- 1Department of General Surgery, Second Affiliated Hospital Zhejiang University School of Medicine, Hangzhou, China
- 2Department of Surgical Ward 1, Ningbo Women and Children’s Hospital, Ningbo, China
- 3College of Information Engineering, Zhejiang University of Technology, Hangzhou, China
Background and Objectives: Pancreatic cancer (PC) is one of the deadliest cancers worldwide although substantial advancement has been made in its comprehensive treatment. The development of artificial intelligence (AI) technology has allowed its clinical applications to expand remarkably in recent years. Diverse methods and algorithms are employed by AI to extrapolate new data from clinical records to aid in the treatment of PC. In this review, we will summarize AI’s use in several aspects of PC diagnosis and therapy, as well as its limits and potential future research avenues.
Methods: We examine the most recent research on the use of AI in PC. The articles are categorized and examined according to the medical task of their algorithm. Two search engines, PubMed and Google Scholar, were used to screen the articles.
Results: Overall, 66 papers published in 2001 and after were selected. Of the four medical tasks (risk assessment, diagnosis, treatment, and prognosis prediction), diagnosis was the most frequently researched, and retrospective single-center studies were the most prevalent. We found that the different medical tasks and algorithms included in the reviewed studies caused the performance of their models to vary greatly. Deep learning algorithms, on the other hand, produced excellent results in all of the subdivisions studied.
Conclusions: AI is a promising tool for helping PC patients and may contribute to improved patient outcomes. The integration of humans and AI in clinical medicine is still in its infancy and requires the in-depth cooperation of multidisciplinary personnel.
1 Introduction
Pancreatic cancer (PC) is one of the deadliest malignancies worldwide. When all tumor stages are included, its 5-year survival rate is 3%–15%, which is the lowest among all cancer types (1, 2). Although pancreatic ductal adenocarcinoma is relatively rare, with an incidence of 8 to 12 cases per 100,000 per year and a 1.3% lifetime risk of developing the disease, the number of cancer deaths caused by PC ranks seventh overall (3). It ranks third in the United States, second only to colon cancer and lung cancer (1). Moreover, the incidence rate of PC has been increasing in recent years, and the mortality rate has also been rising over the past 10 years (1, 4). Therefore, we must actively explore feasible methods to improve the prognosis of patients. Wherever possible, this review focuses on pancreatic adenocarcinoma. However, it should be understood that when the term “pancreatic cancer” is used, the majority of instances are pancreatic ductal adenocarcinomas.
Artificial intelligence (AI) refers to any technique involving the use of a computer system to emulate human behavior (5). Computer vision, convolutional neural networks, and natural language processing have all seen tremendous advancements in data processing and big data technology, which makes AI become a hot spot and help innovate many fields in recent years, including the medical field. There have been some exciting achievements with AI in radiology, pathology, ophthalmology, and dermatology in the medical field. The combination of AI and modern medical treatments is where medical development is headed in the future.
Compared with the subjects mentioned above, the application of AI in the PC field is in its initial stages. However, the existing research results have shown that AI has the ability to optimize the PC diagnostic and therapeutic processes. Our team believes that combining AI with the diagnostic and treatment technology used today may help improve the prognosis of patients. The goals of this study are to summarize AI’s use in various aspects of the diagnosis and treatment of PC cases and also to discuss its limitations and possible future research directions.
2 Overview of AI
AI is a branch of computer science. It was formally put forward by scientists in 1956. To avoid the question of what “intelligence” is, Alan Turing, the father of AI, tends to test the thinking ability of machines only by comparing the behavior of machines and humans. Given the definition in terms of behavior, AI is a form of technology through which people attempt to use computers to imitate human behavior, especially thinking and decision-making processes (Figure 1).
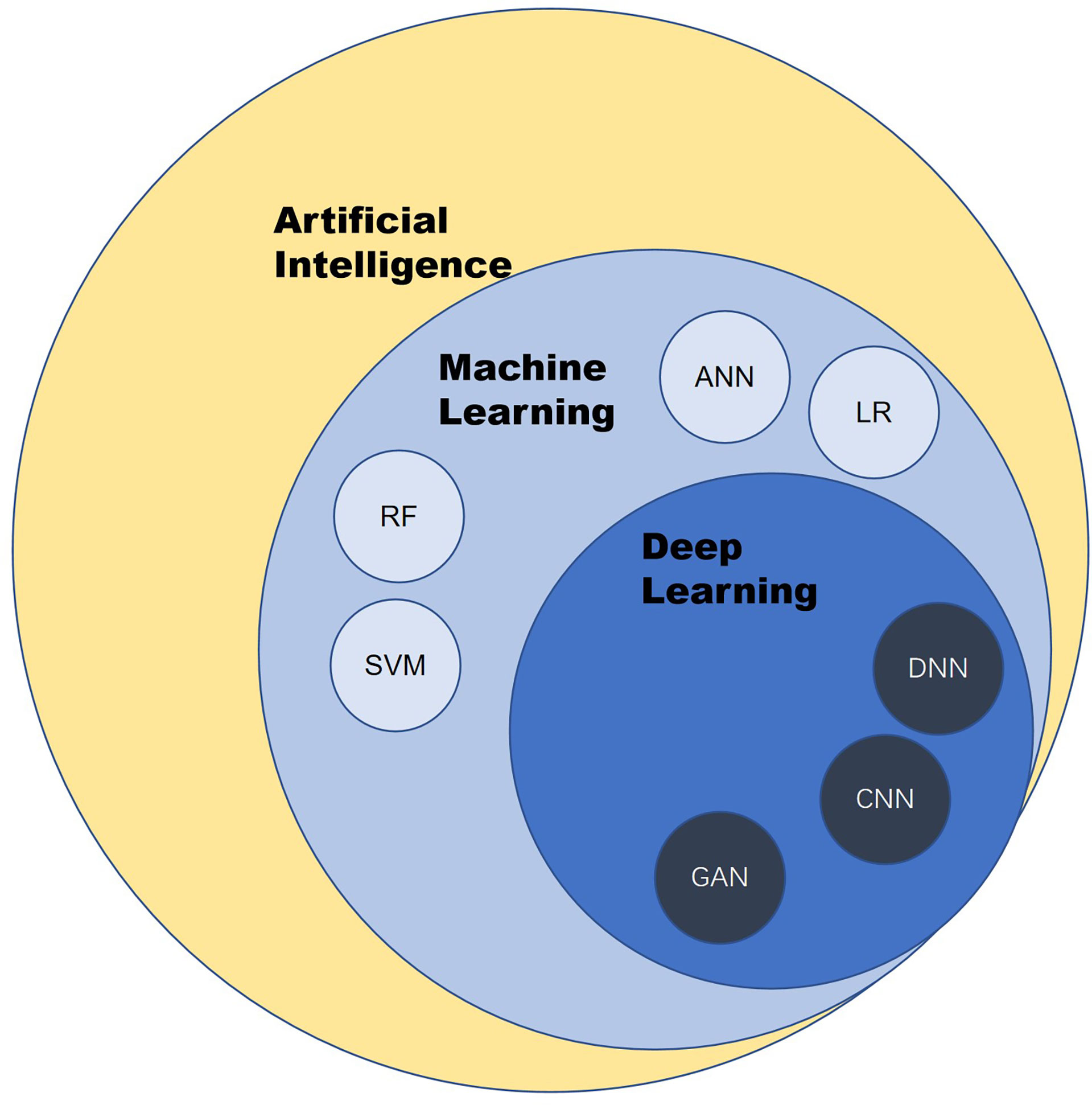
Figure 1 Overview of artificial intelligence. ANN, artificial neural network; CNN, convolutional neural network; LR, logistic regression; RF, random forest; SVM, support vector machine; DNN, deep neural network; GAN, generative adversarial network.
Machine learning (ML) and deep learning are the products of the development of AI. ML is a subset of AI techniques that attempt to apply statistics to data problems to discover new knowledge by generalizing from examples. Deep learning is a subset of ML that uses a collection of sophisticated algorithms known as neural networks to enable machines to analyze and learn in the same way that humans do, allowing them to identify text, pictures, audio, and other input (5).
An artificial neural network (ANN) is a computing model made up of interconnected units containing a high number of neurons (6). The most basic ANN consists of three layers (7) (Figure 2).
A feedforward neural network with a deep structure and convolution computation is known as a convolutional neural network (8). It has a remarkable capacity to process image information, making it useful for AI technologies.
In general, AI is an information processing technology. In clinical practice, some of the work performed by doctors, such as diagnosing diseases, making treatment plans, and judging prognosis, also involves processing and integrating existing information. Compared with the human brain, a computer has a larger storage space and faster processing speed. Thus, interesting questions have emerged regarding if medical treatment processes such as these can be carried out using AI, as well as if this technology can perform better than humans.
3 Methods
We did a thorough analysis of the available literature on AI applications for PC. We searched the online databases PubMed and Google Scholar for publications containing the terms artificial intelligence and pancreatic cancer. Only original articles published with the complete text supplied and written in English were selected using the filter. The abstracts of the publications were then scrutinized for subject relevance. We further looked through the reference lists of pertinent literature reviews to find extra relevant papers.
4 Results
According to medical tasks addressed in the research, papers can be grouped into four main categories: risk assessment, diagnosis, treatment, and prognosis prediction.
