- 1Department of Radiology, Chung-Ang University Hospital, Chung-Ang University College of Medicine, Seoul, South Korea
- 2Division of Cardiology, Department of Internal Medicine, Yonsei University College of Medicine, Seoul, South Korea
- 3Department of Biomedical Systems Informatics, Yonsei University College of Medicine, Seoul, South Korea
- 4Department of Radiation Oncology, Yonsei Cancer Center, Yonsei University College of Medicine, Seoul, South Korea
This study aimed to demonstrate clinical feasibility of deep learning (DL)-based fully automated coronary artery calcium (CAC) scoring software using non-electrocardiogram (ECG)-gated chest computed tomography (CT) from patients with cancer. Overall, 913 patients with colorectal or gastric cancer who underwent non-contrast-enhanced chest CT between 2013 and 2015 were included. Agatston scores obtained by manual segmentation of CAC on chest CT were used as reference. Reliability of automated CAC score acquisition was evaluated using intraclass correlation coefficients (ICCs). The agreement for cardiovascular disease (CVD) risk stratification was assessed with linearly weighted k statistics. ICCs between the manual and automated CAC scores were 0.992 (95% CI, 0.991 and 0.993, p<0.001) for total Agatston scores, 0.863 (95% CI, 0.844 and 0.880, p<0.001) for the left main, 0.964 (95% CI, 0.959 and 0.968, p<0.001) for the left anterior descending, 0.962 (95% CI, 0.956 and 0.966, p<0.001) for the left circumflex, and 0.980 (95% CI, 0.978 and 0.983, p<0.001) for the right coronary arteries. The agreement for cardiovascular risk was excellent (k=0.946, p<0.001). Current DL-based automated CAC software showed excellent reliability for Agatston score and CVD risk stratification using non-ECG gated CT scans and might allow the identification of high-risk cancer patients for CVD.
Introduction
The coronary artery calcium (CAC) score has emerged as a useful imaging biomarker for predicting the risk of major cardiovascular events (1). Recent advances in dedicated software that can automatically detect and quantify coronary artery calcification have led to wider clinical application of CAC (2, 3). Traditionally, the CAC score is calculated on electrocardiogram (ECG)-gated CAC-scoring computed tomography (CT); however, studies on lung cancer screening, such as the Dutch-Belgian randomized lung cancer screening trial and the National Lung Screening Trial, have shown a significant association between the CAC assessed on low-dose chest CT and clinical outcome (4, 5). Additionally, recent studies have shown that CAC scores obtained from non-ECG-gated CT are significantly correlated with those obtained from ECG-gated CAC-scoring CT (6–8). These results provide strong evidence for the potential application of CAC scores assessed using non-ECG-gated chest CT in various clinical scenarios.
In particular, CAC assessment could have additional clinical value in patients with cancer. Indeed, the number of cancer survivors is increasing, and cardiovascular disease (CVD) is one of the leading causes of morbidity and mortality in patients with various cancers (9, 10). Most patients with cancer undergo longitudinal CT follow-up for cancer surveillance; however, CAC assessment from non-ECG-gated chest CTs and its clinical application in cancer patients are challenging in real-world practice. As detection of tiny pulmonary metastasis or primary cancer among vast volume of chest CT images is of great importance in patients with cancers, CAC lesions are easily overlooked from non-ECG gated CT scans. We believe that the application of deep learning algorithm-based automated CAC scoring software to non-ECG-gated chest CTs, especially those from patients with cancer, could have a great clinical impact on patient management and prognostication.
Thus, the aim of this study was to validate the recently released deep learning algorithm-based CAC scoring software for non-ECG-gated chest CT scans from patients with cancer and demonstrate its reliability and clinical applicability in a real-world setting.
Materials and methods
The study was performed in accordance with relevant guidelines and regulations and complied with the Declaration of Helsinki. This study was approved by the institutional review board of Chung-Ang University Hospital. Due to the retrospective nature of the study, the need for patient consent for the use of clinical data was waived by the institutional review board of Chung-Ang University Hospital.
Study population
We identified 975 patients diagnosed with colorectal or gastric cancer and performed baseline chest CT scans, including non-contrast-enhanced CT images, between 2013 and 2015 at our institution. Of the 975 patients, 61 were excluded due to the following reasons: five for poor image quality; 53 for previous coronary stent insertion; one for previous coronary artery bypass graft; three for having cardiac implantable electronic devices. Finally, 913 patients (633 men and 280 women; median age, 68.3 years) were included in the analysis (Figure 1).
Reference calcium scoring
For reference CAC score determination, non-enhanced chest CT images were analyzed for the presence and extent of CAC according to the Agatston method (11) using dedicated software (Aquarius Workstation, TeraRecon, Inc., San Mateo, CA, USA) by two experienced radiology technologists with at least seven years of experience in CAC scoring. The software overlays CAC lesions having more than 130 Hounsfield units with colors, and the observers manually labeled the CAC lesions according to their anatomical locations (i.e., left main [LM], left anterior descending artery [LAD], left circumflex artery [LCx], and right coronary artery [RCA]) by visually confirming each CAC lesion.
