- 1Division of Oral and Maxillofacial Surgery, Faculty of Dentistry, University of Hong Kong, Hong Kong, Hong Kong, SAR, China
- 2Oral Cancer Research Theme, Faculty of Dentistry, University of Hong Kong, Hong Kong, Hong Kong, SAR, China
- 3Department of Oral and Maxillofacial Pathology and Biology, Faculty of Dentistry, University of Lagos, Lagos, Nigeria
- 4Division of Applied Oral Sciences and Community Dental Care, Faculty of Dentistry, University of Hong Kong, Hong Kong, Hong Kong, SAR, China
- 5Clinical Artificial Intelligence Research Theme, Faculty of Dentistry, University of Hong Kong, Hong Kong, Hong Kong, SAR, China
- 6College of Medicine and Dentistry, James Cook University, Cairns, Queensland, Australia
Background: The impact and utility of machine learning (ML)-based prediction tools for cancer outcomes including assistive diagnosis, risk stratification, and adjunctive decision-making have been largely described and realized in the high income and upper-middle-income countries. However, statistical projections have estimated higher cancer incidence and mortality risks in low and lower-middle-income countries (LLMICs). Therefore, this review aimed to evaluate the utilization, model construction methods, and degree of implementation of ML-based models for cancer outcomes in LLMICs.
Methods: PubMed/Medline, Scopus, and Web of Science databases were searched and articles describing the use of ML-based models for cancer among local populations in LLMICs between 2002 and 2022 were included. A total of 140 articles from 22,516 citations that met the eligibility criteria were included in this study.
Results: ML-based models from LLMICs were often based on traditional ML algorithms than deep or deep hybrid learning. We found that the construction of ML-based models was skewed to particular LLMICs such as India, Iran, Pakistan, and Egypt with a paucity of applications in sub-Saharan Africa. Moreover, models for breast, head and neck, and brain cancer outcomes were frequently explored. Many models were deemed suboptimal according to the Prediction model Risk of Bias Assessment tool (PROBAST) due to sample size constraints and technical flaws in ML modeling even though their performance accuracy ranged from 0.65 to 1.00. While the development and internal validation were described for all models included (n=137), only 4.4% (6/137) have been validated in independent cohorts and 0.7% (1/137) have been assessed for clinical impact and efficacy.
Conclusion: Overall, the application of ML for modeling cancer outcomes in LLMICs is increasing. However, model development is largely unsatisfactory. We recommend model retraining using larger sample sizes, intensified external validation practices, and increased impact assessment studies using randomized controlled trial designs
Systematic review registration: https://www.crd.york.ac.uk/prospero/display_record.php?RecordID=308345, identifier CRD42022308345.
Introduction
An estimated 20 million new cancer cases and 10 million cancer-related deaths occur annually (1). By 2040, the incidence rate is extrapolated to increase by 47% with the highest excess relative risks (64 – 95%) to be observed in low- and middle-income countries. Moreover, mortality estimates are expected to parallel the increase in case occurrence (1). Precision prevention, diagnosis, risk stratification, and treatment are now being advocated as contemporaneous strategies to mitigate the incidence, morbidity, and mortality of patients (2). This involves ultramodern tools and approaches that uncover personalized profiles of patients and tumors, ultimately, assisting in tailoring specific interventions to patients while avoiding the need to administer generic intervention strategies to all individuals (3, 4).
Artificial intelligence (AI) plays a central role in the administration of personalized medicine for cancer patients. Digital systems based on AI can provide objective judgments that simulate human intelligence while considering patient- or tumor-related factors (5, 6). Applications of AI in oncological management include medical/pathological image or video analysis, natural language processing of free-full text electronic health record (EHR) reports, robots, and chatbots as intervention assistants and information resources, affective computing for digital health assistants, automated treatment planning and scheduling, and machine learning (ML) models for the prediction of cancer-related outcomes (7–15). ML-based models have been proposed or evaluated regarding their ability to perform individualized diagnosis, risk stratification, tumor profiling, assisted screening, treatment selection, and disease prognosis prediction (7, 14–18). However, many of the predictive intelligent models have been constructed for and using populations in upper-middle and high-income countries in line with the current distributions of cancer burden (19).
With the expected surge in cancer incidence and mortality among low- and lower-middle-income countries (1), it is imperative to assess the construction and utilization status of ML-based models and platforms in readiness for their implementation in precision cancer interventions in these settings. As external validation of ML-based models available in the Global North may not see fruition in many developing countries owing to the disparities in ethnicity, variability in clinical and molecular cancer subtypes and unavailability of several high-precision advanced cancer predictors, indigenous models developed specifically for these populations may take precedence (19–21). Therefore, in this study, we evaluate the utilization, methodology of model construction, and implementation phases performed for ML-based models for cancer outcomes in low- and lower-middle-income populations.
Methods
This scoping review sourced for studies between January 2002 and March 2022 that reported on ML-based models for cancer-related outcomes in low- or lower-middle-income countries (LLMICs). The composition of LLMICs was according to the 2022 World Bank classification of countries by income with 27 and 55 countries in the low and lower-middle income groups respectively (Figure 1) (22). ML-based models considered were those constructed on the backend of traditional machine learning and deep learning algorithms. Also, cancer-related outcomes cuts across risk stratification, screening, diagnosis, treatment selection, treatment complications, and prognosis of any malignancy which were assessed exclusively among LLMIC populations. Original retrospective or prospective studies were included in the review provided: (i) they were conducted exclusively among local populations in LLMICs for all model implementation phases, (ii) the outcomes were assessed among clinical patients or tumors linked to clinical patients, (iii) they featured an ML-based model/platform/software for which internal validation of models had been performed upon development (Phases I and II studies) (23).
Studies reporting multinational cohorts (even if they included LLMICs) or those that used LLMIC populations only for external validation were excluded. Further excluded, were studies featuring models constructed on public datasets or studies for which the development of the models among LLMICs was not described or referenced. However, for public datasets obtained from LLMIC populations, we only included the first study where the cohorts were initially recruited and analyzed for model construction. Studies with suspected data fabrication/falsification, incomplete reporting to assess development/validation population and ML modeling, and unclear stratification of malignant or high-risk classes (in the case of screening and risk stratification) were not selected. Duplicate studies (i.e., studies that used different models for the same outcome on a particular patient cohort), in-vitro studies, animal studies, commentaries, and editorials were also excluded. For duplicate reports, only the study on the models with the best performance was considered in this review.
Articles were sourced from PubMed, Medline, Web of Science, and Scopus electronic databases using nonspecific but pertinent combinations of search keywords for complete retrieval of related texts (Supplementary Table 1). Automated and manual deduplication of citations were then performed. We adopted a two-stage strategy for article selection. In one stage, we removed articles unrelated to the review objectives, studies based on inferential statistical associations, and those not comprising LLMIC populations. Subsequently, full-length texts of screened articles were obtained and assessed twice for each study according to the inclusion and exclusion criteria. These selection stages were performed by two independent authors (JA and AA) and discrepancies were resolved by consensus following discussions and agreement between both reviewers was the basis for the final study selection. During the second-stage selection, a complementary manual search of the references and citations of studies was performed to facilitate the inclusion of studies that may have been missed during electronic database searching. The agreement between both reviewers in selecting articles was near perfect with a κ value of 0.907 (p < 0.001) (19).
Data charting was done using full texts of selected articles. Items sourced included the author names, publication year, study LLMIC, study design, sample size, cancer types, outcomes, ML-model type, model development approach, features, implementation phases (23), and performance measures. For models with multiple validation populations, the mean of their accuracies was calculated and utilized in this study. Data collection was performed independently and in duplicate with the original full texts referred to for discordant entries.
Risk of bias assessment and quality rating of selected studies were performed using the Prediction model Risk of Bias Assessment tool (PROBAST) (24). All four original risk of bias domains were retained and scored as high, low, or unclear based on the total signaling questions per domain. The overall risk of bias ratings was given as ‘high’ if at least one domain was rated as ‘high/unclear’ and ‘low’ if all four domains were rated as ‘low’.
Qualitative synthesis was performed at the level of the studies and models for all LLMICs found. Assumptions were not made for missing data. Descriptive statistics were calculated as median, interquartile range, and frequencies. Pearson’s Chi-square test was used to determine significant differences for categorical variables while Kruskal-Wallis and Mann-Whitney U tests were used for continuous variables. Probability values below 5% were considered statistically significant. SPSS v 27 was used for all analyses. Reporting of this review was in line with the Preferred Reporting Items for Systematic Reviews and Meta-analysis extension for scoping reviews (PRISMA-ScR) (25) guidelines and the protocol registered with the International prospective register of systematic reviews (PROSPERO) with registration number CRD42022308345.
Results
Upon removal of duplicates, 22,516 citations were screened leaving 311 articles for full-text evaluation. Altogether, 171 articles were excluded for reasons presented in Figure 2 and 140 articles were included in this review. These articles emanated from 133 studies as model development and validation were reported in two separate articles by three studies while two studies reported their model construction in three different articles. Likewise, a total of 137 models for various cancer-related outcomes in LLMICs were extracted from these studies (26–165). Citations of included articles are listed in Supplementary Table 2.
General characteristics of studies
Studies were published between 2005 and 2022, and of 133 studies, 81.2% were published from 2018 onward (Figure 3A) (26–165). Of the 82 countries that make up LLMICs in this review, only 13 (15.8%) had at least a study included in this review (Figure 3B). All but two studies from Ethiopia and Sudan were conducted in lower-middle-income countries. Majority of the studies were from Indian (55.6%) and Iranian (17.3%) populations while four sub-Saharan African countries and the Philippines had only one study included. Irrespective of the number of centers involved, retrospective cohorts were often used for model constructions than prospective cohorts (68.4% vs 31.6%; Figure 3C). At least, outcomes from 19 different cancer types were modeled. However, five studies included patients with different cancer types. Only two studies involved patients with sarcomas (osteosarcoma and uterine sarcoma) while other studies involved those with carcinomas, leukemias, and lymphomas. Breast cancer (32.3%), head and neck cancer (14.3%), and brain tumors (9%) were the three most common malignancies by subtypes (Figure 3D). Further, we noticed a strong agreement between common malignancies used for ML modeling and the three most-incident tumors [based on GLOBOCAN estimates 2020 (1)] in each LLMIC represented (κ = 0.833, p=0.003).
