- 1Department of Oral and Maxillofacial Surgery, Guanghua School of Stomatology, Hospital of Stomatology, Sun Yat-sen University, Guangzhou, China
- 2Guangdong Provincial Key Laboratory of Stomatology, Sun Yat-sen University, Guangzhou, China
Background: The purpose of this study was to identify the prognostic value of cuproptosis and copper metabolism–related genes, to clarify their molecular and immunological characteristics, and to elucidate their benefits in head and neck squamous cell carcinoma (HNSCC).
Methods: The details of human cuproptosis and copper metabolism–related genes were searched and filtered from the msigdb database and the latest literature. To identify prognostic genes associated with cuproptosis and copper metabolism, we used least absolute shrinkage and selection operator regression, and this coefficient was used to set up a prognostic risk score model. HNSCC samples were divided into two groups according to the median risk. Afterwards, the function and immune characteristics of these genes in HNSCC were analyzed.
Results: The 14-gene signature was constructed to classify HNSCC patients into low-risk and high-risk groups according to the risk level. In the The Cancer Genome Atlas (TCGA) cohort, the overall survival (OS) rate of the high-risk group was lower than that of the low-risk group (P < 0.0001). The area under the curve of the time-dependent Receiver Operator Characteristic (ROC) curve assessed the good performance of the genetic signature in predicting OS and showed similar performance in the external validation cohort. Gene Ontology and Kyoto Encyclopedia of Genes and Genomes enrichment assays and Protein-Protein Interaction (PPI) protein networks have been used to explore signaling pathways and potential mechanisms that were markedly active in patients with HNSCC. Furthermore, the 14 cuproptosis and copper metabolism-related genes were significantly correlated with the immune microenvironment, suggesting that these genes may be linked with the immune regulation and development of HNSCC.
Conclusions: Our results emphasize the significance of cuproptosis and copper metabolism as a predictive biomarker for HNSCC, and its expression levels seem to be correlated with immune- related features; thus, they may be a possible biomarker for HNSCC prognosis.
Introduction
Head and neck squamous cell carcinoma (HNSCC) originates from the epithelial cells of the mouth, pharynx, and larynx, which is a kind of malignant tumor with high incidence (1, 2). Smoking, alcohol consumption, viral infection, environmental pollutants, and genetics are all risk factors for HNSCC (1). Since the beginning of this century, great progress has been made in the research of immunotherapy and other targeted drugs related to HNSCC, which have been applied in clinic. However, the five-year survival rate of HNSCC is only 50% (3, 4). In addition, due to the asymptomatic character of HNSCC and the lack of early detection, the risk of recurrence and metastasis of cancer patients is still high (5). Therefore, it is particularly critical to uncover new biomarkers for HNSCC patients and to better customize the prevention, screening, and treatment of HNSCC.
Copper is a trace element that has a significant impact on human health. It exists in organisms in the form of Cu (I) and Cu (II). Existing experimental results show that copper plays an important role in being an intracellular antioxidant, cellular respiration, nerve signal transduction, and the development of extracellular matrix (6). However, at high concentrations, copper can also cause the destruction of intracellular lipids, proteins, and nucleic acids (7). Unlike the known mechanisms of cell death, copper-dependent cell death depends on mitochondrial respiration, which occurs through the direct combination of copper with the fatty acylation component of the tricarboxylic acid (TCA) cycle (8). At present, some antitumor drugs can specifically induce cancer cell death, but how to overcome drug resistance is still an important problem to be solved. Platinum-based antitumor drugs (cisplatin and carboplatin) are commonly used to treat multiple types of solid tumors, including HNSCC. However, there is sufficient evidence to suggest that the long-term use of platinum-based antitumor agents in HNSCC patients can develop drug resistance (9–11). Cuproptosis and copper metabolism may be beneficial to the treatment of drug-resistant HNSCC. Copper homeostasis imbalance can be observed in the progression of HNSCC, and copper metabolism–related genes are related to the sensitivity of HNSCC to platinum compounds (9, 12). Copper can enhance the sensitivity of HNSCC patients to cisplatin by combining with copper ionophore disulfide (13). Therefore, it is very important to explore new forms of regulatory cell death, such as cuproptosis, and to find practical and effective prognostic markers for HNSCC. Thus, Tsvetkov et al. suggest that there may be a window that allows an increased concentration of copper in cells to be used to selectively kill cancer cells (14). The activation of the copper-induced cell death pathway may surpass the current drug resistance of chemotherapeutic drugs and open a new research field for new tumor therapies. At present, researchers have great interest in the relationship between cuproptosis and tumor, and its function in HNSCC needs to be further clarified (15–18). Therefore, the role of the entire cuproptosis and copper metabolism–related gene repertoire, in the prognosis of HNSCC, needs to be investigated.
In this study, HNSCC transcripts from several patient cohorts were downloaded from the public database, and 14 genetic signatures of cuproptosis and copper metabolism were identified by bioinformatics analysis. These markers related to cuproptosis and copper metabolism may become an effective index for predicting the prognosis of HNSCC patients
Method
Data collection
Through a comprehensive analysis of the source literature, we screened 139 genes related to cuproptosis and copper metabolism from the msigdb database and added recently reported genes to integrate them for the next step. The TCGA database was used to obtain patient clinicopathological data (https://portal.gdc.cancer.gov/). The data of 546 HNSCC samples were downloaded, including 502 cancer samples and 44 precancerous samples. All 44 adjacent normal tissue specimens belong to the normal group. The phenotype information, survival data (version: 12-06-2021), and RNA sequence data (version: 12-06-2021) of HNSCC patients, cervical cancer Cervical squamous cell carcinoma andendocervical adenocarcinoma (CESC) patients and esophageal cancer (ESCA) patients were acquired from the public database UCSC Xena (https://xenabrowser.net/datapages/). Those without RNA-seq data were excluded.
Construction and validation of the cuproptosis and copper metabolism–related gene prognostic index
Univariate Cox regression analysis (P<0.05) was applied to sift prognostic-related genes; 35 genes were identified, and 14 differentially related genes were identified by least absolute shrinkage and selection operator (LASSO) regression. Complete the above steps through the glmnet package. The coefficient (β) of LASSO regression corresponding to each gene was performed to construct the risk score for predicting the prognosis. Risk score = (coefficient Gene1 × expression of Gene1) + (coefficient Gene2 × expression of Gene2) +……+ (coefficient Gene n × expression Gene n), and the intermediate value of risk score is used to distinguish high-risk groups from low-risk groups. The prognostic power of the index was assessed by Kaplan-Meier (K-M) survival curves with log-rank tests. In order to test the independent prognostic accuracy of the index, we established 1-year, 3-year and 5-year time-dependent ROC charts, analyzed them with survival ROC software, and calculated the area under the curve (AUC).
Differentially expressed gene identification and bioinformatics analysis
The Wilcoxon function in R is used to calculate differentially expressed genes (DEGs) between clustering subgroups False discovery rate (FDR<0.05 and |log2FC|>1). According to all the data of DEGs, Gene Ontology (GO) and Kyoto Encyclopedia of Genes and Genomes (KEGG) analyses were studied. Then, the function enrichment analysis of DEGs protein–protein interaction network was fulfilled through the Search tool for the retrieval of interacting genes/proteins (STRING) database (https://string-db.org/), and the online calculation and prediction were carried out. On this basis, the visibility of PPI is analyzed by using Cytoscape. Principal component analysis (PCA) was used for realizing the dimensionality reduction and visualization of signature genes. In addition, the potential biological functions were studied by gene set enrichment analysis (GSEA 4.1.0), and P < 0.05 and FDR < 0.05 were considered statistically significant.
Tumor immune microenvironment analysis
In order to clarify the prognostic characteristics of the tumor immune microenvironment, the high-risk group and low-risk group were compared. We applied the CIBERSORTx website (https://cibersortx.stanford.edu/) to compare and reckon the abundance of 22 immune cells in the two populations. Platform xCell (xCell signature) (19) was used to calculate the proportion of immune cell infiltration in the tumor environment.
Construction of the predictive nomogram
Using the “rms” R package, a nomogram was established to predict the 1–5 year survival rate of HNSCC patients. The calibration curve is used to evaluate the prediction ability of nomogram. The closer to the 45° line, the better the prediction ability. Then, the independent prognostic factors were compared by decision curve analysis (DCA) to explore the difference of the combined nomogram in clinical benefit.