Automated calcium scoring with software
The automated CAC score was calculated using a commercially available deep-learning-based automatic software (AVIEW CAC, Coreline Soft, Co. Mapo-gu, Seoul, Republic of Korea). The software is an atlas-based CAC acquisition tool, empowered by DL technology (2). For development of the software, the spatial information of coronary and non-coronary regions manually labeled on coronary CT angiography was transfer to non-enhanced CAC scoring CT images using image registration. Then, DL algorithm was developed based on a 3-dimensional U-net architecture for segmentation of coronary and non-coronary regions on CAC scoring CTs (2, 12, 13). Calcium was detected when the potential lesion was in contact with the coronary region, and it did not belong to other structures. The software automatically calculated the CAC score from the uploaded CT images, and the results could be downloaded without the need to open the CT images. In addition, the automatically labeled mask for each CAC lesion was saved by the software.
Per-lesion comparisons
For cases showing substantial differences in CAC scores (outside the 95% limits of agreement [LOA] on the Bland–Altman analyses), a direct comparison of the measured coronary calcium between the manual and fully automated methods was performed by a cardiovascular radiologist (C.M.J., eight years of experience). The cause and location of each mismatched lesion were analyzed. Then, the mismatched lesions were classified into specific categories, namely, mislabeling of the coronary artery (i.e., LAD calcification labeled as LM), false-positive findings (i.e., aortic wall or valve calcification labeled as CAC), and false-negative findings (i.e., missed CAC on the fully automated method).
Statistical analyses
The reliability and correlation of the Agatston scores obtained by the fully automated method in comparison with the manual acquisition were evaluated using the intraclass correlation coefficient (ICC), and the Spearman correlation coefficient, respectively. and their agreement was assessed by examining Bland–Altman plots with 95% LOA. Interobserver variability for manual measurements was also assessed using an ICC. An ICC ≤ 0.40 was designated as poor, 0.41–0.60 as moderate, 0.61–0.80 as good, and ≥ 0.81 as excellent agreement (14). In addition, participants were classified into four commonly used CVD risk groups based on Agatston scores as follows: 0 (absent), 0 < CAC ≤ 100 (low), 100 < CAC ≤ 400 (intermediate), and 400 ≤ CAC (high) (15). The reliability of the classification was assessed using the Cohen linearly weighted k statistics, and agreement was assessed using the proportion of participants assigned to the same category by manual and automatic scoring. Values of 0–0.20 were considered as slight, 0.21–0.40 as fair, 0.41–0.60 as moderate, 0.61–0.80 as substantial, and 0.81–1.00 as almost-perfect agreement (16). Statistical analyses were performed using MedCalc software version 19.1.3 (MedCalc Software, Ltd., Ostend, Belgium) and Statistical Package for the Social Sciences version 26 (IBM Corp. Armonk, NY, USA). Statistical significance was set at p-value < 0.05.
Results
Baseline patient characteristics and CT acquisition parameters
The baseline patient characteristics are summarized in Table 1. All CT scans were obtained from patients with colorectal (n=446) or stomach cancer (n=467) for the evaluation of pulmonary metastasis. CT scans were obtained using 256-channel detector CT in 50.6% (462/913), 64-channel detector CT in 38.8% (354/913), and fewer than 64 channels in 10.6% (97/913). All CT images were reconstructed with a soft tissue kernel, and 98.7% (901/913) of the CT scans were obtained at 120 kVp. Regarding slice thickness, 514 of 913 (59.7%) CT scans were reconstructed with a 3-mm slice thickness, 212 of 913 (23.2%) CT scans were reconstructed with a 2.5-mm slice thickness, and 120 of 913 (13.1%) were reconstructed with a 3.75-mm slice thickness.
Reliability of the Agatston score measurement
For the Agatston score measurement, the ICC of the total score (per-patient analysis) was 0.992 (95% confidence interval [CI], 0.991 and 0.993, p<0.001) between the manual and fully automated methods. On per-vessel analysis, the ICC was 0.863 (95% CI, 0.844 and 0.880, p<0001) for the LM, 0.964 (95% CI, 0.959 and 0.968, p<0.001) for the LAD, 0.962 (95% CI, 0.956 and 0.966, p<0.001) for the LCx, and 0.980 (95% CI, 0.978 and 0.983, p<0001) for the RCA (Table 2). In terms of correlation, the Spearman’s correlation coefficient was 0.977 (95% CI, 0.974 and 0.980, p<0.001) for total Agatston score, 0.736 (95% CI, 0.705 and 0.764, p<0.001) for LM, 0.906 (95% CI, 0.893 and 0.917, p<0.001) for LAD, 0.897 (95% CI, 0.884 and 0.909, p<0.001) for LCx, and 0.935 (95% CI, 0.927 and 0.943, p<0.001) for RCA, respectively. When analyzing agreement of scores based on CAC groups, all ICCs were excellent, with 0.912 (95% CI, 0.894–0.926, p<0.001) for the absent plus low CAC groups (CAC ≤ 100), 0.939 (95% CI, 0.923–0.952, p<0.001) for the intermediate CAC group (100 < CAC ≤ 400), and 0.856 (95% CI, 0.789–0.902, p<0.001) for the high CAC group (400 < CAC) (Supplementary Table S1).