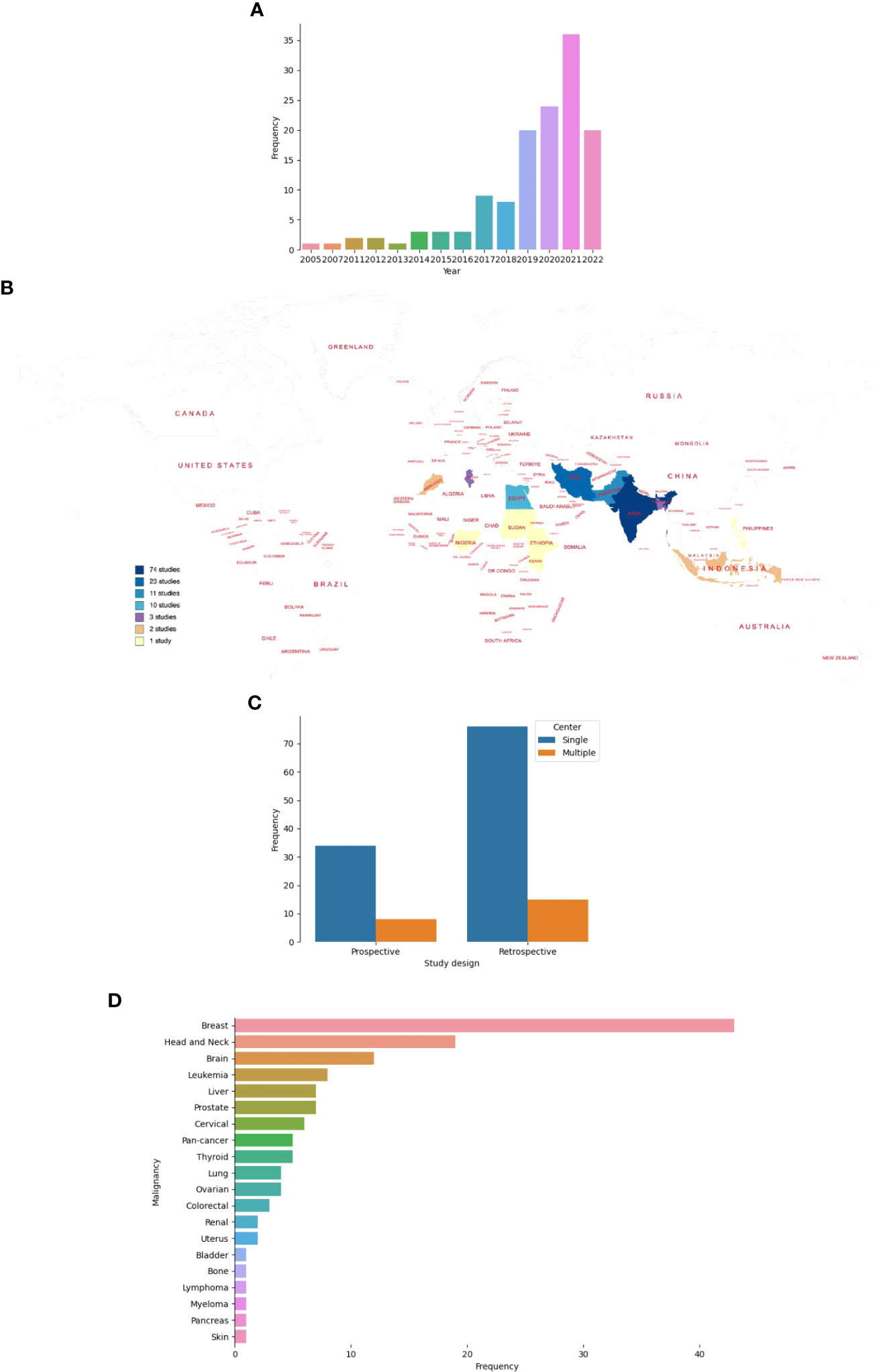
Figure 3 Characteristics of studies on ML-based models for cancer outcomes in LLMICs. (A) Bar plot showing the frequency of studies by publication year (B) Geographic distribution of studies on ML-models for cancer in LLMICs by country (C) Bar plot showing the study design and number of centers involved during model construction (D) Plot showing the frequency of cancers for which models were developed in the LLMIC population.
Risk of bias assessment for studies involving ML-based models in LLMICs
Only four studies (3.0%) had a low overall risk of bias across all four domains of the PROBAST tool (Supplementary Table 3). Proportion of studies with high and low risk of bias in each domain are plotted in Figure 4. More ML studies (93.2%) had a low risk of bias in their evaluation of outcomes while a majority of studies (85.7%) had a high risk of bias regarding the analytical methodology and technical approach to model construction.
Of the 114 studies with a high risk of bias in the ‘analysis’ domain, most studies did not have an event-per-variable (EPV) of at least 20 during training or at least 100 patients in each outcome category during model validation (n = 96). Moreover, other studies did not perform any data resampling methods (cross-validation, bootstrapping, or jackknife) using the training cohort to assess model stability or did not implement imbalanced class correction for model outcomes when necessitated. Due to the retrospective cohort design of many ML studies for cancers, predictors for cross-sectional outcomes such as diagnosis and treatment selection were selected while the outcome of the patients was known leading to their high risk of bias rating in the ‘predictors’ domain. Of note, 35.3% of studies had an unclear risk of bias in the ‘participants’ domain due to gross under-reporting of the inclusion and exclusion criteria used in the selection of patients for which outcomes were evaluated.
Evaluating the methods of ML-based model construction for cancer outcomes in LLMIC populations
Of 137 models, 74 (54%) were developed on the backend of traditional machine learning algorithms while 53 (38.6%) were based on deep learning (Figure 5A). Ten studies utilized both methods as a deep hybrid learning platform. Novel architectures were used for the development of 21 models (15.3%) which were mostly deep learning (95.2%) than deep hybrid or traditional machine learning models (Figure 5B; p<0.001). For studies that reported datasets used by the number of patients (n = 102), cohort sizes ranged from 10 to 5025 patients. A decreasing trend in the proportion of studies was noted with an increase in the number of patients used for model construction. More models were based on predictive features obtained from and for <200 patients (72.6%) than 200 to 500 (13.7%) or 500 to 1000 patients (9.8%). Only four models utilized 1000 patients or more. Stratifying the cohort size by the different ML techniques, all model types mostly involved < 200 patients (Figure 5C). Sample size estimation for model training and validation was only performed for 4 models (2.9%) (Supplementary Table 4).
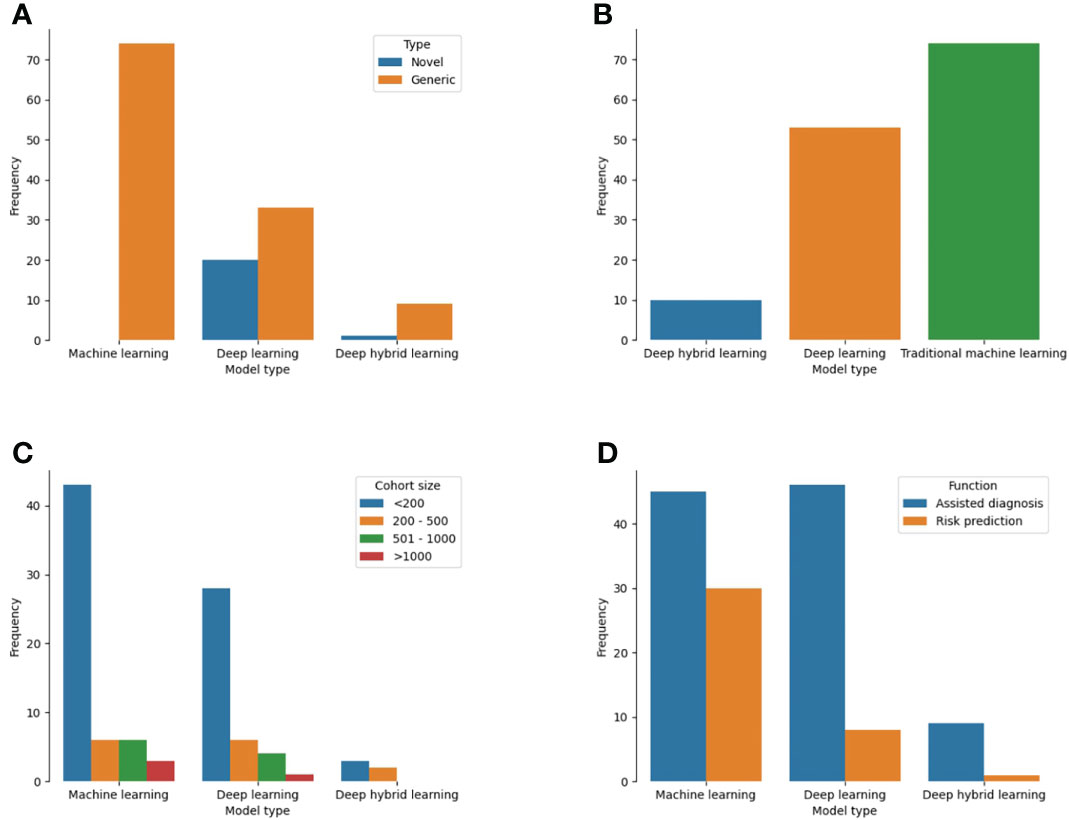
Figure 5 Plot showing the different techniques used for ML-based model construction for cancer patients in LLMICs (A) Model types (B) Novelty of backend algorithm used for model construction (C) Patient cohort size for model construction (D) Functions of the ML-based models.
Single cancer-related outcomes were considered in 134 models including cancer diagnosis (70.1%), overall prognosis/treatment response (14.6%), screening (8.0%), and treatment (5.1%). For the three models with multiple outcomes, two considered both cancer screening and diagnosis while one was used for tumor diagnosis and prognosis prediction (Supplementary Table 4). Tasks for which models were designed to perform included assisted diagnosis (70.8%), risk prediction/stratification (26.3%), and assisted treatment selection (0.7%). These functions were significantly different among the three types of ML techniques used for model construction as more traditional ML models than deep learning and deep hybrid learning (40% vs 14.8% vs 10%) were developed for risk prediction/stratification (Figure 4D, p = 0.003). Similarly, deep learning (85.2%) and deep hybrid learning (90%) models were mostly fashioned to assist in cancer diagnosis.
Features used for model development among LLMIC populations were radiomics (40.2%), clinical (33.6%), pathological (30.7%), and molecular (16.1%) in nature. Of the radiomics datasets, 29 models used magnetic resonance imaging, 15 models utilized computed tomography scans, 10 models used mammograms, and 5 models used ultrasound scans (Supplementary Table 4). Also, biomarkers used as molecular features were proteomic (n=16), genomic (n=3), or metabolomic (n=3) in nature (Supplementary Table 4). Clinical and radiomic features were commonly used for ML model construction for cancer outcomes in LLMICs before 2018 while more recent years have seen higher incorporation of pathological and molecular features (Figure 6A). As realistic models for cancer outcomes often involve multidimensional features, only 23 models (16.8%) used at least two of the four feature categories with the combinations of clinical, pathological, and molecular variables being more common.
