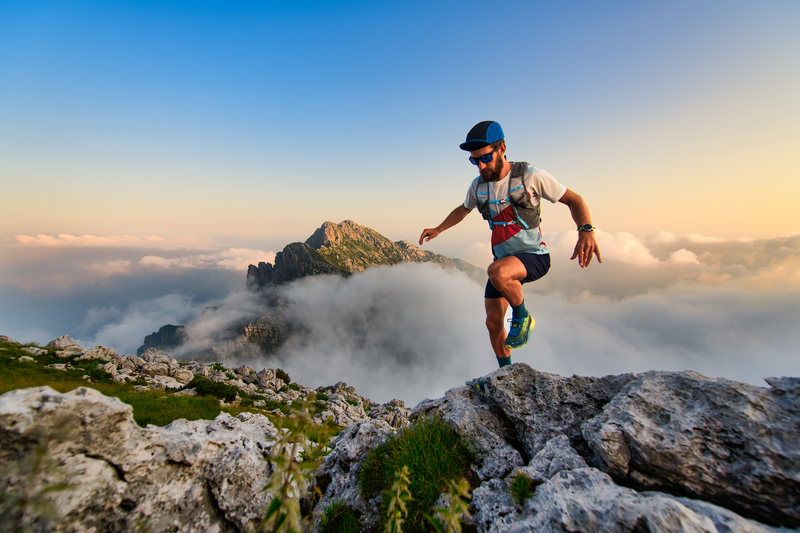
94% of researchers rate our articles as excellent or good
Learn more about the work of our research integrity team to safeguard the quality of each article we publish.
Find out more
ORIGINAL RESEARCH article
Front. Oncol. , 09 August 2022
Sec. Breast Cancer
Volume 12 - 2022 | https://doi.org/10.3389/fonc.2022.946758
Background: Breast cancer (BC) is one of the most common malignant tumors in women and poses a serious threat to their health. Compound Kushen injection (CKI) has shown therapeutic effects on a variety of cancers, including BC, and it can significantly improve the lives of patients. However, the underlying mechanism remains unclear and needs to be fully elucidated.
Methods: The active constituents of CKI were identified through a literature review, and the anti-BC targets of CKI were determined using multiple databases and a ChIP data analysis. Subsequently, the target was analyzed on the DAVID database through GO and KEGG to identify the key pathway that CKI affects to exhibit anti-BC activity. In addition, MCF-7 and MDA-MB-231 cells were treated with CKI for 24 and 48 hours at five concentrations, and the effects of CKI on cell proliferation and apoptosis were measured using MTT and annexin V/propidium iodide staining assays, respectively. The genes and protein identified to be involved in this pathway were verified using real-time quantitative PCR (qPCR) and western blot(WB) in BC cells.
Results: Twelve CKI anti-BC targets were obtained by a comprehensive analysis of the targets collected in the databases and results from the ChIP analysis. Bioinformatics analysis was performed for 12 targets. KEGG analysis showed that the 12 targets were mainly related to the VEGF, ErbB, and TNF signaling pathways. We focused our study on the VEGF signaling pathway as the p-value for the VEGF signaling pathway was the lowest among the three pathways. In vitro experiments showed that CKI significantly inhibited the proliferation of BC cells and induced apoptosis. Furthermore, qPCR and WB experiments showed that the expression of VEGF signaling pathway genes PIK3CA and NOS3 were significantly increased meanwhile SRC was significantly decreased after CKI intervention.
Conclusion: CKI significantly inhibited the proliferation of BC cells and induced apoptosis. The main mechanism for the anti-BC effect of CKI may be that it regulates the VEGF signaling pathway by increasing the expression of PIK3CA, SRC, and NOS3. Macrozamin and lamprolobine may be the main active components of CKI against BC.
Breast cancer (BC) is a malignant tumor originating in mammary epithelial tissues. It is one of the most common malignant tumors in women and seriously threatens their health (1). The latest “Global Cancer Report 2020,” published by the World Health Organization showed that BC has become the most common cancer among women worldwide. In 2018, there were 2.1 million new cases of BC and 627,000 deaths (2). According to the 2020 Cancer Statistics published by the American Cancer Society in CA: A Cancer Journal for Clinicians, it was estimated that there would be 1,806,590 new cancer cases and 606,520 cancer deaths in 2020, ranking BC with the highest new incidence rate among female cancers. It is estimated that by 2030, the number of BC cases will reach 2.64 million, and the number of deaths may reach more than 1.7 million (3). Commonly used BC treatment methods include surgery, chemotherapy, radiation therapy, and endocrine therapy (4). However, even if early-stage BC patients are treated with the abovementioned treatments, 30%-40% of patients still experience recurrence and metastasis (5). In addition, chemotherapy often leads to different degrees of adverse reactions in patients, which further reduces its efficacy (6). Therefore, identifying effective drugs to treat BC remains an important task for drug researchers and doctors.