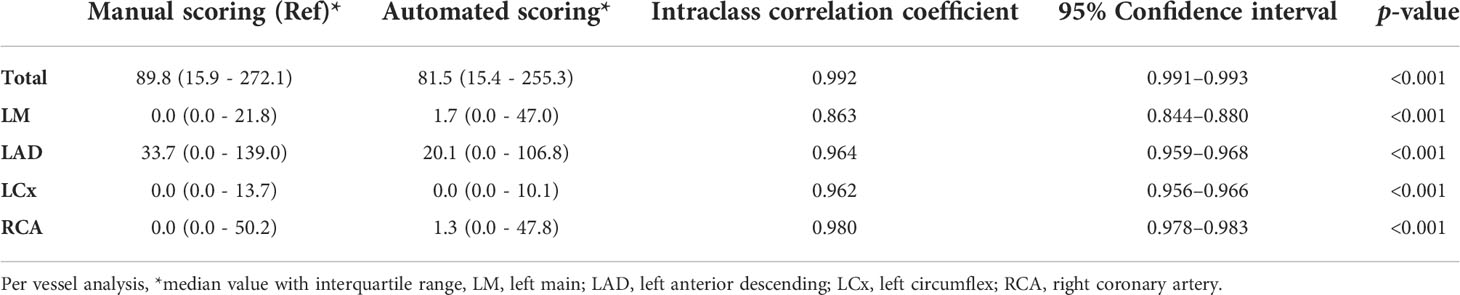
Table 2 Reliability for calculating the Agatston score using deep learning-based fully automated software and manual scoring on chest computed tomography.
Bland–Altman analyses comparing the CAC scores obtained by the manual and fully automated methods were performed (Figure 2). For the total Agatston score, 31 of 913 cases fell outside the 95% LOA. In terms of per-vessel analysis, 42 cases of LM, 33 cases of LAD, 24 cases of LCx, and 24 cases of RCA were outside the 95% LOA.
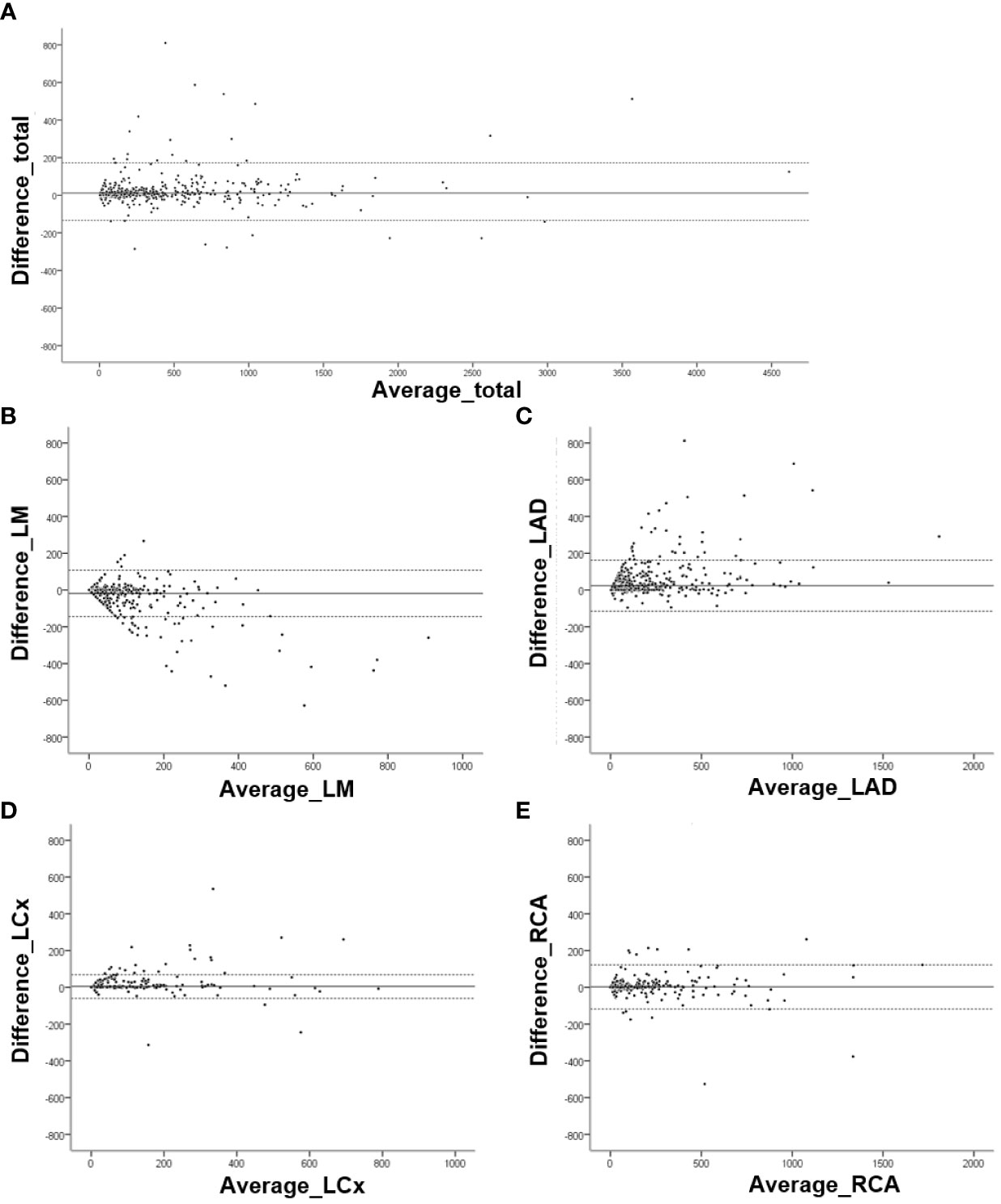
Figure 2 Bland–Altman analysis for Agatston scores obtained by manual and fully automated methods. Graphs for (A) Total Agatston score (mean difference, 12.08; 95% limits of agreement [LOA], -133.59 and 157.74), (B) left main (mean difference, -18.70; 95% LOA, -145.23 and 107.83), (C) left anterior descending (mean difference, 23.56; 95% LOA, -115.14 and 162.27), (D) left circumflex (mean difference, 4.55; 95% LOA, -59.88 and 68.97), and (E) right coronary (mean difference, 2.09; 95% LOA, 87.73 and 91.91) arteries.