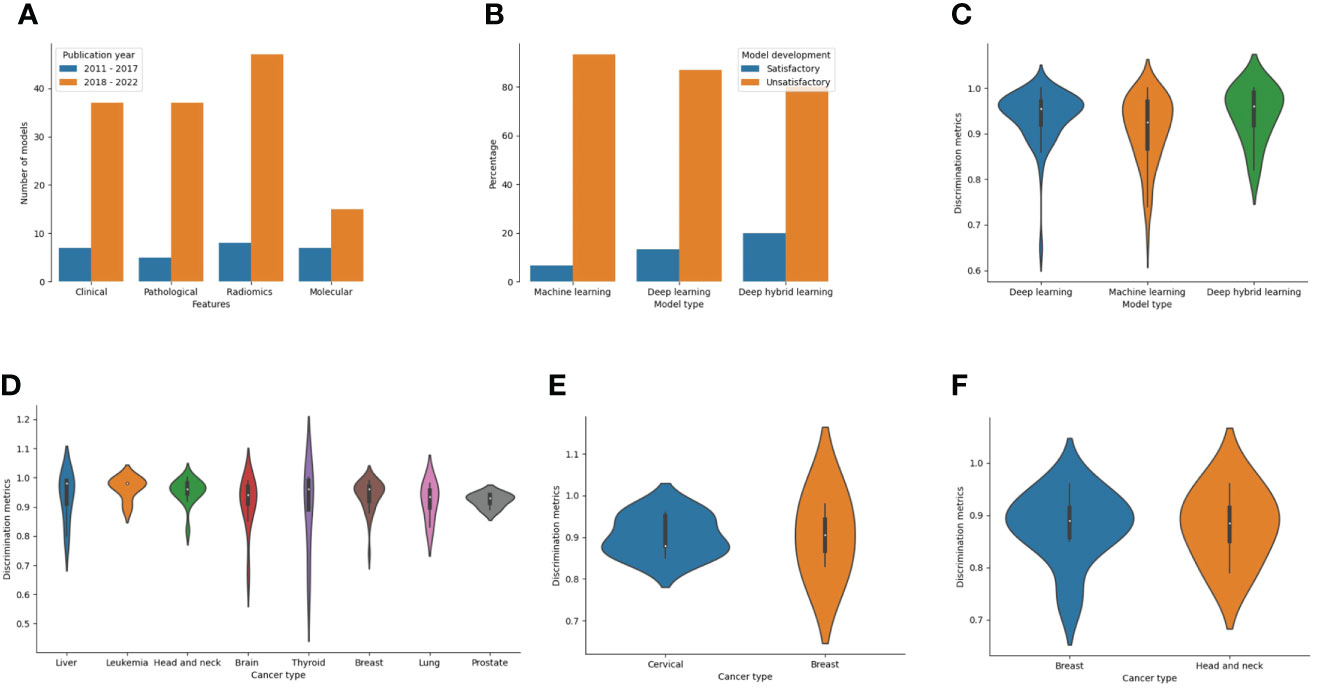
Figure 6 Bar and violin plots evaluating the methods used for model development and summary model performances (A) Plot of features used for model construction by publication year (B) Plot of ML technique by the rating of model development processes (C) Plot showing summary accuracy estimates for the three different ML techniques (D) Plot showing summary accuracy for ML-based models for diagnosis according to the cancer types (E) Plot showing summary accuracy for ML-based models for cancer screening according to the cancer types (F) Plot showing summary accuracy for ML-based models for cancer prognosis according to the cancer types.
Comparison of different algorithms was often conducted before optimal model selection (106 models [77.4%]) and many models were evaluated alongside other ML models (75.2%) than traditional statistical models (13.1%). Of 107 models in which performance comparisons were done, five studies (4.7%) found that the models selected or optimized had an equivalent performance compared to others employed while one ML-based model was reported to have displayed no additional benefit in predictive performance following model comparison (Supplementary Table 4). Other ML-based models (93.5%) were deemed to have positive findings which motivated their selection for proposed tasks.
Considering the model development strategies underwent during construction, 123 of 137 models (89.8%) were deemed unsatisfactory in their approach. This was mostly due to a combination of inadequate sampling and improper technique (47.2%) than inadequate sampling (37.7%) or improper techniques (14.8%) alone (Supplementary Table 4). Common shortfalls in modeling techniques included the use of test-train splitting without nested cross-validation or bootstrapping during model training, not using feature selection even in the event of a low EPV, and lack of data augmentation or imbalance class correction when warranted. The lack of imbalanced class correction points to a limitation in the outcome class parity which may mean that the quality of data used for modeling is low. Although not statistically significant, stratifying the models based on the techniques showed that a higher proportion of deep hybrid models were satisfactory than deep learning or traditional ML models (20% vs 13.2% vs 6.8%, p=0.274) (Figure 6B). Also, according to the publication year, 1 of 25 models (4%) published before 2018 was satisfactory while for studies within the last five years, 13 of 112 models (11.6%) were satisfactory.
Of 124 models that were constructed after the establishment of the Transparent reporting of a multivariate prediction model for individual prognosis or diagnosis (TRIPOD) guideline or Standards for reporting diagnostic accuracy (STARD) guideline, only one model (79) followed the STARD guideline for model and performance reporting (Supplementary Table 4). None of the other models alluded to have followed any established guidelines during construction.
Status of implementation, performance, and clinical impact of ML models in LLMICs
Generally, 128 (93.4%) models have completed phase I (data pre-preprocessing) and II (development and internal validation) implementation strategies only. Further, only 6 models (4.4%) constructed for cancer outcomes in LLMIC populations have been externally validated (phase III implementation) all of which were within the last five years. These included models for breast cancer diagnosis (85, 86, 152), lung cancer diagnosis (51, 52), head and neck cancer diagnosis (124), breast cancer metastasis (47, 56, 128), liver cancer risk prediction (78), and treatment response in colorectal cancer (148). Of these models, only two (47, 56, 85, 152) sufficiently fulfilled the TRIPOD criteria for external validation based on the sample size. Also, none of the deep learning or deep hybrid learning models found have been assessed using external validation.
Software for end-user interactions (phase IV implementation) has been designed for four ML-based models; however, three of these models were not even validated in an independent population before software development. Only one independently-validated ML-based model for breast cancer diagnosis before software development has also had a clinical impact assessment performed (phase V implementation). However, this was conducted using an observational study design (85).
The overall discriminatory performance (AUC/Accuracy) of the ML-based cancer models in LLMICs ranged from 0.65 – 1.00 for 134 models with a median (IQR) discriminatory performance of 0.94 (0.89 – 0.97). Both models based on deep hybrid learning and deep learning had median discrimination metrics (IQR) of 0.96 (0.90 – 0.99) and 0.96 (0.92 – 0.97) which was significantly higher than the median discrimination metrics of traditional ML models 0.93 (0.86 - 0.97) (Figure 6C; p = 0.041). Median accuracies according to the different cancer subtypes and outcomes (cancer screening, diagnosis, and prognosis) from at least three different models are shown in Figures 6D–F. For tumor diagnosis, while most median discrimination metrics were high, performance was highest for the use of ML models in leukemia diagnosis (0.96 [0.92 – 0.98]) than others. Summary discrimination for the incorporation of ML in cancer screening was higher for breast than cervical cancer screening while using ML-based models among LLMIC populations for breast cancer and head and neck cancer prognostication yielded an equivalent median accuracy of 0.89.
Discussion
Utilization of ML-based models for cancer outcomes in LLMICs
Assessing the methodology and implementation status of ML-based models and platforms for cancer outcomes in LLMICs is crucial to improving the prevention, diagnosis, and treatment of cancer in developing nations considering a predicted rise in disease incidence and mortality. This review found a recent increase in the construction of ML-based models for cancers among LLMIC populations that is in line with the general surge of ML application worldwide (166–168). However, intelligent model applications are chiefly limited to some lower-middle-income countries like India, Iran, Pakistan, and Egypt than others. Our study showed that ML-based models were not being applied for cancer prediction among indigenous populations in about 70 countries with a majority of these found to be within sub-Saharan Africa. This observation is likely inseparable from the overall challenges in health information technology and data management that preclude effective medical data accrual of indigenous populations within these countries (19, 169, 170). This reason is further bolstered by the wide use of public databases for ML modeling of cancer outcomes from authors affiliated with many lower-middle-income countries (19). Other reasons for the low popularity of ML-based models in many LLMICs may include the lack of expertise, personnel, and resources required to generate ML-based cancer models and a lack of awareness by stakeholders on the optimal ability of AI tools in predictive and classification health tasks compared to traditional methods (171, 172).
Evaluating data and ML model construction for cancer outcomes in LLMICs
While standard ML algorithms were mostly used for model construction of cancer outcomes, the application of deep learning and deep hybrid learning models were found to be increasing in LLMICs. Of note, the quality of techniques performed during ML model development as well as the overall risk of bias for the studies was largely unsatisfactory. Though many models had excellent discriminatory performance in this study, this translates that many of these models are not currently streamlined enough to be considered for pivotal evaluation of their clinical impact in cancer management in LLMICs. The suboptimal models in this review were found to be a culmination of one of the following challenges: model overfitting, reduced cohort size, not incorporating methods to augment data or correct outcome imbalance, lack of model stability assessment, and cessation of further model validity assessment using external cohorts (23, 24, 173, 174). It is recommended that future studies involving ML-based models for cancer outcomes in LLMICs consider estimating sufficient cohort sizes for model development and validation while considering dimensionality reduction as well as data augmentation/balancing techniques in the event of reduced patient availability (175–177). Furthermore, other shortfalls may be addressed by compliance with standard guidelines for reporting protocols and studies for clinical models and their performances including the IJMEDI (173), TRIPOD (174, 178), STARD (179), SPIRIT-AI (180), and CONSORT-AI (181) statements.
Data quality assessments such as label parity, noisy data identification, outlier detection, and multicollinearity were sparsely assessed by the ML models. However, assessment of class parity based on the need for correction of imbalanced data which could be deduced from the modeling approach showed that this was infrequently performed when needed in the models. Considering the cohort sizes and the lack of class imbalance correction, this suggests that the quality of data used for ML modeling in LLMICs is low. However, this cannot be verified in the models given that other measures of data quality were not intentionally assessed in this study. This represents an additional task to be conducted in future ML models for cancer outcomes in LLMICs. For structured data, authors of ML-based models should consider the using of opensource quality assessment APIs such as the Data Quality Toolkit (182).
Degree of Implementation of ML-based models for cancer outcomes in LLMICs
Regarding the cancer types for which outcomes were modeled using ML algorithms, breast cancer and head and neck cancer (mostly oral cancer) were the most common in line with the majority of LLMICs represented in this review (1). However, outcomes for some cancers uniformly common in many developing countries and have infectious causes related to poverty and awareness such as Kaposi’s sarcoma, Burkitt’s lymphoma, and gastric cancer have not been considered (1, 183–185). Hopefully, with an increase in cancer ML-based model development among more LLMICs in the future, outcomes for these specific malignancies will be further explored for indigenous patients. Likewise, the future should see an increasing application of ML-based models for other cancers associated with low socioeconomic status like cervical and liver cancer (1).
Only a few models in this review have been optimized for software development and even rare was the impact assessment of this software. Of note, none of the models developed for cancer outcomes among LLMIC populations have been evaluated for clinical efficacy and impact in a randomized controlled trial. This is unsurprising given the recency in which model development and performance were performed. However, stakeholders in LLMICs should ensure that ML-based models for cancer outcomes undergo rigorous evaluation in this manner before clinical implementation if applicable (19, 23, 186). Also, challenges to implementation other than model performance including settings for applications, exact application in cancer care, data standardization, and availability of features for prediction should be assessed during these rigorous evaluations.