Genomic variance analysis
In order to identify the genomic variation, the distribution of all somatic mutant genes was compared. Somatic mutation data were downloaded from the Genomic data commons (GDC) database (https://portal.gdc.cancer.gov/). A comparison of tumor mutation burden (TMB) was conducted between the high-risk group and low-risk group.
Statistical analysis
All the statistical analyses of this study were performed using R software. The Wilcoxon test was used to selected DEGs of the two groups. Kaplan–Meier survival analysis and the Cox regression model were used for univariate survival analysis and multivariate survival analysis, respectively. Wilcoxon test was used to determine whether there was a difference in the proportion of somatic mutations. Bilateral P < 0.05 was considered significant.
Results
Construction the prognostic signature of cuproptosis and copper metabolism–related genes and validating its predictive performance
In this study, our bioinformatics analysis is based on the workflow shown in Figure 1. A total of 546 samples from the TCGA database were used; 44 normal samples, incomplete prognostic information, and OS less than 30 days were excluded; and 493 cancer samples were eventually included in the study. To establish a novel prognostic model, 35 genes related to prognosis were selected from 139 genes by univariate Cox analysis, and then, the appropriate genes related to cuproptosis and copper metabolism were selected by LASSO regression analysis (Figure 2A and Additional Figure 1). Finally, based on the optimal value of λ, a 14-gene signature was identified (Figures 2B, C). Figure 2D shows the K-M curve of each gene.
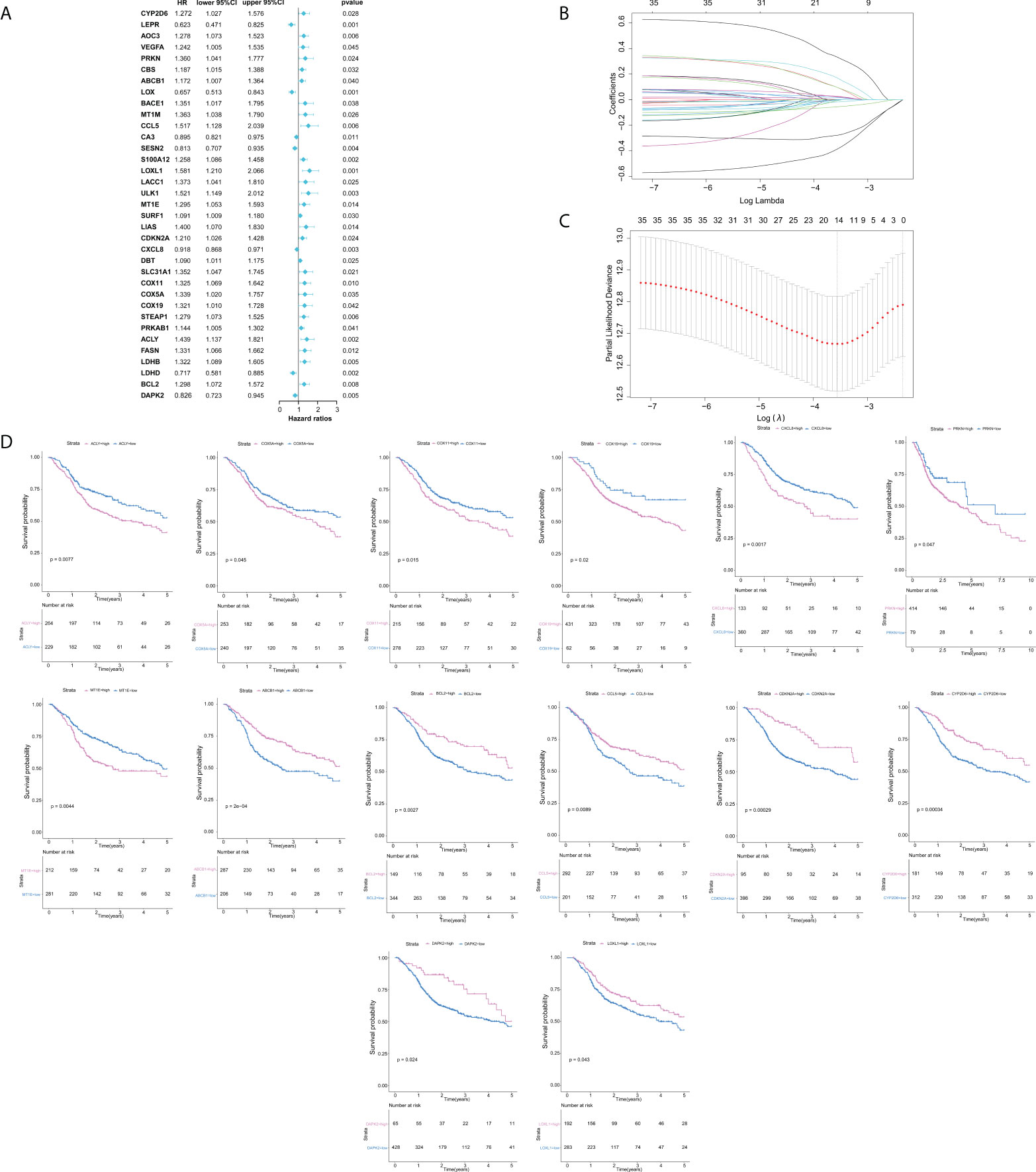
Figure 2 Construction of cuproptosis and copper metabolism–related gene prognostic signature. (A) Cuproptosis and copper metabolism–related genes of Cox analysis. (B, C) Least absolute shrinkage and selection operator analysis was performed to select the optimum genes to construct a 14-gene signature. (D) K-M curve of 14 genes.
As mentioned above, 14 gene-based prognostic signatures were used to compute the risk score for each sample (Figures 3A, B). The formula is as follows: risk score = (-0.3847 × expression of CYP2D6) + (0.1679 × expression of PRKN) + (-0.2781 × expression of ABCB1) + (-0.0177 × expression of CCL5) + (-0.0066 × expression of LOXL1) + (0.0252 × expression of MT1E) + (-0.0554 × expression of CDKN2A) + (0.1500 × expression of CXCL8) + (0.3308 × expression of COX11) + (0.0942 × expression of COX5A) + (0.0945 × expression of COX19) + (0.0222 × expression of ACLY) + (-0.0005 × expression of BCL2) + (-0.0047 × expression of DAPK2). All patients were grouped into a high-risk group (n=246) or a low-risk group (n=247) according to the median risk score. Kaplan–Meier survival analysis indicated that the OS of high-risk groups was markedly lower than that of low-risk groups (Kaplan–Meier survival analysis P < 0.0001, Figure 3D). The ROC curve showed that the risk score development in our present study had a good predictive value of 0.70, 0.69, and 0.63 for 1-year AUC, 3-year AUC, and 5-year AUC, respectively (Figure 3C). Therefore, according to the survival curve, we finally determined that 14 genes related to cuproptosis and copper metabolism were significantly correlated with prognosis: ACLY, COX5A, COX11, COX19, CXCL8, PRKN, and MTIE were negatively correlated with OS, while ABCB1, BCL2, CCL5, CDKN2A, CYP2D6, DAPK2, and LOXL1 were positively correlated with OS. Similarly, PCA confirmed that these 14 genes have implications for OS prediction (Additional Figure 1). In conclusion, the above results suggest that the model composed of cuproptosis and copper metabolism–related genes may be a credible and valuable indicator for predicting the prognosis of HNSCC.