The inter-reader agreement between the two readers for calculating the manual Agatston scores was excellent (ICC for the total Agatston score, 0.999; 95% CI, 0.999 and 1.000). For per-vessel agreement, the ICC was 0.997 (95% CI, 0.996 and 0.999, p<0.001) for LM, 0.999 (95% CI, 0.998 and 1.000, p<0.001) for LAD, 0.999 (95% CI, 0.999 and 1.000, p<0.001) for LCx, 0.998 (95% CI, 0.996 and 1.000, p<0.001) for RCA, respectively.
Risk category assessment
Risk category assessment was analyzed according to CAC-based risk stratification using deep learning-based fully automated software and manual scoring on chest CT. The overall reliability of the CVD risk groups was almost perfect (k=0.946, p<0.001) between the manual and fully automated scoring methods (Table 3). The majority of patients were assigned to the same CVD risk category (869/913, 95.2%), and 42 patients (4.6%) were assigned to the neighboring risk group.
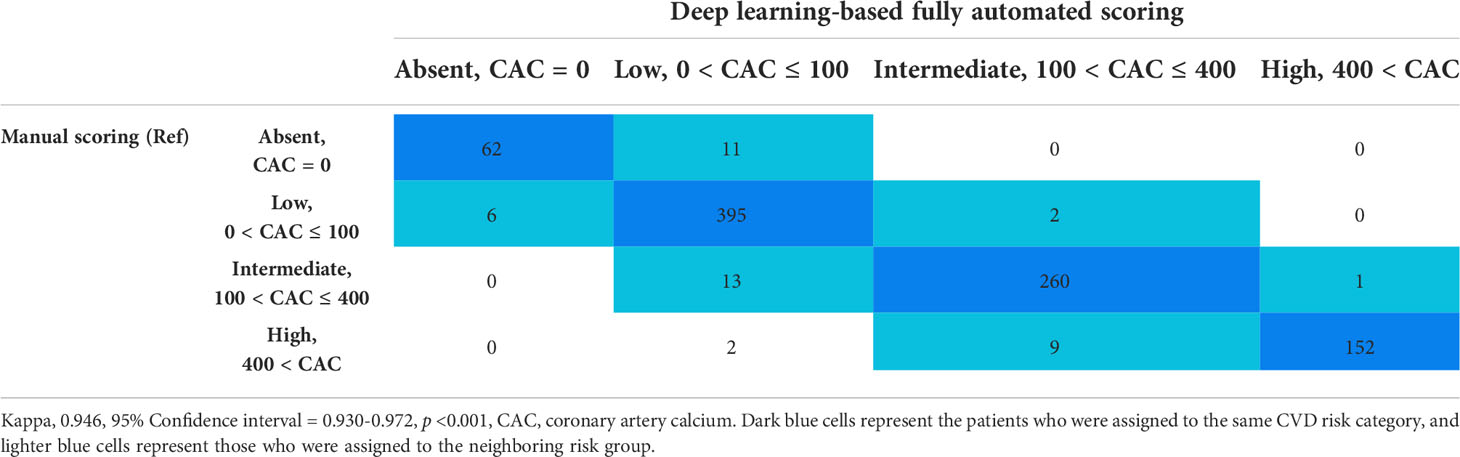
Table 3 Reliability of CAC-based risk stratification using deep learning-based fully automated software and manual scoring on chest computed tomography.