We will introduce the characteristics of each model and their performance one by one in the following sections. The performance of the models below is generally measured by accuracy, sensitivity, and specificity values and the area under the receiver operating characteristic curve (AUC).
Because each study is conducted in different settings, it is not advisable to compare the performance measurements provided in each study. We highly advise the readers to read each article in order to reach their own judgments.
Tables 1 and 2 describe some of the technical terminologies that will be mentioned below.
4.1 Predicting PC through risk factors
There are two types of risk factors for PC: modifiable and non-modifiable risk factors. Smoking, drinking, a history of chronic pancreatitis, dietary variables, and a history of certain infections such as hepatitis B, hepatitis C, and Helicobacter pylori are among the modifiable risk factor (9). The latter includes age, gender, ethnicity, blood group, gut bacteria, family history, and genetic susceptibility (9). For these numerous risk factors, there is no standard scale to determine the risk of PC in an individual. This causes clinicians not able to analyze all risk factors together, leading to wasted information and delayed diagnosis.
Prediagnosis symptoms include new-onset diabetes (10, 11), weight loss (12), jaundice, upper abdominal pain, etc., and these often appear a few months to a few years before PC is diagnosed (13). However, because of the non-specificity of these symptoms, doctors are often unable to link them to PC (14, 15). This also leads to wasted information and delayed diagnosis.
One of the reasons for the high mortality rate of PC is its lagging diagnosis. The majority (80%–85%) of people with PC have locally progressed or distant metastasis when they are diagnosed, and only a few patients have tumors that can be surgically removed (15%–20%) (16). AI can help solve this diagnosis delay problem caused by the waste of information. After obtaining the information on the above risk factors from the electronic health records and establishing the corresponding algorithm, this information can be fully used as input for the algorithm. The algorithm then weighs each risk factor and exports the possibility of PC. Some AI algorithms, such as unsupervised ML (17), can even summarize risk factors from electronic health records on their own. Using these algorithms, high-risk groups can be identified and screened to reduce the diagnosis time.
AI has been used in the analysis of electronic health data of a PC patient in several research studies (18–24). The relevant literature can be found in Table 3. Most of these studies include AI that analyzes electronic health records to help identify PC high-risk groups months to several years earlier than patients who did not get the help of AI predictions.
4.2 Diagnosis of PC through imaging pictures
The most often utilized imaging procedures in PC diagnosis are computed tomography (CT), magnetic resonance imaging (MRI), and endoscopic ultrasonography (EUS). In a complete diagnostic process, the pancreas image is first obtained using corresponding instruments and then interpreted by radiologists, who then give the final diagnosis. The objectivity of this process will inevitably be affected by the participation of radiologists. Unlike machines, the performance of human brains generally varies, particularly in image recognition. Several circumstances, such as weariness, stress, or a lack of expertise, might cause a lesion to be missed or misdiagnosed. Applying AI can achieve the following objectives: 1) shorten the time spent on image interpretation and improve work efficiency, 2) reduce labor intensity for radiologists, 3) improve the accuracy of diagnosis, and 4) diagnose the disease in an earlier stage and improve patient prognosis.
Compared with research focusing on electronic health records, research on combining AI and imaging is more prevalent and includes more mature technology. In the following, the application of AI in EUS, CT, and MRI will be introduced.
4.2.1 EUS
EUS is substantially better than trans-abdominal ultrasonography (US), CT, or MRI for obtaining high-resolution pictures of the pancreas (25). However, the endoscopist’s expertise and technical proficiency have a significant role in the diagnostic performance of EUS, which affects the objectivity and stability of interpretation, and AI will assist in resolving this issue.
As shown in Table 4, some studies reported the application of AI for the analysis of PC EUS images (26–33). Although few studies have been done, it has been claimed that using ML and DL to image the pancreas with EUS can produce results that are on par with or better than those made by endoscopists.
Intraductal papillary mucinous neoplasms (IPMNs) are precursor lesions of PC (34). To discriminate between benign and malignant IPMNs, Kuwahara et al. (35) designed a convolutional neural network (CNN), and their model achieved an accuracy of 94.0%. In a similar study, Machicado et al. (36) designed two CNN algorithms to help with IPMN diagnosis and risk classification. Compared with the existing guidelines [the American Gastroenterological Association (AGA) and revised Fukuoka guidelines], both algorithms yielded higher performance for diagnosis.
Zhang et al. (37) explored assistance with interpreting real-time ultrasonograms to help doctors reduce the missed diagnosis rate. Two algorithms were developed: one to help locate the detector and one to help segment the pancreatic region. Using these algorithms, the accuracy of trainee station recognition increased from 67.2% to 78.4%.
The ROI of the above experiments was drawn manually, typically by senior imaging scholars, while some experiments utilized an automated drawing of the ROI. Iwasa et al. (38) evaluated the capability of deep learning for the automatic segmentation of pancreatic tumors on contrast-enhanced endoscopic ultrasound video images. Their algorithm achieved the median intersection over the union of all cases of 0.77.
4.2.2 CT
The most often utilized imaging modality for the first examination of suspicious PC is the CT (39). Up to 34 months before the diagnosis of PDAC, the initial signs of PC, such as pancreatic parenchyma inhomogeneity and loss of typical fatty marbling of the pancreas, have been documented on retrospective CT evaluation (40). These subtle changes are difficult to recognize with the naked eye, which further emphasizes the necessity to possibly implement AI.
There are two phases in using AI for picture analysis of a PC. The initial step is to use the abdominal CT picture to get the contour of the pancreas, a process referred to as segmentation. The second step is to analyze the region generated by segmentation. In the following studies, most of the focus is on the second step, while the first step is usually completed by experienced imaging experts using manual segmentation methods.
4.2.2.1 Research focusing on analyzing the ROI
A summary of the most recent works describing the combination of AI with CT images to diagnose PDAC can be found in Table 5 (41, 42, 44–46). The performance of these models in the table is satisfying.
Some experiments have also explored the possibility of using CT images to predict the malignant potential of IPMNs. Qiu et al. (47) employed a support vector machine (SVM) to discriminate different histopathological grades of PDAC. The SVM achieved an overall accuracy of 86%. Hanania et al. (48) conducted a texture analysis of pancreatic images of IPMN patients. Within the gray-level co-occurrence matrix (GLCM), they discovered 14 imaging biomarkers and established corresponding logistic regression models to predict histopathological grade within cyst contours. The best logistic regression yielded an AUC of 0.96. In another similar experiment, Permuth et al. (49) combined a plasma-based miRNA genomic classifier data with radiomic features, and their algorithm revealed an AUC = 0.92.
Due to its strong computing power, AI processes information very quickly. Liu et al. (46) employed a faster region-based convolution network model to accurately read CT images and diagnose PC. Their system was able to acquire medical reports in about 200 ms per picture, which is much less time than imaging professionals take for diagnosis, and the AUC was 0.9632.
4.2.2.2 Research focusing on dividing ROI
Segmentation of the pancreas is often not satisfactory because while comprising just a small proportion of CT pictures, this organ is typically very changeable in form, size, and placement.
The richer feature convolutional network (RCF) is an algorithm used for the automated segmentation of images, but its ability to segment the pancreas is poor. Fu et al. (50) extended the RCF and generated a novel pancreas segmentation network. Finally, their algorithm achieved a 36% Dice similarity coefficient (DSC) value in testing data. Zhou et al. (51) built a fixed-point model that shrank the input region using an anticipated segmentation mask. Their algorithm achieved 82.37% DSC.
Muscle atrophy and decreased muscle density 2 to 4 months after diagnosis were linked to a worse survival rate in individuals with advanced PC (52). However, manual measurements of body composition are too time-consuming. Hsu et al. (53) designed an ANN that was able to quantify the tissue components. The detector analysis took 1 ± 0.5 s and the DSC values for visceral fat, subcutaneous fat, and muscle were 0.80, 0.92, and 0.85, respectively. This algorithm can help doctors save a lot of time while maintaining considerable accuracy.
4.2.2.3 Research focusing on dividing and analyzing the ROI
Chu et al. (54) conducted an experiment that divided PC diagnosis into two steps. Firstly, the algorithm recognized the boundaries of all organs in the abdomen and then identified the PC tissue (54, 55). In the first step, the algorithm’s pancreas segmentation accuracy was 87.8% ± 3.1%. In the second step, the algorithm had 94.1% sensitivity and 98.5% specificity. Liu et al. (56) also proposed a two-stage architecture in which the pancreas was first segmented into a binary mask, then compressed into a shape vector, and anomaly classification was conducted. Finally, they achieved a specificity of 90.2% and a sensitivity of 80.2%. It even picked up on a few difficult instances that radiologists would normally overlook, which showed promise for clinical applications.
4.2.3 MRI
Compared with CT, there are still few studies using MRI images as input data.
Corral et al. (57) employed a deep learning protocol to classify IPMNs. According to the malignant degree of the lesion, IPMNs can be classified as healthy pancreas, low-grade IPMN, or high-grade IPMN with adenocarcinoma. In their algorithm, a whole pancreas image was used as the input, with the malignant degree of the pancreas being the output. Their algorithm’s sensitivity and specificity for detecting dysplasia were 92% and 52%, respectively. Their method has a sensitivity and specificity of 75% and 78%, respectively, for detecting high-grade dysplasia or malignancy. According to this study, deep learning may offer diagnostic accuracy comparable to, if not greater than, existing radiographic recommendations for identifying IPMNs.
