- 1Department of Ultrasound in Medicine, Zhejiang University School of Medicine Second Affiliated Hospital, Zhejiang University, Hangzhou, China
- 2Research Center of Ultrasound in Medicine and Biomedical Engineering, Zhejiang University School of Medicine Second Affiliated Hospital, Zhejiang University, Hangzhou, China
- 3Research Center for Life Science and Human Health, Binjiang Institute of Zhejiang University, Hangzhou, China
Background: Conventional ultrasound (CUS) is the first choice for discrimination benign and malignant lymphadenectasis in supraclavicular lymph nodes (SCLNs), which is important for the further treatment. Radiomics provide more comprehensive and richer information than radiographic images, which are imperceptible to human eyes.
Objective: This study aimed to explore the clinical value of CUS-based radiomics analysis in preoperative differentiation of malignant from benign lymphadenectasis in CUS suspected SCLNs.
Methods: The characteristics of CUS images of 189 SCLNs were retrospectively analyzed, including 139 pathologically confirmed benign SCLNs and 50 malignant SCLNs. The data were randomly divided (7:3) into a training set (n=131) and a validation set (n=58). A total of 744 radiomics features were extracted from CUS images, radiomics score (Rad-score) built were using least absolute shrinkage and selection operator (LASSO) logistic regression. Rad-score model, CUS model, radiomics-CUS (Rad-score + CUS) model, clinic-radiomics (Clin + Rad-score) model, and combined CUS-clinic-radiomics (Clin + CUS + Rad-score) model were built using logistic regression. Diagnostic accuracy was assessed by receiver operating characteristic (ROC) curve analysis.
Results: A total of 20 radiomics features were selected from 744 radiomics features and calculated to construct Rad-score. The AUCs of Rad-score model, CUS model, Clin + Rad-score model, Rad-score + CUS model, and Clin + CUS + Rad-score model were 0.80, 0.72, 0.85, 0.83, 0.86 in the training set and 0.77, 0.80, 0.82, 0.81, 0.85 in the validation set. There was no statistical significance among the AUC of all models in the training and validation set. The calibration curve also indicated the good predictive performance of the proposed nomogram.
Conclusions: The Rad-score model, derived from supraclavicular ultrasound images, showed good predictive effect in differentiating benign from malignant lesions in patients with suspected supraclavicular lymphadenectasis.
1 Introduction
Supraclavicular lymphadenectasis has been frequently observed in patients with benign diseases such as reactive hyperplasia, tuberculosis, granulomatous inflammation, etc., and malignant diseases such as lung cancer metastasis, breast cancer metastasis, esophageal cancer metastasis, etc (1). Thus, it is important to distinguish between benign and malignant supraclavicular lymph nodes (SCLNs) for the further treatment of patients (2, 3). Computerized tomography (CT) examination is based on the density of lymph nodes and surrounding soft tissue to distinguish, the enlarged supraclavicular lymph nodes are often indistinguishable from the surrounding tissues and muscles on plain CT scan (4). Due to the fixed and superficial location of the supraclavicular region and clear supraclavicular anatomical structure, high frequency conventional ultrasound (CUS) has its unique advantages for SCLNs examination. CUS is preferred as the first choice for SCLNs with high resolution, low cost and no radiation. However, there is overlap between benign and malignant images for atypical CUS features.
Radiomics, a process of converting radiographic images into quantifiable information, can provide more comprehensive and richer information than radiographic images, which are imperceptible to human eyes (5, 6). Imaging examination is one of the routine steps in routine clinical diagnosis, so radiomics research based on images has certain feasibility. Some studies showed that radiomics analysis on CUS images can effectively predict malignant parotid gland lesions (7). Some studies have shown that image feature-based radiomics extraction has objective characteristics and great value in predicting central lymph node metastasis in papillary thyroid carcinoma patients with Hashimoto’s thyroiditis (8). However, the clinical value of CUS-based radiomics analysis to differentiate of benign and malignant SCLNs was unknown.
Therefore, the purpose of this investigation was to extract the radiomics parameters from supraclavicular CUS images and to establish predictive a nomogram radiomics score (Rad-score) model to noninvasively identify benign and malignant SCLNs.
2 Materials and methods
2.1 Patients
Between January 2021 and July 2022, a total of 189 patients participated in the retrospective study, including pathologically confirmed 50 benign lesions and 139 malignant lesions. The inclusion criteria were as follows: (1) patients with CUS suspected SCLNs; (2) patients who underwent pathological examination within 2 weeks after a SCLNs CUS examination; (3) patients who had high-quality ultrasound image; (4) patients who had definite pathological findings. The exclusion criteria were as follows: (1) patients who underwent previous SCLN treatment (resection biopsy, radiotherapy, chemotherapy); (2) patients who had poor ultrasound image quality; (3) patients who had incomplete clinical data. (4) patients who missed histopathological results. (5) lesion larger than 5 cm in diameter due to the limited width of the US probe.
2.2 Ultrasound examination and pathological examination
The CUS examination was performed using GE LOGIQ E9, Esaote Mylab 90, Toshiba Aplio 500, Mindray Resona 7, and Philips iU22 with corresponding high-frequency linear array probes. Each patient was placed in the supine position while lying on the examination bed. Then, the neck was fully extended, and the patient was told to breathe calmly. The SCLNs were examined by 3-year experience radiologist in superficial CUS. If there were multiple lesions, then the most suspicious would be first recommended for further pathological examination. If the most suspicious one is not suitable for biopsy, the largest one (short diameter) would be second recommended for further pathological examination. The boundary (clear and unclear), shape (long/short diameter < 2, long/short diameter ≥ 2), calcification (with calcification, without calcification), hilus (present or absent), margin (well-defined and ill-defined), structure (cystic or hyperechoic nodule, no cystic and hyperechoic nodule) of the SCLNs were observed and recorded. The most suspected supraclavicular lymphadenectasis were included in the study. CUS suspected SCLN was submitted to ultrasound guided biopsy at ultrasound department. The pathological section was read by a pathologist with more than 5 years of experience. For the pathological benign SCLN, we followed up at least for 6 months. No benign SCLN had progression on ultrasound image.
