- 1The Fourth Department of Medical Oncology, Harbin Medical University Cancer Hospital, Harbin, China
- 2Department of Thoracic Surgery, Harbin Medical University Cancer Hospital, Harbin, China
Lung adenocarcinoma (LUAD) is a remarkably heterogeneous and aggressive disease with dismal prognosis of patients. The identification of promising prognostic biomarkers might enable effective diagnosis and treatment of LUAD. Aberrant activation of epithelial-mesenchymal transition (EMT) is required for LUAD initiation, progression and metastasis. With the purpose of identifying a robust EMT-related gene signature (E-signature) to monitor the survival outcomes of LUAD patients. In The Cancer Genome Atlas (TCGA) database, least absolute shrinkage and selection operator (LASSO) analysis and cox regression analysis were conducted to acquire prognostic and EMT-related genes. A 4 EMT-related and prognostic gene signature, comprising dickkopf-like protein 1 (DKK1), lysyl oxidase-like 2 (LOXL2), matrix Gla protein (MGP) and slit guidance ligand 3 (SLIT3), was identified. By the usage of datum derived from TCGA database and Western blotting analysis, compared with adjacent tissue samples, DKK1 and LOXL2 protein expression in LUAD tissue samples were significantly higher, whereas the trend of MGP and SLIT3 expression were opposite. Concurrent with upregulation of epithelial markers and downregulation of mesenchymal markers, knockdown of DKK1 and LOXL2 impeded the migration and invasion of LUAD cells. Simultaneously, MGP and SLIT3 silencing promoted metastasis and induce EMT of LUAD cells. In the TCGA-LUAD set, receiver operating characteristic (ROC) analysis indicated that our risk model based on the identified E-signature was superior to those reported in literatures. Additionally, the E-signature carried robust prognostic significance. The validity of prediction in the E-signature was validated by the three independent datasets obtained from Gene Expression Omnibus (GEO) database. The probabilistic nomogram including the E-signature, pathological T stage and N stage was constructed and the nomogram demonstrated satisfactory discrimination and calibration. In LUAD patients, the E-signature risk score was associated with T stage, N stage, M stage and TNM stage. GSEA (gene set enrichment analysis) analysis indicated that the E-signature might be linked to the pathways including GLYCOLYSIS, MYC TARGETS, DNA REPAIR and so on. In conclusion, our study explored an innovative EMT based prognostic signature that might serve as a potential target for personalized and precision medicine.
Introduction
Lung adenocarcinoma (LUAD), as the predominant histological type of lung cancer, has biological characteristics of strong aggressiveness and heterogeneity (1–3). In spite of optimized treatment methods including surgery, chemotherapy, radiotherapy, immunotherapy and targeted therapy, prognosis of LUAD patients remains dismal, because of cancer progression (4, 5). Collectively, it is imperative to distinguish populations at a high-risk of LUAD for early intervention and improving clinical outcome. At present, the combination of clinicopathological features and TNM staging system is the consensus criterion for determining treatment options and predicting relapse of LUAD, however this criterion restrains the provision of optimal clinical care to patients (6). Hence, identifying reliable biomarkers for optimizing the prognosis of LUAD is urgently needed.
Metastases are the primary cause of LUAD-associated mortality (7, 8). Migratory tumor cells escape from the primary site, remodel the basement membrane to engage with peritumoural stroma, undergo intravasation, endure shear stress in circulation and adapt to the tumor microenvironment of distant metastasis (9, 10). In total, the metastatic process generally involves three distinct phases: dissemination, dormancy and colonization (11). Epithelial mesenchymal transition (EMT) of cancer cells is a fundamental event during the multistep process participated in cancer metastasis (12, 13). Moreover, EMT activation confers on tumor cells to acquire plasticity with more aggressive phenotype, which exerts a decisive function on the malignant cancer progression (14, 15). Accumulating evidence has revealed that EMT process was closely implicated in tumorigenicity, angiogenesis and drug resistance (16–18). EMT results in a series of changes during epithelial tumor cells transforming into mesenchymal cells, including loss of tight junctions, cell polarity, cytoskeletal reorganization, and increase of cell viability (19). EMT is controlled or induced by a number of factors such as exosomal circRNAs, varied transcripts (Twist, Snail, ZEB1, et al.), microRNAs (miR212, mir200 family, et al.) and cellular oncogenic pathways (EGFR signaling pathway, et al.) (20–22). There is a significant link between the aberrant expression of genes related with EMT and poor clinical outcomes in LUAD patients (13, 23).
Since the advent of next generation sequencing, research on bioinformatic analysis has flourished (24; 25). For instance, The Cancer Genome Atlas (TCGA) database and Gene Expression Omnibus (GEO) database, such public databases are desirable to access transcriptomic information, that advances the efficient methods to select gene signatures (26–28). Numerous studies have attempted to construct the risk model to get biological characteristics or prognostic appraisal in malignant tumors, which had potential clinical impact (29–31). Interestingly, EMT-related gene signature (E-signature) could reveal the prognostic consequences in various types of cancer (32–34). Nevertheless, the existence of heterogeneity in samples among diversified studies on various tumours resulting in different risk models (35). Recently, some researchers reported the prognostic significance of E-signature, however they did not validate the biological functions of EMT-related genes with in vitro experiments in LUAD and their studies remains rudimentary to some extent (36–38).
In the current study, candidate genes involved in EMT process were identified based on TCGA-LUAD training dataset and validated using cell-based assays in vitro and clinical tissue samples. Then, the E-signature which can accurately predict the prognosis of LUAD patients was developed by us. Meanwhile, the nomogram affirmed the feasibility in clinical application of the E-signature. Subgroup analysis suggested that E-Signature could be conducive to identify patients with adverse events at high risk. The E-signature is closely related to multiple pathways associated with cancer progression, as well. In conclusion, the E-signature could be used as an inspiring molecular indicator for evaluation of clinical prognosis in LUAD patients.
Materials and methods
Data capturing and processing
Entire design attached to our research was presented in Figure 1. TCGA website (http://portal.gdc.cancer.gov) were used to obtain raw microarray data and matched clinical of LUAD patients. Expression profiles were processed with robust multiarray average (RMA) algorithm (29). Differential expression analysis was performed by “limma” package. GEO database(https://www.ncbi.nlm.nih.gov/geo/) (Table 1) were used as independent external verification sets, including GSE30219, GSE37745, GSE50081 and GSE8894 (https://www.ncbi.nlm.nih.gov/geo/) (Table 1). Besides, we retrieved “HALLMARK EPITHELIAL MESENCHYMAL TRANSITION” gene list encompassing 200 genes in the MsigDB (https://www.gsea-msigdb.org/gsea/msigdb/index.jsp).
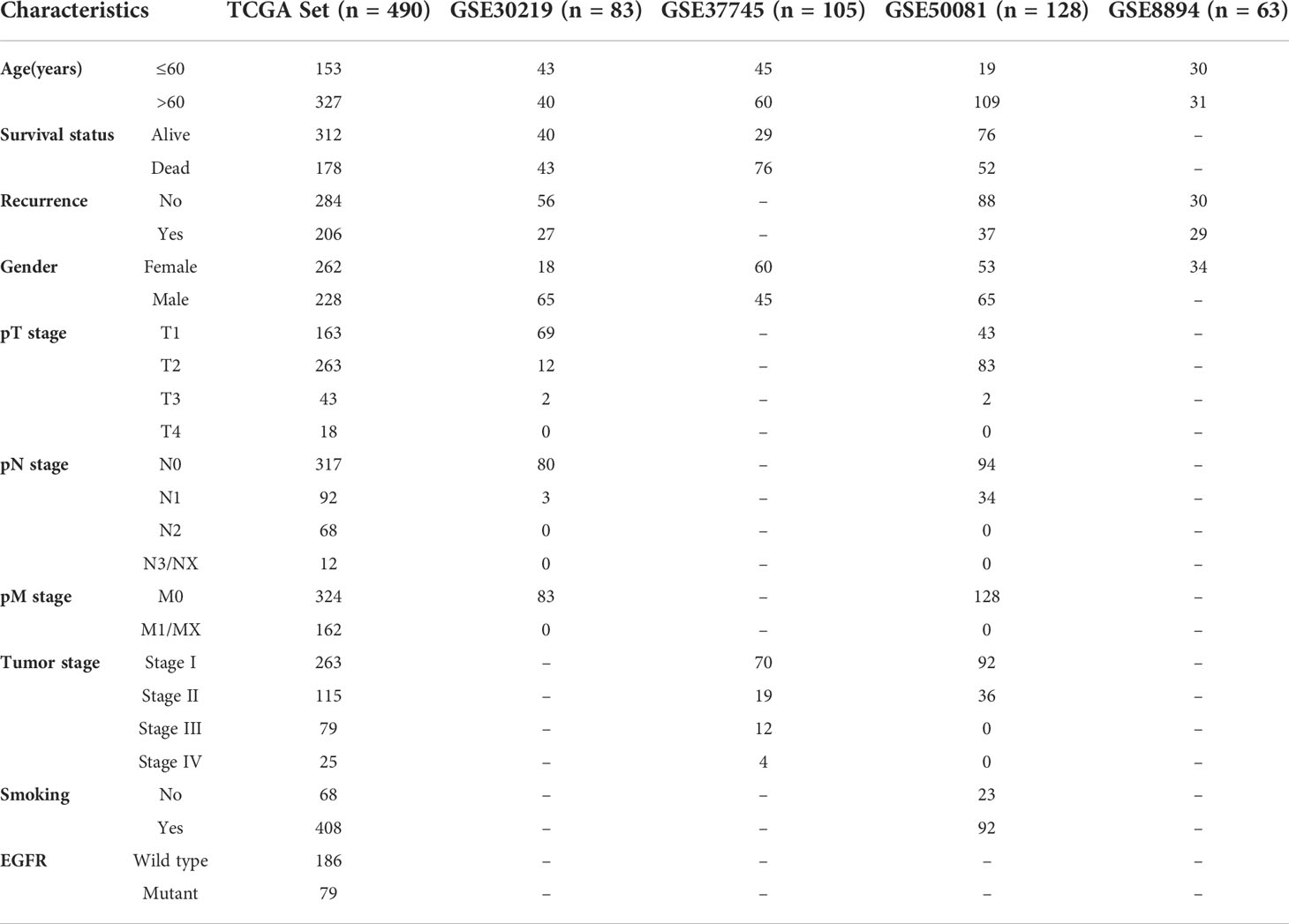
Table 1 Various clinicopathological characteristics of patients with LUAD in TCGA training cohort and GEO datasets.