Unsupervised learning is the process of solving pattern recognition problems using training samples with unknown categories. Because radiologists are needed to get annotations for the majority of medical imaging operations, obtaining labels to develop machine learning models is time-consuming and costly. Hussein et al. (17) explored an unsupervised learning algorithm. They proposed a new clustering algorithm and tested it for the categorization of IPMNs. The accuracy, sensitivity, and specificity to identify benign or malignant tissues were 58.04%, 58.61%, and 41.67% respectively. Although the performance of their algorithm was not satisfying, this is one of the earliest and largest studies of an IPMN classification computer-aided diagnostic system.
In general, it is difficult to obtain medical images. It leads to a small training set for developing an AI algorithm, negatively affecting its performance. A generative adversarial network (GAN) is an image augmentation technology that can generate high-quality synthetic images from existing ones (58). Gao et al. (59) employed this technique to process their training set and expanded the initial 10,293 patches intercepted from MRI imaging to 35,735. With the help of GAN, they optimized their network.
A three-dimensional (3D) image of a tumor provides vital information about the tumor phenotype and microenvironment. However, making reasonable use of 3D picture information is tough. A 3D neural network, on the one hand, necessitates a significant number of processing resources due to its multiple parameters and complex connections between these parameters. The information in two-dimensional (2D) slices, on the other hand, is insufficient to completely reflect the 3D properties of a tumor. Chen et al. (60) developed a method for the automatic prediction of TP53 mutations in PC. While converting 3D images to 2D images, their spiral transformation technology could reduce the computation for the 3D image but still utilize its information. Finally, their algorithm achieved an AUC of 0.74. Their methods for using 3D information with a small sample size and successful multimodal fusion are possible medical imaging analysis paradigms.
Some research has focused on the automatic segmentation of pancreatic MRI images. Zheng et al. (61) proposed a 2D deep learning-based method to segment such images. Based on the shadowed set theory, the suggested technique defined the uncertain regions of pancreatic MRI images. Finally, they achieved a DSC of 84.37%.
4.3 AI for PC treatment
Liu et al. (62) employed a method to help with pancreatic adaptive radiotherapy, which can enhance treatment accuracy and, as a result, reduce gastrointestinal toxicity. They developed this method for cone-beam CT to synthetic CT generation, and the synthetic CT pictures may be able to produce accurate dosage calculations that are equivalent to the planning CT images.
Patients with advanced PC may benefit from echoendoscopic celiac plexus neurolysis as treatment for cancer pain. The efficiency of this procedure is limited; it frequently necessitates repeating therapy, and the outcome of such therapy is not consistent (63, 64). Facciorusso et al. (65) built an ANN model to predict pain response in a patient who underwent repeat echoendoscopic celiac plexus neurolysis (rCPN). They classified the treatment response as effective or ineffective according to the change degree and duration of the visual simulation scale (VAS). Their algorithm achieved an AUC of 0.94. It meant that this algorithm can identify accurately patients likely to benefit from rCPN and exclude those who are not sensitive to the treatment.
Nasief et al. (66) developed a delta-radiomic process for early prediction of PC treatment response based on ML. They analyzed daily CTs recorded during standard CT-guided chemoradiation treatment for PC patients to derive delta-radiomic characteristics. They added a new feature to standard deviation difference termed normalized entropy (NESTD). This new feature can be utilized to enhance organ boundary recognition and offer a method for standardizing contour validation. The output of their model is good or bad response, and their best-performing prediction model achieved an AUC of 0.94.
4.4 AI for predicting PC prognosis
PC is among the most lethal cancers. Even if surgery is performed, the estimated survival time is quite limited (67, 68). Additionally, adverse reactions are commonly encountered with surgery, chemotherapy, and radiotherapy (69–71). Therefore, predicting the prognosis of patients according to existing information, measuring the risks and benefits brought by treatment, and then choosing an appropriate treatment scheme are of great importance for improving the health and wellbeing of people. For decision-making, many predictive evaluation methods or risk scores have been established, including perioperative mortality risk (72), postsurgery complications (73), and survival prediction (74, 75). However, the performance of these systems did not meet expectations, and some clinical data were obtained using invasive operations such as surgery. Additional reliable prognostic indicators are urgently needed. The powerful data processing ability of AI gives it the potential to solve these problems. There are studies describing the computer-based quantitative evaluation of tumor morphology on diagnostic imaging in various cancer types, and it is progressively showing promise in describing the underpinning of tumor biology (76–79).
CT texture analysis is a postprocessing technique that can assess attenuation values and tumor heterogeneity in a user-defined ROI on CT images. In patients with non-small cell lung cancer, esophageal cancer, and metastatic renal cell carcinoma, baseline and first posttherapy alterations in CT texture analysis parameters of tumors have been linked to survival (80–82). This technology is used in many of the studies introduced below.
4.4.1 Predicting patient outcomes after the operation
Mu et al. (83) developed a deep learning model based on preoperative CT to anticipate clinically relevant postoperative pancreatic fistula (CR-POPF) following pancreatoduodenectomy and investigate the biological foundations of their model. Within 1–2 min, the model could generate output (with CR-POPF or not) with exceptional performance (AUC of 0.90). In a similar study, Kambakamba et al. (84) and their best classifier “REPTree” achieved an AUC of 0.95.
Lee et al. (85) used AI approaches to analyze the recurrence of PC after surgery. They compared the random forest model with the Cox proportional hazards model. The random forest and Cox model’s C-index averages were 0.68 and 0.77, respectively, in this study. This is the first study to use AI and multicenter registry data to forecast disease-free survival following PC surgery. The results of this methodological investigation show that AI can be a useful decision-support system for patients undergoing PC surgery.
4.4.2 Survival time prediction
Chakraborty et al. (86) quantified the heterogeneity of PDAC in CT images using texture analysis to predict patient 2-year survival rates. The proposed features obtained an AUC of 0.90 using a customized feature selection approach and a naive Bayes classifier.
Tong et al. (87) established ANN models to predict the 8-month survival rates of PC patients with unresectable tumors using clinical factors. Their ANN model with the best result achieved an AUC of 0.92. In a similar study, Walczak et al. (88) developed an ANN model that predicts PC patients’ 7-month survival, and their algorithm achieved 91% sensitivity and 38% specificity.
Integrating mRNA profiling, DNA methylation, and corresponding clinical information together, Tang et al. (89) established CNN models to predict the 5-year survival rates of PC patients, and their best algorithm achieved an AUC of 0.937.
Yue et al. (90) stratified the risks of PC patients by performing a quantitative analysis of pre- and postradiotherapy positron emission tomography-computed tomography (PET-CT) images and determining the predictive usefulness of textural differences in predicting patients’ therapeutic response. Based on the multivariate analytic risk score, the patients were divided into two groups: a low-risk group with a longer mean OS (29.3 months) and a high-risk group with a shorter mean OS (17.7 months). With log-rank P = 0.001, the multivariate analysis resulted in substantial risk stratification. In a similar study, Cozzi et al. (91) appraised the ability of a radionics signature that was extracted from CT images to anticipate patient outcomes following stereotactic body radiation (SBRT). The patients were stratified into two groups based on the radiomics signature—a low-risk group with a longer mean OS (14.4 months) and a high-risk group with a shorter mean OS (9.0 months)—and their best model achieved an AUC of 0.73.
Smith et al. (92) offered a unique Bayesian statistical method and used it to predict real lymph node ratio statuses and OS in patients who underwent radical oncologic resection. The predictor variables were obtained from the NCI SEER cancer registry. The C-index for the predictive performance was 0.65, which showed that its accuracy was low. They also developed a web application with a point-and-click interface for entering patient baseline data and seeing the posterior estimated survival statistics, such as median survival time and survival rate, and the LNR from their model.
Kaissis et al. (93) developed a supervised ML algorithm to predict OS in patients with PC by employing diffusion-weighted imaging-derived radiomic features. For the prediction of OS, their algorithm has a sensitivity of 87%, specificity of 80%, and AUC of 90%.
4.4.3 Exploring the factors related to prognosis
We separated the subsequent experiments into three groups based on the treatment received by the test subjects: In the first group, all of the patients underwent surgery. In the second group, none of the patients underwent surgery but received radiotherapy and chemotherapy. In the third group, some patients underwent surgery and some underwent radiotherapy and chemotherapy.
4.4.3.1 The first group
Mucins (MUC) are important in pancreatic tumor development and invasion. Yokoyama et al. (94) built models based on the methylation state of three mucin genes (MUC1, MUC2, and MUC4), and they found that their model outperformed tumor size, lymph node metastasis, distant metastasis, and age in predicting OS and can be used to supplement the TNM staging system’s prognostic value.
Cassinotto et al. (95) assessed the effectiveness of quantitative imaging biomarkers for evaluating pathologic tumor aggressiveness and predicting disease-free survival (DFS) by employing CT texture analysis. They concluded that on CT scans tumors that are more hypoattenuating in the portal venous phase are more likely to be aggressive, with a higher tumor grade, more lymph node invasion, and a shorter DFS.
