- 1Department of Ultrasound, The First Affiliated Hospital of Nanjing Medical University, Nanjing, Jiangsu, China
- 2Department of Cardiovascular Ultrasound, Nanjing First Hospital, Nanjing Medical University, Nanjing, Jiangsu, China
Objectives: To develop and validate a nomogram to predict the overall survival (OS) of patients with primary nodal diffuse large B-cell lymphoma(N-DLBCL) based on radiomic features and clinical features.
Materials and methods: A retrospective analysis was performed on 145 patients confirmed with N-DLBCL and they were randomly assigned to training set(n=78), internal validation set(n=33), external validation set(n=34). First, a clinical model (model 1) was established according to clinical features and ultrasound (US) results. Then, based on the radiomics features extracted from conventional ultrasound images, a radiomic signature was constructed (model 2), and the radiomics score (Rad-Score) was calculated. Finally, a comprehensive model was established (model 3) combined with Rad-score and clinical features. Receiver operating characteristic (ROC) curves were employed to evaluate the performance of model 1, model 2 and model 3. Based on model 3, we plotted a nomogram. Calibration curves were used to test the effectiveness of the nomogram, and decision curve analysis (DCA) was used to asset the nomogram in clinical use.
Results: According to multivariate analysis, 3 clinical features and Rad-score were finally selected to construct the model 3, which showed better predictive value for OS in patients with N-DLBCL than mode 1 and model 2 in training (AUC,0. 891 vs. 0.779 vs.0.756), internal validation (AUC, 0.868 vs. 0.713, vs.0.756) and external validation (AUC, 914 vs. 0.866, vs.0.789) sets. Decision curve analysis demonstrated that the nomogram based on model 3 was more clinically useful than the other two models.
Conclusion: The developed nomogram is a useful tool for precisely analyzing the prognosis of N-DLBCL patients, which could help clinicians in making personalized survival predictions and assessing individualized clinical options.
Introduction
Diffuse large B-cell lymphoma (DLBCL) is the most common subtype of lymphoma, accounting for about 30% to 40% of the total incidence of all non-Hodgkin’s lymphomas (NHL) (1, 2). According to the site of origin, DLBCL can be divided into primary nodal diffuse large B-cell lymphoma (N-DLBCL) and primary extranodal diffuse large B-cell lymphoma (EN-DLBCL) (3). DLBCL has obvious heterogeneity in morphology, immunophenotype, genetics and clinical manifestations (4). Nowadays, the treatment of DLBCL has made great progress, immunochemical therapy of rituximab, cyclophosphamide, doxorubicin, vincristine and prednisone (R-CHOP) is the preferred treatment regimen for DLBCL (5). The application of rituximab increased the 5-year survival rate of DLBCL by at least 15% and the cure rate significantly, but there were still more than 30% of patients with primary drug resistance or relapse (6). These recurrent or refractory patients had a poor prognosis and high mortality (7). How to identify these patients as early as possible, accurately predict their efficacy and prognosis, and carry out individualized treatment? It has been an urgent clinical problem to be solved.
At present, 18F-deoxyglucose (FDG) positron emission tomography/computed tomography (PET/CT) has been widely used in evaluating the prognosis of DLBCL (8). Several studies (9, 10) have tested the use of metabolic intensity to predict Progression-Free Survival (PFS) and overall survival (OS) in patients with lymphoma. The most used parameter is the maximum standard uptake value (SUVmax) because it provides a method of measurement independent of the observer (11). However, the reliability of SUVmax may be affected by many factors, such as the attenuation of injection dose, the time between injection and imaging acquisition, partial volume effect and technical characteristics and parameters (12). Recently, new indicators for estimating the overall tumor load based on PET/CT staging, such as metabolic tumor volume (MTV) or total lesion glycolysis (TLG), have been used to predict PFS and OS in patients with lymphoma (13). In addition, international prognostic index (IPI) is currently used for estimating pretreatment risk, while IPI only comes from the clinicopathological features before treatment, which lacks the information to reflect the functional and metabolic characteristics of the tumor. Hence, the IPI often does not reliably predict the individual patient outcome (14).Therefore, the above evaluation indicators fail to capture the heterogeneity of tumors, which is a key prognostic factor for the progression, recurrence, and drug resistance of DLBCL, and closely related to tumor invasiveness, metastasis, and molecular characteristics. This limitation is a major challenge in DLBCL treatment. Therefore, we need to find a new imaging method, which can not only evaluate the treatment effect of patients in real time and dynamically, but capture the heterogeneity of metabolism in the tumor, to help clinicians modify the treatment plan in time and accurately predict the clinical outcome of DLBCL.
Ultrasound (US) can evaluate the shape, size, echo texture and blood flow pattern of lymph nodes in real time and dynamically (15). Radiomic is a method that uses complex computer algorithms to extract a large amount of data from images routinely obtained in the clinical environment, revealing hidden features of tumor from various imaging modes (16, 17), which can assist doctors to make the most accurate diagnosis by means of deeper mining and analysis of massive image data (18, 19). Hence, heterogeneity-related parameters provided by images could contribute to more personalized treatment and reduce the occurrence of toxicity. In this way, the possibility of favorable outcomes is increased, and intensive treatment programs can be provided for high-risk patients (20). Radiomic of 18F-FDG PET/CT have been demonstrated to be useful in predicting the outcomes of DLBCL and Hodgkin’s lymphoma (21, 22). Radiomic based on ultrasound has a good application prospect in the evaluation of curative effect and prognosis of other malignant tumors such as breast and gastrointestinal tumors (23–25). In lymphoma, radiomic shows hope in the differential diagnosis of lymphoma from other Lymph node diseases (26). To our knowledge, no previous study has associated radiomic signatures based on ultrasound with the outcome of patients with N-DLBCL.
This study was aimed at developing and validating the nomogram by radiomic features on ultrasound imaging to predict OS of patients with N-DLBCL more accurately and provide new ideas for personalized clinical treatment and visual evaluation of N-DLBCL.
Patients
Patients newly confirmed with DLBCL and treated in our medical central from August 2009 to October 2021 were retrospectively analyzed. Exclusion criteria: ①Patients with other malignant tumors; ②Patients with Ann arbor IE staging; ③Patients treated in other hospitals or relapsed patients; ④Patients without complete US and clinical data. Inclusion criteria: ①Patients who underwent PET-CT; ②Lymph nodes with a maximum SUV value; ③Lymph nodes with core needle biopsy or resection biopsy. Finally, 111 patients with a total of 111 lymph nodes were included in this study. They were randomly assigned to training set(n=78) and internal validation set(n=33) (7:3 ratio). Besides, the independent external validation set consists of 34 patients from the other two institutions who meet the above exclusion and inclusion criteria. Histopathological diagnosis was based on the result of core needle biopsy or lymph node excision (excision biopsy is required only if the ultrasound-guided biopsy results are uncertain). Clinical variables of each patient were recorded, including gender, age at diagnosis, Bulky disease, B symptoms, Ann Arbor stage, Eastern Cooperative Oncology Group (ECOG), lactate dehydrogenase (LDH) level, serum β2-microglobulin (β2-MG) level, serum hemoglobin (HB) level, extra-nodal involvement, international prognostic index (IPI), state after first-line standardized chemotherapy, POD24, BCL6, BCL2 and treatment regimens. Disease staging was conducted in accordance with the Ann Arbor system. Bulky disease was defined as a nodal mass larger than 10 cm in diameter. State after first-line standardized chemotherapy were separated into two response categories as complete response(CR) and incomplete response (ICR, including partial response, stable and progression) (27).
The study was approved by the Institutional Ethics Committee of our hospital [Ethical number 2022-SR-058], and because it is a retrospective analysis, the requirement of written informed consent was waived.