LASSO Cox regression analysis
To yield the independent prognostic EMT factor, univariate analysis and multivariate analysis were showed with P< 0.05 as a threshold. least absolute shrinkage and selection operator (LASSO) analysis was capable for reducing the dimension (39). 80% TCGA samples after preprocessing were randomized to the training dataset. our prognostic model preserved the advantage of subset shrinkage and maintained a high accuracy rate based on the penalty parameter λ (40). Risk score was calculated from expression of related gene and associated coefficient. Analytical formula for risk score assessment was derived on the basis of the EMT related gene signature = , in this formula, Expri represents gene expression of patient, and coefi represents the multivariate regression coefficient.
Survival analysis
According to median risk scores, all LUAD samples were divided into two groups, involving in high-risk and low-risk groups in different cohorts. Kaplan Meier (KM) curves were plotted to compare the prognostic difference (41). The area under the curve (AUC) which performed from receiver operating characteristic (ROC) curve analysis for overall survival (OS) was employed to designate the predictive efficiency (42).
Establishment and validation of the nomogram
The significant variables from the multivariate models were introduced to draw the graphical nomogram by utilizing “rms” and “nomogramEx” packages (43). The calibration curves for probability of OS showed that match condition between prediction by nomogram and actual observation (44). Decision curve analysis (DCA) was utilized to evaluate the ability of the predictive model in view of clinical applicability (44).
Gene set variation analysis (GSVA)
Single-sample gene set enrichment analysis (ssGSEA) scores were gained by “ClusterProfiler” and “GSVA” R package to recognize the correlation between risk scores and enriched biological processes (45). The results with a cut-off criterion of P value< 0.05 were statistical significance.
Collection of LUAD tissues and cell culture
Under the premise that ethical clearance and approval have been obtained, 5 pairs of fresh tumor samples and matched normal tumor-adjacent samples were dissected from 5 LUAD patients who underwent lobectomy but did not receive radiotherapy and chemotherapy at Harbin Medical University Cancer Hospital between May 2021 and June 2021.
Human LUAD cell lines (A549 and NCI-H1299) were cultured with RPMI-1640 including 10% fetal bovine serum (FBS) and 1% penicillin/streptomycin, and the culture environment needed to be maintained at 37°C and containing 5% carbon dioxide.
Small interfering RNA transfection
SiRNAs of dickkopf-like protein 1 (DKK1), lysyl oxidase-like 2 (LOXL2), matrix Gla protein (MGP) and slit guidance ligand 3 (SLIT3) were made by Hanyinbt (Shanghai, China), in addition, these target sequences were presented as: DKK1#1, 5’-GCUUCACACUUGUCAGAGAtt-3’; DKK1#2, 5’-GGCUCUCAUGGACUAGAAAtt-3’; LOXL2#1, 5’-CAUACAAUACCAAAGUGUAtt-3’; LOXL2#2, 5’-GGGUGGAGGUGUACUAUGAtt-3’; MGP#1, 5’-CCCUACUGCUGCUACACAATT-3’; MGP#2, 5’-GAUAAGUAAUGAAAGUGCATT-3’; SLIT3#1, 5’-CGCGAUUUGGAGAUCCUUAtt-3’; SLIT3#2, 5’-GUACAAAGAGCCAGGAAUATT-3’. All corresponding negative control siRNA sequences were completed by Hanyinbt Company.
When the density of A549 and H1299 cells reached 60-70% in 6-well dishes, the transfection mixture was prepared by fully mixing 120μL riboFECT™CP Buffer, 12μL riboFECT™CP Reagent and 5μL siRNA, and incubated at room temperature for 15min. Finally, 3mL RPMI-1640 containing 10% FBS was added into the 6-well dishes. Follow-up experiments were carried out after ensuring the efficiency of knockout.
Western blotting
Lysate of tissues or cells was centrifuged for 15 min (4°C, 14000rpm) and we measured protein concentrations using the BCA protein analysis kit. Electrophoretic separation and electro-transfer of protein samples with the comparable quality, membrane blocking and incubate overnight (primary antibody, 4°C). PVDF membranes containing proteins are incubated with secondary antibodies for 1 hour at room temperature. The bands on membrane were exposed by CL Xposure film (Thermo Fisher Scientific). Specific antibodies included: DKK1 (21112-1-AP, Proteintech Group Inc., Wuhan, China), LOXL2 (AB179810, Abcam), MGP (10734-1-AP, Proteintech Group Inc., Wuhan, China), SLIT3 (DF9909, Affinity Biosciences, Jiangsu, China), E-Cadherin (20874-1-AP, Proteintech Group Inc., Wuhan, China), N-Cadherin (22018-1-AP, Proteintech Group Inc., Wuhan, China), Vimentin (10366-1-AP, Proteintech Group Inc., Wuhan, China), β-actin (AF7018, Affinity Biosciences, Jiangsu, China).
Detection of cancer cell migration and invasion
Use the tip of a 10 μL pipette to form a scratch on a six-well plate covered with LUAD cells. By comparing the rate of wound healing and taking pictures under the microscope, the cell migration rate was finally counted. Transwell assay was conducted with the Corning Inc. transwell chamber. The invasion experiment required the participation of the matrigel matrix involved. Cell suspension arranged in the upper chamber contained 2x104 cells, besides, ingredient of the lower chamber was 600μL RPMI-1640 complemented with 10% FBS. The fixation of methanol and staining of 0.1% crystal violet for observing the migration and invasion efficiency after 24h or 48h, respectively. Fields were randomly selected in each membrane for capturing.
Statistical methods
Data processing was focused on GraphPad Prism 8.0.2 software. Typicality of data in the study could be reflected with at least three independent experiments completed. Continuous data conforming to a normal distribution were analyzed by Student’s t-test, and subgroup differences were counted using the χ2 test. All results are expressed as mean ± SEM (* P< 0.05, ** P< 0.01, *** P< 0.001).
Results
The identification and validation with in vitro experiments of 4 prognostic EMT-related genes comprising the E-signature
The 200 genes linked to EMT were acquired from the “HALLMARK_EPITHELIAL_MESENCHYMAL_TRANSITION” gene list in the MsigDB. Univariate Cox analyses systematically deduced 68 genes significantly relevant to prognosis based on TCGA-LUAD training cohort. To exploit an optimal model for testing risk, the LASSO analysis was used in its broadest sense to summary the results of each dimensionality reduction and count the occurrence frequency and standard deviation distribution of each probe. Standard deviation (SD) calculations may comprehensively describe the distribution characteristics of data, which is generally understood to measure the deviation degree (46). Four EMT-related genes, including dickkopf-like protein 1 (DKK1), lysyl oxidase-like 2 (LOXL2), matrix Gla protein (MGP) and slit guidance ligand 3 (SLIT3), were comprehensively screened depending on the following criteria (Figure 2A). SD of candidate mRNAs was greater than the median and frequency was well over 500 (Figure 2A). Patients with high DKK1 and LOXL2 expression had the shorter overall survival (OS) according to a Kaplan-Meier analysis, whereas ones with high expression of MGP or SLIT3 had longer OS (Figure 2B). Based on TCGA-LUAD transcriptome data, differential expression analysis showed that with the comparison of normal samples, DKK1 and LOXL2 expression were significantly increased in tumor tissues, whereas MGP and SLIT3 were markedly overexpressed in non-tumoral tissues (Figure 2C). The same results were achieved from the collected clinical tissue samples by western blotting (Figure 2D).