Limitations
The findings of this review should be interpreted while considering the following limitations: (i) Our search strategy may have missed studies not indexed in the popular electronic databases used in this study. However, the search was limited to these databases to ensure the inclusion of mostly high-quality studies which were not presented in predatory journals (ii) Articles from LLMICs in satisfactory indigenous journals only available in print forms or reported in national languages other than English may have also been missed (iii) Due to the large number of studies included in the scoping review, the characteristics of individual studies was not provided within the main text but presented in Supplementary Table 4 (iv) This study recognizes that the challenges identified regarding the application of ML-based models for cancer outcomes in LLMICs may not be specific to these areas. Therefore, future studies should focus on comparing the objectives of this review across regions and settings of different income categories.
Conclusions
A recent increase in the application of ML-based prevention and intervention for cancer in LLMICs was observed in this study. This surge was also associated with only little improvement in the implementation progress of ML-based models in a few of these countries. Generally, ML-based models were mostly generated among certain lower-middle-income countries like India, Iran, Pakistan, and Egypt than others and were chiefly employed for diagnostic outcomes. This showcases the need to intensify model development and/or external validation endeavors in all low-income countries and many lower-middle-income countries, especially within sub-Saharan Africa. Overall, given the suboptimal methods used for the development of existing models, their lack of external validation and clinical impact assessments, and the lack of adherence to standard guidelines, many ML-based models for cancer outcomes among indigenous patients in LLMICs are not presently streamlined enough for pivotal evaluation and clinical application. We recommend model retraining using larger sample sizes and intensified external validation practices for ML-based models developed for cancer among LLMIC populations.
Data availability statement
The original contributions presented in the study are included in the article/Supplementary Material. Further inquiries can be directed to the corresponding author.
Author contributions
JA conceptualized the study, performed the search, study selection, risk of bias assessment, and wrote the original draft of the manuscript. AA assisted in study concepts, study selection, risk of bias assessment, and manuscript review and editing. MK-M was involved in study methodology, interpretation of results, machine learning modelling consultations, and manuscript review and editing. PT and Y-XS contributed to the interpretation of results, critical review of the manuscript, and provided supervision. All authors contributed to the article and approved the submitted version.
Conflict of interest
The authors declare that the research was conducted in the absence of any commercial or financial relationships that could be construed as a potential conflict of interest.
Publisher’s note
All claims expressed in this article are solely those of the authors and do not necessarily represent those of their affiliated organizations, or those of the publisher, the editors and the reviewers. Any product that may be evaluated in this article, or claim that may be made by its manufacturer, is not guaranteed or endorsed by the publisher.
Supplementary material
The Supplementary Material for this article can be found online at: https://www.frontiersin.org/articles/10.3389/fonc.2022.976168/full#supplementary-material
References
1. Sung H, Ferlay J, Siegel RL, Laversanne M, Soerjomataram I, Jemal A, et al. Global cancer statistics 2020: GLOBOCAN estimates of incidence and mortality worldwide for 36 cancers in 185 countries. CA Cancer J Clin (2021) 71(3):209–49. doi: 10.3322/caac.21660
2. Krzyszczyk P, Acevedo A, Davidoff EJ, Timmins LM, Marrero-Berrios I, Patel M, et al. The growing role of precision and personalized medicine for cancer treatment. Technol (Singap World Sci) (2018) 6(3-4):79–100. doi: 10.1142/S2339547818300020
3. Shin SH, Bode AM, Dong Z. Precision medicine: the foundation of future cancer therapeutics. Precis Oncol (2017) 1(1):12. doi: 10.1038/s41698-017-0016-z
4. Tsimberidou AM, Eggermont AM, Schilsky RL. Precision cancer medicine: the future is now, only better. Am Soc Clin Oncol Educ Book (2014) 34:61–9. doi: 10.14694/EdBook_AM.2014.34.61
5. Bhinder B, Gilvary C, Madhukar NS, Elemento O. Artificial intelligence in cancer research and precision medicine. Cancer Discovery (2021) 11(4):900–15. doi: 10.1158/2159-8290.CD-21-0090
6. Dlamini Z, Francies FZ, Hull R, Marima R. Artificial intelligence (AI) and big data in cancer and precision oncology. Comput Struct Biotechnol J (2020) 18:2300–11. doi: 10.1016/j.csbj.2020.08.019
7. Cuocolo R, Caruso M, Perillo T, Ugga L, Petretta M. Machine learning in oncology: A clinical appraisal. Cancer Lett (2020) 481:55–62. doi: 10.1016/j.canlet.2020.03.032
8. Fessele KL. The rise of big data in oncology. Semin Oncol Nurs (2018) 34(2):168–76. doi: 10.1016/j.soncn.2018.03.008
9. Farina E, Nabhen JJ, Dacoregio MI, Batalini F, Moraes FY. An overview of artificial intelligence in oncology. Future Sci OA (2022) 8(4):FSO787. doi: 10.2144/fsoa-2021-0074
10. Chua IS, Gaziel-Yablowitz M, Korach ZT, Kehl KL, Levitan NA, Arriaga YE, et al. Artificial intelligence in oncology: Path to implementation. Cancer Med (2021) 10(12):4138–49. doi: 10.1002/cam4.3935
11. Hamamoto R, Suvarna K, Yamada M, Kobayashi K, Shinkai N, Miyake M, et al. Application of artificial intelligence technology in oncology: Towards the establishment of precision medicine. Cancers (Basel) (2020) 12(12):3532. doi: 10.3390/cancers12123532
12. Xu L, Sanders L, Li K, Chow JCL. Chatbot for health care and oncology applications using artificial intelligence and machine learning: Systematic review. JMIR Cancer (2021) 7(4):e27850-e. doi: 10.2196/27850
13. Hu Y, Strong VE. Robotic surgery and oncologic outcomes. JAMA Oncol (2020) 6(10):1537–9. doi: 10.1001/jamaoncol.2020.2996
14. Zhang H, Zhang N, Wu W, Zhou R, Li S, Wang Z, et al. Machine learning-based tumor-infiltrating immune cell-associated lncRNAs for predicting prognosis and immunotherapy response in patients with glioblastoma. Briefings Bioinf (2022) 23 (6):bbac386. doi: 10.1093/bib/bbac386
15. Zhang N, Zhang H, Wu W, Zhou R, Li S, Wang Z, et al. Machine learning-based identification of tumor-infiltrating immune cell-associated lncRNAs for improving outcomes and immunotherapy responses in patients with low-grade glioma. Theranostics (2022) 12(13):5931–48. doi: 10.7150/thno.74281
16. Kourou K, Exarchos KP, Papaloukas C, Sakaloglou P, Exarchos T, Fotiadis DI. Applied machine learning in cancer research: A systematic review for patient diagnosis, classification and prognosis. Comput Struct Biotechnol J (2021) 19:5546–55. doi: 10.1016/j.csbj.2021.10.006
17. Lehmann J, Cofala T, Tschuggnall M, Giesinger JM, Rumpold G, Holzner B. Machine learning in oncology–perspectives in patient-reported outcome research. Der Onkologe (2021) 27(2):150–5. doi: 10.1007/s00761-021-00916-9
18. Bertsimas D, Wiberg H. Machine learning in oncology: Methods, applications, and challenges. JCO Clin Cancer Inf (2020) 4):885–94. doi: 10.1200/CCI.20.00072
19. Adeoye J, Akinshipo A, Thomson P, Su Y-X. Artificial intelligence-based prediction for cancer-related outcomes in Africa: Status and potential refinements. J Glob Health (2022) 12:03017–. doi: 10.7189/jogh.12.03017
20. Kelly CJ, Karthikesalingam A, Suleyman M, Corrado G, King D. Key challenges for delivering clinical impact with artificial intelligence. BMC Med (2019) 17(1):195. doi: 10.1186/s12916-019-1426-2
21. Riley RD, Ensor J, Snell KIE, Debray TPA, Altman DG, Moons KGM, et al. External validation of clinical prediction models using big datasets from e-health records or IPD meta-analysis: opportunities and challenges. BMJ (2016) 353:i3140. doi: 10.1136/bmj.i3140
22. The World Bank. World bank country and lending groups 2022 (2022). Available at: https://datahelpdesk.worldbank.org/knowledgebase/articles/906519-world-bank-country-and-lending-groups.