US image acquisition
The LogiqE9 ultrasound machine (GE Healthcare), with a 15–4 MHz linear probe (Super Linear™ SL15-4) was employed in ultrasonic examination. Two experienced radiologists used standardized institutional protocols to independently record and review all preoperative US features. If the radiologist had a different opinion on the conclusion, the final decision was made between them after a discussion. The patient was taken in a comfortable posture to fully expose the site of examination. The lymph node that underwent biopsy at the site of onset was selected as the target lymph node. The following parameters of lymph nodes were observed and measured, including size (cross-section, longitudinal-section), the ratio of the longitudinal diameter to the short axis (Solbiati index, SI>2, SI<2), sharp (regular, irregular), visibility of the hilum (present, absent), border (clear, unclear), Adler grade of blood flow (grades 0-3) (28).
ROI segmentation and radiomics features extraction
The US images of all DLBCL patients were export from the Ultrasonic instrument. The maximum longitudinal-section area of images was manually segmented by two ultrasound experts (more than 5 years of experience) using open-source software (ITK-SNAP 3.8.0; http://www.itksnap.org) to generate a region of interest (ROI) containing all the segmented lesions. A total of 464 radiomics features were extracted from the US images, including 90 first-order features and 374 texture features. The first-order features include shape, size, and strength features and texture feature extraction is based on four texture matrices, including grey level cooccurrence matrix (GLCM), grey level run-length matrix (GLRLM), grey level size zone matrix (GLSZM) and gray level dependence matrix (GLDM). All radiomics features were analyzed and mined by PyRadiomic open-source software package.
Radiomics features selection and signature calculation
One radiologist randomly selected 20 lesions from the training cohort to draw ROI again, and the other radiologist repeated it independently within three weeks. The stability of the feature is determined by calculating the inter-observe correlation coefficient (ICC). Radiomics features with ICC lower than 0.75 were excluded from the final feature data set (18).
To obtain the optimal subset of radiomics features, minimal redundancy maximum relevancy (mRMR) and the least absolute shrinkage selection operator (LASSO) with 10-fold cross-validation (the criteria as maximum area under the ROC curve) was further used to select most candidate radiomic features. Finally, 10 radiomics features were screened out. Therefore, the radiomics “Radcore” is calculated according to formula (1) (29).
where β0 is the constant term in the regression, βi is logistic regression coefficient, and χi the value of selected features.
Construction of clinical model, radiomics model, and combined model
Univariate and multivariate COX regression analysis were used to analyze the influence of clinical variables, US characteristics. Then the characteristics of p< 0.05 in multivariate analysis were used to establish the model 1(clinical and US characteristics) of the training set. The optimal radiomics feature subset obtained by LASSO Logistic regression method was used to construct model 2 (radiomics features) in the training set. ROC curves were used to determine the cut-off value of each group feature, and the continuous variables were transformed into classified variables. The radiological signals constructed were mixed with clinical factors, and the univariate analysis and minimum Akaike information criterion (AIC) criterion COX regression analysis were obtained in turn. Model 3 (combined features) was constructed based on COX regression coefficient.
Model performance assessment
Three established models were validated using independent internal and external datasets. The discriminant ability of each model was analyzed by receiver operating characteristic curve (ROC), and area under curve (AUC), sensitivity, specificity of them were obtained.
Development and validation of the nomogram
According to the model 3, a nomogram, convenient for clinical application, was plotted. The model correction was evaluated by correction curve analysis and Hosmer-Lemeshow test. Decision curve analysis (DCA) was used to evaluate the clinical usefulness and net benefit of the predictive model in validation set. Delong test was used to compare the AUC of each pair of models.
Patients’ treatment and follow-up
Patients diagnosed with N-DLBCL received standardized R-CHOP chemotherapy (n=105) or approved clinical drug verification(n=40). The follow-up data were obtained by electronic medical records and telephone interviews. Overall survival (OS) refers to the time from diagnosis until death due to any cause.
Statistical analysis
The classification variables were expressed by the number of cases, using chi-square test (χ2) or Fisher exact test. A SPSS software (version 25.0) was used for univariate analysis and multivariate analysis. R software (version 3.6.1, R Project for Statistical Computing, www.r-project.org) was used for radiomic features analysis. The LASSO logistic regression method was implemented using the glmnet package in R software. Two-sided p value less than 0.05 was assumed to indicate statistical significance.
Result
Patient characteristics
One hundred and forty-five patients with 145 lymph nodes were enrolled in this study, including 78 males and 67 females, ranging from the age of 21 to 85 (mean age, 58 ± 12). The research flowchart is shown in Figure 1. The median follow-up time was 36 months (range, 3–137 months). By the date of the last follow-up, a total of 41 patients had died, with a total survival rate of 71.7%. 1-year survival rate, 3-year survival rate and 5-year survival rate were 88.3%, 80.6% and 73.8% respectively. The clinical and ultrasonic features of the training set and verification set were summarized in Table 1. All the characteristics were not statistically significant between the two sets.
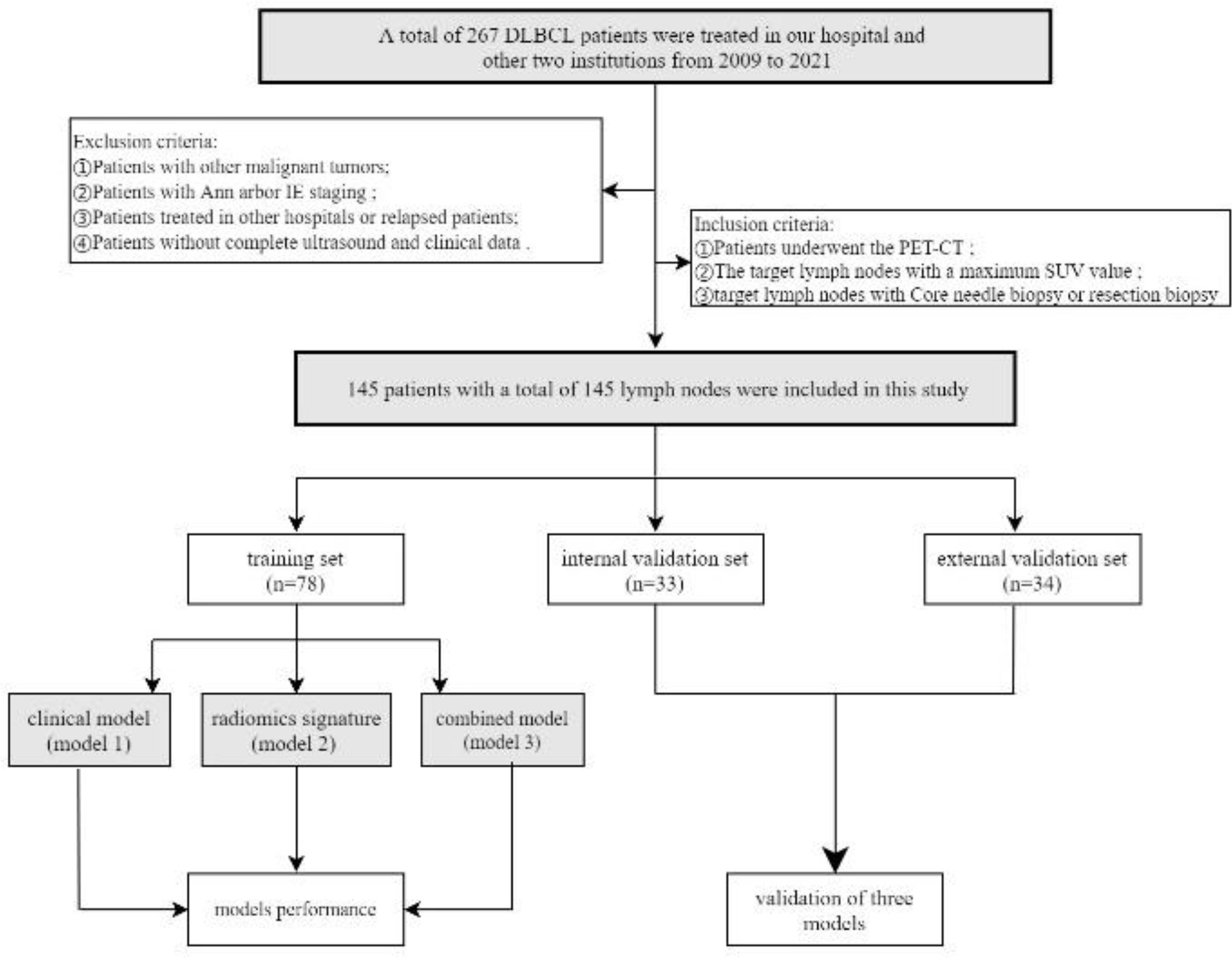
Figure 1 Flow chart of patients’recruitment pathway. DLBCL, Diffuse large B-cell lymphoma; PET-CT, Positron emission tomography/computed tomography. According to the inclusion and exclusion criteria, 145 patients with a total of 145 lymph nodes were included in this study. The patients of our institutions were randomly assigned to training set(n=78) and internal validation set(n=33) (7:3 ratio). The independent external validation set(n=34) from the other two institutions.