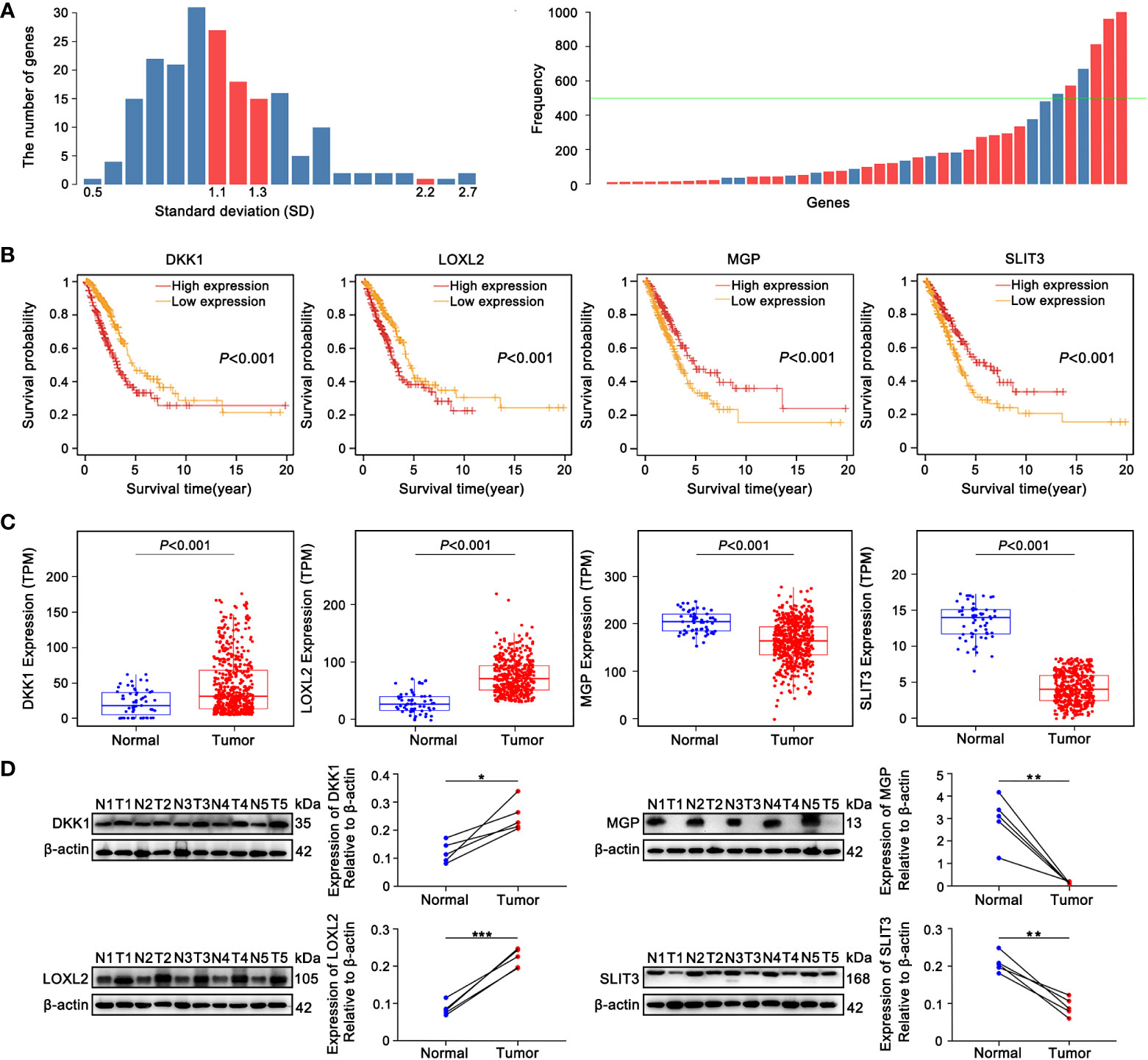
Figure 2 The identification 4 prognostic EMT-related genes comprising the E-signature. (A) The standard deviation distribution of all mRNAs. In the left panel, location corresponding to the red columns were standard deviation (SD) of genes with frequency greater than 500, where SD was used as the abscissa and the vertical axis represented the number of genes. The right panel showed that the gene frequency distribution chart obtained by LASSO analysis. The green baseline meant that frequency was equal to 500, the part exceeded the green line was genes with frequency greater than 500. (B) KM curves showed the overall survival (OS) of patients grouped according to expression patterns of 4 prognostic EMT-related genes comprising the E-signature in TCGA-LUAD datasets. (C) Differential expression analysis of DKK1, LOXL2, MGP and SLIT3 originated from TCGA-LUAD dataset. (D) The expression of 4 genes in fresh LUAD tumor samples (T) and adjacent normal-frozen tissues (N) detected by Western blot (*P< 0.05, **P< 0.01, ***P< 0.001).
The functions and roles of these four genes in LUAD metastasis and EMT remain to be clarified (47–50). We used cell-based assays in vitro to examine the effect of these four genes on EMT and metastasis, respectively. In A549 and H1299 cells, Gene-specific siRNAs were used with three independent siRNAs for knockdown of each gene. Successful knockdown of these four genes was validated by Western blotting in A549 (Figure 3A) and NCI-H1299 cell lines (Figure S1A). Moreover, as illustrated in Figure 3A and Figure S1A, knockdown of DKK1 or si-LOXL2 enhanced the expression of E-cadherin and inhibited the mesenchymal markers’ expression, including N-cadherin and Vimentin. MGP or SLIT3 knockdown led to downregulation of epithelium-derived markers and upregulation of mesenchymal markers (Figure 3A; Figure S1A). Furthermore, wound healing and Transwell assays revealed silencing of DKK1 and LOXL2 suppressed the ability of migration and invasion in LUAD cells, but silencing of MGP and SLIT3 had the opposite effect (Figures 3B, C; Figures S1B, C). Our experimental data indicated that the four EMT-related genes could regulate metastasis and EMT program.
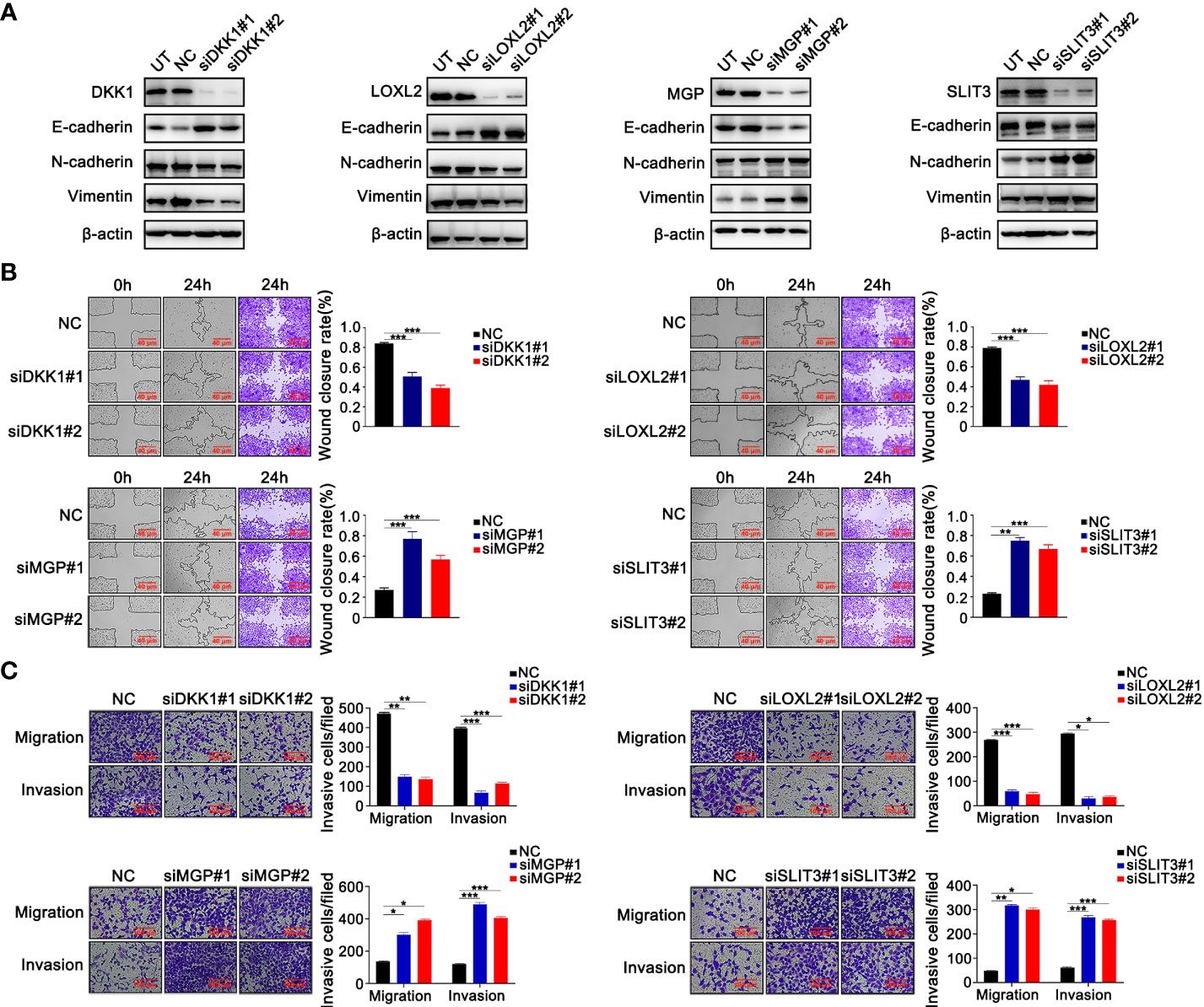
Figure 3 The validation experiments of 4 prognostic EMT-related genes comprising the E-signature in vitro. (A) Western blotting confirmed that silencing 4 genes respectively caused alterations of EMT‐related protein expression in H1299 cells. (B) The effect of silencing four genes on H1299 cell migration confirmed by wound-healing assays. (C) Transferability and invasiveness of H1299 cells were evaluated. *P < 0.05; **P < 0.01; ***P < 0.001. Data wereobtained from three independent experiments.
Construction of the prognostic E-signature in LUAD
Based on multivariate Cox regression analysis, the independent prognostic signature still composed of four EMT genes was generated. Scoring formula as follows: Risk Score = 0.308×expDKK1+0.299×expLOXL2-0.084×expMGP-0.165×expSLIT3 (Figure 4A). The statistical correlation between risk model and 4 genes expression was assessed by the Pearson correlation metric (Figure 4B). Risk score was converted into Z-score, taking 0 served as the optimal boundary value to divide the samples into two groups, in which z score of the high-risk subgroup was greater than 0, and the rest belonged to the low-risk subgroup. The permutation of risk scores, survival status and four genes expression levels were displayed (Figure 4C). These results demonstrated that the risk E-signature was a deleterious indicator of prognosis.
