- 1Department of Hepatobiliary Surgery, Renmin Hospital of Wuhan University, Wuhan, China
- 2Department of Gastrointestinal Surgery, Tongji Hospital, Tongji Medical College in Huazhong University of Science and Technology, Wuhan, China
- 3Department of Oncology, Renmin Hospital of Wuhan University, Wuhan, China
- 4Department of Orthopedics, Renmin Hospital of Wuhan University, Wuhan, China
- 5Department of neurosurgery, Renmin Hospital of Wuhan University, Wuhan, China
Background: The goal is to establish and validate an innovative prognostic risk stratification and nomogram in patients of hepatocellular carcinoma (HCC) with microvascular invasion (MVI) for predicting the cancer-specific survival (CSS).
Methods: 1487 qualified patients were selected from the Surveillance, Epidemiology and End Results (SEER) database and randomly assigned to the training cohort and validation cohort in a ratio of 7:3. Concordance index (C-index), area under curve (AUC) and calibration plots were adopted to evaluate the discrimination and calibration of the nomogram. Decision curve analysis (DCA) was used to quantify the net benefit of the nomogram at different threshold probabilities and compare it to the American Joint Committee on Cancer (AJCC) tumor staging system. C-index, net reclassification index (NRI) and integrated discrimination improvement (IDI) were applied to evaluate the improvement of the new model over the AJCC tumor staging system. The new risk stratifications based on the nomogram and the AJCC tumor staging system were compared.
Results: Eight prognostic factors were used to construct the nomogram for HCC patients with MVI. The C-index for the training and validation cohorts was 0.785 and 0.776 respectively. The AUC values were higher than 0.7 both in the training cohort and validation cohort. The calibration plots showed good consistency between the actual observation and the nomogram prediction. The IDI values of 1-, 3-, 5-year CSS in the training cohort were 0.17, 0.16, 0.15, and in the validation cohort were 0.17, 0.17, 0.17 (P<0.05). The NRI values of the training cohort were 0.75 at 1-year, 0.68 at 3-year and 0.67 at 5-year. The DCA curves indicated that the new model more accurately predicted 1-year, 3-year, and 5-year CSS in both training and validation cohort, because it added more net benefit than the AJCC staging system. Furthermore, the risk stratification system showed the CSS in different groups had a good regional division.
Conclusions: A comprehensive risk stratification system and nomogram were established to forecast CSS for patients of HCC with MVI.
Introduction
Hepatocellular carcinoma (HCC) is the main histological type (approximately 90%) of primary hepatocellular carcinoma (1). HCC rank as the sixth most frequent malignancy and the third leading cause for cancer-related mortality worldwide (2).The 5-year survival rate for HCC is only 18% (3).
Currently, surgery is the preferred treatment for HCC, but the high postoperative recurrence and low long-term survival remain to be solved (4, 5). Most HCC patients are losing surgical treatment due to the advanced stage at the time of diagnosis. Different subtypes and molecular heterogeneities of HCC make their clinical prognosis significantly different (6). Microvascular infiltration (MVI) is mainly a nest of cancer cells seen microscopically in the lumen of the blood vessels lining the endothelium, mainly in the branches of the portal vein next to the cancer (7). Most patients with early-stage relapsed liver cancer eventually pathologically MVI positive, which is one of the key prognostic factors for recurrence after liver resection or liver transplantation in HCC patients (8–12). Further studies of pathological grading of MVI found that the higher the grouping level of MVI, the shorter the median survival of HCC patients (13, 14). At the same time, tumor morphology, diameter, and number are important factors in predicting vascular infiltration (15–17). Therefore, HCC patients with MVI require personalized predictive models. Research teams have established clinical predictive models that combine clinical features, laboratory indicators, and imaging features to more accurately predict MVI (18–22).
It should be noted that the nomogram has been extensively used to predict the prognosis of cancer patients, driving personalized medicine and helping clinicians to predict prognosis (23–25). There have been many studies on prediction models for liver cancer, but few studies have been done to construct a nomogram to predict the prognosis of HCC patients with microvascular invasion. This study aimed to establish prediction models for CSS in HCC patients with MVI and to verify their predictive performance.
Patients and methods
Patients selection and study variables
SEER*Stat 8.3.6 software was applied to extract data on patients diagnosed with hepatocellular carcinoma and microvascular infiltration from the SEER18 registry database (1975-2019) from 2010-2015 and clinically relevant data. This study used the National Cancer Institute Surveillance, Epidemiology and End Results database, which contains patient follow-up information and is updated once a year. Inclusion criteria: (a) primary hepatocellular carcinoma; (b) complete follow-up information; (c) clear cause of death. Exclusion criteria: (a) unknown tumor primary location; (b) lack of follow-up information; (c) unknown tumor infiltration; (d) unknown cause of death or death from other causes; (e) unknown tumor stage; (f) Distant metastases. The scrubbing process is shown in the flowchart.
Arrangement of patient data
The study cohort listed the clinical characteristics of hepatocellular carcinoma with microvascular infiltration and the survival characteristics of patients after diagnosis. All variables were expressed as sum of cases and percentages after inclusion of baseline characteristics. Included categories were: age, sex, race, tumor size, tumor number, pathological grade, AJCC stage, AFP value, surgery, chemotherapy, radiotherapy, cause of death classification, month of survival, and survival status. Unfortunately, the database is limited and does not contain information about hepatocirrhosis, lymph node enlargement, pseudocapsule, portal vein tumor thrombosis, HBsAg or HCVab status, ALT, AST, and GGT. Consequently, this study did not include these indicators in the study. In addition, the 7th edition AJCC-TNM staging criteria were adopted.
Construction and validation of the nomogram model
The Total cases were randomly divided into two groups in the ratio of 7:3, including a training cohort (70% of the total cases) and a validation cohort (30% of the total cases). The training cohort was employed for model construction and the validation cohort for validation. The variables of the establishment of the nomogram were screened by univariate and multivariate Cox regression analyses (P<0.05). Consistency index (C-index), subject operating characteristic curve (ROC), calibration curve and decision curve analysis (DCA) were used to validate the nomogram. The C-index was used to reflect the performance and predictive accuracy of the nomogram, while ROC represented the sensitivity and specificity of the nomogram. Calibration plots (1000 self-help weight samples) were plotted for 1, 3 and 5 years to evaluate the predictive power of the model. DCA was plotted to evaluate the clinical utility of the nomogram. In addition, NRI, C-index, IDI were used to evaluate the advantages of the new model.
A new risk stratification related to the nomogram
Based on the scoring of independent prognostic variables, this study calculated the total score for each patient. The optimal cut-off values were calculated based on the X-tile software to classify patients into low-, middle-, and high-risk groups. The ability of the traditional AJCC staging system and the new risk stratification system to identify different risk groups was compared by KM survival curves.
Statistical analysis
The data is extracted from the SEER*Stat software (version 8.3.9.2). R version 3.6.3 and related software packages was applied to data analysis. The best cut-off value for the total score was applied by X-Tile (version 3.6.1). Chi-square test was used to assess the differences of distribution of the two cohorts. When P-value is less than 0.05, it is statistically significant.
Results
Patient characteristics
1487 eligible HCC patients with MVI were included in the research (the training cohort: 1043, the validation cohort: 444) (Figure 1). 790 were male and 1297 were white. Nearly half 871 had tumors smaller than 5 cm. most 915 cases were AFP positive, 98 received radiotherapy, and 683 received chemotherapy. Table 1 summarizes the baseline demographic characteristics and features of patients with hepatocellular carcinoma combined with microvascular infiltration in the training and validation cohorts, with no differences in distribution between the two groups.