Compound Kushen injection (CKI) consists of a mixture of natural compounds extracted from Radix Sophorae Flavescentis and Rhizoma Heterosmilacis (mass ratio = 7:3). China has used CKI in combination with chemotherapy for the treatment of stomach, liver, and non-small cell lung cancers since 1995 (7). The main component of CKI is derived from S. flavescens, which contains matrine, oxymatrine, and other components with pharmacological activities. The extract from S. flavescens exhibits various pharmacological activities, including enhancing immunity, possessing anti-inflammatory, antiviral, and antiallergy properties, and providing cardiovascular protection; it also affects cancer significantly, such as inhibiting cancer cell proliferation, inducing cell cycle arrest, accelerating apoptosis, restraining angiogenesis, inducing cell differentiation, inhibiting cancer metastasis and invasion, reversing multidrug resistance, and preventing or reducing chemotherapy- and/or radiotherapy-induced toxicity when combined with chemotherapeutic drugs (8, 9). Clinical practice has shown that the use of CKI in chemotherapy treatments improved the functional status of and quality of life in patients after BC surgery and reduced adverse reactions (10). However, the mechanism of CKI in the treatment of BC remains unclear. CKI has multiple components and targets, which makes it difficult for traditional research methods to reveal its complex mechanism in the treatment of BC. Network pharmacology, with its characteristics of “multigene and multitarget” coincides with the complexity of traditional Chinese medicine therapy. Therefore, with the continuous development of systems biology in Chinese medicine research, network pharmacology has become a hot topic in the field of Chinese medicine (11).
This study aimed to explore the mechanism of CKI in the treatment of BC using network pharmacology and Gene Expression Omnibus (GEO) data analysis and to verify the results using in vitro experiments. A scheme of the study protocol is shown in Figure 1.
Following literature search, CKI was analyzed by LC-MS/MS, and 21 active components were identified (12). After searching the PubChem database, 16 active components were selected for subsequent network pharmacology analysis (Table 1).
The three-dimensional structures of the 16 active compounds were uploaded to the PharmMapper database (13–15) (http://www.lilab-ecust.cn/pharmmapper/). Based on the reverse pharmacophore matching method, the pharmacophore model of the drug was selected, and the final 100 protein conformations were set to obtain the target name, gene name, UniProt ID, and other results related to the 16 active compounds in CKI. Simultaneously, the active compounds of CKI were uploaded to the SwissTargetPrediction database (http://www.swisstargetprediction.ch/). The 16 targets identified from the database were combined and imported into the UniProt database (https://www.Uniprot.org/). Species “Homo sapiens” was selected, and repeated non-human and irregular targets were eliminated to obtain the protein targets related to CKI active components.
The targets of BC were searched for in the DisgeNET (https://www.disgenet.org/), CTD (http://ctd.mdibl.org/), Gene Cards (https://www.genecards.org/), OMIM (Https://omim.org/), and PharmGKB databases. To reduce false-positive results, the DisgeNET database selected genes with a score greater than 0.4. The CTD database selected genes with markers/mechanisms or therapeutic markers with an inference score greater than 100. The Gene Cards database selected genes scoring in the top 1%. The targets of the five databases were merged, and duplicate targets were removed. In addition, we searched the GEO database to find relevant ChIP data using “Compound Kushen Injection AND Breast Cancer” as the search phrase. The inclusion criteria were as follows: (1) the samples were human tissue or cells, and (2) a control group and a CKI treatment group were included. Subsequently, the raw data that met the criteria were downloaded and analyzed using HISAT2 and StringTie. The differential genes in the control and CKI treatment groups that overlapped with the genes identified from the abovementioned five database searches were deemed to be BC-related targets.
The intersection of CKI and BC-related proteins was used to obtain potential CKI anti-BC targets. The potential targets of anti-BC CKI were imported into the STRING (http://string-db.org/) database, the species was limited to humans, and the protein interaction relationship file of potential targets was obtained. Node1, node2, and the combined score were extracted from the protein interaction relationship file and imported into Cytoscape 3.8.2, to visualize the relationship between CKI components and targets.
The protein names of selected CKI anti-BC targets were converted into gene symbols and imported into the David 6.8 database (https://david.ncifcrf.gov/) for GO and KEGG pathway enrichment analyses. The results were downloaded, and the data was sorted and visualized using the ggplot2 package in the R software.
Using TCGA database (http://gepia.cancer-pku.cn/), which includes 1085 BC tissues and 291 normal breast tissues (matched TCGA normal and GTEx data), mRNA expression of PIK3CA, SRC, and NOS3 in breast invasive carcinoma tissues and normal breast tissues was analyzed by Gene Expression Profiling Interactive Analysis (GEPIA).
CKI was purchased from the Shanxi Zhendong Group. RPMI 1640 and Dulbecco’s modified Eagle’s medium (DMEM) were purchased from Gibco (USA). Thiazolyl blue tetrazolium bromide was purchased from Solarbio (China); Annexin V-FITC detection kit from Beyotime Biotechnology (China); fetal bovine serum from Gibco (USA); TRIzol reagent from Ambion (Carlsbad, CA, USA); and qPCR kit from TaKaRa (Japan). The PCR primers were purchased from Sangon Biotech Co., Ltd. (China). PIK3CA, SRC, NOS3 primary antibodies were purchased from Abclonal(Wuhan, China).