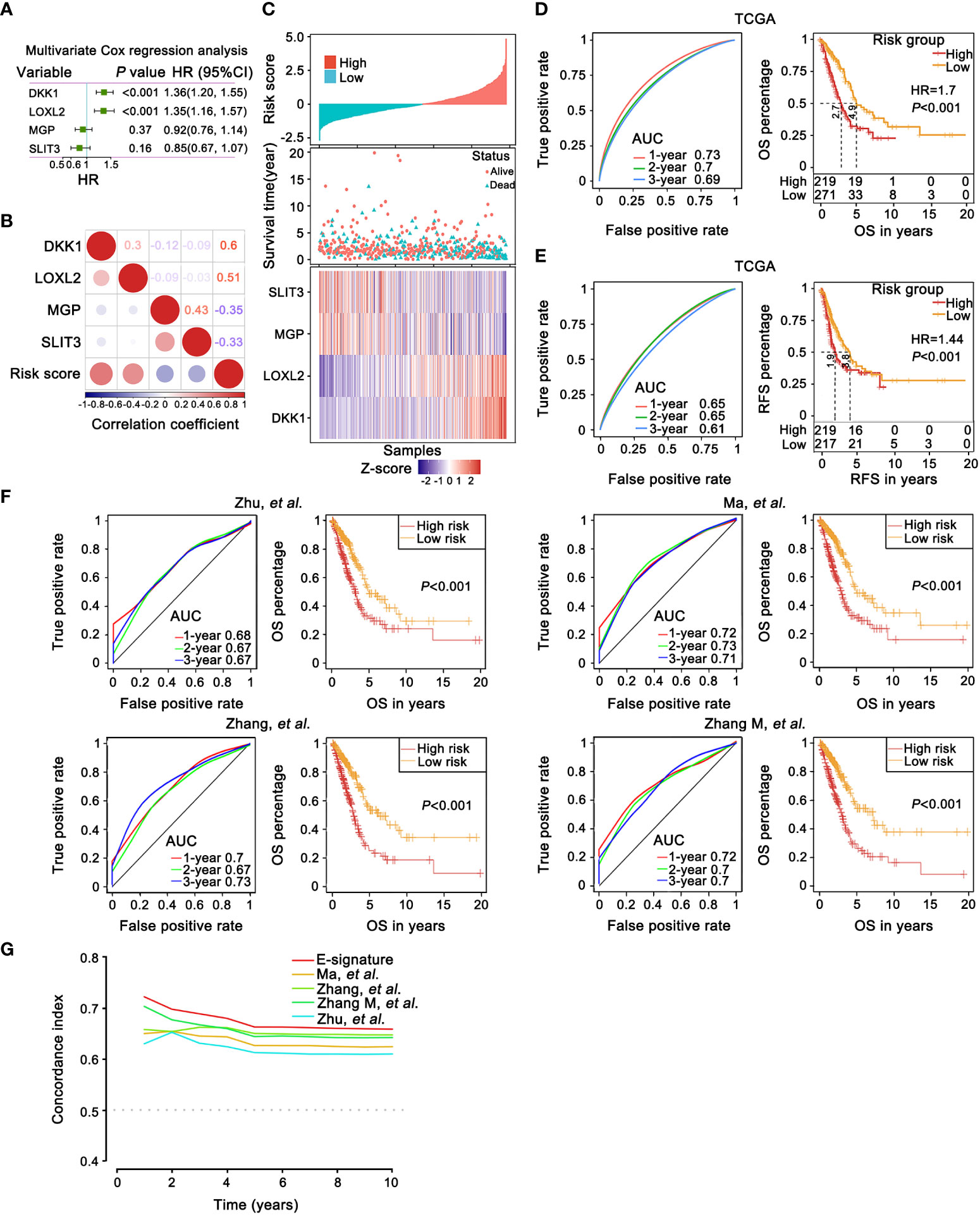
Figure 4 The prognostic robustness and clinical usefulness of the E-signature in the internal training set. (A) Evaluating the impact of 4 EMT-related genes on OS by means of forest plot. (B) Relatedness plot reported Pearson correlation values of each comparison. The bar color indicated Pearson corr. Values below the map calculated between risk score and genes in the matrix. (C) The risk score, survival time and status of each sample in TCGA-LUAD cohorts. The heatmap listed expression status of each gene involved in the signature. (D, E) The time-dependent receiver operating characteristic (ROC) curves for the 1-, 2- and 3-year OS (D) and relapse free survival (RFS) prediction (E) by the E-signature. Significant survival difference between high- and low-threshold group. (F) A comparison with previously reported E-signature models by KM survival analysis and ROC curve. (G) The E-signature had the highest concordance index (C-index) as opposed to other reported models, which proved that it could accurately predict prognosis.
Furthermore, based on TCGA training dataset, the prognostic accuracy of E-signature was appraised by time-dependent ROC analysis, the AUC was 0.73 (1-year), 0.7 (2-year) and 0.69 (3-year), respectively (Figure 4D). Referring to the training set, in comparison with the high-risk group, the low-risk group revealed a significantly longer OS (Figure 4D). The ROC and KM curve also revealed that our model exhibited good sensitivity and specificity in predicting TCGA-LUAD recurrence free survival (RFS, Figure 4E). Compared with recently published lung cancer prognostic models in the literatures (Figure 4F) including Zhu (51), Ma (52), Zhang (53) and Zhang M (54), our E-signature had better specificity by ROC curve analysis especially at 1-year (Figure 4F). Similarly, the E-signature predicted OS better than either the known signatures alone, with a better calibration and classification accuracy (Figure 4G).
External validation of the prognostic E-signature
For further emphasizing the robustness of the constructed signature, GSE30219, GSE37745 and GSE50081 external validation sets were used for ROC analysis and KM analysis. In line with the training set, E-signature had strong predictive accuracy (GSE30219 [1-year: 0.74; 2-year: 0.69; 3-year: 0.73]; GSE37745 [1-year: 0.65; 2-year: 0.66; 3-year: 0.6]; GSE50081 [1-year: 0.72; 2-year: 0.68; 3-year: 0.68]) and patients in the group with high-risk showed a predominant association with frustrating OS (Figure S2A). Next, we opt GSE30219, GSE50081 and GSE8894 datasets and executed the identical methods to validate the prognostic potential of E-signature in RFS. Likewise, the E-signature maintained ideal sensitivity and specificity as a prognostic indicator (GSE30219 [1-year: 0.87; 2-year: 0.76; 3-year: 0.80]; GSE50081 [1-year: 0.67; 2-year: 0.68; 3-year: 0.68]; GSE8894 [1-year: 0.71; 2-year: 0.68; 3-year: 0.68]) and high-risk patients had significantly worse RFS relative to the group at low risk (Figure S2B).
Establishment of a nomogram based on the E-signature
clinicopathological characteristics, such as T stage, N stage, M stage, TNM stage, age, gender, and smoking history, and risk score were incorporated as covariates into univariate and multivariate Cox regression analyses. Risk score, T stage and N stage were demonstrated the independent prognostic factors by forest plot for OS (Figures 5A).
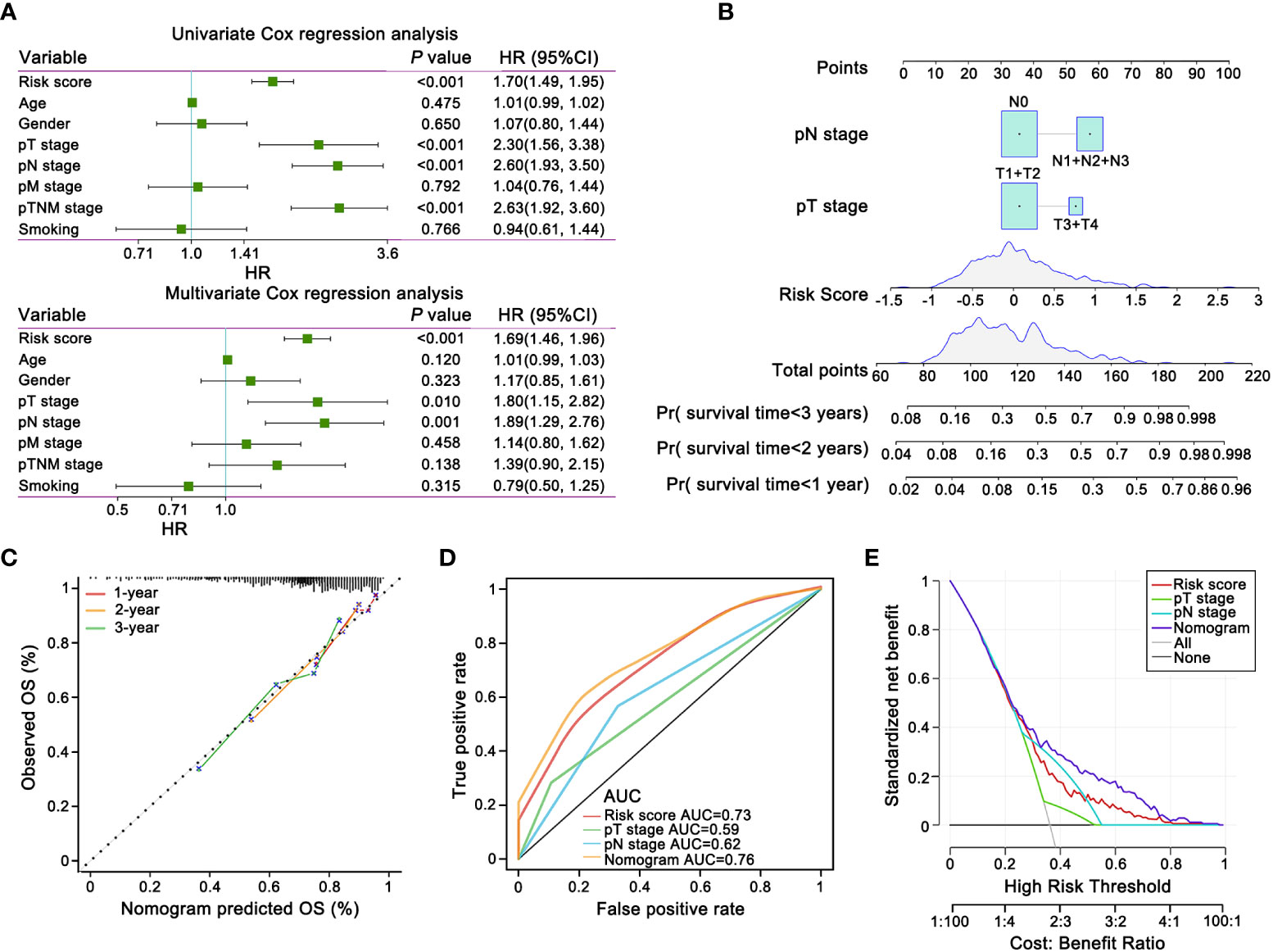
Figure 5 The prognostic nomogram and decision curve analysis (DCA) of the risk score based on E-signature. (A) The forest plot showed that the signature was independent from other risk factors for prognostic prediction. (B) Nomogram predicted risk of secondary progression. Each variable axis, containing T stage, N stage and risk score, corresponded to the characteristic attribute score of single sample. The final score was summarized on the total score axis and the likelihood of 1-, 2-, and 3 years OS is determined on the survival axis. (C) The nomogram yielded an accurate predictive capability that was extremely close to actual survival was presented by the calibration plots. X-axis represented the predicted value of survival probability and y-axis represented actual survival possibility. (D) ROC curves represented that the E-signature ranked first among all the parameters. (E) DCA curves graphically verified the E-signature brought more net benefit of survival than other clinical indexes. Solid lines indicated net benefit of the predictive model within the threshold probabilities range. The black and grey line respectively represented the hypothesis that none or all patients would experience.