Model 1: Clinical features and US features
In the training set, univariate analysis in Table 2 showed that five variables were related to OS. Multivariate analysis showed that hilus, extra-nodal involvement, state after first-line standardized chemotherapy were independent predictors of OS (p<0.05) (Table 3). The diagnostic performance of this model was moderate with an AUC of 0.779 (95% CI, 0.660-0.897). The sensitivity and specificity were 73.6% and 81.4%, respectively.
Model 2: Radiomics signature
After intra-observer and inter-observer reliability analysis, 340 stable features with ICC score larger than 0.75 were retained for follow-up analysis, and finally 10 radiomics features were selected into the LASSO Logistic regression model (Figures 2, 3). ICC values are provided in the supplementary information. Table 4 displays that variables A to J represent 10 selected radiomic features, Rad-score=−1.317+0.403×A+0.094×B+(−0.349) ×C+(−0.081) ×D+0.005×E+(−0.0.310) ×F+0.682×G+0.036×H+(−0.161) ×I+0.005×J. The discriminative ability of radiomics model was low with an AUC of 0.756 (95% CI, 0.622-0.889). The sensitivity and specificity are 93.2%, 52.6% respectively.
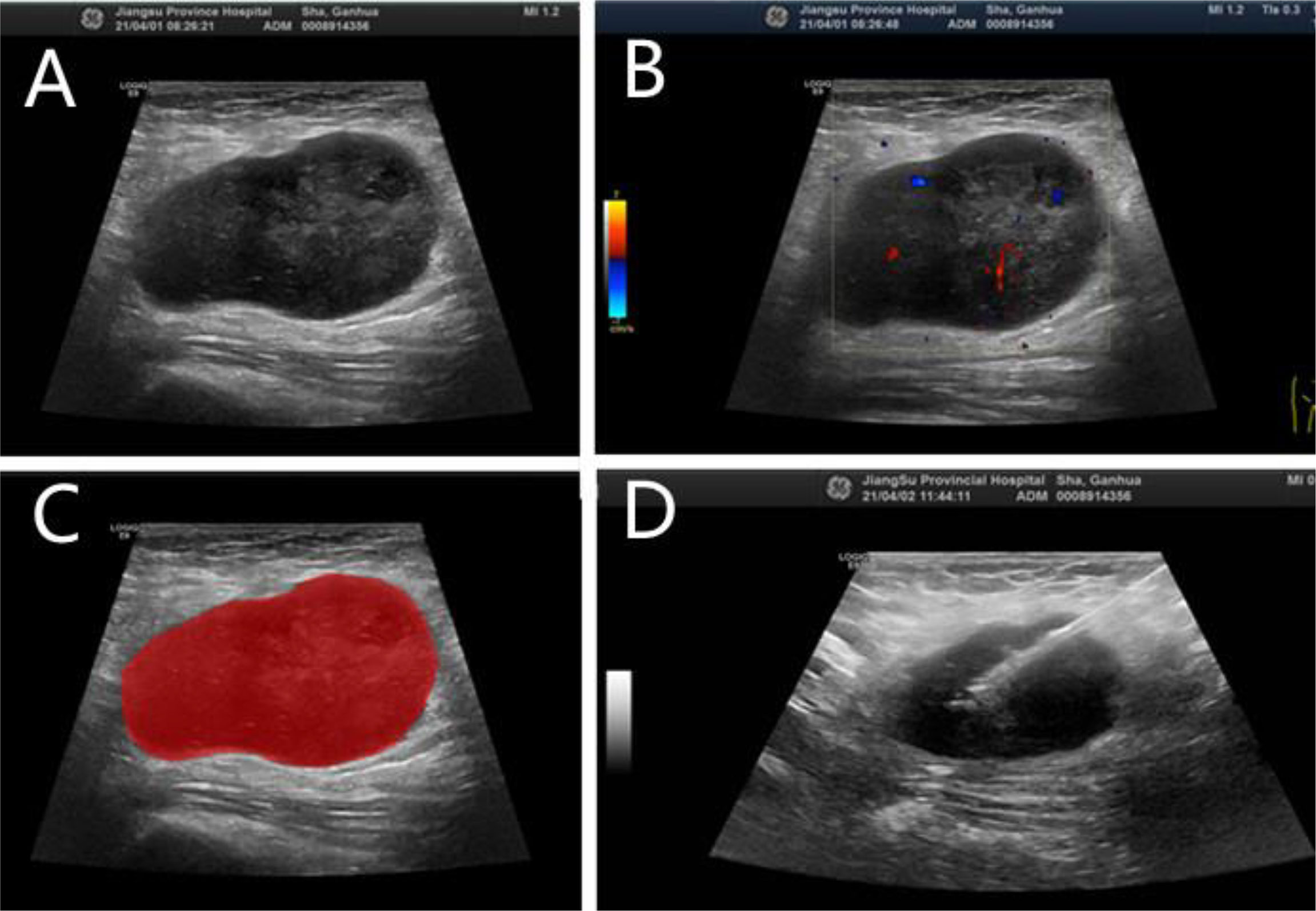
Figure 2 N-DLBCL in a 58-year-old man. (A) Gray-scale ultrasonic image. (B) CDFI image. (C) Radiomic ROI segmentation segmentation of the mass. (D) Ultrasound-guided core needle biopsy.