23. de Hond AAH, Leeuwenberg AM, Hooft L, Kant IMJ, Nijman SWJ, van Os HJA, et al. Guidelines and quality criteria for artificial intelligence-based prediction models in healthcare: A scoping review. NPJ Digit Med (2022) 5(1):2. doi: 10.1038/s41746-021-00549-7
24. Moons KGM, Wolff RF, Riley RD, Whiting PF, Westwood M, Collins GS, et al. PROBAST: A tool to assess risk of bias and applicability of prediction model studies: Explanation and elaboration. Ann Intern Med (2019) 170(1):W1–w33. doi: 10.7326/M18-1377
25. Tricco AC, Lillie E, Zarin W, O'Brien KK, Colquhoun H, Levac D, et al. PRISMA extension for scoping reviews (PRISMA-ScR): Checklist and explanation. Ann Intern Med (2018) 169(7):467–73. doi: 10.7326/M18-0850
26. Aatresh AA, Alabhya K, Lal S, Kini J, Saxena PUP. LiverNet: efficient and robust deep learning model for automatic diagnosis of sub-types of liver hepatocellular carcinoma cancer from H&E stained liver histopathology images. Int J Comput Assist Radiol Surg (2021) 16(9):1549–63. doi: 10.1007/s11548-021-02410-4
27. Abdollahi H, Mahdavi SR, Mofid B, Bakhshandeh M, Razzaghdoust A, Saadipoor A, et al. Rectal wall MRI radiomics in prostate cancer patients: prediction of and correlation with early rectal toxicity. Int J Radiat Biol (2018) 94(9):829–37. doi: 10.1080/09553002.2018.1492756
28. Abdollahi H, Mofid B, Shiri I, Razzaghdoust A, Saadipoor A, Mahdavi A, et al. Machine learning-based radiomic models to predict intensity-modulated radiation therapy response, Gleason score and stage in prostate cancer. Radiol Med (2019) 124(6):555–67. doi: 10.1007/s11547-018-0966-4
29. Abdollahi H, Mostafaei S, Cheraghi S, Shiri I, Rabi Mahdavi S, Kazemnejad A. Cochlea CT radiomics predicts chemoradiotherapy induced sensorineural hearing loss in head and neck cancer patients: A machine learning and multi-variable modelling study. Phys Med (2018) 45:192–7. doi: 10.1016/j.ejmp.2017.10.008
30. Acharya V, Ravi V, Pham TD, Chakraborty C. Peripheral blood smear analysis using automated computer-aided diagnosis system to identify acute myeloid leukemia. IEEE Trans Eng Manage. (2021) 1–14. doi: 10.1109/TEM.2021.3103549
31. Agarwal P, Yadav A, Mathur P, Pal V, Chakrabarty A. BID-net: An automated system for bone invasion detection occurring at stage T4 in oral squamous carcinoma using deep learning. Comput Intell Neurosci (2022) 2022:4357088. doi: 10.1155/2022/4357088
32. Ahammed Muneer KV, Rajendran VR, K PJ. Glioma tumor grade identification using artificial intelligent techniques. J Med Syst (2019) 43(5):113. doi: 10.1007/s10916-019-1228-2
33. Ahmed J, Rehman MA. CANCER PREVENTION INITIATIVE: AN INTELLIGENT APPROACH FOR THYROID CANCER TYPE DIAGNOSTICS. Jurnal Teknologi (2016) 78(4-3):31–9. doi: 10.11113/jt.v78.8237
34. Al Amin A, Parvin S, Kadir MA, Tahmid T, Alam SK, Siddique-e Rabbani K. Classification of breast tumour using electrical impedance and machine learning techniques. Physiol Meas (2014) 35(6):965–74. doi: 10.1088/0967-3334/35/6/965
35. Al-Dhabyani W, Fahmy A, Gomaa M, Khaled H. Deep learning approaches for data augmentation and classification of breast masses using ultrasound images. Int J Adv Comput Sci Appl (2019) 10(5):618–27. doi: 10.14569/IJACSA.2019.0100579
36. Ali M, Sarwar A, Sharma V, Suri J. Artificial neural network based screening of cervical cancer using a hierarchical modular neural network architecture (HMNNA) and novel benchmark uterine cervix cancer database. Neural Comput Appl (2019) 31(7):2979–93. doi: 10.1007/s00521-017-3246-7
37. Allahloubi NMA, Zekri ARN, Ragab M, Mohanad M, Ahmed OS, Eid S, et al. Estrogen receptor gene polymorphism as a possible genetic risk factor for treatment response in ER-positive breast cancer patients. Biochem Genet (2022) 60(6):1963–85. doi: 10.1007/s10528-022-10199-3
38. Amin J, Sharif M, Raza M, Saba T, Anjum MA. Brain tumor detection using statistical and machine learning method. Comput Methods Programs Biomed (2019) 177:69–79. doi: 10.1016/j.cmpb.2019.05.015
39. Amiri S, Akbarabadi M, Abdolali F, Nikoofar A, Esfahani AJ, Cheraghi S. Radiomics analysis on CT images for prediction of radiation-induced kidney damage by machine learning models. Comput Biol Med (2021) 133:104409. doi: 10.1016/j.compbiomed.2021.104409
40. Ara SR, Alam F, Rahman MH, Akhter S, Awwal R, Hasan K. Bimodal multiparameter-based approach for benign-malignant classification of breast tumors. Ultrasound Med Biol (2015) 41(7):2022–38. doi: 10.1016/j.ultrasmedbio.2015.01.023
41. Asaduzzaman S, Ahmed MR, Rehana H, Chakraborty S, Islam MS, Bhuiyan T. Machine learning to reveal an astute risk predictive framework for gynecologic cancer and its impact on women psychology: Bangladeshi perspective. BMC Bioinf (2021) 22(1):213. doi: 10.1186/s12859-021-04131-6
42. Assari Z, Mahloojifar A, Ahmadinejad N. A bimodal BI-RADS-guided GoogLeNet-based CAD system for solid breast masses discrimination using transfer learning. Comput Biol Med (2022) 142: 105160. doi: 10.1016/j.compbiomed.2021.105160
43. Asuntha A, Srinivasan A. Deep learning for lung cancer detection and classification. Multimed Tools Appl (2020) 79(11-12):7731–62. doi: 10.1007/s11042-019-08394-3
44. Aswiga RV, Aishwarya R, Shanthi AP. Augmenting transfer learning with feature extraction techniques for limited breast imaging datasets. J Digit Imaging (2021) 34(3):618–29. doi: 10.1007/s10278-021-00456-z
45. Ayyad SM, Badawy MA, Shehata M, Alksas A, Mahmoud A, Abou El-Ghar M, et al. A new framework for precise identification of prostatic adenocarcinoma. Sensors (Basel) (2022) 22(5): 1848. doi: 10.3390/s22051848
46. Babu T, Singh T, Gupta D, Hameed S. Colon cancer prediction on histological images using deep learning features and Bayesian optimized SVM. J Intell Fuzzy Syst (2021) 41(5):5275–86. doi: 10.3233/JIFS-189850
47. Bakre MM, Ramkumar C, Attuluri AK, Basavaraj C, Prakash C, Buturovic L, et al. Clinical validation of an immunohistochemistry-based CanAssist-breast test for distant recurrence prediction in hormone receptor-positive breast cancer patients. Cancer Med (2019) 8(4):1755–64. doi: 10.1002/cam4.2049
48. Bal A, Das M, Satapathy SM, Jena M, Das SK. BFCNet: a CNN for diagnosis of ductal carcinoma in breast from cytology images. Pattern Anal Appl (2021) 24(3):967–80. doi: 10.1007/s10044-021-00962-4
49. Ben Youssef Y, Abdelmounim E, Zbitou J, Elharoussi M, Boujida MN. Comparison machine learning algorithms in abnormal mammograms classification. Int J Comput Sci Netw Secur (2017) 17(5):19–25.
50. Bhowmik A, Ghosh B, Pal M, Paul RR, Chatterjee J, Chakraborty S. Portable, handheld, and affordable blood perfusion imager for screening of subsurface cancer in resource-limited settings. Proc Natl Acad Sci U.S.A. (2022) 119(2): e2026201119. doi: 10.1073/pnas.2026201119
51. Binson VA, Subramoniam M, Mathew L. Noninvasive detection of COPD and lung cancer through breath analysis using MOS sensor array based e-nose. Expert Rev Mol Diagn (2021) 21(11):1223–33. doi: 10.1080/14737159.2021.1971079
52. Binson VA, Subramoniam M, Sunny Y, Mathew L. Prediction of pulmonary diseases with electronic nose using SVM and XGBoost. IEEE Sensors J (2021) 21(18):20886–95. doi: 10.1109/JSEN.2021.3100390
53. Buddhavarapu VG, Jothi JAA. An experimental study on classification of thyroid histopathology images using transfer learning. Pattern Recognit Lett (2020) 140:1–9. doi: 10.1016/j.patrec.2020.09.020
54. Bukhari SUK, Bokhari SKA, Syed A, Hussain SS, Armaghan SU, Shah SSH. The diagnostic accuracy of convolutional neural network architectures for the diagnosis of brain cancer. Pakistan J Med Health Sci (2020) 14(3):1037–9.
55. Butola A, Ahmad A, Dubey V, Srivastava V, Qaiser D, Srivastava A, et al. Volumetric analysis of breast cancer tissues using machine learning and swept-source optical coherence tomography. Appl Opt (2019) 58(5):A135–a41. doi: 10.1364/AO.58.00A135
56. Chandra Doval D, Mehta A, Somashekhar SP, Gunda A, Singh G, Bal A, et al. The usefulness of CanAssist breast in the assessment of recurrence risk in patients of ethnic Indian origin. Breast (2021) 59:1–7. doi: 10.1016/j.breast.2021.05.007
57. Chandra Joshi R, Mishra R, Gandhi P, Pathak VK, Burget R, Dutta MK. Ensemble based machine learning approach for prediction of glioma and multi-grade classification. Comput Biol Med (2021) 137:104829. doi: 10.1016/j.compbiomed.2021.104829
58. Daoud B, Morooka K, Kurazume R, Leila F, Mnejja W, Daoud J. 3D segmentation of nasopharyngeal carcinoma from CT images using cascade deep learning. Comput Med Imaging Graph (2019) 77:101644. doi: 10.1016/j.compmedimag.2019.101644
59. Das A, Acharya UR, Panda SS, Sabut S. Deep learning based liver cancer detection using watershed transform and Gaussian mixture model techniques. Cogn Syst Res (2019) 54:165–75. doi: 10.1016/j.cogsys.2018.12.009
60. Das D, Mahanta LB. A comparative assessment of different approaches of segmentation and classification methods on childhood medulloblastoma images. J Med Biol Eng (2021) 41(3):379–92. doi: 10.1007/s40846-021-00612-4
61. Das DK, Mitra P, Chakraborty C, Chatterjee S, Maiti AK, Bose S. Computational approach for mitotic cell detection and its application in oral squamous cell carcinoma. Multidimens Syst Signal Process (2017) 28(3):1031–50. doi: 10.1007/s11045-017-0488-6
62. Das N, Hussain E, Mahanta LB. Automated classification of cells into multiple classes in epithelial tissue of oral squamous cell carcinoma using transfer learning and convolutional neural network. Neural Netw (2020) 128:47–60. doi: 10.1016/j.neunet.2020.05.003
63. Dese K, Raj H, Ayana G, Yemane T, Adissu W, Krishnamoorthy J, et al. Accurate machine-Learning-Based classification of leukemia from blood smear images. Clin Lymphoma Myeloma Leuk (2021) 21(11):e903–e14. doi: 10.1016/j.clml.2021.06.025
64. Devakumar D, Sunny G, Sasidharan BK, Bowen SR, Nadaraj A, Jeyseelan L, et al. Framework for machine learning of CT and PET radiomics to predict local failure after radiotherapy in locally advanced head and neck cancers. J Med Phys (2021) 46(3):181–8. doi: 10.4103/jmp.JMP_6_21