The nomogram could intuitively and effectively display the influence of the risk model on the prognostic outcome (55). A multi-scale nomogram was constructed on account of independent prognostic factors, which effectively predicted 1-year, 2-year and 3-year OS probabilities of LUAD patients. The scores corresponding to the parameters were calculated to obtain the total points. Thus, high total score was significantly correlated to worse outcome (Figure 5B). Furthermore, calibration curve implied well performance of the nomogram at predicting survival capacity in LUAD patients (Figure 5C). ROC curve analysis and Decision Curve Analysis (DCA) showed that, compared with other independent variables, the nomogram model classifier had the distinctly superior accuracy and net benefit rate (Figures 5D, E).
Correlation between the prognostic model and clinicopathologic features
To further clarify the clinical implication of the EMT signature, we tested the relationship between risk score and clinicopathologic variables by the Chi-square test. LUAD patients were classified into different subgroups under diverse clinical properties, including T stage, N stage, M stage, tumor stage, gender, age, smoking history, status of EGFR. Notably, there was a gradual upward trend in the proportion of high-risk score patients with increase of malignant grade of pathological T stage, pathological N stage, pathological M stage and tumor stage. Regrettably, other clinicopathological characteristics showed no obvious difference in the distribution (Figure 6A). Subsequently, the risk model was also applied to each subgroup for KM survival analysis. We observed that subgroup with high-risk presented dramatically worse OS, suggesting that our specific signature had a precise predictive value (Figure 6B).
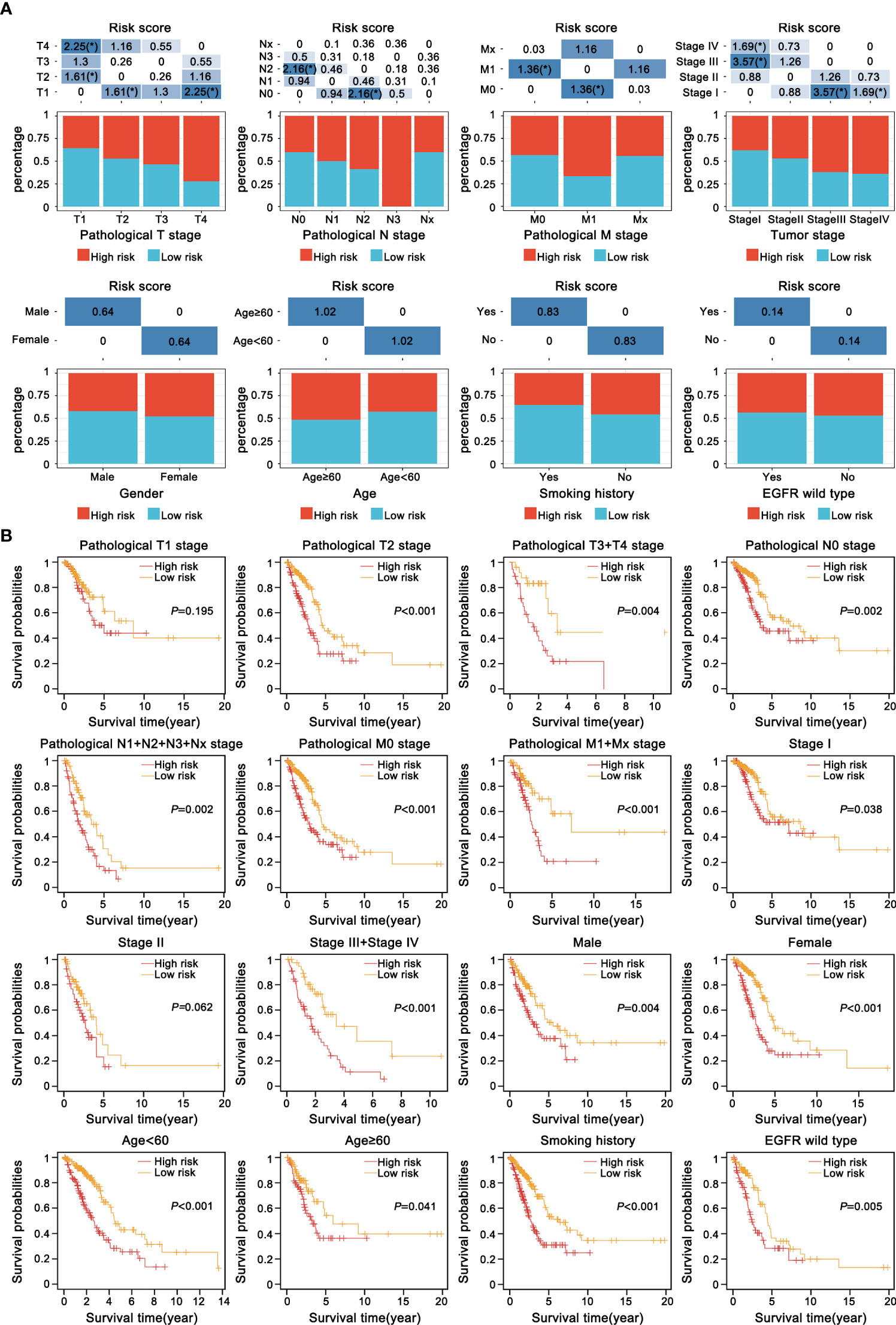
Figure 6 Correlation between the current prognostic E-signature and clinicopathologic attributes. (A) The distribution of samples with different risk scores classified by clinicopathologic traits, including T stage, N stage, M stage, tumour stage, gender, age, smoking history and EGFR mutation. *P < 0.05. (B) KM analysis of each subgroup was stratified by the E-signature to visualize the prognostic value of clinical parameters classification.
Functional annotation and enrichment analysis of the E-signature
The association between risk score and EMT biomarkers was assessed based on the TCGA database (Figure 7A). In order to further observe biological functions of the risk model, ssGSEA method was applied to derive scores of multifarious molecular pathways. The heatmap of hierarchical cluster analysis showed E-signature was enriched in “EPITHELIAL MESENCHYMAL TRANSITION” and other carcinogenic pathways, while metabolic-related pathways such as” HEME METABOLISM” and “BILE ACID METABOLISM” were inversely regulated by our signature (Figure 7B). Moreover, the correlation map visualized the KEGG pathways extracted by correlation coefficient > 0.3 statistically significant associated with the E-signature (Figure 7C). Taken together, the constructed specific signature played a compelling role in promoting tumor development.
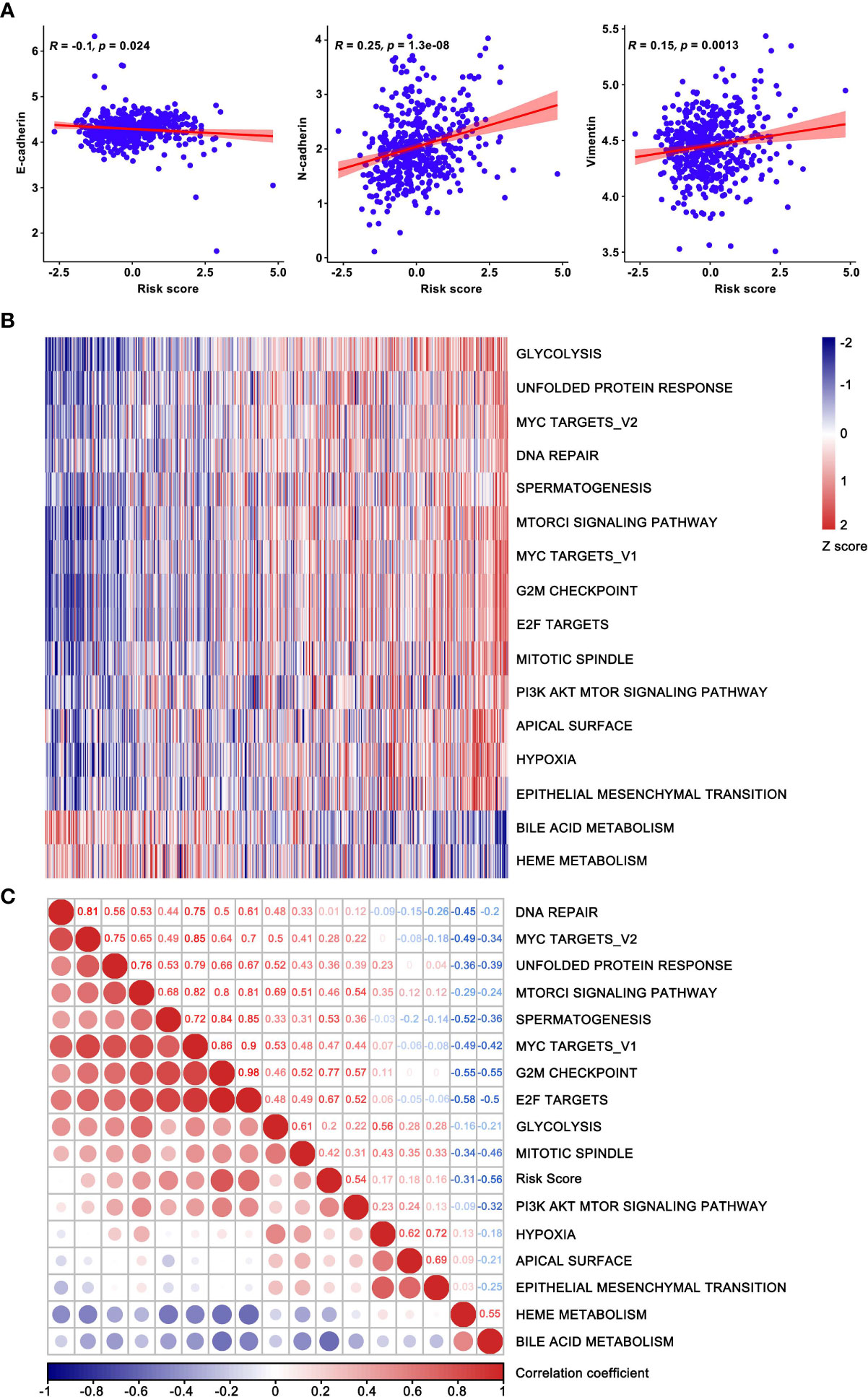
Figure 7 Functional enrichment analysis based on the current prognostic model. (A) The correlation between risk score and EMT biomarkers. (B) Hierarchical clustering analysis was used for heatmap plotting, showing KEGG pathways correlated with the model and coefficients were greater than 0.3. (C) The correlation map confirmed the high-risk group retained the oncogenic pathways. The risk score increased successively from left to right, where the enriched pathways and risk score were used as the abscissa.