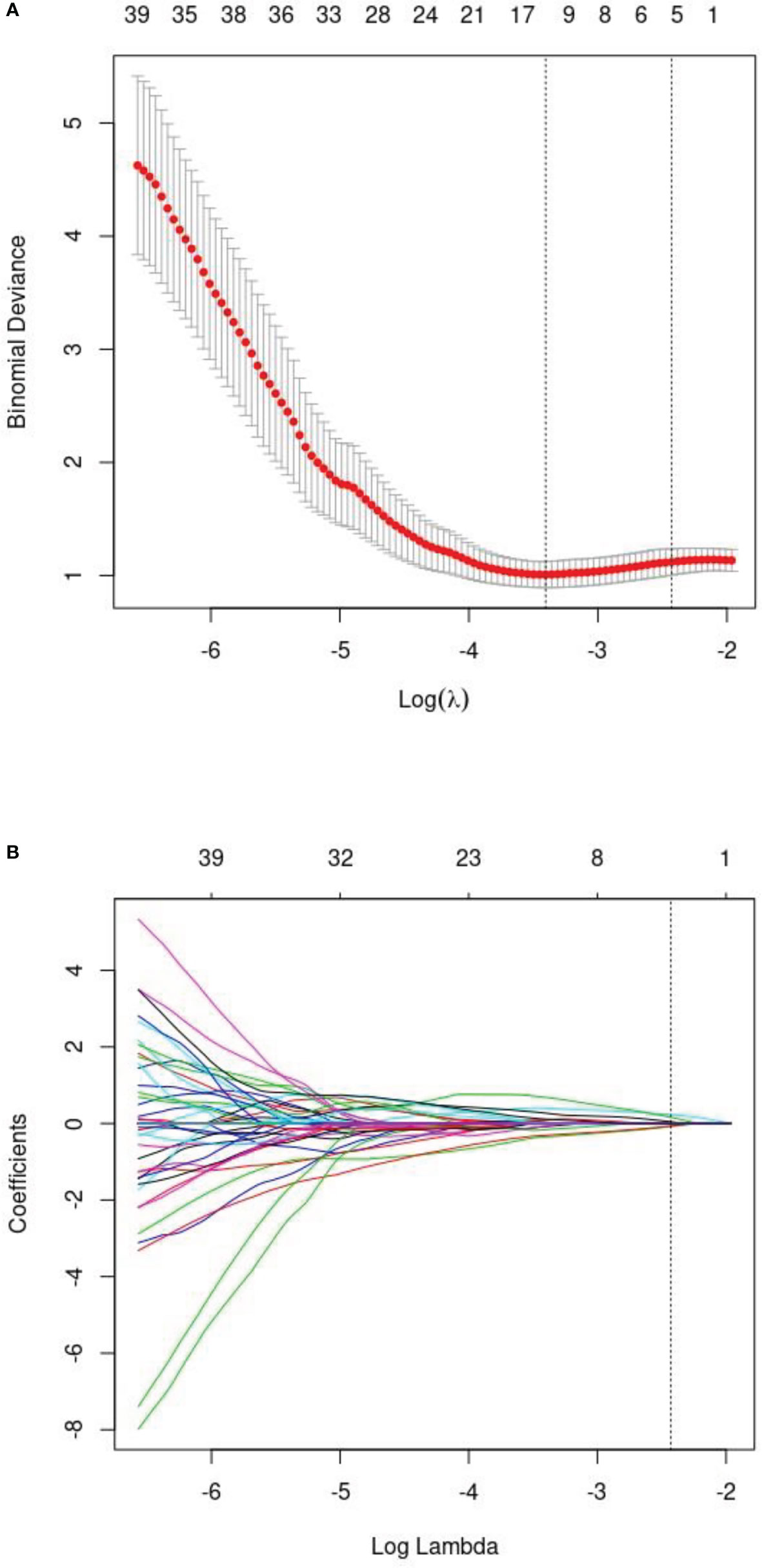
Figure 3 (A) The least absolute shrinkage andselection operator (LASSO) logistic regression for radiomics features selection and signature construction. (B) On the basis of minimum criteria for the least cross-validation binominal deviance, a tuning parameter (l) was selected via 10-fold cross validation.according to 10-fold cross-validation, 10 radiomic features were obtained.
Model 3: Comprehensive model
A comprehensive model was constructed based on multivariate Cox analysis of significant risk factors. The risk factors included Rad-score(p=0.012) and hilus (p=0.020), Extra-nodal involvement (p=0.027), state after first-line (p=0.023). The diagnostic efficiency of combined model is significantly improved, with an AUC of 0.891 (95% CI, 0.807-0.975). The sensitivity and specificity were 89.8% and 73.7% respectively.
Validation and diagnostic performance comparison of three models
Three established models were validated using independent datasets. In the internal validation set, the AUC, sensitivity, and specificity of model 1 were 0.713(95%CI,0.532-0.894), 59.1%, 81.8%. The AUC, sensitivity, specificity of model 2 were 0.756(95%CI,0.593-0.919), 54.5%, 73.2%; The AUC, sensitivity, specificity of model 3 were 0.868(95%CI,0.746-0.990), 81.8%, 81.8%. In the external validation set, the AUC, sensitivity, and specificity of model 1 were 0.866(95%CI,0.808-0.925), 87.7%, 80.8%. The AUC, sensitivity, specificity of model 2 were 0.789(95%CI,0.714-0.863), 92.7%, 60.6%; The AUC, sensitivity, specificity of model 3 were 0.914(95%CI,0.868-0.960), 95.1%, 81.7%. Figure 4 shows the ROC curves of each model for both the training and validation sets, Table 5 shows the cut-off value, sensitivity, specificity, and AUC of each mode. The AUC of the comprehensive model was significantly higher than that of model 1 or model 2 in both the training and validation sets.
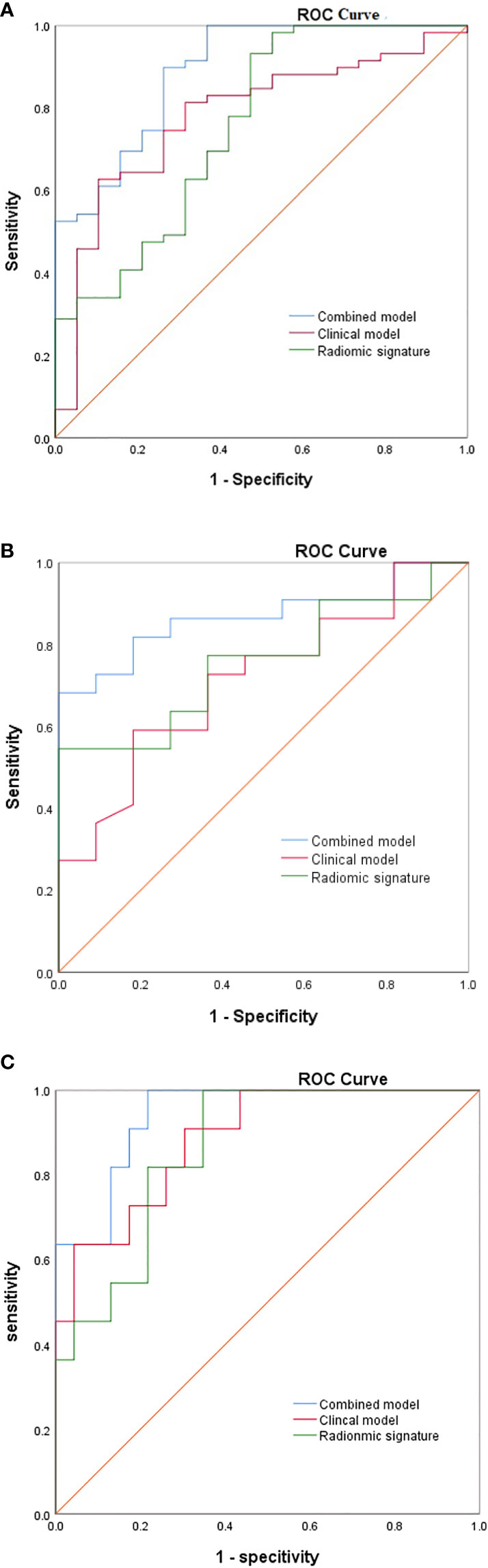
Figure 4 The ROC of the three model from (A) the training cohorts, (B) the internal validation cohort, (C) the external validation cohort.
Development and performance of the nomogram
A nomogram (Figure 5A) was conducted based on the comprehensive model, and favorable calibrations of the nomogram were confirmed both in the training (Figure 5B), the internal validation set (Figure 5C), the internal validation set (Figure 5D). Hosmer–Lemeshow test possessed a p value of 0.334, 0.738 and 0.679, respectively. The DCA (Figures 6A, B) indicated that the nomogram had a higher diagnostic efficiency than model 1 or model 2.