2.3 Region of interest (ROI) segmentation and feature extraction
Firstly, the patients were randomly divided into the training and validation groups in a 7:3 ratio. The jpg format ultrasound images were exported from the imaging system and imported into PyCharm software (Community edition 2022.2). All images were resampled and normalized before feature extraction (7). Then, the ROI was manually segmented by an experienced radiologist and confirmed by another one by using Labelme (3.16.7) software package. Both were blinded to the pathological results before performing image annotation, and consensus was reached by discussion in cases of disagreement. Finally, the radiomics data was extracted by python package pyradiomics (V3.0.1) (https://pyradiomics.readthedocs.io/en/latest/features.html) and 744 radiomics features from SCLNs were extracted in this study.
2.4 Radiomics feature selection
Radiomics features were extracted from lesions after image processing with different filters by using the open-source Pyradiomics package V3.0.1 and were divided into the following classes: (a) first-order statistics; (b) shape-based features; (c) high-order features, including gray-level co-occurrence matrix (GLCM), gray-level size zone matrix (GLSZM), gray-level run length matrix (GLRLM), gray-level dependence matrix (GLDM), and neighboring gray tone difference matrix (NGTDM). Firstly, independent t-test was used to select significant features with statistically significant difference (P < 0.05). Then, the least absolute shrinkage and selection operator (LASSO) was used to select nonzero coefficients by 10-fold cross validation. Finally, Rad-score was calculated based on the selected features.
2.5 Models construction
The clinical and CUS data were analyzed by multivariate analysis firstly to select the statistically significant predictors of distinguishing between benign and malignant lesions and follow by logistic regression analysis. The Rad-score model, CUS model, radiomics-CUS (Rad-score + CUS) model, clinic-radiomics (Clin + Rad-score) model, and combined CUS-clinic-radiomics (Clin + CUS + Rad-score) model were established both in training and validation sets. The calibration curve was used to evaluate the calibration ability. The prediction accuracy of the models was represented by the receiver operating characteristic (ROC) curve and was quantified by the area under the ROC curve (AUC) in both the training and test sets.