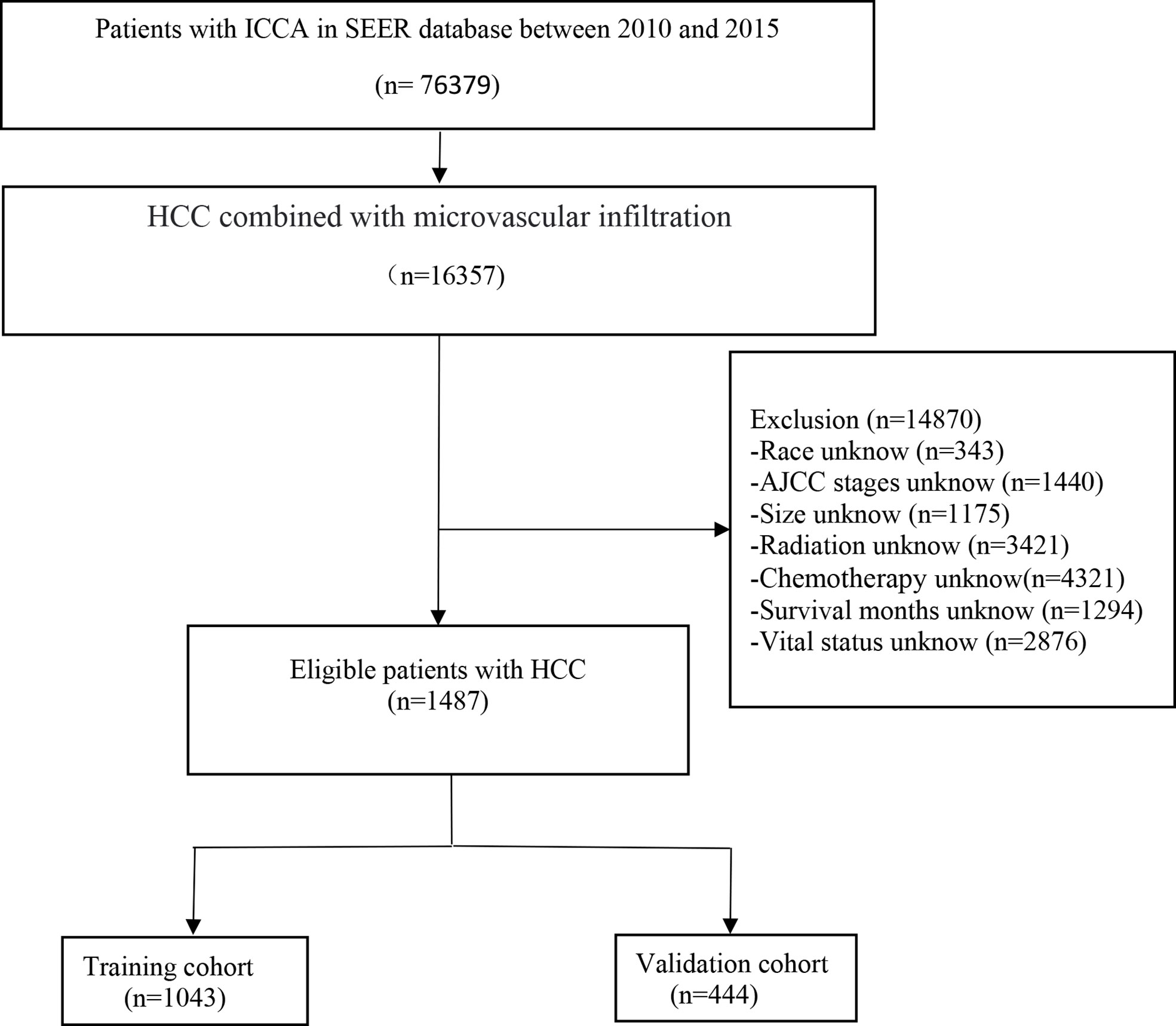
Figure 1 The flowchart of the hepatocellular carcinoma with microvascular infiltration identified in the SEER database.
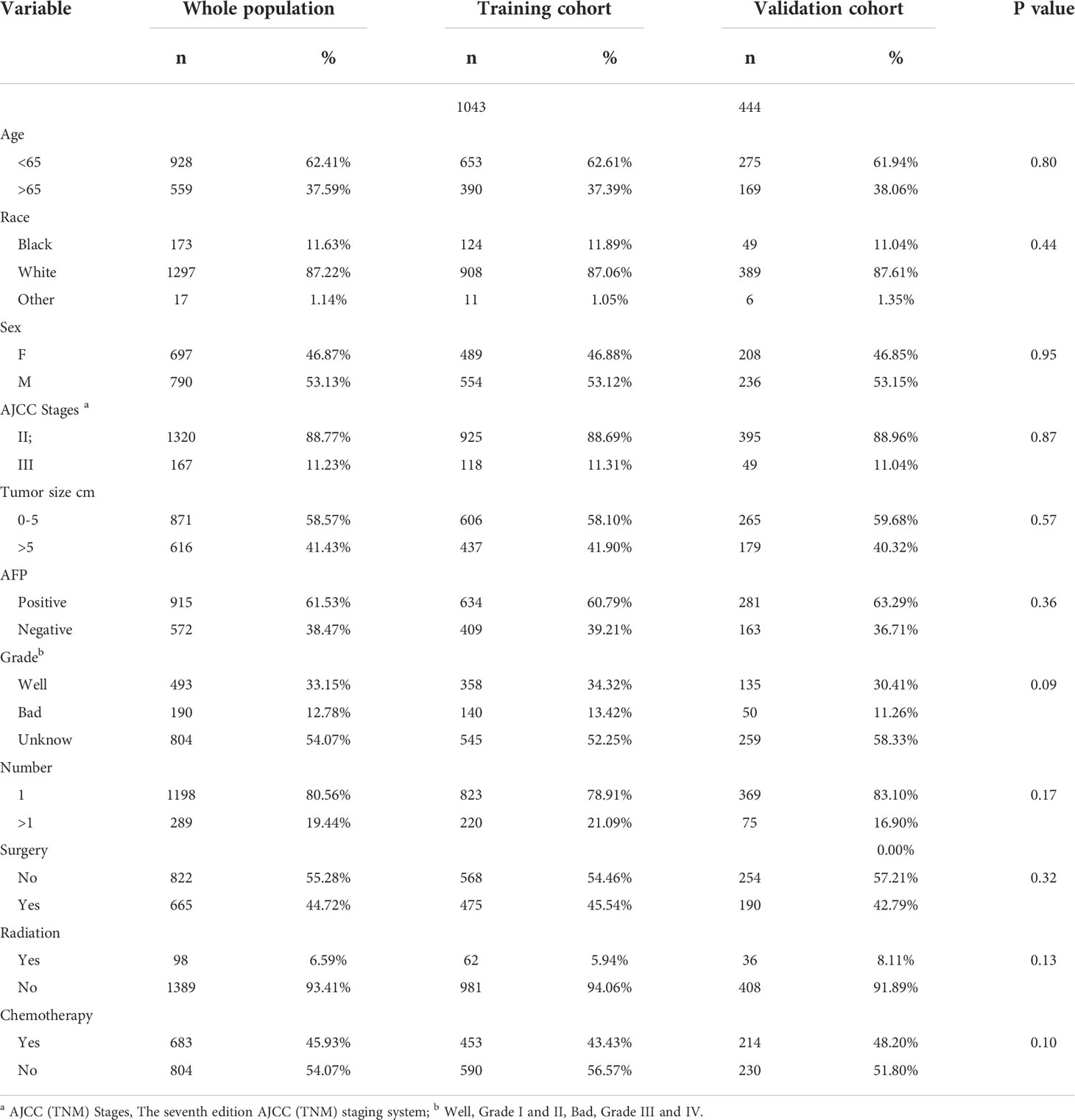
Table 1 Demographics and clinical and pathology characteristics of the patients with hepatocellular carcinoma and microvascular infiltration.