Per-lesion comparison for the cases out of 95% LOA
Per-lesion analysis was performed for cases that were out of the 95% LOA in the Bland–Altman analyses. Overall, 85 cases were reviewed (31 cases for total Agatston score, 42 cases for LM, 33 cases for LAD, 24 cases for LCX, and 24 cases for RCA), and 90 mismatched lesions were identified. Forty percent (36/90) of the mismatched lesions were classified as misnaming of the coronary artery (35 cases of LAD calcification labeled as LM and one case of LCx calcification labeled as LM). Forty out of 90 (44.4%) mismatched lesions were false-negative CACs, which were not detected on automated software, located in the LM (n=2), LAD (n=7), LCx (n=15), and RCA (n=16). False-positive findings included aortic wall or valve calcification (n = 10), mitral annular calcification (n=1), and streaky artifacts with cardiac motion (n=3) (Table 4 and Figure 3).
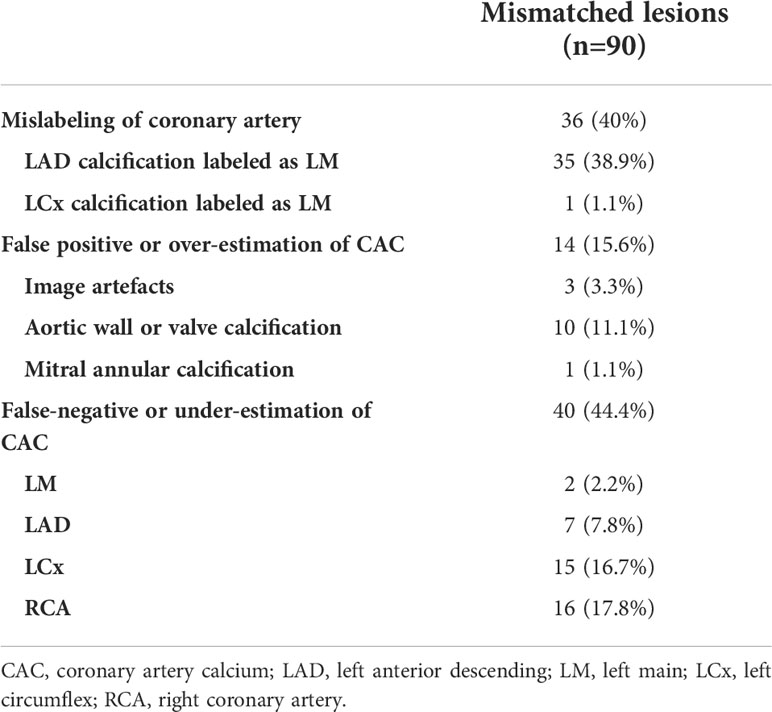
Table 4 Per-lesion analysis for the mismatched CACs in the cases outside of 95% limits of agreement on Bland–Altman analysis.
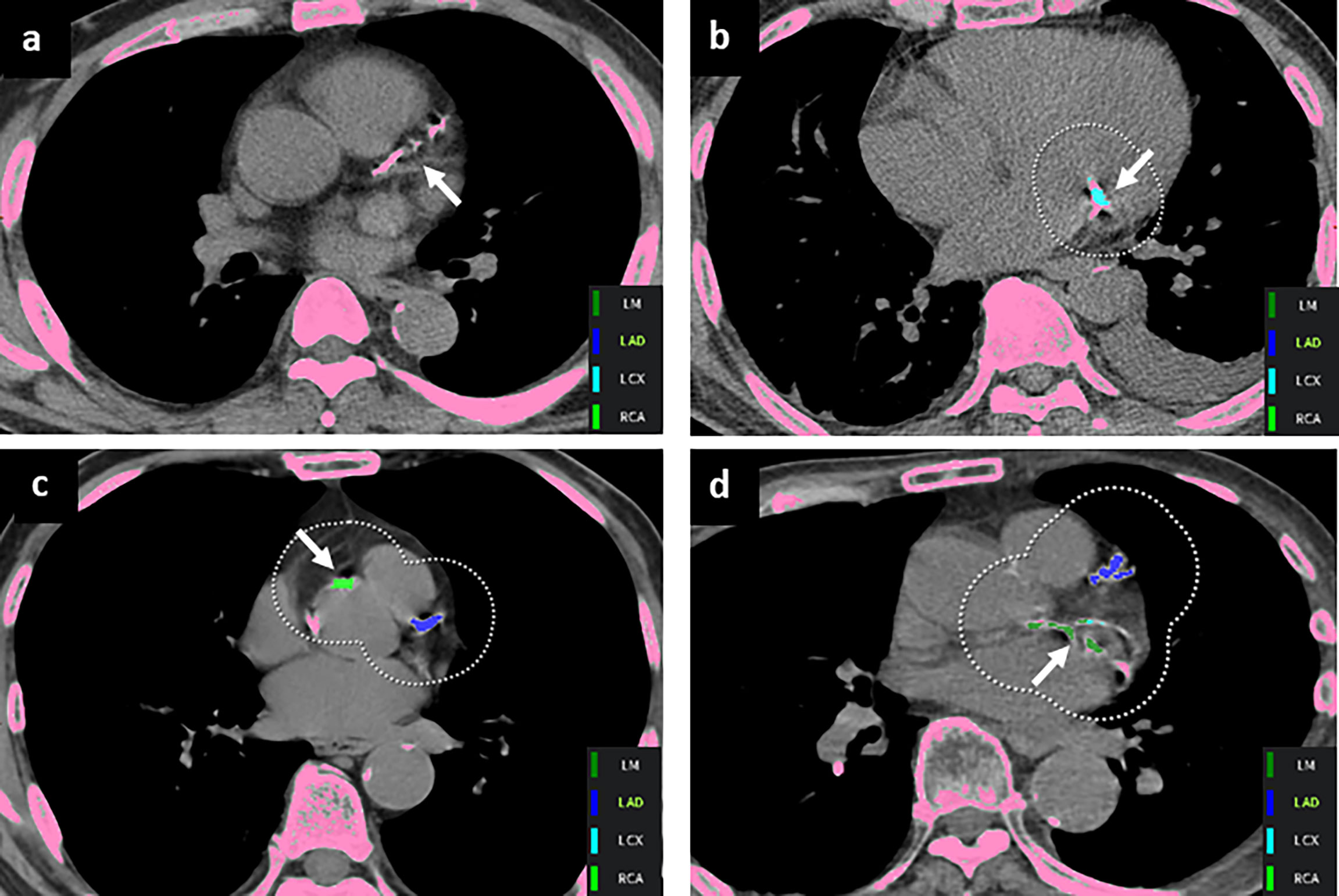
Figure 3 Representative mismatched coronary artery calcium lesions. (A) A false-negative finding was noted in the left anterior descending artery (arrow). False-positive lesions were annotated with arrows in the (B) mitral annulus and (C) aorta wall. (D) CAC on the left circumflex artery was mislabeled as the left main artery (arrow).
Discussion
Our study demonstrated the clinical feasibility of a fully automated CAC scoring software using non-ECG-gated chest CT scans in patients with gastric and colorectal cancer. Our results showed high reliability for the coronary calcium score measurement and high accuracy for the assignment of risk categories using the fully automated CAC software, currently a commercially available software, compared with conventional manual scoring systems, even with non-ECG-gated chest CTs. The current results can contribute to the wider application of CAC quantification in clinical practice, especially for patients with cancer, as majority of them routinely undergo chest CT for metastasis evaluation.
Previous studies have shown that CAC on non-ECG gated chest CT has prognostic significance (4, 17–19). However, different CAC grading methods were used in many of these studies, leading to a limited general application of the results in routine clinical practice (4, 17–20). Recently, Gal and colleagues showed a strong correlation between an automatically quantified CAC obtained by radiotherapy planning CT and the risk of CVD in patients with breast cancer (Bragatston study) (21).Their study suggested that the CAC score could serve as a surrogate marker for the timely identification of high-risk patients with CVD. To