2.6 Statistical analysis
Statistical analyzes were performed using statistical software for Windows version 23.0 (SPSS Inc., Chicago, IL, USA) and R software version 4.2.1 (R project for statistical computing). Quantitative data with abnormal distribution was expressed as median (interquartile range 25th, 75th percentile). Quantitative data with normal distribution was expressed as mean ± standard deviation. Wilcox test was conducted to compare the data displaying an abnormal distribution. Statistical analysis was by chi-squared test when comparing categorical variables. The LASSO method constructed a penalty function by adding constraint conditions, and a prediction model was constructed by performing a 10-fold cross-validation. The models were built using logistic regression and the diagnostic performance for differentiating between benign and malignant lesions was using ROC. DeLong’s test was used to evaluate different ROC curves. Calibration curves were constructed to assess the predictive value of different models. A P value of less than 0.05 was considered statistically significant.
3 Results
3.1 Basic information of patients with SCLNs lymphadenectasis
A total of 189 patients were recruited (Figures 1, 2), including 50 pathologically confirmed benign (16 males and 34 females; age 59.5 (44.5-67.75) years) and 139 malignant (73 males and 66 females; age 65 (53.5-70) years). The specific pathological findings of 50 benign and 139 malignant SCLNs lymphadenectasis was showing (Supplemental Table 1). The median follow-up after biopsy for benign SCLNs lymphadenectasis was 10 (range, 6-20) months. No benign SCLN had progression on ultrasound image. The SCLNs malignant rates of the training set and the validation set were 70.23% and 81.03%, respectively. There were no significant differences in patient age, sex, location, pathology, diameter, tumor history, boundary, margin, calcification, shape, hilus, structure, and rad-score between the training set and the validation set (P>0.05), as shown in Table 1.
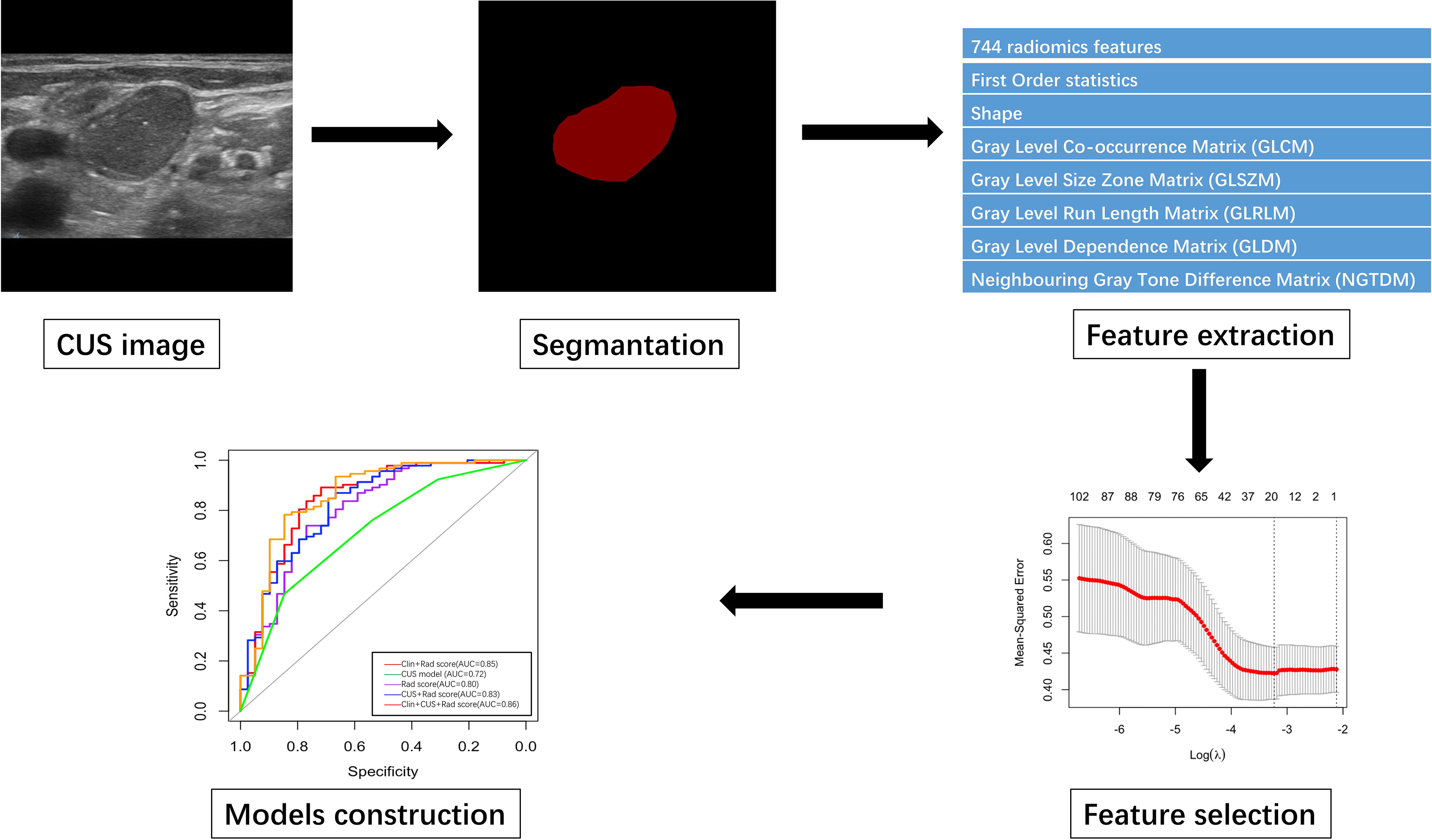
Figure 2 The flowchart of CUS segmentation, feature extraction, feature selection and models construction.
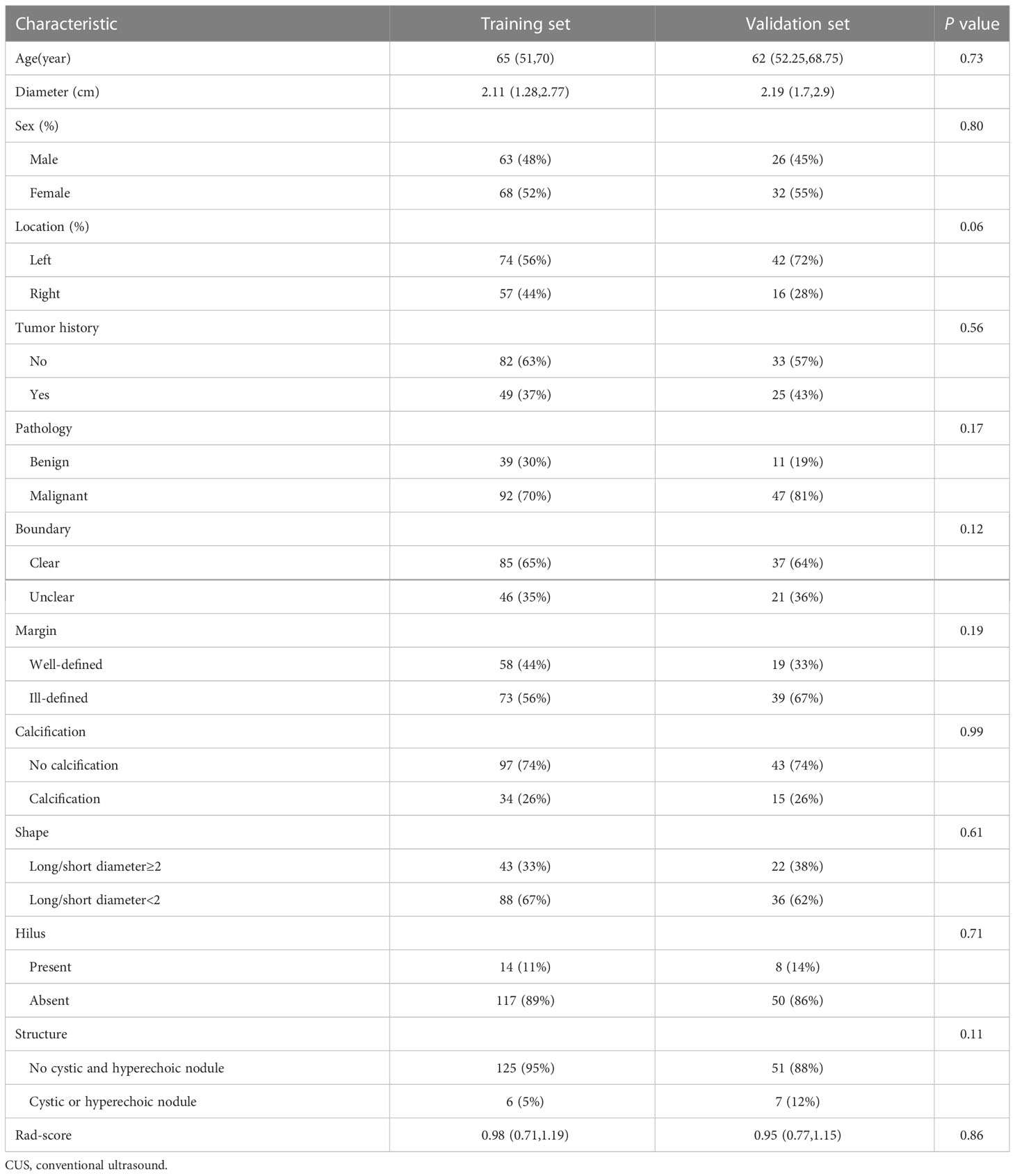
Table 1 Clinic-CUS characteristics of suspected supraclavicular lymphadenectasis between training and validation set.