MCF-7 and MCF-10A were cultured in RPMI 1640, and MDA-MB-231 cells was cultured in Dulbecco’s modified Eagle’s media, containing 10% fetal bovine serum, 100 U/mL penicillin, and 0.1 mg/mL streptomycin at 37°C and 5% CO2. The cells were divided into 10 groups for each cell line. MCF-7 and MDA-MB-231 cells in the log phase were seeded into 96-well plates at a density of 2×104 cells/well. After 24 hours, cells were introduced to media containing CKI (calculated based on the 20.8 mg/mL total alkaloids of CKI)—MCF-7 cells were cultured with 0, 2, 4, 6, and 8 mg/mL, and MDA-MB-231 cells were cultured with 0, 1, 2, 4, and 8 mg/mL of CKI. Each group contained six parallel replicates. The culture was continued for 24 hours and 48 hours, upon which the media was exchanged for one containing MTT. After a 4-hour incubation at 37°C, the supernatant was discarded, and DMSO (100 µL) was added to each well to dissolve the formazan. The plates were then shaken for 10 minutes before the absorbance (OD) was measured at 490 nm using a microplate reader to calculate the survival rate.
MCF-7, MCF-10A and MDA-MB-231 cells were cultured in 6-well trays and treated with a solution containing 2 mg/mL CKI. After 48 hours of treatment, the cells were harvested, and the rate of apoptosis was measured using an Annexin V-FITC detection kit according to the manufacturer’s instructions. The stained cells were sorted and data acquired on an BD FACSCanto Plus (BD Biosciences, NJ, USA) and the data were analysed using FlowJo software V10 (TreeStar Inc.,OR, USA).
MCF-7 and MDA-MB-231 cells were treated with a solution containing 2 mg/mL CKI. After 48 hours of treatment, the media were discarded and the cells washed with PBS for five times. Total RNA from each group was extracted using the TRIzol reagent, and the absorbance was measured at 260 nm to determine the total RNA concentration. Random hexamer primers and a SuperScript III reverse transcriptase kit were used to obtain complementary DNA (cDNA). After reverse transcription, a 20-μL aliquot was used for qPCR to detect the relative expression of mRNA. The results were analyzed using the 2-ΔΔCt method. The primer sequences are tabulated in Table 2.
MCF-7 and MDA-MB-231 cells were treated with a solution containing 2 mg/mL CKI. After 48 hours of treatment, the total protein were extracted from the MCF-7 and MDA-MB-231 cells, respectively. The bicinchoninic acid (BCA) protein assay kit was used to analyze the concentrations for total protein according to the manufacturer’s protocol. Protein from MCF-7 and MDA-MB 231 cells was isolated using SDS-PAGE with a 10% separating gel by loading 20μg of protein per lane. Then, the proteins were transferred onto a PVDF membrane. Afterward, the membranes were blocked with 5% fat-free milk in TBST buffer for 40min, followed by incubation with different primary antibodies including PIK3CA, SRC, NOS3 and β-actin overnight at 4°C. The next day, PVDF membranes were washed three times in TBST buffer and incubated with an anti-rabbit secondary antibody for 50min at 37°C. Finally, the relative expressions of various proteins were quantified by Image-J. The grey densities of the protein bands were normalized by using β-actin density as an internal control.
Molecular docking was performed using SYBYL-X 2.0 software (Tripos, St. Louis, MO, USA). The structures of the 12 proteins listed in Table 1 used for molecular docking were searched from the UniProt database (https://www.uniprot.org/). For the protein preparation, eutectic ligands and water molecules contained in the protein structure were removed, residues were repaired, side chains were fixed, and hydrogenation was performed. In parallel, small molecules related to the 12 targets were minimized in energy, the force field was set, and the algorithm was optimized. Finally, Surflex-Dock in SYBYL-X 2.0 was used to dock individual small molecules into the corresponding protein crystal structures. The binding mode with a C_Score ≥ 4 and total score ≥ 5 was selected for subsequent analysis; PyMol and LigPlot were used to visualize the results.
SPSS 20.0 was used to perform statistical analysis. Differences between the groups were assessed using a Student’s t-test. The data are presented as mean ± SE, and comparisons with a p-value < 0.05 were considered to be statistically significant.
A total of 683 human CKI targets were collected from the PharmMapper and SwissTargetPrediction databases. Additionally, the results obtained from the DisgeNET, CTD, Gene Cards, OMIM, and PharmGKB databases showed that there was a total of 512 BC-related targets. Subsequently, according to the ChIP analysis inclusion criteria, one chip meeting the criteria was screened: GSE78512. In this data set, we selected the MCF-7 control group and the treatment group with the most pronounced effect, which was the group treated with 2 mg/mL CKI for 48 hours, for the follow-up study. As the mRNA expression was not analyzed in the literature, raw data were analyzed. Raw data counts were exported for a gene difference analysis. The results showed 2143 differentially expressed genes between the MCF-7 control group and CKI treatment group (Figure 2). Finally, we took the intersection of CKI targets, BC-related targets and differentially expressed genes in GSE78512. Then, 12 potential anti-BC targets of CKI were found (Figure 3).
Figure 2 Gene difference analysis of chip GSE78512. Notes: The –log10(padj) was set equal to 10 when it was greater than 10.
Figure 3 Venn diagram for CKI prediction of target genes and disease-related genes. * represent the potential anti-BC targets of CKI.
The STRING database was used to generate data for 12 target-related protein-protein interaction(PPI) network (Figure 4A). Subsequently, interaction relationships among the 12 targets and those with the CKI active components were imported into Cytoscape to construct a functional-related protein interaction network (Figure 4B).