date, CAC has not been fully assessed on chest CT scans due to several factors, including the use of non-ECG-gated CT scans, time-consuming quantification processes, and the lack of generalized grading methods or quantification tools. Our study showed that deep learning-based fully automated CAC scoring software is a fast and accurate tool, potentially enabling the implementation of CVD risk-mitigating strategies in routine clinical practice.
To date, several studies have reported the excellent performance of deep learning models for automated CAC scoring on ECG-gated CT scans (2, 22, 23). Furthermore, several recent studies have demonstrated the application of automated CAC quantification models to non-ECG-gated CT scans, originally used for non-cardiac indications (3, 24). The performance of the deep learning-based automated software used in our study for CAC score acquisition and CVD risk stratification using routine chest CT scans was comparable to or even superior to that of previous studies using deep learning algorithms (3, 24).
Nevertheless, the presence of outliers was still observed. Eighty-five cases outside the 95% LOA on the per vessel analysis revealed 90 mismatched lesions. Forty of these 90 mismatched lesions were CACs that were not depicted as such by the automated software, resulting in false-negative results. In addition, an overestimation of the CAC score resulted from 14 mismatched lesions, which were subsequently found to be calcium deposits in the aortic wall, aortic valve, and mitral annulus. Anatomical errors and misnaming of the coronary arteries were also observed in 36 lesions. Further studies are warranted to eliminate the occurrence of outliers in the deep-learning algorithm.
Our study has several limitations. First, the study was retrospective and conducted at a single center. Second, the CAC was assessed using the Agatston method, and other scales, such as the volume or mass score, were not compared. Third, we did not investigate the cardiovascular outcomes of the participants; thus, the correlation between clinical outcomes and automatically calculated CAC scores could not be evaluated. Finally, we lacked ECG-gated CAC scoring CT as a reference standard. The ground-truth CAC score should be obtained from paired ECG-gated CT; however, previous studies have already shown a strong correlation between the CAC score obtained from non-ECG-gated CT and that obtained from ECG-gated CAC scoring CT (6–8). Thus, as a next step, we focused on the comparison between the manual and fully automatic methods using the same dataset. Further multicenter studies validating the use of automatic CAC scoring software with various CT images and direct comparisons with paired ECG-gated CAC scoring CT should be performed.