3.2 Univariate analysis of clinical features, CUS features, and Rad-score for malignant lesions
Benign lesions were more likely to be found with no tumor history (P<0.05, Table 2) in the training set. The validation set also found the same findings. For CUS features, well-defined margin, clear boundary, and long/short diameter≥2 shape were commonly observed in benign lesions (all P<0.05) in the training set. The same results were also found in the validation set.
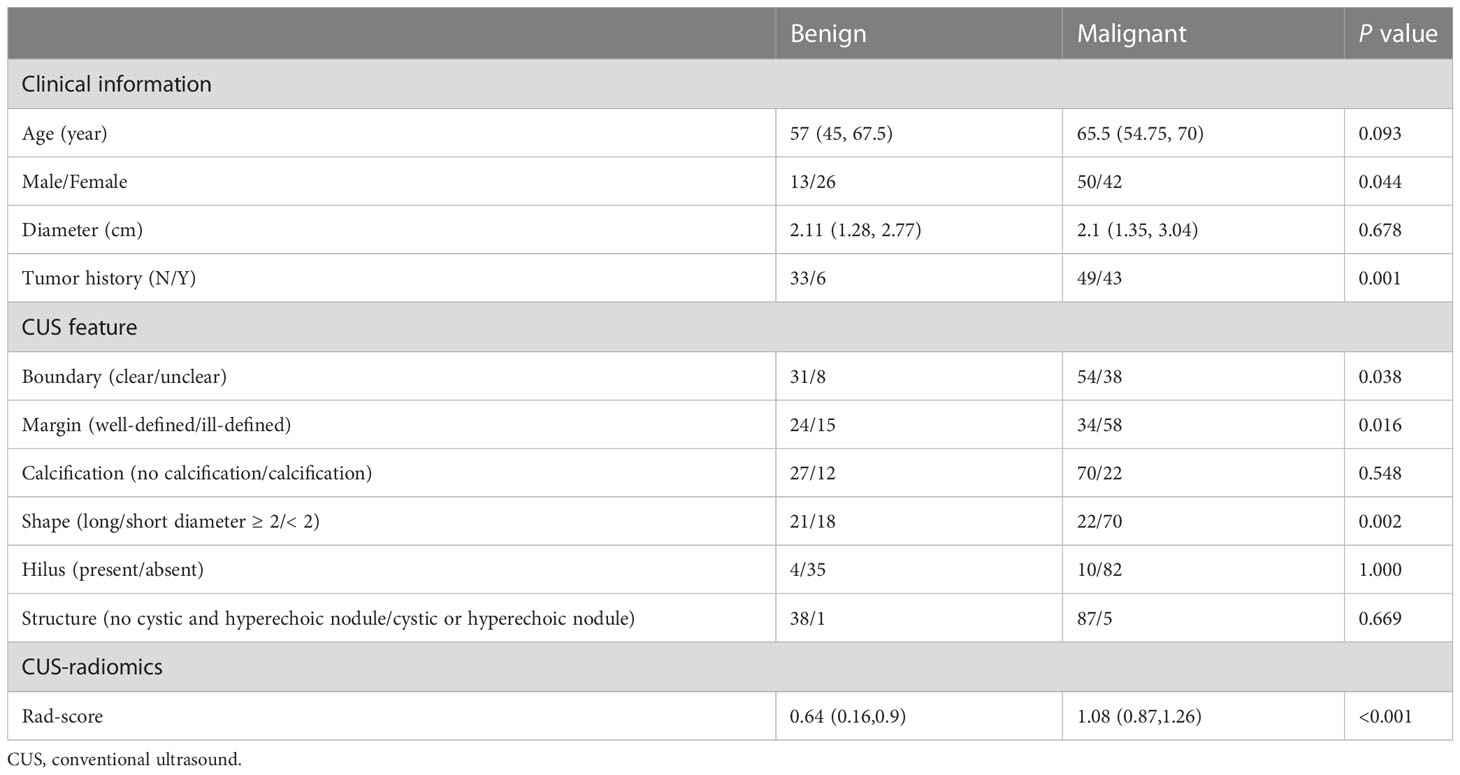
Table 2 Univariate analysis of clinical information, CUS features, and Rad-score for distinguishing benign from malignant lesions in the training set.
Seven hundred and forty-four radiomic features were extracted from each ROI, and a total of 20 radiomic features (Supplemental Table 2) with non-zero coefficients were screened out based on t test and LASSO (Figure 3). The Rad-score was calculated between benign and malignant lesions (Supplemental Table 3). The results showed that patients with benign lesions had lower Rad-score than patients with malignant lesions, which was statistically significant in both training (0.64(0.16, 0.9) vs. 1.08(0.87, 1.26), P < 0.05) and validation sets (0.63(0.13, 0.89) vs. 0.98(0.81, 1.24), P < 0.05).