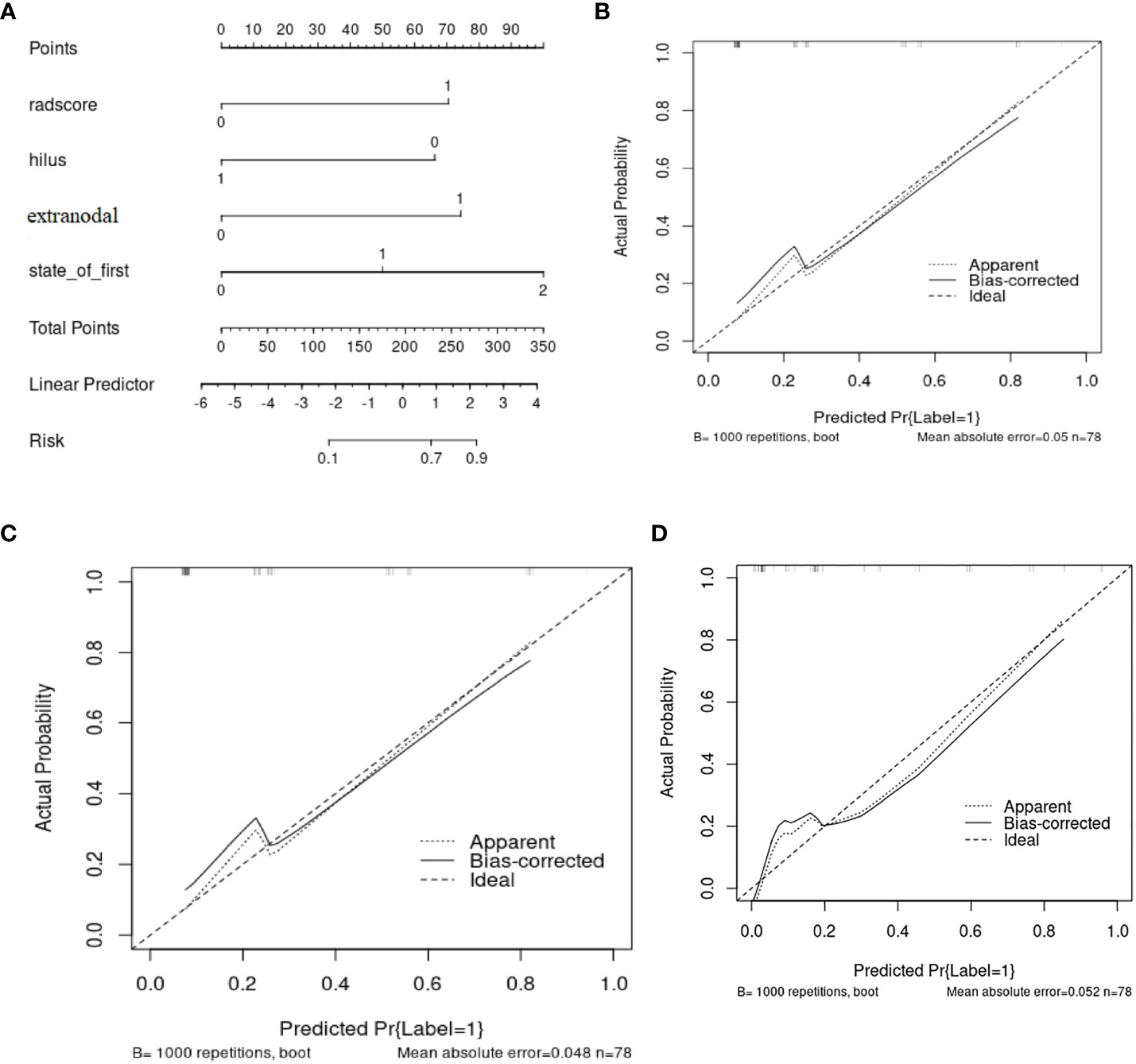
Figure 5 (A) The nomogram base on the model 3 and the calibration curve from (B) the training cohorts, (C) the internal validation cohort, (D) the internal validation cohort.
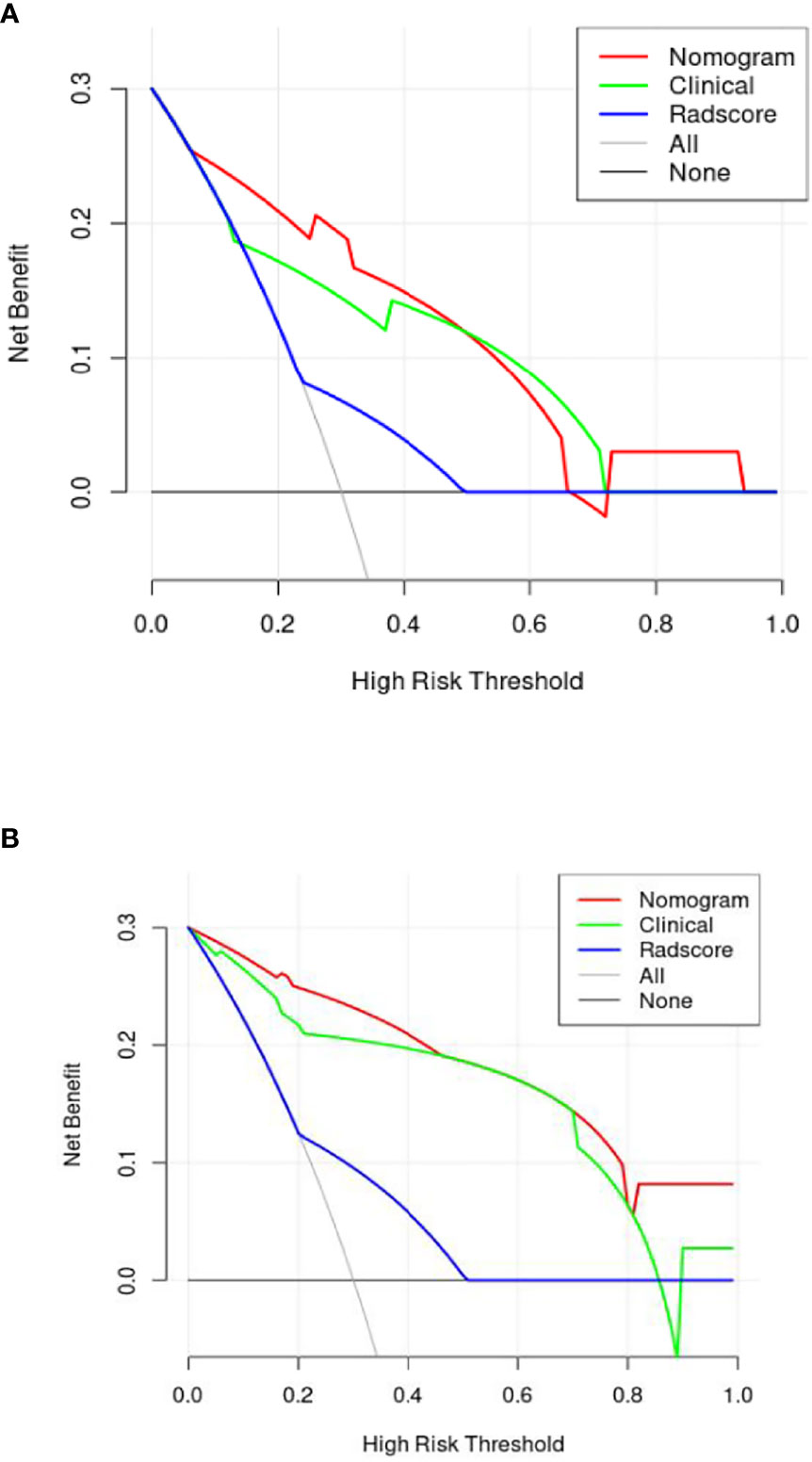
Figure 6 The decision curve [(A) is internal verification, (B) is external verification] analysis of the combined nomogram for prediction overall survival in patients with primary nodal Diffuse large B-cell lymphoma. The DCA indicated that the nomogram had a higher diagnostic efficiency than the clinical model and radiomics signature.
Discussion
This study established three models for predicting the prognosis of patients with N-DLBCL, and it was found that the model with the combination of radiomic and clinical features had good predictive value. On this basis, we developed a nomogram based on the combined model, and verified the nomogram. The results showed that the nomogram could well predict the OS of patients with N-DLBCL. Thus, nomograms can be used by clinicians to make precise and individualized medical decisions.