65. Dheeba J, Jaya T, Singh NA. Breast cancer risk assessment and diagnosis model using fuzzy support vector machine based expert system. J Exp Theor Artif Intell (2017) 29(5):1011–21. doi: 10.1080/0952813X.2017.1280088
66. El Agouri H, Azizi M, El Attar H, El Khannoussi M, Ibrahimi A, Kabbaj R, et al. Assessment of deep learning algorithms to predict histopathological diagnosis of breast cancer: first Moroccan prospective study on a private dataset. BMC Res Notes (2022) 15(1):66. doi: 10.1186/s13104-022-05936-1
67. Fathi E, Rezaee MJ, Tavakkoli-Moghaddam R, Alizadeh A, Montazer A. Design of an integrated model for diagnosis and classification of pediatric acute leukemia using machine learning. Proc Inst Mech Eng H. (2020) 234(10):1051–69. doi: 10.1177/0954411920938567
68. Fathi Kazerooni A, Nabil M, Zeinali Zadeh M, Firouznia K, Azmoudeh-Ardalan F, Frangi AF, et al. Characterization of active and infiltrative tumorous subregions from normal tissue in brain gliomas using multiparametric MRI. J Magn Reson Imaging (2018) 48(4):938–50. doi: 10.1002/jmri.25963
69. Ferjaoui R, Cherni MA, Boujnah S, Kraiem NEH, Kraiem T. Machine learning for evolutive lymphoma and residual masses recognition in whole body diffusion weighted magnetic resonance images. Comput Methods Programs Biomed (2021) 209:106320. doi: 10.1016/j.cmpb.2021.106320
70. Figueroa KC, Song B, Sunny S, Li S, Gurushanth K, Mendonca P, et al. Interpretable deep learning approach for oral cancer classification using guided attention inference network. J BioMed Opt (2022) 27(1): 015001. doi: 10.1117/1.JBO.27.1.015001
71. Gangil T, Shahabuddin AB, Rao BD, Palanisamy K, Chakrabarti B, Sharan K. Predicting clinical outcomes of radiotherapy for head and neck squamous cell carcinoma patients using machine learning algorithms. J Big Data (2022) 9(1): 1–19. doi: 10.1186/s40537-022-00578-3
72. Gehlot S, Gupta A, Gupta R. A CNN-based unified framework utilizing projection loss in unison with label noise handling for multiple myeloma cancer diagnosis. Med Image Anal (2021) 72:102099. doi: 10.1016/j.media.2021.102099
73. Giri S, Bose JC, Chandrasekar A, Tiwary BK, Gajalakshmi P, Chatterjee S. Increased plasma nitrite and von willebrand factor indicates early diagnosis of vascular diseases in chemotherapy treated cancer patients. Cardiovasc Toxicol (2019) 19(1):36–47. doi: 10.1007/s12012-018-9471-1
74. Gnanasekaran VS, Joypaul S, Sundaram PM, Chairman DD. Deep learning algorithm for breast masses classification in mammograms. Iet Image Process (2020) 14(12):2860–8. doi: 10.1049/iet-ipr.2020.0070
75. Gollapalli K, Ray S, Srivastava R, Renu D, Singh P, Dhali S, et al. Investigation of serum proteome alterations in human glioblastoma multiforme. Proteomics (2012) 12(14):2378–90. doi: 10.1002/pmic.201200002
76. Gupta M, Gupta A, Yadav V, Parvaze SP, Singh A, Saini J, et al. Comparative evaluation of intracranial oligodendroglioma and astrocytoma of similar grades using conventional and T1-weighted DCE-MRI. Neuroradiology (2021) 63(8):1227–39. doi: 10.1007/s00234-021-02636-8
77. Hammouda K, Khalifa F, Soliman A, Ghazal M, El-Ghar MA, Badawy MA, et al. A multiparametric MRI-based CAD system for accurate diagnosis of bladder cancer staging. Comput Med Imaging Graph (2021) 90:101911. doi: 10.1016/j.compmedimag.2021.101911
78. Hashem S, ElHefnawi M, Habashy S, El-Adawy M, Esmat G, Elakel W, et al. Machine learning prediction models for diagnosing hepatocellular carcinoma with HCV-related chronic liver disease. Comput Methods Programs Biomed (2020) 196:105551. doi: 10.1016/j.cmpb.2020.105551
79. Holmström O, Linder N, Kaingu H, Mbuuko N, Mbete J, Kinyua F, et al. Point-of-Care digital cytology with artificial intelligence for cervical cancer screening in a resource-limited setting. JAMA Netw Open (2021) 4(3):e211740. doi: 10.1001/jamanetworkopen.2021.1740
80. Hussain E, Mahanta LB, Das CR, Talukdar RK. A comprehensive study on the multi-class cervical cancer diagnostic prediction on pap smear images using a fusion-based decision from ensemble deep convolutional neural network. Tissue Cell (2020) 65:101347. doi: 10.1016/j.tice.2020.101347
81. Jajodia A, Gupta A, Prosch H, Mayerhoefer M, Mitra S, Pasricha S, et al. Combination of radiomics and machine learning with diffusion-weighted MR imaging for clinical outcome prognostication in cervical cancer. Tomography (2021) 7(3):344–57. doi: 10.3390/tomography7030031
82. Jajroudi M, Enferadi M, Homayoun AA, Reiazi R. MRI-Based machine learning for determining quantitative and qualitative characteristics affecting the survival of glioblastoma multiforme. MagnetReson Imaging (2022) 85:222–7. doi: 10.1016/j.mri.2021.10.023
83. James BL, Sunny SP, Heidari AE, Ramanjinappa RD, Lam T, Tran AV, et al. Validation of a point-of-Care optical coherence tomography device with machine learning algorithm for detection of oral potentially malignant and malignant lesions. Cancers (2021) 13(14): 3583. doi: 10.3390/cancers13143583
84. Jha S, Mehta AK. An evolutionary algorithm based feature selection and fuzzy rule reduction technique for the prediction of skin cancer. Concurr Comput-Pract Exp (2022) 34(5): e6694. doi: 10.1002/cpe.6694
85. Kakileti ST, Madhu HJ, Krishnan L, Manjunath G, Sampangi S, Ramprakash HV. Observational study to evaluate the clinical efficacy of thermalytix for detecting breast cancer in symptomatic and asymptomatic women. JCO Glob Oncol (2020) 6:1472–80. doi: 10.1200/GO.20.00168
86. Kakileti ST, Madhu HJ, Manjunath G, Wee L, Dekker A, Sampangi S. Personalized risk prediction for breast cancer pre-screening using artificial intelligence and thermal radiomics. Artif Intell Med (2020) 105:101854. doi: 10.1016/j.artmed.2020.101854
87. Kamath SD, Mahato KK. Optical pathology using oral tissue fluorescence spectra: classification by principal component analysis and k-means nearest neighbor analysis. J BioMed Opt (2007) 12(1):014028. doi: 10.1117/1.2437738
88. Kamath SD, Ray S, Mahato KK. Photoacoustic spectroscopy of ovarian normal, benign, and malignant tissues: a pilot study. J BioMed Opt (2011) 16(6):067001. doi: 10.1117/1.3583573
89. Kansal S, Goel S, Bhattacharya J, Srivastava V. Generative adversarial network-convolution neural network based breast cancer classification using optical coherence tomographic images. Laser Phys (2020) 30(11): 115601. doi: 10.1088/1555-6611/abb596
90. Kashef A, Khatibi T, Mehrvar A. Prediction of cranial radiotherapy treatment in pediatric acute lymphoblastic leukemia patients using machine learning: A case study at MAHAK hospital. Asian Pac J Cancer Prev (2020) 21(11):3211–9. doi: 10.31557/APJCP.2020.21.11.3211
91. Kaur I, Doja MN, Ahmad T. Time-range based sequential mining for survival prediction in prostate cancer. J BioMed Inform (2020) 110:103550. doi: 10.1016/j.jbi.2020.103550
92. Kaur I, Doja MN, Ahmad T, Ahmad M, Hussain A, Nadeem A, et al. An integrated Approach for cancer survival prediction using data mining techniques. Comput Intell Neurosci (2021) 2021:6342226. doi: 10.1155/2021/6342226
93. Kaur R, Juneja M, Mandal AK. Machine learning based quantitative texture analysis of CT images for diagnosis of renal lesions. Biomed Signal Process Control (2021) 64: 102311. doi: 10.1016/j.bspc.2020.102311
94. Kaushik M, Chandra Joshi R, Kushwah AS, Gupta MK, Banerjee M, Burget R, et al. Cytokine gene variants and socio-demographic characteristics as predictors of cervical cancer: A machine learning approach. Comput Biol Med (2021) 134:104559. doi: 10.1016/j.compbiomed.2021.104559
95. Kayal EB, Kandasamy D, Sharma R, Bakhshi S, Mehndiratta A. Segmentation of osteosarcoma tumor using diffusion weighted MRI: a comparative study using nine segmentation algorithms. Signal Image Video Process (2020) 14(4):727–35. doi: 10.1007/s11760-019-01599-x
96. Khan SU, Islam N, Jan Z, Din IU, Khan A, Faheem Y. An e-health care services framework for the detection and classification of breast cancer in breast cytology images as an IoMT application. Future Gener Comput Syst-the Int J Esci (2019) 98:286–96. doi: 10.1016/j.future.2019.01.033
97. Lal S, Das D, Alabhya K, Kanfade A, Kumar A, Kini J. NucleiSegNet: Robust deep learning architecture for the nuclei segmentation of liver cancer histopathology images. Comput Biol Med (2021) 128:104075. doi: 10.1016/j.compbiomed.2020.104075
98. Lotfnezhad Afshar H, Jabbari N, Khalkhali HR, Esnaashari O. Prediction of breast cancer survival by machine learning methods: An application of multiple imputation. Iranian J Public Health (2021) 50(3):598–605. doi: 10.18502/ijph.v50i3.5606
99. Macaulay BO, Aribisala BS, Akande SA, Akinnuwesi BA, Olabanjo OA. Breast cancer risk prediction in African women using random forest classifier. Cancer Treat Res Commun (2021) 28:100396. doi: 10.1016/j.ctarc.2021.100396
100. Mahanta LB, Hussain E, Das N, Kakoti L, Chowdhury M. IHC-net: A fully convolutional neural network for automated nuclear segmentation and ensemble classification for allred scoring in breast pathology. Appl Soft Comput (2021) 103: 107136. doi: 10.1016/j.asoc.2021.107136
101. Mahmood N, Shahid S, Bakhshi T, Riaz S, Ghufran H, Yaqoob M. Identification of significant risks in pediatric acute lymphoblastic leukemia (ALL) through machine learning (ML) approach. Med Biol Eng Comput (2020) 58(11):2631–40. doi: 10.1007/s11517-020-02245-2
102. Mahmood T, Li J, Pei Y, Akhtar F, Rehman MU, Wasti SH. Breast lesions classifications of mammographic images using a deep convolutional neural network-based approach. PloS One (2022) 17(1):e0263126. doi: 10.1371/journal.pone.0263126
103. Majumder B, Baraneedharan U, Thiyagarajan S, Radhakrishnan P, Narasimhan H, Dhandapani M, et al. Predicting clinical response to anticancer drugs using an ex vivo platform that captures tumour heterogeneity. Nat Commun (2015) 6:6169. doi: 10.1038/ncomms7169
104. Majumder SK, Ghosh N, Gupta PK. Relevance vector machine for optical diagnosis of cancer. Lasers Surg Med (2005) 36(4):323–33. doi: 10.1002/lsm.20160