Cox regression to screen independent prognostic factors
Age, AJCC stages, pathological grade, tumor size, number, AFP, surgery, radiation and chemotherapy were significantly identified in univariate COX regression analysis (P<0.05). The multivariate analysis showed that tumor size, age, pathological grade, AFP, radiation, AJCC stages, chemotherapy and surgery (P<0.05) were independent prognostic factors for CSS which were included in the nomogram (Table 2).
Construction and validation of the nomogram
According to the above research results, the nomogram was established to predict CSS at 1, 3, and 5 years for HCC patients with MVI and was validated internally (Figure 2). The C-index for the training and validation cohorts was 0.785 (95% CI: 0.741-0.792) and 0.776 (95% CI: 0.716-0.788), respectively. The ROC, and DCA and calibration curves are shown in Figures 3–5. The ROC curve showed that the 1-, 3-, and 5-year AUC values in the training cohort were 0.759, 0.734, and 0.739, respectively. The 1-, 3-, 5-year td-AUC values in the training cohort were 0.759, 0.734, and 0.739. The td-AUC values of the validation cohort were 0.791 at 1-year, 0.759 at 3-year and 0.765 at 5-year. Furthermore, the DCA curves show good clinical application potential and better positive net benefit in the training and validation cohorts. The calibration curves agreed with the predicted CSS rates at 1, 3, and 5 years.
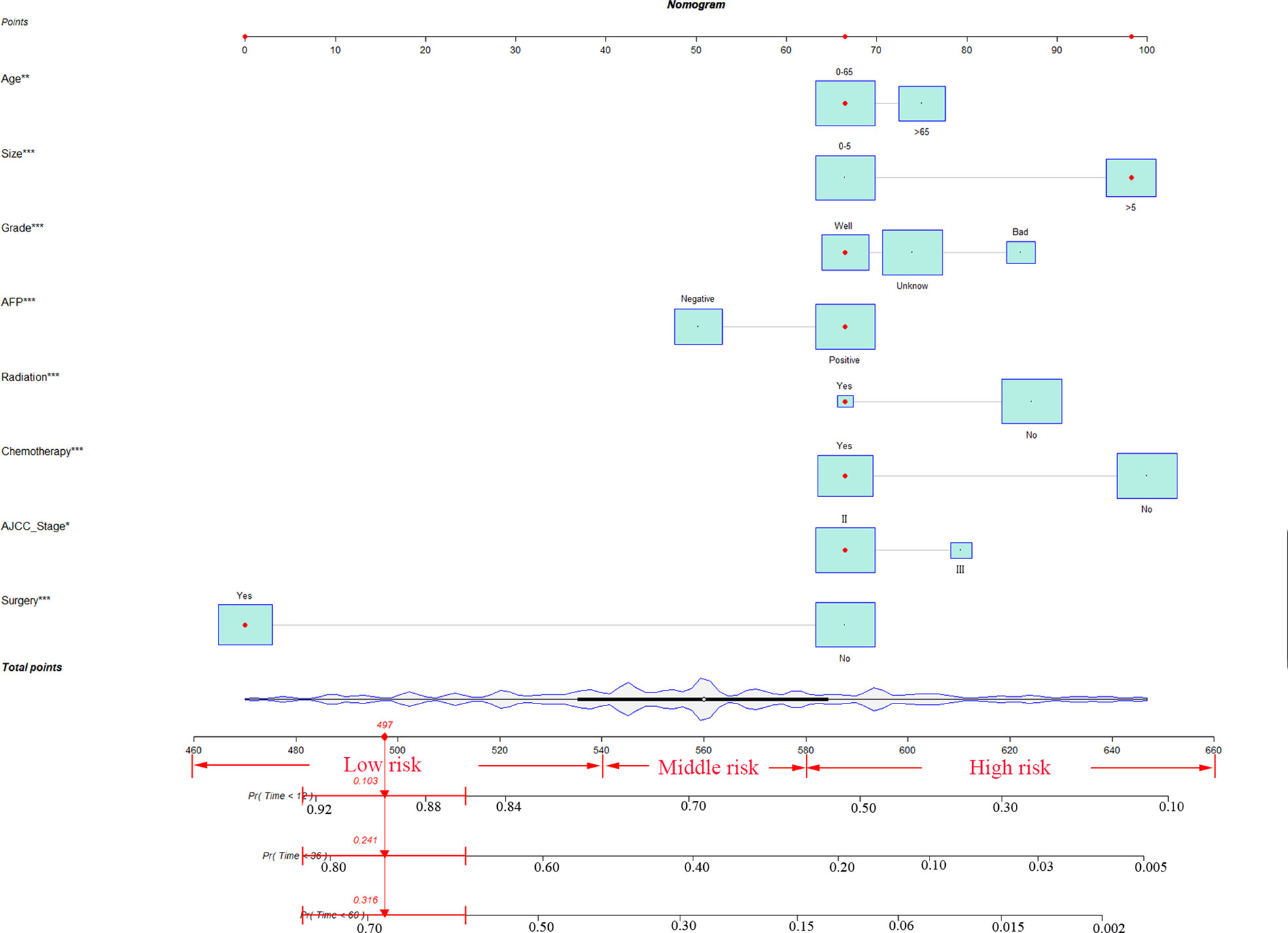
Figure 2 The nomogram for HCC patients with MVI. * P < 0.05, ** P < 0.01, *** P < 0.001, HCC, hepatocellular carcinoma; MVI, microvascular invasion.
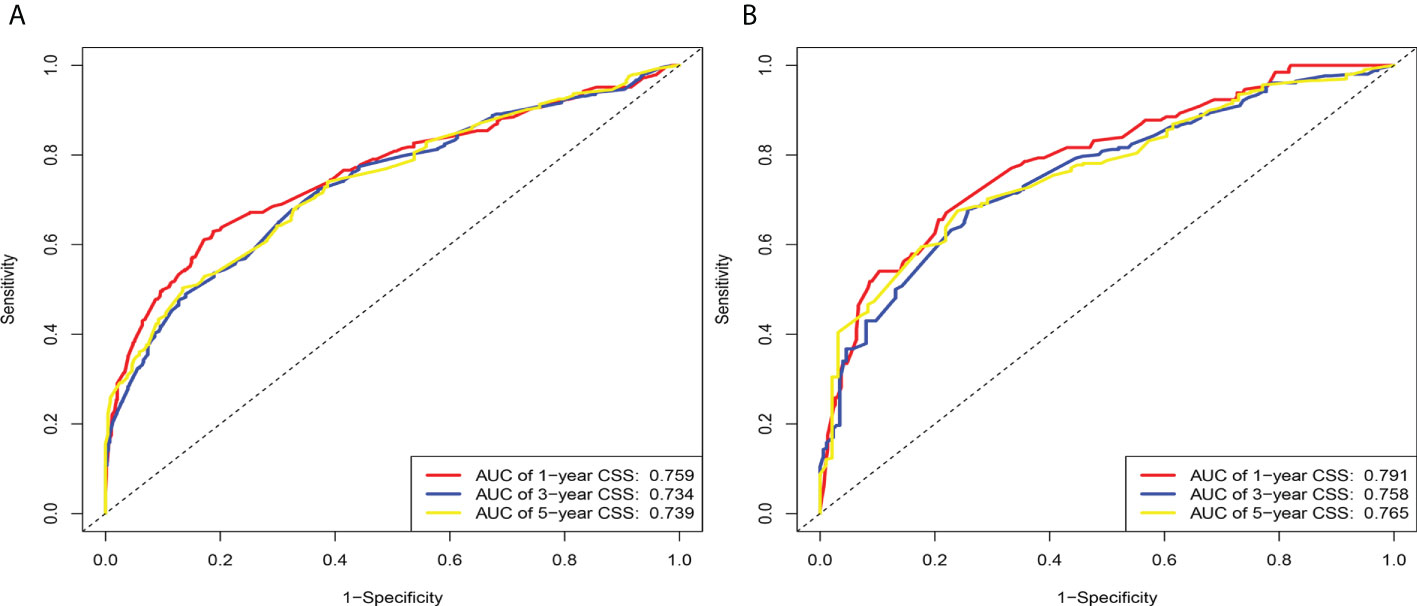
Figure 3 The area under the time-dependent receiver operating characteristic (ROC) curve (td-AUC) based on the nomogram. (A) Based on the training cohorts; (B) Based on the validation cohorts.