In conclusion, we demonstrated excellent reliability for the assessment of CAC scores and CVD risk stratification using deep learning-based fully automated software using non-ECG-gated chest CT scans in patients with cancer. A fast and accurate assessment of CAC can help in the real-world application of CAC quantification on routine chest CTs, which may provide potential opportunities for early prevention in high-risk patients.
Data availability statement
The original contributions presented in the study are included in the article/Supplementary Material. Further inquiries can be directed to the corresponding author.
Ethics statement
The studies involving human participants were reviewed and approved by the Institutional Review Board of Chung-Ang University Hospital. Written informed consent for participation was not required for this study in accordance with the national legislation and the institutional requirements.
Author contributions
Conceptualization of the study was suggested by MC, SY and IC. Data curation was done by JHC, MC, YH and HC. Writing the draft manuscript and preparing figures were done by JHC, WDK and MC. All authors reviewed and edited the manuscript.
Funding
This study was supported by a grant from the National R&D Program for Cancer Control, Ministry of Health & Welfare, Republic of Korea (HA21C0065).
Conflict of interest
The authors declare that the research was conducted in the absence of any commercial or financial relationships that could be construed as a potential conflict of interest.
Publisher’s note
All claims expressed in this article are solely those of the authors and do not necessarily represent those of their affiliated organizations, or those of the publisher, the editors and the reviewers. Any product that may be evaluated in this article, or claim that may be made by its manufacturer, is not guaranteed or endorsed by the publisher.
Supplementary material
The Supplementary Material for this article can be found online at: https://www.frontiersin.org/articles/10.3389/fonc.2022.989250/full#supplementary-material
References
1. Detrano R, Guerci AD, Carr JJ, Bild DE, Burke G, Folsom AR, et al. Coronary calcium as a predictor of coronary events in four racial or ethnic groups. N Engl J Med (2008) 358:1336–45. doi: 10.1056/NEJMoa072100
2. Lee J-G, Kim H, Kang H, Koo HJ, Kang J-W, Kim Y-H, et al. Fully automatic coronary calcium score software empowered by artificial intelligence technology: validation study using three CT cohorts. Korean J Radiol (2021) 22:1764. doi: 10.3348/kjr.2021.0148
3. van Velzen SG, Lessmann N, Velthuis BK, Bank IE, van den Bongard DH, Leiner T, et al. Deep learning for automatic calcium scoring in CT: validation using multiple cardiac CT and chest CT protocols. Radiology (2020) 295:66–79. doi: 10.1148/radiol.2020191621
4. Chiles C, Duan F, Gladish GW, Ravenel JG, Baginski SG, Snyder BS, et al. Association of coronary artery calcification and mortality in the national lung screening trial: A comparison of three scoring methods. Radiology (2015) 276:82–90. doi: 10.1148/radiol.15142062
5. Takx RA, Isgum I, Willemink MJ, van der Graaf Y, de Koning HJ, Vliegenthart R, et al. Quantification of coronary artery calcium in nongated CT to predict cardiovascular events in male lung cancer screening participants: results of the NELSON study. J Cardiovasc Comput Tomogr (2015) 9:50–7. doi: 10.1016/j.jcct.2014.11.006
6. Kim JY, Suh YJ, Han K, Choi BW. Reliability of coronary artery calcium severity assessment on non-Electrocardiogram-Gated CT: A meta-analysis. Korean J Radiol (2021) 22:1034. doi: 10.3348/kjr.2020.1047
7. Suh YJ, Lee JW, Shin SY, Goo JM, Kim Y, Yong HSJEr. Coronary artery calcium severity grading on non-ECG-gated low-dose chest computed tomography: A multiple-observer study in a nationwide lung cancer screening registry. Eur Radiol (2020) 30:3684–91. doi: 10.1007/s00330-020-06707-x
8. Budoff MJ, Nasir K, Kinney GL, Hokanson JE, Barr RG, Steiner R, et al. Coronary artery and thoracic calcium on noncontrast thoracic CT scans: comparison of ungated and gated examinations in patients from the COPD gene cohort. J Cardiovasc Comput Tomogr (2011) 5:113–8. doi: 10.1016/j.jcct.2010.11.002
9. Youn J-C, Chung W-B, Ezekowitz JA, Hong JH, Nam H, Kyoung D-S, et al. Cardiovascular disease burden in adult patients with cancer: An 11-year nationwide population-based cohort study. Int J Cardiol (2020) 317:167–73. doi: 10.1016/j.ijcard.2020.04.080
10. Sturgeon KM, Deng L, Bluethmann SM, Zhou S, Trifiletti DM, Jiang C, et al. A population-based study of cardiovascular disease mortality risk in US cancer patients. Eur Heart J (2019) 40:3889–97. doi: 10.1093/eurheartj/ehz766