Attiyeh et al. (96) generated a survival prediction model for resected PDAC patients using preoperative serum cancer antigen 19-9 levels, CT texture features, and the Brennan score. Finally, the concordance index of their model was 0.73. In another similar study, Choi et al. (97) measured texture analysis parameters from T2-weighted images of patients. In their study, following the multivariate Cox analysis, only tumor size continued to be a significant predictor.
Yun et al. (98) conducted a texture analysis of preoperative contrast-enhanced CT imaging of patients undergoing curative resection. They discovered that weaker CT texture analysis scores are linked to a reduced chance of survival. In a similar study, Eilaghi et al. (99) found that longer OS is connected with less inverse difference normalized and greater dissimilarity. Kim et al. (100) analyzed the gray-level non-uniformity (GLN) values of their images, and they found that GLN values were correlated with recurrence-free survival.
Ciaravino et al. (101) assessed how useful CT texture analysis was for evaluating tissue changes in PC that had been shrunk and removed after chemotherapy. At least two CT scans were necessary for the patients in this investigation (one before chemotherapy and one after chemotherapy), and patients received surgical treatment after chemotherapy. They found that the only parameter that was statistically different between CT 1 and CT 2 was kurtosis. In a similar study, Kim et al. (102) found that higher subtracted entropy and lower subtracted GLCM entropy are predictors of a favorable outcome. These two studies showed that patients’ prognoses can be predicted by quantitative analysis of PC pictures before and after chemotherapy.
4.4.3.2 The second group
Sandrasegaran et al. (103) evaluated the efficacy of CT texture analysis (CTTA) in forecasting the prognosis of patients with unresectable PC. The mean value of positive pixels (MPP), kurtosis, entropy, and skewness were selected as the CTTA parameters. In a multivariate Cox proportional hazard analysis, MPP was the only CTTA parameter that showed significance. Additionally, Kaplan–Meier statistics suggested that patients with high MPP and high kurtosis values had worse prognoses. In a similar study, Cheng et al. (104) found that higher standard deviation (SD) values were closely linked to progression-free survival and OS, indicating higher intratumoral heterogeneity. This could help predict better survival outcomes in patients with unresectable PC.
Cui et al. (105) did a quantitative study of PET-CT scans for patients who had locally progressed PC and were receiving SBRT and generated a signature. After multivariate analysis, the suggested signature was shown to be the sole relevant prognostic indicator, scoring 0.66 on the C-index.
4.4.3.3 The third group
Hayward et al. (106) constructed predictive models for the clinical performance of PC patients using ML techniques and compared the results with those of the linear and logistic regression techniques. They concluded that for most target attributes, such as survival time, Bayesian techniques provided the best overall performance.
During the administration of chemoradiation treatment for PC, Chen et al. (107) looked into radiation-induced alterations in quantitative CT characteristics of malignancies and reported that patients with good responses tended to have different texture features (such as volume, skewness, and kurtosis) compared with those with poor tumor responses.
5 Discussion
5.1 Future perspectives
In the 21st century, computer science is progressing exponentially. This has brought great changes to many other fields, including the medical field. The combination of big data and AI is referred to by some as the fourth industrial revolution (108). Daily diagnosis and treatment activities produce a large variety of medical data, such as imaging, vital signs, laboratory examination results, and more. For physicians, it is difficult to manually integrate all data and conduct a comprehensive analysis. Such situations that involve processing a large amount of data are the strength and advantage of using AI.
For the role of AI in the future, one possibility is that this technology will serve as an assistant to physicians that can provide advice and assist with their final decision-making. This could be very helpful when physicians are fatigued and stressed, and may also contribute to medical education. A second possibility is that AI will fully substitute for a physician. The abovementioned studies demonstrate that AI has the potential to surpass human beings, and in this situation, doctors can be replaced. When AI can completely replace humans in this regard, these individuals may feel liberated to do other work. Moreover, as a kind of software, these technologies can be easily shared worldwide and bring advanced technologies to areas with underdeveloped medical technology.
5.2 Challenges
Although the future of AI looks promising, a series of problems need to be addressed to efficiently achieve these goals.
1) The total amount of data is relatively small. The incidence rate of PC is very low (109), which makes it difficult to collect cases. Furthermore, many existing clinical data are not labeled or annotated. As a result, most PDAC experiments are retrospective and single center and have small sample sizes, making them vulnerable to selection bias and recall bias. To address this problem, a multi-agency cooperative model must be established, and prospective, double-blind, multicenter studies can be conducted. This would allow training sets to be representative and improve the performance of AI. Various data augmentation algorithms, such as GAN, can also help solve such problems and increase the amount of the original data.
2) Existing data are not fully utilized. With CT images as an example, most existing studies select the ROI within the section with the largest tumor diameter, resulting in only a 2D section being analyzed. The tumor is a 3D structure, so a 2D section cannot fully represent it. This may be a contributing factor to why the existing models do not perform well. 3D structures are not utilized as input data because ROI selection is done completely manually. The selection of a 2D structure has taken some time (about 3 min per imaging) and would take even longer for a 3D structure. Additionally, 3D structures would provide a very large amount of data. Even a strong computer would need a considerable amount of time to process such data. Therefore, to solve this problem, we need to optimize the algorithm, improve computing power, and develop automatic segmentation of 3D ROIs. Moreover, the existing research often only analyzes imaging results and does not consider the additional data. In fact, we can combine imaging with clinical features (such as weight loss, jaundice, and upper abdominal pain) and laboratory examination (such as CA199) to form hybrid biomarkers to optimize the performance of the model.
3) There is no unified standard for each process involved with obtaining and processing data. For example, standards involved with contrast-enhanced CT images frequently differ, including the type, concentration, and injection speed of the contrast agent; the equipment for obtaining images; scanning parameters; the selection of final images; the format and pixels transformed into analyzable data; and the selection of ROIs. This variability in standards prevents meaningful comparisons between experimental results and makes cooperation among institutions quite difficult. Therefore, experts should establish a reasonable and unified standardized process, which would greatly accelerate the progress of AI in the diagnosis and treatment of PC.
4) The ethical issues involved with medical data also require attention. In most of the abovementioned studies, the data are typically anonymized and the informed consent step is omitted. This would not be an issue in small sample retrospective studies but could be if AI is widely used in clinical practice. AI is an evolving and iterative system that requires the addition of data from new patients in the clinic. While being diagnosed and treated by AI, new patients also expose their data to AI, and the developers of the technology can profit from it. This raises the questions of how benefits should be distributed and if new patients should receive a share.
The storage, sharing, and management of patient data also require urgent attention. Three different models, the centralized, federated, and hybrid models, have been established for data exchange, each with its own set of benefits and drawbacks (see references for details) (110–114). For instance, the biggest disadvantage of the centralized model is that storing information on a server can increase the danger of a security breach because the individual source no longer has control over the data.
5) AI output interpretability is not satisfactory and is referred to as the “black box” (115). The black box model is a system that does not reveal its internal mechanism. In machine learning, black box models describe models that cannot be understood by looking at parameters, such as deep neural networks. People can only directly observe data input and the resulting output from the model, and it is difficult for people to fully understand how the data are being processed. This makes it difficult for people to modify the internal structure of the model to improve the performance of the algorithm. It also makes it difficult for AI to gain the trust of professionals and patients. Some studies (116) employed new technologies, such as the visual analysis approach and eye tracker to increase the interpretability of the model, providing us with a good solution.
6) The process is not automated enough. People’s impression of AI is always linked to full automation, but at this stage, most experiments can only be defined as semiautomatic. Humans often participate in some part of the process. For example, most ROI selections in CT images are manually produced by senior imaging experts. This takes a considerable amount of time, which is contrary to one of the original intentions of using AI. Although some studies have already focused on the segmentation of abdominal organs (50, 51, 54, 55, 61, 117–121), the performance of these algorithms is far below the level of imaging experts. If complete automation is to be achieved, emphasis should be placed on researching accurate organ segmentation methods.
7) The functions of existing AI are too limited. Ideally, AI, like human beings, can interpret pancreatic images to judge the occupied space, the location and nature of this space, and whether surgery is possible. Combined with other clinical information, AI can judge the prognosis of patients. However, AI in the existing research often has only one function. In future studies, we can aim to combine multiple models for AI to be more comprehensive in the PC diagnostic and treatment processes.
5.3 Conclusions
AI has the potential to help PC patients and contribute to improved patient outcomes. However, the integration of AI and human intelligence in clinical medicine is still in its infancy. Research to date has reported that the performance of AI could be superior to the standard statistical methods or even humans. Yet, AI still has its limitations, including those that we discussed above. Solving these problems requires the in-depth cooperation of multidisciplinary personnel. In the future, these limitations will be individually addressed, and AI will become an indispensable clinical auxiliary tool.
Author contributions
All authors listed have made a substantial, direct, and intellectual contribution to the work and approved it for publication.
Funding
This work was supported by Zhejiang Province Bureau of Health (No. 2020366835) and Funds of Science Technology Department of Zhejiang Province (No. 2020C03074). We thank J. Iacona, Ph.D., from Liwen Bianji (Edanz) (www.liwenbianji.cn) for editing the English text of a draft of this manuscript.