Figure 4 Protein-CKI active ingredients interaction network. Notes: (A) Protein-protein interaction (PPI) network of 12 CKI anti-BC targets. (B) CKI active ingredients- anti-BC targets interaction network.
The anti-BC targets of CKI were imported into the David database for Gene Ontology(GO) and KEGG analyses. The results showed that the 12 targets were involved in 43 biological processes (BP), 4 cell components (CC), and 9 molecular functions (MF), including, but not limited to, events related to transcription (DNA-templated) and regulation of the apoptotic process, actions occurring in the cytosol, mitochondria, and nucleus, and activities associated with protein, ATP, and transcription factor binding. The top 10 items involved in each gene function were selected and drawn into a bar graph using R 4.10 software (Figure 5). In addition, enrichment analysis results of the KEGG pathway showed that 12 targets were involved in regulating 30 signal pathways; 20 signal pathways with minimum p-values were selected to draw bubble maps (Figure 6). The vascular endothelial growth factor (VEGF), epidermal growth factor receptor (ErbB), and tumor necrosis factor (TNF) signaling pathways are the three main pathways related to BC.
Among the signaling pathways related to BC in enrichment analysis results of the KEGG pathway, the VEGF signaling pathway, which had the smallest p-value (P=0.0023), was selected for an in-depth study. The KEGG analysis showed that the genes involved in the regulation of the VEGF signaling pathway in anti-BC targets of CKI were PIK3CA, SRC, and NOS3. Subsequently, data mining on TCGA database found that BC tissues showed decreased PIK3CA and increased SRC expression compared to the expression of these genes in normal tissues(P>0.05); in addition, the BC tissues showed decreased significantly NOS3 compared to the expression of these genes in normal tissues(P<0.05) (Figure 7A). The results of the chip GSE78512 analysis showed that after CKI treatment, the expression of PIK3CA and NOS3 increased and the expression of SRC decreased in MCF-7 cells (Figure 7B). Comparing the expression of PIK3CA, SRC, and NOS3 in normal breast tissue and breast cancer tissue in TCGA database, it can be found that after CKI treatment, the expression of PIK3CA, SRC, and NOS3 in BC cells tends to be in normal tissue, suggesting that CKI may regulate the VEGF signaling pathway by reversing the abnormal expression of PIK3CA, SRC, and NOS3.
Figure 7 The mRNA expression of PIK3CA, SRC and NOS3 in breast cancer from TCGA data and chip GSE78512. Notes: (A) The mRNA expression of PIK3CA, SRC and NOS3 in breast cancer from TCGA data. (B) The mRNA expression of PIK3CA, SRC and NOS3 in breast cancer from chip GSE78512.*P < 0.05, compared with normal breast tissue group or MCF-7 control group. Thanks again for your meticulous review.
To explore the effect of CKI on the proliferation of MCF-7 and MDA-MB-231 cells, we used an MTT assay to measure cell viability after treatment with various doses of CKI. The proliferation of MCF-7 and MDA-MB-231 cells were significantly inhibited, and the IC50 of CKI for MCF-7 and MDA-MB-231 cells after 24 hours (48 hours) of treatment was 1.16 (1.15) mg/mL and 3.57 (1.78) mg/mL, respectively (Figure 8). An annexin V/propidium iodide assay was used to quantify apoptosis when MCF-10A, MCF-7 and MDA-MB-231 cells were treated with CKI. Compared to untreated cells, the percentage of apoptotic cells did not change significantly in MCF-10A; however, the percentage of apoptotic cells increased in MCF-7 and MDA-MB-231 cells treated with CKI, indicating that CKI-treated BC induced apoptosis without affecting normal cells (Figure 9).
Figure 8 Inhibition of MCF-7 and MDA-MB-231 proliferation. Notes: (A) The inhibition of MCF-7 proliferation (% of Control). (B) The inhibition of MDA-MB-231 proliferation (% of Control). n=3. Data are presented as means ± SD.
Figure 9 CKI induces apoptosis of MCF-7 cells and MDA-MB-231 cells. Notes: (A) Induction of apoptosis in MCF-10A cells with CKI treatment. (B) Induction of apoptosis in MCF-7 cells with CKI treatment. (C) Induction of apoptosis in MDA-MB-231 cells with CKI treatment. The level of apoptosis was determined by measuring the levels of Annexin V-FITC and PI staining. n=3. Data are presented as means ± SD. *P < 0.05, compared with MCF-10A(MCF-7 or MDA-MB-231) group.
To provide further evidence, we performed the same in vitro experiments as for chip GSE78512. The experiments were performed with MDA-MB-231 cells as well to further corroborate our conclusion. In addition, we also carried out corresponding experiments at the protein level.The results showed that the expression of PIK3CA and NOS3 mRNA and protein in MCF-7 and MDA-MB-231 cells were significantly increased and the expression of SRC mRNA were significantly decreased after treatment with CKI (Figures 10, 11). Since PIK3CA, SRC, and NOS3 were enriched in the VEGF signaling pathway, our results indicated that CKI may regulate VEGF signaling pathway in the treatment of BC.