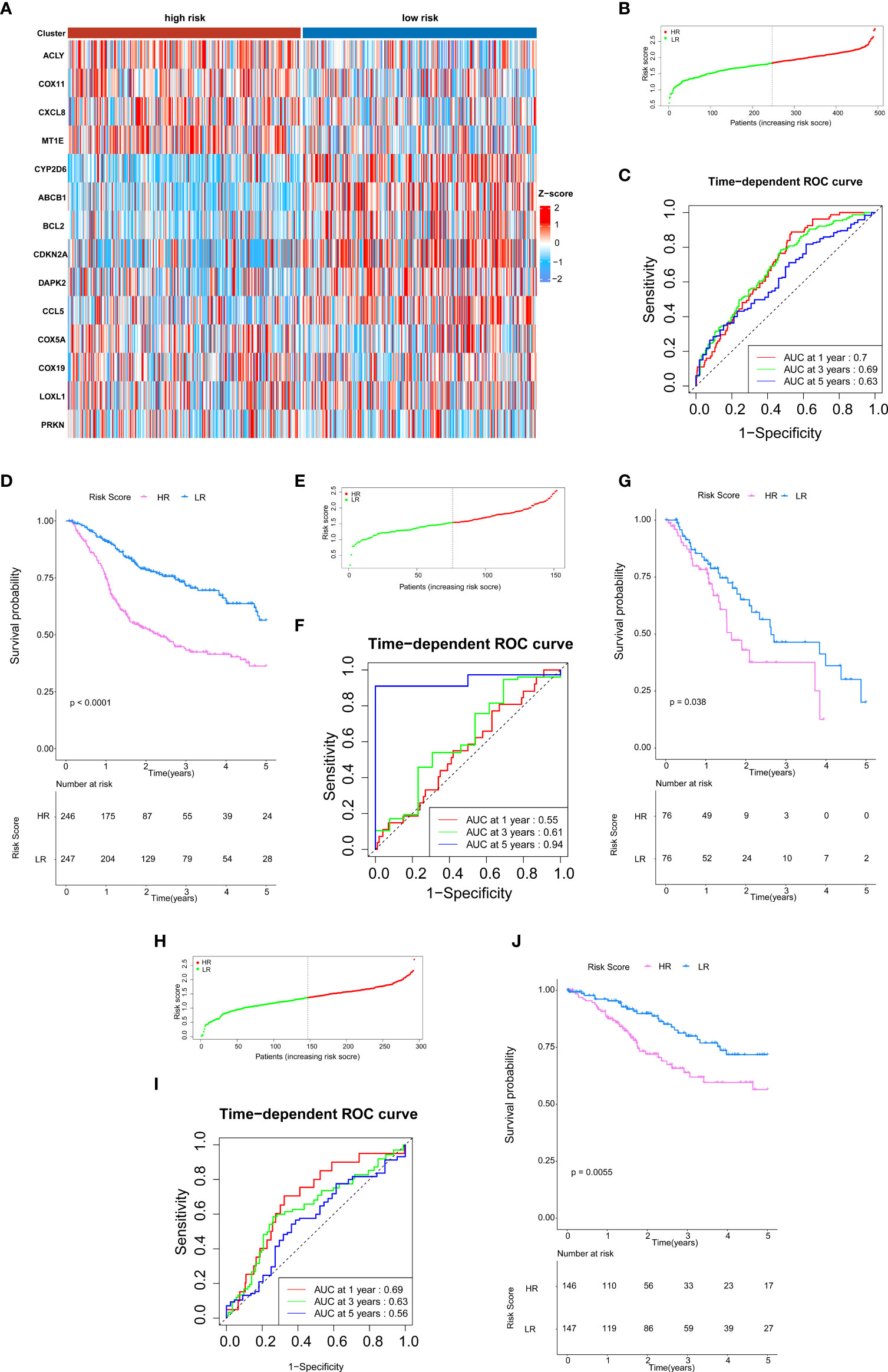
Figure 3 Validation of cuproptosis and copper metabolism–related gene prognostic signature. (A)Heat map representing the expression level of the prognostic genes based on the high-risk group and the low-risk group of head and neck squamous cell carcinoma (HNSCC) patients. (B, E, H) Association between the risk score and the distribution of high- (red) and low- (green) risk groups of HNSCC patients (B), esophageal cancer (ESCA) patients (E), and CESC patients (H). (C, F, I) The ROC curves for evaluating the prognostic value by the 14-gene signature in the TCGA cohort of HNSCC patients (C), ESCA patients (F), and CESC patients (I). (D, G, J) The overall survival (OS) rates of different groups of HNSCC patients (D), ESCA patients (G), and CESC patients (J).
We intended to select the available HNSCC sample cohort to verify the above results. However, the model genes were incomplete in any other HNSCC cohort of Gene expression omnibus (GEO) databases. Therefore, we selected other cohorts for validation. All three malignancies contain an epithelial origin (1, 20, 21). On one hand, ESCA and HNSCC have high homology; they have a similar metastatic behavior and have common internal resistance to conventional systemic therapy (22). On the other hand, esophagus is a high-risk site for HNSCC patients to develop secondary primary malignant tumors (23, 24). Based on these correlations, we selected the available cohorts of ESCA samples from the TCGA database for verification, including 152 patients with ESCA (Figures 3E–G). For HNSCC and CESC, studies have shown that their immune activation transcriptome has a certain correlation (25). Therefore, we also validated our model in the CESC cohort (Figures 3H–J). The results of this validation queue were consistent with the results we obtained before, which showed that it had excellent reliability and repeatability.
Differentially expressed gene identification and bioinformatics analysis
To further investigate the possible biological functions of 14 genes related to cuproptosis and copper metabolism, we used the “clusterProfiler” package for GO annotations and KEGG pathway enrichment analysis. Figure 2 lists the enriched GO terms and KEGG pathways. DEGs were significantly enriched in immunoglobulin production, the detection of chemicals, stimulus involved in sensory, olfactory pathways, T-cell receptor complexes, and plasma membrane signals (Figures 4A, B). The top three significantly enriched molecular function terms include olfactory receptor activity, antigen binding, and neurotransmitter receptor activity (Figure 4C). In addition, KEGG analysis revealed that the significant enrichment pathway of these DEGs was the olfactory transduction pathway (Figure 4D). The PPI network of DEGs was acquired through the application of the online STRING tool. The results showed that the CD19 molecule (CD19) was the most important gene (Figure 4E). The gene coding protein is a member of immunoglobulin gene superfamily. Its coding protein is cell surface protein, and its expression is limited to B-cell lymphocytes. Moreover, we used GSEA to explore the potential biological functions of 14 genes involved in cuproptosis and copper metabolism. The results of GSEA confirm these findings (Figure 4F).
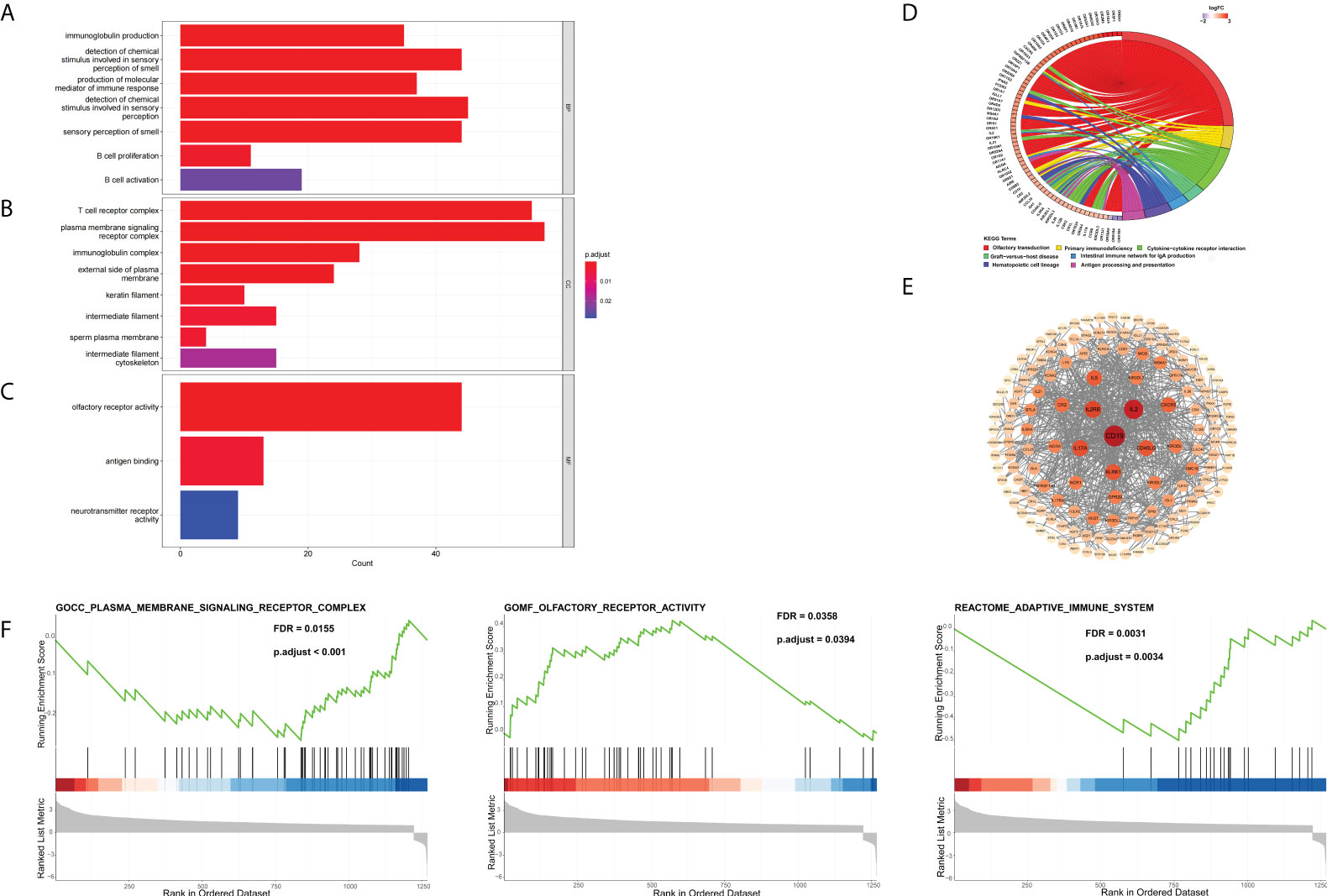
Figure 4 DEG Identification and bioinformatics analysis. (A–D) The enriched GO (A) BP, (B) CC, and (C) MF terms as well as (D) KEGG pathways. GO, gene ontology; KEGG, Kyoto Encyclopedia of Genes and Genomes; DEG, differentially expressed gene; BP, biological process; CC, cellular component; MF, molecular function. (E) The PPI network downloaded from the STRING database indicated the interactions among the candidate genes. (F) Gene set enrichment analysis.
