- 1Department of Radiology, Hospital of Chengdu University of Traditional Chinese Medicine, Chengdu, Sichuan, China
- 2Toxicology Department, West China-Frontier PharmaTech Co., Ltd. (WCFP), Chengdu, Sichuan, China
Objective: The aim of this study was to assess the diagnostic performance of radiomics-based MRI in predicting microvascular invasion (MVI) in hepatocellular carcinoma (HCC).
Method: The databases of PubMed, Cochrane library, Embase, Web of Science, Ovid MEDLINE, Springer, and Science Direct were searched for original studies from their inception to 20 August 2022. The quality of each study included was assessed according to the Quality Assessment of Diagnostic Accuracy Studies 2 and the radiomics quality score. The pooled sensitivity, specificity, positive likelihood ratio (PLR), negative likelihood ratio (NLR), and diagnostic odds ratio (DOR) were calculated. The summary receiver operating characteristic (SROC) curve was plotted and the area under the curve (AUC) was calculated to evaluate the diagnostic accuracy. Sensitivity analysis and subgroup analysis were performed to explore the source of the heterogeneity. Deeks’ test was used to assess publication bias.
Results: A total of 15 studies involving 981 patients were included. The pooled sensitivity, specificity, PLR, NLR, DOR, and AUC were 0.79 (95%CI: 0.72–0.85), 0.81 (95%CI: 0.73–0.87), 4.1 (95%CI:2.9–5.9), 0.26 (95%CI: 0.19–0.35), 16 (95%CI: 9–28), and 0.87 (95%CI: 0.84–0.89), respectively. The results showed great heterogeneity among the included studies. Sensitivity analysis indicated that the results of this study were statistically reliable. The results of subgroup analysis showed that hepatocyte-specific contrast media (HSCM) had equivalent sensitivity and equivalent specificity compared to the other set. The least absolute shrinkage and selection operator method had high sensitivity and specificity than other methods, respectively. The investigated area of the region of interest had high specificity compared to the volume of interest. The imaging-to-surgery interval of 15 days had higher sensitivity and slightly low specificity than the others. Deeks’ test indicates that there was no publication bias (P=0.71).
Conclusion: Radiomics-based MRI has high accuracy in predicting MVI in HCC, and it can be considered as a non-invasive method for assessing MVI in HCC.
Introduction
Hepatocellular carcinoma (HCC) is the most common primary liver malignant tumor, which is also the third leading cause of cancer death (1, 2). Hepatectomy and liver transplantation are still the main treatments for HCC (3, 4). Despite curative therapies, the prognosis of HCC patients remains poor, with 5-year recurrence rates reaching 50%–70% after hepatectomy and <35% after liver transplantation (5–7). It was proven that 15.0%–57.1% of patients presented microvascular invasion (MVI) after hepatectomy, which is a well-established risk factor for postoperative recurrence (8–10). In addition, the 5-year survival rate of patients with MVI significantly declined (11). For the MVI-positive patients, a wide resection margin is recommended. Therefore, an accurate prediction of MVI before operation is of great importance for clinical treatment decision and prognosis evaluation.
MVI is defined as the cancer cell nest in small vessels lined with endothelium, which is visible only under microscopy (12). Conventional imaging methods are of limited value and pose a challenge for non-invasive diagnosis in assessing MVI in HCC. In recent years, radiomics has been widely applied in the tumor diagnosis, the evaluation of response to treatment, and prognosis prediction. As a new and non-invasive technology, radiomics can high-throughput-extract features from large quantities of images to improve diagnostic or prognostic accuracy, which is also effective to preoperatively predict MVI (13). As imaging markers, the extracted radiomics feature can reflect the microscopic pathological changes of the tumor (Supplementary Figure S1), which is promising in the diagnosis of carcinomas (14).
MRI can also provide better soft-tissue resolution, multiparameters, and more stable features for assessing tumor heterogeneity. Previous similar studies have included CT-, MRI-, and US-combined radiomics original studies (13–15). Although they made a subgroup analysis of different imaging modalities, the number of MRI-based radiomics studies included was small. There is no unified conclusion regarding the accuracy of radiomics-based MRI for predicting MVI in HCCs. The current meta-analysis aimed to comprehensively and systematically assess the accuracy of radiomics-based MRI in evaluating the MVI of HCCs.
Materials and methods
Patients, public-involvement patients, and the public were not involved in this study.
Searching strategies
The literature search was independently performed by two radiologists. The databases were searched from their inception to 20 August 2022 including PubMed, Cochrane Library, Embase, Web of Science, Ovid MEDLINE, Springer, and ScienceDirect. The search terms were “hepatocellular carcinoma,” “liver malignant tumor,” “liver cancer,” “liver cell carcinoma,” “texture analysis,” “radiomics,” “advanced analysis,” etc. The titles and abstracts were searched for their relevance. Disagreements were discussed and resolved to reach a consensus. In addition, the search strategy is presented in detail in Supplementary File 1.