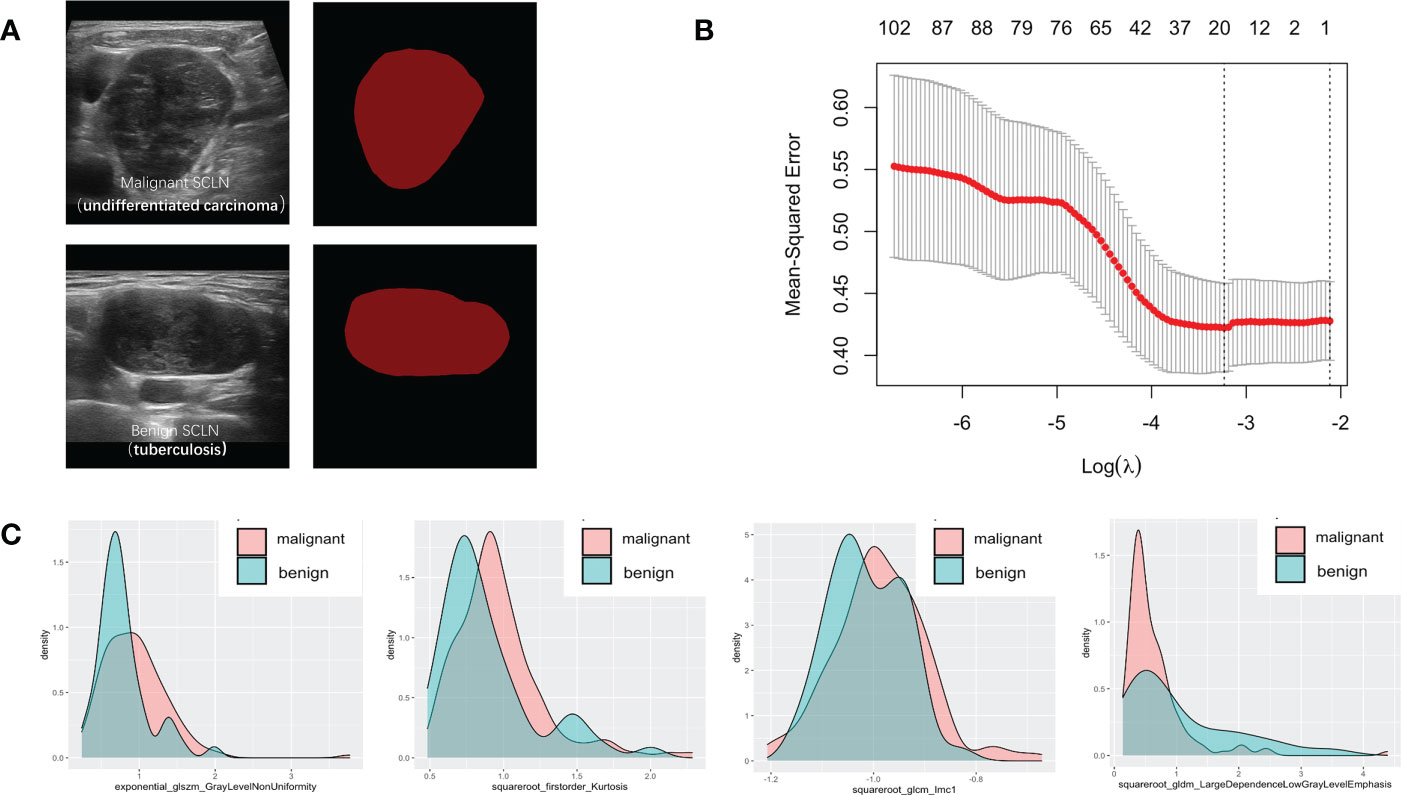
Figure 3 Variables extracted from benign and malignant supraclavicular lymph node. (A) The ROI of benign and malignant supraclavicular lymph node. (B) The Mean-square error plot of LASSO regression in supraclavicular lymph node. (C) The density plots between extracted radiomics variables in benign and malignant supraclavicular lymph node.
3.3 Multivariate analysis of variables for predicting malignant lesions
In the multivariate analysis of clinical information, CUS features and Rad-score characteristics, tumor history (OR=4.88, 95%CI 1.65-17.24), long/short diameter < 2 shape (OR=2.69, 95%CI 1.04-7.14), Rad-score (OR=15.57, 95%CI 5.20-64.49) were significantly correlated with pathological results (P<0.05). However, sex, boundary, and the margin were not independent signatures for predicting malignant lesions (Supplemental Table 4).
3.4 Performance, construction and validation of nomogram
The Rad-score model, Clin + Rad-score model, Rad-score + CUS model, and Clin + CUS + Rad-score model were constructed based on clinical information, CUS features and Rad-score (Figure 4). The AUC of CUS model, Rad-score model, Clin + Rad-score model, Rad-score + CUS model, and Clin + CUS + Rad-score model were 