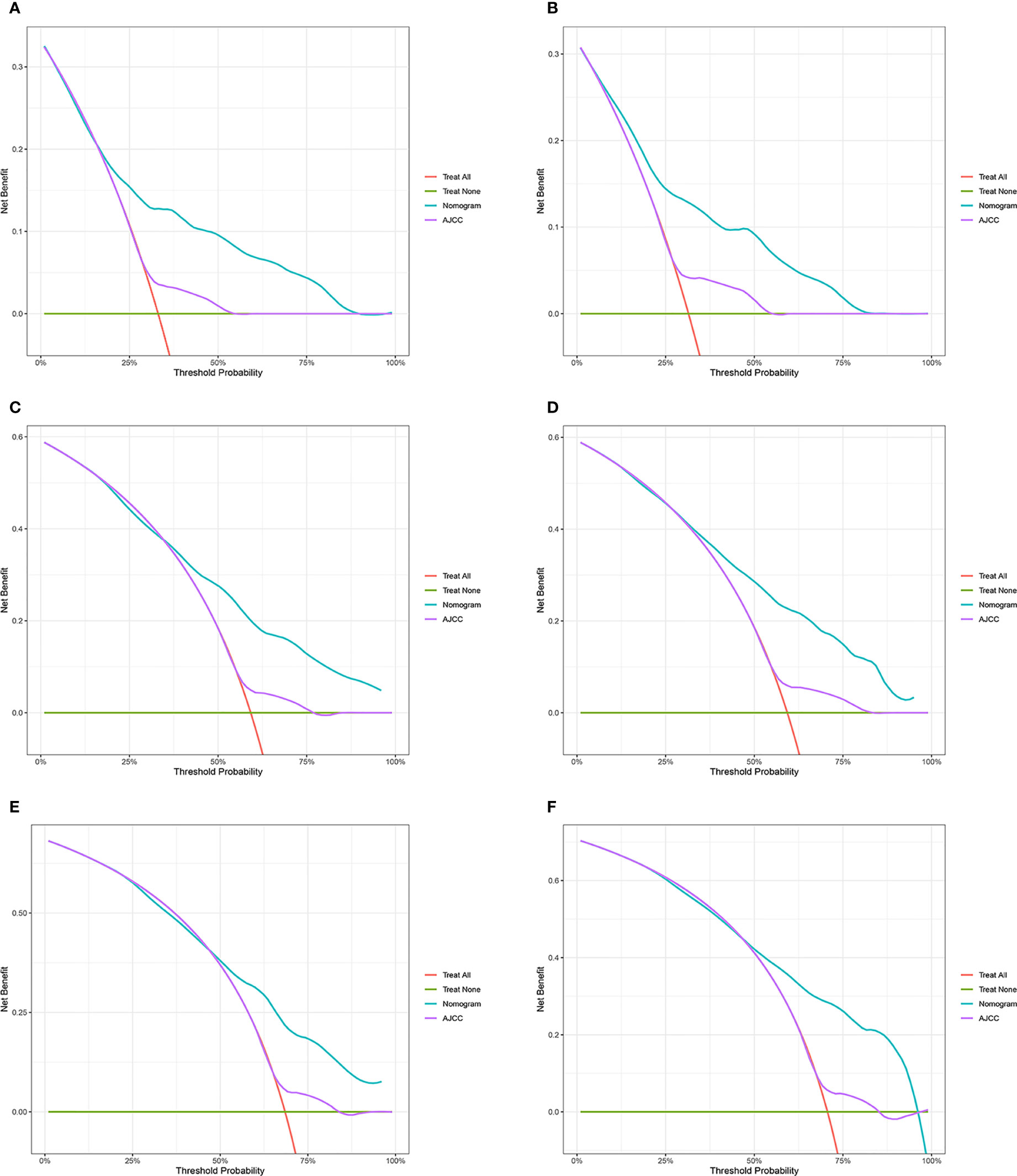
Figure 4 Decision curve analysis of the nomogram and AJCC tumor staging for the cancer-specific survival prediction of HCC patients with MVI. (A, C, E) 1-, 3- and 5-year cancer-specific survival benefits based on the training cohorts. (B, D, F) 1-, 3- and 5-year cancer-specific survival benefits based on the validation cohort. HCC, hepatocellular carcinoma; MVI, microvascular invasion.
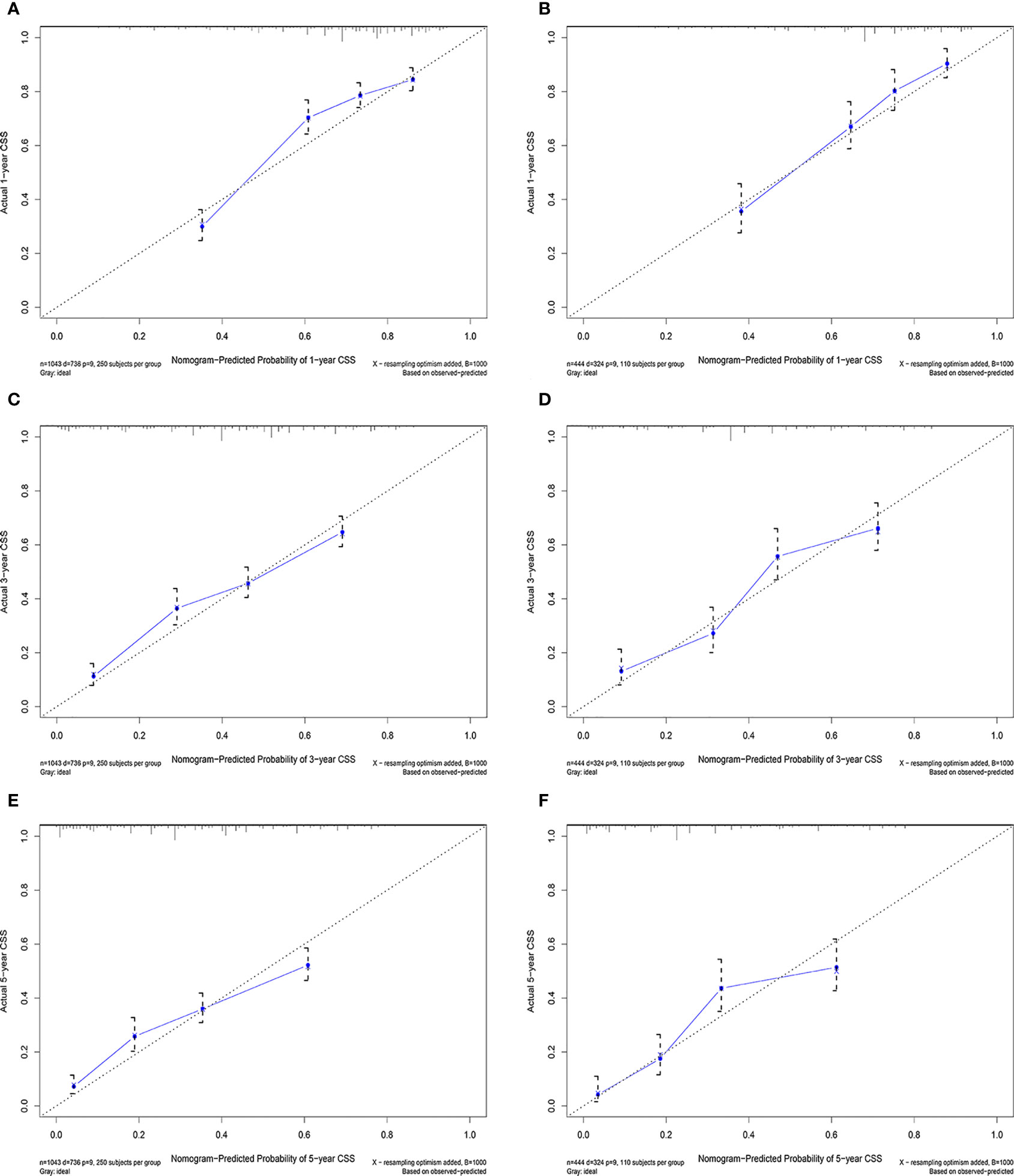
Figure 5 Calibration curves in the training (A, C, E) and validation (B, C, F) cohorts for 1-year, 3-year, and 5-year cancer-specific survival.