Study selection
Studies were selected according to the following criteria: (1) original research studies. (2) HCC patients with MVI were confirmed by biopsy or histopathology. (3) Data were available and could be extracted for calculating the true-positive (TP), false-positive (FP), true-negative (TN), and false-negative (FN) values. (4) MRI-based radiomics was applied to predict MVI in HCC. (5) English literature: the excluding criteria were case reports, reviews, abstracts, meta-analyses, insufficient calculable data, or animal studies.
Data extraction
The relevant information extracted from the original study was as follows: the first author, the year of publication, country and language, sample size, research type, gold standard, the age of patients, TP, FP, FN, TN, MRI field strengths, and radiomics software. When there is a disagreement in the process of document screening and data extraction, the third radiologist will discuss and resolve it.
Quality assessment of included studies
The quality of each study was assessed on the basis of the Quality Assessment of Diagnostic Accuracy Studies 2 (QUADAS-2) guideline and the radiomics quality score (RQS) (16, 17), which is recommended by the Cochrane collaboration web. The QUADAS-2 tool consists of four parts: (1) patient selection; (2) index test; (3) reference standard; and (4) flow and timing. The RQS checklist is described in Supplemental Table S1.
Statistical analysis
Meta-analysis was performed by Stata version 15.1, and Review Manager software, version 5.3. We adopted a bivariate random effects model to calculate the pooled estimates in advance. The Cochran-Q method and inconsistency index (I2) were used to investigate heterogeneity among the studies. If I2 > 50%, P < 0.05, the observed heterogeneity was significant. If I2 < 50%, P > 0.05, the observed heterogeneity was not significant. If there were obvious heterogeneity, the Spearman’s correlation coefficient was used to assess the threshold effect between the sensitivity logit and the specificity logit. If there were no threshold effect, sensitivity analysis and subgroup analysis were performed to further investigate the cause of the heterogeneity.
Pooled sensitivity (Sen), specificity (Spec), PLR, NLR, and DOR were calculated to assess the diagnostic performance of radiomics-based MRI. The summary receiver operating characteristic (SROC) curve was plotted, and the area under the curve (AUC) was calculated. Deeks’ test was used to evaluate publication bias, and P > 0.05, which indicates that there was no significant bias.
Clinical utility
A Fagan plot was used to evaluate the clinical utility, which demonstrated the posttest probability (P post) of MVI when pretest probabilities were calculated.
Results
Research and selection of studies
A total of 661 relevant studies were initially identified from multidatabases, and 229 duplicated articles were excluded. Additionally, 385 records were removed after reading their titles and abstracts and being deemed irrelevant. Subsequently, after reading the full texts, 28 articles were found to be reviews or not related to the MVI of HCC, and 4 articles were unavailable for data extraction. Ultimately, 15 articles were included (18–32). The literature search process is shown in Figure 1.
Study characteristics
The characteristics of the included studies are shown in Tables 1, 2. All 15 studies were retrospective cohort studies. The total number of patients was 981. From the included studies, the number of MVIs and no MVIs were reported and the pathological histology was used as reference standards. Six studies used hepatocyte-specific contrast media (HSCM). The LASSO method and other methods were used as the method for selection in 11 studies and 4 studies, respectively.
Quality assessment and publication bias
The quality of the included studies was evaluated according to the QUADAS-2 checklist, and the results are shown in detail in Table 3. It was observed that the ‘index test’ in the ‘risk of bias’ and ‘applicability concerns’ revealed uncertain shortcomings, which may suggest bias regarding inclusion. Overall, the quality of all included studies was satisfactory. Deeks’ funnel plot asymmetry test was used to assess the potential publication bias. The results indicated that there was no significant bias (P = 0.71), which are shown in Figure 2. The 15 studies reached a mean ± standard deviation RQS of 14.80 ± 1.57, median 16, and range 12–17. The average percentage RQS was 20.6% with a maximum of 47.2%. The RQS individual scores and inter-rater agreement are presented in Supplemental Tables S2, S3.The RQS was reached with good inter-rater agreement (ICC 0.977, 95% CI 0.934–0.992).