11. Agatston AS, Janowitz WR, Hildner FJ, Zusmer NR, Viamonte M, Detrano RJJotACoC. Quantification of coronary artery calcium using ultrafast computed tomography. J Am Coll Cardiol (1990) 15:827–32. doi: 10.1016/0735-1097(90)90282-T
12. Sartoretti T, Gennari AG, Sartoretti E, Skawran S, Maurer A, Buechel RR, et al. Fully automated deep learning powered calcium scoring in patients undergoing myocardial perfusion imaging. J Nucl Cardiol (2022). doi: 10.1007/s12350-022-02940-7
13. Vonder M, Zheng S, Dorrius MD, van der Aalst CM, de Koning HJ, Yi J, et al. Deep Learning for Automatic Calcium Scoring in Population-Based Cardiovascular Screening. JACC Cardiovasc Imaging (2022) 15(2):366–7. doi: 10.1016/j.jcmg.2021.07.012
14. Bartko JJ. The intraclass correlation coefficient as a measure of reliability. J Psychol Rep (1966) 19:3–11. doi: 10.2466/pr0.1966.19.1.3
15. Budoff MJ, Shaw LJ, Liu ST, Weinstein SR, Tseng PH, Flores FR, et al. Long-term prognosis associated with coronary calcification: observations from a registry of 25,253 patients. J Am Coll Cardiol (2007) 49:1860–70. doi: 10.1016/j.jacc.2006.10.079
16. Fleiss JL. Measuring nominal scale agreement among many raters. J psychol Bull (1971) 76:378. doi: 10.1037/h0031619
17. Jacobs PC, Gondrie MJ, van der Graaf Y, de Koning HJ, Isgum I, van Ginneken B, et al. Coronary artery calcium can predict all-cause mortality and cardiovascular events on low-dose CT screening for lung cancer. Am J Roentgenol (2012) 198:505–11. doi: 10.2214/AJR.10.5577
18. Zhu Y, Yip R, Shemesh J, Jirapatnakul AC, Yankelevitz DF, Henschke CIJEr. Combined aortic valve and coronary artery calcifications in lung cancer screening as predictors of death from cardiovascular disease. Eur Radiol (2020) 30:6847–57. doi: 10.1007/s00330-020-07049-4
19. Shemesh J, Henschke CI, Shaham D, Yip R, Farooqi AO, Cham MD, et al. Ordinal scoring of coronary artery calcifications on low-dose CT scans of the chest is predictive of death from cardiovascular disease. Radiology (2010) 257:541–8. doi: 10.1148/radiol.10100383
20. Einstein AJ, Johnson LL, Bokhari S, Son J, Thompson RC, Bateman TM, et al. Agreement of visual estimation of coronary artery calcium from low-dose CT attenuation correction scans in hybrid PET/CT and SPECT/CT with standard agatston score. J Am Coll Cardiol (2010) 56:1914–21. doi: 10.1016/j.jacc.2010.05.057
21. Gal R, van Velzen SGM, Hooning MJ, Emaus MJ, van der Leij F, Gregorowitsch ML, et al. Identification of risk of cardiovascular disease by automatic quantification of coronary artery calcifications on radiotherapy planning CT scans in patients with breast cancer. JAMA Oncol (2021) 7:1024–32. doi: 10.1001/jamaoncol.2021.1144
22. Martin SS, van Assen M, Rapaka S, Hudson HT Jr, Fischer AM, Varga-Szemes A, et al. Evaluation of a deep learning-based automated CT coronary artery calcium scoring algorithm. JACC Cardiovasc Imaging (2021) 13:524–6. doi: 10.1016/j.ejrad.2020.109420
23. Zhang N, Yang G, Zhang W, Wang W, Zhou Z, Zhang H, et al. Fully automatic framework for comprehensive coronary artery calcium scores analysis on non-contrast cardiac-gated CT scan: Total and vessel-specific quantifications. Eur J Radiol (2021) 134:109420. doi: 10.1016/j.ejrad.2020.109420
Keywords: coronary artery calcium score (CACS), chest CT, artificial intelligence, accuracy, cancer patient, risk stratification
Citation: Choi JH, Cha MJ, Cho I, Kim WD, Ha Y, Choi H, Lee SH, You SC and Chang JS (2022) Validation of deep learning-based fully automated coronary artery calcium scoring using non-ECG-gated chest CT in patients with cancer. Front. Oncol. 12:989250. doi: 10.3389/fonc.2022.989250
Received: 08 July 2022; Accepted: 30 August 2022;
Published: 20 September 2022.
Edited by:
Rohit Moudgil, Cleveland Clinic, United StatesReviewed by:
Damian Craiem, Favaloro University, ArgentinaGerman Gonzalez, Sierra Research S.L., Spain
Copyright © 2022 Choi, Cha, Cho, Kim, Ha, Choi, Lee, You and Chang. This is an open-access article distributed under the terms of the Creative Commons Attribution License (CC BY). The use, distribution or reproduction in other forums is permitted, provided the original author(s) and the copyright owner(s) are credited and that the original publication in this journal is cited, in accordance with accepted academic practice. No use, distribution or reproduction is permitted which does not comply with these terms.
*Correspondence: Min Jae Cha, YXN0ZXJpc20zNUBuYXZlci5jb20=; Iksung Cho, aWtzdW5nY2hvLm1kQGdtYWlsLmNvbQ==