Figure 10 The mRNA expression of PIK3CA, SRC and NOS3 in MCF-7 cells and MDA-MB-231 cells. Notes: (A) The expression levels of PIK3CA mRNA in MCF-7 cells; (B) The expression levels of SRC mRNA in MCF-7 cells; (C) The expression levels of NOS3 mRNA in MCF-7 cells; (D) The expression levels of PIK3CA mRNA in MDA-MB-231 cells; (E) The expression levels of SRC mRNA in MDA-MB-231 cells; (F) The expression levels of NOS3 mRNA in MDA-MB-231 cells. n=3. Data are presented as means ± SD. *P < 0.05, compared with MCF-7(MDA-MB-231) group.
Figure 11 The protein expression of PIK3CA, SRC and NOS3 in MCF-7 cells and MDA-MB-231 cells. Notes: (A) The expression levels of PIK3CA, SRC and NOS3 protein in MCF-7 cells; (B) The expression levels of PIK3CA, SRC and NOS3 protein in MDA-MB-231 cells. Lane-I, III, V represented MCF-7 (MDA-MB-231) cells group; Lane-II, IV, V represented MCF-7 (MDA-MB-231) cells with 2 mg/ml CKI treatment group. n=3. Data are presented as means ± SD. *P < 0.05, compared with MCF-7(MDA-MB-231) group.
To further elucidate the possible interactions between the active components of CKI and predicted targets related to the VEGF signaling pathway, we performed molecular docking simulations of the interactions between the active components of CKI and the predicted targets. The results showed that PIK3CA, SRC, and NOS3 interact well with the active small molecules in CKI; the docking scores are tabulated in Table 3. PIK3CA binds to macrozamin possibly by interacting with the residues His-670, Ser-629, Asn-756, and Arg-818 through hydrogen bonds and the residues Gln-630, Leu-632, Ile-633, Phe-666, Leu-755, Leu-814, Gln-815, Pro-835, Tyr-836, and Gly-837 via hydrophobic interactions (Figure 12A). SRC binds to macrozamin possibly by interacting with the residues Ser-248, Gln-251, Arg-291, Tyr-326, Tyr-340, and Glu-396 through hydrogen bonds and with the residues Glu-339, Ser-342, and Val-399 via hydrophobic interactions (Figure 12B). NOS3 binds to lamprolobine possibly by interacting with the residue His-461 through hydrogen bonds, and the residues Ser-102, Arg-365, His-461, Gln-462, Glu-463, Trp-74, Val-104, Trp-445, Ala-446, Trp-447, and Phe-460 via hydrophobic effects (Figure 12C). The docking results further suggest that the VEGF signaling pathway may be involved in CKI therapy for BC.
Table 3 Docking information of VEGF signaling pathway-related predicted target with active components of CKI.
Figure 12 Molecular docking simulations of the interactions between the active components of CKI and the predicted targets. Notes: (A) PIK3CA-macrozamin; (B) SRC-macrpzamin; (C) NOS3-lamprolobine.
This study explored the mechanism of CKI in the treatment of BC through network pharmacology and GEO database analysis combined with in vitro experimentation. First, we collected 683 CKI and 512 BC-related targets through multiple database analyses. To avoid false-positive results, we searched the GEO database for chips related to CKI treatment of BC to verify the collected targets. According to our research criteria for chip selection, one chip was found: GSE78512. After analyzing GSE78512, 2143 differentially expressed genes were identified, and 12 CKI anti-BC targets were obtained after comprehensive analysis with targets collected from the database. Bioinformatics analysis was then performed for 12 targets. GO analysis showed that these 12 targets were mainly related to apoptosis and cell proliferation. KEGG analysis showed that the 12 targets were mainly related to the VEGF, ErbB, and TNF signaling pathways. Among them, the VEGF signaling pathway had the lowest p-value, which became the focus of our attention.
VEGF is a mitogen that is specific to endothelial cells. Its structure is a homologous dimer glycoprotein linked through disulfide bonds that can induce physiological and pathological angiogenesis (16). Studies have shown that VEGF binds to VEGFR2 on the surface of endothelial cells and triggers a cascade of signal transduction via the PI3K and mitogen-activated protease (MAPK) pathways. These pathways, involving key messengers nitric oxide and cyclic cGMP, contribute to endothelial mitosis, promote endothelial cell survival through the apoptotic inhibitor protein pathway, and promote endothelial proliferation, leading to angiogenesis (17, 18). Additionally, VEGF effectively increases the permeability of endothelial cells and enhances the ability of tumor cells to metastasize (19). Many studies on the inhibition of BC proliferation and metastasis and the mechanisms of action described are related to the VEGF signaling pathway. Wang et al. found that cystathionine-γ-lyase can promote BC metastasis by regulating the VEGF signaling pathway (20). Lee et al. studied the role of VEGF in brain metastases originating from BC. Human brain microvascular endothelial cells were used to build an in vitro model of blood vessels, and using VEGF inhibitors, BC metastasis to the brain was suppressed (21). Ghattass et al. used drugs targeting hypoxia-inducible factor-1α in vivo and in vitro to demonstrate their antimetastatic effect on BC and verified that the mechanism of drug-induced cell damage was related to the downregulation of VEGF expression (22). In addition, Tian et al. showed that asiatic acid could inhibit angiogenesis and vascular permeability by acting on the VEGF/VEGFR2 signaling pathway, thereby inhibiting the growth and metastasis of BC in mice (23). In this study, we compared the relative expression of CKI anti-BC targets involved in the VEGF signaling pathway in BC and normal tissues from the TCGA database. Then, through the chip GSE78512 raw data analysis, it was found that CKI can reverse the expression of the anti-BC targets in BC cells. Furthermore, through in vitro experiments, we showed that CKI can inhibit proliferation and induce apoptosis of BC cells. These results suggest that CKI may exert its anti-BC effect by regulating the VEGF signaling pathway to inhibit proliferation and induce apoptosis in BC cells.