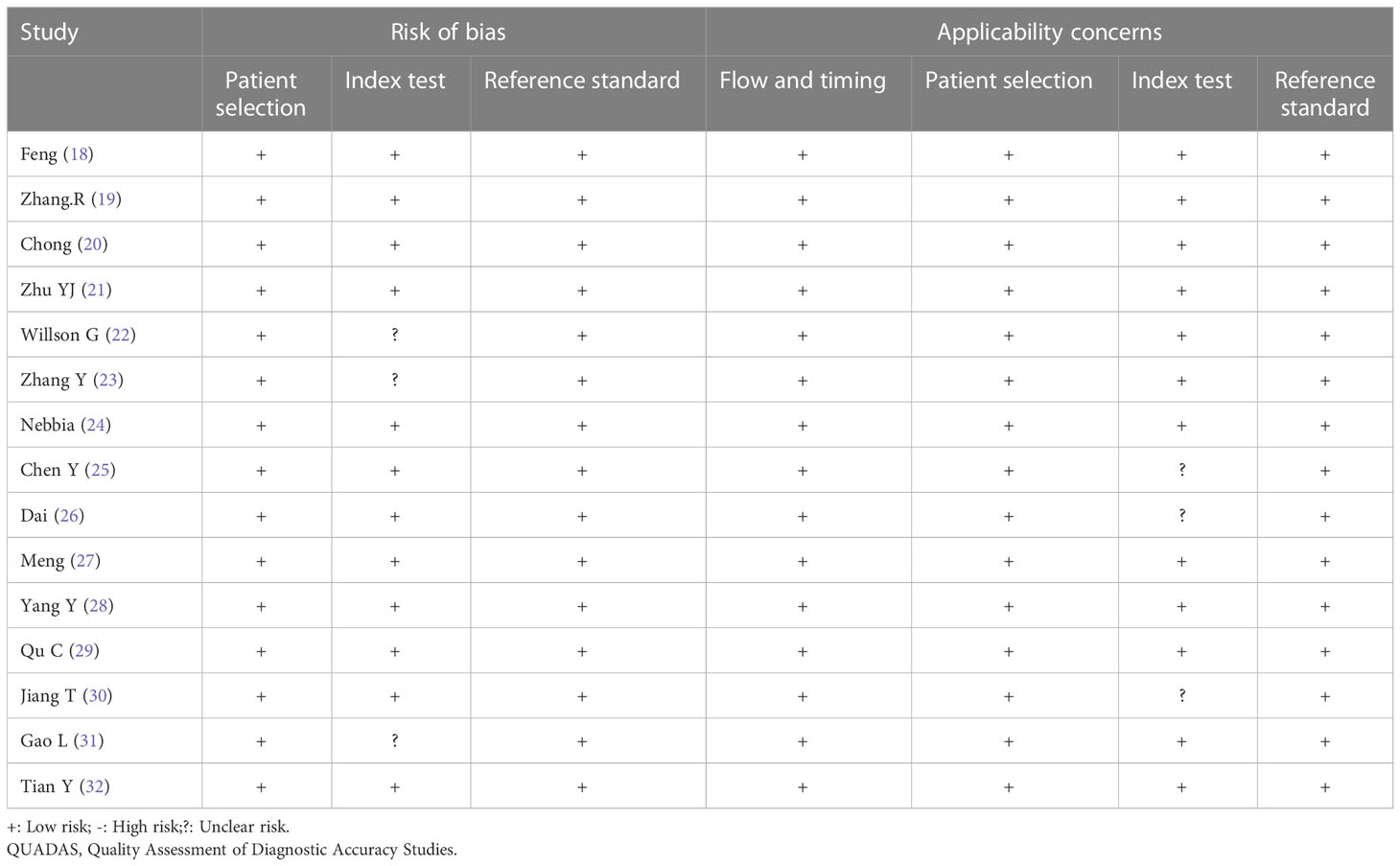
Table 3 Results of the Quality Assessment of Diagnostic Accuracy Studies 2 (QUADAS-2) quality assessment of included studies.
Meta-analysis
The results of the meta-analysis are presented in Figures 3, 4. Pooled sensitivity and specificity were 0.79 (95% CI 0.72–0.85) and 0.81 (95% CI 0.73–0.87), respectively. The values of PLR, NLR, and DOR were 4.1 (95% CI 2.9–5.9), 0.26 (95% CI 0.19–0.35), and 16 (95% CI 9–28), respectively. The AUC of SROC was 0.87 (95% CI 0.84–0.89). These findings indicated that radiomics-based MRI has a high diagnostic performance for evaluating MVI in HCC.