Analysis of tumor immune microenvironment
Through DEG analysis, we found that these genes play a role in immune-related pathways. As a result, we explored the connection between the cuproptosis and copper metabolism–related signature and the tumor immune microenvironment. We used the CIBERSORTx algorithm and xCell to compare the distribution of immune cells in different groups. It was observed that M2 macrophages, naïve CD4 T cells, resting memory CD4 T cells, resting NK cells, activated mast cells, eosinophils, M0 macrophages, cancer cells, and lymph vessels were more abundant in the high-risk group, while Th17 cells, DC, naïve B cells, plasma cells, CD8 T cells, follicular helper T cells, activated NK cells, activated memory CD4 T cells, M1 macrophages, and resting mast cells were more abounding in the low-risk group (Figure 5A, Additional Figure 2). We also displayed the characteristics related to the immune landscape of the two groups (Figure 5B, Additional Figure 2). Immune-related functions such as human leukocyte antigen, immune checkpoint, and inflammation-related promotion between the two groups were usually more significant in low-risk populations (Figure 5C).
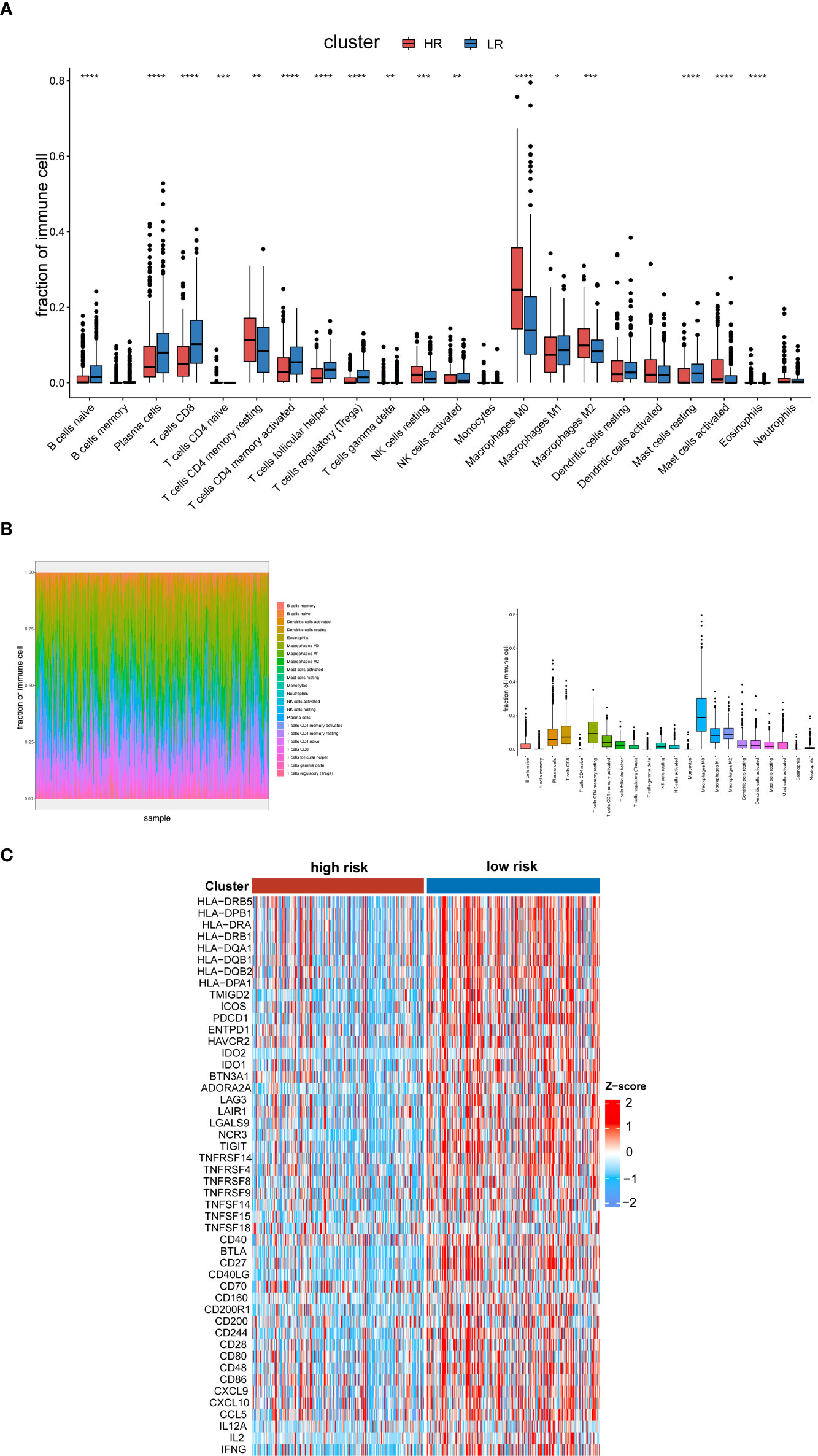
Figure 5 Analysis of the tumor immune microenvironment. (A) Analysis of the abundance of immune cells via the CIBERSORTx algorithm based on the TCGA cohort. (B) The characteristics related to the immune landscape of two groups via the CIBERSORTx algorithm. (C) Heat map of human leukocyte antigen, immune checkpoint, and inflammation-related promotion in the low- and high-risk groups in the TCGA databases. The p-values were shown as: *p < 0.05; **p < 0.01; ***p < 0.001. ****p < 0.0001.
Establishment and evaluation of the predictive nomogram
Multivariate Cox regression was used for determining whether the risk characteristics were independent prognostic factors (Figure 6A). We analyzed the heat map of the association between the prognostic characteristics of cuproptosis and copper metabolism related genes and clinicopathological manifestations, which can be used for the clinical management of HNSCC patients (Figure 6B). To further enhance the predictive ability, a predictive nomogram ground on the integration of the risk score and pathological stage was constructed in the TCGA cohort (Figure 6C). The calibration curve of the nomogram showed that the standard curves for predicting and observing 1-year, 3-year, and 5-year results in the cohort were fairly consistent (Figures 6D–F). In addition, we used DCA analysis to evaluate the predicted values of nomogram for the TCGA cohort 1, 3, and 5 years in clinical decision-making (Figures 6G–I).