Clinical value of the new nomogram compared to the tumor stage based on AJCC staging
The NRI, IDI and C-index were included in the analysis to compare the advantages and limitations of the nomogram and the traditional AJCC tumor staging system. The results showed that the C-index related to the nomogram was higher than that of the AJCC staging system (Figure 6). The IDI values of 1-, 3-, 5-year CSS in the training cohort were 0.17, 0.16, 0.15, and in the validation cohort were 0.17, 0.17, 0.17 (P<0.05), indicated that the established nomogram significantly outperformed AJCC staging system. The NRI values of the training cohort were 0.75 (95% CI= 0.59-0.84) at 1-year, 0.68 (95% CI= 0.56-0.83) at 3-year and 0.67 (95% CI= 0.67) at 5-year (Table 3). The net benefit of the nomogram was compared to that of the AJCC staging system. The DCA curves showed that the nomogram better predicted 1-, 3-, and 5-year CSS in the all cohorts because the new model showed more net benefit than AJCC staging system.
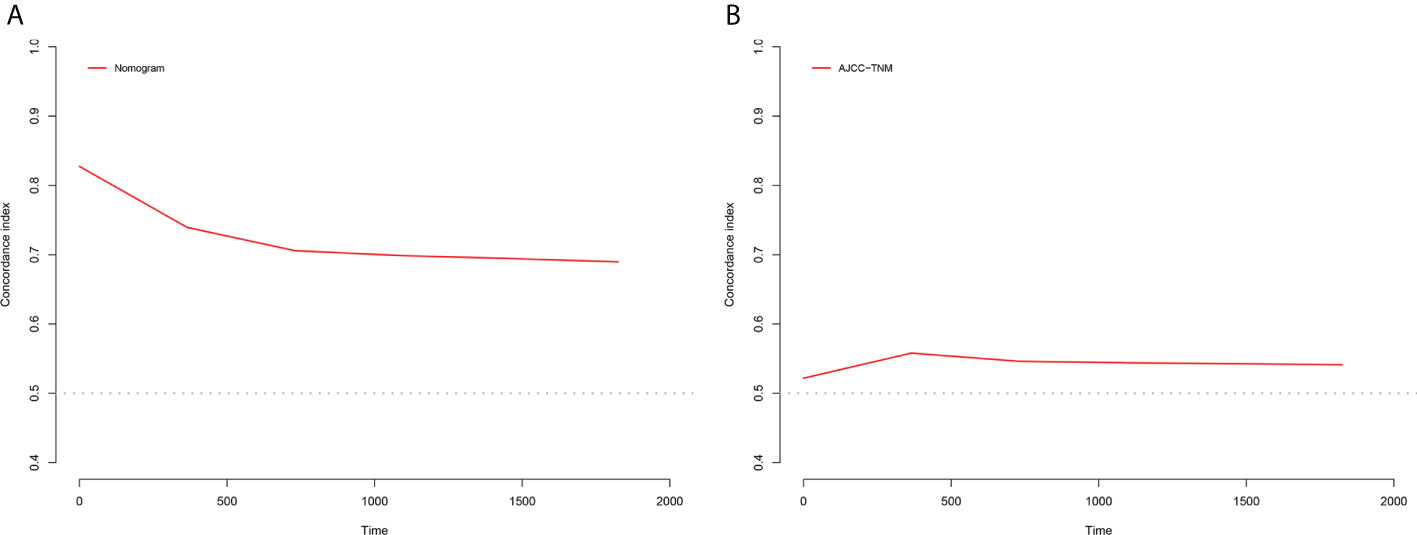
Figure 6 C-index results, (A) The C-index based on the nomogram; (B) The C-index based on the AJCC tumor staging.
Establishment of a stratified risk system based on the nomogram
According to the analysis results of X-tile software, all patients were re-divided into three groups (low risk: total score <540, middle risk: 540≤ total score <580, and high risk: total score ≥580) based on the nomogram (Figure 7). Kaplan-Meier curves suggested that the new classification system has a more satisfactory capability than traditional AJCC staging (Figure 8).