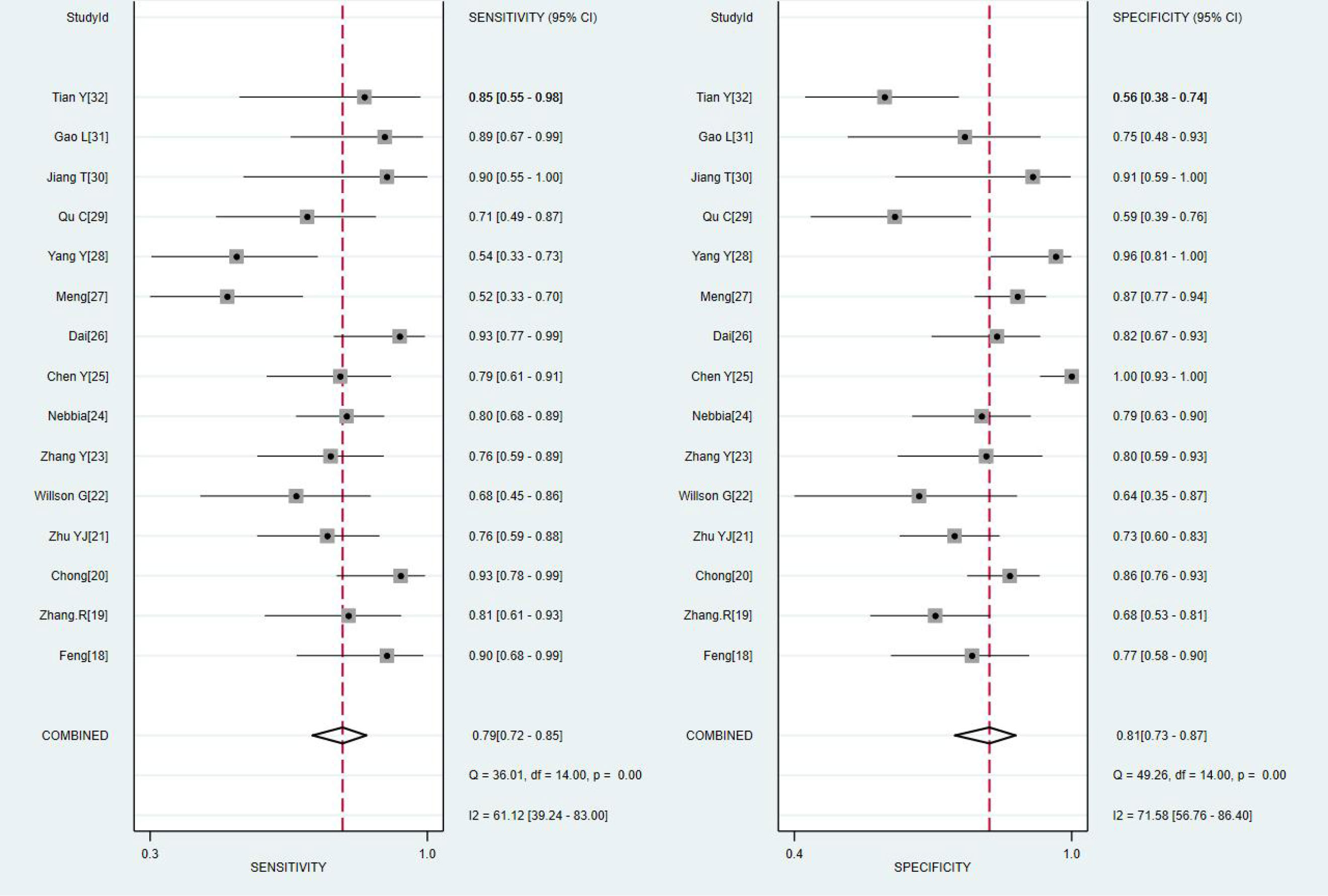
Figure 3 Coupled forest plots of the sensitivity and specificity of radiomics-based microvascular invasion (MRI) for predicting the MVI of hepatocellular carcinoma (HCC).
Exploration of heterogeneity
Heterogeneity was tested using Cochran-Q and I2. In Figure 3, the P-value of the Cochran-Q test was 0.00 (P < 0.05), and I2 was 61.12% in pooled sensitivity. Additionally, the P-value of the Cochran-Q test was 0.00 (P < 0.05), and I2 was 71.58% in pooled specificity. These results indicated that there was significant heterogeneity in pooled sensitivity and specificity among the included studies.
The result of sensitivity analysis showed that the bivariate model was moderately robust in goodness-of-fit and bivariate normality analyses (Supplemental Figure S2A, B). Influence analysis and outlier detection identified two outliers (Supplemental Figure S2C, D). After we excluded these outliers, the overall results did not change significantly, which suggested that the results of this study were statistically reliable.
Subgroup analysis was performed by comparing included studies with different variables. Six studies using HSCM had equivalent sensitivity (0.737 vs. 0.729) and specificity (0.816 vs. 0.820) compared to nine studies using the other. There were 11 studies with the LASSO method that had high sensitivity (0.775 vs. 0.620) and high specificity (0.842 vs. 0.765) than other methods. There were 11 studies using the investigated area of VOI that had equivalent sensitivity (0.731 vs. 0.730) and low specificity (0.814 vs. 0.844) than those studies with ROI. The imaging-to-surgery interval of 15 days had higher sensitivity (0.823 vs. 0.682) and slightly low specificity (0.790 vs. 0.837) than the others. The details of the subgroup analysis are shown in Table 4 and Figures 5A–D.