Discussion
LUAD is the accepted common classification of lung cancer, accompanied by high prevalence and fatality (2, 56). In cancer patients, metastasis is primary cause of shorten survival and high mortality, and often has already occurred at the time of diagnosis (57, 58). During the procedure of the classical invasion-metastasis cascade, transformation from tumor epithelial cells to mesenchymal cells with the invasion and migration capacity (59). Subsequently, mesenchymal cells locally invade the surrounding matrix and extracellular matrix (ECM), transport and stay in distant organ tissues, eventually extravasate and proliferate to form metastasis (60). The induction and ultimate success of this process depends on EMT and its key regulators (59). Regulation of EMT markers expression, for instance, N-cadherin, Vimentin and E-cadherin, ultimately affects tumor progression, metastasis and drug resistance (61, 62). To date, considerable studies have shown that the marked association of sophisticated regulation of EMT with poor prognosis of lung cancer patients (63, 64). To break down this barrier in clinical settings, molecular biological characteristics should be adequately considered, and circulating tumor markers, TNM staging and other indicators are not accurate enough in predicting the survival of LUAD patients (65, 66). Similarly, single-gene biomarkers are unable to achieve a satisfying prediction result (67). Therefore, a multigene panel might be a promising and reliable method for precision and individualized treatment of LUAD patients.
With rapid advancements in high-throughput sequencing, abundant studies have used data from large communal databases, for example, TCGA and GEO databases, to construct the prognostic signatures for identification of patient risk stratification, guidance of treatment regimens, precise prognostic assessment, and improvement of clinical efficacy (68, 69). Previous studies have reported risk-prediction models based on EMT in LUAD (37, 38). Although the conventional analytical methods used were similar, the genes required for construction of the signatures were fundamentally different, mainly due to the different databases and screening processes. In terms of bioinformatics analysis, our study not only plotted KM curves, ROC curves and nomogram to improve the accuracy and specificity of prognosis prediction, but also generated calibration curves for the nomogram to further prove effective clinical utility of E-signature. Secondly, the DCA (decision curve) was drawn for clarifying clinical feasibility of the risk model and prognostic significance of our signature was emphasized by comparing with known prognostic models (51–53). In addition, compared with the previously reported EMT models, we conducted in vitro experiments in-depth to verify a dramatically correlation between our signature and EMT procedure. Collectively, the specific E-signature we developed could predict the cancer process more comprehensively and accurately, with more stable and robust predictive performance and superior clinical practice (37, 38).
In this study, the E-signature was found to be composed of DKK1, LOXL2, MGP and SLIT3. It is reported that DKK1 (a secreted protein) contains the cysteine-rich domain and is a member of the family of Dickkopf, (70). DKK1 has emerged as an indispensable regulatory factor in multiple cancers and commonly existed as an inhibitor of the Wnt pathway (71–73). Nevertheless, DKK1 acting as a tumor promoter, which played a critical role in the cancer progression (74–76). LOXL2 belonging to the LOX family, has the typical function of catalyzing the cross-link of elastin and collagen in the ECM and has attracted much attention in cancer biology (48). Plenty of studies have revealed that LOXL2 participated in tumor progression, metastasis, poor prognosis and chemoradiotherapy resistance in varied cancers, e.g., lung cancer, breast cancer, pancreatic cancer and colorectal cancer (77–80). MGP, an extracellular matrix protein, whose well-defined function is still unknown, and currently acts as a double-edged sword in cancers (49, 81–84). Upregulation of MGP promoted cancer proliferation, migration and invasion, that was linked with unfavorable prognosis (49, 81–83). Whereas, MGP reversed chemotherapy resistance and received favorable survival outcomes in estrogen receptor positive breast cancer (84). SLIT3, a secreted protein, is widely distributed in normal tissues and mainly participates in the Slit/Robo pathway (85). SLIT3 has rarely been reported in human cancers, which could inhibit the progression of thyroid cancer (86).
Conclusion
In summary, we developed and validated a trustworthy and powerful signature, which could serve as an independent and promising biomarker to optimize prognosis and surveillance protocols for individual LUAD patients.
Data availability statement
The datasets presented in this study can be found in online repositories. The names of the repository/repositories and accession number(s) can be found in the article/Supplementary Material.
Ethics statement
The study conducted in accordance with the guidelines of the Declaration of Helsinki, and was reviewed and approved by the Ethics Committee of Harbin Medical University. The need for written informed consent for participation was not required for this study in accordance with the national legislation and institutional requirements.
Author contributions
LC and YX designed and supervised the research. YMC completed the first draft of manuscript. XW and JN collected pathological information of LUAD patients. YWC provided technical support for bioinformatics and RG carried out experiments, besides, WL offered auxiliary services. LZ performed statistical analysis and conducted the figures. YMC revised the manuscript and figures. All authors contributed to the article and approved the submitted version.
Conflict of interest
The authors declare that the research was conducted in the absence of any commercial or financial relationships that could be construed as a potential conflict of interest.
Publisher’s note
All claims expressed in this article are solely those of the authors and do not necessarily represent those of their affiliated organizations, or those of the publisher, the editors and the reviewers. Any product that may be evaluated in this article, or claim that may be made by its manufacturer, is not guaranteed or endorsed by the publisher.
Supplementary material
The Supplementary Material for this article can be found online at: https://www.frontiersin.org/articles/10.3389/fonc.2022.974614/full#supplementary-material
References
1. Siegel RL, Miller KD, Fuchs HE, Jemal A. Cancer statistics 2022. CA Cancer J Clin (2022) 72(1):7–33. doi: 10.3322/caac.21708
2. Sung H, Ferlay J, Siegel RL, Laversanne M, Soerjomataram I, Jemal A, et al. Global cancer statistics 2020: Globocan estimates of incidence and mortality worldwide for 36 cancers in 185 countries. CA Cancer J Clin (2021) 71(3):209–49. doi: 10.3322/caac.21660
3. Jang HR, Shin SB, Kim CH, Won JY, Xu R, Kim DE, et al. Plk1/Vimentin signaling facilitates immune escape by recruiting Smad2/3 to pd-L1 promoter in metastatic lung adenocarcinoma. Cell Death Differ (2021) 28(9):2745–64. doi: 10.1038/s41418-021-00781-4
4. Stinchcombe TE, Bradley JD. Thoracic oncology: Current standard therapy and future developments. J Clin Oncol (2022) 40(6):527–9. doi: 10.1200/JCO.21.02396
5. Liang W, Liu J, He J. Driving the improvement of lung cancer prognosis. Cancer Cell (2020) 38(4):449–51. doi: 10.1016/j.ccell.2020.09.008
6. Fujikawa R, Muraoka Y, Kashima J, Yoshida Y, Ito K, Watanabe H, et al. Clinicopathologic and genotypic features of lung adenocarcinoma characterized by the international association for the study of lung cancer grading system. J Thorac Oncol (2022) 17(5):700–7. doi: 10.1016/j.jtho.2022.02.005
7. Ganesh K, Massague J. Targeting metastatic cancer. Nat Med (2021) 27(1):34–44. doi: 10.1038/s41591-020-01195-4
8. Cheng WC, Chang CY, Lo CC, Hsieh CY, Kuo TT, Tseng GC, et al. Identification of theranostic factors for patients developing metastasis after surgery for early-stage lung adenocarcinoma. Theranostics (2021) 11(8):3661–75. doi: 10.7150/thno.53176
9. Han Y, Wang D, Peng L, Huang T, He X, Wang J, et al. Single-cell sequencing: A promising approach for uncovering the mechanisms of tumor metastasis. J Hematol Oncol (2022) 15(1):59. doi: 10.1186/s13045-022-01280-w
10. Weiss F, Lauffenburger D, Friedl P. Towards targeting of shared mechanisms of cancer metastasis and therapy resistance. Nat Rev Cancer (2022) 22(3):157–73. doi: 10.1038/s41568-021-00427-0