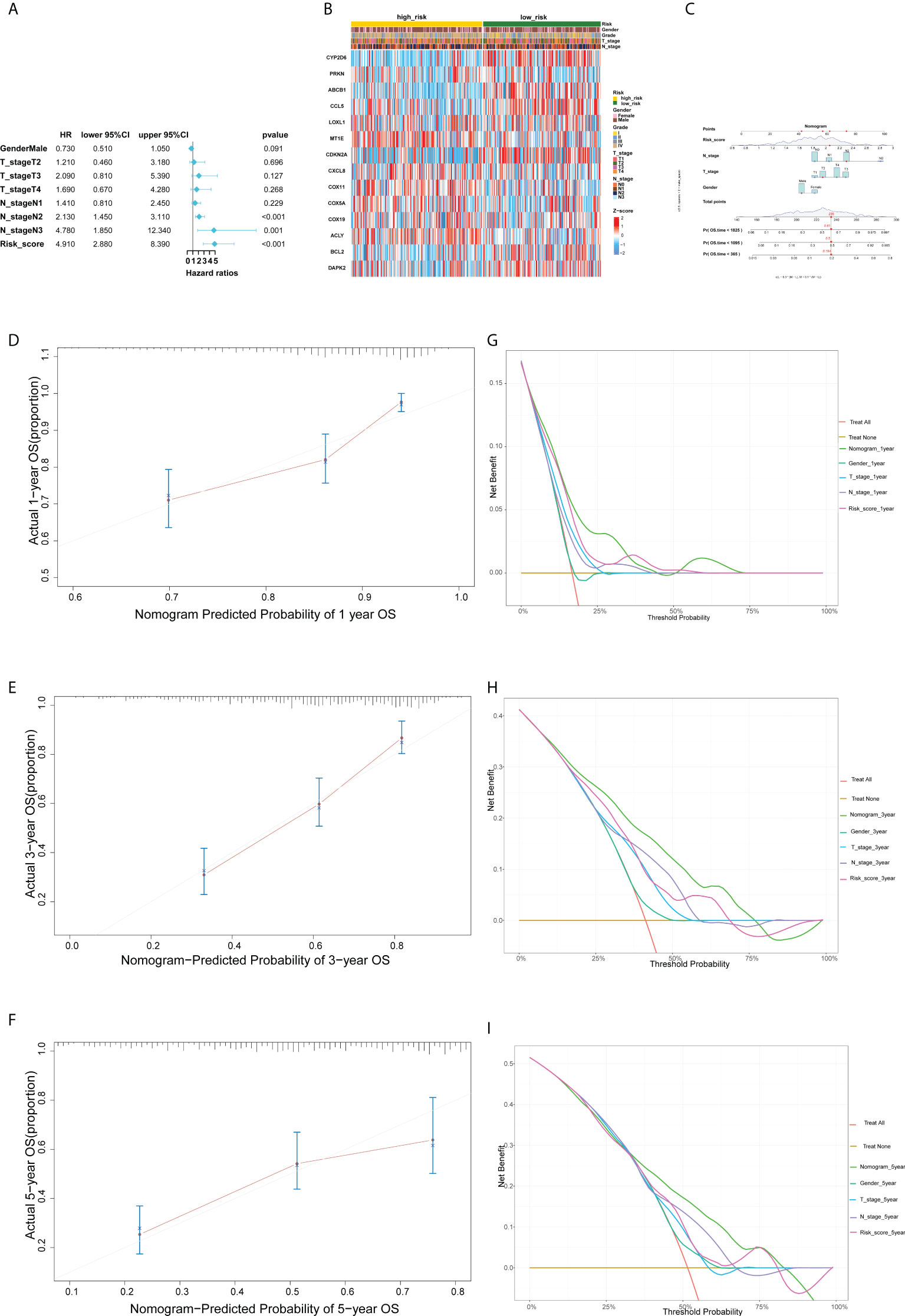
Figure 6 Establishment and evaluation of the predictive nomogram.(A)Multivariate Cox regression analysis of the clinicopathological features in the TCGA cohort. (B) Heat map for cuproptosis and copper metabolism–related gene prognostic signature and clinicopathological manifestations. (C) A nomogram for both clinic-pathological factors and prognostic cuproptosis and copper metabolism–related genes. (D–F) Plots depict the calibration of nomograms based on the risk score in terms of the agreement between predicted and observed 1-year (D), 3-year (E), and 5-year (F) outcomes in the TCGA cohort. (G, H, I) Decision curve analyses of the nomograms based on OS in two cohorts for 1 year (G), 3 years (H), and 5 years (I).
Genomic variance analysis in the TCGA cohort
In order to explore whether the difference of the copper metabolism gene expression between the two groups is related to gene variation, we conducted genomic variance analysis. We found that CDKN2A and ABCB1 had a high mutation probability in HNSCC (Figure 7A). The results showed that TMB in high-risk patients was higher than that in low-risk groups (P < 0.05, Figure 7B). The OS of patients with low risk and low TMB is higher than that of patients with high risk and high TMB (P < 0.0001, Figure 6C).
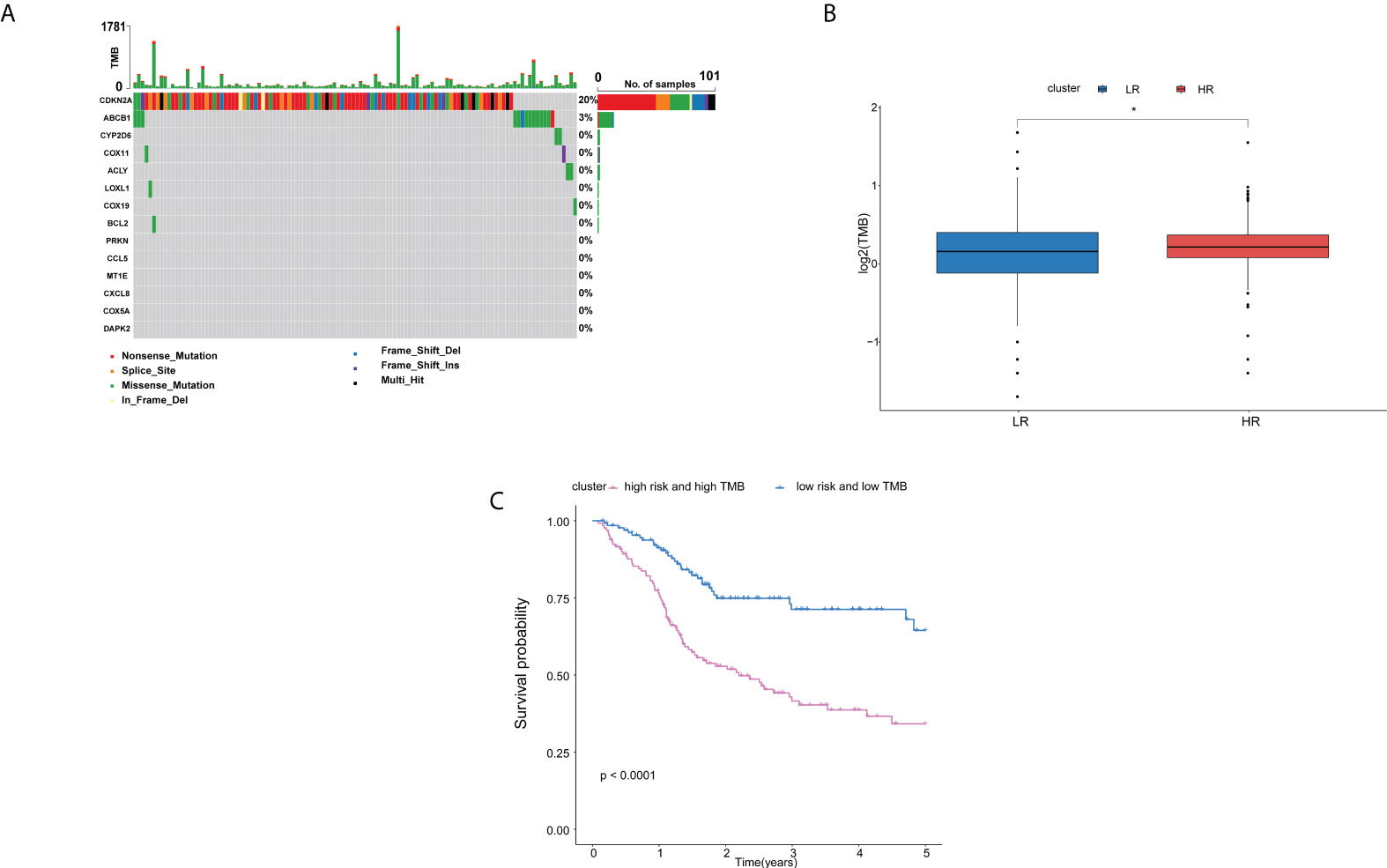
Figure 7 Analysis of the differences of genomics between high- and low-risk groups in the TCGA cohort.(A) Waterfall chart depicting the differential signature genes in somatic mutations of the HNSCC. (B) Boxplots displaying the TMB of the high-risk patients compared to low-risk patients. (C) OS rates in low-risk patients with low TMB were higher than those in high-risk patients with high TMB. Adjusted P-values were shown as: ns, not significant; *P < 0.05.