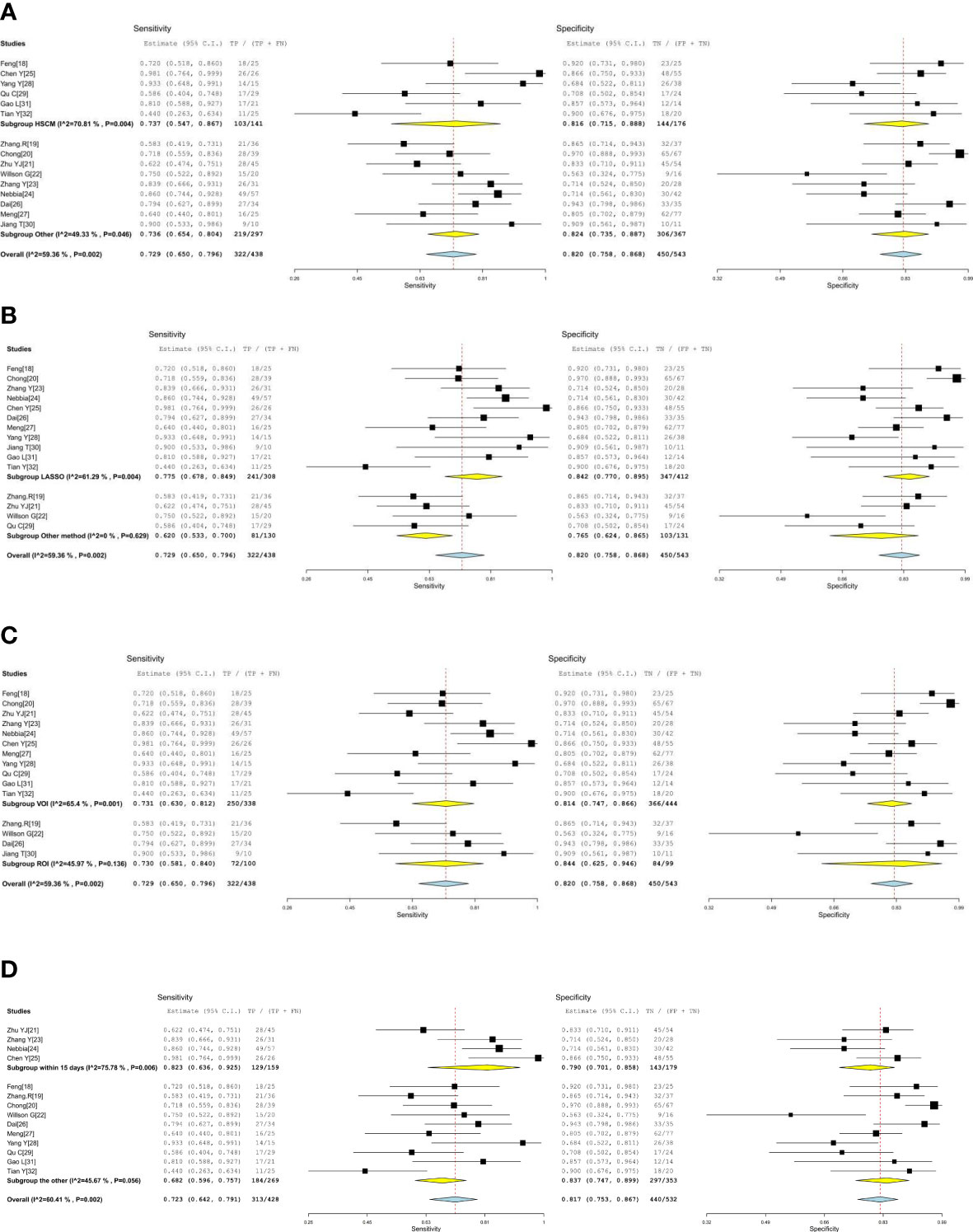
Figure 5 (A, B) The forest plots of subgroup analysis. (C, D) The forest plots of subgroup analysis.
Evaluation of clinical utility
The clinical utility of radiomics-based MRI was evaluated by using the likelihood ratio to simulate a Fagan nomogram. The results are shown in Figure 6. With a 20% pretest probability of MVI, the posttest probabilities of MVI and given positive and negative results of radiomics-based MRI are 51% and 6%, respectively. The Fagan nomogram revealed that the posttest probability increased by 31% in positive pretest patients but decreased by 14% in patients with a negative pretest, indicating that radiomics based-MRI was useful in clinical practice.
Discussion
MVI is defined as the presence of cancer cells in the portal vein, hepatic vein, or a large capsular vessel of the surrounding hepatic tissue lined by the endothelium, which is visible on microscopy (12). MVI is recognized as the strongest independent predictor of the early recurrence and poor prognosis of HCC (8–10). Previous studies found that some conventional imaging features, such as the tumor margin, size, number, capsule, shape, apparent diffusion coefficient values, and enhancement pattern, may contribute to the diagnosis of MVI before surgery (33). However, imaging features have some limitations, such as the fact that the reviews of medical images rely on subjective experience. The quantitative radiomics features can reflect the microscopic pathological changes of HCC by extracting features from the overall level of the tumor on the basis of conventional imaging images and evaluating the internal heterogeneity of the tumor (34, 35). Several previous similar studies have demonstrated that radiomics has high accuracy in evaluating the MVI in HCC; however, all of these studies analyzed CT-, MRI-, and ultrasound-based radiomics (13–15). This meta-analysis demonstrates that radiomics-based MRI has high diagnostic performance for predicting the MVI of HCC and can be used as a reliable and quantitative method for the non-invasive diagnosis of MVI in clinical practice. MRI can provide better soft-tissue resolution, multiparameters, and more stable features for assessing tumor heterogeneity.
