 10949:109490D. doi: 10.1117/12.2512598
14. Manni F, van der Sommen F, Fabelo H, Zinger S, Shan C, Edström E, et al. Hyperspectral imaging for glioblastoma surgery: improving tumor identification using a deep spectral-spatial approach. Sensors (Basel) (2020) 20(23):6955. doi: 10.3390/s20236955
15. Lam KHB, Leon AJ, Hui W, Lee SC, Batruch I, Faust K, et al. Topographic mapping of the glioblastoma proteome reveals a triple-axis model of intra-tumoral heterogeneity. Nat Commun (2022) 13(1):116. doi: 10.1038/s41467-021-27667-w
16. Li X, Strasser B, Neuberger U, Vollmuth P, Bendszus M, Wick W, et al. Deep learning super-resolution magnetic resonance spectroscopic imaging of brain metabolism and mutant isocitrate dehydrogenase glioma. Neuro-Oncol Adv (2022) 4(1):vdac071. doi: 10.1093/noajnl/vdac071
17. Latini F, Fahlström M, Berntsson SG, Larsson E-M, Smits A, Ryttlefors M. A novel radiological classification system for cerebral gliomas: the brain-grid. PloS One (2019) 14:e0211243. doi: 10.1371/journal.pone.0211243
18. Cho H-h, Lee S-h, Kim J, Park H. Classification of the glioma grading using radiomics analysis. PeerJ (2018) 6:e5982. doi: 10.7717/peerj.5982
19. Melin BS, Barnholtz-Sloan JS, Wrensch MR, Johansen C, Il'yasova D, Kinnersley B, et al. Genome-wide association study of glioma subtypes identifies specific differences in genetic susceptibility to glioblastoma and non-glioblastoma tumors. Nat Genet (2017) 49(5):789–94. doi: 10.1038/ng.3823
20. Karami Fath M, Karimfar N, Fazlollahpour Naghibi A, Shafa S, Ghasemi Shiran M, Ataei M, et al. Revisiting characteristics of oncogenic extrachromosomal DNA as mobile enhancers on neuroblastoma and glioma cancers. Cancer Cell Int (2022) 22(1):200. doi: 10.1186/s12935-022-02617-8
21. Garofano L, Migliozzi S, Oh YT, D'Angelo F, Najac RD, Ko A, et al. Pathway-based classification of glioblastoma uncovers a mitochondrial subtype with therapeutic vulnerabilities. Nat Cancer (2021) 2(2):141–56. doi: 10.1038/s43018-020-00159-4
22. Yanovich-Arad G, Ofek P, Yeini E, et al. Proteogenomics of glioblastoma associates molecular patterns with survival. Cell Rep (2021) 34:108787. doi: 10.1016/j.celrep.2021.108787
23. Ramazzotti D, Lal A, Wang B, Batzoglou S, Sidow A. Multi-omic tumor data reveal diversity of molecular mechanisms that correlate with survival. Nat Commun (2018) 9(1):4453. doi: 10.1038/s41467-018-06921-8
24. Rathore S, Akbari H, Rozycki M, Abdullah KG, Nasrallah MP, Binder ZA, et al. Radiomic MRI signature reveals three distinct subtypes of glioblastoma with different clinical and molecular characteristics, offering prognostic value beyond IDH1. Sci Rep (2018) 8(1):5087. doi: 10.1038/s41598-018-22739-2
25. Mobadersany P, Yousefi S, Amgad M, Gutman DA, Barnholtz-Sloan JS, Velázquez Vega JE, et al. Predicting cancer outcomes from histology and genomics using convolutional networks. Proc Natl Acad Sci USA (2018) 115(13):E2970–9. doi: 10.1073/pnas.1717139115
26. Chen RJ, Lu MY, Wang J, Williamson DFK, Rodig SJ, Lindeman NI, et al. Pathomic fusion: an integrated framework for fusing histopathology and genomic features for cancer diagnosis and prognosis. IEEE Trans Med Imag (2022) 41(4):757–70. doi: 10.1109/TMI.2020.3021387
27. Boehm KM, Khosravi P, Vanguri R, Gao J, Shah SP. Harnessing multimodal data integration to advance precision oncology. Nat Rev Cancer (2022) 22:114–26. doi: 10.1038/s41568-021-00408-3
28. Cheerla A, Gevaert O. Deep learning with multimodal representation for pancancer prognosis prediction. Bioinformatics (2019) 35:i446–54. doi: 10.1093/bioinformatics/btz342
29. Liang C, Li Y, Luo J. A novel method to detect functional microRNA regulatory modules by bicliques merging. IEEE/ACM Trans Comput Biol Bioinform (2016) 13:549–56. doi: 10.1109/TCBB.2015.2462370
30. Mao XG, Xue XY, Wang L, Lin W, Zhang X. Deep learning identified glioblastoma subtypes based on internal genomic expression ranks. BMC Cancer (2022) 22:86. doi: 10.1186/s12885-022-09191-2
31. Tran KA, Kondrashova O, Bradley A, Williams ED, Pearson JV, Waddell N. Deep learning in cancer diagnosis, prognosis and treatment selection. Genome Med (2021) 13:152. doi: 10.1186/s13073-021-00968-x
Keywords: glioma, glioblastoma, classification, artificial intelligence, machine learning
Citation: Hill CS and Pandit AS (2023) Moving towards a unified classification of glioblastomas utilizing artificial intelligence and deep machine learning integration. Front. Oncol. 13:1063937. doi: 10.3389/fonc.2023.1063937
Received: 07 October 2022; Accepted: 24 April 2023;
Published: 23 June 2023.
Edited by:
Marco Scarpa, University Hospital of Padua, ItalyReviewed by:
Riki Kawaguchi, University of California, Los Angeles, United StatesCopyright © 2023 Hill and Pandit. This is an open-access article distributed under the terms of the Creative Commons Attribution License (CC BY). The use, distribution or reproduction in other forums is permitted, provided the original author(s) and the copyright owner(s) are credited and that the original publication in this journal is cited, in accordance with accepted academic practice. No use, distribution or reproduction is permitted which does not comply with these terms.
*Correspondence: Ciaran Scott Hill, Y2lhcmFuLmhpbGxAdWNsLmFjLnVr
Disclaimer: All claims expressed in this article are solely those of the authors and do not necessarily represent those of their affiliated organizations, or those of the publisher, the editors and the reviewers. Any product that may be evaluated in this article or claim that may be made by its manufacturer is not guaranteed or endorsed by the publisher.
Research integrity at Frontiers
Learn more about the work of our research integrity team to safeguard the quality of each article we publish.