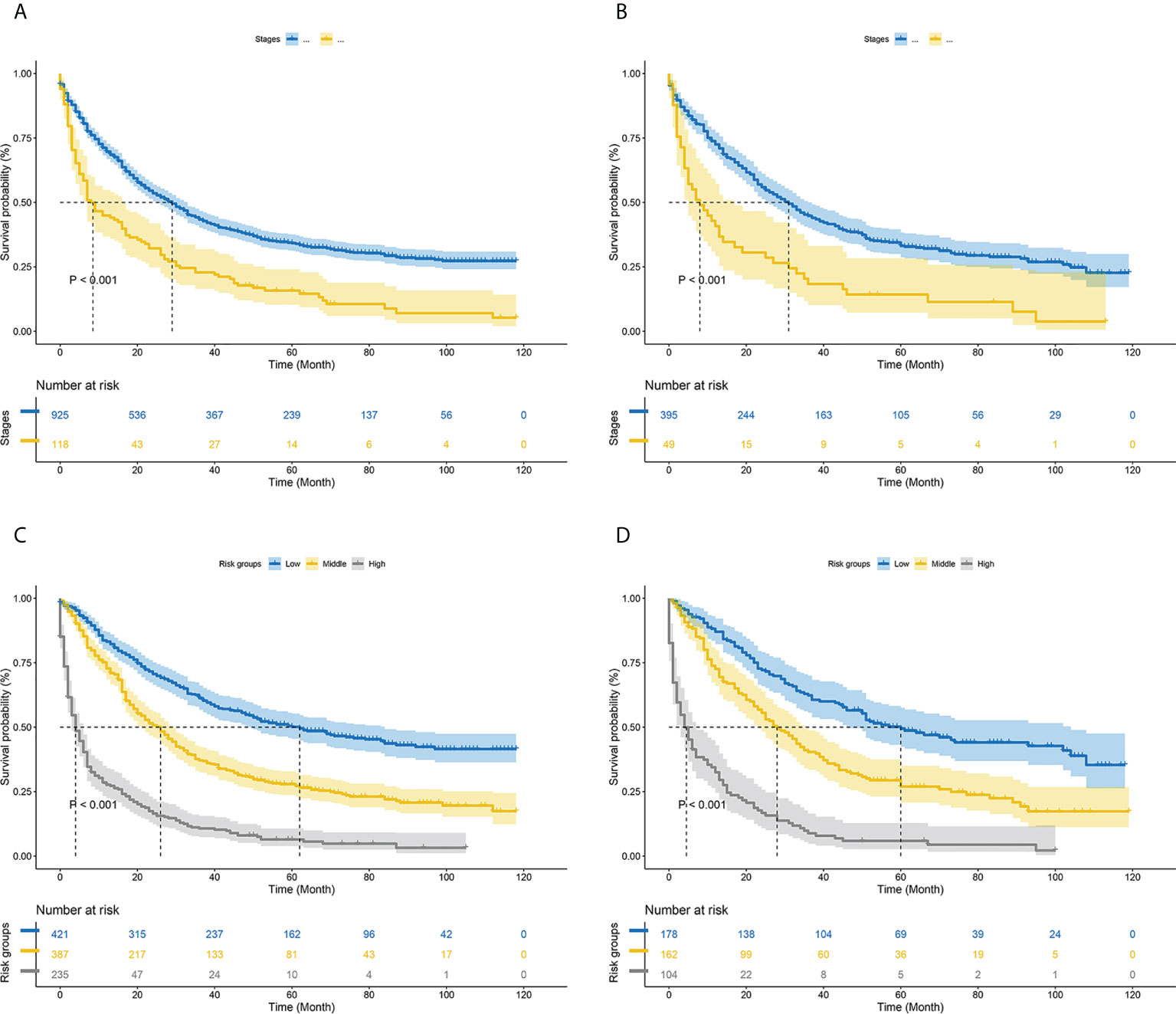
Figure 8 Kaplan–Meier curves of cancer-specific survival for new risk classification and the AJCC tumor staging (A) The AJCC tumor staging in the training cohort; (B) The AJCC tumor staging in the validation cohort; (C) The new risk classification in the training cohort; (D) The new risk classification in the validation cohort.
Discussion
HCC is one of the most common malignant tumors worldwide. MVI still exists in the residual liver after radical resection of liver cancer, and the detection rate is about 40% (26). HCC combined with MVI is more likely to invade blood vessels and lymphatic vessels, leading to early tumor spread and metastasis, which is an important factor predicting the prognosis of HCC (8, 9). Therefore, this study aimed to construct a nomogram to predict the prognosis of patients with HCC complicated with microvascular invasion. According to the results of multivariate COX regression analysis of the model group, 9 predictors including age, tumor size, pathological grade, AFP, radiation, chemotherapy, AJCC stages and surgery were included in the nomogram model that predicted the prognosis of MVI in HCC patients. The verification of the nomogram shows that the standard curve in the calibration curve of the model group and the validation group is basically close to the calibration prediction curve, indicating that the model has good discrimination and calibration ability. Based on the total score, patients were divided into low risk, middle risk, and high risk groups (the best cut-off values were selected by the X-tile software). In addition, Kaplan-Meier curves also showed that the new nomogram have more satisfactory discriminative power than traditional staging systems in stratifying the prognosis of HCC patients with MVI.
Previous studies have proposed some factors that may affect CSS in HCC patients complicated with MVI, such as age, tumor size, pathological grade and adjuvant therapy. However, these studies did not include additional clinical variables that affect the prognosis of HCC patients with MVI, and the small size of the total number of cases included in these studies inevitably biased the findings (27, 28). This study takes these factors into full consideration. Age and AFP are known significant risk factors, with lower AFP levels and longer median survival in older patients (29–31). Nathan (32) and Hirokawa (33) reported that tumor size is an independent risk factor for prognosis in HCC patients with MVI, which is consistent with the findings in this study that tumor diameter greater than 5 cm was an independent risk factor. The abnormal differentiation of tumor is one of the main characteristics of cancer cells, which is characterized by abnormal function and naive shape, and has strong proliferation and infiltration ability. Poorly differentiated cancer cells tend to have stronger vascular invasion capabilities, so the pathological grade is associated with the prognosis of MVI-HCC (34). Surgical resection is currently the treatment of choice for HCC patients combined with MVI who are not eligible for liver transplantation. As can be seen in the nomogram, there is a clear survival benefit from surgery. Hepatectomy is generally considered an important measure to improve patient prognosis, and hepatectomy still has a significant survival advantage for partial BCLC stage B HCC (35). In solitary small hepatocellular carcinoma with MVI, surgery rather than radiofrequency ablation should be used for initial treatment, and overall and disease-free survival with anatomic resection is significantly better than limited resection (36, 37). Adjuvant therapy is a controversial factor. Postoperative adjuvant TACE can reduce the tumor recurrence rate and help to improve the overall survival rate and tumor-free survival rate of liver cancer patients with MVI or multinodular tumors (38, 39). In patients with early (≤12 months) recurrence of mvi-positive HCC, TACE provided better overall survival than surgery or RFA (40). However, in many retrospective studies, postoperative adjuvant radiotherapy improved local control of HCC patients with MVI and was superior to TACE or conservative therapy, especially for patients with non-anatomical liver resection (NAR) (41, 42). In this study, our nomogram showed that adjuvant chemotherapy and radiotherapy could improve the postoperative prognosis of HCC patients with MVI.
The nomogram in this study integrates more factors into a quantitative model and has been shown to be superior to the AJCC in predicting prognosis and planning clinical strategies. Generally, the AJCC staging system has been closely related to OS. However, different outcomes were observed among patients in the same stage, which may be related to age, tumor size, adjuvant therapy and other factors. Therefore, we compared the nomogram involving multiple variables with tumor staging based on conventional AJCC criteria. The positive IDI and NRI of the nomogram demonstrated that the nomogram had superior predictive performance than the AJCC staging criteria alone. Furthermore, DCA revealed that the nomogram had better clinical utility and benefit in predicting CSS than conventional AJCC criteria. We established a risk stratification system, which could distinctly classify all patients into three risk prognostic groups according to their nomogram TPs. The cancer-specific survival curves presented that the new risk stratification system was superior to the conventional AJCC criteria in identifying different risk groups. Due to the poor prognosis of high-risk patients, we should pay more attention to patients with TP>580. These results suggest that the current nomograms are convenient and useful to assess the prognosis of HCC patients with MVI.
Despite the good performance of the nomogram, there are some limitations of this study. First, SEER did not publish data on virus-related variables, markers of inflammation, surgical margins. Therefore, these variables were not evaluated in this study. Second, the accuracy of the nomogram may be further enhanced by incorporating preoperative MVI prediction models and incorporating other prognostic biomarkers (43, 44). Finally, this study requires multicentre clinical data from other countries to verify the application value of the nomogram.
Conclusions
Based on the seer database, a comprehensive nomogram and an innovative risk stratification system were established to predict CSS for HCC patients with MVI. Internal validation proved that the risk classification system possesses promising application capabilities. Compared to AJCC staging, the system is valid to differentiate between different risk groups, which may serve as an efficient tool for individualised treatment of patients. However, this result requires validation with data from other countries.
Data availability statement
The original contributions presented in the study are included in the article/Supplementary Material. Further inquiries can be directed to the corresponding authors.