However, obvious heterogeneity between included studies was noted. HSCM gadoxetate disodium was proven effective to assess the presence of MVI. The study demonstrated that the specificity of the hepatobiliary phase of gadolinium ethoxybenzyl diethylenetriamine pentaacetic acid Gd-EOB-DTPA-enhanced MRI combined with tumor margins and low signal intensity around the tumor to predict MVI is as high as 92.5% (36), but the contrast agent is expensive and not widely used in clinical practice. Subgroup analysis found that different contrast media (HSCM and others), the investigated area, and the method for selection were not the factors of significant heterogeneity. Furthermore, different imaging-to-surgery interval times have different. Therefore, the procedure and method should be standardized by conducting further research.
This study still has some limitations: (1) MRI scanning parameters (including the scanner machine model, field strength, and radiomics software) have not yet been unified; external datasets and different MRI scanning parameters are necessary for confirming the prediction value of the radiomics model. (2) Only English literatures of studies were included, which may result in applicable articles not being included in the review. (3) There was great heterogeneity in pooled estimates between the included studies. All of these factors may reduce the reliability of the results of this study. In the future, a large number of unified and standardized prospective studies are still needed to confirm the value of radiomics based-MRI in predicting the MVI of HCC.
Conclusion
In conclusion, this study demonstrated that radiomics based on MRI has high accuracy for predicting MVI in HCC, and it can be used as a reliable method to predict the presence of MVI in HCC before surgery in clinical applications.
Data availability statement
The raw data supporting the conclusions of this article will be made available by the authors, without undue reservation.
Author contributions
GL and WY have contributed equally to this work and share first Authorship. GL and WY completed manuscript together. GL collected validation group data. SL and MX processed the data and the statistics. ML gave the support of everything we need. All authors did literature researches. All authors contributed to the article and approved the submitted version.
Conflict of interest
Author ML was employed by West China-Frontier PharmaTech Co., Ltd.
The remaining authors declare that the research was conducted in the absence of any commercial or financial relationships that could be construed as a potential conflict of interest.
Publisher’s note
All claims expressed in this article are solely those of the authors and do not necessarily represent those of their affiliated organizations, or those of the publisher, the editors and the reviewers. Any product that may be evaluated in this article, or claim that may be made by its manufacturer, is not guaranteed or endorsed by the publisher.
Supplementary material
The Supplementary Material for this article can be found online at: https://www.frontiersin.org/articles/10.3389/fonc.2022.960944/full#supplementary-material
References
1. Bray F, Ferlay J, Soerjomataram I, Siegel RL, Torre LA, Jemal A. Global cancer statistics 2018: GLOBOCAN estimates of incidence and mortality worldwide for 36 cancers in 185 countries. CA Cancer J Clin (2018) 68:394–424. doi: 10.3322/caac.21492
2. Forner A, Reig M, Bruix J. Hepatocellular carcinoma. Lancet (2018) 391(10127):1301–14. doi: 10.1016/S0140-6736(18)30010-2
3. Bakr S, Echegaray S, Shah R, Kamaya A, Louie J, Napel S, et al. Noninvasive radiomics signature based on quantitative analysis of computed tomography images as a surrogate for microvascular invasion in hepatocellular carcinoma: a pilot study. J Med Imaging (Bellingham) (2017) 4:41303. doi: 10.1117/1.JMI.4.4.041303
4. Zheng Y, Cai Q, Peng L, Sun S, Wang S, Zhou J. Related factors of hepatocellular carcinoma recurrence associated with hyperglycemia after liver transplantation. Transplant Proc (2020) 53:177–92. doi: 10.1016/j.transproceed.2020.10.027
5. Mazzaferro V, Sposito C, Zhou J, Pinna AD, De Carlis L, Fan J, et al. Metroticket 2.0 model for analysis of competing risks of death after liver transplantation for hepatocellular carcinoma. Gastroenterology. (2018) 154(1):128–39. doi: 10.1053/j.gastro.2017.09.025