Conflict of interest
The authors declare that the research was conducted in the absence of any commercial or financial relationships that could be construed as a potential conflict of interest.
Publisher’s note
All claims expressed in this article are solely those of the authors and do not necessarily represent those of their affiliated organizations, or those of the publisher, the editors and the reviewers. Any product that may be evaluated in this article, or claim that may be made by its manufacturer, is not guaranteed or endorsed by the publisher.
References
1. Siegel RL, Miller KD, Jemal A. Cancer statistics, 2020. CA Cancer J Clin (2020) 70(1):7–30. doi: 10.3322/caac.21590
2. Arnold M, Rutherford MJ, Bardot A, Ferlay J, Andersson TML, Myklebust TÅ, et al. Progress in cancer survival, mortality, and incidence in seven high-income countries 1995–2014 (ICBP SURVMARK-2): a population-based study. Lancet Oncol (2019) 20(11):1493–505. doi: 10.1016/S1470-2045(19)30456-5
3. Bray F, Ferlay J, Soerjomataram I, Siegel RL, Torre LA, Jemal A, et al. Global cancer statistics 2018: GLOBOCAN estimates of incidence and mortality worldwide for 36 cancers in 185 countries. CA Cancer J Clin (2018) 68(6):394–424. doi: 10.3322/caac.21492
4. Ward EM, Sherman RL, Henley SJ, Jemal A, Siegel DA, Feuer EJ, et al. Annual report to the nation on the status of cancer, featuring cancer in men and women age 20-49 years. J Natl Cancer Inst (2019) 111(12):1279–97. doi: 10.1093/jnci/djz106
6. Zhang Z. Artificial neural network. In: Multivariate time series analysis in climate and environmental research Springer International (2018). p. 1–35.
7. Shahid N, Rappon T, Berta W. Applications of artificial neural networks in health care organizational decision-making: A scoping review. PLoS One (2019) 14(2):e0212356. doi: 10.1371/journal.pone.0212356
8. Gu J, Wang Z, Kuen J, Ma L, Shahroudy A, Shuai B, et al. Recent advances in convolutional neural networks. Pattern Recognition (2018) 77:354–77. doi: 10.1016/j.patcog.2017.10.013
9. Midha S, Chawla S, Garg PK. Modifiable and non-modifiable risk factors for pancreatic cancer: A review. Cancer Lett (2016) 381(1):269–77. doi: 10.1016/j.canlet.2016.07.022
10. Huang BZ, Pandol SJ, Jeon CY, Chari ST, Sugar CA, Chao CR, et al. New-onset diabetes, longitudinal trends in metabolic markers, and risk of pancreatic cancer in a heterogeneous population. Clin Gastroenterol Hepatol (2020) 18(8):1812–1821.e7. doi: 10.1016/j.cgh.2019.11.043
11. Pannala R, Basu A, Petersen GM, Chari ST. New-onset diabetes: a potential clue to the early diagnosis of pancreatic cancer. Lancet Oncol (2009) 10(1):88–95. doi: 10.1016/S1470-2045(08)70337-1
12. Hart PA, Kamada P, Rabe KG, Srinivasan S, Basu A, Aggarwal G, et al. Weight loss precedes cancer-specific symptoms in pancreatic cancer-associated diabetes mellitus. Pancreas (2011) 40(5):768–72. doi: 10.1097/MPA.0b013e318220816a
13. Paparrizos J, White RW, Horvitz E. Screening for pancreatic adenocarcinoma using signals from web search logs: Feasibility study and results. J Oncol Pract (2016) 12(8):737–44. doi: 10.1200/JOP.2015.010504
14. Macdonald S, Macleod U, Campbell NC, Weller D, Mitchell E. Systematic review of factors influencing patient and practitioner delay in diagnosis of upper gastrointestinal cancer. Br J Cancer (2006) 94(9):1272–80. doi: 10.1038/sj.bjc.6603089
15. Walter FM, Mills K, Mendonça SC, Abel GA, Basu B, Carroll N, et al. Symptoms and patient factors associated with diagnostic intervals for pancreatic cancer (SYMPTOM pancreatic study): a prospective cohort study. Lancet Gastroenterol Hepatol (2016) 1(4):298–306. doi: 10.1016/S2468-1253(16)30079-6
16. Singhi AD, Koay EJ, Chari ST, Maitra A. Early detection of pancreatic cancer: Opportunities and challenges. Gastroenterology (2019) 156(7):2024–40. doi: 10.1053/j.gastro.2019.01.259
17. Hussein S, Kandel P, Bolan CW, Wallace MB, Bagci U. Lung and pancreatic tumor characterization in the deep learning era: Novel supervised and unsupervised learning approaches. IEEE Trans Med Imaging (2019) 38(8):1777–87. doi: 10.1109/TMI.2019.2894349
18. Xiaodong Li, Gao P, Huang CJ, Hao S, Ling XB, Han Y, et al. A deep-learning based prediction of pancreatic adenocarcinoma with electronic health records from the state of Maine.World academy of science, engineering and technology. Int J Med Health Sci (2020) 14(11):358–65. Available at this link: https://publications.waset.org/10011557/a-deep-learning-based-prediction-of-pancreatic-adenocarcinoma-with-electronic-health-records-from-the-state-of-maine.
19. Appelbaum L, Cambronero JP, Stevens JP, Horng S, Pollick K, Silva G, et al. Development and validation of a pancreatic cancer risk model for the general population using electronic health records: An observational study. Eur J Cancer (2021) 143:19–30. doi: 10.1016/j.ejca.2020.10.019
20. Malhotra A, Rachet B, Bonaventure A, Pereira SP, Woods LM. Can we screen for pancreatic cancer? identifying a sub-population of patients at high risk of subsequent diagnosis using machine learning techniques applied to primary care data. PLoS One (2021) 16(6):e0251876. doi: 10.1371/journal.pone.0251876
21. Muhammad W, Hart GR, Nartowt B, Farrell JJ, Johung K, Liang Y, et al. Pancreatic cancer prediction through an artificial neural network. Front Artif Intell (2019) 2: 2. doi: 10.3389/frai.2019.00002
22. Placido D, Yuan B, Hjaltelin JX, Haue AD, Chmura PJ, Yua C, et al. Pancreatic cancer risk predicted from disease trajectories using deep learning. doi: 10.1101/2021.06.27.449937 (Accessed June 28, 2021)
23. Zhao D, Weng C. Combining PubMed knowledge and EHR data to develop a weighted bayesian network for pancreatic cancer prediction. J BioMed Inform (2011) 44(5):859–68. doi: 10.1016/j.jbi.2011.05.004
24. Hsieh MH, Sun LM, Lin CL, Hsieh MJ, Hsu CY, Kao CH. Development of a prediction model for pancreatic cancer in patients with type 2 diabetes using logistic regression and artificial neural network models. Cancer Manag Res (2018) 10:6317–24. doi: 10.2147/CMAR.S180791
25. Kitano M, Yoshida T, Itonaga M, Tamura T, Hatamaru K, Yamashita Y. Impact of endoscopic ultrasonography on diagnosis of pancreatic cancer. J Gastroenterol (2019) 54(1):19–32. doi: 10.1007/s00535-018-1519-2
26. Norton ID, Zheng Y, Wiersema MS, Greenleaf J, Clain JE, Dimagno EP. Neural network analysis of EUS images to differentiate between pancreatic malignancy and pancreatitis. Gastrointest Endosc (2001) 54(5):625–9. doi: 10.1067/mge.2001.118644
27. Tonozuka R, Itoi T, Nagata N, Kojima H, Sofuni A, Tsuchiya T, et al. Deep learning analysis for the detection of pancreatic cancer on endosonographic images: a pilot study. J Hepatobiliary Pancreat Sci (2021) 28(1):95–104. doi: 10.1002/jhbp.825
28. Saftoiu A, Vilmann P, Gorunescu F, Gheonea DI, Gorunescu M, Ciurea T, et al. Neural network analysis of dynamic sequences of EUS elastography used for the differential diagnosis of chronic pancreatitis and pancreatic cancer. Gastrointest Endosc (2008) 68(6):1086–94. doi: 10.1016/j.gie.2008.04.031
29. Saftoiu A, Vilmann P, Gorunescu F, Janssen J, Hocke M, Larsen M, et al. Efficacy of an artificial neural network-based approach to endoscopic ultrasound elastography in diagnosis of focal pancreatic masses. Clin Gastroenterol Hepatol (2012) 10(1):84–90.e1. doi: 10.1016/j.cgh.2011.09.014
30. Saftoiu A, Vilmann P, Dietrich CF, Iglesias-Garcia J, Hocke M, Seicean A, et al. Quantitative contrast-enhanced harmonic EUS in differential diagnosis of focal pancreatic masses (with videos). Gastrointest Endosc (2015) 82(1):59–69. doi: 10.1016/j.gie.2014.11.040