105. Malek M, Gity M, Alidoosti A, Oghabian Z, Rahimifar P, Seyed Ebrahimi SM, et al. A machine learning approach for distinguishing uterine sarcoma from leiomyomas based on perfusion weighted MRI parameters. Eur J Radiol (2019) 110:203–11. doi: 10.1016/j.ejrad.2018.11.009
106. Malek M, Tabibian E, Rahimi Dehgolan M, Rahmani M, Akhavan S, Sheikh Hasani S, et al. A diagnostic algorithm using multi-parametric MRI to differentiate benign from malignant myometrial tumors: Machine-learning method. Sci Rep (2020) 10(1):7404. doi: 10.1038/s41598-020-64285-w
107. Mathialagan P, Chidambaranathan M. Computer vision techniques for upper aero-digestive tract tumor grading classification-addressing pathological challenges. Pattern Recognit Lett (2021) 144:42–53. doi: 10.1016/j.patrec.2021.01.002
108. Mohamed N, van de Goor R, El-Sheikh M, Elrayah O, Osman T, Nginamau ES, et al. Feasibility of a portable electronic nose for detection of oral squamous cell carcinoma in Sudan. Healthc (Basel) (2021) 9(5): 534. doi: 10.3390/healthcare9050534
109. Mohebian MR, Marateb HR, Mansourian M, Mananas MA, Mokarian F. A hybrid computer-aided-diagnosis system for prediction of breast cancer recurrence (HPBCR) using optimized ensemble learning. Comput Struct Biotechnol J (2017) 15:75–85. doi: 10.1016/j.csbj.2016.11.004
110. Mokni R, Gargouri N, Damak A, Sellami D, Feki W, Mnif Z. An automatic computer-aided diagnosis system based on the multimodal fusion of breast cancer (MF-CAD). Biomed Signal Process Control (2021) 69: 102914. doi: 10.1016/j.bspc.2021.102914
111. Montazeri M, Montazeri M, Montazeri M, Beigzadeh A. Machine learning models in breast cancer survival prediction. Technol Health Care (2016) 24(1):31–42. doi: 10.3233/THC-151071
112. Mostafaei S, Abdollahi H, Dehkordi SK, Shiri I, Razzaghdoust A, Moghaddam SHZ, et al. CT imaging markers to improve radiation toxicity prediction in prostate cancer radiotherapy by stacking regression algorithm. Radiol Medica (2020) 125(1):87–97. doi: 10.1007/s11547-019-01082-0
113. Mungle T, Tewary S, Das DK, Arun I, Basak B, Agarwal S, et al. MRF-ANN: a machine learning approach for automated ER scoring of breast cancer immunohistochemical images. J Microsc (2017) 267(2):117–29. doi: 10.1111/jmi.12552
114. Nachimuthu DS, Baladhandapani A. Multidimensional texture characterization: on analysis for brain tumor tissues using MRS and MRI. J Digit Imaging (2014) 27(4):496–506. doi: 10.1007/s10278-013-9669-5
115. Naeem S, Ali A, Qadri S, Mashwani WK, Tairan N, Shah H, et al. Machine-learning based hybrid-feature analysis for liver cancer classification using fused (MR and CT) images. Appl Sci-Basel (2020) 10(9): 3134. doi: 10.3390/app10093134
116. Nanditha BR, Kiran AG, Chandrashekar HS, Dinesh MS, Murali S. An ensemble deep neural network approach for oral cancer screening. Int J Online Biomed Eng (2021) 17(2):121–34. doi: 10.3991/ijoe.v17i02.19207
117. Nassar A, Lymona AM, Lotfy MM, Youssef ASE, Mohanad M, Manie TM, et al. Tumor mutation burden prediction model in Egyptian breast cancer patients based on next generation sequencing. Asian Pac J Cancer Prev (2021) 22(7):2053–9. doi: 10.31557/APJCP.2021.22.7.2053
118. Naushad SM, Dorababu P, Rupasree Y, Pavani A, Raghunadharao D, Hussain T, et al. Classification and regression tree-based prediction of 6-mercaptopurine-induced leucopenia grades in children with acute lymphoblastic leukemia. Cancer Chemother Pharmacol (2019) 83(5):875–80. doi: 10.1007/s00280-019-03803-8
119. Nawandhar A, Kumar N, Veena R, Yamujala L. Stratified squamous epithelial biopsy image classifier using machine learning and neighborhood feature selection. Biomed Signal Process Control (2020) 55: 101671. doi: 10.1016/j.bspc.2019.101671
120. Nayak A, Baidya Kayal E, Arya M, Culli J, Krishan S, Agarwal S, et al. Computer-aided diagnosis of cirrhosis and hepatocellular carcinoma using multi-phase abdomen CT. Int J Comput Assist Radiol Surg (2019) 14(8):1341–52. doi: 10.1007/s11548-019-01991-5
121. Negahbani F, Sabzi R, Pakniyat Jahromi B, Firouzabadi D, Movahedi F, Kohandel Shirazi M, et al. PathoNet introduced as a deep neural network backend for evaluation of ki-67 and tumor-infiltrating lymphocytes in breast cancer. Sci Rep (2021) 11(1):8489. doi: 10.1038/s41598-021-86912-w
122. Nisa M, Buzdar SA, Khan K, Ahmad MS. Deep convolutional neural network based analysis of liver tissues using computed tomography images. Symmetry-Basel (2022) 14(2): 383. doi: 10.3390/sym14020383
123. Panigrahi S, Bhuyan R, Kumar K, Nayak J, Swarnkar T. Multistage classification of oral histopathological images using improved residual network. Math Biosci Eng (2022) 19(2):1909–25. doi: 10.3934/mbe.2022090
124. Paul A, Srivastava S, Roy R, Anand A, Gaurav K, Husain N, et al. Malignancy prediction among tissues from oral SCC patients including neck invasions: a (1)H HRMAS NMR based metabolomic study. Metabolomics (2020) 16(3):38. doi: 10.1007/s11306-020-01660-8
125. Paydar S, Pourahmad S, Azad M, Bolandparvaz S, Taheri R, Ghahramani Z, et al. The evolution of a malignancy risk prediction model for thyroid nodules using the artificial neural network. Middle East J Cancer (2016) 7(1):47–52.
126. Punithavathy K, Poobal S, Ramya MM. Performance evaluation of machine learning techniques in lung cancer classification from PET/CT images. Fme Trans (2019) 47(3):418–23. doi: 10.5937/fmet1903418P
127. Rahman TY, Mahanta LB, Das AK, Sarma JD. Automated oral squamous cell carcinoma identification using shape, texture and color features of whole image strips. Tissue Cell (2020) 63:101322. doi: 10.1016/j.tice.2019.101322
128. Ramkumar C, Buturovic L, Malpani S, Attuluri AK, Basavaraj C, Prakash C, et al. Development of a novel proteomic risk-classifier for prognostication of patients with early-stage hormone receptor-positive breast cancer. biomark Insights (2018) 13: 1177271918789100. doi: 10.1177/1177271918789100
129. Ranjith G, Parvathy R, Vikas V, Chandrasekharan K, Nair S. Machine learning methods for the classification of gliomas: Initial results using features extracted from MR spectroscopy. Neuroradiol J (2015) 28(2):106–11. doi: 10.1177/1971400915576637
130. Rashmi R, Prasad K, Udupa CBK. BCHisto-net: Breast histopathological image classification by global and local feature aggregation. Artif Intell Med (2021) 121:102191. doi: 10.1016/j.artmed.2021.102191
131. Reda I, Khalil A, Elmogy M, Abou El-Fetouh A, Shalaby A, Abou El-Ghar M, et al. Deep learning role in early diagnosis of prostate cancer. Technol Cancer Res Treat (2018) 17:1533034618775530. doi: 10.1177/1533034618775530
132. Reda I, Shalaby A, Elmogy M, Elfotouh AA, Khalifa F, El-Ghar MA, et al. A comprehensive non-invasive framework for diagnosing prostate cancer. Comput Biol Med (2017) 81:148–58. doi: 10.1016/j.compbiomed.2016.12.010
133. Rehman A, Abbas N, Saba T, Rahman SIU, Mehmood Z, Kolivand H. Classification of acute lymphoblastic leukemia using deep learning. Microsc Res Tech (2018) 81(11):1310–7. doi: 10.1002/jemt.2313
134. Rehman KU, Li JQ, Pei Y, Yasin A, Ali S, Saeed Y. Architectural distortion-based digital mammograms classification using depth wise convolutional neural network. Biology-Basel (2022) 11(1): 15. doi: 10.3390/biology11010015
135. Sadewo W, Rustam Z, Hamidah H, Chusmarsyah AR. Pancreatic cancer early detection using twin support vector machine based on kernel. Symmetry-Basel (2020) 12(4): 667. doi: 10.3390/sym12040667
136. Saha M, Chakraborty C, Arun I, Ahmed R, Chatterjee S. An advanced deep learning approach for ki-67 stained hotspot detection and proliferation rate scoring for prognostic evaluation of breast cancer. Sci Rep (2017) 7(1):3213. doi: 10.1038/s41598-017-03405-5
137. Saikia AR, Bora K, Mahanta LB, Das AK. Comparative assessment of CNN architectures for classification of breast FNAC images. Tissue Cell (2019) 57:8–14. doi: 10.1016/j.tice.2019.02.001
138. Samuri SM, Nova TV, Rahmatullah B, Li WS, Al-Qaysi ZT. CLASSIFICATION MODEL FOR BREAST CANCER MAMMOGRAMS. Iium Eng J (2022) 23(1):187–99. doi: 10.31436/iiumej.v23i1.1825
139. Sanyal P, Barui S, Deb P, Sharma HC. Performance of a convolutional neural network in screening liquid based cervical cytology smears. J Cytol (2019) 36(3):146–51. doi: 10.4103/JOC.JOC_201_18
140. Sathya DJ, Geetha K. Mass classification in breast DCE-MR images using an artificial neural network trained via a bee colony optimization algorithm. Scienceasia (2013) 39(3):294–305. doi: 10.2306/scienceasia1513-1874.2013.39.294
141. Savala R, Dey P, Gupta N. Artificial neural network model to distinguish follicular adenoma from follicular carcinoma on fine needle aspiration of thyroid. Diagn Cytopathol (2018) 46(3):244–9. doi: 10.1002/dc.23880
142. Sengupta A, Ramaniharan AK, Gupta RK, Agarwal S, Singh A. Glioma grading using a machine-learning framework based on optimized features obtained from T(1) perfusion MRI and volumes of tumor components. J Magn Reson Imaging (2019) 50(4):1295–306. doi: 10.1002/jmri.26704
143. Sengupta D, Ali SN, Bhattacharya A, Mustafi J, Mukhopadhyay A, Sengupta K. A deep hybrid learning pipeline for accurate diagnosis of ovarian cancer based on nuclear morphology. PloS One (2022) 17(1):e0261181. doi: 10.1371/journal.pone.0261181
144. Sepandi M, Taghdir M, Rezaianzadeh A, Rahimikazerooni S. Assessing breast cancer risk with an artificial neural network. Asian Pac J Cancer Prev (2018) 19(4):1017–9. doi: 10.22034/APJCP.2018.19.4.1017
145. Shaban M, Khurram SA, Fraz MM, Alsubaie N, Masood I, Mushtaq S, et al. A novel digital score for abundance of tumour infiltrating lymphocytes predicts disease free survival in oral squamous cell carcinoma. Sci Rep (2019) 9(1):13341. doi: 10.1038/s41598-019-49710-z
146. Shaikh TA, Ali R, Beg MMS. Transfer learning privileged information fuels CAD diagnosis of breast cancer. Mach Vision Appl (2020) 31(1–2): 1–23. doi: 10.1007/s00138-020-01058-5
147. Sharafeldeen A, Elsharkawy M, Khaled R, Shaffie A, Khalifa F, Soliman A, et al. Texture and shape analysis of diffusion-weighted imaging for thyroid nodules classification using machine learning. Med Phys (2022) 49(2):988–99. doi: 10.1002/mp.15399