6. Kardashian A, Florman SS, Haydel B, Ruiz RM, Klintmalm GB, Lee DD, et al. Liver transplantation outcomes in a U.S. multicenter cohort of 789 patients with hepatocellular carcinoma presenting beyond Milan criteria. Hepatology. (2020) 72(6):2014–28. doi: 10.1002/hep.31210
7. Vogel A, Cervantes A, Chau I, Daniele B, Llovet JM, Meyer T, et al. Hepatocellular carcinoma: ESMO clinical practice guidelines for diagnosis, treatment and follow-up. Ann Oncol (2019) 30(5):871–3. doi: 10.1093/annonc/mdy308
8. Rodríguez-Perálvarez M, Luong TV, Andreana L, Meyer T, Dhillon AP, Burroughs AK. A systematic review of microvascular invasion in hepatocellular carcinoma: Diagnostic and prognostic variability. Ann Surg Oncol (2013) 20:325–39. doi: 10.1245/s10434-012-2513-1
9. Imamura H, Matsuyama Y, Tanaka E, Ohkubo T, Hasegawa K, Miyagawa S, et al. Risk factors contributing to early and late phase intrahepatic recurrence of hepatocellular carcinoma after hepatectomy. J Hepatol (2003) 38:200–7. doi: 10.1016/s0168-8278(02)00360-4
10. Du M, Chen L, Zhao J, Tian F, Zeng H, Tan Y, et al. Microvascular invasion (MVI) is a poorer prognostic predictor for small hepatocellular carcinoma. BMC Cancer (2014) 14:38. doi: 10.1186/1471-2407-14-38
11. Wakai T, Shirai Y, Sakata J, Kaneko K, Cruz PV, Akazawa K, et al. Anatomic resection independently improves long-term survival in patients with T1-T2 hepatocellular carcinoma. Ann Surg Oncol (2007) 14:1356–65. doi: 10.1245/s10434-006-9318-z
12. Lei Z, Li J, Wu D, Xia Y, Wang Q, Si A, et al. Nomogram for preoperative estimation of microvascular invasion risk in hepatitis b virus-related hepatocellular carcinoma within the Milan criteria. JAMA Surg (2016) 151(4):356–63. doi: 10.1001/jamasurg.2015.4257
13. Zhong X, Long H, Su L, Zheng R, Wang W, Duan Y, et al. Radiomics models for preoperative prediction of microvascular invasion in hepatocellular carcinoma: A systematic review and meta-analysis. Abdom Radiol (NY). (2022) 47(6):2071–88. doi: 10.1007/s00261-022-03496-3
14. Li L, Wu C, Huang Y, Chen J, Ye D, Su Z, et al. Radiomics for the preoperative evaluation of microvascular invasion in hepatocellular carcinoma: A meta-analysis. Front Oncol (2022) 12:831996. doi: 10.3389/fonc.2022.831996
15. Wang Q, Li C, Zhang J, Hu X, Fan Y, Ma K, et al. Radiomics models for predicting microvascular invasion in hepatocellular carcinoma: A systematic review and radiomics quality score assessment. Cancers (Basel). (2021) 13(22):5864. doi: 10.3390/cancers13225864
16. Whiting PF, Rutjes AW, Westwood ME, Mallett S, Deeks JJ, Reitsma JB, et al. QUADAS-2: A revised tool for the quality assessment of diagnostic accuracy studies. Ann Intern Med (2011) 155(8):529–36. doi: 10.7326/0003-4819-155-8-201110180-00009
17. Lambin P, Leijenaar RTH, Deist TM, Peerlings J, de Jong EEC, van Timmeren J, et al. Radiomics: the bridge between medical imaging and personalized medicine. Nat Rev Clin Oncol (2017) 14(12):749–62. doi: 10.1038/nrclinonc.2017.141
18. Feng ST, Jia Y, Liao B, Huang B, Zhou Q, Li X, et al. Preoperative prediction of microvascular invasion in hepatocellular cancer: a radiomics model using gd-EOB-DTPA-enhanced MRI. Eur Radiol (2019) 29(9):4648–59. doi: 10.1007/s00330-018-5935-8
19. Zhang R, Xu L, Wen X, Zhang J, Yang P, Zhang L, et al. A nomogram based on bi-regional radiomics features from multimodal magnetic resonance imaging for preoperative prediction of microvascular invasion in hepatocellular carcinoma. Quant Imaging Med Surg (2019) 9(9):1503–15. doi: 10.21037/qims.2019.09.07
20. Chong HH, Yang L, Sheng RF, Yu Y, Wu DJ, Rao XS, et al. Multi-scale and multi-parametric radiomics of gadoxetate disodium-enhanced MRI predicts microvascular invasion and outcome in patients with solitary hepatocellular carcinoma ≤ 5 cm. Eur Radiol (2021) 31(7):4824–38. doi: 10.1007/s00330-020-07601-2
21. Zhu YJ, Feng B, Wang S, Wang LM, Wu JF, Ma XH, et al. Model-based three-dimensional texture analysis of contrast-enhanced magnetic resonance imaging as a potential tool for preoperative prediction of microvascular invasion in hepatocellular carcinoma. Oncol Lett (2019) 18:720–32. doi: 10.3892/ol.2019.10378
22. Wilson GC, Cannella R, Fiorentini G, Shen C, Borhani A, Furlan A, et al. Texture analysis on preoperative contrast-enhanced magnetic resonance imaging identifies microvascular invasion in hepatocellular carcinoma. HPB (Oxford) (2020) 22:1622–30. doi: 10.1016/j.hpb.2020.03.001