31. Ozkan M, Cakiroglu M, Kocaman O, Kurt M, Yilmaz B, Can G, et al. Age-based computer-aided diagnosis approach for pancreatic cancer on endoscopic ultrasound images. Endosc Ultrasound (2016) 5(2):101–7. doi: 10.4103/2303-9027.180473
32. Udristoiu AL, Cazacu IM, Gruionu LG, Gruionu G, Iacob AV, Burtea DE, et al. Real-time computer-aided diagnosis of focal pancreatic masses from endoscopic ultrasound imaging based on a hybrid convolutional and long short-term memory neural network model. PLoS One (2021) 16(6):e0251701. doi: 10.1371/journal.pone.0251701
33. Marya P, Powers PD, Chari ST, Gleeson FC, Leggett CL, Dayyeh A, et al. Utilisation of artificial intelligence for the development of an EUS-convolutional neural network model trained to enhance the diagnosis of autoimmune pancreatitis. Gut (2020) 0:1–10. doi: 10.1136/gutjnl-2020-322821
34. Brosens LA, Hackeng WM, Offerhaus GJ, Hruban RH, Wood LD. Pancreatic adenocarcinoma pathology: changing "landscape". J Gastrointest Oncol (2015) 6(4):358–74. doi: 10.3978/j.issn.2078-6891.2015.032
35. Kuwahara T, Hara K, Mizuno N, Okuno N, Matsumoto S, Obata M, et al. Usefulness of deep learning analysis for the diagnosis of malignancy in intraductal papillary mucinous neoplasms of the pancreas. Clin Transl Gastroenterol (2019) 10(5):1–8. doi: 10.14309/ctg.0000000000000045
36. Machicado JD, Chao WL, Carlyn DE, Pan TY, Poland S, Alexander VL, et al. High performance in risk stratification of intraductal papillary mucinous neoplasms by confocal laser endomicroscopy image analysis with convolutional neural networks (with video). Gastrointest Endosc (2021) 94(1):78–87.e2. doi: 10.1016/j.gie.2020.12.054
37. Zhang J, Zhu L, Yao L, Ding X, Chen D, Wu H, et al. Deep learning-based pancreas segmentation and station recognition system in EUS: development and validation of a useful training tool (with video). Gastrointest Endosc (2020) 92(4):874–885.e3. doi: 10.1016/j.gie.2020.04.071
38. Iwasa Y, Iwashita T, Takeuchi Y, Ichikawa H, Mita N, Uemura S, et al. Automatic segmentation of pancreatic tumors using deep learning on a video image of contrast-enhanced endoscopic ultrasound. J Clin Med (2021) 10(16):3589. doi: 10.3390/jcm10163589
39. Tempero MA, Malafa MP, Al-Hawary M, Behrman SW, Benson AB, Cardin DB, et al. Pancreatic adenocarcinoma, version 2.2021, NCCN clinical practice guidelines in oncology. J Natl Compr Canc Netw (2021) 19(4):439–57. doi: 10.6004/jnccn.2014.0106
40. Gonoi W, Hayashi TY, Okuma H, Akahane M, Nakai Y, Mizuno S, et al. Development of pancreatic cancer is predictable well in advance using contrast-enhanced CT: a case-cohort study. Eur Radiol (2017) 27(12):4941–50. doi: 10.1007/s00330-017-4895-8
41. Chu LC, Park S, Kawamoto S, Fouladi DF, Shayesteh S, Zinreich ES, et al. Utility of CT radiomics features in differentiation of pancreatic ductal adenocarcinoma from normal pancreatic tissue. AJR Am J Roentgenol (2019) 213(2):349–57. doi: 10.2214/AJR.18.20901
42. Park S, Chu LC, Hruban RH, Vogelstein B, Kinzler KW, Yuille AL, et al. Differentiating autoimmune pancreatitis from pancreatic ductal adenocarcinoma with CT radiomics features. Diagn Interv Imaging (2020) 101(9):555–64. doi: 10.1016/j.diii.2020.03.002
43. Liu K-L, Li S, Guo YT, Zhou YP, Zhang ZD, Li S, et al. Deep learning to distinguish pancreatic cancer tissue from non-cancerous pancreatic tissue: a retrospective study with cross-racial external validation. Lancet Digital Health (2020) 2(6):e303–13. doi: 10.1016/S2589-7500(20)30078-9
44. Ren S, Zhang J, Chen J, Cui W, Zhao R, Qiu W, et al. Evaluation of texture analysis for the differential diagnosis of mass-forming pancreatitis from pancreatic ductal adenocarcinoma on contrast-enhanced CT images. Front Oncol (2019) 9: 1171. doi: 10.3389/fonc.2019.01171
45. Qureshi G, Gaddam S, Wachsman AM, Wang L, Azab L, Asadpour V, et al. Predicting pancreatic ductal adenocarcinoma using artificial intelligence analysis of pre-diagnostic computed tomography imag. Cancer Biomarkers (2022) 33:211–7. doi: 10.3233/CBM-210273
46. Liu SL, Wu T, Chen P-T, Tsai YM, Roth H, Wu M-S, et al. Establishment and application of an artificial intelligence diagnosis system for pancreatic cancer with a faster region-based convolutional neural network. Chin Med J (Engl) (2019) 132(23):2795–803. doi: 10.1097/CM9.0000000000000544
47. Qiu W, Duan N, Chen X, Ren S, Zhang Y, Wang Z, et al. Pancreatic ductal adenocarcinoma: Machine learning-based quantitative computed tomography texture analysis for prediction of histopathological grade. Cancer Manag Res (2019) 11:9253–64. doi: 10.2147/CMAR.S218414
48. Alexander N, Hanania L, Bantis LE, Feng Z, Wang H, Tamm EP, Katz MH, et al. Quantitative imaging to evaluate malignant potential of IPMNs. Oncotarget (2016) 7(52):85776–84. doi: 10.18632/oncotarget.11769
49. Jennifer B, Permuth J, Choi J, Balarunathan Y, Kim J, Chen DT, Chen L, et al. Combining radiomic features with a miRNA classifier may improve prediction of malignant pathology for pancreatic intraductal papillary mucinous neoplasms. Oncotarget (2016) 7(52):85785–97. doi: 10.18632/oncotarget.11768
50. Fu M, Wu W, Hong X, Liu Q, Jiang J, Ou Y, et al. Hierarchical combinatorial deep learning architecture for pancreas segmentation of medical computed tomography cancer images. BMC Syst Biol (2018) 12(Suppl 4):56. doi: 10.1186/s12918-018-0572-z
51. Zhou Y, Xie L, Shen W, Wang Y, Fishman EK, Yuille AL. A Fixed-Point Model for Pancreas Segmentation in Abdominal CT Scans. MICCAI (2017), 693–701.
52. Babic A, Rosenthal MH, Bamlet WR, Takahashi N, Sugimoto M, Danai LV, et al. Postdiagnosis loss of skeletal muscle, but not adipose tissue, is associated with shorter survival of patients with advanced pancreatic cancer. Cancer Epidemiol Biomarkers Prev (2019) 28(12):2062–9. doi: 10.1158/1055-9965.EPI-19-0370
53. Hsu TH, Schawkat K, Berkowitz SJ, Wei JL, Makoyeva A, Legare K, et al. Artificial intelligence to assess body composition on routine abdominal CT scans and predict mortality in pancreatic cancer- a recipe for your local application. Eur J Radiol (2021) 142:109834. doi: 10.1016/j.ejrad.2021.109834
54. Wang Y, Zhou Y, Shen W, Park S, Fishman EK, Yuill AL. Abdominal multi-organ segmentation with organ-attention networks and statistical fusion. arXiv (2018) 55(2019):88–102. doi: 10.1016/j.media.2019.04.005
55. Zhu Z, Xia Y, Xie L, Fishman EK, Yuille AL. Multi-scale coarse-to-Fine segmentation for screening pancreatic ductal adenocarcinoma. arXiv (2019), 3–12. doi: 10.1007/978-3-030-32226-7_1
56. Liu F, Xie L, Xia Y, Fishman E, Yuille A. Joint shape representation and classification for detecting PDAC. arXiv (2019), 212–20. doi: 10.1007/978-3-030-32692-0_25
57. Corral JE, Hussein S, Kandel P, Bolan CW, Bagci U, Wallace MB. Deep learning to classify intraductal papillary mucinous neoplasms using magnetic resonance imaging. Pancreas (2019) 48(6):805–10. doi: 10.1097/MPA.0000000000001327
58. Radford A, Metz L, Chintala S. Unsupervised representation learning with deep convolutional generative adversarialnetworks. arXiv (2016). doi: 10.48550/arXiv.1511.06434
59. Gao X, Wang X. Performance of deep learning for differentiating pancreatic diseases on contrast-enhanced magnetic resonance imaging: A preliminary study. Diagn Interv Imaging (2020) 101(2):91–100. doi: 10.1016/j.diii.2019.07.002
60. Chen X, Lin X, Shen Q, Qian X. Combined spiral transformation and model-driven multi-modal deep learning scheme for automatic prediction of TP53 mutation in pancreatic cancer. IEEE Trans Med Imaging (2021) 40(2):735–47. doi: 10.1109/TMI.2020.3035789
61. Zheng H, Chen Y, Yue X, Ma C, Liu X, Yang P, et al. Deep pancreas segmentation with uncertain regions of shadowed sets. Magn Reson Imaging (2020) 68:45–52. doi: 10.1016/j.mri.2020.01.008