Discussion
In this study, we divided HNSCC into two risk subgroups with different TME characteristics based on 14 genes related to cuproptosis and copper metabolism. The characteristics of subgroup survival analysis were also well displayed according to clinical characteristics such as the tumor grade and stage. These results show the cuproptosis and copper metabolism-related genes signature can be considered as a validated dependable model for HNSCC.
All genes are involved in copper homeostasis and transport, oxidative stress, and the TCA cycle (26–34). COX11 is a copper transfer factor, while COX19 facilitates the transfer of copper (26). It was found that among multiple MT subtypes, MT1E was significantly upregulated in transcription after copper treatment, and its expression change can be used as an indicator of intracellular copper ion variation (6). The interaction between copper chelate and ABCB1 inhibited its mediated transport and downregulated the expression of ABCB1 (27). The genetic variation of PRKN is closely related to the pathogenesis of Parkinson’s disease, mainly manifested in mitochondrial dysfunction (35–37). CYP2D6, DAPK2, BCL2, RANTES, and IL-8 are all related to the redox characteristics of copper (29, 34, 38). LOX inactivation due to copper metabolism disorders or genetic mutations leads to the dysfunction of connective tissue (39). The enzymatic activity of ACLY has important functions in the TCA cycle (40). In addition, CDKN2A is a key gene regulating cuproptosis and has a high mutation probability in HNSCC (41).
The enrichment analysis of the two groups displayed that the 14 genes related to cuproptosis and copper metabolism were significantly enriched in immunocytes, tumorigenesis, such as immune response, intermediate filament, B-cell proliferation, and so on (42–46) The correlation analysis of the tumor immune microenvironment revealed that the activation of cells in the high-risk group had the characteristics of “cold tumor”, such as naïve CD4 T cells, resting NK cells, and M0 macrophages. On the contrary, in the low-risk group, Th17 cells, DC, B cells, plasma cells, CD8 T cells, activated memory CD4 T cells, follicular helper T cells, activated NK cells, and M1 macrophage cells were more abundant and had a longer survival time. In addition, we investigated and studied the relevance between cuproptosis and copper metabolism–related genes, immune activity, and immune checkpoints. Our results show that there is a close interaction between copper metabolism and immunity. Immunity may play a crucial part in the occurrence of cuproptosis. Cuproptosis may affect the immune state of HNSCC, thus affecting the occurrence and development of tumor. Previous studies have shown that the imbalance of copper metabolism and the changes of copper protein levels are closely related to cancer (47). The dysfunction of copper metabolic proteins may be the initiation of processes such as the generation of pretransfer niche, immune defense escape, and angiogenesis. However, the potential mechanism of inducing the cuproptosis behavior of tumor cells and enhancing antitumor immune response needs to be further clarified, and our research will help to provide new ideas into the exploration of potential tumor-targeted therapies.
Interestingly, using GO, KEGG, and GSEA enrichment analyses, we found that 14 genes associated with cuproptosis and copper metabolism were significantly enriched in the olfactory transduction pathway. In mammals, copper is highly concentrated in brain regions, including the major organs of olfactory receptors (48). The destruction of copper homeostasis is the basis of neurodegenerative diseases, which can be manifested as olfactory dysfunction (49). Patients with Alzheimer’s disease show serious olfactory defects in the early stage, and olfactory dysfunction may occur in patients with Wilson’s disease with neurological symptoms (48, 50). Recent studies have shown that olfactory receptor–related genes not only act on proprioceptive nerves but also play a crucial role in promoting tissue inflammation and cancer metastasis (51). At the same time, combined with the correlation between copper metabolism and tumor in this study, we speculate that there may be a potential relationship between copper metabolism–related genes and olfactory receptors on tumors. This discovery may offer new ideas for the diagnosis and treatment of HNSCC.
In recent years, the establishment of prognostic models for HNSCC has developed rapidly. Some researchers have developed a prognostic signature of regulatory cell death–related genes in HNSCC (52–54). Compared with ferroptosis, pyroptosis, and apoptosis, cuproptosis is a new concept. In this paper, we preliminarily discussed the role of cuproptosis in HNSCC and constructed a robust correlation model to predict the prognosis of HNSCC patients. Some researchers have paid attention to the TMB, which is related to the immunotherapeutic response of HNSCC (55). Therefore, the prognostic value of TMB in HNSCC researchers has been studied (55, 56). Interestingly, we found that the difference of copper metabolism gene expression in HNSCC patients was related to TMB, and the internal relationship between them remains to be explored. As an immunogenic tumor, the establishment of immune checkpoint–related gene signature is helpful to determine which HNSCC patients can benefit from immunotherapy (57, 58). In this study, we explored the possible role of cuproptosis in the progression of HNSCC from the perspective of immunity. We found that cuproptosis may be connected with the immune status of HNSCC and may thus play a role in the occurrence and development of tumors. The in-depth study of their internal mechanism may help to provide new ideas for tumor immunotherapy.
As with all studies, our study also had limitations. Our bioinformatics analysis identified potential cancer diagnostic genes associated with cuproptosis and copper metabolism, but its accuracy remains to be verified for each HNSCC subtype. In addition, our current research results are obtained from databases, and a series of biological experiments should be conducted to further examine the molecular mechanisms involved in cuproptosis and copper metabolism–related genes that affect the prognosis of HNSCC patients.
Data availability statement
Publicly available datasets were analyzed in this study. This data can be found here: HNSCC, CESC, ESCA cohort from TCGA database, https://portal.gdc.cancer.gov/.
Author contributions
SZ and JH conceived and designed the study. HL, SZ, and XL performed the data analysis. SZ, LZ, HL, and YY analyzed and interpreted the results. LZ wrote the original manuscript. SZ and JH reviewed and revised the manuscript. All authors contributed to the article and approved the submitted version.
Funding
This research was supported by the National Natural Science Foundation of China (grant numbers 81874128 and 82072994); Sun Yat-Sen University Clinical Research 5010 Program (grant numbers 2015018).
Conflict of interest
The authors declare that the research was conducted in the absence of any commercial or financial relationships that could be construed as a potential conflict of interest.
Publisher’s note
All claims expressed in this article are solely those of the authors and do not necessarily represent those of their affiliated organizations, or those of the publisher, the editors and the reviewers. Any product that may be evaluated in this article, or claim that may be made by its manufacturer, is not guaranteed or endorsed by the publisher.