23. Zhang Y, Shu Z, Ye Q, Chen J, Zhong J, Jiang H, et al. Preoperative prediction of microvascular invasion in hepatocellular carcinoma via multi-parametric MRI radiomics. Front Oncol (2021) 11:633596. doi: 10.3389/fonc.2021.633596
24. Nebbia G, Zhang Q, Arefan D, Zhao X, Wu S. Pre-operative microvascular invasion prediction using multi-parametric liver MRI radiomics. J Digit Imaging. (2020) 33(6):1376–86. doi: 10.1007/s10278-020-00353-x
25. Chen Y, Xia Y, Tolat PP, Long L, Jiang Z, Huang Z, et al. Comparison of conventional gadoxetate disodium-enhanced MRI features and radiomics signatures with machine learning for diagnosing microvascular invasion. AJR Am J Roentgenol (2021) 216:1–11. doi: 10.2214/AJR.20.23255
26. Dai H, Lu M, Huang B, Tang M, Pang T, Liao B, et al. Considerable effects of imaging sequences, feature extraction, feature selection, and classifiers on radiomics-based prediction of microvascular invasion in hepatocellular carcinoma using magnetic resonance imaging. Quant Imaging Med Surg (2021) 11(5):1836–53. doi: 10.21037/qims-20-218
27. Meng XP, Wang YC, Zhou JY, Yu Q, Lu CQ, Xia C, et al. Comparison of MRI and CT for the prediction of microvascular invasion in solitary hepatocellular carcinoma based on a non-radiomics and radiomics method: Which imaging modality is better? J Magn Reson Imaging (2021) 54(2):526–36. doi: 10.1002/jmri.27575
28. Yang Y, Fan W, Gu T, Yu L, Chen H, Lv Y, et al. Radiomic features of multi-ROI and multi-phase MRI for the prediction of microvascular invasion in solitary hepatocellular carcinoma. Front Oncol (2021) 11:756216. doi: 10.3389/fonc.2021.756216
29. Qu C, Wang Q, Li C, Xie Q, Cai P, Yan X, et al. A radiomics model based on gd-EOB-DTPA-Enhanced MRI for the prediction of microvascular invasion in solitary hepatocellular carcinoma ≤ 5 cm. Front Oncol (2022) 12:831795. doi: 10.3389/fonc.2022.831795
30. Jiang T, He S, Yang H, Dong Y, Yu T, Luo Y, et al. Multiparametric MRI-based radiomics for the prediction of microvascular invasion in hepatocellular carcinoma. Acta Radiol (2022) 30, 210336510. doi: 10.1177/02841851221080830
31. Gao L, Xiong M, Chen X, et al. Multi-region radiomic analysis based on multi-sequence MRI can preoperatively predict microvascular invasion in hepatocellular carcinoma. Front Oncol (2022) 12:818681. doi: 10.3389/fonc.2022.818681
32. Tian Y, Hua H, Peng Q, Zhang Z, Wang X, Han J, et al. Preoperative evaluation of gd-EOB-DTPA-Enhanced MRI radiomics-based nomogram in small solitary hepatocellular carcinoma (≤3 cm) with microvascular invasion: A two-center study. J Magn Reson Imaging (2022) 56(5):1459–1472. doi: 10.1002/jmri.28157
33. Suh YJ, Kim MJ, Choi JY, Park MS, Kim KW. Preoperative prediction of the microvascular invasion of hepatocellular carcinoma with diffusion-weighted imaging. Liver Transpl (2012) 18:1171–8. doi: 10.1002/lt.23502
34. Davnall F, Yip CS, Ljungqvist G, Selmi M, Ng F, Sanghera B, et al. Assessment of tumor heterogeneity: An emerging imaging tool for clinical practice? Insights Imaging (2012) 3:573–89. doi: 10.1007/s13244-012-0196-6
35. Lewis S, Hectors S, Taouli B. Radiomics of hepatocellular carcinoma. Abdominal Radiol (New York) (2021) 46:111–23. doi: 10.1007/s00261-019-02378-5
Keywords: hepatocellular carcinoma, microvascular invasion, MRI, radiomics, meta-analysis
Citation: Liang G, Yu W, Liu S, Zhang M, Xie M, Liu M and Liu W (2023) The diagnostic performance of radiomics-based MRI in predicting microvascular invasion in hepatocellular carcinoma: A meta-analysis. Front. Oncol. 12:960944. doi: 10.3389/fonc.2022.960944
Received: 03 June 2022; Accepted: 23 December 2022;
Published: 31 January 2023.
Edited by:
Jakub Nalepa, Silesian University of Technology, PolandReviewed by:
Llewellyn Padayachy, University of Pretoria, South AfricaRenguo Guan, Sun Yat-sen University Cancer Center (SYSUCC), China
Copyright © 2023 Liang, Yu, Liu, Zhang, Xie, Liu and Liu. This is an open-access article distributed under the terms of the Creative Commons Attribution License (CC BY). The use, distribution or reproduction in other forums is permitted, provided the original author(s) and the copyright owner(s) are credited and that the original publication in this journal is cited, in accordance with accepted academic practice. No use, distribution or reproduction is permitted which does not comply with these terms.
*Correspondence: Mingguo Xie, xmg6806@163.com