62. Liu Y, Lei Y, Wang T, Fu Y, Tang X, Curran WJ, et al. CBCT-based synthetic CT generation using deep-attention cycleGAN for pancreatic adaptive radiotherapy. Med Phys (2020) 47(6):2472–83. doi: 10.1002/mp.14121
63. Rykowski JJ, Hilgier M. Efficacy of neurolytic celiac plexus block in varyinglocations of pancreatic cancer: Influence on pain relief. Anesthesiology (2000) 92:347.e54. doi: 10.1097/00000542-200002000-00014
64. McGreevy K, Hurley RW, Erdek MA, Aner MM, Li S, Cohen SP. The effectiveness of repeat celiac plexus neurolysis for pancreatic cancer: a pilot study. Pain Pract (2013) 13(2):89–95. doi: 10.1111/j.1533-2500.2012.00557.x
65. Facciorusso A, Del Prete V, Antonino M, Buccino VR, Muscatiello N. Response to repeat echoendoscopic celiac plexus neurolysis in pancreatic cancer patients: A machine learning approach. Pancreatology (2019) 19(6):866–72. doi: 10.1016/j.pan.2019.07.038
66. Nasief H, Zheng C, Schott D, Hall W, Tsai S, Erickson B, et al. A machine learning based delta-radiomics process for early prediction of treatment response of pancreatic cancer. NPJ Precis Oncol (2019) 3:25. doi: 10.1038/s41698-019-0096-z
67. Beger HG, Rau B, Gansauge F, Poch B, Link KH. Treatment of pancreatic cancer: challenge of the facts. World J Surg (2003) 27(10):1075–84. doi: 10.1007/s00268-003-7165-7
68. Fesinmeyer MD, Austin MA, Li CI, Roos DAJ, Bowen DJ. Differences in survival by histologic type of pancreatic cancer. Cancer Epidemiol Biomarkers Prev (2005) 14(7):1766–73. doi: 10.1158/1055-9965.EPI-05-0120
69. McPhee JT, Hill JS, Whalen GF, Zayaruzny M, Litwin DE, Sullivan ME, et al. Perioperative mortality for pancreatectomy: a national perspective. Ann Surg (2007) 246(2):246–53. doi: 10.1097/01.sla.0000259993.17350.3a
70. Vollmer CM Jr., Sanchez N, Gondek S, McAuliffe J, Kent TS, et al. A root-cause analysis of mortality following major pancreatectomy. J Gastrointest Surg (2012) 16(1):89–102. discussion 102-3. doi: 10.1007/s11605-011-1753-x
71. Liu GF, Li GJ, Zhao H. Efficacy and toxicity of different chemotherapy regimens in the treatment of advanced or metastatic pancreatic cancer: A network meta-analysis. J Cell Biochem (2018) 119(1):511–23. doi: 10.1002/jcb.26210
72. Are C, Afuh C, Ravipati L, Sasson A, and Ullrich F, Smith L, et al. Preoperative nomogram to predict risk of perioperative mortality following pancreatic resections for malignancy. J Gastrointest Surg (2009) 13(12):2152–62. doi: 10.1007/s11605-009-1051-z
73. Braga M, Capretti G, Pecorelli N, Balzano G, Doglioni C, Ariotti R, et al. A prognostic score to predict major complications after pancreaticoduodenectomy. Ann Surg (2011) 254(5):702–7. discussion 707-8. doi: 10.1097/SLA.0b013e31823598fb
74. Dasari BV, Roberts KJ, Hodson J, Stevens L, Smith AM, Hubscher SG, et al. A model to predict survival following pancreaticoduodenectomy for malignancy based on tumour site, stage and lymph node ratio. HPB (Oxford) (2016) 18(4):332–8. doi: 10.1016/j.hpb.2015.11.008
75. Miura T, Hirano S, Nakamura T, Tanaka E, Shichinohe T, Tsuchikawa T, et al. A new preoperative prognostic scoring system to predict prognosis in patients with locally advanced pancreatic body cancer who undergo distal pancreatectomy with en bloc celiac axis resection: a retrospective cohort study. Surgery (2014) 155(3):457–67. doi: 10.1016/j.surg.2013.10.024
76. Zhang H, Graham CM, Elci O, Griswold ME, Zhang X, Khan MA, et al. Locally advanced squamous cell carcinoma of the head and neck: CT texture and histogram analysis allow independent prediction of overall survival in patients treated with induction chemotherapy. Radiology (2013) 269(3):801–09. doi: 10.1148/radiol.13130110
77. Aerts HJ, Velazquez ER, Leijenaar RT, Parmar C, Grossmann P, Carvalho S, et al. Decoding tumour phenotype by noninvasive imaging using a quantitative radiomics approach. Nat Commun (2014) 5:4006. doi: 10.1038/ncomms5006
78. Simpson AL, Adams LB, Allen PJ, D'Angelica MI, DeMatteo RP, Fong Y, et al. Texture analysis of preoperative CT images for prediction of postoperative hepatic insufficiency: a preliminary study. J Am Coll Surg (2015) 220(3):339–46. doi: 10.1016/j.jamcollsurg.2014.11.027
79. Win T, Miles KA, Janes SM, Ganeshan B, Shastry M, Endozo R, et al. Tumor heterogeneity and permeability as measured on the CT component of PET/CT predict survival in patients with non-small cell lung cancer. Clin Cancer Res (2013) 19(13):3591–9. doi: 10.1158/1078-0432.CCR-12-1307
80. Ganeshan B, Panayiotou E, Burnand K, Dizdarevic S, Miles K. Tumour heterogeneity in non-small cell lung carcinoma assessed by CT texture analysis: a potential marker of survival. Eur Radiol (2012) 22(4):796–802. doi: 10.1007/s00330-011-2319-8
81. Yip C, Landau D, Kozarski R, Ganeshan B, Thomas R, Michaelidou A, et al. Primary esophageal cancer: Heterogeneity as potential prognostic biomarker in patients treated with definitive chemotherapy and radiation therapy. Radiology (2014) 270(1):1–46. doi: 10.1148/radiol.13122869
82. Goh V, Ganeshan B, Nathan P, Juttla JK, Vinayan A, Miles KA. Assessment of response to tyrosine kinase inhibitors in metastatic renal cell cancer: CT texture as a predictive biomarker. Radiology (2011) 261(1):165–71. doi: 10.1148/radiol.11110264
83. Mu W, Liu C, Gao F, Qi Y, Lu H, Liu Z, et al. Prediction of clinically relevant pancreatico-enteric anastomotic fistulas after pancreatoduodenectomy using deep learning of preoperative computed tomography. Theranostics (2020) 10(21):9779–88. doi: 10.7150/thno.49671
84. Kambakamba P, Mannil M, Herrera PE, Muller PC, Kuemmerli C, Linecker M, et al. The potential of machine learning to predict postoperative pancreatic fistula based on preoperative, non-contrast-enhanced CT: A proof-of-principle study. Surgery (2020) 167(2):448–54. doi: 10.1016/j.surg.2019.09.019
85. Lee KS, Jang JY, Yu YD, Heo JS, Han HS, Yoon YS, et al. Usefulness of artificial intelligence for predicting recurrence following surgery for pancreatic cancer: Retrospective cohort study. Int J Surg (2021) 93:106050. doi: 10.1016/j.ijsu.2021.106050
86. Chakraborty J, Langdon-Embry L, Cunanan KM, Escalon JG, Allen PJ, Lowery MA, et al. Preliminary study of tumor heterogeneity in imaging predicts two year survival in pancreatic cancer patients. PLoS One (2017) 12(12):e0188022. doi: 10.1371/journal.pone.0188022
87. Tong Z, Liu Y, Ma H, Zhang J, Lin B, Bao X, et al. Development, validation and comparison of artificial neural network models and logistic regression models predicting survival of unresectable pancreatic cancer. Front Bioeng Biotechnol (2020) 8: 196. doi: 10.3389/fbioe.2020.00196
88. Walczak S, Velanovich V. An evaluation of artificial neural networks in predicting pancreatic cancer survival. J Gastrointest Surg (2017) 21(10):1606–12. doi: 10.1007/s11605-017-3518-7
89. Tang B, Chen Y, Wang Y, Nie J. A Wavelet-Based Learning Model Enhances Molecular Prognosis in Pancreatic Adenocarcinoma. BioMed Research International 2021. doi: 10.1155/2021/7865856
90. Yue Y, Osipov A, Fraass B, Sandler H, Zhang X, Nissen N, et al. Identifying prognostic intratumor heterogeneity using pre- and post-radiotherapy 18F-FDG PET images for pancreatic cancer patients. J Gastrointest Oncol (2017) 8(1):127–38. doi: 10.21037/jgo.2016.12.04
91. Cozzi L, Comito T, Fogliata A, Franzese C, Franceschini D, Bonifacio C, et al. Computed tomography based radiomic signature as predictive of survival and local control after stereotactic body radiation therapy in pancreatic carcinoma. PLoS One (2019) 14(1):e0210758. doi: 10.1371/journal.pone.0210758
92. Smith BJ, Mezhir JJ. An interactive Bayesian model for prediction of lymph node ratio and survival in pancreatic cancer patients. J Am Med Inform Assoc (2014) 21(e2):e203–11. doi: 10.1136/amiajnl-2013-002171