Supplementary material
The Supplementary Material for this article can be found online at: https://www.frontiersin.org/articles/10.3389/fonc.2022.955336/full#supplementary-material
References
1. Johnson DE, Burtness B, Leemans CR, Lui VWY, Bauman JE, Grandis JR. Head and neck squamous cell carcinoma. Nat Rev Dis Primers (2020) 6(1):92. doi: 10.1038/s41572-020-00224-3
2. von Witzleben A, Wang C, Laban S, Savelyeva N, Ottensmeier CH. Hnscc: Tumour antigens and their targeting by immunotherapy. Cells (2020) 9(9):2103. doi: 10.3390/cells9092103
3. Solomon B, Young RJ, Rischin D. Head and neck squamous cell carcinoma: Genomics and emerging biomarkers for immunomodulatory cancer treatments. Semin Cancer Biol (2018) 52(Pt 2):228–40. doi: 10.1016/j.semcancer.2018.01.008
4. Queiroz A, Albuquerque-Souza E, Gasparoni LM, de França BN, Pelissari C, Trierveiler M, et al. Therapeutic potential of periodontal ligament stem cells. World J Stem Cells (2021) 13(6):605–18. doi: 10.4252/wjsc.v13.i6.605
5. Kumpitsch C, Moissl-Eichinger C, Pock J, Thurnher D, Wolf A. Preliminary insights into the impact of primary radiochemotherapy on the salivary microbiome in head and neck squamous cell carcinoma. Sci Rep (2020) 10(1):16582. doi: 10.1038/s41598-020-73515-0
6. Ma M, Qiu B, Jin J, Wang J, Nie Y, Liang Y, et al. Establishment of a specific in vivo cu() reporting system based on metallothionein screening. Metallomics (2021) 13(7):mfab035. doi: 10.1093/mtomcs/mfab035
7. Bigagli E, Luceri C, Bernardini S, Dei A, Dolara P. Extremely low copper concentrations affect gene expression profiles of human prostate epithelial cell lines. Chem Biol Interact (2010) 188(1):214–9. doi: 10.1016/j.cbi.2010.06.009
8. Tsvetkov P, Coy S, Petrova B, Dreishpoon M, Verma A, Abdusamad M, et al. Copper induces cell death by targeting lipoylated tca cycle proteins. Science (2022) 375(6586):1254–61. doi: 10.1126/science.abf0529
9. Vyas A, Duvvuri U, Kiselyov K. Copper-dependent Atp7b up-regulation drives the resistance of Tmem16a-overexpressing head-and-Neck cancer models to platinum toxicity. Biochem J (2019) 476(24):3705–19. doi: 10.1042/BCJ20190591
10. Kitamura N, Sento S, Yoshizawa Y, Sasabe E, Kudo Y, Yamamoto T. Current trends and future prospects of molecular targeted therapy in head and neck squamous cell carcinoma. Int J Mol Sci (2020) 22(1):240. doi: 10.3390/ijms22010240
11. Atashi F, Vahed N, Emamverdizadeh P, Fattahi S, Paya L. Drug resistance against 5-fluorouracil and cisplatin in the treatment of head and neck squamous cell carcinoma: A systematic review. J Dent Res Dent Clin Dent Prospects (2021) 15(3):219–25. doi: 10.34172/joddd.2021.036
12. Ressnerova A, Raudenska M, Holubova M, Svobodova M, Polanska H, Babula P, et al. Zinc and copper homeostasis in head and neck cancer: Review and meta-analysis. Curr Med Chem (2016) 23(13):1304–30. doi: 10.2174/0929867323666160405111543
13. Yao W, Qian X, Ochsenreither S, Soldano F, DeLeo AB, Sudhoff H, et al. Disulfiram acts as a potent radio-chemo sensitizer in head and neck squamous cell carcinoma cell lines and transplanted xenografts. Cells (2021) 10(3):517. doi: 10.3390/cells10030517
14. Kahlson MA, Dixon SJ. Copper-induced cell death. Science (2022) 375(6586):1231–2. doi: 10.1126/science.abo3959
15. Bian Z, Fan R, Xie L. A novel cuproptosis-related prognostic gene signature and validation of differential expression in clear cell renal cell carcinoma. Genes (Basel) (2022) 13(5):851. doi: 10.3390/genes13050851
16. Zhang G, Sun J, Zhang X. A novel cuproptosis-related lncrna signature to predict prognosis in hepatocellular carcinoma. Sci Rep (2022) 12(1):11325. doi: 10.1038/s41598-022-15251-1
17. Han J, Hu Y, Liu S, Jiang J, Wang H. A newly established cuproptosis-associated long non-coding rna signature for predicting prognosis and indicating immune microenvironment features in soft tissue sarcoma. J Oncol (2022) 2022:8489387. doi: 10.1155/2022/8489387
18. Lv H, Liu X, Zeng X, Liu Y, Zhang C, Zhang Q, et al. Comprehensive analysis of cuproptosis-related genes in immune infiltration and prognosis in melanoma. Front Pharmacol (2022) 13:930041. doi: 10.3389/fphar.2022.930041
19. Aran D, Hu Z, Butte AJ. Xcell: Digitally portraying the tissue cellular heterogeneity landscape. Genome Biol (2017) 18(1):220. doi: 10.1186/s13059-017-1349-1
20. Domper Arnal MJ, Ferrandez Arenas A, Lanas Arbeloa A. Esophageal cancer: Risk factors, screening and endoscopic treatment in Western and Eastern countries. World J Gastroenterol (2015) 21(26):7933–43. doi: 10.3748/wjg.v21.i26.7933
21. Burd EM. Human papillomavirus and cervical cancer. Clin Microbiol Rev (2003) 16(1):1–17. doi: 10.1128/CMR.16.1.1-17.2003
22. Sproll C, Fluegen G, Stoecklein NH. Minimal residual disease in head and neck cancer and esophageal cancer. Adv Exp Med Biol (2018) 1100:55–82. doi: 10.1007/978-3-319-97746-1_4
23. Kim J, Bowlby R, Mungall AJ, Robertson AG, Odze RD, Cherniack AD, et al. Integrated genomic characterization of oesophageal carcinoma. Nature (2017) 541(7636):169–75. doi: 10.1038/nature20805
24. Businello G, Fassan M, Degasperi S, Traverso G, Scarpa M, Angriman I, et al. Esophageal squamous cell carcinoma metachronous to head and neck cancers. Pathol Res Pract (2021) 219:153346. doi: 10.1016/j.prp.2021.153346
25. Saiz-Ladera C, Baliu-Pique M, Cimas FJ, Manzano A, Garcia-Barberan V, Camarero SC, et al. Transcriptomic correlates of immunologic activation in head and neck and cervical cancer. Front Oncol (2021) 11:714550. doi: 10.3389/fonc.2021.714550
26. Bode M, Woellhaf MW, Bohnert M, van der Laan M, Sommer F, Jung M, et al. Redox-regulated dynamic interplay between Cox19 and the copper-binding protein Cox11 in the intermembrane space of mitochondria facilitates biogenesis of cytochrome c oxidase. Mol Biol Cell (2015) 26(13):2385–401. doi: 10.1091/mbc.E14-11-1526
27. Ghosh RD, Chakraborty P, Banerjee K, Adhikary A, Sarkar A, Chatterjee M, et al. The molecular interaction of a copper chelate with human p-glycoprotein. Mol Cell Biochem (2012) 364(1-2):309–20. doi: 10.1007/s11010-012-1232-z
28. Pan M, Cheng ZW, Huang CG, Ye ZQ, Sun LJ, Chen H, et al. Long-term exposure to copper induces mitochondria-mediated apoptosis in mouse hearts. Ecotoxicol Environ Saf (2022) 234:113329. doi: 10.1016/j.ecoenv.2022.113329
29. Schlegel CR, Georgiou ML, Misterek MB, Stocker S, Chater ER, Munro CE, et al. Dapk2 regulates oxidative stress in cancer cells by preserving mitochondrial function. Cell Death Dis (2015) 6:e1671. doi: 10.1038/cddis.2015.31
30. Hartwig C, Zlatic SA, Wallin M, Vrailas-Mortimer A, Fahrni CJ, Faundez V. Trafficking mechanisms of p-type atpase copper transporters. Curr Opin Cell Biol (2019) 59:24–33. doi: 10.1016/j.ceb.2019.02.009
31. Xu M, Tang H, Zhou X, Chen H, Dong Q, Zhang Y, et al. Effects and mechanisms of Sub-chronic exposure to copper nanoparticles on renal cytochrome P450 enzymes in rats. Environ Toxicol Pharmacol (2018) 63:135–46. doi: 10.1016/j.etap.2018.08.004
32. Yang L, Xing S, Wang K, Yi H, Du B. Paeonol attenuates aging mrc-5 cells and inhibits epithelial-mesenchymal transition of premalignant hacat cells induced by aging mrc-5 cell-conditioned medium. Mol Cell Biochem (2018) 439(1-2):117–29. doi: 10.1007/s11010-017-3141-7