Author contributions
Conceptualization: DY, MZ, XX, YS. Data curation: GZ, YH, XX. Formal analysis: JP, XX, YS. Writing-original draft: DY, MZ, FZ. Writing-review and editing: YD, YH. All authors contributed to the article and approved the submitted version.
Conflict of interest
The authors declare that the research was conducted in the absence of any commercial or financial relationships that could be construed as a potential conflict of interest.
Publisher’s note
All claims expressed in this article are solely those of the authors and do not necessarily represent those of their affiliated organizations, or those of the publisher, the editors and the reviewers. Any product that may be evaluated in this article, or claim that may be made by its manufacturer, is not guaranteed or endorsed by the publisher.
Supplementary material
The Supplementary Material for this article can be found online at: https://www.frontiersin.org/articles/10.3389/fonc.2022.987603/full#supplementary-material
References
1. Forner A, Reig M, Bruix J. Hepatocellular carcinoma. Lancet (2018) 391(10127):1301–14. doi: 10.1016/S0140-6736(18)30010-2
2. Yang JD, Hainaut P, Gores GJ, Amadou A, Plymoth A, Roberts LR. A global view of hepatocellular carcinoma: trends, risk, prevention and management. Nat Rev Gastroenterol Hepatol (2019) 16(10):589–604. doi: 10.1038/s41575-019-0186-y
3. Islami F, Ward EM, Sung H, Cronin KA, Tangka FKL, Sherman RL, et al. Annual report to the nation on the status of cancer, part 1: National cancer statistics. J Natl Cancer Inst (2021) 113(12):1648–69. doi: 10.1093/jnci/djab131
4. Orcutt ST, Anaya DA. Liver resection and surgical strategies for management of primary liver cancer. Cancer Control (2018) 25(1):1073274817744621. doi: 10.1177/1073274817744621
5. Kohli R, Cortes M, Heaton ND, Dhawan A. Liver transplantation in children: state of the art and future perspectives. Arch Dis Child (2018) 103(2):192–8. doi: 10.1136/archdischild-2015-310023
6. Gao Q, Zhu H, Dong L, Shi W, Chen R, Song Z, et al. Integrated proteogenomic characterization of HBV-related hepatocellular carcinoma. Cell (2019) 179(2):561–77.e22. doi: 10.1016/j.cell.2019.08.052
7. Rodríguez-Perálvarez M, Luong TV, Andreana L, Meyer T, Dhillon AP, Burroughs AK. A systematic review of microvascular invasion in hepatocellular carcinoma: diagnostic and prognostic variability. Ann Surg Oncol (2013) 20(1):325–39. doi: 10.1245/s10434-012-2513-1
8. Lim KC, Chow PK, Allen JC, Chia GS, Lim M, Cheow PC, et al. Microvascular invasion is a better predictor of tumor recurrence and overall survival following surgical resection for hepatocellular carcinoma compared to the Milan criteria. Ann Surg (2011) 254(1):108–13. doi: 10.1097/SLA.0b013e31821ad884
9. Roayaie S, Blume IN, Thung SN, Guido M, Fiel MI, Hiotis S, et al. A system of classifying microvascular invasion to predict outcome after resection in patients with hepatocellular carcinoma. Gastroenterology (2009) 137(3):850–5. doi: 10.1053/j.gastro.2009.06.003
10. Mehta N, Heimbach J, Harnois DM, Sapisochin G, Dodge JL, Lee D, et al. Validation of a risk estimation of tumor recurrence after transplant (RETREAT) score for hepatocellular carcinoma recurrence after liver transplant. JAMA Oncol (2017) 3(4):493–500. doi: 10.1001/jamaoncol.2016.5116
11. Iguchi T, Shirabe K, Aishima S, Wang H, Fujita N, Ninomiya M, et al. New pathologic stratification of microvascular invasion in hepatocellular carcinoma: Predicting prognosis after living-donor liver transplantation. Transplantation (2015) 99(6):1236–42. doi: 10.1097/TP.0000000000000489
12. Pommergaard HC, Rostved AA, Adam R, Thygesen LC, Salizzoni M, Gómez Bravo MA, et al. Vascular invasion and survival after liver transplantation for hepatocellular carcinoma: a study from the European liver transplant registry. HPB (Oxford) (2018) 20(8):768–75. doi: 10.1016/j.hpb.2018.03.002
13. Pawlik TM, Delman KA, Vauthey JN, Nagorney DM, Ng IO, Ikai I, et al. Tumor size predicts vascular invasion and histologic grade: Implications for selection of surgical treatment for hepatocellular carcinoma. Liver Transpl (2005) 11(9):1086–92. doi: 10.1002/lt.20472
14. Sumie S, Nakashima O, Okuda K, Kuromatsu R, Kawaguchi A, Nakano M, et al. The significance of classifying microvascular invasion in patients with hepatocellular carcinoma. Ann Surg Oncol (2014) 21(3):1002–9. doi: 10.1245/s10434-013-3376-9
15. Rhee H, Chung T, Yoo JE, Nahm JH, Woo HY, Choi GH, et al. Gross type of hepatocellular carcinoma reflects the tumor hypoxia, fibrosis, and stemness-related marker expression. Hepatol Int (2020) 14(2):239–48. doi: 10.1007/s12072-020-10012-6
16. Xu LX, He MH, Dai ZH, Yu J, Wang JG, Li XC, et al. Genomic and transcriptional heterogeneity of multifocal hepatocellular carcinoma. Ann Oncol (2019) 30(6):990–7. doi: 10.1093/annonc/mdz103
17. Mo HY, Zhong JH, Li LQ. Comment on tumor size as a prognostic factor for solitary HCC after resection. J Surg Oncol (2016) 113(5):593. doi: 10.1002/jso.24173
18. Wang L, Jin YX, Ji YZ, Mu Y, Zhang SC, Pan SY. Development and validation of a prediction model for microvascular invasion in hepatocellular carcinoma. World J Gastroenterol (2020) 26(14):1647–59. doi: 10.3748/wjg.v26.i14.1647
19. Li S, Zeng Q, Liang R, Long J, Liu Y, Xiao H, et al. Using systemic inflammatory markers to predict microvascular invasion before surgery in patients with hepatocellular carcinoma. Front Surg (2022) 9:833779. doi: 10.3389/fsurg.2022.833779
20. Zhong X, Peng J, Xie Y, Shi Y, Long H, Su L, et al. A nomogram based on multi-modal ultrasound for prediction of microvascular invasion and recurrence of hepatocellular carcinoma. Eur J Radiol (2022) 151:110281. doi: 10.1016/j.ejrad.2022.110281
21. Yao W, Yang S, Ge Y, Fan W, Xiang L, Wan Y, et al. Computed tomography radiomics-based prediction of microvascular invasion in hepatocellular carcinoma. Front Med (Lausanne) (2022) 9:819670. doi: 10.3389/fmed.2022.819670
22. Hong SB, Choi SH, Kim SY, Shim JH, Lee SS, Byun JH, et al. MRI Features for predicting microvascular invasion of hepatocellular carcinoma: A systematic review and meta-analysis. Liver Cancer (2021) 10(2):94–106. doi: 10.1159/000513704
23. Chen S, Wang C, Gu Y, Ruan R, Yu J, Wang S. Prediction of microvascular invasion and its M2 classification in hepatocellular carcinoma based on nomogram analyses. Front Oncol (2021) 11:774800. doi: 10.3389/fonc.2021.774800
24. He C, Zhao C, Zhang Y, Chen C, Lin X. An inflammation-index signature predicts prognosis of patients with intrahepatic cholangiocarcinoma after curative resection. J Inflam Res (2021) 14:1859–72. doi: 10.2147/JIR.S311084