Molecular docking is an established in silico structure-based method widely used in drug discovery. Docking enables the identification of novel compounds of therapeutic interest and predicts ligand-target interactions at the molecular level (24). To further provide evidence that the mechanism of CKI treatment in BC was related to the VEGF signaling pathway, we carried out molecular docking experiments between the active components of CKI and the targets (PIK3CA, SRC, and NOS3) involved in the VEGF signaling pathway.
PIK3CA gene was detected by Volinia et al. In 1994 using in situ hybridization (25), and it is the only gene in the PI3K family that can undergo somatic mutation and cause cancer. PIK3CA gene mutation leads to the growth and transformation of mammary epithelial cells and fibroblasts, and inhibits apoptosis, which is closely related to the occurrence and development of tumors (26, 27). About 20-40% of breast cancers contain PIK3CA mutations, and the frequency of PIK3CA mutations is second only to TP53 mutations in breast cancer (28). Therefore, regulating the expression of PIK3CA may help improve breast cancer development. SRC family kinases are group of nine non-receptor tyrosine kinases of which SRC is the prototype (29). A key regulator of cell-matrix and cell-cell adhesions, SRC has been implicated in a number of cancer-associated phenotypes, including proliferation, migration and invasion (30, 31). Disease-associated upregulation of SRC kinase activity has been demonstrated in many malignancies, including breast, colon and gastric cancer. In breast cancer, elevated SRC expression is associated with poor prognosis (32). In addition, members of the SRC family may phosphorylate NOS3, leading to a decrease in NOS3 activity (33, 34). Studies have shown that NOS3 may play an important role in tumor progression via angiogenesis or apoptosis (35). Therefore, SRC and NOS3 play an important role in the progression of breast cancer.
In our study, the results showed that PIK3CA-macrozamin, SRC-macrpzamin, and NOS3-lamprolobine had favorable binding energies, further indicating that CKI may treat BC by regulating the VEGF signaling pathway.
Unfortunately, although we found through network pharmacology and GEO database that CKI anti-BC may regulate VEGF signaling pathway, and proved that gene/protein expression levels enriched in VEGF signaling pathway could be reversed by CKI treatment at nucleic acid and protein levels. However, whether CKI has an effect on normal endothelial cells is lacking in this study.
In summary, CKI could inhibit proliferation and induce apoptosis of BC cells, and the therapeutic mechanism of CKI against BC is likely to be closely related to its regulation of the VEGF signaling pathway by reversing the expression of PIK3CA, SRC, and NOS3. In addition, network pharmacology may be used to predict key therapeutic targets. We found that macrozamin and lamprolobine may be the active compounds in CKI that act against BC.
Publicly available datasets were analyzed in this study. This data can be found here: GEO:GSE78512.
YY completed most of the research and drafted manuscripts. BZ, QL, and DW plotted all of the charts. YT, YL, and LW analyzed the data. LL did the experiment in vitro. HH and FB designed the research and reviewed the manuscript. All authors contributed to the article and approved the submitted version.
Our study was supported by National Natural Science Foundation of China (No.81960756, No.81360689), Guangxi natural science foundation (No.2022GXNSFDA035063, No.2018GXNSFAA050078), Innovation and Entrepreneurship Training Program for College Students (202110601023) and Guangxi Zhuang Autonomous Region Health and Family Planning Commission Self-financed Scientific Research Project (Z20211128, Z20210927).
The authors declare that the research was conducted in the absence of any commercial or financial relationships that could be construed as a potential conflict of interest.
All claims expressed in this article are solely those of the authors and do not necessarily represent those of their affiliated organizations, or those of the publisher, the editors and the reviewers. Any product that may be evaluated in this article, or claim that may be made by its manufacturer, is not guaranteed or endorsed by the publisher.
BC, breast cancer; CKI, Compound Kushen injection; ErbB, epidermal growth factor receptor; GEO, Gene Expression Omnibus; GEPIA, Gene Expression Profiling Interactive Analysis; GO, Gene Ontology; KEGG, Kyoto Encyclopedia of Genes and Genomes; PPI, protein-protein interaction; TCGA, The Cancer Genome Atlas; TNF, tumor necrosis factor; VEGF, vascular endothelial growth factor.