0.72, 0.80, 0.85, 0.83 and 0.86 in the training set, respectively (Figure 5; Table 3). The AUC of CUS model, Rad-score model, Clin + Rad-score model, Rad-score + CUS model, and Clin + CUS + Rad-score model were 0.80, 0.77, 0.82, 0.81 and 0.85 in the validation set. By the Delong test, there was no significant difference in the AUC of the Rad-score between the training set and the validation set (p=0.70). There were no significant differences among all models in terms of AUC. A nomogram that contains Rad-score, shape and tumor history variables describing SCLNs to predict the malignant lesion with CUS suspected supraclavicular lymphadenectasis.
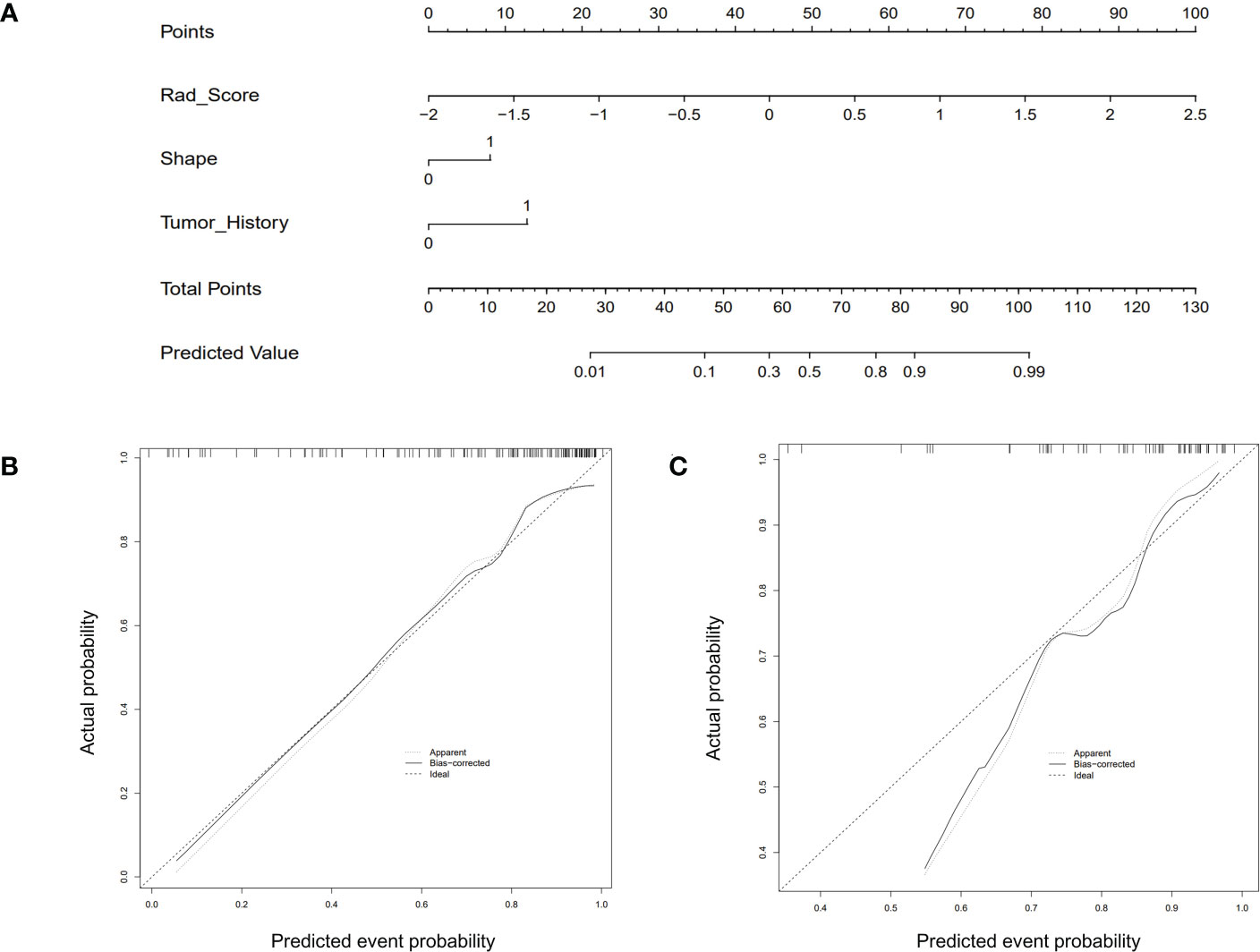
Figure 4 The CUS-based radiomics nomogram and calibration curves of the nomogram. (A) Integrating Rad-score, shape, and tumor history, the CUS-based nomogram was established. Calibration curves of the nomogram in the training (B) and testing (C) set.
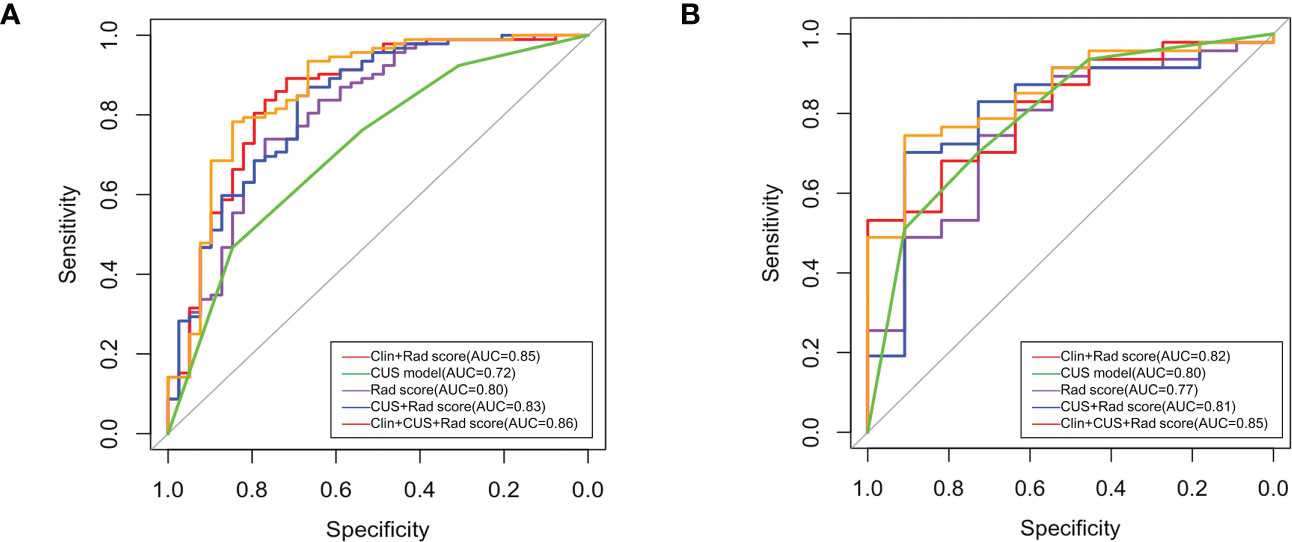
Figure 5 Receiver operating characteristic (ROC) curves of all models in training set (A) and validation set (B).
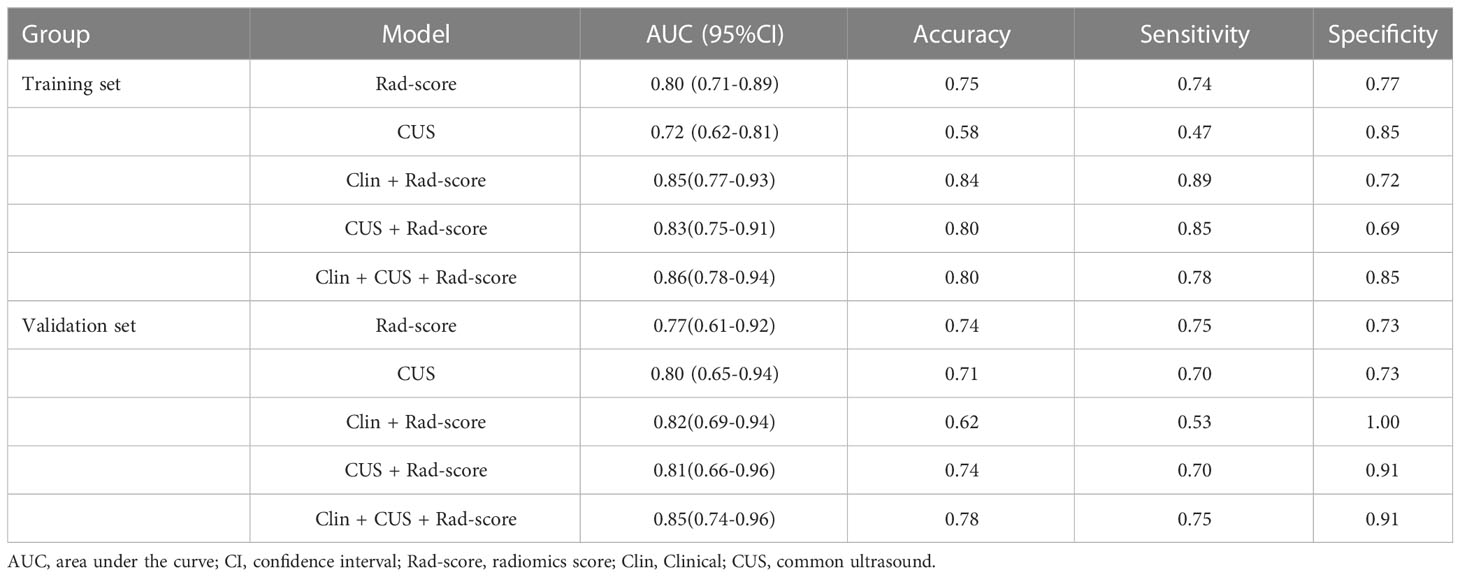
Table 3 The ROC, accuracy, sensitivity, and specificity of five models in the training set and validation set.