All N-DLBCL patients in our study were adults, which was in line with that reported in the literature for Chinese studies, Sun et al. (30)showed that the mean age of DLBCL patients was 51.6 years. Besides, consistent with the previous study (30, 31), most of the DLBCL cohort in this study was male patients, who accounted for 53.7% (78/145). The 5-year survival rate of 145patients in this study was 73.8% (107/145), which was almost the same as the report (74.8%) of Xia et al. (32), but higher than previous reports (43%-52%) (33). The reason is that the subjects in this study and Xia et al. are all primary intranodal DLBCL. Moo-Kon Song et al. (34) reported EN- DLBCL, such as originating from the gastric, intestinal tract and so on are even worse, especially in the non-GCB type. Some studies (3, 35) also have demonstrated that the involvement of the extranodal tissue may lead to a worse prognosis for patients with nodal lesions. The results of univariate and multivariate analysis showed state after the 6 cycles standardized treatment was the prediction factors of OS. After 6 cycles of standardized treatment, according to the results of PET-CT assessment, the CR rate of patients was 62.1% (90/145) in our study, which was lower than that reported by Ivan et al (36). At present, the effect of BCL-6 gene translocation on the prognosis of DLBCL patients is still controversial. Most scholars believe that the prognosis of BCL-6 positive expression is better, but some scholars hold the opposite view. Akyurek et al. (37) found that BCL-6 gene translocation can affect the OS of DLBCL patients(p=0.04), but there is no significant effect on PFS. This phenomenon is more significant in non-GCB DLBCL patients. In addition, foreign studies (38, 39) conclude that MYC/BCL2 co-expression in DLBCL is associated with an aggressive clinical course, which is more common in the ABC subtype, and contributes to the overall inferior prognosis of patients with ABC-DLBCL. However, in this study, probably due to the small sample size, BCL2 and BCL6 were not predictors of OS. For the same reason, the results of multivariate analysis showed that the other clinical features reported in the literature, including IPI score, LDH level, HB level, β2-MG level, Bulky disease, ECOG, Arbor stage, POD 24, were not associated with OS in the training group and the verification group.
US is also a commonly used diagnostic imaging technique, which possess a higher sensitivity in the detection of superficial enlarged lymph nodes. The feature of lymph nodes can be evaluated according to the hilum, shape, border, size, echo texture and blood flow pattern of lymph nodes (40). In this study, univariate analysis found that the lymphatic hilum structure and boundary is related to OS, while multivariate study found that the absence of lymphatic hilum is an independent predictor of OS. B lymphocytes mainly settle in the superficial cortex of the lymph nodes, some studies demonstrated that the abnormal lymphocytes of DLBLC in the early stage grew locally and did not invade the whole lymph node, but in the late stage, the abnormal lymphocytes infiltrated into the whole lymph node, resulting in the disappearance of the lymphatic hilum or eccentric and thin stripe under pressure (41). At the same time, studies have shown that patients with stage III-IV have a poor prognosis (3, 34). Therefore, in model 1, the absence of lymphatic hilum is a predictor of OS (training set, p=0.045; internal validation set, p=0.012; external validation set, p=0.016). From the previous literatures, in lymphoma, the disease frequently arises inside the lymph node, and (depending on the aggressiveness and natural history of the tumor) it may never reach the subcapsular area, or it may progress in a centrifugal fashion to invade the whole lymph node; in high-grade aggressive lymphomas, the neoplastic cells may even reach the lymph node from outside (as with metastasis) when the disease originates in another lymph node of the group and subsequently infiltrates the remaining nodes, the lesions fused with each other and the boundary was not clear (42), which may explain why in univariate analysis, the boundary is related to OS (training set, p=0.012; internal validation set, p=0.033; external validation set, p=0.048). The univariate and multivariate analysis showed that other ultrasonic features were not related to OS (p≥0.05).
18F-FDG PET/CT has been widely used for diagnosis, staging and response assessment in DLBCL (43). In recent years, there are also many literatures (12, 14, 44) to predict the prognosis of DLBCL based on 18F-FDG PET/CT baseline radiomic features. However, we have not seen a specific explanation about which target lymph nodes should be selected as the object of study, nor the criteria for selection. In this study, the target lymph nodes with the highest SUV value on PET-CT and ultrasound-guided pathological biopsy (Figure 2) were selected as the objects of study to reduce false positive and false negative and improve accuracy. As far as we know, there have been no radiomics based on ultrasound images to predict the prognosis of DLBCL, and the literatures on molecular imaging radiomics of DLBCL are also very limited. Parvez et al. (45) found that GLNGLSZM correlated with disease free survival, and that kurtosis correlated with OS. Aide et al. (4, 46) found that skewness of skeletal heterogeneity was a prognostic factor for PFS, and long-zone high gray level emphasis from GLSZM was a prognostic parameter for 2-year event-free survival. Meanwhile, Cottereau et al. (47) reported that the radiomic feature characterizing lesion dissemination was associated with PFS and OS. Our study found that radiomic features related to OS included two first-order features, two GLCM, four GLSZM, a GLSZM and a GLRLM, which is consistent with the above literature reports. In addition, Wang et al. (39) reported that radiomics are not superior to traditional imaging parameters. In our study, the diagnostic efficacy of radiomic signature (training set, AUC=0.756; internal validation set, AUC=0.756; external validation set, AUC=0.789) is lower than that of clinical model (training set, AUC=0.779; internal validation set, AUC=0.713; external validation set, AUC=0.866), but it can be used as a supplementary index of clinical model, and the diagnostic efficacy of combined model (training set, AUC=0.891; internal validation set, AUC=0.868; external validation set, AUC=0.914) is higher than radiomic signature or clinical model.
Still, there are some limitations in our study. First, this was a retrospective study, and the sample size was relatively small. But we have been collecting more cases and collaborating with other medical centers to expand the sample size, using external verification sets to further validate the nomogram. Second, the radiomic features were only extracted from gray-scale ultrasound images, and hopefully in the future, we can extract them from multimode ultrasound images such as elastography and contrast-enhanced ultrasound.
In conclusion, based on clinic and radiomic features, we have developed and validated a nomogram to predict OS of patients with N-DLBCL. The established nomograms can provide a visualized estimation of risk for each prognostic factor, to assist clinicians take personalized treatment for N-DLBCL patients and improve their prognosis.
Data availability statement
The original contributions presented in the study are included in the article/Supplementary Material. Further inquiries can be directed to the corresponding authors.
Ethics statement
The studies involving human participants were reviewed and approved by The study was approved by the Institutional Ethics Committee of the First Affiliated Hospital of Nanjing Medical University [Ethical number 2022-SR-058], and the requirement of written informed consent was waived. Written informed consent for participation was not required for this study in accordance with the national legislation and the institutional requirements.
Author contributions
HD designed the study and wrote the manuscript. YY, WL, YZ and WC collected the data. LL and HS analyzed the data. HD, PZ, and XY evaluated US images and segmented lesions. All authors contributed to the article and approved the submitted version.
Acknowledgments
Thanks to all the authors for their contributions to this article.
Conflict of interest
The authors declare that the research was conducted in the absence of any commercial or financial relationships that could be construed as a potential conflict of interest.
Publisher’s note
All claims expressed in this article are solely those of the authors and do not necessarily represent those of their affiliated organizations, or those of the publisher, the editors and the reviewers. Any product that may be evaluated in this article, or claim that may be made by its manufacturer, is not guaranteed or endorsed by the publisher.