33. Kang Z, Qiao N, Liu G, Chen H, Tang Z, Li Y. Copper-induced apoptosis and autophagy through oxidative stress-mediated mitochondrial dysfunction in Male germ cells. Toxicol In Vitro (2019) 61:104639. doi: 10.1016/j.tiv.2019.104639
34. MacGregor HJ, Kato Y, Marshall LJ, Nevell TG, Shute JK. A copper-hydrogen peroxide redox system induces dityrosine cross-links and chemokine oligomerisation. Cytokine (2011) 56(3):669–75. doi: 10.1016/j.cyto.2011.08.025
35. Zhu YF, Aredo B, Chen B, Zhao CX, He YG, Ufret-Vincenty RL. Mice with a combined deficiency of superoxide dismutase 1 (Sod1), dj-1 (Park7), and parkin (Prkn) develop spontaneous retinal degeneration with aging. Invest Ophth Vis Sci (2019) 60(12):3740–51. doi: 10.1167/iovs.19-27212
36. Aboud AA, Tidball AM, Kumar KK, Neely MD, Han B, Ess KC, et al. Park2 patient neuroprogenitors show increased mitochondrial sensitivity to copper. Neurobiol Dis (2015) 73:204–12. doi: 10.1016/j.nbd.2014.10.002
37. Pickrell AM, Youle RJ. The roles of Pink1, parkin, and mitochondrial fidelity in parkinson's disease. Neuron (2015) 85(2):257–73. doi: 10.1016/j.neuron.2014.12.007
38. Yang F, Pei R, Zhang Z, Liao J, Yu W, Qiao N, et al. Copper induces oxidative stress and apoptosis through mitochondria-mediated pathway in chicken hepatocytes. Toxicol In Vitro (2019) 54:310–6. doi: 10.1016/j.tiv.2018.10.017
39. Lin W, Xu L, Li G. Molecular insights into lysyl oxidases in cartilage regeneration and rejuvenation. Front Bioeng Biotechnol (2020) 8:359. doi: 10.3389/fbioe.2020.00359
40. Noe JT, Rendon BE, Geller AE, Conroy LR, Morrissey SM, Young LEA, et al. Lactate supports a metabolic-epigenetic link in macrophage polarization. Sci Adv (2021) 7(46):eabi8602. doi: 10.1126/sciadv.abi8602
41. McBride S, Sherman E, Tsai CJ, Baxi S, Aghalar J, Eng J, et al. Randomized phase ii trial of nivolumab with stereotactic body radiotherapy versus nivolumab alone in metastatic head and neck squamous cell carcinoma. J Clin Oncol Off J Am Soc Clin Oncol (2021) 39(1):30–7. doi: 10.1200/jco.20.00290
42. Kim SS, Shen S, Miyauchi S, Sanders PD, Franiak-Pietryga I, Mell L, et al. B cells improve overall survival in hpv-associated squamous cell carcinomas and are activated by radiation and pd-1 blockade. Clin Cancer Res (2020) 26(13):3345–59. doi: 10.1158/1078-0432.CCR-19-3211
43. Spector ME, Bellile E, Amlani L, Zarins K, Smith J, Brenner JC, et al. Prognostic value of tumor-infiltrating lymphocytes in head and neck squamous cell carcinoma. JAMA Otolaryngol Head Neck Surg (2019) 145(11):1012–9. doi: 10.1001/jamaoto.2019.2427
44. Bisheshar SK, De Ruiter EJ, Devriese LA, Willems SM. The prognostic role of nk cells and their ligands in squamous cell carcinoma of the head and neck: A systematic review and meta-analysis. Oncoimmunology (2020) 9(1):1747345. doi: 10.1080/2162402X.2020.1747345
45. Cillo AR, Kurten CHL, Tabib T, Qi Z, Onkar S, Wang T, et al. Immune landscape of viral- and carcinogen-driven head and neck cancer. Immunity (2020) 52(1):183–99.e9. doi: 10.1016/j.immuni.2019.11.014
46. Komi DEA, Redegeld FA. Role of mast cells in shaping the tumor microenvironment. Clin Rev Allergy Immunol (2020) 58(3):313–25. doi: 10.1007/s12016-019-08753-w
47. Serra M, Columbano A, Ammarah U, Mazzone M, Menga A. Understanding metal dynamics between cancer cells and macrophages: Competition or synergism? Front Oncol (2020) 10:646. doi: 10.3389/fonc.2020.00646
48. Horning MS, Trombley PQ. Zinc and copper influence excitability of rat olfactory bulb neurons by multiple mechanisms. J Neurophysiol (2001) 86(4):1652–60. doi: 10.1152/jn.2001.86.4.1652
49. Adamson SX, Zheng W, Agim ZS, Du S, Fleming S, Shannahan J, et al. Systemic copper disorders influence the olfactory function in adult rats: Roles of altered adult neurogenesis and neurochemical imbalance. Biomolecules (2021) 11(9):1315. doi: 10.3390/biom11091315
50. Mueller A, Reuner U, Landis B, Kitzler H, Reichmann H, Hummel T. Extrapyramidal symptoms in wilson's disease are associated with olfactory dysfunction. Mov Disord (2006) 21(9):1311–6. doi: 10.1002/mds.20989
51. Orecchioni M, Kobiyama K, Winkels H, Ghosheh Y, McArdle S, Mikulski Z, et al. Olfactory receptor 2 in vascular macrophages drives atherosclerosis by Nlrp3-dependent il-1 production. Science (2022) 375(6577):214–21. doi: 10.1126/science.abg3067
52. He F, Chen Z, Deng W, Zhan T, Huang X, Zheng Y, et al. Development and validation of a novel ferroptosis-related gene signature for predicting prognosis and immune microenvironment in head and neck squamous cell carcinoma. Int Immunopharmacol (2021) 98:107789. doi: 10.1016/j.intimp.2021.107789
53. Zhu W, Zhang J, Wang M, Zhai R, Xu Y, Wang J, et al. Development of a prognostic pyroptosis-related gene signature for head and neck squamous cell carcinoma patient. Cancer Cell Int (2022) 22(1):62. doi: 10.1186/s12935-022-02476-3
54. Huang J, Huo H, Lu R. A novel signature of necroptosis-associated genes as a potential prognostic tool for head and neck squamous cell carcinoma. Front Genet (2022) 13:907985. doi: 10.3389/fgene.2022.907985
55. Zhang L, Li B, Peng Y, Wu F, Li Q, Lin Z, et al. The prognostic value of tmb and the relationship between tmb and immune infiltration in head and neck squamous cell carcinoma: A gene expression-based study. Oral Oncol (2020) 110:104943. doi: 10.1016/j.oraloncology.2020.104943
56. Wu F, Du Y, Hou X, Cheng W. A prognostic model for oral squamous cell carcinoma using 7 genes related to tumor mutational burden. BMC Oral Health (2022) 22(1):152. doi: 10.1186/s12903-022-02193-3
57. Song D, Tian J, Han X, Li X. A model of seven immune checkpoint-related genes predicting overall survival for head and neck squamous cell carcinoma. Eur Arch Otorhinolaryngol (2021) 278(9):3467–77. doi: 10.1007/s00405-020-06540-4
Keywords: cuproptosis, copper metabolism-related genes, gene signature, nomogram, prognosis, head and neck squamous cell carcinoma
Citation: Zhang S, Zhang L, Lu H, Yao Y, Liu X and Hou J (2022) A cuproptosis and copper metabolism–related gene prognostic index for head and neck squamous cell carcinoma. Front. Oncol. 12:955336. doi: 10.3389/fonc.2022.955336
Received: 28 May 2022; Accepted: 01 August 2022;
Published: 22 August 2022.
Edited by:
Jungsu S. Oh, University of Ulsan, South KoreaReviewed by:
Haolong Li, University of California, San Francisco, United StatesSunghoon Kwon, Konkuk University, South Korea
Copyright © 2022 Zhang, Zhang, Lu, Yao, Liu and Hou. This is an open-access article distributed under the terms of the Creative Commons Attribution License (CC BY). The use, distribution or reproduction in other forums is permitted, provided the original author(s) and the copyright owner(s) are credited and that the original publication in this journal is cited, in accordance with accepted academic practice. No use, distribution or reproduction is permitted which does not comply with these terms.
*Correspondence: Jingsong Hou, aG91anNAbWFpbC5zeXN1LmVkdS5jbg==
†These authors have contributed equally to this work