4 Discussion
SCLN metastasis is of great significance for treatment decision-making and prognostic evaluation (9–11). Among all imaging methods, CUS is considered the most convenient method for assessing the characteristics of SCLN. However, the diagnostic value of CUS in assessing lymph nodes is controversial. Zheng et al.’s study reported that the diagnostic performance of axillary CUS was poor with an AUC of 0.585-0.719 (12). In our study, several clinical and ultrasound imaging features were associated with the differential diagnosis of benign and malignant lesions. Benign SCLNs were more likely to have no tumor history, which was consistent with previous research (13). For CUS features, well-defined margin, clear boundary, and long/short diameter ≥ 2 shape were commonly observed in benign lesions, but also occasionally seen in malignant lesions (14). The overlapping of CUS features and clinic data brings some difficulties in the diagnosis of benign and malignant lymph nodes.
CUS-based radiomics could provide a large number of quantitative image features from ultrasonic images, which tend to be hard for the naked eyes to recognize (7). Seven hundred and forty-four radiomics features were selected from SCLN. These included first-order statistics and high-order features with various filters (NGTDM, GLRLM, GLSZM, GLCM, and GLDM). First-order statistics, also known as grayscale histogram features, are mainly used to perform statistical calculations on the entire image or the ROI within the image, and are used to describe the grayscale of the image. Second-order statistics refer to the spatial relationship between the intensities of each voxel. Higher-order statistics are used for feature extraction and image preprocessing such as wavelet decomposition, Fourier transform and other filtering (15). The software automatically extracts radiomics features to compensate for errors introduced by manual and subjective measurements. In our results, the AUCs of Rad-score model, Clin + Rad-score model, Rad-score + CUS model, and Clin + CUS + Rad-score model were 0.80, 0.85, 0.83, 0.86 in the training set and 0.77, 0.82, 0.81, 0.85 in the validation set. There were no significant differences among all models in terms of AUC. Zhou et al. (16) developed an ultrasound radiomics nomogram to identify central lymph node metastasis in patient papillary thyroid cancer, the AUCs for the training set, internal validation set, and external validation set were 0.816 and 0.858, respectively. Radiomics based on analysis of CUS images showed good performances as other routine methods. Through the Rad-score model, we could distinguish benign and malignant lesions when supraclavicular lymphadenopathy is suspected on ultrasonography.
This study has some limitations. First, the overall sample size is small. The sample size should be expanded in future studies. Second, this was a single-center retrospective study with good predictive power, which may indicate the need for an external validation set to validate this predictive model. Third, the study included several different ultrasound machines that could have affected the findings. Fourth, the biopsy specimen had a possibility of false negative. In the future, large, multi-center clinical studies, more subgroups and enhanced ultrasound images are needed to further confirm the findings of this study.
5 Conclusion
In conclusion, the radiomics nomogram, derived from CUS, showed favorable prediction efficacy for differentiating benign from malignant in patients with suspected supraclavicular lymphadenectasis. The Rad-score model improves the differentiation of the benign lesion from malignant lesion.
Data availability statement
The raw data supporting the conclusions of this article will be made available by the authors, without undue reservation.
Ethics statement
The retrospective study was approved by the ethics consultant committee of Zhejiang University School of Medicine Second Affiliated Hospital. Written informed consent for participation was not required for this study in accordance with the national legislation and the institutional requirements.
Author contributions
JL and PJ contributed equally to this study. JL and PJ were responsible for the conception and design of this study. JL and JC contributed to the data analysis and writing of the manuscript. YC, FQ, and TW contributed to data collection. YH, YZ, HP, and PJ contributed to data analysis and manuscript preparation. PH contributed to writing-reviewing. All authors contributed to the article and approved the submitted version.
Funding
This study was funded by the National Natural Science Foundation of China (Grants No. 81420108018), Natural Science Foundation nonprofit research projects of Zhejiang Province of China (Grants No.LGF19H180020) and China ultrasound physician technology star program (Grants No.KJXX2018003).
Acknowledgments
We thank the National Natural Science Foundation of China, Natural Science Foundation nonprofit research projects of Zhejiang Province of China, China ultrasound physician technology star program for support.
Conflict of interest
The authors declare that the research was conducted in the absence of any commercial or financial relationships that could be construed as a potential conflict of interest.
Publisher’s note
All claims expressed in this article are solely those of the authors and do not necessarily represent those of their affiliated organizations, or those of the publisher, the editors and the reviewers. Any product that may be evaluated in this article, or claim that may be made by its manufacturer, is not guaranteed or endorsed by the publisher.