Supplementary material
The Supplementary Material for this article can be found online at: https://www.frontiersin.org/articles/10.3389/fonc.2022.991948/full#supplementary-material
References
1. Bray F, Ferlay J, Soerjomataram I, Siegel RL, Torre LA, Jemal A. Global cancer statistics 2018: GLOBOCAN estimates of incidence and mortality worldwide for 36 cancers in 185 countries. CA: Cancer J Clin (2018) 68:394–424. doi: 10.3322/caac.21492
2. Liu W, Liu J, Song Y, Zeng X, Wang X, Mi L, et al. Burden of lymphoma in China, 2006-2016: an analysis of the global burden of disease study 2016. J Hematol Oncol (2019) 12:115. doi: 10.1186/s13045-019-0785-7
3. Shi Y, Han Y, Yang J, Liu P, He X, Zhang C, et al. Clinical features and outcomes of diffuse large b-cell lymphoma based on nodal or extranodal primary sites of origin: Analysis of 1,085 WHO classified cases in a single institution in China. Chin J Cancer Res (2019) 31:152–61. doi: 10.21147/j.issn.1000-9604.2019.01.10
4. Aide N, Fruchart C, Nganoa C, Gac AC, Lasnon C. Baseline (18)F-FDG PET radiomic features as predictors of 2-year event-free survival in diffuse large b cell lymphomas treated with immunochemotherapy. Eur radiology. (2020) 30:4623–32. doi: 10.1007/s00330-020-06815-8
5. Nowakowski GS, Blum KA, Kahl BS, Friedberg JW, Baizer L, Little RF, et al. Beyond RCHOP: A blueprint for diffuse Large b cell lymphoma research. J Natl Cancer Institute (2016) 108–16. doi: 10.1093/jnci/djw257
6. Gleeson M, Counsell N, Cunningham D, Lawrie A, Clifton-Hadley L, Hawkes E, et al. Prognostic indices in diffuse large b-cell lymphoma in the rituximab era: an analysis of the UK national cancer research institute r-CHOP 14 versus 21 phase 3 trial. Br J Haematol (2021) 192:1015–9. doi: 10.1111/bjh.16691
7. Sehn LH, Berry B, Chhanabhai M, Fitzgerald C, Gill K, Hoskins P, et al. The revised international prognostic index (R-IPI) is a better predictor of outcome than the standard IPI for patients with diffuse large b-cell lymphoma treated with r-CHOP. Blood (2007) 109:1857–61. doi: 10.1182/blood-2006-08-038257
8. Lue KH, Wu YF, Lin HH, Hsieh TC, Liu SH, Chan SC, et al. Prognostic value of baseline radiomic features of (18)F-FDG PET in patients with diffuse Large b-cell lymphoma. Diagnostics (Basel Switzerland) (2020) 11–23. doi: 10.3390/diagnostics11010036
9. Chen Y, Zhou M, Liu J, Huang G. Prognostic value of bone marrow FDG uptake pattern of PET/CT in newly diagnosed diffuse Large b-cell lymphoma. J Cancer. (2018) 9:1231–8. doi: 10.7150/jca.23714
10. Ollila TA, Olszewski AJ. Extranodal diffuse Large b cell lymphoma: Molecular features, prognosis, and risk of central nervous system recurrence. Curr Treat options Oncol (2018) 19:38. doi: 10.1007/s11864-018-0555-8
11. Takeda K, Takanami K, Shirata Y, Yamamoto T, Takahashi N, Ito K, et al. Clinical utility of texture analysis of 18F-FDG PET/CT in patients with stage I lung cancer treated with stereotactic body radiotherapy. J Radiat Res (2017) 58:862–9. doi: 10.1093/jrr/rrx050
12. Zhou Y, Ma XL, Pu LT, Zhou RF, Ou XJ, Tian R, et al. Prediction of overall survival and progression-free survival by the (18)F-FDG PET/CT radiomic features in patients with primary gastric diffuse Large b-cell lymphoma. Contrast media Mol imaging. (2019) 2019:5963607. doi: 10.1155/2019/5963607
13. Xie M, Wu K, Liu Y, Jiang Q, Xie Y. Predictive value of f-18 FDG PET/CT quantization parameters in diffuse large b cell lymphoma: A meta-analysis with 702 participants. Med Oncol (Northwood London England). (2015) 32:446. doi: 10.1007/s12032-014-0446-1
14. Zhang X, Chen L, Jiang H, He X, Feng L, Ni M, et al. A novel analytic approach for outcome prediction in diffuse large b-cell lymphoma by [(18)F]FDG PET/CT. Eur J Nucl Med Mol imaging. (2022) 49:1298–310. doi: 10.1007/s00259-021-05572-0
15. Cui XW, Hocke M, Jenssen C, Ignee A, Klein S, Schreiber-Dietrich D, et al. Conventional ultrasound for lymph node evaluation, update 2013. Z Gastroenterol (2014) 52:212–21. doi: 10.1055/s-0033-1356153
16. Dissaux G, Visvikis D, Da-Ano R, Pradier O, Chajon E, Barillot I, et al. Pretreatment (18)F-FDG PET/CT radiomics predict local recurrence in patients treated with stereotactic body radiotherapy for early-stage non-small cell lung cancer: A multicentric study. J Nucl Med (2020) 61:814–20. doi: 10.2967/jnumed.119.228106
17. Jiang Y, Yuan Q, Lv W, Xi S, Huang W, Sun Z, et al. Radiomic signature of (18)F fluorodeoxyglucose PET/CT for prediction of gastric cancer survival and chemotherapeutic benefits. Theranostics (2018) 8:5915–28. doi: 10.7150/thno.28018
18. Zhou Y, Su GY, Hu H, Ge YQ, Si Y, Shen MP, et al. Radiomics analysis of dual-energy CT-derived iodine maps for diagnosing metastatic cervical lymph nodes in patients with papillary thyroid cancer. Eur radiology. (2020) 30:6251–62. doi: 10.1007/s00330-020-06866-x
19. Peng H, Dong D, Fang MJ, Li L, Tang LL, Chen L, et al. Prognostic value of deep learning PET/CT-based radiomics: Potential role for future individual induction chemotherapy in advanced nasopharyngeal carcinoma. Clin Cancer Res (2019) 25:4271–9. doi: 10.1158/1078-0432.Ccr-18-3065
20. Forgacs A, Pall Jonsson H, Dahlbom M, Daver F, DiFranco MD, Opposits G, et al. A study on the basic criteria for selecting heterogeneity parameters of F18-FDG PET images. PloS One (2016) 11:e0164113. doi: 10.1371/journal.pone.0164113
21. Zhou Y, Zhu Y, Chen Z, Li J, Sang S, Deng S, et al. Radiomic features of (18)F-FDG PET in Hodgkin lymphoma are predictive of outcomes. Contrast media Mol imaging. (2021) 2021:6347404. doi: 10.1155/2021/6347404
22. de Jesus FM, Yin Y, Mantzorou-Kyriaki E, Kahle XU, de Haas RJ, Yakar D, et al. Machine learning in the differentiation of follicular lymphoma from diffuse large b-cell lymphoma with radiomic [(18)F]FDG PET/CT features. Eur J Nucl Med Mol imaging. (2022) 49:1535–43. doi: 10.1007/s00259-021-05626-3
23. Lu W, Zhong L, Dong D, Fang M, Dai Q, Leng S, et al. Radiomic analysis for preoperative prediction of cervical lymph node metastasis in patients with papillary thyroid carcinoma. Eur J Radiol (2019) 118:231–8. doi: 10.1016/j.ejrad.2019.07.018
24. Jiang M, Li C, Tang S, Lv W, Yi A, Wang B, et al. Nomogram based on shear-wave elastography radiomics can improve preoperative cervical lymph node staging for papillary thyroid carcinoma. Thyroid (2020) 30:885–97. doi: 10.1089/thy.2019.0780
25. Conti A, Duggento A, Indovina I, Guerrisi M, Toschi N. Radiomics in breast cancer classification and prediction. Semin Cancer Biol (2021) 72:238–50. doi: 10.1016/j.semcancer.2020.04.002
26. Liu Y, Chen J, Zhang C, Li Q, Zhou H, Zeng Y, et al. Ultrasound-based radiomics can classify the etiology of cervical lymphadenopathy: A multi-center retrospective study. Front Oncol (2022) 12:856605. doi: 10.3389/fonc.2022.856605