93. Kaissis G, Ziegelmayer S, Lohofer F, Algul H, Eiber M, Weichert W, et al. A machine learning model for the prediction of survival and tumor subtype in pancreatic ductal adenocarcinoma from preoperative diffusion-weighted imaging. Eur Radiol Exp (2019) 3(1):41. doi: 10.1186/s41747-019-0119-0
94. Yokoyama S, Hamada T, Higashi M, Matsuo K, Maemura K, Kurahara H, et al. Predicted prognosis of patients with pancreatic cancer by machine learning. Clin Cancer Res (2020) 26(10):2411–21. doi: 10.1158/1078-0432.CCR-19-1247
95. Cassinotto C, Chong J, Zogopoulos G, Reinhold C, Chiche L, Lafourcade JP, et al. Resectable pancreatic adenocarcinoma: Role of CT quantitative imaging biomarkers for predicting pathology and patient outcomes. Eur J Radiol (2017) 90:152–8. doi: 10.1016/j.ejrad.2017.02.033
96. Attiyeh MA, Chakraborty J, Doussot A, Langdon-Embry L, Mainarich S, Gonen M, et al. Survival prediction in pancreatic ductal adenocarcinoma by quantitative computed tomography image analysis. Ann Surg Oncol (2018) 25(4):1034–42. doi: 10.1245/s10434-017-6323-3
97. Choi MH, Lee YJ, Yoon SB, Choi JI, Jung SE, Rha SE. MRI Of pancreatic ductal adenocarcinoma: texture analysis of T2-weighted images for predicting long-term outcome. Abdom Radiol (NY) (2019) 44(1):122–30. doi: 10.1007/s00261-018-1681-2
98. Yun G, Kim YH, Lee YJ, Kim B, Hwang JH, Choi DJ, et al. Tumor heterogeneity of pancreas head cancer assessed by CT texture analysis: association with survival outcomes after curative resection. Sci Rep (2018) 8(1):7226. doi: 10.1038/s41598-018-25627-x
99. Eilaghi A, Baig S, Zhang Y, Zhang J, Karanicolas P, Gallinger S, et al. CT texture features are associated with overall survival in pancreatic ductal adenocarcinoma - a quantitative analysis. BMC Med Imaging (2017) 17(1):38. doi: 10.1186/s12880-017-0209-5
100. Kim HS, Kim YJ, Kim KG, Park JS. Preoperative CT texture features predict prognosis after curative resection in pancreatic cancer. Sci Rep (2019) 9(1):17389. doi: 10.1038/s41598-019-53831-w
101. Ciaravino V, Cardobi N, Capelli DERRP, Melisi D, Simionato F, et al. CT texture analysis of ductal adenocarcinoma downstaged after chemotherapy. Anticancer Res (2018) 38(8):4889–95. doi: 10.21873/anticanres.12803
102. Kim BR, Kim JH, Ahn SJ, Joo I, Choi SY, Park SJ. CT prediction of resectability and prognosis in patients with pancreatic ductal adenocarcinoma after neoadjuvant treatment using image findings and texture analysis. Eur Radiol (2019) 29(1):362–72. doi: 10.1007/s00330-018-5574-0
103. Sandrasegaran K, Lin Y, Asare-Sawiri M, Taiyini T, Tann M. CT texture analysis of pancreatic cancer. Eur Radiol (2019) 29(3):1067–73. doi: 10.1007/s00330-018-5662-1
104. Cheng SH, Cheng YJ, Jin ZY, Xue HD. Unresectable pancreatic ductal adenocarcinoma: Role of CT quantitative imaging biomarkers for predicting outcomes of patients treated with chemotherapy. Eur J Radiol (2019) 113:188–97. doi: 10.1016/j.ejrad.2019.02.009
105. Cui Y, Song J, Pollom E, Alagappan M, Shirato H, Chang DT, et al. Quantitative analysis of (18)F-fluorodeoxyglucose positron emission tomography identifies novel prognostic imaging biomarkers in locally advanced pancreatic cancer patients treated with stereotactic body radiation therapy. Int J Radiat Oncol Biol Phys (2016) 96(1):102–9. doi: 10.1016/j.ijrobp.2016.04.034
106. Hayward J, Alvarez SA, Ruiz C, Sullivan M, Tseng J, Whalen G. Machine learning of clinical performance in a pancreatic cancer database. Artif Intell Med (2010) 49(3):187–95. doi: 10.1016/j.artmed.2010.04.009
107. Chen X, Oshima K, Schott D, Wu H, Hall W, Song Y, et al. Assessment of treatment response during chemoradiation therapy for pancreatic cancer based on quantitative radiomic analysis of daily CTs: An exploratory study. PLoS One (2017) 12(6):e0178961. doi: 10.1371/journal.pone.0178961
108. Danuser Y, Kendzia MJ. Technological advances and the changing nature of work: Deriving a future skills set. Adv Appl Sociol (2019) 09(10):463–77. doi: 10.4236/aasoci.2019.910034
109. Siegel RL, Miller KD, Fuchs HE, Jema A. Cancer statistics, 2021. CA Cancer J Clin (2021) 71(1):7–33. doi: 10.3322/caac.21654
110. Young MR, Abrams N, Ghosh S, Rinaudo JAS, Marquez G, Srivastava S. Prediagnostic image data, artificial intelligence, and pancreatic cancer: A tell-tale sign to early detection. Pancreas (2020) 49(7):882–6. doi: 10.1097/MPA.0000000000001603
111. Deist TM, Dankers F, Ojha P, Marshall Scott M, Janssen T, Faivre-Finn C, et al. Distributed learning on 20 000+ lung cancer patients - the personal health train. Radiother Oncol (2020) 144:189–200. doi: 10.1016/j.radonc.2019.11.019
112. Guinney J, Saez-Rodriguez J. Alternative models for sharing confidential biomedical data. Nat Biotechnol (2018) 36(5):391–2. doi: 10.1038/nbt.4128
113. Chang K, Balachandar N, Lam C, Yi D, Brown J, Beers A, et al. Distributed deep learning networks among institutions for medical imaging. J Am Med Inform Assoc (2018) 25(8):945–54. doi: 10.1093/jamia/ocy017
114. Crimi A, Bakas S, Kuijf H, Keyvan F, Reyes M, Walsum TVBrainlesion: Glioma, Multiple Sclerosis, Stroke and Traumatic Brain Injuries. Springer Nature Switzerland (2019).
115. Price WN. Big data and black-box medical algorithms. Sci Transl Med (2018) 10:471. doi: 10.1126/scitranslmed.aao5333
116. Dmitriev K, Marino J, Baker K, Kaufman AE. Visual analytics of a computer-aided diagnosis system for pancreatic lesions. IEEE Trans Vis Comput Graph (2021) 27(3):2174–85. doi: 10.1109/TVCG.2019.2947037
117. Suman G, Panda A, Korfiatis P, Edwards ME, Garg S, Blezek DJ, et al. Development of a volumetric pancreas segmentation CT dataset for AI applications through trained technologists: a study during the COVID 19 containment phase. Abdom Radiol (NY) (2020) 45(12):4302–10. doi: 10.1007/s00261-020-02741-x
118. Park S, Chu LC, Fishman EK, Yuille AL, Vogelstein B, Kinzler KW, et al. Annotated normal CT data of the abdomen for deep learning: Challenges and strategies for implementation. Diagn Interv Imaging (2020) 101(1):35–44. doi: 10.1016/j.diii.2019.05.008
119. Liu Y, Lei Y, Fu Y, Wang T, Tang X, Jiang X, et al. CT-based multi-organ segmentation using a 3D self-attention U-net network for pancreatic radiotherapy. Med Phys (2020) 47(9):4316–24. doi: 10.1002/mp.14386
120. Boers TGW, Hu Y, Gibson E, Barratt DC, Bonmati E, Krdzalic J, et al. Interactive 3D U-net for the segmentation of the pancreas in computed tomography scans. Phys Med Biol (2020) 65(6):065002. doi: 10.1088/1361-6560/ab6f99
Keywords: pancreatic adenocarcinoma (PC), artificial intelligence (AI), machine learning (ML), artificial neural network (ANN), future perspectives
Citation: Chen X, Fu R, Shao Q, Chen Y, Ye Q, Li S, He X and Zhu J (2022) Application of artificial intelligence to pancreatic adenocarcinoma. Front. Oncol. 12:960056. doi: 10.3389/fonc.2022.960056
Received: 02 June 2022; Accepted: 24 June 2022;
Published: 22 July 2022.
Edited by:
Xiangsong Wu, Shanghai Jiao Tong University School of Medicine, ChinaReviewed by:
Hongcheng Sun, Shanghai General Hospital, ChinaYuhua Zhang, University of Chinese Academy of Sciences, China
Copyright © 2022 Chen, Fu, Shao, Chen, Ye, Li, He and Zhu. This is an open-access article distributed under the terms of the Creative Commons Attribution License (CC BY). The use, distribution or reproduction in other forums is permitted, provided the original author(s) and the copyright owner(s) are credited and that the original publication in this journal is cited, in accordance with accepted academic practice. No use, distribution or reproduction is permitted which does not comply with these terms.
*Correspondence: Jinhui Zhu, MjUxMjAxNkB6anUuZWR1LmNu