Supplementary material
The Supplementary Material for this article can be found online at: https://www.frontiersin.org/articles/10.3389/fonc.2023.1048205/full#supplementary-material
References
1. Zhang W, Peng J, Zhao S, Wu W, Yang J, Ye J, et al. Deep learning combined with radiomics for the classification of enlarged cervical lymph nodes. J Cancer Res Clin Oncol (2022), 148(10):2773–2780. doi: 10.1007/s00432-022-04047-5
2. Yu J, Deng Y, Liu T, Zhou J, Jia X, Xiao T, et al. Lymph node metastasis prediction of papillary thyroid carcinoma based on transfer learning radiomics. Nat Commun (2020) 11(1):4807. doi: 10.1038/s41467-020-18497-3
3. Coopey SB. Supraclavicular and contralateral axillary lymph node involvement in breast cancer patients. Ann Surg Oncol (2022), 29(10):6100–6105. doi: 10.1245/s10434-022-12134-7
4. Morawitz J, Bruckmann NM, Dietzel F, Ullrich T, Bittner AK, Hoffmann O, et al. Comparison of nodal staging between CT, MRI, and [(18)F]-FDG PET/MRI in patients with newly diagnosed breast cancer. Eur J Nucl Med Mol Imag. (2022) 49(3):992–1001. doi: 10.1007/s00259-021-05502-0
5. Xv Y, Lv F, Guo H, Liu Z, Luo D, Liu J, et al. A CT-based radiomics nomogram integrated with clinic-radiological features for preoperatively predicting WHO/ISUP grade of clear cell renal cell carcinoma. Front Oncol (2021) 11:712554. doi: 10.3389/fonc.2021.712554
6. Bernatowicz K, Grussu F, Ligero M, Garcia A, Delgado E, Perez-Lopez R. Robust imaging habitat computation using voxel-wise radiomics features. Sci Rep (2021) 11(1):20133. doi: 10.1038/s41598-021-99701-2
7. Li Q, Jiang T, Zhang C, Zhang Y, Huang Z, Zhou H, et al. A nomogram based on clinical information, conventional ultrasound and radiomics improves prediction of malignant parotid gland lesions. Cancer Lett (2022) 527:107–14. doi: 10.1016/j.canlet.2021.12.015
8. Jin P, Chen J, Dong Y, Zhang C, Chen Y, Zhang C, et al. Ultrasound-based radiomics nomogram combined with clinical features for the prediction of central lymph node metastasis in papillary thyroid carcinoma patients with hashimoto’s thyroiditis. Front Endocrinol (Lausanne). (2022) 13:993564. doi: 10.3389/fendo.2022.993564
9. Chen SC, Shen SC, Yu CC, Huang TS, Lo YF, Chang HK, et al. Long-term outcomes of breast cancer patients who underwent selective neck dissection for metachronous isolated supraclavicular nodal metastasis. Cancers (Basel) (2021) 14(1):164. doi: 10.3390/cancers14010164
10. Diao K, Andring LM, Barcenas CH, Singh P, Carisa Le-Petross H, Reed VK, et al. Contemporary outcomes after multimodality therapy in patients with breast cancer presenting with ipsilateral supraclavicular node involvement. Int J Radiat Oncol Biol Phys (2022) 112(1):66–74. doi: 10.1016/j.ijrobp.2021.08.026
11. Li Y, Wang T, Fu YF, Shi YB, Wang JY. Computed tomography-guided biopsy for sub-centimetre lung nodules: Technical success and diagnostic accuracy. Clin Respir J (2020) 14(7):605–10. doi: 10.1111/crj.13172
12. Zheng X, Yao Z, Huang Y, Yu Y, Wang Y, Liu Y, et al. Deep learning radiomics can predict axillary lymph node status in early-stage breast cancer. Nat Commun (2020) 11(1):1236. doi: 10.1038/s41467-020-15027-z
13. Gronkiewicz JJ, Vade A. Cervical lymph node fine needle aspiration in patients with no history of malignancy. Ultrasound Q (2013) 29(4):323–6. doi: 10.1097/RUQ.0b013e3182a44eff
14. Ye F, Gong Y, Tang K, Xu Y, Zhang R, Chen S, et al. Contrast-enhanced ultrasound characteristics of preoperative central cervical lymph node metastasis in papillary thyroid carcinoma. Front Endocrinol (Lausanne). (2022) 13:941905. doi: 10.3389/fendo.2022.941905
15. Linsalata S, Borgheresi R, Marfisi D, Barca P, Sainato A, Paiar F, et al. Radiomics of patients with locally advanced rectal cancer: Effect of preprocessing on features estimation from computed tomography imaging. BioMed Res Int (2022) 2022:2003286. doi: 10.1155/2022/2003286
Keywords: supraclavicular lymph node, radiomics, ultrasound, nomogram, lymphadenectasis
Citation: Luo J, Jin P, Chen J, Chen Y, Qiu F, Wang T, Zhang Y, Pan H, Hong Y and Huang P (2023) Clinical features combined with ultrasound-based radiomics nomogram for discrimination between benign and malignant lesions in ultrasound suspected supraclavicular lymphadenectasis. Front. Oncol. 13:1048205. doi: 10.3389/fonc.2023.1048205
Received: 19 September 2022; Accepted: 24 February 2023;
Published: 09 March 2023.
Edited by:
Lorenz Kadletz-Wanke, Medical University of Vienna, AustriaReviewed by:
Jianhua Zhou, Sun Yat-sen University Cancer Center (SYSUCC), ChinaLun Li, Fudan University, China
Copyright © 2023 Luo, Jin, Chen, Chen, Qiu, Wang, Zhang, Pan, Hong and Huang. This is an open-access article distributed under the terms of the Creative Commons Attribution License (CC BY). The use, distribution or reproduction in other forums is permitted, provided the original author(s) and the copyright owner(s) are credited and that the original publication in this journal is cited, in accordance with accepted academic practice. No use, distribution or reproduction is permitted which does not comply with these terms.
*Correspondence: Pintong Huang, aHVhbmdwaW50b25nQHpqdS5lZHUuY24=
†These authors have contributed equally to this work