11. Massague J, Ganesh K. Metastasis-initiating cells and ecosystems. Cancer Discovery (2021) 11(4):971–94. doi: 10.1158/2159-8290.CD-21-0010
12. Vieugue P, Blanpain C. Recording emt activity by lineage tracing during metastasis. Dev Cell (2020) 54(5):567–9. doi: 10.1016/j.devcel.2020.07.011
13. Yang J, Antin P, Berx G, Blanpain C, Brabletz T, Bronner M, et al. Guidelines and definitions for research on epithelial-mesenchymal transition. Nat Rev Mol Cell Biol (2020) 21(6):341–52. doi: 10.1038/s41580-020-0237-9
14. Dongre A, Weinberg RA. New insights into the mechanisms of epithelial-mesenchymal transition and implications for cancer. Nat Rev Mol Cell Biol (2019) 20(2):69–84. doi: 10.1038/s41580-018-0080-4
15. Lambert AW, Weinberg RA. Linking emt programmes to normal and neoplastic epithelial stem cells. Nat Rev Cancer (2021) 21(5):325–38. doi: 10.1038/s41568-021-00332-6
16. Liang H, Yu T, Han Y, Jiang H, Wang C, You T, et al. Lncrna ptar promotes emt and invasion-metastasis in serous ovarian cancer by competitively binding mir-101-3p to regulate Zeb1 expression. Mol Cancer (2018) 17(1):119. doi: 10.1186/s12943-018-0870-5
17. Lourenco AR, Ban Y, Crowley MJ, Lee SB, Ramchandani D, Du W, et al. Differential contributions of pre- and post-emt tumor cells in breast cancer metastasis. Cancer Res (2020) 80(2):163–9. doi: 10.1158/0008-5472.CAN-19-1427
18. Huang T, Song X, Xu D, Tiek D, Goenka A, Wu B, et al. Stem cell programs in cancer initiation, progression, and therapy resistance. Theranostics (2020) 10(19):8721–43. doi: 10.7150/thno.41648
19. Gu Y, Zhang J, Zhou Z, Liu D, Zhu H, Wen J, et al. Metastasis patterns and prognosis of octogenarians with nsclc: A population-based study. Aging Dis (2020) 11(1):82–92. doi: 10.14336/AD.2019.0414
20. Singh M, Yelle N, Venugopal C, Singh SK. Emt: Mechanisms and therapeutic implications. Pharmacol Ther (2018) 182:80–94. doi: 10.1016/j.pharmthera.2017.08.009
21. Nieto MA, Huang RY, Jackson RA, Thiery JP. Emt: 2016. Cell (2016) 166(1):21–45. doi: 10.1016/j.cell.2016.06.028
22. Lamouille S, Xu J, Derynck R. Molecular mechanisms of epithelial-mesenchymal transition. Nat Rev Mol Cell Biol (2014) 15(3):178–96. doi: 10.1038/nrm3758
23. Hu Z, Cunnea P, Zhong Z, Lu H, Osagie OI, Campo L, et al. The Oxford classic links epithelial-to-Mesenchymal transition to immunosuppression in poor prognosis ovarian cancers. Clin Cancer Res (2021) 27(5):1570–9. doi: 10.1158/1078-0432.CCR-20-2782
24. Consortium ITP-CAoWG. Pan-cancer analysis of whole genomes. Nature (2020) 578(7793):82–93. doi: 10.1038/s41586-020-1969-6
25. Liu J, Lichtenberg T, Hoadley KA, Poisson LM, Lazar AJ, Cherniack AD, et al. An integrated tcga pan-cancer clinical data resource to drive high-quality survival outcome analytics. Cell (2018) 173(2):400–16 e11. doi: 10.1016/j.cell.2018.02.052
26. Chang JT, Lee YM, Huang RS. The impact of the cancer genome atlas on lung cancer. Transl Res (2015) 166(6):568–85. doi: 10.1016/j.trsl.2015.08.001
27. Hutter C, Zenklusen JC. The cancer genome atlas: Creating lasting value beyond its data. Cell (2018) 173(2):283–5. doi: 10.1016/j.cell.2018.03.042
28. Yan S, Wong KC. Gesgnext: Gene expression signature extraction and meta-analysis on gene expression omnibus. IEEE J BioMed Health Inform (2020) 24(1):311–8. doi: 10.1109/JBHI.2019.2896144
29. Wang S, Xiong Y, Zhang Q, Su D, Yu C, Cao Y, et al. Clinical significance and immunogenomic landscape analyses of the immune cell signature based prognostic model for patients with breast cancer. Brief Bioinform (2021) 22(4):1093–107. doi: 10.1093/bib/bbaa311
30. Chen R, Goodison S, Sun Y. Molecular profiles of matched primary and metastatic tumor samples support a linear evolutionary model of breast cancer. Cancer Res (2020) 80(2):170–4. doi: 10.1158/0008-5472.CAN-19-2296
31. Liu M, Tong L, Liang B, Song X, Xie L, Peng H, et al. A 15-gene signature and prognostic nomogram for predicting overall survival in non-distant metastatic oral tongue squamous cell carcinoma. Front Oncol (2021) 11:587548. doi: 10.3389/fonc.2021.587548
32. Feng C, Liu S, Shang Z. Identification and validation of an emt-related lncrna signature for hnscc to predict survival and immune landscapes. Front Cell Dev Biol (2021) 9:798898. doi: 10.3389/fcell.2021.798898
33. Yu H, Gu D, Yue C, Xu J, Yan F, He X. An immune cell-based signature associating with emt phenotype predicts postoperative overall survival of escc. Front Oncol (2021) 11:636479. doi: 10.3389/fonc.2021.636479
34. Du Y, Wang B, Jiang X, Cao J, Yu J, Wang Y, et al. Identification and validation of a stromal emt related lncrna signature as a potential marker to predict bladder cancer outcome. Front Oncol (2021) 11:620674. doi: 10.3389/fonc.2021.620674
35. Lin WP, Xing KL, Fu JC, Ling YH, Li SH, Yu WS, et al. Development and validation of a model including distinct vascular patterns to estimate survival in hepatocellular carcinoma. JAMA Netw Open (2021) 4(9):e2125055. doi: 10.1001/jamanetworkopen.2021.25055
36. Han Y, Wong FC, Wang D, Kahlert C. An in silico analysis reveals an emt-associated gene signature for predicting recurrence of early-stage lung adenocarcinoma. Cancer Inform (2022) 21:11769351221100727. doi: 10.1177/11769351221100727
37. Tang Y, Jiang Y, Qing C, Wang J, Zeng Z. Systematic construction and validation of an epithelial-mesenchymal transition risk model to predict prognosis of lung adenocarcinoma. Aging (Albany NY) (2020) 13(1):794–812. doi: 10.18632/aging.202186
38. Shi J, Wang Z, Guo J, Chen Y, Tong C, Tong J, et al. Identification of a three-gene signature based on epithelial-mesenchymal transition of lung adenocarcinoma through construction and validation of a risk-prediction model. Front Oncol (2021) 11:726834. doi: 10.3389/fonc.2021.726834
39. Blanco JL, Porto-Pazos AB, Pazos A, Fernandez-Lozano C. Prediction of high anti-angiogenic activity peptides in silico using a generalized linear model and feature selection. Sci Rep (2018) 8(1):15688. doi: 10.1038/s41598-018-33911-z
40. Wysocki AC, Rhemtulla M. On penalty parameter selection for estimating network models. Multivar Behav Res (2021) 56(2):288–302. doi: 10.1080/00273171.2019.1672516
41. Zhang JH, Hou R, Pan Y, Gao Y, Yang Y, Tian W, et al. A five-microrna signature for individualized prognosis evaluation and radiotherapy guidance in patients with diffuse lower-grade glioma. J Cell Mol Med (2020) 24(13):7504–14. doi: 10.1111/jcmm.15377
42. Wang Q, Wang Z, Li G, Zhang C, Bao Z, Wang Z, et al. Identification of idh-mutant gliomas by a prognostic signature according to gene expression profiling. Aging (Albany NY) (2018) 10(8):1977–88. doi: 10.18632/aging.101521
43. Balachandran VP, Gonen M, Smith JJ, DeMatteo RP. Nomograms in oncology: More than meets the eye. Lancet Oncol (2015) 16(4):e173–80. doi: 10.1016/S1470-2045(14)71116-7
44. Mo S, Cai X, Zhou Z, Li Y, Hu X, Ma X, et al. Nomograms for predicting specific distant metastatic sites and overall survival of colorectal cancer patients: A Large population-based real-world study. Clin Transl Med (2020) 10(1):169–81. doi: 10.1002/ctm2.20
45. Yu J, Liu TT, Liang LL, Liu J, Cai HQ, Zeng J, et al. Identification and validation of a novel glycolysis-related gene signature for predicting the prognosis in ovarian cancer. Cancer Cell Int (2021) 21(1):353. doi: 10.1186/s12935-021-02045-0
46. Macaskill P. Standard deviation and standard error: Interpretation, usage and reporting. Med J Aust (2018) 208(2):63–4. doi: 10.5694/mja17.00633
47. Qi L, Sun B, Liu Z, Li H, Gao J, Leng X. Dickkopf-1 inhibits epithelial-mesenchymal transition of colon cancer cells and contributes to colon cancer suppression. Cancer Sci (2012) 103(4):828–35. doi: 10.1111/j.1349-7006.2012.02222.x
48. Wen B, Xu LY, Li EM. Loxl2 in cancer: Regulation, downstream effectors and novel roles. Biochim Biophys Acta Rev Cancer (2020) 1874(2):188435. doi: 10.1016/j.bbcan.2020.188435
49. Gong C, Zou J, Zhang M, Zhang J, Xu S, Zhu S, et al. Upregulation of mgp by Hoxc8 promotes the proliferation, migration, and emt processes of triple-negative breast cancer. Mol Carcinog (2019) 58(10):1863–75. doi: 10.1002/mc.23079
50. Zhang C, Guo H, Li B, Sui C, Zhang Y, Xia X, et al. Effects of Slit3 silencing on the invasive ability of lung carcinoma A549 cells. Oncol Rep (2015) 34(2):952–60. doi: 10.3892/or.2015.4031
51. Zhu J, Wang M, Hu D. Development of an autophagy-related gene prognostic signature in lung adenocarcinoma and lung squamous cell carcinoma. PeerJ (2020) 8:e8288. doi: 10.7717/peerj.8288
52. Ma B, Geng Y, Meng F, Yan G, Song F. Identification of a sixteen-gene prognostic biomarker for lung adenocarcinoma using a machine learning method. J Cancer (2020) 11(5):1288–98. doi: 10.7150/jca.34585