1. Fahad Ullah M. Breast cancer: Current perspectives on the disease status. Adv Exp Med Biol (2019) 1152:51–64. doi: 10.1007/978-3-030-20301-6_4
2. Okamo PJT. World cancer report: Prevention and treatment are both needed in the battle against cancer.
3. Siegel RL, Miller KD, Jemal A. Cancer statistics, 2020. CA. Cancer J Clin (2020) 70(1):7–30. doi: 10.3322/caac.21590
4. Peart O. Breast intervention and breast cancer treatment options. Radiol Technol (2015) 86(5):535M–58M.
5. Brenner H, Gondos A, Arndt V. Recent major progress in long-term cancer patient survival disclosed by modeled period analysis. J Clin Oncol (2007) 25(22):3274–80. doi: 10.1200/JCO.2007.11.3431
6. Mackey JR, Martin M, Pienkowski T, Rolski J, Guastalla JP, Sami A, et al. Adjuvant docetaxel, doxorubicin, and cyclophosphamide in node-positive breast cancer: 10-year follow-up of the phase 3 randomised BCIRG 001 trial. Lancet Oncol (2013) 14(1):72–80. doi: 10.1016/S1470-2045(12)70525-9
7. Tan CJ, Zhao Y, Goto M, Hsieh KY, Yang XM, Morris-Natschke SL, et al. Alkaloids from oxytropis ochrocephala and antiproliferative activity of sophoridine derivatives against cancer cell lines. Bioorg Med Chem Lett (2016) 26(5):1495–7. doi: 10.1016/j.bmcl.2015.09.010
8. Yu L, Zhou Y, Yang Y, Lu F, Fan Y. Efficacy and safety of compound kushen injection on patients with advanced colon cancer: A meta-analysis of randomized controlled trials. Evidence-Based Complement Altern Med: eCAM(2017) (2017) 7102514. doi: 10.1155/2017/7102514
9. Wang W, You RL, Qin WJ, Hai LN, Fang MJ, Huang GH, et al. Anti-tumor activities of active ingredients in compound kushen injection. Acta Pharmacol Sin (2015) 36(6):676–9. doi: 10.1038/aps.2015.24
10. Ao M, Xiao X, Li Q. Efficacy and safety of compound kushen injection combined with chemotherapy on postoperative patients with breast cancer: A meta-analysis of randomized controlled trials. Medicine (2019) 98(3):e14024. doi: 10.1097/MD.0000000000014024
11. Barabási AL, Gulbahce N, Loscalzo J. Network medicine: a network-based approach to human disease. Nat Rev Genet (2011) 12(1):56–68. doi: 10.1038/nrg2918
12. Ma Y, Gao H, Liu J, Chen L, Wang Z. Identification and determination of the chemical constituents in a herbal preparation, compound kushen injection. hplc lc-dad-ms/ms (2014) 37(2). doi: 10.1080/10826076.2012.738623
13. Liu X, Ouyang S, Yu B, Liu Y, Huang K, Gong J, et al. PharmMapper server: a web server for potential drug target identification using pharmacophore mapping approach. Nucleic Acids Res (2010) 38(Web Server issue):W609–614. doi: 10.1093/nar/gkq300
14. Wang X, Pan C, Gong J, Liu X, Li H. Enhancing the enrichment of pharmacophore-based target prediction for the polypharmacological profiles of drugs. J Chem Inf Model (2016) 56(6):1175–83. doi: 10.1021/acs.jcim.5b00690
15. Wang X, Shen Y, Wang S, Li S, Zhang W, Liu X, et al. PharmMapper 2017 update: a web server for potential drug target identification with a comprehensive target pharmacophore database. Nucleic Acids Res (2017) 45(W1):W356–w360. doi: 10.1093/nar/gkx374
16. Leung DW, Cachianes G, Kuang WJ, Goeddel DV, Ferrara N. Vascular endothelial growth factor is a secreted angiogenic mitogen. Sci (New York NY)(1989) (4935) 246:1306–9. doi: 10.1126/science.2479986
17. Ricard N, Bailly S, Guignabert C, Simons M. The quiescent endothelium: signalling pathways regulating organ-specific endothelial normalcy. Nat Rev Cardiol (2021) 18(8):565–80. doi: 10.1038/s41569-021-00517-4
18. Gerber HP, McMurtrey A, Kowalski J, Yan M, Keyt BA, Dixit V, et al. Vascular endothelial growth factor regulates endothelial cell survival through the phosphatidylinositol 3’-kinase/Akt signal transduction pathway. requirement for flk-1/KDR activation. J Biol Chem (1998) 273(46):30336–43. doi: 10.1074/jbc.273.46.30336
19. Dvorak HF. Biology d. reconciling VEGF with VPF: The importance of increased vascular permeability for stroma formation in tumors, healing wounds, and chronic inflammation. Front Cell Dev Biol (2021) 9:660609. doi: 10.3389/fcell.2021.660609
20. Wang L, Shi H, Liu Y, Zhang W, Duan X, Li M, et al. Cystathionine−γ−lyase promotes the metastasis of breast cancer via the VEGF signaling pathway. Int J Oncol (2019) 55(2):473–87. doi: 10.3892/ijo.2019.4823
21. Lee TH, Avraham HK, Jiang S, Avraham S. Vascular endothelial growth factor modulates the transendothelial migration of MDA-MB-231 breast cancer cells through regulation of brain microvascular endothelial cell permeability. J Biol Chem (2003) 278(7):5277–84. doi: 10.1074/jbc.M210063200