148. Shayesteh S, Nazari M, Salahshour A, Sandoughdaran S, Hajianfar G, Khateri M, et al. Treatment response prediction using MRI-based pre-, post-, and delta-radiomic features and machine learning algorithms in colorectal cancer. Med Phys (2021) 48(7):3691–701. doi: 10.1002/mp.14896
149. Shayesteh SP, Alikhassi A, Fard Esfahani A, Miraie M, Geramifar P, Bitarafan-Rajabi A, et al. Neo-adjuvant chemoradiotherapy response prediction using MRI based ensemble learning method in rectal cancer patients. Phys Med (2019) 62:111–9. doi: 10.1016/j.ejmp.2019.03.013
150. Shehata M, Alksas A, Abouelkheir RT, Elmahdy A, Shaffie A, Soliman A, et al. A comprehensive computer-assisted diagnosis system for early assessment of renal cancer tumors. Sensors (Basel) (2021) 21(14): 4928. doi: 10.3390/s21144928
151. Shukla RS, Aggarwal Y. Nonlinear heart rate variability based artificial intelligence in lung cancer prediction. J Appl Biomed (2018) 16(2):145–55. doi: 10.1016/j.jab.2017.12.002
152. Singh A, Bhat V, Sudhakar S, Namachivayam A, Gangadharan C, Pulchan C, et al. Multicentric study to evaluate the effectiveness of thermalytix as compared with standard screening modalities in subjects who show possible symptoms of suspected breast cancer. BMJ Open (2021) 11(10):e052098. doi: 10.1136/bmjopen-2021-052098
153. Singh BK, Verma K, Panigrahi L, Thoke AS. Integrating radiologist feedback with computer aided diagnostic systems for breast cancer risk prediction in ultrasonic images: An experimental investigation in machine learning paradigm. Expert Syst Appl (2017) 90:209–23. doi: 10.1016/j.eswa.2017.08.020
154. Singh D, Kumar V, Das CJ, Singh A, Mehndiratta A. Characterisation of prostate cancer using texture analysis for diagnostic and prognostic monitoring. NMR Biomed (2021) 34(6):e4495. doi: 10.1002/nbm.4495
155. Subbaiah RM, Dey P, Nijhawan R. Artificial neural network in breast lesions from fine-needle aspiration cytology smear. Diagn Cytopathol (2014) 42(3):218–24. doi: 10.1002/dc.23026
156. Tapak L, Shirmohammadi-Khorram N, Amini P, Alafchi B, Hamidi O, Poorolajal J. Prediction of survival and metastasis in breast cancer patients using machine learning classifiers. Clin Epidemiol Global Health (2019) 7(3):293–9. doi: 10.1016/j.cegh.2018.10.003
157. Tekchandani H, Verma S, Londhe ND, Jain RR, Tiwari A. Computer aided diagnosis system for cervical lymph nodes in CT images using deep learning. Biomed Signal Process Control (2022) 71: 103158. doi: 10.1016/j.bspc.2021.103158
158. Tewary S, Arun I, Ahmed R, Chatterjee S, Chakraborty C. AutoIHC-scoring: a machine learning framework for automated allred scoring of molecular expression in ER- and PR-stained breast cancer tissue. J Microsc (2017) 268(2):172–85. doi: 10.1111/jmi.12596
159. Tewary S, Arun I, Ahmed R, Chatterjee S, Mukhopadhyay S. AutoIHC-analyzer: computer-assisted microscopy for automated membrane extraction/scoring in HER2 molecular markers. J Microsc (2021) 281(1):87–96. doi: 10.1111/jmi.12955
160. Thakran S, Gupta RK, Singh A. Characterization of breast tumors using machine learning based upon multiparametric magnetic resonance imaging features. Nmr Biomed (2022) 35(5):e4665. doi: 10.1002/nbm.4665
161. Tomas RC, Sayat AJ, Atienza AN, Danganan JL, Ramos MR, Fellizar A, et al. Detection of breast cancer by ATR-FTIR spectroscopy using artificial neural networks. PloS One (2022) 17(1):e0262489. doi: 10.1371/journal.pone.0262489
162. Torkaman A, Charkari NM, Aghaeipour M. An approach for leukemia classification based on cooperative game theory. Anal Cell Pathol (Amst) (2011) 34(5):235–46. doi: 10.1155/2011/212174
163. Vaka AR, Soni B, Reddy KS. Breast cancer detection by leveraging machine learning. Ict Express (2020) 6(4):320–4. doi: 10.1016/j.icte.2020.04.009
164. Vazifehdan M, Moattar MH, Jalali M. A hybrid Bayesian network and tensor factorization approach for missing value imputation to improve breast cancer recurrence prediction. J King Saud University-Computer Inf Sci (2019) 31(2):175–84. doi: 10.1016/j.jksuci.2018.01.002
165. Zadeh HG, Pakdelazar O, Haddadnia J, Rezai-Rad G, Mohammad-Zadeh M. Diagnosing breast cancer with the aid of fuzzy logic based on data mining of a genetic algorithm in infrared images. Middle East J Cancer (2012) 3(4):119–29.
166. Kourou K, Exarchos TP, Exarchos KP, Karamouzis MV, Fotiadis DI. Machine learning applications in cancer prognosis and prediction. Comput Struct Biotechnol J (2015) 13:8–17. doi: 10.1016/j.csbj.2014.11.005
167. Levine AB, Schlosser C, Grewal J, Coope R, Jones SJM, Yip S. Rise of the machines: Advances in deep learning for cancer diagnosis. Trends Cancer (2019) 5(3):157–69. doi: 10.1016/j.trecan.2019.02.002
168. Tran KA, Kondrashova O, Bradley A, Williams ED, Pearson JV, Waddell N. Deep learning in cancer diagnosis, prognosis and treatment selection. Genome Med (2021) 13(1):152. doi: 10.1186/s13073-021-00968-x
169. Zayyad MA, Toycan M. Factors affecting sustainable adoption of e-health technology in developing countries: an exploratory survey of Nigerian hospitals from the perspective of healthcare professionals. PeerJ (2018) 6:e4436. doi: 10.7717/peerj.4436
170. Andersen L. Artificial intelligence in international development: Avoiding ethical pitfalls. J Public Int Affairs (2019).
171. De-Arteaga M, Herlands W, Neill DB, Dubrawski A. Machine learning for the developing world. ACM Trans Manage Inf Syst (TMIS) (2018) 9(2):1–14. doi: 10.1145/3210548
172. Guo J, Li B. The application of medical artificial intelligence technology in rural areas of developing countries. Health equity (2018) 2(1):174–81. doi: 10.1089/heq.2018.0037
173. Cabitza F, Campagner A. The need to separate the wheat from the chaff in medical informatics: Introducing a comprehensive checklist for the (self)-assessment of medical AI studies. Int J Med Inf (2021) 153:104510. doi: 10.1016/j.ijmedinf.2021.104510
174. Collins GS, Reitsma JB, Altman DG, Moons KG. Transparent reporting of a multivariable prediction model for individual prognosis or diagnosis (TRIPOD): the TRIPOD statement. Br J Cancer (2015) 112(2):251–9. doi: 10.1038/bjc.2014.639
175. Riley RD, Collins GS, Ensor J, Archer L, Booth S, Mozumder SI, et al. Minimum sample size calculations for external validation of a clinical prediction model with a time-to-event outcome. Stat Med (2022) 41(7):1280–95. doi: 10.1002/sim.9275
176. Riley RD, Debray TPA, Collins GS, Archer L, Ensor J, van Smeden M, et al. Minimum sample size for external validation of a clinical prediction model with a binary outcome. Stat Med (2021) 40(19):4230–51. doi: 10.1002/sim.9025
177. Archer L, Snell KIE, Ensor J, Hudda MT, Collins GS, Riley RD. Minimum sample size for external validation of a clinical prediction model with a continuous outcome. Stat Med (2021) 40(1):133–46. doi: 10.1002/sim.8766
178. Collins GS, Dhiman P, Andaur Navarro CL, Ma J, Hooft L, Reitsma JB, et al. Protocol for development of a reporting guideline (TRIPOD-AI) and risk of bias tool (PROBAST-AI) for diagnostic and prognostic prediction model studies based on artificial intelligence. BMJ Open (2021) 11(7):e048008. doi: 10.1136/bmjopen-2020-048008
179. Bossuyt PM, Reitsma JB, Bruns DE, Gatsonis CA, Glasziou PP, Irwig L, et al. STARD 2015: an updated list of essential items for reporting diagnostic accuracy studies. Bmj (2015) 351:h5527. doi: 10.1136/bmj.h5527
180. Cruz Rivera S, Liu X, Chan A-W, Denniston AK, Calvert MJ, Ashrafian H, et al. Guidelines for clinical trial protocols for interventions involving artificial intelligence: the SPIRIT-AI extension. Lancet Digit Health (2020) 2(10):e549–e60. doi: 10.1136/bmj.m3210
181. Liu X, Cruz Rivera S, Moher D, Calvert MJ, Denniston AK, Chan A-W, et al. Reporting guidelines for clinical trial reports for interventions involving artificial intelligence: the CONSORT-AI extension. Nat Med (2020) 26(9):1364–74. doi: 10.1038/s41591-020-1034-x
182. Gupta N, Patel H, Afzal S, Panwar N, Mittal RS, Guttula S, et al. Data quality toolkit: Automatic assessment of data quality and remediation for machine learning datasets. arXiv: USA (Cornell Tech) (2021).
183. Motlhale M, Sitas F, Bradshaw D, Chen WC, Singini MG, de Villiers CB, et al. Epidemiology of kaposi’s sarcoma in sub-Saharan Africa. Cancer Epidemiol (2022) 78:102167. doi: 10.1016/j.canep.2022.102167
184. Chalya PL, Mbunda F, Rambau PF, Jaka H, Masalu N, Mirambo M, et al. Kaposi’s sarcoma: a 10-year experience with 248 patients at a single tertiary care hospital in Tanzania. BMC Res Notes (2015) 8(1):440. doi: 10.1186/s13104-015-1348-9
185. Magrath I. Epidemiology: clues to the pathogenesis of burkitt lymphoma. Br J Haematol (2012) 156(6):744–56. doi: 10.1111/j.1365-2141.2011.09013.x
Keywords: artificial intelligence, cancer, machine learning, low-income countries, lower-middle-income countries
Citation: Adeoye J, Akinshipo A, Koohi-Moghadam M, Thomson P and Su Y-X (2022) Construction of machine learning-based models for cancer outcomes in low and lower-middle income countries: A scoping review. Front. Oncol. 12:976168. doi: 10.3389/fonc.2022.976168
Received: 23 June 2022; Accepted: 14 November 2022;
Published: 01 December 2022.
Edited by:
Dana Kristjansson, Norwegian Institute of Public Health (NIPH), NorwayReviewed by:
Hao Zhang, Chongqing Medical University, ChinaRasheed Alabi, University of Helsinki, Finland
Copyright © 2022 Adeoye, Akinshipo, Koohi-Moghadam, Thomson and Su. This is an open-access article distributed under the terms of the Creative Commons Attribution License (CC BY). The use, distribution or reproduction in other forums is permitted, provided the original author(s) and the copyright owner(s) are credited and that the original publication in this journal is cited, in accordance with accepted academic practice. No use, distribution or reproduction is permitted which does not comply with these terms.
*Correspondence: Yu-Xiong Su, richsu@hku.hk