53. Zhang L, Zhang Z, Yu Z. Identification of a novel glycolysis-related gene signature for predicting metastasis and survival in patients with lung adenocarcinoma. J Transl Med (2019) 17(1):423. doi: 10.1186/s12967-019-02173-2
54. Zhang M, Zhu K, Pu H, Wang Z, Zhao H, Zhang J, et al. An immune-related signature predicts survival in patients with lung adenocarcinoma. Front Oncol (2019) 9:1314. doi: 10.3389/fonc.2019.01314
55. Tian S, Li Q, Li R, Chen X, Tao Z, Gong H, et al. Development and validation of a prognostic nomogram for hypopharyngeal carcinoma. Front Oncol (2021) 11:696952. doi: 10.3389/fonc.2021.696952
56. Adib E, Nassar AH, Abou Alaiwi S, Groha S, Akl EW, Sholl LM, et al. Variation in targetable genomic alterations in non-small cell lung cancer by genetic ancestry, sex, smoking history, and histology. Genome Med (2022) 14(1):39. doi: 10.1186/s13073-022-01041-x
57. Klein CA. Cancer progression and the invisible phase of metastatic colonization. Nat Rev Cancer (2020) 20(11):681–94. doi: 10.1038/s41568-020-00300-6
58. Jiang C, Zhang N, Hu X, Wang H. Tumor-associated exosomes promote lung cancer metastasis through multiple mechanisms. Mol Cancer (2021) 20(1):117. doi: 10.1186/s12943-021-01411-w
59. Yeung KT, Yang J. Epithelial-mesenchymal transition in tumor metastasis. Mol Oncol (2017) 11(1):28–39. doi: 10.1002/1878-0261.12017
60. Lu J. The warburg metabolism fuels tumor metastasis. Cancer Metastasis Rev (2019) 38(1-2):157–64. doi: 10.1007/s10555-019-09794-5
61. Zhang N, Ng AS, Cai S, Li Q, Yang L, Kerr D. Novel therapeutic strategies: Targeting epithelial-mesenchymal transition in colorectal cancer. Lancet Oncol (2021) 22(8):e358–e68. doi: 10.1016/S1470-2045(21)00343-0
62. Wu J, He Z, Yang XM, Li KL, Wang DL, Sun FL. Rccd1 depletion attenuates tgf-Beta-Induced emt and cell migration by stabilizing cytoskeletal microtubules in nsclc cells. Cancer Lett (2017) 400:18–29. doi: 10.1016/j.canlet.2017.04.021
63. Pan Z, Cai J, Lin J, Zhou H, Peng J, Liang J, et al. A novel protein encoded by Circfndc3b inhibits tumor progression and emt through regulating snail in colon cancer. Mol Cancer (2020) 19(1):71. doi: 10.1186/s12943-020-01179-5
64. Yang S, Liu Y, Li MY, Ng CSH, Yang SL, Wang S, et al. Foxp3 promotes tumor growth and metastasis by activating Wnt/Beta-catenin signaling pathway and emt in non-small cell lung cancer. Mol Cancer (2017) 16(1):124. doi: 10.1186/s12943-017-0700-1
65. Zhang Z, Chen J, Zhu S, Zhu D, Xu J, He G. Construction and validation of a cell cycle-related robust prognostic signature in colon cancer. Front Cell Dev Biol (2020) 8:611222. doi: 10.3389/fcell.2020.611222
66. Mo S, Dai W, Zhou Z, Gu R, Li Y, Xiang W, et al. Comprehensive transcriptomic analysis reveals prognostic value of an emt-related gene signature in colorectal cancer. Front Cell Dev Biol (2021) 9:681431. doi: 10.3389/fcell.2021.681431
67. Nguyen LC, Naulaerts S, Bruna A, Ghislat G, Ballester PJ. Predicting cancer drug response in vivo by learning an optimal feature selection of tumour molecular profiles. Biomedicines (2021) 9(10):1319–43. doi: 10.3390/biomedicines9101319
68. Qi L, Li T, Shi G, Wang J, Li X, Zhang S, et al. An individualized gene expression signature for prediction of lung adenocarcinoma metastases. Mol Oncol (2017) 11(11):1630–45. doi: 10.1002/1878-0261.12137
69. Qi L, Li Y, Qin Y, Shi G, Li T, Wang J, et al. An individualised signature for predicting response with concordant survival benefit for lung adenocarcinoma patients receiving platinum-based chemotherapy. Br J Cancer (2016) 115(12):1513–9. doi: 10.1038/bjc.2016.370
70. D’Amico L, Mahajan S, Capietto AH, Yang Z, Zamani A, Ricci B, et al. Dickkopf-related protein 1 (Dkk1) regulates the accumulation and function of myeloid derived suppressor cells in cancer. J Exp Med (2016) 213(5):827–40. doi: 10.1084/jem.20150950
71. Jiang J, Li J, Yao W, Wang W, Shi B, Yuan F, et al. Foxc1 negatively regulates Dkk1 expression to promote gastric cancer cell proliferation through activation of wnt signaling pathway. Front Cell Dev Biol (2021) 9:662624. doi: 10.3389/fcell.2021.662624
72. Zhuang X, Zhang H, Li X, Li X, Cong M, Peng F, et al. Differential effects on lung and bone metastasis of breast cancer by wnt signalling inhibitor Dkk1. Nat Cell Biol (2017) 19(10):1274–85. doi: 10.1038/ncb3613
73. Niu J, Li XM, Wang X, Liang C, Zhang YD, Li HY, et al. Dkk1 inhibits breast cancer cell migration and invasion through suppression of beta-Catenin/Mmp7 signaling pathway. Cancer Cell Int (2019) 19:168. doi: 10.1186/s12935-019-0883-1
74. Lyros O, Lamprecht AK, Nie L, Thieme R, Gotzel K, Gasparri M, et al. Dickkopf-1 (Dkk1) promotes tumor growth Via akt-phosphorylation and independently of wnt-axis in barrett’s associated esophageal adenocarcinoma. Am J Cancer Res (2019) 9(2):330–46.
75. Feng Y, Zhang Y, Wei X, Zhang Q. Correlations of Dkk1 with pathogenesis and prognosis of human multiple myeloma. Cancer biomark (2019) 24(2):195–201. doi: 10.3233/CBM-181909
76. Shen Q, Yang XR, Tan Y, You H, Xu Y, Chu W, et al. High level of serum protein Dkk1 predicts poor prognosis for patients with hepatocellular carcinoma after hepatectomy. Hepat Oncol (2015) 2(3):231–44. doi: 10.2217/hep.15.12
77. Ferreira S, Saraiva N, Rijo P, Fernandes AS. Loxl2 inhibitors and breast cancer progression. Antioxid (Basel) (2021) 10(2):312–27. doi: 10.3390/antiox10020312
78. Li R, Li H, Zhu L, Zhang X, Liu D, Li Q, et al. Reciprocal regulation of Loxl2 and Hif1alpha drives the warburg effect to support pancreatic cancer aggressiveness. Cell Death Dis (2021) 12(12):1106. doi: 10.1038/s41419-021-04391-3
79. Peng DH, Ungewiss C, Tong P, Byers LA, Wang J, Canales JR, et al. Zeb1 induces Loxl2-mediated collagen stabilization and deposition in the extracellular matrix to drive lung cancer invasion and metastasis. Oncogene (2017) 36(14):1925–38. doi: 10.1038/onc.2016.358
80. Zheng GL, Liu YL, Yan ZX, Xie XY, Xiang Z, Yin L, et al. Elevated Loxl2 expression by Linc01347/Mir-328-5p axis contributes to 5-fu chemotherapy resistance of colorectal cancer. Am J Cancer Res (2021) 11(4):1572–85.
81. Sterzynska K, Klejewski A, Wojtowicz K, Swierczewska M, Andrzejewska M, Rusek D, et al. The role of matrix gla protein (Mgp) expression in paclitaxel and topotecan resistant ovarian cancer cell lines. Int J Mol Sci (2018) 19(10):2901–17. doi: 10.3390/ijms19102901
82. Huang C, Wang M, Wang J, Wu D, Gao Y, Huang K, et al. Suppression mgp inhibits tumor proliferation and reverses oxaliplatin resistance in colorectal cancer. Biochem Pharmacol (2021) 189:114390. doi: 10.1016/j.bcp.2020.114390
83. Li X, Wei R, Wang M, Ma L, Zhang Z, Chen L, et al. Mgp promotes colon cancer proliferation by activating the nf-kappab pathway through upregulation of the calcium signaling pathway. Mol Ther Oncol (2020) 17:371–83. doi: 10.1016/j.omto.2020.04.005
84. Tuo YL, Ye YF. Mgp is downregulated due to promoter methylation in chemoresistant er+ breast cancer and high mgp expression predicts better survival outcomes. Eur Rev Med Pharmacol Sci (2017) 21(17):3871–8.
85. Ni W, Liu T, Wang HY, Liu LH, Chen GX. [Expression of Slit3/Robo signal pathway in mouse aortic smooth muscle cell and its impact on proliferation and migration]. Zhonghua Xin Xue Guan Bing Za Zhi (2016) 44(6):542–7. doi: 10.3760/cma.j.issn.0253-3758.2016.06.016
Keywords: lung adenocarcinoma, EMT, signature, prognosis, nomogram
Citation: Cui Y, Wang X, Zhang L, Liu W, Ning J, Gu R, Cui Y, Cai L and Xing Y (2022) A novel epithelial-mesenchymal transition (EMT)-related gene signature of predictive value for the survival outcomes in lung adenocarcinoma. Front. Oncol. 12:974614. doi: 10.3389/fonc.2022.974614
Received: 21 June 2022; Accepted: 30 August 2022;
Published: 15 September 2022.
Edited by:
Tao Sun, Nankai University, ChinaReviewed by:
Yiping Zou, Tianjin Medical University Cancer Institute and Hospital, ChinaLiming Chen, Nanjing Normal University, China
Chunlin Ou, Xiangya Hospital, Central South University, China
Copyright © 2022 Cui, Wang, Zhang, Liu, Ning, Gu, Cui, Cai and Xing. This is an open-access article distributed under the terms of the Creative Commons Attribution License (CC BY). The use, distribution or reproduction in other forums is permitted, provided the original author(s) and the copyright owner(s) are credited and that the original publication in this journal is cited, in accordance with accepted academic practice. No use, distribution or reproduction is permitted which does not comply with these terms.
*Correspondence: Ying Xing, eGluZ3lpbmdAaHJibXUuZWR1LmNu; Li Cai, Y2FpbGlAZW1zLmhyYm11LmVkdS5jbg==
†These authors have contributed equally to this work