27. Coskun N, Okudan B, Uncu D, Kitapci MT. Baseline 18F-FDG PET textural features as predictors of response to chemotherapy in diffuse large b-cell lymphoma. Nucl Med Commun (2021) 42:1227–32. doi: 10.1097/mnm.0000000000001447
28. Giovagnorio F, Galluzzo M, Andreoli C, De CM, David V. Color Doppler sonography in the evaluation of superficial lymphomatous lymph nodes. J Ultrasound Med (2002) 21:403–8. doi: 10.7863/jum.2002.21.4.403
29. Yu Y, Tan Y, Xie C, Hu Q, Ouyang J, Chen Y, et al. Development and validation of a preoperative magnetic resonance imaging radiomics-based signature to predict axillary lymph node metastasis and disease-free survival in patients with early-stage breast cancer. JAMA Netw Open (2020) 3:e2028086. doi: 10.1001/jamanetworkopen.2020.28086
30. Sun J, Yang Q, Lu Z, He M, Gao L, Zhu M, et al. Distribution of lymphoid neoplasms in China: analysis of 4,638 cases according to the world health organization classification. Am J Clin Pathol (2012) 138:429–34. doi: 10.1309/ajcp7yltqpusdq5c
31. Aoki R, Karube K, Sugita Y, Nomura Y, Shimizu K, Kimura Y, et al. Distribution of malignant lymphoma in Japan: analysis of 2260 cases, 2001-2006. Pathol Int (2008) 58:174–82. doi: 10.1111/j.1440-1827.2007.02207.x
32. Xia Y, Huang S, Wang Y, Lei D, Wang Y, Yang H, et al. Prognostication of primary tumor location in early-stage nodal diffuse Large b-cell lymphoma: An analysis of the SEER database. Cancers (Basel) (2021) 13(2):3954. doi: 10.3390/cancers13163954
33. Colomo L, López-Guillermo A, Perales M, Rives S, Martínez A, Bosch F, et al. Clinical impact of the differentiation profile assessed by immunophenotyping in patients with diffuse large b-cell lymphoma. Blood (2003) 101:78–84. doi: 10.1182/blood-2002-04-1286
34. Song MK, Chung JS, Sung-Yong O, Lee GW, Kim SG, Seol YM, et al. Clinical impact of bulky mass in the patient with primary extranodal diffuse large b cell lymphoma treated with r-CHOP therapy. Ann Hematol (2010) 89:985–91. doi: 10.1007/s00277-010-0964-7
35. Castillo JJ, Winer ES, Olszewski AJ. Sites of extranodal involvement are prognostic in patients with diffuse large b-cell lymphoma in the rituximab era: an analysis of the surveillance, epidemiology and end results database. Am J Hematol (2014) 89:310–4. doi: 10.1002/ajh.23638
36. Dlouhy I, Karube K, Enjuanes A, Salaverria I, Nadeu F, Ramis-Zaldivar JE, et al. Revised international prognostic index and genetic alterations are associated with early failure to r-CHOP in patients with diffuse large b-cell lymphoma. Br J Haematol (2022) 196:589–98. doi: 10.1111/bjh.17858
37. Akyurek N, Uner A, Benekli M, Barista I. Prognostic significance of MYC, BCL2, and BCL6 rearrangements in patients with diffuse large b-cell lymphoma treated with cyclophosphamide, doxorubicin, vincristine, and prednisone plus rituximab. Cancer (2012) 118:4173–83. doi: 10.1002/cncr.27396
38. Hu S, Xu-Monette ZY, Tzankov A, Green T, Wu L, Balasubramanyam A, et al. MYC/BCL2 protein coexpression contributes to the inferior survival of activated b-cell subtype of diffuse large b-cell lymphoma and demonstrates high-risk gene expression signatures: a report from the international DLBCL rituximab-CHOP consortium program. Blood (2013) 121:4021–4031; quiz 4250. doi: 10.1182/blood-2012-10-460063
39. Li M, Zhang QL, Zhao W, Huang X, Gong LP, Shi Q, et al. [The incidence of high-grade b-cell lymphoma with MYC and BCL2 and/or BCL6 rearrangements in diffuse large b-cell lymphoma]. Zhonghua Xue Ye Xue Za Zhi. (2021) 42:124–8. doi: 10.3760/cma.j.issn.0253-2727.2021.02.006
40. Youk JH, Kwak JY, Lee E, Son EJ, Kim JA. Grayscale ultrasound radiomic features and shear-wave elastography radiomic features in benign and malignant breast masses. Ultraschall der Med (Stuttgart Germany 1980). (2020) 41:390–6. doi: 10.1055/a-0917-6825
41. Trenker C, Neesse A, Görg C. Sonographic patterns of renal lymphoma in b-mode imaging and in contrast-enhanced ultrasound (CEUS)–a retrospective evaluation. Eur J Radiol (2015) 84:807–10. doi: 10.1016/j.ejrad.2014.12.027
42. Rettenbacher T. Sonography of peripheral lymph nodes part 2: Doppler criteria and typical findings of distinct entities. Ultraschall der Med (Stuttgart Germany 1980). (2014) 35:10–27; quiz 28-32. doi: 10.1055/s-0033-1355593
43. McKay MJ, Taubman KL, Lee S, Scott AM. Radiotherapy planning of lymphomas: role of metabolic imaging with PET/CT. Ann Nucl Med (2022) 36:162–71. doi: 10.1007/s12149-021-01703-7
44. Eertink JJ, van de Brug T, Wiegers SE, Zwezerijnen GJC, Pfaehler EAG, Lugtenburg PJ, et al. (18)F-FDG PET baseline radiomics features improve the prediction of treatment outcome in diffuse large b-cell lymphoma. Eur J Nucl Med Mol imaging. (2022) 49:932–42. doi: 10.1007/s00259-021-05480-3
45. Parvez A, Tau N, Hussey D, Maganti M, Metser U. (18)F-FDG PET/CT metabolic tumor parameters and radiomics features in aggressive non-hodgkin's lymphoma as predictors of treatment outcome and survival. Ann Nucl Med (2018) 32:410–6. doi: 10.1007/s12149-018-1260-1
46. Aide N, Talbot M, Fruchart C, Damaj G, Lasnon C. Diagnostic and prognostic value of baseline FDG PET/CT skeletal textural features in diffuse large b cell lymphoma. Eur J Nucl Med Mol imaging. (2018) 45:699–711. doi: 10.1007/s00259-017-3899-6
Keywords: diffuse large B-cell lymphoma, overall survival, radiomic, nomograms, predict
Citation: Deng H, Zhou Y, Lu W, Chen W, Yuan Y, Li L, Shu H, Zhang P and Ye X (2022) Development and validation of nomograms by radiomic features on ultrasound imaging for predicting overall survival in patients with primary nodal diffuse large B-cell lymphoma. Front. Oncol. 12:991948. doi: 10.3389/fonc.2022.991948
Received: 12 July 2022; Accepted: 14 November 2022;
Published: 07 December 2022.
Edited by:
Jiansong Ji, Lishui Central Hospital, ChinaReviewed by:
Ming Xu, The First Affiliated Hospital of Sun Yat-sen University, ChinaLian-Ming Wu, Shanghai Jiao Tong University, China
Copyright © 2022 Deng, Zhou, Lu, Chen, Yuan, Li, Shu, Zhang and Ye. This is an open-access article distributed under the terms of the Creative Commons Attribution License (CC BY). The use, distribution or reproduction in other forums is permitted, provided the original author(s) and the copyright owner(s) are credited and that the original publication in this journal is cited, in accordance with accepted academic practice. No use, distribution or reproduction is permitted which does not comply with these terms.
*Correspondence: Xinhua Ye, ultrasoundye@163.com; Pingyang Zhang, zhpy28@126.com
†These authors have contributed equally to this work and share first authorship