22. Ghattass K, El-Sitt S, Zibara K, Rayes S, Haddadin MJ, El-Sabban M, et al. The quinoxaline di-n-oxide DCQ blocks breast cancer metastasis in vitro and in vivo by targeting the hypoxia inducible factor-1 pathway. Mol Cancer (2014) 13:12. doi: 10.1186/1476-4598-13-12
23. Tian M, Chen K, Huang J, Chu D, Li J, Huang K, et al. Asiatic Acid inhibits angiogenesis and vascular permeability through the VEGF/VEGFR2 signaling pathway to inhibit the growth and metastasis of breast cancer in mice. Phytother Res (2021) 278(7):5277–8. doi: 10.1002/ptr.7292
Pinzi L, Rastelli G. Molecular docking: Shifting paradigms in drug discovery. Int J Mol Sci (2019) 20(18). doi: 10.3390/ijms20184331
25. Volinia S, Hiles I, Ormondroyd E, Nizetic D, Antonacci R, Rocchi M, et al. Molecular cloning, cDNA sequence, and chromosomal localization of the human phosphatidylinositol 3-kinase p110α (PIK3CA) gene. Genomics (1994) 24(3):472–7. doi: 10.1006/geno.1994.1655
26. Astle MV, Hannan KM, Ng PY, Lee RS, George AJ, Hsu AK, et al. AKT induces senescence in human cells via mTORC1 and p53 in the absence of DNA damage: implications for targeting mTOR during malignancy. Oncogene (2012). doi: 10.1038/onc.2011.394
27. Samuels Y, Diaz LA, Schmidt-Kittler O, Cummins JM, Delong L, Cheong I, et al. Mutant PIK3CA promotes cell growth and invasion of human cancer cells. Cancer Cell (2005) 7(6):561–73. doi: 10.1016/j.ccr.2005.05.014
28. Saal LH, Holm K, Maurer M, Memeo L, Su T, Wang X, et al. PIK3CA mutations correlate with hormone receptors, node metastasis, and ERBB2, and are mutually exclusive with PTEN loss in human breast carcinoma. Cancer Res (2005) 65(7):2554–9. doi: 10.1158/0008-5472-CAN-04-3913
29. Gallick FMJ, Gary E. SRC family nonreceptor tyrosine kinases as molecular targets for cancer therapy. Anti-Cancer Agents Med Chem (Formerly Curr Med Chem - Anti-Cancer Agents) (2007) 7(6):651–9. doi: 10.2174/187152007784111278
30. Avizienyte E, Frame MC. Src and FAK signalling controls adhesion fate and the epithelial-to-mesenchymal transition. Curr Opin Cell Biol (2005) 17(5):542–7. doi: 10.1016/j.ceb.2005.08.007
31. Nam K, Oh S, Lee KM, Yoo SA, Shin I. CD44 regulates cell proliferation, migration, and invasion via modulation of c-src transcription in human breast cancer cells. Cell Signal (2015) 27(9):1882–94. doi: 10.1016/j.cellsig.2015.05.002
32. Ottenhoff-Kalff AE, Rijksen G, van Beurden EA, et al. Characterization of protein tyrosine kinases from human breast cancer: involvement of the c-src oncogene product. Cancer Res (1992) 52(17):4773–8.
33. García-Cardeña G, Fan R, Stern DF, et al. Endothelial nitric oxide synthase is regulated by tyrosine phosphorylation and interacts with caveolin-1. J Biol Chem (1996) 271(44):27237–40. doi: 10.1074/jbc.271.44.27237
34. Takenouchi Y, Oo ML, Senga T, Watanabe Y, Machida K, Miyazaki K, et al. Tyrosine phosphorylation of NOS3 in a breast cancer cell line and src-transformed cellsOncol Rep. (2004) 11(5):1059–62.
Keywords: compound kushen injection, breast cancer, network pharmacology, gene expression omnibus, therapeutic mechanism
Citation: Ye Y, Zhang B, Liang Q, Wang D, Bai F, Li Y, Wei L, Li L, Huang H and Tang Y (2022) Exploring the pharmacological mechanism of compound kushen injection in the treatment of breast cancer using in vitro experiments: Coupling network pharmacology with GEO database. Front. Oncol. 12:946758. doi: 10.3389/fonc.2022.946758
Received: 18 May 2022; Accepted: 18 July 2022;
Published: 09 August 2022.
Edited by:
Dirk Geerts, University of Amsterdam, NetherlandsReviewed by:
Blassan George, University of Johannesburg, South AfricaCopyright © 2022 Ye, Zhang, Liang, Wang, Bai, Li, Wei, Li, Huang and Tang. This is an open-access article distributed under the terms of the Creative Commons Attribution License (CC BY). The use, distribution or reproduction in other forums is permitted, provided the original author(s) and the copyright owner(s) are credited and that the original publication in this journal is cited, in accordance with accepted academic practice. No use, distribution or reproduction is permitted which does not comply with these terms.
*Correspondence: Yunxia Tang, dGFuZ3l1bnhpYTE5ODRAMTI2LmNvbQ==; Huixue Huang, aGh4MTIzQGhvdG1haWwuY29t
†These authors have contributed equally to this work
Disclaimer: All claims expressed in this article are solely those of the authors and do not necessarily represent those of their affiliated organizations, or those of the publisher, the editors and the reviewers. Any product that may be evaluated in this article or claim that may be made by its manufacturer is not guaranteed or endorsed by the publisher.
Research integrity at Frontiers
Learn more about the work of our research integrity team to safeguard the quality of each article we publish.