25. Adhoute X, Pénaranda G, Raoul JL, Edeline J, Blanc JF, Pol B, et al. Barcelona Clinic liver cancer nomogram and others staging/scoring systems in a French hepatocellular carcinoma cohort. World J Gastroenterol (2017) 23(14):2545–55. doi: 10.3748/wjg.v23.i14.2545
26. Li Z, Lei Z, Xia Y, Li J, Wang K, Zhang H, et al. Association of preoperative antiviral treatment with incidences of microvascular invasion and early tumor recurrence in hepatitis b virus-related hepatocellular carcinoma. JAMA Surg (2018) 153(10):e182721. doi: 10.1001/jamasurg.2018.2721
27. Lin S, Ye F, Rong W, Song Y, Wu F, Liu Y, et al. Nomogram to assist in surgical plan for hepatocellular carcinoma: a prediction model for microvascular invasion. J Gastrointest Surg (2019) 23(12):2372–82. doi: 10.1007/s11605-019-04140-0
28. Xu L, Li L, Wang P, Zhang M, Zhang Y, Hao X, et al. Novel prognostic nomograms for hepatocellular carcinoma patients with microvascular invasion: Experience from a single center. Gut Liver (2019) 13(6):669–82. doi: 10.5009/gnl18489
29. Cucchetti A, Piscaglia F, Grigioni AD, Ravaioli M, Cescon M, Zanello M, et al. Preoperative prediction of hepatocellular carcinoma tumour grade and micro-vascular invasion by means of artificial neural network: a pilot study. J Hepatol (2010) 52(6):880–8. doi: 10.1016/j.jhep.2009.12.037
30. Ma L, Deng K, Zhang C, Li H, Luo Y, Yang Y, et al. Nomograms for predicting hepatocellular carcinoma recurrence and overall postoperative patient survival. Front Oncol (2022) 12:843589. doi: 10.3389/fonc.2022.843589
31. Motoyama H, Kobayashi A, Yokoyama T, Shimizu A, Sakai H, Furusawa N, et al. Impact of advanced age on the short- and long-term outcomes in patients undergoing hepatectomy for hepatocellular carcinoma: a single-center analysis over a 20-year period. Am J Surg (2015) 209(4):733–41. doi: 10.1016/j.amjsurg.2014.05.026
32. Nathan H, Schulick RD, Choti MA, Pawlik TM. Predictors of survival after resection of early hepatocellular carcinoma. Ann Surg (2009) 249(5):799–805. doi: 10.1097/SLA.0b013e3181a38eb5
33. Hirokawa F, Hayashi M, Miyamoto Y, Asakuma M, Shimizu T, Komeda K, et al. Outcomes and predictors of microvascular invasion of solitary hepatocellular carcinoma. Hepatol Res (2014) 44(8):846–53. doi: 10.1111/hepr.12196
34. Sumie S, Kuromatsu R, Okuda K, Ando E, Takata A, Fukushima N, et al. Microvascular invasion in patients with hepatocellular carcinoma and its predictable clinicopathological factors. Ann Surg Oncol (2008) 15(5):1375–82. doi: 10.1245/s10434-008-9846-9
35. Kim H, Ahn SW, Hong SK, Yoon KC, Kim HS, Choi YR, et al. Survival benefit of liver resection for Barcelona clinic liver cancer stage b hepatocellular carcinoma. Br J Surg (2017) 104(8):1045–52. doi: 10.1002/bjs.10541
36. Fan LF, Zhao WC, Yang N, Yang GS. Alpha-fetoprotein: the predictor of microvascular invasion in solitary small hepatocellular carcinoma and criterion for anatomic or non-anatomic hepatic resection. Hepatogastroenterology (2013) 60(124):825–36. doi: 10.1016/j.ejso.2019.06.031
37. Lee S, Kang TW, Song KD, Lee MW, Rhim H, Lim HK, et al. Effect of microvascular invasion risk on early recurrence of hepatocellular carcinoma after surgery and radiofrequency ablation. Ann Surg (2021) 273(3):564–71. doi: 10.1097/SLA.0000000000003268
38. Chen ZH, Zhang XP, Zhou TF, Wang K, Wang H, Chai ZT, et al. Adjuvant transarterial chemoembolization improves survival outcomes in hepatocellular carcinoma with microvascular invasion: A systematic review and meta-analysis. Eur J Surg Oncol (2019) 45(11):2188–96. doi: 10.1016/j.ejso.2019.06.031
39. Wang H, Du PC, Wu MC, Cong WM. Postoperative adjuvant transarterial chemoembolization for multinodular hepatocellular carcinoma within the Barcelona clinic liver cancer early stage and microvascular invasion. Hepatobil Surg Nutr (2018) 7(6):418–28. doi: 10.21037/hbsn.2018.09.05
40. Jin YJ, Lee JW, Lee OH, Chung HJ, Kim YS, Lee JI, et al. Transarterial chemoembolization versus surgery/radiofrequency ablation for recurrent hepatocellular carcinoma with or without microvascular invasion. J Gastroenterol Hepatol (2014) 29(5):1056–64. doi: 10.1111/jgh.12507
41. Wang L, Chen B, Li Z, Yao X, Liu M, Rong W, et al. Optimal postoperative adjuvant treatment strategy for HBV-related hepatocellular carcinoma with microvascular invasion: a propensity score analysis. Onco Targets Ther (2019) 12:1237–47. doi: 10.2147/OTT.S179247
42. Yang J, Liang H, Hu K, Xiong Z, Cao M, Zhong Z, et al. The effects of several postoperative adjuvant therapies for hepatocellular carcinoma patients with microvascular invasion after curative resection: a systematic review and meta-analysis. Cancer Cell Int (2021) 21(1):92. doi: 10.1186/s12935-021-01790-6
43. Lei Z, Li J, Wu D, Xia Y, Wang Q, Si A, et al. Nomogram for preoperative estimation of microvascular invasion risk in hepatitis b virus-related hepatocellular carcinoma within the Milan criteria. JAMA Surg (2016) 151(4):356–63. doi: 10.1001/jamasurg.2015.4257
Keywords: hepatocellular carcinoma, microvascular invasion, cancer-specific survival, nomogram, risk stratification, prognostic model
Citation: Yang D, Zhu M, Xiong X, Su Y, Zhao F, Hu Y, Zhang G, Pei J and Ding Y (2022) Clinical features and prognostic factors in patients with microvascular infiltration of hepatocellular carcinoma: Development and validation of a nomogram and risk stratification based on the SEER database. Front. Oncol. 12:987603. doi: 10.3389/fonc.2022.987603
Received: 11 August 2022; Accepted: 29 August 2022;
Published: 14 September 2022.
Edited by:
Zisis Kozlakidis, International Agency For Research On Cancer (IARC), FranceReviewed by:
Hengkai Chen, First Affiliated Hospital of Fujian Medical University, ChinaBing Mao, Henan Provincial People’s Hospital, China
Copyright © 2022 Yang, Zhu, Xiong, Su, Zhao, Hu, Zhang, Pei and Ding. This is an open-access article distributed under the terms of the Creative Commons Attribution License (CC BY). The use, distribution or reproduction in other forums is permitted, provided the original author(s) and the copyright owner(s) are credited and that the original publication in this journal is cited, in accordance with accepted academic practice. No use, distribution or reproduction is permitted which does not comply with these terms.
*Correspondence: Youming Ding, dingym62@163.com; Yong Hu, hyrm2017@126.com
†These authors have contributed equally to this work